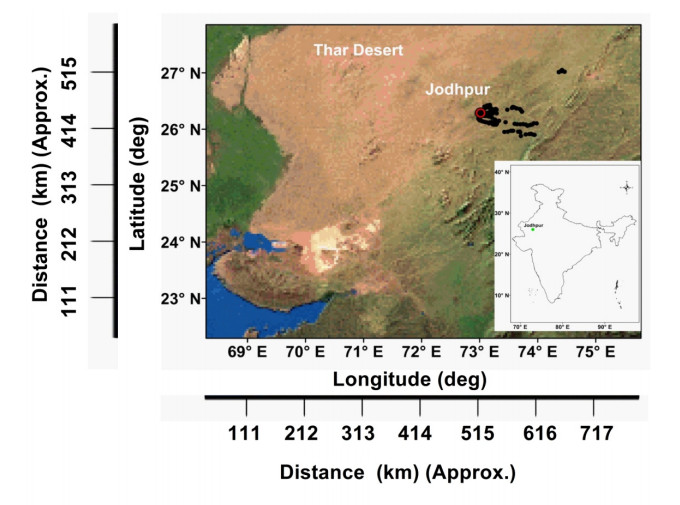
We evaluate temperature and humidity profiles retrieved from an INSAT-3DR sounder against radiosonde measurements for one year during 2017–2018. This evaluation is carried out in terms of slope, intercept, bias, Root Mean Square Error (RMSE), and correlation coefficient in temperature and relative humidity profiles. This comparison provided first-hand information about the performance of INSAT-3DR sounder retrievals. This validation exercise is unique in terms of comparing with the high resolution (1 Hz) radiosonde measurements performed in the afternoon time when there is maximum convection driven by solar radiation. 276 pairs of co-located temperatures have been compared. INSAT-3DR temperature retrievals are strongly linearly correlated to radiosonde measurements, with the slope as 1.001 and correlation coefficient of 0.99, while the mean temperature bias between INSAT-3DR and radiosonde is -0.23°C with RMSE of 1.9°C. Comparison of Relative humidity retrieved from INSAT-3DR to the radiosonde data results in RMSE of 9.8% with a slope of 0.99 with a correlation coefficient of 0.77. The collocation of data points for validation is done matching in space [Latitude, Longitude, Pressure level (height)] and time (±30 minutes). The effect of relaxing the space and time window on the validation statistics is also studied for the first time.
Citation: Hareef Baba Shaeb Kannemadugu, Manish Verma, Dibyendu Dutta, Lianne Rachel Johnson, Srinivasa Rao, Seshasai MVR. Comparison of temperature and humidity profiles retrieved from INSAT-3DR sounder with high resolution radiosonde measurements[J]. AIMS Geosciences, 2021, 7(2): 180-193. doi: 10.3934/geosci.2021011
[1] | Adeeba Ayaz, Maddu Rajesh, Shailesh Kumar Singh, Shaik Rehana . Estimation of reference evapotranspiration using machine learning models with limited data. AIMS Geosciences, 2021, 7(3): 268-290. doi: 10.3934/geosci.2021016 |
[2] | Manogaran Madhiarasan . Long-term wind speed prediction using artificial neural network-based approaches. AIMS Geosciences, 2021, 7(4): 542-552. doi: 10.3934/geosci.2021031 |
[3] | Inthuorn Sasanakul, Sarah Gassman, Pitak Ruttithivaphanich, Siwadol Dejphumee . Characterization of shear wave velocity profiles for South Carolina Coastal Plain. AIMS Geosciences, 2019, 5(2): 303-324. doi: 10.3934/geosci.2019.2.303 |
[4] | Leszek J. Kaszubowski . Seismic profiling of the sea-bottom in recognition of geotechnical conditions. AIMS Geosciences, 2020, 6(2): 199-230. doi: 10.3934/geosci.2020013 |
[5] | Evgeniy V. Torgashov, Aleksandra V. Varnavina . Site Characterization during Bridge Foundation Construction Using Electrical Resistivity Tomography. AIMS Geosciences, 2016, 2(3): 201-213. doi: 10.3934/geosci.2016.3.201 |
[6] | Gerardo W Quirós, Patricia M Peters, Kuat C Gan . Combining NSP- and CPTu-based Nkt to evaluate undrained shear strength. AIMS Geosciences, 2023, 9(1): 95-122. doi: 10.3934/geosci.2023007 |
[7] | Thomas Fauchez, Giada Arney, Ravi Kumar Kopparapu, Shawn Domagal Goldman . Explicit cloud representation in the Atmos 1D climate model for Earth and rocky planet applications. AIMS Geosciences, 2018, 4(4): 180-191. doi: 10.3934/geosci.2018.4.180 |
[8] | Natascha T. Torres, Thomas Steinsberger, Helen Droz-Georget, Beat Müller, Helmut Brandl, Peter C. Hauser, Gerhard Furrer . A Novel Method to Quantify Bioavailable Elements and Mobile ATP on Rock Surfaces and Lichens. AIMS Geosciences, 2016, 2(3): 245-258. doi: 10.3934/geosci.2016.3.245 |
[9] | Quentin Fiacre Togbévi, Luc Ollivier Sintondji . Hydrological response to land use and land cover changes in a tropical West African catchment (Couffo, Benin). AIMS Geosciences, 2021, 7(3): 338-354. doi: 10.3934/geosci.2021021 |
[10] | Corey A. Palmer, Katherine P. Markstein, Lawrence H. Tanner . Experimental test of temperature and moisture controls on the rate of microbial decomposition of soil organic matter: preliminary results. AIMS Geosciences, 2019, 5(4): 886-898. doi: 10.3934/geosci.2019.4.886 |
We evaluate temperature and humidity profiles retrieved from an INSAT-3DR sounder against radiosonde measurements for one year during 2017–2018. This evaluation is carried out in terms of slope, intercept, bias, Root Mean Square Error (RMSE), and correlation coefficient in temperature and relative humidity profiles. This comparison provided first-hand information about the performance of INSAT-3DR sounder retrievals. This validation exercise is unique in terms of comparing with the high resolution (1 Hz) radiosonde measurements performed in the afternoon time when there is maximum convection driven by solar radiation. 276 pairs of co-located temperatures have been compared. INSAT-3DR temperature retrievals are strongly linearly correlated to radiosonde measurements, with the slope as 1.001 and correlation coefficient of 0.99, while the mean temperature bias between INSAT-3DR and radiosonde is -0.23°C with RMSE of 1.9°C. Comparison of Relative humidity retrieved from INSAT-3DR to the radiosonde data results in RMSE of 9.8% with a slope of 0.99 with a correlation coefficient of 0.77. The collocation of data points for validation is done matching in space [Latitude, Longitude, Pressure level (height)] and time (±30 minutes). The effect of relaxing the space and time window on the validation statistics is also studied for the first time.
