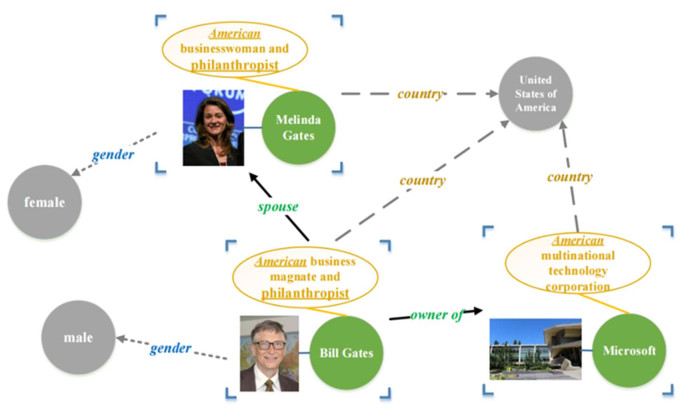
Knowledge graph embedding aims to learn representation vectors for the entities and relations. Most of the existing approaches learn the representation from the structural information in the triples, which neglects the content related to the entity and relation. Though there are some approaches proposed to exploit the related multimodal content to improve knowledge graph embedding, such as the text description and images associated with the entities, they are not effective to address the heterogeneity and cross-modal correlation constraint of different types of content and network structure. In this paper, we propose a multi-modal content fusion model (MMCF) for knowledge graph embedding. To effectively fuse the heterogenous data for knowledge graph embedding, such as text description, related images and structural information, a cross-modal correlation learning component is proposed. It first learns the intra-modal and inter-modal correlation to fuse the multimodal content of each entity, and then they are fused with the structure features by a gating network. Meanwhile, to enhance the features of relation, the features of the associated head entity and tail entity are fused to learn relation embedding. To effectively evaluate the proposed model, we compare it with other baselines in three datasets, i.e., FB-IMG, WN18RR and FB15k-237. Experiment result of link prediction demonstrates that our model outperforms the state-of-the-art in most of the metrics significantly, implying the superiority of the proposed method.
Citation: Shi Liu, Kaiyang Li, Yaoying Wang, Tianyou Zhu, Jiwei Li, Zhenyu Chen. Knowledge graph embedding by fusing multimodal content via cross-modal learning[J]. Mathematical Biosciences and Engineering, 2023, 20(8): 14180-14200. doi: 10.3934/mbe.2023634
[1] | Hanming Zhai, Xiaojun Lv, Zhiwen Hou, Xin Tong, Fanliang Bu . MLSFF: Multi-level structural features fusion for multi-modal knowledge graph completion. Mathematical Biosciences and Engineering, 2023, 20(8): 14096-14116. doi: 10.3934/mbe.2023630 |
[2] | Xiaowen Jia, Jingxia Chen, Kexin Liu, Qian Wang, Jialing He . Multimodal depression detection based on an attention graph convolution and transformer. Mathematical Biosciences and Engineering, 2025, 22(3): 652-676. doi: 10.3934/mbe.2025024 |
[3] | Xiaodong Zhu, Liehui Jiang, Zeng Chen . Cross-platform binary code similarity detection based on NMT and graph embedding. Mathematical Biosciences and Engineering, 2021, 18(4): 4528-4551. doi: 10.3934/mbe.2021230 |
[4] | Guoli Wang, Pingping Wang, Jinyu Cong, Benzheng Wei . MRChexNet: Multi-modal bridge and relational learning for thoracic disease recognition in chest X-rays. Mathematical Biosciences and Engineering, 2023, 20(12): 21292-21314. doi: 10.3934/mbe.2023942 |
[5] | Yang Liu, Tianran Tao, Xuemei Liu, Jiayun Tian, Zehong Ren, Yize Wang, Xingzhi Wang, Ying Gao . Knowledge graph completion method for hydraulic engineering coupled with spatial transformation and an attention mechanism. Mathematical Biosciences and Engineering, 2024, 21(1): 1394-1412. doi: 10.3934/mbe.2024060 |
[6] | Peng Wang, Shiyi Zou, Jiajun Liu, Wenjun Ke . Matching biomedical ontologies with GCN-based feature propagation. Mathematical Biosciences and Engineering, 2022, 19(8): 8479-8504. doi: 10.3934/mbe.2022394 |
[7] | Paula Mercurio, Di Liu . Identifying transition states of chemical kinetic systems using network embedding techniques. Mathematical Biosciences and Engineering, 2021, 18(1): 868-887. doi: 10.3934/mbe.2021046 |
[8] | Zhijing Xu, Yang Gao . Research on cross-modal emotion recognition based on multi-layer semantic fusion. Mathematical Biosciences and Engineering, 2024, 21(2): 2488-2514. doi: 10.3934/mbe.2024110 |
[9] | Keyue Yan, Tengyue Li, João Alexandre Lobo Marques, Juntao Gao, Simon James Fong . A review on multimodal machine learning in medical diagnostics. Mathematical Biosciences and Engineering, 2023, 20(5): 8708-8726. doi: 10.3934/mbe.2023382 |
[10] | Xinyu Lu, Lifang Wang, Zejun Jiang, Shizhong Liu, Jiashi Lin . MRE: A translational knowledge graph completion model based on multiple relation embedding. Mathematical Biosciences and Engineering, 2023, 20(3): 5881-5900. doi: 10.3934/mbe.2023253 |
Knowledge graph embedding aims to learn representation vectors for the entities and relations. Most of the existing approaches learn the representation from the structural information in the triples, which neglects the content related to the entity and relation. Though there are some approaches proposed to exploit the related multimodal content to improve knowledge graph embedding, such as the text description and images associated with the entities, they are not effective to address the heterogeneity and cross-modal correlation constraint of different types of content and network structure. In this paper, we propose a multi-modal content fusion model (MMCF) for knowledge graph embedding. To effectively fuse the heterogenous data for knowledge graph embedding, such as text description, related images and structural information, a cross-modal correlation learning component is proposed. It first learns the intra-modal and inter-modal correlation to fuse the multimodal content of each entity, and then they are fused with the structure features by a gating network. Meanwhile, to enhance the features of relation, the features of the associated head entity and tail entity are fused to learn relation embedding. To effectively evaluate the proposed model, we compare it with other baselines in three datasets, i.e., FB-IMG, WN18RR and FB15k-237. Experiment result of link prediction demonstrates that our model outperforms the state-of-the-art in most of the metrics significantly, implying the superiority of the proposed method.
Knowledge Graphs are a type of relational graphs that store the factual knowledge in real-world, in which the factual knowledge is in the form of triplets. Existing large-scale knowledge graphs projects include FreeBase [1], YAGO [2] and DBpedia [3], which are effective to support downstream applications such as medical question answering [4], named entity disambiguation [5] and dialogue systems [6] and so on. Therefore, it is an important problem to develop an effective method to represent and store Knowledge Graphs for different applications. In order to provide a numerical representation for knowledge graph, knowledge graph embedding (KGE) aims to translate the entities and relations to a continuous low dimensional vector space [7,8]. Then, the embedded representation can be used as the input for other applications.
Recently, KGE has attracted a great attention in natural language processing, and many KGE models have been proposed. Most of the existing KGE models mainly learn the representation for the relation, head entity and tail entity, based on the structural information of triples [9,10,11]. These models neglect the abundant content associated with the entities and relation, which affects the performance of the learned representation. Usually, many of the nodes in KG may be associated with different modalities of external data, such as text description and images, which provides details of the corresponding entities. These data are also valuable to specify the semantics of the nodes and predict the relation between entities, and hence improve the learning of KGE. For example, Figure 1 shows an example of knowledge subgraph, in which the nodes are associated with multi-modal contents. The image associated with the entity "Bill Gates" is helpful to predict that the "gender" of "Bill Gates" is "male". The text description associated with "Bill Gates" is helpful to predict that the "country" of "Bill Gates" is the "United States of America". Similar observations are the same for the entities "Microsoft" and "Melinda Gates". Therefore, effectively encoding the multimodal data into the learning of knowledge graph embedding provides new clue to improve KGE.
There are some works that attempt to improve the performance of KGE by exploiting the multimodal content. For example, Mousselly-Sergieh et al. [12] propose to align the features of structure, text and image to learn the representation of KG with a translation-based method. Veira et al. [13] replace or add the entity features with text features. Yao et al. [14] use the text associated with the KG triples to finetune the pre-trained model BERT [15] for knowledge graph completion. Although these methods have achieved a certain degree of success, they are not effective to learn the cross-modal correlation in the multimodal data. Compared with the unimodal data, different modalities of data content contained in KGs are heterogeneous and represented in different spaces. It is not appropriate to integrate the features in different spaces directly by element wise addition, multiplication or concatenation. Moreover, the correlation in multimodal data is more complex. There exist intra-modal and inter-modal correlation in them. For example, the different objects in an image are correlated with each other, and also the words in text description. As for the inter-modal correlation, some objects in an image are semantically similar with certain words in the corresponding text description. Therefore, it is nontrivial to encode the intra-modal and inter-modal correlation simultaneously to learn the features for the multimodal content. Meanwhile, the multimodal content and graph structure are also heterogenous, it is difficulty to combine the content and structural information for embedding learning. Finally, a large number of studies have shown that the interaction between entities and relations should not be ignored in KGE models [16,17]. It is desired to effectively encode the interaction between entities and relations in depth into the relation embedding learning.
