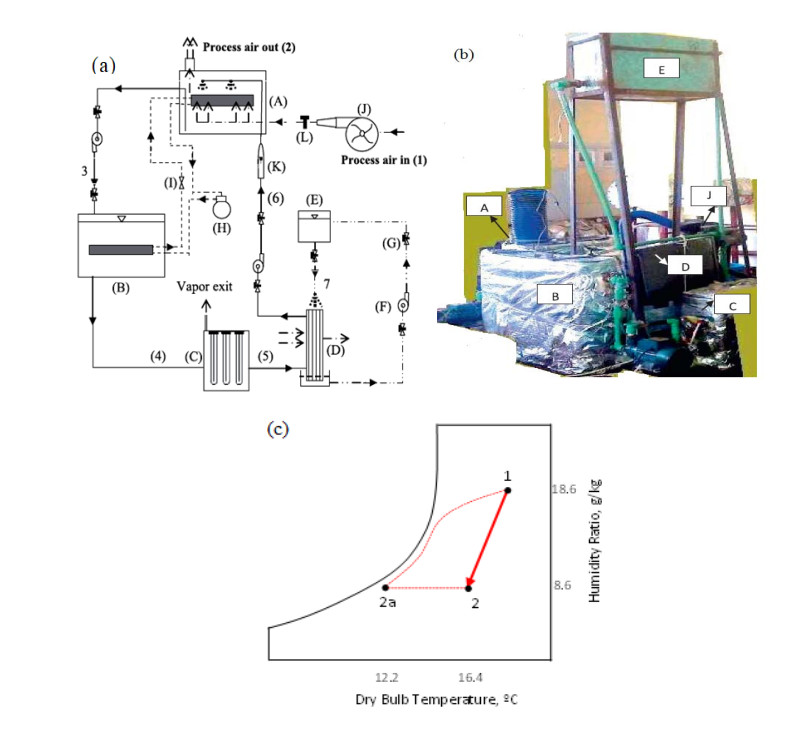



Citation: Ayman A. Aly, B. Saleh, M. M. Bassuoni, M. Alsehli, A. Elfasakhany, Khaled I.E. Ahmed. Artificial neural network model for performance evaluation of an integrated desiccant air conditioning system activated by solar energy[J]. AIMS Energy, 2019, 7(3): 395-412. doi: 10.3934/energy.2019.3.395
[1] | Marwa M. Ibrahim, Amr A. Elfeky, Amal El Berry . Forecasting energy production of a PV system connected by using NARX neural network model. AIMS Energy, 2024, 12(5): 968-983. doi: 10.3934/energy.2024045 |
[2] | Mohamed Hamdi, Hafez A. El Salmawy, Reda Ragab . Optimum configuration of a dispatchable hybrid renewable energy plant using artificial neural networks: Case study of Ras Ghareb, Egypt. AIMS Energy, 2023, 11(1): 171-196. doi: 10.3934/energy.2023010 |
[3] | Muhammad Farhan Hanif, Muhammad Sabir Naveed, Mohamed Metwaly, Jicang Si, Xiangtao Liu, Jianchun Mi . Advancing solar energy forecasting with modified ANN and light GBM learning algorithms. AIMS Energy, 2024, 12(2): 350-386. doi: 10.3934/energy.2024017 |
[4] | Aklilu T. Habtewold, Demiss A. Ambie, Wondwossen B. Eremed . Solar assisted pyrolysis system for High-Density polyethylene plastic waste to fuel conversion. AIMS Energy, 2020, 8(3): 455-473. doi: 10.3934/energy.2020.3.455 |
[5] | Rashid Al Badwawi, Mohammad Abusara, Tapas Mallick . Speed control of synchronous machine by changing duty cycle of DC/DC buck converter. AIMS Energy, 2015, 3(4): 728-739. doi: 10.3934/energy.2015.4.728 |
[6] | Nadwan Majeed Ali, Handri Ammari . Design of a hybrid wind-solar street lighting system to power LED lights on highway poles. AIMS Energy, 2022, 10(2): 177-190. doi: 10.3934/energy.2022010 |
[7] | Hayder S. Al-Madhhachi, Ahmed M. Ajeena, Nihad A. Al-Bughaebi . Dynamic simulation and energy analysis of forced circulation solar thermal system in two various climate cities in Iraq. AIMS Energy, 2021, 9(1): 138-149. doi: 10.3934/energy.2021008 |
[8] | Virendra Sharma, Lata Gidwani . Optimistic use of battery energy storage system to mitigate grid disturbances in the hybrid power system. AIMS Energy, 2019, 7(6): 688-709. doi: 10.3934/energy.2019.6.688 |
[9] | Bilal Akbar, Khuram Pervez Amber, Anila Kousar, Muhammad Waqar Aslam, Muhammad Anser Bashir, Muhammad Sajid Khan . Data-driven predictive models for daily electricity consumption of academic buildings. AIMS Energy, 2020, 8(5): 783-801. doi: 10.3934/energy.2020.5.783 |
[10] | Virendra Sharma, Lata Gidwani . Recognition of disturbances in hybrid power system interfaced with battery energy storage system using combined features of Stockwell transform and Hilbert transform. AIMS Energy, 2019, 7(5): 671-687. doi: 10.3934/energy.2019.5.671 |
Nomenclature: Latin letters
ANN: Artificial Neural Network; BP-ANN: back propagation artificial neural network; COP: coefficient of performance; E: deviation; f: activation function; h: specific enthalpy, kJ/kg; i: index representing input layers; IDACS: integrated desiccant air conditioning system; j: index representing hidden layers; k: index representing output layers; LD: liquid desiccant; LDACS: liquid desiccant air conditioning system; m: number of experimental data; ˙m: mass flow rate (kg/s); n: number of input neurons; SMR: specific moisture removal; T: temperature, ℃; VCRS: vapor compression refrigeration systems; x: concentration; z: hidden neurons; ˙Q: Heat transfer rate, kW; ˙W: Power, kW; Subscripts
a: process air; AH: additional regeneration heat; c: compressor; cond: condenser; da-out: outlet dry air; eva: evaporator; reg: regenerative; s: desiccant solution; Aver: average; exp: experimental; Rel: relative; S: standard; Tran: trained
The liquid desiccant air conditioning systems (LDACS) are alternatives to the traditional vapor compression refrigeration systems (VCRS). These systems conduct air dehumidification with liquid desiccant (LD) to reduce electrical energy consumption. The present dehumidification system has many weak points. The first is poor energy efficiency, and the second is that it produces water and continuously drains during use; this breeds mildew, amebas, or bacteria. The third is that air ventilating during the dehumidification process is difficult in the present dehumidification system of the air-conditioner. Therefore, it is need to develop an alternative method for the dehumidification system. The LDACS have recently gained growing interest from the stand point of reducing energy consumption during the dehumidification. This is because the LDACS can be driven by low-grade renewable energies such as solar energy, geothermal energy, and low temperature waste heat from industrial plants, and it allows isothermal dehumidification and produces no waste water. Furthermore, the LDACS allow reducing the humidity or adding humidity to the air by switching the circulation loop with ventilation during use of the air-conditioners [1]. Numerous investigations have proposed various air handling systems utilizing LD [2,3,4,5]. Also, many studies have been conducted on hybrid cooling systems [6,7,8,9,10].
