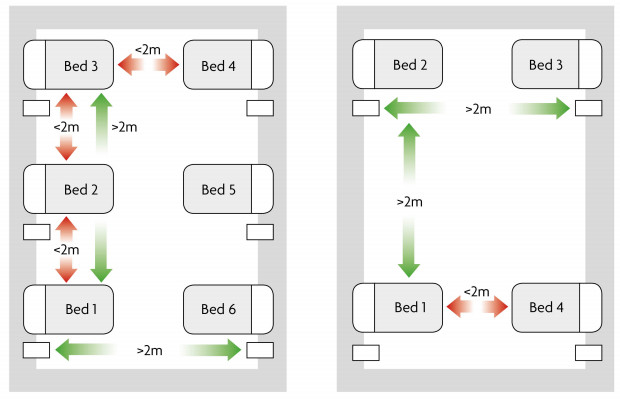
Beta-thalassemia major (β-TM) is a genetic disorder, prevalent especially in the Mediterranean region, Southeast Asia, and the Indian subcontinent. With improvements in management over the years, β-TM has transitioned from a fatal childhood disease to a chronic condition. However, in Pakistan, there is still a lack of a comprehensive national policy and strategic plan, which has resulted in a growing number of β-TM patients, placing a substantial burden on individuals and the national healthcare system. This scoping review is aimed to understand obstacles faced by β-TM patients in Pakistan. For this review, 26 unique articles were identified by using the PRISMA flow guidelines. PubMed and Google Scholar were used with the MESH term Beta-Thalassemia Major AND Pakistan, and the duration was set between 2012–2022. Then, the reviewers created a spreadsheet using Microsoft Excel to add in the data from the studies selected. Inductive and deductive approaches were used for thematic analysis. Additionally, we critically analyzed the current landscape of β-TM in Pakistan. The main challenges in β-TM care in Pakistan are suboptimal transfusion services and a poor complication management. Due to the need of chronic blood transfusions, transfusion-transmitted infection (TTI) incidence within this patient population is high. These largely include hepatitis C, hepatitis B, and the Human immunodeficiency virus (HIV). TTIs impact the quality of life of these patients and their overall survival. Furthermore, psychosocial morbidities are also prevalent in β-TM patients, with increased levels of hostility, anxiety, and depressive symptoms, thus emphasizing the critical need for sustained psychological support. Access to quality treatments is constrained, with notable disparities between public and private sector hospitals. Additionally, the financial burden on β-TM patients is considerable, which contributes to economic strain and more hardships on the already suffering families. The review concludes that the absence of a unified national policy exacerbates these challenges, which results in an escalating burden of β-TM nationwide. To address these issues, essential recommendations include the following: the implementation of a standardized protocol for β-TM care, the enhancement of access to quality care, the provision of iron chelation therapy, and safeguarding safe blood transfusion practices. Prevention programs, along with increased public awareness and education about β-TM and carrier screening, are pivotal. Collaborative efforts with international partners and drawing insights from successful strategies in countries with similar β-TM burdens can aid in mitigating the overall impact of β-TM in Pakistan and improving the quality of life of the affected individuals.
Citation: Ali Hussain Ansari, Saqib Hussain Ansari, Mubarak Jabeen Salman, Muhammad Usman Hussain Ansari, Rawshan Jabeen. A scoping review on the obstacles faced by beta thalassemia major patients in Pakistan- Matter of policy investment[J]. AIMS Public Health, 2024, 11(4): 1105-1124. doi: 10.3934/publichealth.2024057
[1] | David Moreno-Martos, Sean Foley, Benjamin Parcell, Dumitru Trucu, Raluca Eftimie . A computational investigation of COVID-19 transmission inside hospital wards and associated costs. Mathematical Biosciences and Engineering, 2022, 19(7): 6504-6522. doi: 10.3934/mbe.2022306 |
[2] | Qian Shen . Research of mortality risk prediction based on hospital admission data for COVID-19 patients. Mathematical Biosciences and Engineering, 2023, 20(3): 5333-5351. doi: 10.3934/mbe.2023247 |
[3] | Haonan Zhong, Wendi Wang . Mathematical analysis for COVID-19 resurgence in the contaminated environment. Mathematical Biosciences and Engineering, 2020, 17(6): 6909-6927. doi: 10.3934/mbe.2020357 |
[4] | Zhihao Zhang, Ting Zeng, Yijia Wang, Yinxia Su, Xianghua Tian, Guoxiang Ma, Zemin Luan, Fengjun Li . Prediction Model of hospitalization time of COVID-19 patients based on Gradient Boosted Regression Trees. Mathematical Biosciences and Engineering, 2023, 20(6): 10444-10458. doi: 10.3934/mbe.2023459 |
[5] | Sarita Bugalia, Vijay Pal Bajiya, Jai Prakash Tripathi, Ming-Tao Li, Gui-Quan Sun . Mathematical modeling of COVID-19 transmission: the roles of intervention strategies and lockdown. Mathematical Biosciences and Engineering, 2020, 17(5): 5961-5986. doi: 10.3934/mbe.2020318 |
[6] | Sarafa A. Iyaniwura, Musa Rabiu, Jummy F. David, Jude D. Kong . Assessing the impact of adherence to Non-pharmaceutical interventions and indirect transmission on the dynamics of COVID-19: a mathematical modelling study. Mathematical Biosciences and Engineering, 2021, 18(6): 8905-8932. doi: 10.3934/mbe.2021439 |
[7] | Xinmiao Rong, Liu Yang, Huidi Chu, Meng Fan . Effect of delay in diagnosis on transmission of COVID-19. Mathematical Biosciences and Engineering, 2020, 17(3): 2725-2740. doi: 10.3934/mbe.2020149 |
[8] | Jie Bai, Xiunan Wang, Jin Wang . An epidemic-economic model for COVID-19. Mathematical Biosciences and Engineering, 2022, 19(9): 9658-9696. doi: 10.3934/mbe.2022449 |
[9] | Yukun Tan, Durward Cator III, Martial Ndeffo-Mbah, Ulisses Braga-Neto . A stochastic metapopulation state-space approach to modeling and estimating COVID-19 spread. Mathematical Biosciences and Engineering, 2021, 18(6): 7685-7710. doi: 10.3934/mbe.2021381 |
[10] | Ziqiang Cheng, Jin Wang . Modeling epidemic flow with fluid dynamics. Mathematical Biosciences and Engineering, 2022, 19(8): 8334-8360. doi: 10.3934/mbe.2022388 |
Beta-thalassemia major (β-TM) is a genetic disorder, prevalent especially in the Mediterranean region, Southeast Asia, and the Indian subcontinent. With improvements in management over the years, β-TM has transitioned from a fatal childhood disease to a chronic condition. However, in Pakistan, there is still a lack of a comprehensive national policy and strategic plan, which has resulted in a growing number of β-TM patients, placing a substantial burden on individuals and the national healthcare system. This scoping review is aimed to understand obstacles faced by β-TM patients in Pakistan. For this review, 26 unique articles were identified by using the PRISMA flow guidelines. PubMed and Google Scholar were used with the MESH term Beta-Thalassemia Major AND Pakistan, and the duration was set between 2012–2022. Then, the reviewers created a spreadsheet using Microsoft Excel to add in the data from the studies selected. Inductive and deductive approaches were used for thematic analysis. Additionally, we critically analyzed