To tackle these problems, we propose a multi-modal content fusion-based knowledge graph embedding model (MMCF), which encodes the multimodal content based on cross-modal correlation with the structural information to learn embedding representation. In particular, we investigate: 1) how to encode the intra-modal and inter-modal correlation of multimodal content into the embedding of knowledge graph; 2) how to fuse the structure information and data content for embedding learning. As shown in Figure 2, the model mainly contains three modules. The first module is entity embedding, which is proposed to learn the intra-modal and inter-modal correlation, in which the multimodal content is fused based on the cross-modal correlation to obtain a uniform representation. Then, the content representation is fused with the structural features to obtain entity embedding. The second module is relation embedding, which is used to fuse the interaction between entities and relation to obtain relation embedding. The third module is a decoder, which learn a scoring function to fuse the learning of entity embedding and relation embedding. Our model is different from existing fusion models that mainly learn the global features of attribute data and network structure for KGE, which is not effective to capture the latent semantics correlation between different modality of data. In addition, the proposed model can be directly extended to integrate more types of data with existing decoders. To evaluate the model, we supplement the entities with related text and images for two public datasets. Experimental results demonstrate the superiority of our approach. The main contributions are as follows:
• We propose to exploit the fine-grained semantics and correlation in the different modalities of data to improve the embedding of knowledge graph.
• We propose a novel Multi-modal content Fusion model (MMCF) for knowledge graph embedding, in which the text content and visual content are fused with the structure information to learning the embedding of entities and relation.
• Extensive experiments are conducted on three benchmark datasets, and the result demonstrates the superiority of our approach.
In the rest part of this paper, the related existing works is summarized in Section 2. Then, the problem of KGE with multimodal content is formulated in Section 3, followed by Section 4 which presents the detail of our model. The experiments and analysis are provided in Section 5. Finally, the paper is discussed in Section 6 and concluded in Section 7.
There are many knowledge graphs embedding models, which can be roughly divided into two categories: structure-based models, and models fused with external content.
The traditional structure-based models mainly learn the representation for the entities and relations from the triples, which defines a scoring or distance function on each fact to measure its plausibility. Some works propose translational distance models, including MuRE [10], TransE [18], TransR [19], etc. TransE is one of the first proposed models, which considers relation as a translation from the head entity to tail entity in the same vector space. By applying a relation-specific matrix, MuRE proposes a relation-specific distance measuring method. Some other works propose semantic matching models, which defines a similarity-based scoring function to calculate the probability that a triple is a golden triple. They mainly learn latent semantic representation for the entities and relations to measure the plausibility of each fact, such as TuckER [20], HolE [21], CrossE [22], etc. Recently, there are some works based on neural network, which proposes to apply the classic neural network models, i.e., CNN [23] and GNN [24], to learn the deep interaction information between the entities and relations. These approaches mainly include ReInceptionE [25], ConvKB [26], HypER [27], COMPGCN [28], etc. There are also some models that propose to use logical rules [29], and relation paths [30], to further learn the structure information.
These structure-based models exploit different properties of representation space to expect that the learned embedding is effective to preserve the structural information of the original knowledge graph, i.e., the representation is effective and efficient to infer the relationships between entities. Though these approaches achieve great success, they only learn the representation from the structural information of the triples, which can't be directly extended to encode the abundant and valuable content associated with the nodes of KG.
With the development of Web technology and social media, various data are produced for the entities and facts of knowledge graph. Therefore, there are some works that attempt to learn from the external data to improve the embedding of KG. One of the earliest works try to fuse the text information for knowledge base completion [31], in which only the text description is used to initialize the representation of entities. The text representations learned from text and knowledge graph structure are directly combined by a gate strategy in [9]. DKRL [25] propose to use both CBOW and CNN to learn entity representation by combining the text description, and then the objective function proposed by TransE is adopted for joint learning. Similarly, Veira et al. [13] propose to add text features to the entity features directly, which can be built on other KGE models. KG-BERT [14] uses the pre-trained language model BERT [15] to learn the representation of the text description associated with the entities and relations, and the triples are considered as textual sequences. However, these models are not effective to learn the latent correlation between different types of features since they are represented in different spaces.
There are also some works use Nonnegative Matrix Factorization (NMF) to combine different views of data or attribute with the affinity information. For example, the tensor singular value decomposition is used to learn the relation between different views in [32]. The structure information of co-expression and attribute data are fused by NMF in [33]. Li et al. [33] uses Nonnegative Matrix Factorization to fuse the structure information and attribute data, where the attribute contains one modality of content. Ma et al. [34] also uses the joint NMF and self-representation leaning to combine the structure and multi-view data, and [35] uses the joint NMF to combine different networks. These methods need the global network structure, which is not effective to handle new data because it needs a matrix built on the whole network.
Beside the text content or attribute, the visual content is also fused to learn knowledge graph embedding. Based on DKRL, IKRL is proposed to further combine image with the structure information for KGE [36] and the objective function of DKRL is also used for joint learning. Based on IKRL, Mousselly-Sergieh et al. [12] propose a multimodal translation-based approach to leverage both multimodal, i.e., visual and linguistic, and structural information for KG representation learning. These methods mainly align the structural information and other types of features instead of learn the latent correlation between them. More types of data, such as numbers, texts and images are included to learn the embedding of KG in [37], which directly concatenates different types of features into a high-dimensional vector. It is not effective to be applied to embed large-scale KGs.
Though introducing the external information has improved the performance of KGE, there are still some problems remained unsolved. First, these methods mainly regard different type of data as a whole, which neglects the fine-grained semantics and the cross-modal correlation. Second, most of the models mainly combine the text or image features with the entities, which is not effective to model the interaction between the content of entities and relations. However, it has been demonstrated that the interaction contributes greatly to the performance of KGE [38]. Finally, many of the fusion-based models are specifically designed, which can't be extended to other KGE models to include the external content.
Before the introduction of our model, we formulate the problem of KGE. A knowledge graph G is represented by a collection of golden triples denoted as (h, r, t), where h, t ∈ E denote the head and tail entity respectively, and r ∈ R denotes the relation between the head and tail entities. Beside the original structure information, each entity is also associated with other multi-modal content, i.e., the textual description e and image I.
Then, the problem of knowledge graph embedding can be formulated as: (zh,zr,zt)←f(h,r,t), where f(.) is the embedding function which combines the triple structure information and the multimodal content associated with the entities to learn the embedding, zh, zr, zt∈ Rd are the learned representations of the head entity h relation r and tail entity t respectively.
The framework of our embedding model MMCF is showed in Figure 2. As it is shown, MMCF mainly contains three modules, i.e., entity embedding, relation embedding and decoder. The entity embedding component is proposed to learning embedding of entity, in which the intra-modal and inter-modal correlation are encoded to fuse the multimodal content and structure information. The relation embedding module is used to learn the embedding of relation, which includes the features of the head entity and tail entity to supplement the relation feature. The decoder can be any of the existing decoders, such as MuRE [10] and InteractE [11], which learns a score for the input embeddings of a triple by a loss function.
As shown in Figure 2, our model MMCF is mainly comprised of three modules, i.e., the entity embedding module, relation embedding module and decoder. We detail the three modules in this section.
Most of the existing works of knowledge embedding learn the representation mainly based on the triple information [10,18]. Though some works try to include the other types of content for entity embedding, they are not effective to capture the latent correlation between different types of content [25,29]. In this module, we first learn the multimodal content features by exploiting the intra-modal and inter-modal correlation, and then they are fused with the structure features by a gating network to obtain the final representation of entity.
Given an image I, we use Faster R-CNN [39] initialized with ResNet-101 to extract the visual object proposals, which is represented by triple set (oi, li, ai), where oi is the feature vector extracted from the region of interest (ROI) pooling layer in the Region Proposal Network, li is a 4-dimensional representation of the bounding box location, and ai is a one hot representation of the attribute class. These vectors are then combined to formulate the representation of the visual object as follows:
o′i=concat(oi,Wlli,Waai) | (1) |
where concat(.) is the concatenation operation, Wl and Wa are the parameter matrices. Then, a fully connected layer is added to transform the vector o′i to match the textual features, i.e., v={v1,v2,...,vk), where vi denotes the transformation of o′i.
