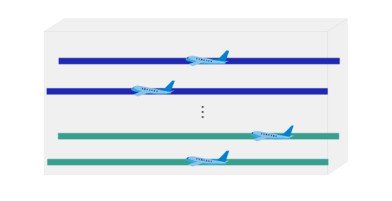
To efficiently handle the projected growth in air traffic, operational concepts such as "corridors-in-the-sky" have been developed in future Air Traffic Management (ATM) plans. Corridors-in-the-sky are defined as reserved airspace where aircraft move in a common direction along parallel lanes on high flight levels. The on and off ramps play an important role in connecting this airspace structure to the jet route. This paper addresses the scheduling problem for flight sequences entering the corridors-in-the-sky via the on-ramp. To satisfy the requirements of various stakeholders, this paper proposes time-based and cost-based aircraft scheduling optimization models for the on-ramp of the corridors-in-the-sky. An improved Particle Swarm Optimization (PSO) algorithm is also developed to solve a case study of the air route B215 in the Beijing control area. Computational results indicate that the optimized models have advantages in reducing the delay time and cost for both single aircraft and flight sequences, compared with the First-come, First-served (FCFS) approach.
Citation: Jie Ren, Shiru Qu, Lili Wang, Lijing Ma, Tingting Lu. Aircraft scheduling optimization model for on-ramp of corridors-in-the-sky[J]. Electronic Research Archive, 2023, 31(6): 3625-3648. doi: 10.3934/era.2023184
[1] | Zhizhou Zhang, Zhenglei Wei, Bowen Nie, Yang Li . Discontinuous maneuver trajectory prediction based on HOA-GRU method for the UAVs. Electronic Research Archive, 2022, 30(8): 3111-3129. doi: 10.3934/era.2022158 |
[2] | Ming Wei, Shaopeng Zhang, Bo Sun . Airport passenger flow, urban development and nearby airport capacity dynamic correlation: 2006-2019 time-series data analysis for Tianjin city, China. Electronic Research Archive, 2022, 30(12): 4447-4468. doi: 10.3934/era.2022226 |
[3] | Chuanyao Li, Yichao Lu, Yuqiang Wang, Gege Jiang . Congestion behavior and tolling strategies in a bottleneck model with exponential scheduling preference. Electronic Research Archive, 2023, 31(2): 1065-1088. doi: 10.3934/era.2023053 |
[4] | Jie Ren, Shiru Qu, Lili Wang, Yu Wang, Tingting Lu, Lijing Ma . Research on en route capacity evaluation model based on aircraft trajectory data. Electronic Research Archive, 2023, 31(3): 1673-1690. doi: 10.3934/era.2023087 |
[5] | Nishui Cai, Guofeng He . Multi-cloud resource scheduling intelligent system with endogenous security. Electronic Research Archive, 2024, 32(2): 1380-1405. doi: 10.3934/era.2024064 |
[6] | Ming Wei, Congxin Yang, Bo Sun, Binbin Jing . A multi-objective optimization model for green demand responsive airport shuttle scheduling with a stop location problem. Electronic Research Archive, 2023, 31(10): 6363-6383. doi: 10.3934/era.2023322 |
[7] | Shuguang Li, Yong Sun, Muhammad Ijaz Khan . Single machine Pareto scheduling with positional deadlines and agreeable release and processing times. Electronic Research Archive, 2023, 31(5): 3050-3063. doi: 10.3934/era.2023154 |
[8] | Dike Hu, Hua Wang, Zijie Huang, Wangqiang Xiao . Research on impact reduction of flexible boundary particle damping honeycomb plate based on discrete element multi body dynamics coupling. Electronic Research Archive, 2023, 31(10): 6303-6326. doi: 10.3934/era.2023319 |
[9] | Gang Cheng, Yijie He . Enhancing passenger comfort and operator efficiency through multi-objective bus timetable optimization. Electronic Research Archive, 2024, 32(1): 565-583. doi: 10.3934/era.2024028 |
[10] | Jingqian Xu, Ma Zhu, Baojun Qi, Jiangshan Li, Chunfang Yang . AENet: attention efficient network for cross-view image geo-localization. Electronic Research Archive, 2023, 31(7): 4119-4138. doi: 10.3934/era.2023210 |
To efficiently handle the projected growth in air traffic, operational concepts such as "corridors-in-the-sky" have been developed in future Air Traffic Management (ATM) plans. Corridors-in-the-sky are defined as reserved airspace where aircraft move in a common direction along parallel lanes on high flight levels. The on and off ramps play an important role in connecting this airspace structure to the jet route. This paper addresses the scheduling problem for flight sequences entering the corridors-in-the-sky via the on-ramp. To satisfy the requirements of various stakeholders, this paper proposes time-based and cost-based aircraft scheduling optimization models for the on-ramp of the corridors-in-the-sky. An improved Particle Swarm Optimization (PSO) algorithm is also developed to solve a case study of the air route B215 in the Beijing control area. Computational results indicate that the optimized models have advantages in reducing the delay time and cost for both single aircraft and flight sequences, compared with the First-come, First-served (FCFS) approach.