the current landscape of β-TM in Pakistan. The main challenges in β-TM care in Pakistan are suboptimal transfusion services and a poor complication management. Due to the need of chronic blood transfusions, transfusion-transmitted infection (TTI) incidence within this patient population is high. These largely include hepatitis C, hepatitis B, and the Human immunodeficiency virus (HIV). TTIs impact the quality of life of these patients and their overall survival. Furthermore, psychosocial morbidities are also prevalent in β-TM patients, with increased levels of hostility, anxiety, and depressive symptoms, thus emphasizing the critical need for sustained psychological support. Access to quality treatments is constrained, with notable disparities between public and private sector hospitals. Additionally, the financial burden on β-TM patients is considerable, which contributes to economic strain and more hardships on the already suffering families. The review concludes that the absence of a unified national policy exacerbates these challenges, which results in an escalating burden of β-TM nationwide. To address these issues, essential recommendations include the following: the implementation of a standardized protocol for β-TM care, the enhancement of access to quality care, the provision of iron chelation therapy, and safeguarding safe blood transfusion practices. Prevention programs, along with increased public awareness and education about β-TM and carrier screening, are pivotal. Collaborative efforts with international partners and drawing insights from successful strategies in countries with similar β-TM burdens can aid in mitigating the overall impact of β-TM in Pakistan and improving the quality of life of the affected individuals.
There can be high mortality and morbidity for patients due to the spread of infections in hospitals (i.e., nosocomial or HAI) from infected patients to healthy patients, either directly through sharing the same rooms and environment, or indirectly through healthcare workers (HCWs) attending infected and susceptible patients. The novel coronavirus disease (COVID-19) that has been reported since January 2020, was shown to cause a relatively high prevalence of nosocomial infections: between 12.5−15% in the UK [1,2] and up to 44% in China [3]. In comparison, other coronaviruses such as SARS (Severe Acute Respiratory Syndrome, 2003) and MERS (Middle East Respiratory Syndrome, 2012), had estimated nosocomial infection prevalence of 36% and 56%, respectively [1].
In UK hospitals, patients symptoms and results of real-time polymerase chain reaction (RT-PCR) inform targeted infection prevention and control (IPC) measures [1,2]. Patients who do not meet the clinical criteria for the COVID-19 disease may not be tested, and are usually admitted into shared bays, i.e., rooms of up to 6 beds that usually share the same bathroom facilities [2]. There can be problems with this approach, as highlighted in a recent study [2] in which it was shown that more than half of the patients who acquired COVID-19 in a hospital setting were identified as having been in the same hospital bay with other infected patients.
There is also the issue of asymptomatic cases, which pose a particular risk for healthcare transmission. Various studies in the literature have estimated the proportion of asymptomatic COVID-19 infections: from 17.9% asymptomatic passengers on board of the Princess Cruises ship [4], to 30.8% asymptomatic Japanese nationals evacuated from Wuhan [5], 50–75% asymptomatic residents of an Italian village (Vo'Euganeo) [6], and even 81% asymptomatic passengers of an un-named cruise ship [7]. Since asymptomatic infections cannot be recognised if they are not confirmed by laboratory tests [5], it is highly likely that asymptomatic patients are found in hospitals, and they can be involved in the spread of COVID-19 inside these hospitals. In fact, a study in Beijing [8] showed that 5% of hospitalised patients were asymptomatic. This complicates the issue of nosocomial transmission of COVID-19, since hospitals do not test/re-test routinely all patients hospitalised for various interventions (not COVID-19 related).
In regard to nosocomial transmissions, a 2015 report by the National Institute for Health Research [9] concluded that while hospital patients preferred a single-room accommodation, staff preferred a mix of single-rooms and shared-rooms accommodation. Moreover, a study examining hospital design [9] mentioned that it was impossible to perform an analysis of the impact of single-room hospital design on the transmission of HAIs, and given that some of the studies they cited showed a decrease in infections while other studies showed an increase in infections, the authors concluded that there was no clear evidence of the impact of single rooms on HAIs. Since some of the published COVID-19 studies [2] emphasised that ≈55% of HAIs were connected with infected patients in shared bays, it is important to understand COVID-19 transmission in these hospital bays.
The common limitation of clinical studies that focus on the spread of COVID-19 inside hospitals (see, for example, [1,2,10]), is that they identify and count only PCR-symptomatic patients and not hospitalised patients that were exposed to the disease but were discharged before developing symptoms. Also, these studies cannot identify contacts between patients and staff, which might have had undiagnosed COVID-19 [2]. To address this lack of information, mathematical modelling and computational simulations are the perfect tool to test various scenarios regarding the mechanisms that can reduce the COVID-19 transmission in hospitals. Currently there are very few mathematical studies that focus on the nosocomial transmission of SARS-CoV-2 (despite the huge number of studies focused on the general viral spread through communities: e.g., from studies that focus only on symptomatic transmission [11,12,13,14,15], to studies that focus on both symptomatic and asymptomatic transmission [16,17,18,19], or transmission during the latent stages of the exposed period and during the symptomatic infectious period [14,20], transmission by various age classes [21,22], the impact of control measures such as school or workplace closures, or self-imposed and government-imposed social distancing measures [11,18,22,23,24,25]). We are aware of only one modelling study in [26], where the authors used a classical SEIR continuous model to investigate the transmission dynamics of COVID-19 in English hospitals, following interactions between patients and HCWs.