The pre-trained language representation model BERT [15] is used to obtain word vectors for the text description. Given a text description document e, we extract the word vectors as e = {w1, w2, …, wm}, where m denotes the number of words in the document. Then, the image object features and textual word embeddings are further processed to learn the intra-modal and inter-modal correlation, as shown in Figure 2.
Usually, the objects in an image and words in a document are correlated with each other. By exploiting the intra-modal correlation, the important information in each modality can be enhanced for representation learning. As for the visual content, we use the self-attention mechanism [40] to learn the intra-modal correlation. Specifically, given a set of objects v={v1,v2,...,vk}, the query, key and value are calculated: Qv=vWQ, Kv=vWK, Vv=vWV, where WQ, WK, and WV are the matrices of parameters. Then, the weighted sum of the value is calculated as follows:
Self−Attent(Qv,Kv,Vv)=softmax(QvKTv√dk)Vv | (2) |
where dk denotes the dimensionality of the visual object vector. We apply the multi-headed attention mechanism to calculate the self -attention h times, and then the values of all heads are concatenated. Then, the Add Norm layers are appended to smooth the result as follows:
O(l)v=Norm(H(l−1)v+M(l)v) | (3) |
H(l)v=max(0,O(l)vW(l)v+b(l)v)W(l)′v+b(l)′v | (4) |
where H(l−1)v is the input before self-attention process, and M(l)v denotes the output of self-attention process. The self-attention and Add Norm process literately to obtain the visual object vectors, and then the vectors are aggregated to obtain the image representation v0∈Rd by an average pooling operation.
As for the textual content, the convolution neural networks [41] is used to learn the intra-modal correlation. Specifically, given the textual vectors input e = {w1, w2, …, wm}, the 1-dim CNN [41] is used to encode the context information. We use three window sizes, i.e., uni-gram, bi-gram and tri-gram, to learn the representation of the i-th word as follows:
ws,i=ReLU(Wswi:i+s−1+bs),s=1,2,3 | (5) |
where ws,i is the output of the i-th word using window size s, Ws is the parameter of filter matrix and bs is the bias parameter. Then, all the word vectors corresponding to the window size s is aggregated using a max-pooling operation to obtain the text representation: ps=max(ws,1,ws,2,...,+ws,m). Finally, p1, p2 and p3 are concatenated to a fully connected layer with a l2 normalization to obtain the final text description embedding e0:
e0=Norm(Weconcat(p1,p2,p3)+be | (6) |
where e0∈Rd is the learned text representation which encodes the intra-modal correlation.
Beside the intra-modal correlation, each object in an image may also correlated with some words in the text description. The inter-correlation is important to supplement the learning of the representation for each other modalities. We use the cross-attention to capture the cross-modal correlation for representation learning. As shown in Figure 2, the input of the cross-attention are the stacked features of image objects v={v1,v2,...,vk} and textual words e = {w1, w2, …, wm}. First, we obtain the query, key and value for the two modalities: Kv=vWK, Qv=vWQ, Vv=vWV, Ke=eWK, Qe=eWQ, Ve=eWV. Then, the visual object and textual word representation encoding the cross-modal correlation are calculated as follows:
v′=softmax(QvKTv)Ve | (7) |
e′=softmax(QeKTe)Vv | (8) |
where v′ denotes the set of visual object representation which captures the inter-modal cross-modal correlation, and e′ denotes the set of textual word representation. By these operations, we can obtain another representation for each textual word and visual object. To obtain the final representation v1∈Rd for the whole image, the learned v′ is passed into an average pool layer. The learned e′ is passed into an 1d-CNN layer followed by a max pool layer to obtain the final representation for the whole text description e1∈Rd.
As discussed above, we have learned multiple features from the multimodal content. Meanwhile, there is also another type of feature which encode the structure information of knowledge graph, which is also the main feature learned in other works [10,18,19]. To capture the structure information, we use the structure-based methods, such as TransE [18], to learn the raw structure feature s0∈Rx of each entity node. In the end, we obtain two types of visual feature v0 and v1, two types of textual feature e0 and e1, and the structural feature s0.
Finally, all these features are fused to obtain a final representation of the entity node. The feature fusion operation is composed of several steps. First, the two types of textual features are fused using multi-modal factorized bilinear pooling (MFB) [42] as follows:
e2 = MFB(e0,e1)=k∑i=1(UTie0∘GTie1) | (9) |
where ◦ denotes the element wise multiplication operation, k denotes the number of factors in MFB. Similarly, the two types of visual features are also fused with MFB to obtain the final representation v2. Then, we fuse the visual feature v2, the textual feature e2 and the structural feature s0 to obtain the final representation of the entity using gating network with softmax function as follows:
(α,β,γ) = softmax(GTev2√d,GTee2√d,GTes0√d) | (10) |
ze = αv2+βe2+γs0 | (11) |
where ze is the final representation of the whole entity, which can be a head entity zh or a tail entity zt. As a result, the learned representation of an entity captures both the structure information and multimodal content, which encodes the content by exploiting the intra-modal and inter-modal correlation. When α and β are trained to be 0, the representation only contains the structural features, which is similar to the existing models [10,18,19]. Therefore, our approach is more effective to learn the representation.
Usually, the relation in knowledge graph exists between a head entity and tail entity, which rarely contains other content information. To improve the representation of relation, we use the multimodal features learned from the head entity and tail entity to enhance the semantics information of relation. Meanwhile, this process can also capture the interaction between entities and the corresponding relation, and thus further improves the representation learning of relation. The Bilinear Network is used to fuse the entity feature and relation structure feature as follows:
zr = σ(WThzh)∘σ(WTtzt)∘σ(WTrrs) + br | (12) |
where σ is a nonlinear activation function, rs is the relation representation learned by other structure-based method [18], zh and zt are the fused representation of the head entity and tail entity respectively. This formulation is also used to learn the structural information of a triple.
Algorithm 1 the training of MMCF |
Input: triples (h, r, t) of a graph G; Output: zh, zr, zt 1: For each entity in G 2: Feature Extraction 2: Extract the visual representation o′i of each object by Eq (1); 6: Extract the word representation wi; 3: Transform o′i to vi by fully-connect layer; 4: Intra-modal Correlation Learning 4: Learn the intra-modal correlation between vi by Eq (2); 5: All the vi s are aggregated to obtain the image representation v0∈Rd; 6: Extract the word representation wi; 7: Use 1-dim CNN to encode the intra-modal correlation of wi by Eq (5); 8: Obtain text description embedding e0∈Rd by Eq (6). 9: Inter-modal Correlation Learning 9: Learn visual representation v1∈Rd based on inter-modal correlation by Eq (7); 10: Learn textual representation e1∈Rd based on inter-modal correlation by Eq (8); 12: Cross-modal Feature Fusion 11: Obtain text representation e2 by fusing e0 and e1 using Eq (9); 12: Obtain visual representation v2 by fusing v0 and v1 using Eq (9); 13: Combine v2, e2, s0 to obtain entity representation zh or zt by Eq (11); 14: end for 15: For each relation in G 16: Learn relation representation zr by Eq (12) 17: end for 18: Minimize ϕ(h,r,t) by Eq (13). |
In the decoder module, a scoring function is applied to learn the representation. Many of current score functions proposed by other works [10,18,19] can be used since our method can be directly extended to these models. For example, we use the MuRE [10] scoring function to calculate a score for a triple as follows:
ϕ(h,r,t) = −d(Rzh,zt+rf)2+bh+bt | (13) |
where d(.) is a Euclidean distance function, R is a relation-specific matrix, bh and bt are the biases of the head and tail entities. The scoring function aim to give a high value to the positive triple, and a small value to the negative triple. With this function, we can train our model on the training dataset to learn the representation of entity and relation. Meanwhile, the other types of decoders proposed by other models can also be used to learn the scoring function, such as InteractE [11] and TransE [12]. The training process of MMCF is shown in Algorithm 1.
To evaluate the performance of our approach, extensive experiments are conducted to compare our approach with other approaches. Meanwhile, the effectiveness of each component in our model is also verified.
Three public datasets are used in the experiments, i.e., FB-IMG [12], WN18RR [43] and FB15k-237 [44]. The dataset FB-IMG has already included high-quality multimodal content to knowledge graph. It contains the embedding representation for entities and relations, and representation of the textual description and images associated with the entities. WN18RR is built on the base of WN18[18]. It removes the inverse relations, which makes the test triples can't be inferred from the inverse of training examples directly. FB15k-237 is a revision of FB15k [18], in which all the inverse relations are also removed. Though the two datasets are widely used in KGE evaluation, they contain only the structure information. To associate the datasets with external multimodal content, Yao et al. [14] download the text description for the triples in WN18RR and FB15k-237. Based on the work [14], we further extend the two datasets with text description and images. The names of entities are used as keywords to crawl the related images from the web search engines, such as Google and Bing. Then, the top-15 images and the text content, such as title, caption, abstract of each entity are downloaded. We manually select the image and the text content which is most related to the corresponding entity to supplement the multimodal content of the two datasets. Since the relation denotes the structure between entities, it is difficulty to be directly described by other multimodal content. Therefore, we use the data of the associated entities to enhance the learning of relation embedding as discussed above. We show the statistics information of these datasets in Table 1.