The Aviation Industry Corporation of China has predicted a significant increase in air transport demand, with projected Revenue Passenger Kilometers (RPK) set to reach 3.3 trillion passenger kilometers by 2039. This represents a substantial 175% increase from 2019 levels, with an annual growth rate of 5.3% [1]. However, this growth will place immense pressure on the Air Traffic Management (ATM) system, which may struggle to meet the demand-capacity imbalance, resulting in safety and congestion issues. To address these challenges, there is a pressing need to enhance the performance of the ATM system, particularly in the areas of airspace capacity and efficiency, such as the Terminal Maneuvering Area (TMA) and Area Control Center (ACC) [2]. Despite efforts to optimize the route network structure, en route traffic congestion in the ACC remains a significant challenge, with aircraft still limited to flying routes defined by ground-based navigation aids, resulting in inflexible and suboptimal use of available airspace [3]. In recent academic literature, optimizing the route network structure has become a crucial issue. In addition, advances in Communication Navigation and Surveillance (CNS) technologies, such as Airborne Separation Assurance System (ASAS), Automated Dependent Surveillance Broadcasting (ADS-B), and Cockpit Display of Traffic Information (CDTI), have revolutionized operating conditions for Air Traffic Controllers (ATCOs) and enabled pilots to self-separate, providing greater flexibility for airspace designers to implement new airspace classes capable of accommodating substantially higher traffic volumes than current airspace structures [4].
To ensure safe and efficient operation in airspace, Corridors-in-the-Sky, also known as Tubes, have been proposed to accommodate increasing and fluctuating traffic volumes, with designs based on traffic demand. The concept of Corridors-in-the-Sky originated from the Dynamic Airspace Super Sector (DASS) proposed by George Mason University in 2003. The DASS system is defined as a structured network of elongated sectors, which can be imagined as thin ribbons of airspace, stretching over the United States and connecting major airports [5]. Since then, several other concepts, such as the Dynamic Multi-Track Airway (DMA), Worm, Flow Corridor, and Bubble, have been proposed to increase airspace capacity and efficiency. The DMA concept proposes a network of high-altitude airways constructed of multiple, closely spaced, parallel tracks to increase en-route capacity. Similarly, the Worm is a concept composed of a sequence of flights moving in trail, also described as a train or follow-the-leader concept [6]. The Flow Corridor is developed as a new type of flexible tube-shaped airspace to better accommodate high levels of traffic for future air transportation systems, with characteristics such as multiple lanes of traffic, self-separated aircraft flying within the lanes, and dynamic deployment [7]. Additionally, the Bubble is proposed as a reserved airspace surrounding an aircraft as it moves, to increase the flexibility of airspace [8].
Regardless of the design concepts, corridors-in-the-sky are regions of airspace that typically consist of long and narrow pathways connecting high-demand airports and cities, in which aircraft move in a common direction or trajectory along parallel lanes. This design is expected to increase both the efficiency and throughput of air traffic while maintaining operational safety. Corridors-in-the-sky allow for effective handling of mixed air traffic, where some aircraft are capable of self-separation and others are not [9]. Figure 1 shows the main characteristics of corridors-in-the-sky [4]:
● Multiple horizontally parallel lanes of traffic are allowed.