Here we present a new study that considers a mathematical modelling approach to investigate the spread of COVID-19 among patients hospitalised in 4-bed versus 6-bed bays, which represents a standard bay structure in many hospitals in the UK; see also Figure 1. To this end we consider a network model that accounts for stochastic fluctuations between interconnected individuals inside the same hospital bay, where every single bed can be occupied by a susceptible, exposed, infectious or a recovered individual. With the help of this model we investigate computationally a variety of scenarios: from variations in the recovery and incubation periods; to the position of infected individuals in specific beds inside 6-bed bays; the effect of removing infected individuals from these bays; the effect of accommodating patients in single rooms; the effect of having asymptomatic individuals; and the role of periodic testing of hospitalised patients.
Since we are interested in investigating the transmission of infectious diseases (including COVID-19) across 4-bed and 6-bed hospital bays, which are the standard bays in many UK hospitals, in the following we consider an individual-based (network) model that tracks susceptible (S; i.e., patients vulnerable to develop an infection), exposed (E; i.e., patients incubating the virus), infected (I; i.e., patients that can transmit the disease) and recovered (R) individuals in each of the 4 or 6 beds inside these hospital bays. Following the approach in [27], each individual In,t is described by a set of characteristics, In,t=[Cn,1,t,Cn,2,t,...,Cn,m,t], where n=1,...N describes the number of individuals in the hospital bay, m is the number of characteristics, and t is the time. These characteristics are as follows:
● Cn,1,t is the epidemiological class. It can be 0 (susceptible), 1 (exposed), 2 (infected) or 3 (recovered).
● Cn,2,t is the bed at which the individual is placed.
● Cn,3,t is the time since the individual acquired the virus (start of the incubation period).
● Cn,4,t is the duration of the incubation period.
● Cn,5,t is the time since the individual has become infectious (virus transmission is now possible).
● Cn,6,t is the time that takes the individual to recover once becomes infectious.
The following rules are used to update each of these states:
● Epidemiological class update:
- A susceptible individual might become exposed with probability β if he/she interacts with an infected individual. Throughout this study we assume that the infection probability β depends on the distance between the susceptible individual and the infectious one. This modelling assumption is consistent with the clinical assumption of predominant transmission of SARS-CoV-2 via respiratory droplets [28]. Some recent studies have suggested that airborne transmission may be possible in certain circumstances, however to date the authors report that there is no perfect experimental data proving or disproving airborne transmission of SARS-CoV-2 [29]. Moreover, the evidence seems inconsistent with airborne transmission especially in well-ventilated spaces [29]. For this reason, and in accordance with current public health recommendations [30], in this study we assume that a distance of at least 2 m distance should be maintained to minimise the spread of SARS-CoV-2. Thus, here we consider the infection probability β to be a fixed number that depends on the distance between beds: probability is 1 if the distance is 0, probability is almost 0 if the distance is greater than 2; see the exponential distribution in Figure 2. The distance dij between two beds i and j (see also Figure 1) is given by matrices Dk=(dij), with i,j=1..k and k∈{4,6}:
D4=(02.565.52.505.5665.502.55.562.50),D6=(01.536.35.75.51.501.55.75.55.731.505.55.76.36.35.75.501.535.75.55.71.501.55.55.76.331.50). | (2.1) |
Therefore, the probability of becoming infected following the "contact" between individuals in beds i and j (where "contact" is understood in a very loose sense) is β(dij); see also Figure 2.
We assume that there are a finite number of "contacts" within a day, and at each time step we simulate a "contact" between an infected individual and a susceptible one. Throughout this study we consider a time step Δt=0.1 days, which corresponds to an assumption of 10 contacts per day. (Note that changing the time step, i.e., the number of "contacts", impacts the speed at which the disease spreads but not the type of spread.)
- An exposed individual becomes infected once his/her incubation period is finished (i.e., Cn,3,t>Cn,4,t).
- An infected individual recovers if the time since he/she has been infected surpasses the expected duration of the infection (i.e., Cn,5,t>Cn,6,t).
● Incubation time update: If the individual is exposed, the incubation time update is increased by Δt at each iteration, until the individual becomes infectious.
● Incubation stage: We describe the duration of the incubation stage using an Erlang distribution with mean 1/σ and shape 2. Since the results obtained with this Erlang distribution are relatively similar to the results obtained with an exponential distribution (not shown here; for details see [31]), throughout this study we use only Erlang distributions.
● Infection time update: If the individual is infected, the infection time update is increased by Δt at each iteration, until the individual recovers.
● Recovery stage: Similar to the incubation stage, to describe the duration of the recovery stage we use an Erlang distribution with mean 1/γ and shape 2.
Before we show the numerical results, we needs to discuss briefly the parameter values for which these results are obtained. Even if this model can be generally applied to the spread of various infectious diseases through hospital bays, here we focus on the spread of COVID-19. Therefore, the parameter values (i.e., mean recovery period, mean incubation period) are chosen for this particular disease, COVID-19, and are summarised in Table 1.
Parameters (& units) | Description | Range | Most common values | References |
σ (day−1) | Incubation rate | 0.071−0.5 | 0.143, 0.192 | [11,12,13,14,15,16,18,19,20,22,23,24,25,32,33,34,35,36,37,38] |
1/σ (day) | Mean incubation period | 2−14 | 7, 5.2 | [11,12,13,14,15,16,18,19,20,22,23,24,25,32,33,34,35,36,37,38] |
γ (day−1) | Recovery rate | 0.055−0.621 | 0.143−0.25 | [11,12,13,14,15,16,18,19,21,22,23,24,32,33,35,36,37,39,40] |
1/γ (day) | Mean recovery / infection period | 1.61−18 | 4−7 | [11,12,13,14,15,16,18,19,21,22,23,24,32,33,35,36,37,39,40] |
For the numerical simulations presented here, we fix 1γ=7 days, 1σ=5.2 days, and we run the simulations up to Tmax=60 days. We run this network model 300 times, and we compute the mean and standard deviation of these 300 runs for individuals in each epidemiological state (i.e., susceptibles, exposed, infectives, recovered), for each of the 4-beds or 6-beds bays. We already mentioned before that the probability of infection β is given by a decaying exponential which depends on the distance between beds (see Figure 2). Finally, as initial conditions for these simulations, we assume that all patients are susceptible, with the exception of the individual placed in bed 2 (see Figure 1), who is exposed. This describes the situation where an individual, not being thought to have the disease (although they were exposed), is introduced into a hospital bay full of patients that can become infected. For simplicity, and to be able to compare the outcome of various simulations, we arbitrarily chose to place the exposed individual in bed 2. However, in Section 3.2 we discuss also what happens when we place the initial exposed individual in a different bed.