Watanabe et al. [1] conducted a systematic assessment of the humidification capability of sixteen types of ionic liquids for finding a suitable desiccant liquid for the LDACS. Among the examined liquids, tributyl phosphonium dimethyl phosphate presented the greatest dehumidification capacity and had a minimum corrosive impact. Also, they found that a 77% (w/w) aqueous solution of this liquid worked as an effective desiccant liquid for the LDACS. Park et al. [11] evaluated the effect of dehumidification with a cascade LD on the energy consumption in an evaporative cooling and LD system. The results showed that the retrofit case with a cascade LD section consumed 12% lower energy under the case of maximum load. The achieved overall primary and thermal coefficients of performance (COP) of the retrofit case were 2.05 and 0.78, respectively. The impact of electrodialysis regenerator of the LDACS on the performance of the system was investigated by Cheng and Jiao [12]. They found that the electric current efficiency correlation of an electrodialysis regenerator of the LDACS depend on many measurements. Also, they concluded that the concentration of the LD at the regenerator inlet increases from 27% to 35% leading to enhance the system COP from 4 to 6.2. Although, the rise of the LD concentration at the regenerator inlet decreases its performance, however, it boosts the system COP. Cheng and Xu [13] investigated, experimentally, a multi-function LD regeneration system to decrease the working cost and the waste of electrode solution of electrodialysis regenerator. They concluded that enhancing the system current efficiency requires significant reduction in both the concentration difference among solutions in the dilute chambers and regeneration chambers and the operational current. A desiccant aided air conditioning (AC) system uses solar energy for desiccant regeneration was investigated by Speerforck et al. [14]. The system achieved the highest energy efficiency ratio at 7.7 (kW/kW). Also they found that the proposed system saves electricity more than 50% compared with the vapor compression chiller. An energy efficient unit utilizing LD was suggested by Adnan et al. [15]. They concluded that the suggested system decreases the energy consumption to approximately 0.3 of that used by traditional AC. The performance of regeneration and absorption columns for a LD vapor refrigeration system was examined by Mohan et al. [16]. They concluded that the decrease of temperature and the increase of the specific humidity of the entry air lead to increase the dehumidification inside the absorber. An integrated desiccant aided AC system was introduced by Jia et al. [17] as a substitute to traditional VCRS. The major feature of the system was removing the sensible heat and moisture by both cooling coil and desiccant, respectively. They concluded that the proposed system save about 37.5% of the electrical energy consumption as compared with traditional VCRS. The performance of a hybrid AC system was evaluated theoretically and experimentally by Jongsoo et al. [18]. They concluded that the proposed hybrid system boosts the COP by about 94% in comparison with traditional VCRS. A solar powered two-stage rotary desiccant cooling unit and a VCRS were simulated by Ge et al. [19]. They concluded that the desiccant cooling system consumes less electrical power and has better supply air quality as compared with traditional VCRS. The performance of a LDACS subjected to variable fresh air ratios was analyzed by Niu et al. [20]. They concluded that the proposed system consumes remarkably less power as compared with traditional VCRS with primary return air. The uppermost energy saving ratio was about 59% with fresh air ratio about 20%, and the lowermost was nearly 4.6% with 100% fresh air. Jiazhen et al. [21] have tested a desiccant wheel aided separate sensible and latent cooling AC system utilizing R410a and CO2 as refrigerant. They concluded that with a 50 ℃ regeneration temperature, the vapor compression cycle COP has boosted by about 7% as compared with that achieved by the base system. Ge et al. [22] experimentally examined the performance of two desiccant coated finned tube heat exchangers, which were coated with polymer and silica gel materials. Their results exhibited that the desiccant coated heat exchanger attains high dehumidification performance under specific operating conditions and overcomes the drawback of adsorption heat. Further, they have shown that the polymer coated heat exchanger performs worse than the silica gel one. Ge et al. [23,24] studied the performance of desiccant coated heat exchanger system utilizing silica gel as desiccant substance and a solar power desiccant coated heat exchanger cooling system. Experimental tests to investigate the performance of a counter flow regenerator using lithium chloride solution as the desiccant were carried out by Mohammad et al. [25]. A single and multilayer artificial neural network (ANN) was used to predict the performance of the regenerator. An ANN model for predicting the performance of a liquid desiccant dehumidifier was proposed by Mohammad et al. [26]. The maximum difference between the ANN and experimental values for water condensation rate and dehumidifier effectiveness were 8.13% and 9.0485%, respectively. A