● Vertically, aircraft on the corridors-in-the-sky follow Reduced Vertical Separation Minima (RVSM) or other requirements to fulfill safety requirements.
● Advanced CNS technology allows for changes in methods of separation, such as self-separation, which potentially reduces separation standards within the corridor, or enables flying in formation.
● Dynamic activation rules allow for the addition or removal of corridor structures as needed throughout the day. While corridors do not have to be restricted to high altitudes, they are most naturally suited for high-altitude airspace.
To ensure operational efficiency, corridors-in-the-sky are typically designed as relatively closed airspaces, with ramps connecting them to jet routes. Aircraft enter or exit the corridors-in-the-sky via on-ramps or off-ramps. The basic structure of corridors-in-the-sky is displayed in Figure 2.
The practical use of corridors-in-the-sky presents many challenges that need to be addressed. Although the preliminary concepts and geometry of corridors-in-the-sky have been extensively studied, existing research often overlooks the operational challenges of the ramps. In this study, our primary objective is to present two aircraft scheduling optimization models for the on-ramp of corridors-in-the-sky. Our goal is to reduce delay costs and improve the airspace capacity. We make the following contributions: (i) the development of an aircraft scheduling model for the on-ramp of corridors-in-the-sky that minimizes the total delayed time of the flight sequence, (ii) the development of an aircraft scheduling model for the on-ramp of corridors-in-the-sky that minimizes the delayed economic cost of the flight sequence based on different types of aircraft, and (iii) the development of an improved particle swarm optimization algorithm to increase resolution efficiency. To validate our proposed approaches, we conduct a case study and compare the results of our two models with a scheduling solution based on the First-come, First-served (FCFS) strategy.
The remainder of this paper is organized as follows: Section 2 discusses previous related work and describes the contributions of our research. Sections 3 and 4 presents our two proposed models as well as the solution approaches, while Section 5 describes the results of the application of these models. Finally, Section 6 summarizes our research conclusions and provides directions for future research.
The literature pertinent to our work primarily focuses on two aspects. Firstly, the focus of our study is the concept of corridors-in-the-sky, which is a new and innovative airspace management concept. Secondly, our problem is primarily an Aircraft Sequencing and Scheduling Problem (ASSP).
In order to address the increasing demands of air traffic, the concept of corridors-in-the-sky has been investigated as a promising approach to decreasing airspace complexity, enhancing capacity, and mitigating delays. Since the introduction of this concept, numerous studies have been conducted to design and evaluate corridors or corridor networks. Researchers from both domestic and international settings have predominantly focused on the operational concepts, structural design, and operational benefits of corridors-in-the-sky.
Regarding the operational concepts of the corridors-in-the-sky, R. Hoffman et al. have made significant contributions by developing and enhancing the current understanding of tube design options and their tradeoffs. They have created a comprehensive list of design issues and some potential alternatives, such as changing lanes/altitude, entering/exiting flow corridors at various points, and crossing of two flow corridors, etc. Furthermore, they conducted some preliminary analysis to gain insights into design issues and principles, and to estimate the capacity-enhancing effects of corridors-in-the-sky for the airspace [10]. Additionally, H. Horst proposed a dual airspace concept to split air traffic in dense traffic areas based on ATCO issues. The resulting airspace consists of a district airspace (similar to the current airspace) and a new Freeway airspace. The Freeways are independent, isolated airspace at high altitude with special rules. There are two proposed Freeways for Europe which follow the main traffic flows in the region and they will absorb significant portions of Core-Europe's intercontinental traffic and some of Europe's long-haul domestic traffic [11].