Dataset | #entities | #relation | #train | #valid | #test |
FB-IMG | 11,757 | 1,231 | 285,850 | 29,580 | 34,863 |
WN18RR | 40,943 | 11 | 86,835 | 3,034 | 3,134 |
FB15k-237 | 14,541 | 237 | 272,115 | 17,535 | 20,466 |
We use the pre-trained language model BERT [13] to obtain a 300-dimensional vector for the text word. The whole text content is finally represented by a 1024-dimensional vector. As for the image, the top-15 objects extracted by the pretrained Fast R-CNN [39] with the highest accuracy are selected and each one is represented by a 2048-dimensional vector. The Adam et al. [45] optimizer is used in the experiments, whose initial learning rate is 1 × 10−4, and then decreases at a rate of 0.9 every 20 epochs. By adjusting the parameters, the model with the highest F1 value in the verification set is finally selected. All of the experiments are conducted on 2 NVIDIA RTX 3090 24 GB.
Usually, the task of link prediction is used to evaluate the quality of the representation learned by the embedding method. It is used to predict the missed facts of knowledge graph, which is also an effective way to solve the problem of incompleteness of KGs. The task of link prediction in KG is formulated as inferring the missed head entity given (−, r, t), or the missed tail entity given (h, r, −). The trained model calculates the plausibility scores of all possible triples in the test set, and then the ranking result of these triples is used for evaluation. In the experiment, we use the popular matrices of Mean Reciprocal Rank (MRR) and H@N to evaluate the ranking result. MRR is the mean reciprocals of all the ranking result of the test samples. HITS@N denotes the hits occur at the N-th position, which denotes the average proportion of positive triples that rank less than N in the ranking list, N = 1/3/10.
To evaluate the performance of our approach, we compare it with two categories of models, i.e., the structure-based models and external information-fused models.
The structure-based models mainly learn the embedding of entity and relation based on the graph structure information, such as the triplet set. In the experiment, MuRE and MuRP [10], InteractE [11], ConvKB [26], HypER [27], DistMult [46] and M2GNN [47] are used as the baselines, and the link prediction result on WN18RR and FB15k-237 published by these models are directly used for comparison. As for the dataset FB-IMG, we also reproduce several baseline modes for comparison, such as MuRE [10], InteractE [11], ComplEx [35], ConvE [48]. There are also some models exploiting external information for knowledge graph embedding, such as the entity-related text descriptions or images. On the datasets WN18RR and FB15k-237, we adopt the baseline model KG-BERT [14] for comparison, which exploits the external text content for KGE and achieves the state-of-the-art performance. On the dataset FB-IMG, there are some other works exploiting the external multimodal content for KGE, such as TransE [12] and MKRL [36]. Accordingly, we compare with these baseline models on FB-IMG.
In the first experiment, we compare the performance of link prediction of our model MMCF with the baseline models on the datasets WN18RR and FB15k-237 which introduces the textual and visual content. We implement MMCF with two decoders MuRE [8] and InteractE [7]. The comparison result is shown in Table 2. From the table, several conclusions can be derived.
Models | WN18RR | FB15k-237 | ||||||
MRR | HITS@1 | HITS@3 | HITS@10 | MRR | HITS@1 | HITS@3 | HITS@10 | |
MuRE | 0.475 | 0.436 | 0.487 | 0.554 | 0.336 | 0.245 | 0.370 | 0.521 |
MuRP | 0.481 | 0.440 | 0.495 | 0.566 | 0.335 | 0.243 | 0.367 | 0.518 |
InteractE | 0.463 | 0.430 | − | 0.528 | 0.354 | 0.263 | − | 0.535 |
ConvKB | 0.249 | 0.057 | 0.417 | 0.524 | 0.243 | 0.155 | 0.371 | 0.421 |
HypER | 0.465 | 0.436 | 0.477 | 0.522 | 0.341 | 0.252 | 0.376 | 0.520 |
DistMult | 0.430 | 0.390 | 0.440 | 0.490 | 0.241 | 0.155 | 0.263 | 0.419 |
M2GNN | 0.485 | 0.444 | 0.498 | 0.572 | 0.362 | 0.275 | 0.398 | 0.565 |
ComplEx | 0.440 | 0.410 | 0.460 | 0.510 | 0.247 | 0.158 | 0.275 | 0.428 |
ConvE | 0.430 | 0.400 | 0.440 | 0.520 | 0.325 | 0.237 | 0.356 | 0.501 |
KG-BERT | − | − | − | 0.524 | − | − | − | 0.420 |
MMCFMuRE | 0.489 | 0.443 | 0.497 | 0.571 | 0.359 | 0.269 | 0.397 | 0.554 |
MMCFInteractE | 0.483 | 0.448 | 0.495 | 0.570 | 0.367 | 0.273 | 0.402 | 0.563 |
First, our model MMCF achieves the best performance of multiple metrices on the two datasets. The result demonstrates that learning the intra-modal and inter-modal correlation to fuse the multimodal content with the structure information is effective to improve the performance of knowledge graph embedding. Second, whichever of the two decoders MuRE [8] and InteractE [7] is applied, MMCF outperforms other models in most of the matrices. Therefore, it also demonstrates that including the multimodal content with cross-modal correlation can improve the performance of the existing KGE models. Meanwhile, MMCFMuRE performs better than MMCFInteractE on WN18RR, which is the same as that MuRE performs better than InteractE. The same observation can also be found in FB15k-237. Therefore, an effective decoder also contributes to the performance of MMCF. Third, compared with other models that include the related content for knowledge graph embedding, such as KG-BERT, our model still improves the performance. It demonstrates that exploiting the intra-modal and inter-modal correlation to fuse multimodal content is more effective than directly fusing the multimodal for KGE.
In the other experiment, we also compare MMCF with the baselines on the dataset FB-IMG. The comparison result is shown in Table 3, where MMCFTreansE denotes that MMCF adopts TransE [12] as the decoder. From the table, it is observed that our modal outperforms than these baselines. The result further demonstrates that the multimodal content of entities supplements the structural information for KGE, and exploiting the intra-modal and inter-modal correlation is also effective to learn the representation for multimodal content.
Models | MRR | HITS@1 | HITS@3 | HITS@10 |
TransE | − | − | − | 0.494 |
MKRL | − | − | − | 0.645 |
MuRE | 0.765 | 0.703 | 0.807 | 0.874 |
InteractE | 0.813 | 0.762 | 0.849 | 0.895 |
ConvKB | 0.449 | 0.337 | 0.513 | 0.621 |
ComplEx | 0.525 | 0.392 | 0.618 | 0.754 |
ConvE | 0.747 | 0.667 | 0.804 | 0.882 |
MMCFTransE | 0.453 | 0.342 | 0.525 | 0.702 |
MMCFMuRE | 0.820 | 0.768 | 0.852 | 0.899 |
MMCFInteractE | 0.819 | 0.771 | 0.849 | 0.897 |
To evaluate the effectiveness of each component in MMCF, we design a set of ablation experiments on multi-modal dataset FB-IMG. Meanwhile, a set of various versions of MMCF are designed as follows:
MMCF-intra. It removes the intra-modal correlation learning components for both the two modalities, and only the inter-modal correlation is encoded to learn the multimodal representation.
MMCF-inter. It removes the inter-modal correlation learning components, and only the intra-modal correlation is encoded to learn the multimodal representation.
MMCF-gating. It removes the gating network, and then the feature output by the cross-modal correlation learning module and the structure feature are added element-wise.
MMCF-text. It removes the text description from the multimodal content, and then only the image is used as the external content of the entities.
MMCF-image. It removes the image from the multimodal content, and then only the textual description is used as the external content of the entities.
MMCF-entity. The features of entities are not fused into the relation feature. It mainly tests whether the entity features are useful for relation representation learning.
Then, the ablation experiment is conducted on WN18RR and FB15k-237 with the decoder MuRE. Table 4 shows the experiment result. From the table, we can obtain several conclusions. First, MMCF with all components to exploit the multi-modal content obtains the best performance. Second, all the components contribute to the performance of MMCF, and the related multimodal content is useful to knowledge graph embedding. MMCF without image obtains the second-best performance, while the performance of MMCF without the text description decrease greatly. This is might because that the text content is more effective to reflect the semantics of entity than image. Third, including the entity features to the relation is effective to improve the learning of relation representation, since the performance of MMCF-entity also decreases greatly. The entity features can enrich the semantics of relation.