Vertical profiles of meteorological parameters (temperature, relative humidity, wind speed and direction, pressure) are important for understanding boundary-layer processes, radiative transfer calculations, air pollution meteorology, Numerical Weather Prediction (NWP), climate monitoring and to validate the same parameters retrieved from satellites. Upper air temperature, winds, and water vapor are also Essential Climate Variables (ECV) [1]. These profiles are measured using balloon-borne radiosondes equipped with sensors measuring temperature and humidity with high vertical resolution and accuracy, whose data are relayed to a receiving station on the ground. However, these profiles can also be retrieved from satellite measured radiances using the first guess profile data from NWP or the climatology. Satellite-based profiles have the advantage of larger spatial and temporal coverage as compared to point observations of radiosonde.
Kaplan [2] laid out the idea of inferring the vertical profile of temperature from remote radiation measurements in the infrared part of the spectrum as this is very sensitive to the vibration and rotation of certain molecules, which absorb/emit the radiation at a similar frequency. Houghton and smith [3] majorly contributed to the theory of atmospheric sounding and the realization of the satellite platform [4,5,6]. The satellite sounders are mainly the High-Resolution Infrared Sounder (HIRS) and the Advanced Microwave Sounding Unit (AMSU), the Atmospheric Infra-Red Sounder (AIRS) [7], the Infrared Atmospheric Sounding Interferometer (IASI) [8], The Cross-Track Infrared Sounder (CrIS) and the Advanced Technology Microwave Sounder (ATMS) onboard the Suomi-NPP [9]. These polar-orbiting sounders profiles though have a good spatial resolution but the poor temporal resolution (time needed to revisit and acquire data for the exact same location) which is related to the precession (the rotation of the orbit plane around the polar axis) of the plane of the orbits. However, geostationary satellites, such as INSAT-3D launched in 2013, offer a high temporal resolution of vertical profiles of temperature and humidity over the Indian region though not at a global scale. INSAT-3DR, launched in 2016, is similar to INSAT -3D with few improvements, such as higher spatial resolution in the visible and thermal infrared bands (https://www.isro.gov.in/Spacecraft/insat-3dr).
It is very important to validate the profiles retrieved from the sounders with the in situ observations i.e. ground truth (Radiosonde). This is to know the biases and the related uncertainties which in turn can be useful for the data assimilation for NWP models, for example, a sensitivity analysis has shown that a 1 K variation of Ts in the model can incur a maximum bias of 29 and 30 W/m2 in sensible and latent heat fluxes [10]. Towards this, Mitra et al. [11] compared INSAT-3D profiles with those of radiosondes (at pressure levels from 1000 hPa to 100 hPa) launched at 00:00 UTC/05:30 LT for about five months in 2014, whose data were collected by Indian Meteorological Department (IMD). Ratnam et al. [12] made a similar comparison using profiles of radiosondes launched at 12:00 UTC/17:30 LT at Gadanki and also utilized IMD Station data (launch time at 00:00 UTC/5:30 LT). The comparison data pairs were obtained by interpolating the radiosonde data about the pressure levels (up to 10 hPa) of INSAT-3D and considering the data available within ± 1° latitude and longitude from a given station and within ± 1 h time window. Singh et al. [13] validated INSAT-3D profiles using radiosonde data (launch time at 00:00 UTC and 12:00 UTC for e.g Durre et al. [14,15]) available at Integrated Global Radiosonde Archive (IGRA). They have used collocation criteria of the time window of 1 hour and a distance window of 0.25° from radiosonde measurements (pressure level up to 100 hPa) as the GPS coordinates of the balloon were not available. However, validation of temperature and humidity retrievals from INSAT-3DR is not yet reported.
In the present study, we compare Temperature (T) and Relative Humidity (RH) profiles retrieved from the INSAT-3DR sounder using the year-round high resolution (1 Hz) radiosonde measurements performed in the afternoon time (09:00 UTC/14:00 LT) at Jodhpur in western India. The collocation of data points is done in matching three dimensions (Latitude, Longitude, and Altitude (pressure up to 0 hPa)) and time (±30 minutes). We also studied for the first time the effect of relaxing the space and time window on the validation statistics.