Structural design of corridors-in-the-sky has been approached through numerical modeling methodologies. A. Yousefi et al. proposed a flow-based modeling approach that uses a set of user-preferred, wind optimal, and unconstrained 4D trajectories (4DT) to allocate corridors-in-the-sky. The authors optimized corridors' coordinates dynamically based on changes in preferred trajectories, resulting in a NAS-wide corridor network that mimics the dynamics of user-preferred trajectories [4]. J. Chen presented the Flow Corridor-based Traffic Management Initiative (FC-TMI), which forms and dissolves flow corridors based on demand, traffic patterns, dynamic weather, and the need to resolve demand-capacity imbalances. The FC-TMI provides structure only when necessary without unnecessarily constraining traffic. It was found that FC-TMI reduced delays (~5–13%) while using a small number of flow corridors (15–27), with only ~300–600 aircraft in the flow corridors [3]. B. Sridhar et al. initiated the concept of a tube network connecting local regions around major airports in the U.S. The authors determined the impacted traffic by a simple tube network via simulation, revealing that connecting local regions captured 82% of flights while connecting only the top 25 airports captured only 17%. The authors concluded that tubes should interconnect airports in clusters seeded by major airports instead of interconnecting just the major airports [12]. C. Han et al. proposed a new tube design methodology that considered flight trajectory clustering to build a tube airspace in China. The authors used the MAKLINK graph and Genetic Algorithm to find conflict-free tube center lines to avoid special use airspace. The results showed that ten tubes would accommodate more than 40% of the total flow and minimize the average extra distance. The authors concluded that building tubes upon airlines rather than major airports is important [13].
The benefits of implementing corridors-in-the-sky can be viewed from several operational perspectives, including a reduction in flight delays, air traffic controller workload, and fuel consumption. N. Takeichi et al. conducted a study analyzing the average fuel consumption and flight time of different aircraft types, using actual flight data based on the optimum Flow Corridor allocation in Japan. They found that the introduction of Flow Corridors resulted in reduced flight time and fuel consumption, with a significant decrease in fuel consumption observed in both the cruise and descent trajectories [14]. M. Xue et al. developed a corridor traffic simulation tool to examine traffic flow within the corridor. They proposed various lane options and operational policies to investigate their impact on complexity, using the number of conflict resolutions as the measure of complexity. The study revealed that heavy traffic could be managed with low complexity under appropriate operational policies and lane options [15]. Y. Morooka et al. proposed a layered air corridor consisting of several fixed altitude and Mach number cruise trajectories. By analyzing a set of constrained optimum trajectories, they calculated the Direct Operational Costs (DOCs) of the layered air corridor and found that it could reduce both DOCs and conflicts compared to conventional operations. [9]. B. Ye et al. proposed an optimal design method to minimize the total flying cost within flow corridors while considering environmental impacts such as contrail and CO2 emissions. Results showed that the optimized seven flow corridors covered 4.03% of flight demand in China and reduced the total flying cost by 3.19% [16]. Y. Zhang et al. developed a hybrid methodology that combined Monte Carlo simulation and dynamic event trees to estimate the collision risk of the flow corridor concept-of-operations. The study found that the collision risk was around 10–9 collisions per flight hour. The overall risk assessment captured the unique characteristics of the flow corridor concept, including self-separation within the corridor, lane change maneuvers, speed adjustments, and the automated separation assurance system [17].
Due to the runway being a primary bottleneck in the airport system, there has been a broad range of studies on ASSP for runways. Dear proposed the use of Position Shifting Constraints (PSC) in combination with the FCFS policy to optimize the arrival schedule [18]. This idea has since been built upon by several researchers, with variations depending on the number of runways, operation modes of the runway, objective functions (minimizing flight tardiness, cost, or maximizing capacity), and solution algorithms (exact and heuristic approaches). R. Prakash et al. presented a data-splitting algorithm to solve the ASSP on a single runway optimally, under both segregated and mixed-mode operations, while considering several realistic constraints such as safety separation standards, wide time-windows, and constrained position shifting, with the objective of maximizing total throughput [19]. A. Lieder et al. proposed a new algorithm capable of creating optimal landing schedules on multiple independent runways for aircraft with positive target landing times and limited time windows. Numerical experiments showed that problems with up to 100 aircraft could be optimally solved within seconds [20]. K. K. H. Ng et al. proposed a constructive heuristic using the artificial bee colony algorithm to resolve the aircraft landing problem, considering speed control for airborne delay and holding patterns in the near terminal area. The computational results showed that the proposed algorithm could resolve the problem in a reasonable amount of time for practical usage [21]. Additionally, J. A. Bennell et al. conducted an extensive literature survey on the problem of ASSP [22].