Models | WN18RR | FB15k-237 | ||||||
MRR | HITS@1 | HITS@3 | HITS@10 | MRR | HITS@1 | HITS@3 | HITS@10 | |
MMCF-intra | 0.471 | 0.426 | 0.479 | 0.554 | 0.342 | 0.256 | 0.384 | 0.544 |
MMCF-inter | 0.475 | 0.429 | 0.482 | 0.556 | 0.348 | 0.258 | 0.389 | 0.546 |
MMCF-gating | 0.480 | 0.435 | 0.491 | 0.566 | 0.353 | 0.265 | 0.396 | 0.552 |
MMCF-text | 0.458 | 0.421 | 0.471 | 0.549 | 0.335 | 0.252 | 0.380 | 0.538 |
MMCF-image | 0.482 | 0.441 | 0.494 | 0.562 | 0.356 | 0.267 | 0.394 | 0.549 |
MMCF-entity | 0.467 | 0.438 | 0.485 | 0.559 | 0.339 | 0.254 | 0.387 | 0.542 |
MMCF | 0.489 | 0.443 | 0.497 | 0.571 | 0.359 | 0.269 | 0.397 | 0.554 |
In this section, we analyze the impact of embedding size and also give a case study. We recode the experiment result by setting the embedding of MMCF with different dimension size {20, 40, 60, 80,100,120,140,160} on WN18RR. From the table, it can be found that the performance is improved greatly with the embedding size increased in the early stage. Then the performance is maintained for a period, and decreases very slowly. Therefore, it demonstrates that a large vector is not always perform better than a small vector of the embedding. Moreover, our model is not very sensitive to the size of the embedding size when the size reaches a certain number.
To visualize the performance in detail, we present an example of ranking list for a query (peach, _hypernym, −). Table 6 shows the result of MMCF and MuRE, where MMCF use MuRE as the decoder. From the table, it can be observed that the correct answer is located in the first position of the ranking list returned by MMCF. MuRE ranks the correct answer in the third position. The other method TransE which also fuse the external multimodal content to embed knowledge graph ranks the correct item in the second position. Therefore, our method obtains the best result in this example. This is might because that stone fruit contain some text and visual content that describe the nature of peach. MMCF can learn the latent correlation between the multimodal content of different entities, which is then more effective to infer the related entity. From the experiment result, it is further demonstrated that the external multi-modal content is useful for knowledge graph embedding.
Embedding Size | MRR | HITS@1 | HITS@3 | HITS@10 |
20 | 0.368 | 0.395 | 0.421 | 0.523 |
40 | 0.476 | 0.428 | 0.468 | 0.551 |
60 | 0.483 | 0.435 | 0.490 | 0.562 |
80 | 0.487 | 0.440 | 0.495 | 0.567 |
100 | 0.489 | 0.443 | 0.497 | 0.571 |
120 | 0.488 | 0.441 | 0.495 | 0.572 |
140 | 0.487 | 0.438 | 0.493 | 0.570 |
160 | 0.485 | 0.435 | 0.490 | 0.565 |
Top-N | MMCF | MuRE | TransE |
1 | stone fruit | structure | fruit tree |
2 | fruit | fruit tree | stone fruit |
3 | citrus fruit | stone fruit | veggie |
4 | fruit tree | seasoning | tree |
5 | structure | computer memory unit | nut |
6 | monocot genus | veggie | citrus fruit |
7 | veggie | citrus fruit | monocot genus |
8 | tree | monocot genus | structure |
9 | produce | tree | root |
10 | root | root | animal |
Our model is built on the exiting KGE model to exploit the external multimodal knowledge to improve the embedding of knowledge graph. Since it learns the inter-modal and intra-modal correlation of different modalities of content, it needs more running time than the traditional KGE algorithm, such as TransE, MuRE and InteractE. The parameter size of our model is about 4.2M. The training of our model is conducted on 2 NVIDIA RTX 3090 24GB, which takes about 12 hours for one dataset. However, the algorithm can be optimized by distributed, parallel and cluster computing.
This study is designed to deeply exploit the external multimodal content for knowledge graph embedding. In reality, there is usually a great volume of different types of data related to entities and relation, such as text description, web pages, medical images, web images, audio and videos and so on. Many of the data can be easily obtained from different sources, such as Web sites, traditional databases and medical datasets, etc. Therefore, it is reasonable to exploit the multimodal data to improve the performance of traditional knowledge graph embedding methods. Accordingly, there are already some works [14,37] to fuse the external data for knowledge graph embedding, which has achieved certain success. However, these methods mainly regard the different types of data as a whole or directly fuse the features of external data with the features learned from knowledge graph. Therefore, these methods are not effective to learn from the multimodal data since different types of data are heterogeneous and there exists cross-modal correlation.
Our method first learns the representation of different modalities of data by exploiting the intra-modal correlation, and then the features of different modalities are fused by encoding the inter-modal correlation. Finally, the features learned from the multimodal content and graph structural information are fused by a gating network. Therefore, our method gives consideration to the characteristic of multimodal data, and thus it is more effective to fuse the multimodal content for knowledge graph embedding. The experiment result also demonstrates the superiority of our method, by comparing with the structure-based methods and multimodal content fusion-based methods. By using the same decoder, our model performs better than the original models MURE, TransE and InteractE. Though we mainly fuse the text description and image for knowledge graph embedding in this paper, the other types of data, such as video and audio, can also be fused by extending our method directly. Moreover, the framework of our method can also be used or revised in other domains which needs to handle multimodal data, such as network embedding, multimodal knowledge graph construction, visual question answering, multimodal data classification and so on. The limitation of our method is that it might be more complex than the structure-based methods and other multimodal content fusion-based methods, since it further learns the fine-granularity cross-modal correlation between different types of data. However, this problem can be alleviated by parallel computing.
In this paper, we propose to learn knowledge graph embedding by exploiting the cross-modal correlation between the multimodal content related to the entities. Specifically, a novel model is proposed to exploit the intra-modal and inter-modal correlation for multimodal representation learning, which then fused with the structure features for entity and relation representation learning. It is different from existing works which learn entity embedding mainly base on the structure information or include the external data as a whole. We evaluate the performance on three datasets, and the result demonstrate the superiority of the proposed model. Meanwhile, our model can be easily combined with other structure-based models, such as MuRE, TransE and InteractE.
In the future works, it is interesting to exploit the multi-modal pre-training models to more effectively learning the context semantics of entity. Moreover, this model can also be combined with other embedding models, such as network embedding.
The authors declare they have not used Artificial Intelligence (AI) tools in the creation of this article.
This work is supported by State Grid Corporation of China Big Data Center Technology Project (SGSJ0000YFJS2200066).
All authors declare no conflicts of interest in this paper.