We start this numerical investigation of our network model by assuming that hospitalised patients are accommodated in shared bays with either 4 beds or 6 beds. In Figure 3 we show the baseline dynamics of this model when an infected patient is hospitalised in bed 2 of a: (a), (b) 4-bed bay; (c), (d) 6-bed bay. We observe that in a 4-bed bay the average number of exposed and infected individuals decreases towards zero faster than in a 6-bed bay (see panels (a), (c)). Moreover, we note that for a 4-bed bay, individuals placed in beds 3 and 4 have an almost zero probability of becoming infected, when the original exposed patient was placed in bed 2 (see Figure 3(b)). For a 6-bed bay, individuals placed in beds 4, 5, and 6 have an almost zero probability of becoming infected, when the original exposed patient was placed in bed 2 (see Figure 3(d)). This means that the distance between the infected bed 2 and the beds on the opposite walls is large enough to avoid the infection.
In a 4-bed bay it does not matter where we place the first exposed individual, as the disease spreads equally in all beds due to the symmetry of bed distribution. However, in a 6-bed bay, it is important where we place the exposed individual: the disease is more likely to reach all the individuals in a room if the exposed individual is placed in beds 2 or 5. Next we compare how the disease evolves depending on where we place the first exposed individual in a 6-bed bay.
In Figure 4 we assume that the first exposed individual is placed in bed 1, which is different from the case in Figure 3(b), (d) where we assumed that the first exposed individual was placed in bed 2.
We see that by placing the first exposed individual at bed 1, the disease spreads a bit slower compared to the case when this individual is placed in bed 2. More precisely, when we place him/her in bed 1, the average number of susceptible individuals decreases and reaches a steady value around t=35 days, whereas when we place him/her in bed 2 the steady value is reached around t=28 days.
The parameters that characterise the transmission of COVID-19, e.g., mean incubation period and mean recovery period, are not fixed, with various studies showing different estimations; see Table 1. Here we focus on a 6-bed bay, and investigate the impact of incubation and recovery rates, showing only results for the minimum and the maximum values of these parameters. When varying the incubation rate we fix the mean recovery period to 1/γ=7 days, and when varying the recovery rate we fix the mean incubation period to 1/σ=5.2 days. (Here we do not vary β, since this parameter is given by the distance between beds; see caption of Figure 2.)
Figure 5 shows model dynamics when we modify the recovery period. The most significant difference between the cases 1/γ=1.61 days and 1/γ=18 days is in the amplitude and shape of the infected curve. For the case where the recovery period is 18 days (Figure 5(c)) there is a time interval during which the number of infected individuals reaches a value of 2 (within one standard deviation). Since the recovery periods are larger (the mean recovery period is 18 days), there is more time in which susceptible individuals can become infected. This also affects the values of the average number of susceptible and recovered individuals. In terms of the probability of being at each epidemiological state per bed, there is a higher probability of becoming infected when the mean recovery period is 18 days (panels (d)), due to larger exposure to infected individuals.
The effects of varying the incubation period are shown in Figure 6. The most significant differences between the cases 1/σ=2 days and 1/σ=14 days can be found in the average numbers of exposed and infected individuals. When the mean incubation period is longer (Figure 6(c)), the mean value for the exposed and infected individuals reaches zero later in time. In terms of the probability of being at each epidemiological state per bed, the probability of being infected is higher for earlier times (t=10 days), when the mean incubation period is shorter (panels (c)).
Here we investigate the effect of removing from the bay every patient that is classified as infected (we remove this patient when the incubation period ends). We do this in order to reproduce the situation in which patients are transferred to separate COVID-19-only bays right at the moment they present clinical symptoms (assuming all of them are symptomatic individuals). Once we remove the infected patient, we place a new susceptible individual in the free bed. We emphasise that we modelled this out of mathematical interest (to keep the same number of patients in a bay and continue the simulations), as the standard clinical practice does not put a new person back in a bay with potential incubating patients.
Since various studies reported pre-symptomatic transmission of SARS-CoV-2 during the incubation period [41,42,43], and following the approach in some published modelling studies [14,20] which accounted for the spread of infection during the latent period, in the following we assume that exposed individuals can transmit the disease, but at a much lower rate compared to the infected ones (we consider here an overlap between exposed and pre-symptomatic cases). Since we could not find in the literature any values for the probability of transmission, in this sub-section we set the probability of infection from the exposed individuals to be 20% of the probability of infection from the infected individuals. In Figure 7 we can see the differences between 4-bed bays and 6-bed bays.
On one hand, in a 4-bed bay the disease disappears after a month. This is when we have 4 susceptible and 0 exposed in all the runs (Figure 7(a)) or the probability of being in the exposed state is zero for times greater than 30 days (Figure 7(b)). On the other hand, in a 6-bed bay the disease disappears after 50 days, which is when the probability of being in the exposed state is zero for t>50 days (Figure 7(d)). Both bays have in common the fact that the individuals across the aisle from the infected bed 2 (i.e., beds 3 and 4 in a 4-bed bay and beds 4, 5 and 6 in a 6-bed bay) do not become infected.
Until now, we have assumed that patients in a hospital bay can become infected by interacting with exposed/infected individuals from the same bay. However, sometimes they have to share bathrooms with individuals from other bays, which increases the probability of becoming infected (it has been shown that the virus can survive for several hours on different surfaces [45]). Moreover, patients interact with hospital staff (doctors, nurses, etc.), who might transmit the disease with a very low probability [44], even if they follow the preventive measures (washing their hands, changing their clothes, wearing masks, etc.).
To implement this aspect in our simulations, we note that the few studies that estimated the secondary attack rates for COVID-19 (defined as the probability that infection occurs among susceptibles, following contacts with an infectious person or infectious environment [46]) have shown wide ranges: from 0.45% in [47], to 35% in [48], and even 68.57% in [49]. The variability in the estimations for these attack rates might have been caused by the small sample sizes considered. To address this issue, a meta-analysis study focused on Taiwan patients [50] estimated a secondary attack rate for the nosocomial spread of SARS-CoV-2 between 0.73–2.93%. The authors of this study also emphasised the low attack rates that characterised the beginning of the epidemics (the data in [50] focused on cases between January and April 2020), and mentioned that higher attack rates were expected if the epidemics would continue. They also emphasised that in Taiwan the nosocomial attack rates were very low, due to staff training and increased awareness as a result of previous experiences with SARS.