The present study utilizes the data from the observations done using balloon-borne Pisharoty GPS radiosondes (Ver. B3) [16,17], which are widely used for atmospheric studies by Indian weather agencies and research institutions [18,19,20]. The sensors used for measuring T and RH have an accuracy of ±1℃ (range −90 to 60℃) and ± 5% (range 0 to 100%) respectively. The data contains atmospheric variables, pressure, temperature, relative humidity, and the position (Latitude, Longitude, and Altitude) of the balloon with a time resolution of about 1 second. These sondes were launched on Wednesdays at about 14:00 local time (July 2017 to May 2018) at Jodhpur (Lat. 26.23° N; Lon. 73.02°E) located at the gateway of the Thar Desert, Rajasthan, India (Figure 1). The afternoon time is suitable to capture fully developed convective activities [18,20], and a total of 22 soundings are considered for this study. We consider soundings which are on clear sky days only. Figure 2a and 2b shows the Temperature and humidity profile obtained from the radiosounding performed on 19/07/2017.
The INSAT-3DR, similar to INSAT-3D, is an advanced meteorological satellite of India configured with an imaging System and an Atmospheric Sounder launched from Sriharikota, Andhra Pradesh, by the Indian launch vehicle GSLV-F05 on 8th Sept 2016 into the geostationary orbit (altitude of ~35,786 km, location at 74° East). The imager has six channels (VIS (0.55-0.75 µm), SWIR (1.55-1.70 µm), NIR (3.80-4.0 µm), WV (6.5-7.1 µm), TIR-1 (10.2-11.2 µm) and TIR-2 (11.5-12.5 µm) and the sounder has 18 IR channels, which consist of longwave and shortwave bands and one visible band (for cloud detection in the daytime) (https://www.mosdac.gov.in/insat-3drpayloads). The important aspect of INSAT-3DR is that the sounder is operated from a geostationary platform i.e. stationary as seen from the ground. Therefore, it is capable to follow the weather pattern because of its time continuity.
This atmospheric sounder gives profiles of temperature and specific humidity at 40 pressure levels (1000,950,920,850,750,700,670,620,570,500,475,430,400,350,300,250,200,150,135,115,100, 85, 70, 60, 50, 30, 25, 20, 15, 10, 7, 5, 4, 3, 2, 1.5, 1, 0.5, 0.2, 0.1 hPa) at hourly interval and 10 km × 10 km resolution over India region. We have used sounder Level-2 data product available at mosdac.gov.in and T and RH profiles from the INSAT-3DR sounder are provided only in clear sky conditions. Figure 3 shows the sample sounder data. The retrieval procedure for T and RH involves a two-step method. In the first step, first guess profiles are derived (using regression and model forecast) and in the second step, accurate profiles are retrieved using a physical retrieval scheme (uses iterative method) [21]. This approach is the same as adopted for GOES (Geostationary Operational Environmental Satellite) [22,23,24]. For the quality control criteria for the level-2 product of INSAT 3DR, at each iterative step in the physical retrieval procedure, convergence tests are carried out for the profile retrievals. Two convergence tests namely the expansion coefficient convergence test and the brightness temperature residual test are carried out at each iteration and if one of the tests fails, then the retrieval is rejected [21].
Data collocation is carried out in two categories. In Category-1, the collocation of data points for validation is done matching exactly (up to one decimal place) in space [Latitude, Longitude, Pressure level (height)] and time (±30 minutes). In category-2, the latitude and longitude are relaxed in steps of 0.05 deg up to 0.25 deg to see the effect of relaxation in space. The various datasets, each consisting of 276 comparison pairs, are obtained from the same in-situ (radiosounding) measurements of category 1, by associating each of them with satellite measurements increasingly distant, increasing or reducing both their latitude and longitude in steps of 0.05° up to 0.25°.Similarly, the time window is relaxed in steps of 30 minutes up to 2 hours to study the effect of time relaxation on validation statistics.
In this section comparison statistics of INSAT-3DR retrieved T and RH profiles with radiosonde measurements are presented.
Figure 4a and 4b shows the collocated temperature profiles measured by radiosondes and retrieved from INSAT-3DR for the 27th December 2017 and whole comparison period respectively and infers that the INSAT-3DR can capture well the general decrease of temperature in the troposphere with a decrease in pressure (increase in Altitude). Above the tropopause (100 hPa) general increase in temperature with a decrease in pressure (Increase in altitude) is also well captured. This signifies the fact that the INSAT-3DR sounder can be used for studying the cold point tropopause altitude and temperature which in turn will decide the possibility of stratospheric intrusions [25].
Figure 4c shows a linear regression plot between Radiosonde measured temperatures vs INSAT-3DR retrieved temperatures for collocation data points (category-1) matched in space and time. 276 pairs of co-located temperatures have been compared. INSAT-3DR temperature retrievals are strongly linearly correlated to radiosonde measurements, with a slope of 1.001 and correlation coefficient of 0.99, while the mean temperature bias between INSAT-3DR and radiosonde is −0.23℃ with Root Mean Square Error (RMSE) of 1.9℃. RMSE of up to 2℃, the higher bias of 0.65 ℃ and similar slope and correlation coefficient (up to 100 hPa) is reported by Singh et al. [13]. Ratnam et al. [12] reported similar results (up to 10 hPa) of bias up to 2 K. Compared to the previous validations, the lower bias in our study is probably due to the smaller width of space and time window used for data collocation, through a larger data set and simultaneous analysis would be needed to confirm this hypothesis, while the negative bias might be due to a greater warm bias of radiosonde temperature sensor due to solar radiation for radiosondes launched at 14:00 LT, compared to those launched at 17:30 LT and 05.30 LT in previous validations, although radiosonde temperature measurements are corrected for solar radiation effects.