[1] | K. Bollacker, C. Evans, P. Paritosh, T. Sturge, J. Taylor, Freebase: a collaboratively created graph database for structuring human knowledge, in 2008 ACM SIGMOD International Conference on Management of Data (SIGKDD), (2008), 1247–1250. https://doi.org/10.1145/1376616.1376746 |
[2] | F. M. Suchanek, G. Kasneci, G. Weikum, Yago: a core of semantic knowledge, in 2007 16th International Conference on World Wide Web (WWW), (2007), 697–706. https://doi.org/10.1145/1242572.1242667 |
[3] |
J. Lehmann, R. Isele, M. Jakob, A. Jentzsch, D. Kontokostas, P. N. Mendes, et al., Dbpedia–a large-scale, multilingual knowledge base extracted from Wikipedia, Semantic Web, 6 (2015), 167–195. https://doi.org/10.3233/SW-140134 doi: 10.3233/SW-140134
![]() |
[4] |
M. Wang, X. He, Z. Zhang, L. Liu, L. Qing, Y. Liu, Dual-process system based on mixed semantic fusion for Chinese medical knowledge-based question answering, Math. Biosci. Eng., 20 (2023), 4912–4939. https://doi.org/10.3934/mbe.2023228 doi: 10.3934/mbe.2023228
![]() |
[5] | Z. Zheng, X. Si, F. Li, E. Y. Chang, X. Zhu, Entity disambiguation with freebase, in 2012 IEEE/WIC/ACM International Joint Conferences on Web Intelligence and Intelligent Agent Technology, (2012), 82–89. https://doi.org/10.1109/WI-IAT.2012.26 |
[6] | S. Moon, P. Shah, A. Kumar, R. Subba, Opendialkg: Explainable conversational reasoning with attention-based walks over knowledge graphs, in 2019 the 57th Annual Meeting of the Association for Computational Linguistics (ACL), (2019), 845–854. https://doi.org/10.18653/v1/P19-1081 |
[7] |
X. Lu, L. Wang, Z. Jiang, S. Liu, J. Lin, MRE: A translational knowledge graph completion model based on multiple relation embedding, Math. Biosci. Eng., 20 (2023), 5881–5900. https://doi.org/10.3934/mbe.2023253 doi: 10.3934/mbe.2023253
![]() |
[8] |
Q. Wang, Z. Mao, B. Wang, L. Guo, Knowledge graph embedding: A survey of approaches and applications, IEEE Trans. Knowl. Data Eng., 29 (2017), 2724–2743. https://doi.org/10.1109/TKDE.2017.2754499 doi: 10.1109/TKDE.2017.2754499
![]() |
[9] | J. Xu, X. Qiu, K. Chen, X. Huang, Knowledge graph representation with jointly structural and textual encoding, in 2017 the 26th International Joint Conference on Artificial Intelligence (IJCAI), (2017), 1318–1324. https://doi.org/10.48550/arXiv.1611.08661 |
[10] |
I. Balaˇzevi´c, C. Allen, T. Hospedales, Multi-relational poincar'e graph embeddings, Adv. Neural Inf. Proces. Syst., 32 (2019), 1168–1179. https://doi.org/10.48550/arXiv.1905.09791 doi: 10.48550/arXiv.1905.09791
![]() |
[11] | S. Vashishth, S. Sanyal, V. Nitin, N. Agrawal, P. Talukdar, Interacte: Improving convolution-based knowledge graph embeddings by increasing feature interactions, in 2020 the 34th AAAI Conference on Artificial Intelligence (AAAI), (2020), 3009–3016. https://doi.org/10.1609/aaai.v34i03.5694 |
[12] | H. Mousselly-Sergieh, T. Botschen, I. Gurevych, S. Roth, A multimodal translation-based approach for knowledge graph representation learning, in 2018 the Seventh Joint Conference on Lexical and Computational Semantics, (2018), 225–234. https://doi.org/10.18653/v1/S18-2027 |
[13] | N. Veira, B. Keng, K. Padmanabhan, A. G. Veneris, Unsupervised embedding enhancements of knowledge graphs using textual associations, in 2019 the 28th International Joint Conference on Artificial Intelligence (IJCAI), (2019), 5218–5225. https://doi.org/10.24963/ijcai.2019/725 |
[14] | L. Yao, C. Mao, Y. Luo, Kg-bert: Bert for knowledge graph completion, preprint, arXiv: 1909.03193. |
[15] | J. Devlin, M. W. Chang, K. Lee, K. Toutanova, Bert: Pre-training of deep bidirectional transformers for language understanding, in 2019 the Conference of the North American Chapter of the Association for Computational Linguistics: Human Language Technologies (NAACL), (2019), 4171–4186. https://doi.org/10.48550/arXiv.1810.04805 |
[16] | M. Schlichtkrull, T. N. Kipf, P. Bloem, R. Van Den Berg, I. Titov, M. Welling, Modeling relational data with graph convolutional networks, in 2018 European Semantic Web Conference, (2018), 593–607. https://doi.org/10.48550/arXiv.1703.06103 |
[17] | S. Vashishth, S. Sanyal, V. Nitin, P. Talukdar, Composition-based multi-relational graph convolutional networks, in 2020 the International Conference on Learning Representations (ICLR), (2020), 121–134. https://doi.org/10.48550/arXiv.1911.03082 |
[18] |
A. Bordes, N. Usunier, A. Garcia-Duran, J. Weston, O. Yakhnenko, Translating embeddings for modeling multi-relational data, Adv. Neural Inf. Process. Syst., 22 (2013), 2787–2795. https://doi.org/10.5555/2999792.2999923 doi: 10.5555/2999792.2999923
![]() |
[19] | Y. Lin, Z. Liu, M. Sun, Y. Liu, X. Zhu, Learning entity and relation embeddings for knowledge graph completion, in 2015 AAAI Conference on Artificial Intelligence (AAAI), (2015), 2181–2187. https://doi.org/10.1609/aaai.v29i1.9491 |
[20] | I. Balazevic, C. Allen, T. Hospedales, Tucker: Tensor factorization for knowledge graph completion. In 2019 the Conference on Empirical Methods in Natural Language Processing and the 9th International Joint Conference on Natural Language Processing (EMNLP-IJCNLP), (2019), 178–189. https://doi.org/10.18653/v1/D19-1522 |
[21] | M. Nickel, L. Rosasco, T. Poggio, Holographic embeddings of knowledge graphs, in 2016 the 30th AAAI Conference on Artificial Intelligence (AAAI), (2016), 1955–1961. https://doi.org/10.1609/aaai.v30i1.10314 |
[22] | W. Zhang, B. Paudel, W. Zhang, A. Bernstein, H. Chen, Interaction embeddings for prediction and explanation in knowledge graphs, in 2019 the 12th ACM International Conference on Web Search and Data Mining (WSDM), (2019), 96–104. https://doi.org/10.1145/3289600.3291014 |
[23] | Y. LeCun, L. Bottou, Y. Bengio, P. Haffffner, Gradient-based learning applied to document recognition, in Proceedings of the IEEE, (1998), 2278–2324. https://doi.org/10.1109/5.726791 |
[24] |
Z. Wu, S. Pan, F. Chen, G. Long, C. Zhang, S. Y. Philip, A comprehensive survey on graph neural networks, IEEE Trans. Neural Networks Learn. Syst., 6 (2021), 97–109. https://doi.org/10.1109/TNNLS.2020.2978386 doi: 10.1109/TNNLS.2020.2978386
![]() |
[25] | Z. Xie, G. Zhou, J. Liu, X. Huang, Reinceptione: Relation-aware inception network with joint local-global structural information for knowledge graph embedding, in 2020 the 58th Annual Meeting of the Association for Computational Linguistics (ACL), (2020), 5929–5939. https://doi.org/10.18653/v1/2020.acl-main.526 |
[26] | D. Q. Nguyen, T. D. Nguyen, D. Q. Nguyen, D. Phung, A novel embedding model for knowledge base completion based on convolutional neural network, in 2018 the 16th Annual Conference of the North American Chapter of the Association for Computational Linguistics: Human Language Technologies (NAACL-HLT), (2018), 327–333. https://doi.org/10.18653/v1/N18-2053 |
[27] | I. Balaevicx, C. Allen, T. M. Hospedales, Hypernetwork knowledge graph embeddings, in 2019 the 28th International Conference on Artificial Neural Networks, (2019), 553–565. https://doi.org/10.1007/978-3-030-30493-5_52 |