Based on these estimates, for our study we assume that each individual has a probability of 5% of becoming infected each day due to interactions with hospital staff and/or sharing the bathroom.
In Figure 8 we see, for the first time in our simulations, that all patients have become infected in both 4-bed bays and 6-bed bays. Before considering interactions with staff members and sharing bathrooms, individuals at beds 3 and 4 (4-bed bay) and individuals at beds 4, 5 and 6 (6-bed bay) rarely became infected because the distance between beds was large enough to avoid the spread of the disease. However, if there are other ways of catching the disease, these patients will surely become infected. In fact, the probability of being recovered by t=60 days is 1 for all the beds in both bays.
In order to investigate the impact of disease spread following interactions with hospital staff, we now place only one individual in a single-bed room (which has an en-suite bathroom). Thus, the only way the patient can become infected is through interactions with the hospital staff. Since in this case we cannot talk about distance between beds, for the probability of infection β we assume that at the end of each day there is a fixed probability (5%) of becoming infected if the individual is susceptible.
As stated in [51,52], the probability of infection is reduced by 85% when wearing masks. Here, we assume that the probability of infection is 5% per day when staff are wearing masks. Then we assume that the probability of becoming infected when staff are not wearing masks is 90%. In Figures 9(a) and (b) we show the probability of an individual (in a 1-bed room) of being at each epidemiological state when staff are wearing masks (sub-panel (a)) or not wearing masks (sub-panel (b)). It is clear that the use of masks increases the probability of the patient remaining in the susceptible state: from 0% after 10 days when the staff are not wearing masks, to 13% after 10 days when the staff are wearing masks.
Since it has been shown that a large number of the people infected with COVID-19 do not present with symptoms [6], but they can spread the virus efficiently [53] next we investigate the effects these asymptomatic individuals have on model dynamics. To this end, we split the infected individuals into "infected asymptomatic" and "infected symptomatic". Therefore, the epidemiological class Cn,1,t for each individuals can now take the following values: 0 (susceptible), 1 (exposed), 2 (infected asymptomatic), 3 (infected symptomatic) and 4 (recovered). Moreover, after the incubation period has passed, we randomly decide if the new individual is asymptomatic or symptomatic, with a maximum probability of 80% of being asymptomatic as suggested by various studies [7,54,6]. We assume that both exposed and infected asymptomatic individuals can transmit the disease, with the exposed individuals having a lower transmission probability (i.e., 20% lower, as considered also in Sub-section 3.4). We remove the infected symptomatic individuals the moment they become infected, and we remove the infected asymptomatic individuals after they test positive.
Here, we compare the effects of running RT-PCR tests every day (Figure 10) or every 3 days (Figure 11) on hospitalised patients that have not been diagnosed with COVID-19. We see that it is better to run tests more often, as the probability of being susceptible (per bed) is higher when running tests every day (see panels (b), (d)). The results are similar for the 4-bay case and 6-bay case.
When running tests every 3 days (Figure 11), if we look at the infected asymptomatic individuals (in panels (a), (c)), sometimes we see two asymptomatic individuals in a bay. This is much higher compared to the case when tests are run every day, when the level of asymptomatics is zero (Figure 10(a), (c)). Moreover, for the case where tests are performed every 3 days, we note a periodic behaviour in the average number of individuals per time – caused by the removal of asymptomatics after they test positive.
In this study we used modelling and computational approaches to investigate the question of the spread of an infectious disease through single rooms and shared hospital bays. This is an important aspect to be addressed due to the associated hospital costs of having single rooms or shared bays, and due to the very large numbers of patients that need to be hospitalised (with or without disease) in the context of a pandemic, as the one currently caused by SARS-CoV-2.
To investigate the probability that an infectious disease would spread among patients hospitalised in single rooms and in shared hospital bays with 4 beds or 6 beds, we used a simple network model that considered the probability that each bed had a susceptible, exposed, infected (symptomatic or asymptomatic) or recovered individual. We built the model in steps, starting with the four epidemiological classes considered by the majority of early models for COVID-19 (i.e., susceptibles, exposed, infected and recovered; [14,17]). Then, we introduced asymptomatic infected individuals, to investigate their role in disease transmission across hospital bays (and how the results are different from the case where we assumed that all infected individuals are symptomatic – which was the standard assumption at the beginning of this pandemics). With the help of this model we tested a variety of scenarios: the effect of placing the initial exposed individual in different beds inside the 6-bed bay (see Figure 4); the effect of varying the recovery and incubation periods (see Figures 5 and 6); the effect of removing infected individuals from the bays (see Figure 7); the effect of interaction with members of staff and sharing bathrooms, thus increasing the daily probability of infection (see Figure 8); the role of asymptomatic individuals and the effect of frequent testing (every day or every 3 days) that can be used to identify asymptomatic individuals (see Figures 10 and 11); and finally the effect of having only 1 patient per room, in which case the probability of infection depends only on interactions with staff members (see Figure 9).
We showed that patients hospitalised in single rooms have a higher probability to remain susceptible (i.e., not become infected) compared to patients in 4-bed bays; see Figures 9(a) and 8. We also showed that periodic testing combined with removing infected individuals from the bays reduces the probability of exposure and infection; see Figure 4.
We conclude our theoretical study by emphasising that healthcare associated infectious diseases seem to spread slower in single (1-bed) rooms versus shared bays. Moreover, the COVID-19 infection spreads slower in 4-bed bays compared to 6-bed bays. This predictive result is important since a 2015 report by National Institute for Health Research on the impact of single-room accommodation on patient and staff experiences [9] concluded that there was no clear evidence of a reduction in HAIs through single rooms: while many studies showed a reduction in infection rates, other studies showed no differences or higher infection rates. In our theoretical study we have shown that, from an epidemiological point of view, there are differences in the disease transmission when there is a single patient per room, or multiple patients per room. Moreover, when there are multiple room occupancies, it is important to keep the number of patients as low as possible (e.g., 4 instead of 6), to slow down the spread of infectious diseases.
Regarding periodic testing, current guidance [58] advocates for routine testing of asymptomatic HCWs every 7 days, depending on available testing capacity. Moreover, it is suggested that elective patients are to be tested for COVID-19 no more than 72 hours before admission, and cancer patients receiving long-course treatments should be tested every week [58]. The guidance from Health Protection Scotland [56] is that all patients over 70 years of age should tested for COVID-19 on admission to hospital. Moreover, the advice is that if the test results are negative, then the patients should be tested every 4 days until they are discharged [56]. Our simulation results investigated the effects of testing every 1–3 days. However, we emphasise that patient choice would need to be taken into account when developing policies for more frequent testing, as sampling has been reported to be uncomfortable. In addition, resources for testing would need to be increased in order for this to be feasible.