Figure 4d shows the profile of mean collocated temperature biases between INSAT-3DR and radiosondes [(T3DR-TR)mean] for the whole comparison period, with the corresponding standard deviations (horizontal bars). The bias can be positive or negative from 1000 hPa to 300 hPa, ranging within ±3℃ up to 500 hPa and within ±2℃ from 500 hPa to 300 hPa. From 300 hPa to 100 hPa the temperature bias is positive, with values typically lower than 2℃ and in some cases higher, up to more than 3℃. Above 100 hPa, in the upper troposphere and lower stratosphere (UTLS) region, the bias can be positive, with values typically lower than 2℃ and in some cases higher, up to more than 3℃, or negative, with values typically lower than 3℃ and in some cases higher, up to more than 4℃. So the biases observed need to be taken care of while using the temperature profiles retrieved from INSAT-3DR data for different studies for boundary layer (below about 850 hPa), free troposphere, and UTLS region.
We also study for the first time the dependence of validation statistics on the space and time window selected for the comparison data sets, as the different authors follow different space and time windows. Table. 1 shows the different statistics with the variation of latitude and longitude in the steps of ±0.05 deg up to 0.25 degree and the variation of the time window in steps of 30 minutes up to 2 hours. It is observed that there is a variation in RMSE (up to about 10%) and bias (up to about 60%), but not much in slope and correlation coefficient, when the space window is varied. These variations are lower when the time window is varied (up to about 3% and 50% for RMSE and bias, respectively).
Distance (±) deg | slope | intercept | RMSE (SD) | N | Bias | r |
0 | 1.001 | −0.20677 | 1.90726 | 276 | −0.2 | 0.99846 |
−0.05 | 0.997 | −0.456 | 1.84 | 276 | −0.4 | 0.99855 |
−0.1 | 0.999 | −0.30995 | 1.87084 | 276 | −0.3 | 0.99851 |
−0.15 | 0.998 | −0.38977 | 1.81382 | 276 | −0.3 | 0.9986 |
−0.2 | 0.994 | −0.61181 | 1.7414 | 276 | −0.5 | 0.9987 |
−0.25 | 0.997 | −0.47134 | 1.77265 | 276 | −0.4 | 0.99868 |
0.05 | 0.999 | −0.35961 | 1.93632 | 276 | −0.3 | 0.99841 |
0.1 | 0.998 | −0.41297 | 1.94912 | 276 | −0.4 | 0.99838 |
0.15 | 0.997 | −0.43234 | 1.88191 | 276 | −0.4 | 0.99849 |
0.2 | 0.997 | −0.41859 | 1.86106 | 276 | −0.3 | 0.99852 |
0.25 | 0.996 | −0.41439 | 1.85309 | 276 | −0.3 | 0.99853 |
Time (hr) | ||||||
0.5 | 1.001 | −0.20677 | 1.90726 | 276 | −0.2 | 0.99846 |
1 | 0.999 | −0.30147 | 1.86207 | 279 | −0.3 | 0.99847 |
1.5 | 0.993 | −0.5528 | 1.91093 | 421 | −0.3 | 0.9982 |
2 | 0.991 | −0.67313 | 1.92643 | 536 | −0.4 | 0.99811 |
Figure 5a and 5b shows the collocated relative humidity profiles measured by radiosondes and retrieved from INSAT-3DR for 27th December 2017 and the whole comparison period respectively. The relative humidity is high within the tropopause, where it varies from 10% up to 50%, but drastically decreases above, where it is less than 10%. Below the tropopause, where RH is considerably high, INSAT-3DR can capture well the RH variation with the pressure of radiosondes, although there is a difference in RH values. In contrast, above the tropopause, where RH is very low, RH variations with the pressure of INSAT-3DR and radiosondes do not match.
Figure 5c shows a linear regression plot between radiosonde measured relative humidity vs. INSAT-3DR retrieved relative humidity for collocation data points (category-1) matched in space and time. 276 pairs of co-located relative humidity values have been compared. INSAT-3DR relative humidity retrievals show a good linear correlation with radiosonde measurements, with a correlation coefficient of 0.77 and slope of 0.99. The mean relative humidity bias between INSAT-3DR and radiosonde is −0.06% with an RMSE of 9.8%, which reveals that the relative humidity values retrieved from INSAT-3DR data need to be used with caution while assimilating in NWP models. RMSE of up to 20%, the higher bias of 0.87% and slope of 0.75, and correlation coefficient (up to 200 hpa) of 0.82 are reported by Singh et al. [13]. Ratnam et al. [12] reported similar results (up to 300 hPa) with a mean difference in water vapor of 20–30 %. Compared to previous validations, the lower bias and RMSE in our study might be due to the smaller width of space and time window used for data collocation; however, a larger data set and simultaneous analysis would be needed to confirm this hypothesis. This could be related to the high variability of relative humidity in the spatial and temporal domain, particularly in the lower troposphere. The diurnal variation in water vapor in the atmosphere is influenced by local geographical conditions such as land/sea breeze or mountain/valley or large water bodies such as rivers/lakes that change circulation patterns and temperature [26]. In addition to this, air mass changes at a larger scale also influence the moisture availability in the atmosphere. Variation in air temperature controls temporal variability in the atmospheric water vapor via the Clausius-Clapeyron equation and through a different type of convection i.e. shallow convection, turbulent flux within the boundary layer, and deep convection. Hence, smaller width of the space and time window is advisable for data collocation. Also, a plausible cause for the disagreement between in-situ and satellite measurements of temperature and relative humidity could be the scaling effect that is traced in the in-situ data because they are characterized by higher resolution [27].