thorough investigation on parameters that having the potential impact on performance of the desiccant enhanced evaporative air conditioning system was conducted by Sohani et al. [27]. Five soft computing and statistical tools were used to predict the overall performance of the system. An experimental study of a dehumidifier was carried out and the effects of inlet parameters of air, water and solution on the performance of the dehumidifier were discussed by Cheng et al. [28]. The average absolute difference of the theoretical and experimental values is less than 8% for both moisture and enthalpy effectiveness. The solar assisted liquid desiccant dehumidifier and regenerative indirect evaporative cooling semi-centralized air conditioning system was investigated by Chen et al. [29]. The system model was developed by solving the heat and mass transfer equations of each component integrally in a closed loop.
For design and energy saving objectives, an accurate model to evaluate the performance of integrated desiccant air conditioning system (IDACS) powered by solar energy will be very helpful. Consequently, in the present study, ANN technique is introduced to assess the IDACS performance. The presented IDACS system uses a VCRS integrated to the desiccant solution system in a new method. The evaporator of the VCRS is submerged in the strong desiccant solution and a pass of air is blown inside it to enhance heat transfer process. The performance of IDACS is predicted using back propagation artificial neural network (BP-ANN). The IDACS contains a LD dehumidification cycle combined with a VCRS. The primary objective of the present work is to establish a BP-ANN model to assess the IDACS performance. The integrated system performance is evaluated using its controlling parameters, i.e., COP, outlet dry air temperature (Tda-out), and specific moisture removal (SMR). The training of the BP-ANN is achieved using experimental results previously published by Bassuoni [30]. The influences of some selected operating parameters, i.e., regeneration temperature, desiccant solution temperature in the condenser and evaporator are studied and discussed. Furthermore, the concentration of strong solution on the system performance are studied and discussed as well.
Figure 1a displays a schematic diagram of the IDACS. The system includes basically a VCRS combined with a LD dehumidification unit. Figure 1b illustrates the system experimental set-up. As can be observed from Figure 1a, the IDACS comprises of four subsystems; process air subsystem, desiccant solution subsystem, cooling water subsystem, and VCRS. The VCRS has refrigeration capacity equal 5.27 kW and utilizes 134a as a working fluid. The condenser and evaporator boxes are manufactured from stainless steel sheet with 0.5 mm thickness and 60 × 60 × 25 cm dimensions. Calcium chloride is utilized as a desiccant in the dehumidification unit. The evaporative heat exchanger is utilized to cool the strong desiccant solution to the desired condition (point 6). In the process air subsystem, the ambient air (point 1) is forced to the evaporator using air fan. In the evaporator, the air is cooled and dehumidified to the desired temperature and relative humidity to feed the space to be conditioned (point 2). The ambient air temperature and relative humidity at the experiment time fluctuated between 41–42 ℃, and 46–48%, respectively.
The desiccant solution subsystem is a heat driven unit, in which a heat supply is required for this subsystem to regenerate the desiccant solution. A low-grade renewable heat source at a temperature of approximately 30–70 ℃ can be utilized. Renewable energy sources like; solar energy and waste and geothermal heats can be utilized for the regeneration process. The strong solution (point 6) is sprayed into the evaporator box of the VCRS, and then the diluted desiccant solution (point 3) is fed to the condenser (B). The diluted desiccant solution is heated in the condenser (point 4) to use waste heat from VCRS and accordingly reduce the required heat for the desiccant solution regeneration. A solar heater (C) is utilized to regenerate the solution totally to the desired concentration. In the cooling water subsystem, a heat exchanger (D) evaporative type with effectiveness equal 0.85 is utilized to pre-cool the strong solution through the process (5–6), after which the strong solution is fed into the evaporator. A cooling water tank (E) is used to feed the necessary cooling water (point 7) for pre-cooling process. The cooling water temperature is fixed at approximately 24 ℃ using a cooling coil with a digital thermostat inserted inside the supplying tank. Figure 1a, b are taken from Bassuoni [30]. Figure 1c shows the process air psychometric chart. The process 1–2 (solid line) represents the IDACS process. The process 1-2a-2 (dashed line) denotes the equivalent traditional AC system with reheat. The process 1-2a is a cooling process with dehumidification and the process 2a-2 is a heating process to the required temperature of the supply air. More details about the IDACS, its working principle, and measuring instruments uncertainties can be found at Bassuoni [30].