Finally, since frequent RT-PCR testing may not be economically wise, laboratories certified under the Clinical Laboratory Improvement Amendments [57] may use a specimen pooling strategy, which allows laboratories to test more samples with fewer testing material and thus lower costs. This pooling strategy means combining respiratory samples from different people and conducting one laboratory test on the combined pool of samples [57].
To conclude, in this study we have shown that transmission could be reduced by reducing the number of patients per room and by carrying out more regular testing. It is likely that a number of strategies will be required to reduce the risk of COVID-19 transmission and ensure the safe delivery of healthcare.
It is a pleasure to acknowledge Wai-Lum Sung (graphic designer at the University of Aberdeen) for his assistance in the preparation of Figure 1, and Kathryn Brechin the Associate Nurse Director of Ninewells Hospital who created the first draft of Figure 1.
The authors declare no conflict of interest.
[1] | Hashim S, Sarwar M, Arsalan A, et al. (2018) Frequency of carrier screening and preventive orientation among first degree relatives of Thalassaemia patients. J Pak Med Assoc 68: 50-54. |
[2] | Debnath M (2021) Prevalence of thalassemia in Indian population-A review. North Bengal Anthropologist 2021: 99-112. |
[3] | Khalid A, Butt AMK, Shahid R, et al. (2020) Thalassemia: Current Situation in Pakistan. Lahore Garrison Univ J Life Sci 4: 309-318. https://doi.org/10.54692/lgujls.2020.0404126 |
[4] |
Ali N, Moiz B, Bin Azhar W, et al. (2012) Carrier detection for beta-thalassemia trait in general Pakistani population: a way forward. Hematology 17: 237-240. https://doi.org/10.1179/1607845412Y.0000000002 ![]() |
[5] | Safdar S, Mirbahar A, Sheikh MA, et al. (2017) Economic Burden of Thalassemia on Parents of Thalassemic Children: A Multi-Centre Study. Pakistan J Med Res . |
[6] |
Black ML, Sinha S, Agarwal S, et al. (2010) A descriptive profile of β-thalassaemia mutations in India, Pakistan and Sri Lanka. J Community Genet 1: 149-157. https://doi.org/10.1007/s12687-010-0026-9 ![]() |
[7] |
Waheed U, Saba N, Wazeer A, et al. (2021) A systematic review and meta-analysis on the epidemiology of hepatitis B and hepatitis C virus among beta-thalassemia major patients in Pakistan. J Lab Physicians 13: 270-276. https://doi.org/10.1055/s-0041-1731110 ![]() |
[8] |
Riaz M, Tiller J, Ajmal M, et al. (2019) Implementation of public health genomics in Pakistan. Eur J Hum Genet 27: 1485-1492. https://doi.org/10.1038/s41431-019-0428-z ![]() |
[9] | Ejaz MS, Baloch S, Arif F (2015) Efficacy and adverse effects of oral chelating therapy (deferasirox) in multi-transfused Pakistani children with β-thalassemia major. Pakistan J Med Sci 31: 621. https://doi.org/10.12669/pjms.313.6972 |
[10] | Sharif Y, Irshad S, Muazzam A, et al. (2021) Assessment of patients with β-thalassemia major, undergoing tertiary care at a regional thalassemia center in Pakistan. Pakistan J Zool 53: 245. https://doi.org/10.17582/journal.pjz/20190630160603 |
[11] | Asif N, Hassan K (2016) Management of thalassemia in Pakistan. J Islamabad Med Dent Coll 5: 152-153. |
[12] |
Ahmed Kiani R, Anwar M, Waheed U, et al. (2016) Epidemiology of transfusion transmitted infection among patients with β-thalassaemia major in Pakistan. J Blood Transfus 2016: 8135649. https://doi.org/10.1155/2016/8135649 ![]() |
[13] | Nazir S, Faraz A, Shahzad N, et al. (2014) Prevalence of HCV in β-thalassemia major patients visiting tertiary care hospitals in Lahore–Pakistan. Adv Life Sci 1: 197-201. |
[14] |
Ehsan H, Wahab A, Shafqat MA, et al. (2020) Prevalence of transfusion transmissible infections in beta-thalassemia major patients in Pakistan: a systematic review. Blood 136: 23-25. https://doi.org/10.1182/blood-2020-138911 ![]() |
[15] |
Yasmeen H, Hasnain S (2018) Quality of life of Pakistani children with β-thalassemia major. Hemoglobin 42: 320-325. https://doi.org/10.1080/03630269.2018.1553183 ![]() |
[16] | Adil A, Sobani ZA, Jabbar A, et al. (2012) Endocrine complications in patients of beta thalassemia major in a tertiary care hospital in Pakistan. J Pak Med Assoc 62: 307-310. |
[17] | Khalil S, Khan HS, Akhtar P (2016) Status of hepatitis B and C in beta thalassemia major patients. J Islamabad Med Dent Coll 5: 71-73. |
[18] |
Ahmed S, Ayub M, Naeem M, et al. (2021) Thalassemia patients from baluchistan in Pakistan are infected with multiple hepatitis B or C virus strains. Am J Trop Med Hyg 104: 1569. https://doi.org/10.4269/ajtmh.20-0740 ![]() |
[19] |
Manzoor A, Khizar N, Abbasi NB, et al. (2022) Frequency of Hypoparathyroidism in Children Presenting with Beta-Thalassemia Major in Tertiary Care Center. Pak J Med Health Sci 16: 955-958. https://doi.org/10.53350/pjmhs22161955 ![]() |
[20] | Siddiqui SH, Ishtiaq R, Sajid F, et al. (2014) Quality of life in patients with thalassemia major in a developing country. J Coll Physicians Surg Pak 24: 477-480. |
[21] |
Hoodbhoy Z, Ehsan L, Alvi N, et al. (2020) Establishment of a thalassaemia major quality improvement collaborative in Pakistan. Arch Dis Child 105: 487-493. https://doi.org/10.1136/archdischild-2018-315743 ![]() |