Figure 5d shows the profile of mean collocated relative humidity biases between INSAT-3DR and radiosondes [(RH3DR-RHR)mean] for the whole comparison period with the corresponding standard deviations (horizontal bars). From 1000 hPa to 620 hPa the bias can be positive, with values typically lower than 25%, or negative, with values lower than 15%. Above 620 hPa up to 250 hPa, the bias is negative, with values lower than 10%. Above 250 hPa, in the upper troposphere and lower stratosphere (UTLS), the bias can be positive, with values typically lower than 20% and in some cases higher, up to 40%, or negative, with values lower than 10%. The observed biases could be related to the dependence of retrieval quality on surface emissivity, undetected clouds, and the influence of moisture content in the atmosphere [13]. Radiosonde measurements are also known to have a dry bias in a relatively cold and dry environment such as the UTLS region [28]. The differences also can arise due to the vertical resolution differences between the Radiosonde measurements and satellite sounder retrievals. So the biases observed need to be taken care of while using the relative humidity profiles retrieved from INSAT-3DR data for different studies involving different pressure levels.
Table 2 shows the different statistics with the variation of latitude and longitude in the steps of ± 0.05 deg up to 0.25 degree and the variation of the time window in steps of 30 minutes up to 2 hours. It is observed that there is a variation in RMSE (about 10%), Bias (about 90%), and correlation coefficient (5%), but not much in slope, when the space window is varied. These variations are higher when the time window is varied (about 30% for slope, about 32% for RMSE, about 95% for bias, and about 22% for correlation coefficient). Therefore, the validation statistics of relative humidity profiles retrieved from satellite data, in particular, the bias to radiosonde measurements, depends significantly on the space and time window selected for the comparison of data sets. The data collocation criteria might be the major factor behind the differences in the validation statistics reported by us and other authors [11,12,13], in addition to a difference between INSAT 3D and 3DR satellite platforms and equipment.
Distance (±) deg | slope | intercept | RMSE (SD) | N | Bias | r |
0 | 0.99262 | 0.15803 | 9.76068 | 276 | 0.06 | 0.77047 |
−0.05 | 1.03651 | −0.25206 | 8.80229 | 276 | 0.23312 | 0.81364 |
−0.1 | 1.01596 | 0.17311 | 9.6484 | 276 | 0.38517 | 0.78122 |
−0.15 | 1.01746 | 0.14665 | 9.75326 | 276 | 0.37867 | 0.77837 |
−0.2 | 1.01429 | 0.35328 | 9.89702 | 276 | 0.54316 | 0.77288 |
−0.25 | 1.00325 | 0.37309 | 9.82383 | 276 | 0.41661 | 0.77095 |
0.05 | 1.01799 | 0.0117 | 9.61059 | 276 | 0.25078 | 0.78302 |
0.1 | 1.01444 | 0.04735 | 9.41379 | 276 | 0.23921 | 0.78819 |
0.15 | 1.01322 | −0.03403 | 9.23815 | 276 | 0.14159 | 0.79342 |
0.2 | 1.01153 | −0.12006 | 8.85482 | 276 | 0.03313 | 0.80517 |
0.25 | 1.00965 | 0.074 | 9.31834 | 276 | 0.20221 | 0.78982 |
Time (hr) | ||||||
0.5 | 0.99262 | 0.15803 | 9.76068 | 276 | 0.06 | 0.77047 |
1 | 0.74143 | 5.4424 | 13.39716 | 279 | 1.23112 | 0.59909 |
1.5 | 0.70612 | 3.288 | 12.31716 | 421 | 1.17149 | 0.58493 |
2 | 0.86713 | 1.51131 | 14.25768 | 536 | 0.51987 | 0.61437 |
We compared the vertical profiles of temperature and relative humidity retrieved from the INSAT-3DR sounder (available as a product) with year-round (2017–2018) measurements from radiosondes launched at afternoon time (14:00 LT) over Jodhpur. The main findings are as follows.
1) INSAT-3DR can capture well the general trend of temperature variation in the troposphere and lower stratosphere and due to its high temporal resolution can be used in studies of diurnal variation of the thermo dynamical state of the atmosphere.
2) INSAT-3DR temperature retrievals are linearly correlated to radiosonde measurements, with a correlation coefficient of 0.99. The mean temperature bias between INSAT-3DR and radiosondes is −0.23℃ with an RMSE of 1.9℃. The temperature bias is higher in the troposphere, below 500 hPa, where it ranges within ±3℃, and in UTLS, above 100 hPa, where it can exceed 3℃ in absolute value. These results reveal that the temperature profiles retrieved from INSAT-3DR data should be used with caution in studies involving different pressure levels, as well as for their assimilation in NWP models.