[28] | S. Vashishth, S. Sanyal, V. Nitin, P. Talukdar, Composition-based multi-relational graph convolutional networks, in 2020 the International Conference on Learning Representations (ICLR), (2020), 321–334. https://doi.org/10.48550/arXiv.1911.03082 |
[29] | W. Y. Wang, W. W. Cohen, Learning first-order logic embeddings via matrix factorization, in 2016 the 25th International Joint Conference on Artificial Intelligence (IJCAI), (2016), 2132–2138. https://doi.org/10.5555/3060832.3060919 |
[30] |
B. Jagvaral, W. K. Lee, J. S. Roh, M. S. Kim, Y. T. Park, Path-based reasoning approach for knowledge graph completion using cnn-bilstm with attention mechanism, Expert Syst. Appl., 142 (2020), 112960. https://doi.org/10.1016/j.eswa.2019.112960 doi: 10.1016/j.eswa.2019.112960
![]() |
[31] |
R. Socher, D. Chen, C. D. Manning, A. Ng, Reasoning with neural tensor networks for knowledge base completion, Adv. Neural Inf. Process. Syst., 2013 (2013), 926–934. https://doi.org/10.5555/2999611.2999715 doi: 10.5555/2999611.2999715
![]() |
[32] |
X. Gao, Y. Wang, W. Hou, Z. Liu, X. Ma, Multi-view Clustering for integration of gene expression and methylation data with tensor decomposition and self-representation learning, IEEE/ACM Trans. Comput. Biol. Bioinf., 2022 (2022). https://doi.org/10.1109/TCBB.2022.3229678 doi: 10.1109/TCBB.2022.3229678
![]() |
[33] |
D. Li, S. Zhang, X. Ma, Dynamic module detection in temporal attributed networks of cancers, IEEE/ACM Trans. Comput. Biol. Bioinf., 4 (2022), 2219–2230. https://doi.org/10.1109/TCBB.2021.3069441 doi: 10.1109/TCBB.2021.3069441
![]() |
[34] |
X. Ma, W. Zhao, W. Wu, Layer-specific modules detection in cancer multi-layer networks, IEEE/ACM Trans. Comput. Biol. Bioinf., 2022 (2022). https://doi.org/10.1109/TCBB.2022.3176859 doi: 10.1109/TCBB.2022.3176859
![]() |
[35] |
X. Gao, X. Ma, W. Zhang, J. Huang, H. Li, Y. Li, et al., multi-view clustering with self-representation and structural constraint, IEEE Trans. Big Data, 4 (2022), 882–893. https://doi.org/10.1109/TBDATA.2021.3128906 doi: 10.1109/TBDATA.2021.3128906
![]() |
[36] | R. Xie, Z. Liu, H. Luan, M. Sun, Image-embodied knowledge representation learning, in 2017 the 26th International Joint Conference on Artificial Intelligence (IJCAI), (2017), 3140–3146. https://doi.org/10.24963/ijcai.2017/438 |
[37] | P. Pezeshkpour, L. Chen, S. Singh, Embedding multimodal relational data for knowledge base completion, in 2018 the Conference on Empirical Methods in Natural Language Processing (EMNLP), (2018), 3208–3218. https://doi.org/10.18653/v1/D18-1359 |
[38] | J. Yuan, N. Gao, J. Xiang, Transgate: knowledge graph embedding with shared gate structure, in 2019 the AAAI Conference on Artificial Intelligence (AAAI), (2019), 3100–3107. https://doi.org/10.1609/AAAI.V33I01.33013100 |
[39] |
S. Ren, K. He, R. Girshick, J. Sun, Faster R-CNN: Towards real-time object detection with region proposal networks, IEEE Trans. Pattern Anal. Mach. Intell., 39 (2017), 1137–1149. https://doi.org/10.1109/TPAMI.2016.2577031 doi: 10.1109/TPAMI.2016.2577031
![]() |
[40] |
A. Vaswani, N. Shazeer, N. Parmar, J. Uszkoreit, L. Jones, A. N. Gomez, et al., Attention is all you need, Adv. Neural Inf. Process. Syst., (2017), 5998–6008. https://doi.org/10.48550/arXiv.1706.03762 doi: 10.48550/arXiv.1706.03762
![]() |
[41] | Y. Kim, Convolutional neural networks for sentence classification, preprint, arXiv: 1408.5882. |
[42] | Z. Yu, J. Yu, J. Fan, D. Tao, Multi-modal factorized bilinear pooling with co-attention learning for visual question answering, in 2017 the IEEE International Conference on Computer Vision (ICCV), (2017), 1821–1830. https://doi.org/10.1109/ICCV.2017.202 |
[43] | T. Dettmers, M. Pasquale, S. Pontus, S. Riedel, Convolutional 2d knowledge graph embeddings, in 2018 the 32th AAAI Conference on Artificial Intelligence (AAAI), (2018), 1811–1818. https://doi.org/10.1609/aaai.v32i1.11573 |
[44] | K. Toutanova, D. Chen, Observed versus latent features for knowledge base and text inference, in 2015 the 3rd workshop on continuous vector space models and their compositionality, (2015), 57–66. https://doi.org/10.18653/v1/W15-4007 |
[45] |
D. Kingma, J. Ba, Adam: A method for stochastic optimization, Comput. Sci., 34 (2014), 56–67. https://doi.org/10.48550/arXiv.1412.6980 doi: 10.48550/arXiv.1412.6980
![]() |
[46] | B. Yang, S. W. Yih, X. He, J. Gao, L. Deng, Embedding entities and relations for learning and inference in knowledge bases, in 2015 International Conference on Learning Representations (ICLR), (2015), 345–358. https://doi.org/10.48550/arXiv.1412.6575 |
[47] | S. Wang, X. Wei, C. N. Santos, Z. Wang, R. Nallapati, A. Arnold, et al., Mixed-curvature multi-relational graph neural network for knowledge graph completion, in 2021 the International World Wide Web Conference (WWW), (2021), 1761–1771. https://doi.org/10.1145/3442381.3450118 |
[48] | T. Trouillon, J. Welbl, S. Riedel, xE. Gaussier, G. Bouchard, Complex embeddings for simple link prediction, in 2016 the 33rd International Conference on Machine Learning (ICML), (2016), 2071–2080. https://doi.org/10.48550/arXiv.1606.06357 |
1. | Yuchao Zhang, Xiangjie Kong, Zhehui Shen, Jianxin Li, Qiuhua Yi, Guojiang Shen, Bo Dong, A survey on temporal knowledge graph embedding: Models and applications, 2024, 304, 09507051, 112454, 10.1016/j.knosys.2024.112454 | |
2. | Gege Li, Yong Liu, Wei Deng, Yafei Jia, Aoqi Zhang, Difan Qi, 2024, Multi Feature Deep Fusion Based on Relationship Path Enhancement for Multi-Modal Knowledge Graph Completion, 979-8-3315-0658-2, 548, 10.1109/CBASE64041.2024.10824600 |
Algorithm 1 the training of MMCF |
Input: triples (h, r, t) of a graph G; Output: zh, zr, zt 1: For each entity in G 2: Feature Extraction 2: Extract the visual representation o′i of each object by Eq (1); 6: Extract the word representation wi; 3: Transform o′i to vi by fully-connect layer; 4: Intra-modal Correlation Learning 4: Learn the intra-modal correlation between vi by Eq (2); 5: All the vi s are aggregated to obtain the image representation v0∈Rd; 6: Extract the word representation wi; 7: Use 1-dim CNN to encode the intra-modal correlation of wi by Eq (5); 8: Obtain text description embedding e0∈Rd by Eq (6). 9: Inter-modal Correlation Learning 9: Learn visual representation v1∈Rd based on inter-modal correlation by Eq (7); 10: Learn textual representation e1∈Rd based on inter-modal correlation by Eq (8); 12: Cross-modal Feature Fusion 11: Obtain text representation e2 by fusing e0 and e1 using Eq (9); 12: Obtain visual representation v2 by fusing v0 and v1 using Eq (9); 13: Combine v2, e2, s0 to obtain entity representation zh or zt by Eq (11); 14: end for 15: For each relation in G 16: Learn relation representation zr by Eq (12) 17: end for 18: Minimize ϕ(h,r,t) by Eq (13). |
Dataset | #entities | #relation | #train | #valid | #test |
FB-IMG | 11,757 | 1,231 | 285,850 | 29,580 | 34,863 |
WN18RR | 40,943 | 11 | 86,835 | 3,034 | 3,134 |
FB15k-237 | 14,541 | 237 | 272,115 | 17,535 | 20,466 |