[22] | Khan AM, Abbas Z, Ramzan S, et al. (2021) Social epidemiological analysis of risk factors and psychosocial burden of beta thalassemia major (BTM) in Pakistan. Elem Educ Online 20: 2682. |
[23] | Batool I, Ishfaq K, Bajwa RS (2017) Psychosocial burden among thalassemia major patients: An exploratory investigation of south Punjab, Pakistan. Pakistan Ped J 41: 158-162. |
[24] | Sultana R, Humayun S, Noor T, et al. (2016) Impact of thalassaemia on quality of Life. J Soc Obstetricians Gynaecologists Pakistan 6: 156-160. |
[25] | Ishfaq K, Diah NM, Ali J, et al. (2018) Psychosocial problems faced by thalassemia major patients of district Multan, Pakistan. Pakistan Ped J 42: 22-26. |
[26] |
Tanveer T, Masud H, Butt ZA (2018) Are people getting quality thalassemia care in twin cities of Pakistan? A comparison with international standards. Int J Qual Health Care 30: 200-207. https://doi.org/10.1093/intqhc/mzx198 ![]() |
[27] | Saleem N, Anwar A, Shahid NUA, et al. (2021) Perception of parents of thalassemic child to thalassemia in Pakistan. Cureus 13: e17615. https://doi.org/10.7759/cureus.17615 |
[28] |
Biswas B, Naskar NN, Basu K, et al. (2022) Transfusion-transmitted infections, its risk factors and impact on quality of life: An epidemiological study among β-thalassemia major children. Asian J Transfus Sci 16: 99. https://doi.org/10.4103/ajts.AJTS_74_18 ![]() |
[29] |
Zaheer HA, Waheed U, Abdella YE, et al. (2020) Thalassemia in Pakistan: A forward-looking solution to a serious health issue. Glob J Transfus Med 5: 108-110. https://doi.org/10.4103/GJTM.GJTM_72_19 ![]() |
[30] | Bukhari GMJ (2022) Quality of life among beta-thalassemic major children presenting at Federal Government Hospital Islamabad, Pakistan. J Pak Med Assoc 72: 2241-2244. https://doi.org/10.47391/JPMA.5248 |
[31] |
Qamar MK, Shaikh BT (2022) Out of Pocket Expenditure on Thalassemia Major and Its Implications on The Household Economics. J Ayub Med Coll Abbottabad 34: 91-94. https://doi.org/10.55519/JAMC-01-8332 ![]() |
[32] | Al-Moshary M, Al-Mussaed E, Khan A (2019) Prevalence of transfusion transmitted infections and the quality of life in β-thalassemia major patients. Cureus 11: e6129. https://doi.org/10.7759/cureus.6129 |
[33] | Adib-Hajbaghery M, Ahmadi M, Poormansouri S (2015) Health related quality of life, depression, anxiety and stress in patients with beta-thalassemia major. Iran J Ped Hematol Oncol 5: 193. |
[34] |
Ali SA, Azim D, Hassan H, et al. (2021) The impact of COVID-19 on transfusion-dependent thalassemia patients of Karachi, Pakistan: A single-center experience. Transfus Clin Biol 28: 60-67. https://doi.org/10.1016/j.tracli.2020.10.006 ![]() |
[35] |
Ahmed S, Jafri H, Faran M, et al. (2022) Cascade screening for beta-thalassaemia in Pakistan: relatives' experiences of a decision support intervention in routine practice. Eur J Hum Genet 30: 406-412. https://doi.org/10.1038/s41431-021-00974-y ![]() |
[36] |
Khaliq SJH (2022) Thalassemia in Pakistan. Hemoglobin 46: 12-14. https://doi.org/10.1080/03630269.2022.2059670 ![]() |
[37] |
Reed-Embleton H, Arambepola S, Dixon S, et al. (2020) A cost-of-illness analysis of β-Thalassaemia major in children in Sri Lanka–experience from a tertiary level teaching hospital. BMC Pediatr 20: 257. https://doi.org/10.1186/s12887-020-02160-3 ![]() |
[38] |
Nhac-Vu HT, Tran VTN, Nguyen TDT, et al. (2023) Economic burden of Thalassemia treatment: An analysis from the Vietnam Social Security perspective. PLoS One 18: e0293916. https://doi.org/10.1371/journal.pone.0293916 ![]() |
[39] |
Alaei K, Sarwar M, Alaei A (2018) The urgency to mitigate the spread of hepatitis C in Pakistan through blood transfusion reform. Int J Health Policy Manag 7: 207. https://doi.org/10.15171/ijhpm.2017.120 ![]() |
[40] | Ghafoor M, Sabar MF, Sabir F (2021) Prevention programmes and prenatal diagnosis for beta thalassemia in Pakistan: A narrative review. J Pak Med Assoc 71: 326-331. |
[41] |
Gambari R, Waziri AD, Goonasekera H, et al. (2024) Pharmacogenomics of Drugs Used in β-Thalassemia and Sickle-Cell Disease: From Basic Research to Clinical Applications. Int J Mol Sci 25: 4263. https://doi.org/10.3390/ijms25084263 ![]() |
[42] | Rehman MEU, Sabir B, Fazal F, et al. (2022) Impact of COVID-19 restrictions on healthcare delivery for thalassemia major patients: A perspective from Pakistan. Ann Med Surg 82: 104636. https://doi.org/10.1016/j.amsu.2022.104636 |
[43] | Hammad M, Arif R, Bano S, et al. (2023) Aftermath of the COVID-19 pandemic on mental health and well-being of patients with thalassemia major in Pakistan: a qualitative study. Cureus 15: e35048. https://doi.org/10.7759/cureus.35048 |
[44] | Nashwan AJ, Yassin MA, Babu GDJ, et al. (2018) Quality of life among adolescents aged 14 to 18 years with beta-thalassemia major (TM) in Qatar. Acta Biomed 89: 16-26. |
[45] |
ul Hassan Rashid MA, Abbasi SRS, Manzoor MM (2020) Socio-religious prognosticators of psychosocial burden of beta thalassemia major. J Relig Health 59: 2866-2881. https://doi.org/10.1007/s10943-020-01069-6 ![]() |
[46] |
Ahmed S, Saleem M, Sultana N, et al. (2000) Prenatal diagnosis of beta-thalassaemia in Pakistan: experience in a Muslim country. Prenat Diagn 20: 378-383. https://doi.org/10.1002/(SICI)1097-0223(200005)20:5<378::AID-PD815>3.0.CO;2-7 ![]() |