3) INSAT-3DR can capture the relative humidity variation with pressure in the troposphere, while this variation significantly differs from that of radiosondes in the lower stratosphere. INSAT-3DR relative humidity retrievals show a good linear correlation with radiosonde measurements with a correlation coefficient of 0.77, while the mean bias between INSAT-3DR and radiosondes is −0.06% with RMSE of 9.8%. The bias is higher, with values typically lower than 25% and in some cases up to 40%, in the troposphere, below 620 hPa, and in UTLS, above 250 hPa. These biases indicate that the relative humidity profiles retrieved from INSAT-3DR data should be used with caution, and improvements are needed in their retrieval algorithms.
4) The study on the dependence of validations statistics on the selection of space and time window for collocating comparison data sets suggests that there is not much dependence for the temperature (with respect to both space and time window) but there is a significant variation in the case of relative humidity (less with respect to space window and more with respect to time window). Therefore, for the validation of relative humidity retrieved from the INSAT-3DR sounder, the exact matching in space and time (±30 minutes) of satellite and radiosonde data sets is strongly recommended. This will aid in the correct assessment of the performance of satellite retrievals.
Radiosonde launches (at 14:00 LT) are planned from different climatic regions of India under the Radiosonde Network for National Information system for Climate and Environmental Studies (RANN) project. Data from these radiosoundings will be used to consolidate the statistical validation of temperature and humidity profiles retrieved from INSAT-3DR with a larger number of collocated profiles. This exercise will be useful both for the corrections of retrieval algorithms and for data assimilation in NWP models.
The present study is done as a part of the Radiosonde Network for the NICES (RANN) project. We thank RRSC staff, Chief General Manager, Regional centers, and Director, NRSC for the support and encouragement throughout this study. We are also grateful to the Meteorological and Oceanographic Satellite Data Archival Centre (MOSDAC) for hosting the INSAT-3DR data through the website (www.mosdac.gov.in). We thank the academic editor and anonymous reviewers for their critical comments.
The authors declare no conflict of interest.
[1] | GCOS, The Global observing system for climate: Implementation needs, 2016. Available from: https://unfccc.int. |
[2] |
Kaplan LD (1959) Inference of atmospheric structure from remote radiation measurements. J OPT Soc Am 49: 1004-1007. doi: 10.1364/JOSA.49.001004
![]() |
[3] | Houghton JT, Smith SD (1966) Infrared Physics, Oxford: Oxford University Press. |
[4] | Peckham G, Rodgers CD, Houghton JT, et al. (1966) Remote Temperature Sensing of the Earth's Atmosphere Using a Selective Chopper Radiometer, Proceedings of the Symposium on Electromagnetic Sensing of the Earth from Satellites, Miami Beach, Florida. |
[5] |
Ellis P, Holah G, Houghton JT, et al. (1973) Remote Sounding of Atmospheric Temperature from Satellites IV. The Selective Chopper Radiometer for Nimbus 5. Pro R Soc London Ser A 334: 149-170. doi: 10.1098/rspa.1973.0085
![]() |
[6] |
Cracknell AP, Varotsos CA (2021) Editorial Sir John Houghton. Remote Sensing Letters 12: 364-376. doi: 10.1080/2150704X.2021.1881649
![]() |
[7] |
Aumann HH, Chahine MT, Gautier C, et al. (2003) AIRS/AMSU/HSB on the AQUA Mission: Design, Science Objectives, Data Products, and Processing Systems. IEEE Trans Geosci Remote Sens 41: 253-264. doi: 10.1109/TGRS.2002.808356
![]() |
[8] | Blumstein D, Chalon G, Carlier T, et al. (2004) IASI Instrument: Technical Overview and Measured Performances. SPIE 5543: 196. |
[9] |
Han Y, Rivercomb H, Cromp M, et al. (2013) Suomi NPP Crls measurements, sensor data record algorithm, calibration and validation activities, and record data quality. J Geophys Res Atmos 118: 12734-12748. doi: 10.1002/2013JD020344
![]() |
[10] |