In summary, while previous research has made progress in conceptualizing and assessing the impact of corridors-in-the-sky, specific operational details, such as on/off ramp aircraft scheduling, remain understudied. Furthermore, there is a lack of studies on air traffic management scenarios in the terminal maneuvering area and en route, and many proposed models fail to address the needs of all stakeholders. To address these gaps, this paper proposes two aircraft scheduling models for on-ramp corridors-in-the-sky that take into account the interests of all stakeholders involved. By doing so, this paper seeks to contribute to the development of practical solutions for implementing corridors-in-the-sky and maximizing their benefits.
The process of using corridors-in-the-sky for aircraft involves several steps. First, the aircraft departs from the origin airport and heads directly to the conventional jet route. Then, it enters the corridors-in-the-sky through an on-ramp located at the boundary of the airspace and follows the designated route until it reaches the off-ramp. Finally, the aircraft exits the corridors-in-the-sky and returns to the jet route to land at the destination airport.
However, when an aircraft on the jet route approaches the on-ramp, it must maintain a safe distance from other aircraft operating within the corridors-in-the-sky. In such cases, ATCO may instruct the aircraft at the on-ramp to conduct a holding procedure at a designated holding fix. This maneuver keeps the aircraft within a specified airspace while awaiting clearance, ensuring a safe and orderly flow of traffic.
The holding procedure involves flying over the holding fix and then turning to fly outbound for a specified period of time. After completing the outbound leg, the aircraft turns onto the inbound track and intercepts the inbound track to return to the holding fix at the on-ramp of the corridors-in-the-sky. Figure 3 illustrates the holding procedure, with the black line representing the runway-shaped holding pattern and the red point A indicating the holding fix at the on-ramp of the corridors-in-the-sky.
In situations where multiple aircraft enter the corridors-in-the-sky simultaneously, ATCO may assign different holding altitudes to ensure vertical separation between the planes. Additionally, ATCO may instruct aircraft to conduct one or more holding procedures until they are cleared to join the corridors-in-the-sky.
To optimize the sequencing of on-ramp flights and reduce delays, this study proposes two models. The first model aims to minimize the total delayed time, while the second model focuses on minimizing the total economic cost of the delay.
The scheduling problem addressed in this paper involves static/off-line data, where all information related to the aircraft on the jet route preparing to join the corridors-in-the-sky and the aircraft on the corridors-in-the-sky is known in advance. Specifically, we assume that a certain number of aircraft n are on the corridors-in-the-sky passing by the on-ramp during the time period [0, T], and a certain number of aircraft m are on the jet route preparing to join the corridors-in-the-sky via the on-ramp. We use Set A=[T1,T2,…Tl,…,Tn] to represent the time when the aircraft operating on the corridors-in-the-sky arrives at the on-ramp, and Set B=[Ta1,Ta2,…,Tai,…,Taj,…,Tam] to denote the time when the aircraft on the jet route arrives at the holding fix of the on-ramp.
To optimize the scheduling of aircraft entering the corridors-in-the-sky via the on-ramp, we require certain information about the aircraft, such as their serial number, the transition time from the holding fix to the on-ramp, the required minimum safety time interval that the aircraft should maintain on the corridors-in-the-sky, the time for the aircraft to conduct a standard holding procedure, and the status bits of the aircraft used to confirm whether the aircraft is conducting a holding procedure or not.
In addition to the aircraft-specific data, we also need operational data associated with the economic characteristics of the airline and passengers, such as the fuel consumption cost per minute for different types of aircraft, passengers' average delay cost, the maximum passenger capacity of the aircraft, delay cost coefficient, flight occupancy rate, and other related information. By considering both the aircraft-specific and operational data, we aim to develop two aircraft scheduling optimization models that can minimize total delayed time and total economic cost of the delay, respectively.