The ANN is a strategy that can solve the problems of physical systems wherever traditional methods are not easy to apply or wherever the issue cannot be any formulized. ANN training process is improvement of neuron parameters with regard to a defined level operate. Since it is by learning ready to update the first layer information, it is recognized among updated strategies [31,32,33].
An ANN structure of a practical system is a style of extremely advanced and nonlinear fitting model of indefinable shape. Figure 2 shows illustration of the current ANN model that will be used in the current work. The model has four inputs layer (1:4), hidden layer (1:50), and the three outputs layer with neurons y1, y2, and y3. Let τj be the bias for neuron zj and ∅ be the bias for neuron y. Let wij be the weight of the connection of the affiliation from neuron xi to neuron zj and βj be the weight of connection from neuron zj to y. The equations that the ANN estimates are:
y=gA(50∑i=1ziβj+∅) | (1) |
zj=fA(8∑i=1xiWij+τj) | (2) |
where gA and fA are activation functions, which are utilized in ANNs to provide continuous values instead of separate ones. The activation performs utilized in second layer neurons are tan sigmoid functions and in the third layer neurons, the piecewise linear activation function is employed as shown in Figure 3. The Tan sigmoid activation function is:
gA=1−e−2 net1+e−2 net | (3) |
and piecewise linear activation function is:
fA={−1net<−1net|net|≤1+1net>1} | (4) |
The back propagation formula, utilized in this work, updates the weights in the steepest descent direction (sign of the gradient). The flow chart of the learning method is showed in Figure 4.
It is found that 3 layers NNW can approximate any function to any defined accuracy. The network has an input, hidden, and output layers. The training stage is sectioned as follows:
1. forward-propagation phase: X = [x1, x2, x3, x4] is propagating from the first layer to the last layer Y.
Zq=f(n∑j=1vqjxj),n=4 | (5) |
Y=f(k∑q=1wiqZq) | (6) |
where Zq is the data for second layers, f is the activation function, Vqj is the first-to-second connections weights, Y is the ANN output and Wiq is the second-to-last connections weights.
2. back-propagation phase: Equation 7 represents the error difference in the output Y, and the target d:
E=12m∑i=1(di−yi)2 | (7) |
where m is the learned points number.
The weights in second-to-last connections are treated by the gradient-descent methodology as follows:
ΔWiq=−η∂E∂Wiq=−η[∂E∂Y][∂Y∂neti][∂neti∂Wiq]=−η[di−yi][f′(neti)][Zq]=ηδoiZq | (8) |
where η is the learning rate.
For moderate the weights of the input-to-hidden:
ΔVqi=−η∂E∂Vqi=−η[∂E∂netq][∂netq∂Vqj]=ηδVhqxi | (9) |
δoi=−[di−yi][f′(neti)] | (10) |
δhq=[∂E∂Zq][∂zq∂netq] | (11) |
where δoi and δhq are the local and partial errors separately.
The interactions proceed until the execution error diminished to defined range. The ANN execution is controlled by the mean squared error (MSE) and linear correlation coefficient (r). As the NW update its MSE turns out to nearly zero value.
In this study, three significant dependent parameters are selected to characterize the IDACS performance; COP, SMR, and Tda-out. The IDACS COP is represented by the following equation:
COP=˙ma(h1−h2)(˙Wc+˙QAH) | (12) |
where; ṁa is the process air mass flow rate, h1 and h2 are the inlet and outlet air enthalpies, ˙Wc is the required compressor power, and ˙QAH is the additional regeneration heat rate.
The SMR is the removed moisture from the process air for every kilogram of desiccant solution. It is represented by the following equation:
SMR=˙ma(ya1−ya2)˙ms | (13) |
where; ˙ms is the desiccant solution mass flow rate, ya1 and ya2 are the process air humidity ratio at evaporator entrance inlet and exit, respectively.
Four controlling parameters are chosen as independent parameters, namely; desiccant solution temperature in the evaporator and condenser, regenerative temperature (Treg), and desiccant solution concentration (xs). These four independent parameters are selected to be fed in the BP-ANN model to predict the three dependent output parameters. These four independent parameters are chosen because of their significance in the integrated system performance parameters. The BP-ANN technique is utilized to generate the correlation between the four independent parameters and the system performance parameters. Every connection to a neuron has updatable weighting factor connected with it. The algorithm endeavours to limit the least square error between the evaluated and the target output. The desiccant solution temperatures in the evaporator and condenser are denoted hereafter as the evaporator temperature (Teva) and condenser temperature (Tcond), respectively.