Cracknell AP, Varotsos CA (2011) New Aspects of Global Climate-dynamics Research and Remote Sensing Preface. Int J Remote Sens 32: 579-600. doi: 10.1080/01431161.2010.517807
![]() |
[11] | Mitra A, Bhan S, Sharma A, et al. (2015) INSAT-3D vertical profile retrievals at IMDPS, New Delhi. Mausam 66: 687-694. |
[12] |
Ratnam MV, Kumar AH, Jayaraman A (2016) Validation of INSAT-3D sounder data with in situ measurements and other similar satellite observations over India. Atmos Meas Tech 9: 5735-5745. doi: 10.5194/amt-9-5735-2016
![]() |
[13] |
Singh T, Mittal R, Shukla MV (2017) Validation of INSAT-3D temperature and moisture sounding retrievals using matched radiosonde measurements. Int J Remote Sens 3: 3333-3355. doi: 10.1080/01431161.2017.1294776
![]() |
[14] |
Durre I, Yin X (2008) Enhanced radiosonde data for studies of vertical structure. Bull Amer Meteor Soc 89: 1257-1262. doi: 10.1175/2008BAMS2603.1
![]() |
[15] |
Durre I, Yin, X, Vose RS, et al. (2018) Enhancing the Data Coverage in the Integrated Global Radiosonde Archive. J Atmos Oceanic Technol 35: 1753-1770. doi: 10.1175/JTECH-D-17-0223.1
![]() |
[16] | Satyanarayana S (2008) GPS radiosonde development. Technology Development for Atmospheric Research and Applications, ISRO, 142-188. |
[17] |
Divya US, Girija J, Satyanarayana S, et al. (2014) An Advanced Radiosonde System for Aerospace Applications. J Atmos Oceanic Technol 31: 2067-2077. doi: 10.1175/JTECH-D-13-00050.1
![]() |
[18] |
Nair SK, Anurose TJ, Subrahamanyam DB, et al. (2011) Characterization of the vertical structure of coastal atmospheric boundary layer (cabl) over Thumba (8.5N, 76.9E) during different seasons. Adv Meteorol 2011: 1-9. doi: 10.1155/2011/390826
![]() |
[19] |
Kompalli SK, Suresh Babu S, Krishna Moorthy K, et al. (2014) Aerosol black carbon characteristics over Central India: Temporal variation and its dependence on mixed layer height. Atmos Res 147-148: 27-37. doi: 10.1016/j.atmosres.2014.04.015
![]() |
[20] | Kannemadugu HBS (2019) Seasonal Characteristics of Atmospheric Boundary Layer and its Associated Dynamics over Central India. Asia-Pacific J Atmos Sci. |
[21] | Space Application Centre, Ahmedabad, India. INSAT-3D Algorithm Theoretical Basis Development Document (ATBD), 2015. Available from: http://mosdac.gov.in/data/doc/INSAT_3D_ATBD_MAY_2015.pdf. |
[22] |
Li J, Wolf WW, Menzel WP, et al. (2000) Global soundings of the atmosphere from ATOVS measurements: The algorithm and validation. J Appl Meteorol 39: 1248-1268. doi: 10.1175/1520-0450(2000)039<1248:GSOTAF>2.0.CO;2
![]() |
[23] |
Ma XL, Schmit T, Smith W (1999) A non-linear physical retrieval algorithm-Its application to the GOES-8/9 sounder. J Appl Meteor 38: 501-503. doi: 10.1175/1520-0450(1999)038<0501:ANPRAI>2.0.CO;2
![]() |
[24] |
Hayden CM (1988) GOES-VAS simultaneous temperature-moisture retrieval algorithm. J Appl Meteor 27: 705-733. doi: 10.1175/1520-0450(1988)027<0705:GVSTMR>2.0.CO;2
![]() |
[25] |
Suneeth KV, Das SS, Das SK (2017) Diurnal variability of the global tropical tropopause: results inferred from COSMIC observations. Clim Dyn 49: 3277-3292. doi: 10.1007/s00382-016-3512-x
![]() |
[26] |
Meza A, Mendoza MP, Natali C, et al. (2020) Diurnal variation of precipitable water vapor over Central and South America. Geod Geodyn 11: 426-441. doi: 10.1016/j.geog.2020.04.005
![]() |
[27] |
Efstathiou MN, Varotsos CA (2010) On the altitude dependence of the temperature scaling behaviour at the global troposphere. Int J Remote Sens 31: 343-349. doi: 10.1080/01431160902882702
![]() |
[28] |
Vömel H, Selkirk H, Miloshecich, L, et al. (2007) Radiation dry bias of the Vaisala RS92 humidity sensor. J Atmos Oceanic Technol 24: 953-963. doi: 10.1175/JTECH2019.1
![]() |
1. | Hareef baba shaeb Kannemadugu, Sandelger Dorligjav, Biswadip Gharai, Seshasai M.V.R, Satellite-Based Air Pollution Potential Climatology over India, 2021, 232, 0049-6979, 10.1007/s11270-021-05324-8 | |
2. | G. S. Gopikrishnan, J. Kuttippurath, P. K. Thapliyal, M. V. Shukla, Validation of INSAT‐3D and INSAT‐3DR Temperature Profile Retrievals Using Ground‐Based, Satellite, and Reanalysis Data, 2023, 128, 2169-897X, 10.1029/2023JD038912 | |