Table 1 shows the notation and decision variables in these two models.
Notations | Explanation | Unit |
n | The number of aircraft on the corridors-in-the-sky | / |
m | The number of aircraft on the jet route | / |
Tl | The time when the aircraft l on the corridors-in-the-sky arrives at the on-ramp | / |
Tai | The time when the aircraft i on the jet route arrives at the holding fix of the on-ramp | / |
td | The required minimum safety time interval which the aircraft should maintain on the corridors-in-the-sky | Minute |
tg | The transition time of the aircraft from the holding fix to the on-ramp | Minute |
tw | The time for aircraft to conduct a standard holding procedure | Minute |
K | The maximum number of standard holding procedures | / |
C1 | The economic loss of airlines caused by delay | CNY |
Ci, fuel | The fuel consumption cost per minute of flight i | CNY/Minute |
C2 | The economic loss of passengers caused by delay | CNY |
Ci, pas | The average delay cost per person of flight i | CNY/Minute |
ri | The maximum passenger capacity of flight i | / |
qi | The delay cost coefficient of flight i | / |
τ | The flight occupancy rate | / |
Decision variables | Explanation | |
xi | Actual time of joining the corridors-in-the-sky of aircraft i | |
ki | The cumulative number of completing standard holding procedures for aircraft i | |
δij | 1, if aircraft i is scheduled to join the corridors-in-the-sky before aircraft j (i=1,…,m;j=1,…,m;i≠j) 0, otherwise |
The constraints for aircraft scheduling in the on-ramp of the corridors-in-the-sky problem can be expressed as follows:
|xi−Tl|⩾td | (1) |
δij+δji=1,(i=1,…,m;j=1,…,m;i<j) | (2) |
xj⩾xi+δijtd,(i=1,…,m;j=1,…,m;i≠j) | (3) |
xi−Tai=tg+kitw,∀i | (4) |
0≤ki≤K,ki∈Z | (5) |
Constraint (1) enforces a safety temporal interval between any aircraft on the corridors-in-the-sky l and those entering the corridors-in-the-sky from the jet route i. Constraint (2) ensures the order precedence relationship between flights i and j on the jet route. Constraint (3) guarantees the time-based separation requirements between two aircraft entering the corridors-in-the-sky from the jet route. Constraint (4) maintains the time required for an aircraft to enter the corridors-in-the-sky from the holding fix, which consists of both holding time and transition time. Lastly, constraint (5) specifies the minimum and maximum number of holding procedure rounds in integer values.
For ATCOs and airspace managers, aircraft occupancy time, delay time, and airspace utilization are the key factors they consider when sequencing aircraft. To enable the flight sequence to join the corridors-in-the-sky as quickly as possible and minimize the total delayed time of the sequence, we propose a time-based optimization model. The time-based objective function is established as follows:
minZ1=m∑i=1(xi−Tai) | (6) |
here, the objective function Z1 represents the total delayed time of the aircraft sequence joining the corridors-in-the-sky, measured in minutes.
While ATCOs and airspace managers focus on aircraft occupancy time, delay time, and airspace utilization, airlines prioritize the economic losses incurred by themselves and their passengers during aircraft sequencing. To address these concerns and better align with the operational environment, we have developed a cost-based model for on-ramp flight sequencing. The model aims to minimize both the airlines' Flight Operating Cost (FOC) and the economic loss suffered by passengers due to flight delays. By considering the interests of different stakeholders, this model provides a more balanced solution.
Air transportation is a complex system that involves several stakeholders such as airlines, passengers, airports, and air traffic control facilities. In this paper, our focus is on the economic losses incurred by airlines and passengers due to flight delays, as they are the most significant indirect losses in air transportation. The indirect losses to airports and air traffic control facilities are challenging to estimate accurately and are therefore not included in this study.