The experimental measurements results from Bassuoni [30] are utilized to build and approve the BP-ANN model. 70% of the experimental data was used to train the model while the remaining 30% was used for model validation [26]. The total numbers of the tested datasets are twenty-three which are grouped into two sets; training set and validating set. The first set contains 16 datasets (70% of the total datasets) and has been utilized for training the proposed BP-ANN model. The second set contains the rest 7 datasets (30% of the total datasets) and has been utilized to validate the model. The experimental datasets in the second set are chosen so that they are within the range of the first set.
The proposed BP-ANN model is trained and validated with various working conditions as independent parameters and the IDACS performance parameters as dependent parameter. Consequently, the correlations for the system performance parameters can be written as a function of the four dependent parameters as follows:
COP=f(Teva,Tcond,Treg,xs) | (14) |
SMR=f(Teva,Tcond,Treg,xs) | (15) |
Tda−out=f(Teva,Tcond,Treg,xs) | (16) |
The BP-ANN strategy is utilized to find out relation between IDACS performance parameters and the chosen four different input parameters. Unlike the traditional regression-based models, BP-ANN introduce an effective substitute technique to depict complex nonlinear functions between inputs and outputs data sets while not requiring a close information of unexpressed physical connection.
In this study, the experimental results from Bassuoni [30] are utilized to train the BP-ANN model. The experimental data include 23 datasets. 16 datasets are utilized to train the BP-ANN model while the rest 7 datasets are used to examine the predictive mode of the model. The BP-ANN model is verified by matching the trained system performance parameters with the measurements of Bassuoni [30] as displayed in Figure 5. It can be detected from the figure that, the BP-ANN model achieves a very good agreement with the measurements. Later, the BP-ANN model is conducted to exam its prediction performance as shown in Figure 6. It can be observed from the figure, the relationship between the predictive and experimental results are linear. Figures 5 and 6 demonstrate the perfect estimation of the BP-ANN model. It is important to emphasize that the experimental results, not utilized in the model construction, are utilized to inspect the prediction performance of the developed model.
To assess the precision of the BP-ANN model in the prediction of system performance parameters, the relative deviations versus Teva, Tcond, Treg, and strong solution concentration are exhibited in Figure 7. As can be noticed from the figure, the predicted system performance parameters are in a very good agreement with the experimental measurements. The BP-ANN model has shown relative errors in the learning mode for COP, Tda-out, and SMR within ±0.005%, ±0.006%, and ±0.05%, respectively. The prediction average and the standard deviations of the BP-ANN model in the trained mode for COP, Tda-out, and SMR are within (0.0004%, 0.003%), (0.0007%, 0.004%), and (0.005%, 0.017%), respectively. The definition of relative errors, and average and standard deviations are as follow:
Relative error(ERel)=˙mTran−˙mexp˙mexp | (17) |
Averagedeviation(EAver)=1mm∑1{˙mTran−˙mexp˙mexp} | (18) |
Standarddeviation(ES)=√1mm∑1(ERel−EAver)2 | (19) |
Furthermore, the BP-ANN model is examined in the predictive mode as well. The relative errors of the model for COP, Tda-out, and SMR in the predictive mode are about ±0.006%, ±0.006%, and ±0.004%, respectively. The prediction standard and average deviations in the predictive mode for COP, Tda-out, and SMR are (0.001%, 0.004%), (0.003%, 0.003%), and (0.0007%, 0.002%), respectively. According to the obtained results, the BP-ANN method is a helpful tool in prediction IDACS performance parameters. The influences of some selected parameters, i.e., Teva, Tcond, Treg, and strong solution concentration on the IDACS performance parameters are discussed in the next subsections. The basic values of the system operating parameters and their ranges are presented in Table 1. In each case, only varies the parameter whose effect is studied within the given range in Table 1 while the remaining parameters are kept constant and equal to the basic values given in Table 1. The BP-ANN model is utilized to compute the system performance parameters in the following discussion. The analyses are exhibited graphically in Figures 8-11.
Parameter | Basic value | Range |
desiccant solution volume flow rate | 4 L/min | - |
dry air mass flow rate | 0.25 kg/s | - |
desiccant solution temperatures in the evaporator | 17.5 ℃ | 10 ℃–20 ℃ |
desiccant solution temperatures in the condenser | 48 ℃ | 37.8 ℃–51.8 ℃ |
regenerative temperature | 65 ℃ | 60.5 ℃–88 ℃ |
desiccant solution concentration | 0.4 | 0.315–0.42 |
Figure 8 illustrates the influence of Teva on the IDACS performance parameters. The figure shows the experimental values of the COP, SMR, and Tda-out and that predicted by the BP-ANN model. The figure shows very good matching between the measurements and the predicted values. The influence of Teva on the COP and SMR is shown in Figure 8a, b. The COP is improved while SMR declines as Teva growths. As Teva growths from 10 ℃ to 20 ℃, the COP boosts by about 38.5% while SMR reduces by approximately 38.9%. This can be interpreted by the fact that the growth of Teva, results to reduce the capability of desiccant solution for absorbing moisture from the air. This attributed to the reduction of the vapor pressure difference between desiccant solution and air, which leads to decrease SMR. The growth of Teva results to increase the desiccant concentration at evaporator outlet, which results to lower regeneration heat and greater COP. Also the growth of Teva results to growth Tcond, which results in decrease the required regeneration heat from the auxiliary heater. The variation of the supply air temperature against Teva is presented in Figure 8c. As can be detected from the figure, Tda-out is directly proportional with Teva. As Teva increases from 10 ℃ to 20 ℃, the Tda-out increases by approximately 57.7%.