3. | C. Mahesh, Dileep P.K., Anish Kumar M. Nair, K.V.S. Namboodiri, Satellite derived mixing ratio profile, a resourceful indicator of heat waves: INSAT-3DR sounder based analysis, 2024, 73, 02731177, 4125, 10.1016/j.asr.2024.01.010 |
Distance (±) deg | slope | intercept | RMSE (SD) | N | Bias | r |
0 | 1.001 | −0.20677 | 1.90726 | 276 | −0.2 | 0.99846 |
−0.05 | 0.997 | −0.456 | 1.84 | 276 | −0.4 | 0.99855 |
−0.1 | 0.999 | −0.30995 | 1.87084 | 276 | −0.3 | 0.99851 |
−0.15 | 0.998 | −0.38977 | 1.81382 | 276 | −0.3 | 0.9986 |
−0.2 | 0.994 | −0.61181 | 1.7414 | 276 | −0.5 | 0.9987 |
−0.25 | 0.997 | −0.47134 | 1.77265 | 276 | −0.4 | 0.99868 |
0.05 | 0.999 | −0.35961 | 1.93632 | 276 | −0.3 | 0.99841 |
0.1 | 0.998 | −0.41297 | 1.94912 | 276 | −0.4 | 0.99838 |
0.15 | 0.997 | −0.43234 | 1.88191 | 276 | −0.4 | 0.99849 |
0.2 | 0.997 | −0.41859 | 1.86106 | 276 | −0.3 | 0.99852 |
0.25 | 0.996 | −0.41439 | 1.85309 | 276 | −0.3 | 0.99853 |
Time (hr) | ||||||
0.5 | 1.001 | −0.20677 | 1.90726 | 276 | −0.2 | 0.99846 |
1 | 0.999 | −0.30147 | 1.86207 | 279 | −0.3 | 0.99847 |
1.5 | 0.993 | −0.5528 | 1.91093 | 421 | −0.3 | 0.9982 |
2 | 0.991 | −0.67313 | 1.92643 | 536 | −0.4 | 0.99811 |
Distance (±) deg | slope | intercept | RMSE (SD) | N | Bias | r |
0 | 0.99262 | 0.15803 | 9.76068 | 276 | 0.06 | 0.77047 |
−0.05 | 1.03651 | −0.25206 | 8.80229 | 276 | 0.23312 | 0.81364 |
−0.1 | 1.01596 | 0.17311 | 9.6484 | 276 | 0.38517 | 0.78122 |
−0.15 | 1.01746 | 0.14665 | 9.75326 | 276 | 0.37867 | 0.77837 |
−0.2 | 1.01429 | 0.35328 | 9.89702 | 276 | 0.54316 | 0.77288 |
−0.25 | 1.00325 | 0.37309 | 9.82383 | 276 | 0.41661 | 0.77095 |
0.05 | 1.01799 | 0.0117 | 9.61059 | 276 | 0.25078 | 0.78302 |
0.1 | 1.01444 | 0.04735 | 9.41379 | 276 | 0.23921 | 0.78819 |
0.15 | 1.01322 | −0.03403 | 9.23815 | 276 | 0.14159 | 0.79342 |
0.2 | 1.01153 | −0.12006 | 8.85482 | 276 | 0.03313 | 0.80517 |
0.25 | 1.00965 | 0.074 | 9.31834 | 276 | 0.20221 | 0.78982 |
Time (hr) | ||||||
0.5 | 0.99262 | 0.15803 | 9.76068 | 276 | 0.06 | 0.77047 |
1 | 0.74143 | 5.4424 | 13.39716 | 279 | 1.23112 | 0.59909 |
1.5 | 0.70612 | 3.288 | 12.31716 | 421 | 1.17149 | 0.58493 |
2 | 0.86713 | 1.51131 | 14.25768 | 536 | 0.51987 | 0.61437 |
Distance (±) deg | slope | intercept | RMSE (SD) | N | Bias | r |
0 | 1.001 | −0.20677 | 1.90726 | 276 | −0.2 | 0.99846 |
−0.05 | 0.997 | −0.456 | 1.84 | 276 | −0.4 | 0.99855 |
−0.1 | 0.999 | −0.30995 | 1.87084 | 276 | −0.3 | 0.99851 |
−0.15 | 0.998 | −0.38977 | 1.81382 | 276 | −0.3 | 0.9986 |
−0.2 | 0.994 | −0.61181 | 1.7414 | 276 | −0.5 | 0.9987 |
−0.25 | 0.997 | −0.47134 | 1.77265 | 276 | −0.4 | 0.99868 |
0.05 | 0.999 | −0.35961 | 1.93632 | 276 | −0.3 | 0.99841 |
0.1 | 0.998 | −0.41297 | 1.94912 | 276 | −0.4 | 0.99838 |
0.15 | 0.997 | −0.43234 | 1.88191 | 276 | −0.4 | 0.99849 |
0.2 | 0.997 | −0.41859 | 1.86106 | 276 | −0.3 | 0.99852 |
0.25 | 0.996 | −0.41439 | 1.85309 | 276 | −0.3 | 0.99853 |
Time (hr) | ||||||
0.5 | 1.001 | −0.20677 | 1.90726 | 276 | −0.2 | 0.99846 |
1 | 0.999 | −0.30147 | 1.86207 | 279 | −0.3 | 0.99847 |
1.5 | 0.993 | −0.5528 | 1.91093 | 421 | −0.3 | 0.9982 |
2 | 0.991 | −0.67313 | 1.92643 | 536 | −0.4 | 0.99811 |
Distance (±) deg | slope | intercept | RMSE (SD) | N | Bias | r |
0 | 0.99262 | 0.15803 | 9.76068 | 276 | 0.06 | 0.77047 |
−0.05 | 1.03651 | −0.25206 | 8.80229 | 276 | 0.23312 | 0.81364 |
−0.1 | 1.01596 | 0.17311 | 9.6484 | 276 | 0.38517 | 0.78122 |
−0.15 | 1.01746 | 0.14665 | 9.75326 | 276 | 0.37867 | 0.77837 |
−0.2 | 1.01429 | 0.35328 | 9.89702 | 276 | 0.54316 | 0.77288 |
−0.25 | 1.00325 | 0.37309 | 9.82383 | 276 | 0.41661 | 0.77095 |
0.05 | 1.01799 | 0.0117 | 9.61059 | 276 | 0.25078 | 0.78302 |
0.1 | 1.01444 | 0.04735 | 9.41379 | 276 | 0.23921 | 0.78819 |
0.15 | 1.01322 | −0.03403 | 9.23815 | 276 | 0.14159 | 0.79342 |
0.2 | 1.01153 | −0.12006 | 8.85482 | 276 | 0.03313 | 0.80517 |
0.25 | 1.00965 | 0.074 | 9.31834 | 276 | 0.20221 | 0.78982 |
Time (hr) | ||||||
0.5 | 0.99262 | 0.15803 | 9.76068 | 276 | 0.06 | 0.77047 |
1 | 0.74143 | 5.4424 | 13.39716 | 279 | 1.23112 | 0.59909 |
1.5 | 0.70612 | 3.288 | 12.31716 | 421 | 1.17149 | 0.58493 |
2 | 0.86713 | 1.51131 | 14.25768 | 536 | 0.51987 | 0.61437 |