Flight delays can significantly increase the FOC of airlines, resulting in economic losses. The primary costs associated with airline operations during delays include fuel consumption, landing fees, meals, and baggage services. Among these costs, fuel consumption represents a significant proportion, and therefore, we only consider it when calculating the economic losses incurred by airlines due to delays. Moreover, the cost of fuel consumption is directly proportional to the category of the delayed aircraft, determined by its maximum certificated take-off mass or wake turbulence category, as defined by the International Civil Aviation Organization (ICAO) DOC 4444 'Air Traffic Management'. Specifically, the cost of an aircraft delay increases with its takeoff weight [24]. Table 2 in this paper presents the cost of airline delays due to on-ramp sequencing.
Wake turbulence category | Symbol | Maximum takeoff weight (ton) | Operation cost (CNY/minute) |
Heavy | H | > 136 | 468 |
Medium | M | 7-136 | 258 |
Light | L | ≤ 7 | 24 |
The calculation of FOC due to fuel consumption for delayed aircraft is illustrated in this section using three examples: Category-H (CAT-H), Category-M (CAT-M), and Category-L (CAT-L) aircraft. For CAT-H aircraft, we take the A330-200 as an example. The average hourly fuel consumption for this type of aircraft is 4700 kg, and the average price of fuel is around 5700 CNY/ton. Therefore, the average FOC for a CAT-H aircraft can be calculated to be 468 CNY per minute. For CAT-M aircraft, we consider the A320-200 as an example, with an FOC of 258 CNY per minute. Lastly, for CAT-L aircraft, we use the DHC-6 as an example, with an FOC of 24 CNY per minute. By calculating the FOC for different aircraft categories, we can estimate the economic losses of airlines caused by flight delays during on-ramp sequencing.
Using these FOC values, we can calculate the economic losses incurred by airlines due to delayed on-ramp sequencing for the entire flight sequence, as follows:
C1=∑mi=1Ci,fuelti | (7) |
where ti is the time during which the aircraft is held in a holding pattern due to delayed on-ramp sequencing.
Air transportation is highly valued for its ability to provide timely travel, but flight delays can result in economic losses for passengers, which are measured in terms of increased travel time costs. The cost of travel time can vary depending on several factors, such as the type of travel mode and the purpose of travel. In this research article, we aim to examine the economic losses incurred by passengers due to flight delays from two perspectives: the type of flight and whether it is a connecting flight.
To accomplish this goal, we will consider three types of flights: domestic scheduled flights, international scheduled flights, and chartered flights. According to a study [25], the average delay cost per passenger for domestic scheduled flights is around 1 CNY/minute, while for international scheduled flights and chartered flights, it is approximately 2 CNY/minute.
Additionally, we will examine the impact of delay on connecting flights, which can cause a domino effect of delays. To account for this, we introduce a delay cost coefficient for connecting flights.
Overall, the economic losses incurred by passengers due to delay are as follows:
C2=∑mi=1Ci,pasriqiτti | (8) |
here, qi represents the delay cost coefficient, with qi = 2 for connecting flights and qi = 1 for non-connecting flights in this study. τ represents the flight occupancy rate, which is typically set at 75% in practice [26].
Based on the preceding discussion, an optimization model is presented to minimize the total delayed economic cost of flight sequences entering corridors-in-the-sky. The objective function of this cost-based model can be optimized using the following equation:
minZ2=∑mi=1(Ci,fuel+Ci,pasriqiτ)(xi−Tai) | (9) |
here, Ci,fuel+Ci,pasriqiτ represents the delay cost per minute of flight i, and the objective function Z2 refers to the total delayed cost of the aircraft sequence joining the corridors-in-the-sky in CNY. Equation (9) assumes that the delay cost of an aircraft due to the execution of holding procedures is linearly related to the delay time of the flight. Therefore, the priority of flights entering the on-ramp can be determined to optimize the release order and minimize the total delay cost of the entire flight sequence.