[47] |
Ali G, Tariq MA, Shahid K, et al. (2021) Advances in genome editing: The technology of choice for precise and efficient β-thalassemia treatment. Gene Ther 28: 6-15. https://doi.org/10.1038/s41434-020-0153-9 ![]() |
[48] |
Hardouin G, Antoniou P, Martinucci P, et al. (2023) Adenine base editor–mediated correction of the common and severe IVS1-110 (G> A) β-thalassemia mutation. Blood 141: 1169-1179. https://doi.org/10.1182/blood.2022016629 ![]() |
[49] |
Abbas Z, Rahman A, Aslam B, et al. (2024) Precision genome editing offers hope for treatment of β-thalassemia and other genetic disorders. Mol Ther Nucleic Acids 35: 102204. https://doi.org/10.1016/j.omtn.2024.102204 ![]() |
[50] |
Arif T, Farooq A, Ahmad FJ, et al. (2023) Prime editing: A potential treatment option for β-thalassemia. Cell Biol Int 47: 699-713. https://doi.org/10.1002/cbin.11972 ![]() |
[51] |
Halim-Fikri BH, Lederer CW, Baig AA, et al. (2022) Global globin network consensus paper: classification and stratified roadmaps for improved thalassaemia care and prevention in 32 countries. J Pers Med 12: 552. https://doi.org/10.3390/jpm12040552 ![]() |
1. | Konstantinos Giannakou, Maria Kyprianidou, Margarita Christofi, Anastasios Kalatzis, Georgia Fakonti, Mandatory COVID-19 Vaccination for Healthcare Professionals and Its Association With General Vaccination Knowledge: A Nationwide Cross-Sectional Survey in Cyprus, 2022, 10, 2296-2565, 10.3389/fpubh.2022.897526 | |
2. | Lulla Opatowski, Laura Temime, Contributions of modelling for the control of COVID-19 nosocomial transmission, 2022, 41, 23525568, 101054, 10.1016/j.accpm.2022.101054 | |
3. | Wenyu Chen, Ming Yao, Liang Dong, Pingyang Shao, Ye Zhang, Binjie Fu, The application framework of big data technology during the COVID-19 pandemic in China, 2022, 150, 0950-2688, 10.1017/S0950268822000577 | |
4. | Jordana Vaz Hendler, Patricia Miranda do Lago, Gabriel Cardozo Müller, João Carlos Santana, Jefferson Pedro Piva, Liane Esteves Daudt, Risk factors for severe COVID-19 infection in Brazilian children, 2021, 25, 14138670, 101650, 10.1016/j.bjid.2021.101650 | |
5. | Tom Dudding, Sadiyah Sheikh, Florence Gregson, Jennifer Haworth, Simon Haworth, Barry G. Main, Andrew J. Shrimpton, Fergus W. Hamilton, Anthony J. Ireland, Nick A. Maskell, Jonathan P. Reid, Bryan R. Bzdek, Mark Gormley, Markus Metsälä, A clinical observational analysis of aerosol emissions from dental procedures, 2022, 17, 1932-6203, e0265076, 10.1371/journal.pone.0265076 | |
6. | David Moreno-Martos, Sean Foley, Benjamin Parcell, Dumitru Trucu, Raluca Eftimie, A computational investigation of COVID-19 transmission inside hospital wards and associated costs, 2022, 19, 1551-0018, 6504, 10.3934/mbe.2022306 | |
7. | Meghann Gallouche, Caroline Landelle, Sylvie Larrat, Aurélie Truffot, Jean-Luc Bosson, Alban Caporossi, Towards real-time monitoring of COVID-19 nosocomial clusters using SARS-CoV-2 genomes in a university hospital of the French Alps, 2023, 26669919, 104650, 10.1016/j.idnow.2023.104650 | |
8. | F. McAndrew, R.G. Abeysuriya, R. Sacks-Davis, M. Sammann, D.M. Lister, D. West, S.S. Majumdar, N. Scott, Admission screening testing of patients and staff N95 respirators are cost-effective in reducing COVID-19 hospital-acquired infections, 2024, 152, 01956701, 81, 10.1016/j.jhin.2024.06.015 | |
9. | Sreejita Ghosh, Dibyajit Lahiri, Moupriya Nag, Rina Rani Ray, Debasmita Bhattacharya, 2024, Chapter 7, 978-981-99-9461-8, 145, 10.1007/978-981-99-9462-5_7 | |
10. | D.R.M. Smith, S. Chervet, T. Pinettes, G. Shirreff, S. Jijón, A. Oodally, K. Jean, L. Opatowski, S. Kernéis, L. Temime, How have mathematical models contributed to understanding the transmission and control of SARS-CoV-2 in healthcare settings? A systematic search and review, 2023, 141, 01956701, 132, 10.1016/j.jhin.2023.07.028 | |
11. | George Shirreff, Anne C.M. Thiébaut, Bich-Tram Huynh, Guillaume Chelius, Antoine Fraboulet, Didier Guillemot, Lulla Opatowski, Laura Temime, Hospital population density and risk of respiratory infection: is close contact density dependent?, 2024, 17554365, 100807, 10.1016/j.epidem.2024.100807 |
Parameters (& units) | Description | Range | Most common values | References |
σ (day−1) | Incubation rate | 0.071−0.5 | 0.143, 0.192 | [11,12,13,14,15,16,18,19,20,22,23,24,25,32,33,34,35,36,37,38] |
1/σ (day) | Mean incubation period | 2−14 | 7, 5.2 | [11,12,13,14,15,16,18,19,20,22,23,24,25,32,33,34,35,36,37,38] |
γ (day−1) | Recovery rate | 0.055−0.621 | 0.143−0.25 | [11,12,13,14,15,16,18,19,21,22,23,24,32,33,35,36,37,39,40] |
1/γ (day) | Mean recovery / infection period | 1.61−18 | 4−7 | [11,12,13,14,15,16,18,19,21,22,23,24,32,33,35,36,37,39,40] |
Parameters (& units) | Description | Range | Most common values | References |
σ (day−1) | Incubation rate | 0.071−0.5 | 0.143, 0.192 | [11,12,13,14,15,16,18,19,20,22,23,24,25,32,33,34,35,36,37,38] |
1/σ (day) | Mean incubation period | 2−14 | 7, 5.2 | [11,12,13,14,15,16,18,19,20,22,23,24,25,32,33,34,35,36,37,38] |
γ (day−1) | Recovery rate | 0.055−0.621 | 0.143−0.25 | [11,12,13,14,15,16,18,19,21,22,23,24,32,33,35,36,37,39,40] |
1/γ (day) | Mean recovery / infection period | 1.61−18 | 4−7 | [11,12,13,14,15,16,18,19,21,22,23,24,32,33,35,36,37,39,40] |