The correlation of the IDACS performance parameters as a function of Tcond is shown in Figure 9. The figure shows the measurements of the COP, SMR, and Tda-out and the values computed by the model. The figure approves the high accuracy of the BP-ANN model in predicting the IDACS performance parameters. The results show that the COP drops with the growth of Tcond as displayed in Figure 9a. This is due to the fact of increasing Tcond leads to increase the required compressor power resulting in a COP decrease. As Tcond increases from 37.8 ℃ to 51.8 ℃, the COP declines by approximately 25%. As presented in Figure 9b, the SMR growths with the growth of Tcond until Tcond reaches approximately 48 ℃ then SMR starts to decline. This can be interpreted by that the increase of Tcond leads to increase the cooling coil temperature, which results in decrease the capability of the desiccant solution for absorbing moisture from air. The influence of Tcond on the Tda-out is presented in Figure 9c. As can be detected from the figure, Tda-out is directly proportional with Tcond. As Tcond increases from 37.8 ℃ to 51.8 ℃, the Tda-out increases by approximately 37%.
The variation of the IDACS performance parameters as a function of Treg is illustrated in Figure 10. The figure shows the measured values of the performance parameters and the values computed by the model. The measurements and computed values are matching very well as can be observed from the figure. As can be detected from Figure 10a, the COP enhances as Treg increases till Treg reaches approximately 71 ℃ then COP declines with the growth of Treg. This can be interpreted as follows; the growth of Treg leads to growth the concentration of the strong solution and consequently boosts the SMR as displayed in Figure 10b. As the strong solution concentration rises the capability of the desiccant solution for absorbing moisture growths, resulting in large latent load removing capability by the IDACS. This leads to boost COP. With further increase of Treg, the required regeneration energy at the equal solution flow rate increases, which results in a decline of COP. As shown in Figure 10b, the SMR growths with the increase of Treg. As Treg growths from 60.5 ℃ to 88 ℃, the SMR increases by nearly 33.3%. The influence of Treg on the Tda-out is displayed in Figure 10c. The Tda-out is directly proportional to Tda-out. As Treg growths from 60.5 ℃ to 88 ℃, the Tda-out increases by almost 36.6%.
Figure 11 shows the variation of the IDACS performance parameters as a function of strong solution concentration. The figure shows the measured values of the performance parameters and the values computed by the model. The figure displays the high capability of the BP-ANN model to reproduce the measured values. As can be detected from Figure 11a, b, and c the COP, SMR, and Tda-out are directly proportional with the strong solution concentration. This can be interpreted as follows, the growth of strong solution concentration leads to boost the capability of desiccant solution for absorbing moisture. The removed cooling load from the process air increases as the absorbed moisture from the air increases. This leads to increase the system performance parameters. As strong solution concentration increases from 0.315 to 0.42 leads to increase the COP, SMR, and Tda-out by nearly 50%, 28% and 40.2%; respectively.
The performance of an integrated desiccant air conditioning system powered by solar energy is investigated utilizing BP-ANN. The system performance is characterized by the system coefficient of performance, outlet dry air temperature, and specific moisture removal. The integrated system has basically four controlling parameters; desiccant solution temperature in the evaporator and condenser, regeneration temperature, and desiccant solution concentration. These four parameters are utilized to establish the BP-ANN model to assess the system performance parameters. The model shows very small relative differences in the trained mode for system coefficient of performance, outlet dry air temperature, and specific moisture removal within ±0.005%, ±0.006%, and ±0.05%, respectively. The predictive performance of the proposed BP-ANN model is inspected as well showing good correlation with experimental measurements. The relative differences of the system performance parameters calculated by the BP-ANN model in the prediction mode from the measurements are within ±0.006%, ±0.006%, and ±0.004% for system coefficient of performance, outlet dry air temperature, and specific moisture removal, respectively. The system performance is affected in a various extents by the working parameters. Accordingly, the effects of regeneration temperature, desiccant solution temperature in the condenser and evaporator, and strong solution concentration on the system performance are examined. The system COP is directly proportional to the desiccant solution temperature in the evaporator, strong solution concentration, and regeneration temperature (until 71 ℃ then it is reversed) while inversely proportional to desiccant solution temperature in the condenser. More and wider range of experimental data are needed to expand the application range of the proposed ANN model.
This study is supported by Taif University under research grant 1-439-6073. The authors would like to thank Taif University for the financial support.
All authors declare no conflict of interest in this paper.