Models | WN18RR | FB15k-237 | ||||||
MRR | HITS@1 | HITS@3 | HITS@10 | MRR | HITS@1 | HITS@3 | HITS@10 | |
MuRE | 0.475 | 0.436 | 0.487 | 0.554 | 0.336 | 0.245 | 0.370 | 0.521 |
MuRP | 0.481 | 0.440 | 0.495 | 0.566 | 0.335 | 0.243 | 0.367 | 0.518 |
InteractE | 0.463 | 0.430 | − | 0.528 | 0.354 | 0.263 | − | 0.535 |
ConvKB | 0.249 | 0.057 | 0.417 | 0.524 | 0.243 | 0.155 | 0.371 | 0.421 |
HypER | 0.465 | 0.436 | 0.477 | 0.522 | 0.341 | 0.252 | 0.376 | 0.520 |
DistMult | 0.430 | 0.390 | 0.440 | 0.490 | 0.241 | 0.155 | 0.263 | 0.419 |
M2GNN | 0.485 | 0.444 | 0.498 | 0.572 | 0.362 | 0.275 | 0.398 | 0.565 |
ComplEx | 0.440 | 0.410 | 0.460 | 0.510 | 0.247 | 0.158 | 0.275 | 0.428 |
ConvE | 0.430 | 0.400 | 0.440 | 0.520 | 0.325 | 0.237 | 0.356 | 0.501 |
KG-BERT | − | − | − | 0.524 | − | − | − | 0.420 |
MMCFMuRE | 0.489 | 0.443 | 0.497 | 0.571 | 0.359 | 0.269 | 0.397 | 0.554 |
MMCFInteractE | 0.483 | 0.448 | 0.495 | 0.570 | 0.367 | 0.273 | 0.402 | 0.563 |
Models | MRR | HITS@1 | HITS@3 | HITS@10 |
TransE | − | − | − | 0.494 |
MKRL | − | − | − | 0.645 |
MuRE | 0.765 | 0.703 | 0.807 | 0.874 |
InteractE | 0.813 | 0.762 | 0.849 | 0.895 |
ConvKB | 0.449 | 0.337 | 0.513 | 0.621 |
ComplEx | 0.525 | 0.392 | 0.618 | 0.754 |
ConvE | 0.747 | 0.667 | 0.804 | 0.882 |
MMCFTransE | 0.453 | 0.342 | 0.525 | 0.702 |
MMCFMuRE | 0.820 | 0.768 | 0.852 | 0.899 |
MMCFInteractE | 0.819 | 0.771 | 0.849 | 0.897 |
Models | WN18RR | FB15k-237 | ||||||
MRR | HITS@1 | HITS@3 | HITS@10 | MRR | HITS@1 | HITS@3 | HITS@10 | |
MMCF-intra | 0.471 | 0.426 | 0.479 | 0.554 | 0.342 | 0.256 | 0.384 | 0.544 |
MMCF-inter | 0.475 | 0.429 | 0.482 | 0.556 | 0.348 | 0.258 | 0.389 | 0.546 |
MMCF-gating | 0.480 | 0.435 | 0.491 | 0.566 | 0.353 | 0.265 | 0.396 | 0.552 |
MMCF-text | 0.458 | 0.421 | 0.471 | 0.549 | 0.335 | 0.252 | 0.380 | 0.538 |
MMCF-image | 0.482 | 0.441 | 0.494 | 0.562 | 0.356 | 0.267 | 0.394 | 0.549 |
MMCF-entity | 0.467 | 0.438 | 0.485 | 0.559 | 0.339 | 0.254 | 0.387 | 0.542 |
MMCF | 0.489 | 0.443 | 0.497 | 0.571 | 0.359 | 0.269 | 0.397 | 0.554 |
Embedding Size | MRR | HITS@1 | HITS@3 | HITS@10 |
20 | 0.368 | 0.395 | 0.421 | 0.523 |
40 | 0.476 | 0.428 | 0.468 | 0.551 |
60 | 0.483 | 0.435 | 0.490 | 0.562 |
80 | 0.487 | 0.440 | 0.495 | 0.567 |
100 | 0.489 | 0.443 | 0.497 | 0.571 |
120 | 0.488 | 0.441 | 0.495 | 0.572 |
140 | 0.487 | 0.438 | 0.493 | 0.570 |
160 | 0.485 | 0.435 | 0.490 | 0.565 |
Top-N | MMCF | MuRE | TransE |
1 | stone fruit | structure | fruit tree |
2 | fruit | fruit tree | stone fruit |
3 | citrus fruit | stone fruit | veggie |
4 | fruit tree | seasoning | tree |
5 | structure | computer memory unit | nut |
6 | monocot genus | veggie | citrus fruit |
7 | veggie | citrus fruit | monocot genus |
8 | tree | monocot genus | structure |
9 | produce | tree | root |
10 | root | root | animal |
Algorithm 1 the training of MMCF |
Input: triples (h, r, t) of a graph G; Output: zh, zr, zt 1: For each entity in G 2: Feature Extraction 2: Extract the visual representation o′i of each object by Eq (1); 6: Extract the word representation wi; 3: Transform o′i to vi by fully-connect layer; 4: Intra-modal Correlation Learning 4: Learn the intra-modal correlation between vi by Eq (2); 5: All the vi s are aggregated to obtain the image representation v0∈Rd; 6: Extract the word representation wi; 7: Use 1-dim CNN to encode the intra-modal correlation of wi by Eq (5); 8: Obtain text description embedding e0∈Rd by Eq (6). 9: Inter-modal Correlation Learning 9: Learn visual representation v1∈Rd based on inter-modal correlation by Eq (7); 10: Learn textual representation e1∈Rd based on inter-modal correlation by Eq (8); 12: Cross-modal Feature Fusion 11: Obtain text representation e2 by fusing e0 and e1 using Eq (9); 12: Obtain visual representation v2 by fusing v0 and v1 using Eq (9); 13: Combine v2, e2, s0 to obtain entity representation zh or zt by Eq (11); 14: end for 15: For each relation in G 16: Learn relation representation zr by Eq (12) 17: end for 18: Minimize ϕ(h,r,t) by Eq (13). |
Dataset | #entities | #relation | #train | #valid | #test |
FB-IMG | 11,757 | 1,231 | 285,850 | 29,580 | 34,863 |
WN18RR | 40,943 | 11 | 86,835 | 3,034 | 3,134 |
FB15k-237 | 14,541 | 237 | 272,115 | 17,535 | 20,466 |
Models | WN18RR | FB15k-237 | ||||||
MRR | HITS@1 | HITS@3 | HITS@10 | MRR | HITS@1 | HITS@3 | HITS@10 | |
MuRE | 0.475 | 0.436 | 0.487 | 0.554 | 0.336 | 0.245 | 0.370 | 0.521 |
MuRP | 0.481 | 0.440 | 0.495 | 0.566 | 0.335 | 0.243 | 0.367 | 0.518 |
InteractE | 0.463 | 0.430 | − | 0.528 | 0.354 | 0.263 | − | 0.535 |
ConvKB | 0.249 | 0.057 | 0.417 | 0.524 | 0.243 | 0.155 | 0.371 | 0.421 |
HypER | 0.465 | 0.436 | 0.477 | 0.522 | 0.341 | 0.252 | 0.376 | 0.520 |
DistMult | 0.430 | 0.390 | 0.440 | 0.490 | 0.241 | 0.155 | 0.263 | 0.419 |
M2GNN | 0.485 | 0.444 | 0.498 | 0.572 | 0.362 | 0.275 | 0.398 | 0.565 |
ComplEx | 0.440 | 0.410 | 0.460 | 0.510 | 0.247 | 0.158 | 0.275 | 0.428 |
ConvE | 0.430 | 0.400 | 0.440 | 0.520 | 0.325 | 0.237 | 0.356 | 0.501 |
KG-BERT | − | − | − | 0.524 | − | − | − | 0.420 |
MMCFMuRE | 0.489 | 0.443 | 0.497 | 0.571 | 0.359 | 0.269 | 0.397 | 0.554 |
MMCFInteractE | 0.483 | 0.448 | 0.495 | 0.570 | 0.367 | 0.273 | 0.402 | 0.563 |
Models | MRR | HITS@1 | HITS@3 | HITS@10 |
TransE | − | − | − | 0.494 |
MKRL | − | − | − | 0.645 |
MuRE | 0.765 | 0.703 | 0.807 | 0.874 |
InteractE | 0.813 | 0.762 | 0.849 | 0.895 |
ConvKB | 0.449 | 0.337 | 0.513 | 0.621 |
ComplEx | 0.525 | 0.392 | 0.618 | 0.754 |
ConvE | 0.747 | 0.667 | 0.804 | 0.882 |
MMCFTransE | 0.453 | 0.342 | 0.525 | 0.702 |
MMCFMuRE | 0.820 | 0.768 | 0.852 | 0.899 |
MMCFInteractE | 0.819 | 0.771 | 0.849 | 0.897 |
Models | WN18RR | FB15k-237 | ||||||
MRR | HITS@1 | HITS@3 | HITS@10 | MRR | HITS@1 | HITS@3 | HITS@10 | |
MMCF-intra | 0.471 | 0.426 | 0.479 | 0.554 | 0.342 | 0.256 | 0.384 | 0.544 |
MMCF-inter | 0.475 | 0.429 | 0.482 | 0.556 | 0.348 | 0.258 | 0.389 | 0.546 |
MMCF-gating | 0.480 | 0.435 | 0.491 | 0.566 | 0.353 | 0.265 | 0.396 | 0.552 |
MMCF-text | 0.458 | 0.421 | 0.471 | 0.549 | 0.335 | 0.252 | 0.380 | 0.538 |
MMCF-image | 0.482 | 0.441 | 0.494 | 0.562 | 0.356 | 0.267 | 0.394 | 0.549 |
MMCF-entity | 0.467 | 0.438 | 0.485 | 0.559 | 0.339 | 0.254 | 0.387 | 0.542 |
MMCF | 0.489 | 0.443 | 0.497 | 0.571 | 0.359 | 0.269 | 0.397 | 0.554 |
Embedding Size | MRR | HITS@1 | HITS@3 | HITS@10 |
20 | 0.368 | 0.395 | 0.421 | 0.523 |
40 | 0.476 | 0.428 | 0.468 | 0.551 |
60 | 0.483 | 0.435 | 0.490 | 0.562 |
80 | 0.487 | 0.440 | 0.495 | 0.567 |
100 | 0.489 | 0.443 | 0.497 | 0.571 |
120 | 0.488 | 0.441 | 0.495 | 0.572 |
140 | 0.487 | 0.438 | 0.493 | 0.570 |
160 | 0.485 | 0.435 | 0.490 | 0.565 |
Top-N | MMCF | MuRE | TransE |
1 | stone fruit | structure | fruit tree |
2 | fruit | fruit tree | stone fruit |
3 | citrus fruit | stone fruit | veggie |
4 | fruit tree | seasoning | tree |
5 | structure | computer memory unit | nut |
6 | monocot genus | veggie | citrus fruit |
7 | veggie | citrus fruit | monocot genus |
8 | tree | monocot genus | structure |
9 | produce | tree | root |
10 | root | root | animal |