[1] |
Watanabe H, Komura T, Matsumoto R, et al. (2019) Design of ionic liquids as liquid desiccant for an air conditioning system. Green Energy Environ 4: 139–145. doi: 10.1016/j.gee.2018.12.005
![]() |
[2] |
Mahmoud KG, Ball HD (1992) Liquid desiccant systems simulation. Int J Refrig 15: 74–80. doi: 10.1016/0140-7007(92)90030-X
![]() |
[3] |
Kessling W, Laevemann E, Peltzer M (1998) Energy storage in open cycle liquid desiccant cooling systems. Int J Refrig 21: 150–156. doi: 10.1016/S0140-7007(97)00045-5
![]() |
[4] |
Elasyed SS, Hamamoto Y, Akisawa A, et al. (2006) Analysis of an air cycle refrigerator driving air conditioning system integrated desiccant system. Int J Refrig 29: 219–228. doi: 10.1016/j.ijrefrig.2005.05.014
![]() |
[5] |
Li Z, Liu XH, Jiang Y, et al. (2005) New type of fresh air processor with liquid desiccant total heat recovery. Energy Build 37: 587–593. doi: 10.1016/j.enbuild.2004.09.017
![]() |
[6] | Studak JW, Peterson JL (1988) A preliminary evaluation of alternative liquid desiccants for a hybrid desiccant air conditioner. In: Proceeding of the fifth annual symposium on improving building energy efficiency in hot and humid climates 13: 155–159. |
[7] | Maclaine-Cross IL (1988) Proposal for a hybrid desiccant air conditioning system. In: Proceedings of the symposium on desiccant cooling applications, ASHRAE Winter Meeting, Dallas, TX. |
[8] | Saunders JH, Wilkinson WH, Landstorm DK, et al. (1989) A hybrid space conditioning system combining a gas-fired chiller and a liquid desiccant dehumidifier. In: Proceeding of the eleventh annual ASME solar energy conference, San Diego, CA, 207–212. |
[9] |
Sick F, Bushulte TK, Klein SA, et al. (1988) Analysis of the seasonal performance of hybrid desiccant cooling systems. Sol Energy 40: 211–217. doi: 10.1016/0038-092X(88)90043-6
![]() |
[10] | Waugaman DG, Kini A, Kettleborough CF (1992) A review of desiccant cooling systems. J Energy Resour Technol 115: 1–8. |
[11] |
Park J, Dong H, Cho H, et al. (2019) Energy benefit of a cascade liquid desiccant dehumidification in a desiccant and evaporative cooling-assisted building air-conditioning system. Appl Therm Eng 147: 291–301. doi: 10.1016/j.applthermaleng.2018.10.101
![]() |
[12] |
Cheng Q, Jiao S (2018) Experimental and theoretical research on the current efficiency of the electrodialysis regenerator for liquid desiccant air-conditioning system using LiCl solution. Int J Refrig 96: 1–9. doi: 10.1016/j.ijrefrig.2018.09.001
![]() |
[13] |
Cheng Q, Xu W (2017) Performance analysis of a novel multi-function liquid desiccant regeneration system for liquid desiccant air-conditioning system. Energy 140: 240–252. doi: 10.1016/j.energy.2017.08.092
![]() |
[14] |
Speerforck A, Ling J, Aute V, et al. (2017) Modeling and simulation of a desiccant assisted solar and geothermal air conditioning system. Energy 141: 2321–2336. doi: 10.1016/j.energy.2017.11.151
![]() |
[15] | Kinsara AA, Elsayed MM, Al-Rabghi OM (1996) Proposed energy efficient air conditioning system using liquid desiccant. Appl Therm Eng 16(1996): 791–806. |
[16] |
Mohan BS, Maiya MP, Tiwari S (2008) Performance characterization of liquid desiccant columns for a hybrid air-conditioner. Appl Therm Eng 28: 1342–1355. doi: 10.1016/j.applthermaleng.2007.10.015
![]() |
[17] |
Jia CX, Dai YJ, Wu JY, et al. (2006) Analysis on a hybrid desiccant air-conditioning system. Appl Therm Eng 26: 2393–2400. doi: 10.1016/j.applthermaleng.2006.02.016
![]() |
[18] |
Jongsoo J, Yamaguchi S, Saito K, et al. (2010) Performance analysis of four-partition desiccant wheel and hybrid dehumidification air-conditioning system. Int J Refrig 33: 496–509. doi: 10.1016/j.ijrefrig.2009.12.001
![]() |
[19] |
Ge TS, Ziegler F, Wang RZ, et al. (2010) Performance comparison between a solar driven rotary desiccant cooling system and conventional vapor compression system. Appl Therm Eng 30: 724–731. doi: 10.1016/j.applthermaleng.2009.12.002
![]() |
[20] |
Niu X, Xiao F, Ge G (2010) Performance analysis of liquid desiccant based air-conditioning system under variable fresh air ratios. Energy Build 42: 2457–2464. doi: 10.1016/j.enbuild.2010.08.027
![]() |
[21] |
Ling J, Kuwabara O, Hwang Y, et al. (2011) Experimental evaluation and performance enhancement prediction of desiccant assisted separate sensible and latent cooling air conditioning system. Int J Refrig 34: 946–957. doi: 10.1016/j.ijrefrig.2010.12.008
![]() |
[22] |
Ge TS, Dai YJ, Wang RZ, et al. (2010) Experimental comparison and analysis on silica gel and polymer coated fin-tube heat exchangers. Energy 35: 2893–2900. doi: 10.1016/j.energy.2010.03.020
![]() |
[23] |
Ge TS, Dai YJ, Wang RZ (2011) Performance study of silica gel coated fin-tube heat exchanger cooling system based on a developed mathematical model. Energy Convers Manage 52: 2329–2338. doi: 10.1016/j.enconman.2010.12.047
![]() |
[24] |
Ge TS, Dai YJ, Wang RZ (2012) Simulation investigation on solar powered desiccant coated heat exchanger cooling system. Appl Energy 93: 532–540. doi: 10.1016/j.apenergy.2011.11.089
![]() |
[25] |
Mohammad AT, Mat SB, Sulaiman MY, et al. (2013) Artificial neural network analysis of liquid desiccant regenerator performance in a solar hybrid air-conditioning system. Appl Therm Eng 59: 389–397. doi: 10.1016/j.applthermaleng.2013.06.006
![]() |
[26] |
Mohammad AT, Mat SB, Sulaiman MY, et al. (2013) Implementation and validation of an artificial neural network for predicting the performance of a liquid desiccant dehumidifier. Energy Convers Manage 67: 240–250. doi: 10.1016/j.enconman.2012.12.005
![]() |
[27] |
Sohani A, Sayyaadi H, Balyani HH, et al. (2016) A novel approach using predictive models for performance analysis of desiccant enhanced evaporative cooling systems. Appl Therm Eng 107: 227–252. doi: 10.1016/j.applthermaleng.2016.06.121
![]() |
[28] |
Cheng X, Peng D, Yin Y, et al. (2019) Experimental study and performance analysis on a new dehumidifier with outside evaporative cooling. Build Environ 148: 200–211. doi: 10.1016/j.buildenv.2018.11.006
![]() |
[29] |
Chen Y, Yang H, Luo Y (2018) Investigation on solar assisted liquid desiccant dehumidifier and evaporative cooling system for fresh air treatment. Energy 143:114–127. doi: 10.1016/j.energy.2017.10.124
![]() |
[30] |
Bassuoni MM (2014) Experimental performance study of a proposed desiccant based air conditioning system. J Adv Res 5: 87–95. doi: 10.1016/j.jare.2012.12.002
![]() |
[31] |
Saleh B, Aly AA (2016) Artificial neural network model for evaluation the effect of surface properties amendment on slurry erosion behavior of AISI 5117 steel. Ind Lubr Tribol 68: 676–682. doi: 10.1108/ILT-12-2015-0190
![]() |
[32] |
Saleh B, Aly AA (2016) Artificial neural network models for depicting mass flow rate of R22, R407C and R410A through electronic expansion valves. Int J Refrig 63: 113–124. doi: 10.1016/j.ijrefrig.2015.11.010
![]() |
[33] | Saleh B, Aly AA, Alogla AF, et al. (2019) Mass flow rate assessment of R22 and R407C-R600a-R290 mixture in adiabetic straight capillary tubes using back propagation artificial neural network. Cienc Tec Vitivinic 34: 31–47. |
1. | Liang Zhang, Liang Zhang, Top-N recommendation algorithm integrated neural network, 2020, 0941-0643, 10.1007/s00521-020-05452-y | |
2. | Sampath Suranjan Salins, S.V. Kota Reddy, Shiva Kumar, Experimental investigation of the influence of vapor compression refrigeration in a multistage reciprocating dehumidifier test rig, 2021, 199, 13594311, 117546, 10.1016/j.applthermaleng.2021.117546 | |
3. | Daniel Chuquin-Vasco, Dennise Chicaiza-Sagal, Cristina Calderón-Tapia, Nelson Chuquin-Vasco, Juan Chuquin-Vasco, Lidia Castro-Cepeda, Forecasting mixture composition in the extractive distillation of n-hexane and ethyl acetate with n-methyl-2-pyrrolidone through ANN for a preliminary energy assessment, 2024, 12, 2333-8334, 439, 10.3934/energy.2024020 | |
4. | Imtiyaz Hussain, Uzair Sajjad, Showkat Ahmad Bhat, Naseem Abbas, Ahsan Ali, Muhammad Sultan, Hafiz Muhammad Ali, Khalid Hamid, Wei-Mon Yan, Najaf Rubab, Potential evaluation of AI-based surrogate models to find the robust and optimal method for thermal performance assessment of desiccant air-conditioning systems, 2025, 25, 25901745, 100782, 10.1016/j.ecmx.2024.100782 |
Parameter | Basic value | Range |
desiccant solution volume flow rate | 4 L/min | - |
dry air mass flow rate | 0.25 kg/s | - |
desiccant solution temperatures in the evaporator | 17.5 ℃ | 10 ℃–20 ℃ |
desiccant solution temperatures in the condenser | 48 ℃ | 37.8 ℃–51.8 ℃ |
regenerative temperature | 65 ℃ | 60.5 ℃–88 ℃ |
desiccant solution concentration | 0.4 | 0.315–0.42 |
Parameter | Basic value | Range |
desiccant solution volume flow rate | 4 L/min | - |
dry air mass flow rate | 0.25 kg/s | - |
desiccant solution temperatures in the evaporator | 17.5 ℃ | 10 ℃–20 ℃ |
desiccant solution temperatures in the condenser | 48 ℃ | 37.8 ℃–51.8 ℃ |
regenerative temperature | 65 ℃ | 60.5 ℃–88 ℃ |
desiccant solution concentration | 0.4 | 0.315–0.42 |