Before formulating the two on-ramp flight sequencing optimization models, several assumptions were made. These assumptions include:
● Outbound timing for holding procedures is based on the holding altitude and assumes a standard rate of turn of 3 degrees per second. It is assumed that the total time required to complete one holding procedure is tw = 5 minutes, with the outbound timing set to one and a half minutes for aircraft above 4250 m (14,000 ft) [27].
● Aircraft will not be disrupted to perform other maneuvers before completing the entire holding procedure.
● ATCO will assign different holding altitudes to maintain vertical separation when multiple aircraft are in the holding procedure, ensuring that the aircraft in the holding area do not interfere with each other.
● After completing the holding procedure, it is assumed that aircraft will take tg=1 minutes to fly from the holding fix to the on-ramp of the corridors-in-the-sky, with negligible differences in aircraft airspeed.
● All aircraft on the corridors-in-the-sky share the same flight level, meaning that they only need to maintain lateral separation.
● The longitudinal separation of aircraft on the corridors-in-the-sky is converted into a time interval of td=1 minutes, based on the requirement of a distance interval of 10 km for jet routes.
These assumptions provide the basis for the two on-ramp flight sequencing optimization models, which seek to improve the efficiency and safety of air traffic flow by optimizing the sequencing of aircraft entering the corridors-in-the-sky.
It is indeed true that metaheuristic algorithms, such as Genetic Algorithm (GA), Simulated Annealing (SA), and Particle Swarm Optimization (PSO), are commonly employed to solve static problems associated with aircraft scheduling. These algorithms are popular because they provide fast and satisfactory results. PSO, in particular, has gained significant popularity due to its simplicity, rapid convergence, and adaptability to complex problems.
In practice, ATCOs frequently use the FCFS strategy in aircraft sequencing and scheduling. However, the FCFS scheme has a significant disadvantage in that a delay in a single aircraft can create a ripple effect, resulting in increased delays among the entire flight sequence. Therefore, this study proposes two optimization models that are compared with the FCFS strategy to demonstrate their advantages in reducing delays and improving overall efficiency in aircraft scheduling. The use of metaheuristic algorithms such as PSO is particularly beneficial in finding optimal solutions to these models quickly and effectively.
The PSO algorithm, first developed by Kennedy and Eberhart, is a stochastic search algorithm that simulates the foraging behavior of bird flocks [28]. In PSO, each optimization problem solution is represented by a "particle" that moves around in the search space. The particle's position corresponds to the solution of the problem being solved, and its adaptation value is determined by the function being optimized. Each particle has a velocity that determines its direction and distance of travel.
At each iteration, particles update their positions and velocities based on two current extremes: their own best-known position (individual extremes Pid) and the swarm's best-known position (global extremes Pgd). This allows the particles to follow the current optimal particle in the solution space. The optimal solution searched by the particle itself is denoted by Pid, while Pgd represents the optimal solution searched by the particle population. The velocity and position updating rules are expressed by Eqs (10)–(12) as follows:
Vt+1id=ωVtid+η1 rand ()(Ptid−Xtid)+η2 rand ()(Ptid−Xtid) | (10) |
Pt+1i={Xt+1i,F(Xt+1i)≥F(Pti)Pti,F(Xt+1i)<F(Pti) | (11) |
Pt+1g={Pt+1i,F(Pt+1i)≥F(Ptg)Ptg,F(Pt+1i)<F(Ptg) | (12) |
where Vid and Xid are the velocity and position of particle i in dimension d, η1 and η2 are the learning parameters of the particle swarm, rand() is a random number uniformly distributed in the range [0,1] thus increasing the randomness for each part of the inheritance degree, and ω is the inertia weight.
Figure 4 shows the process flowchart of the basic PSO algorithm.
Although PSO has traditionally been used to solve continuous problems, it is now being increasingly applied to solve discrete problems as well. The basic idea of the discrete particle swarm optimization algorithm is to enable the continuous exchange of information between particles and the population, in order to facilitate the movement of the population towards a better location. However, optimization using the discrete particle swarm algorithm often results in premature convergence and suboptimal solutions, as particles can quickly fly towards the region of the best particles in the population.
To address this issue, we propose an improved PSO algorithm that is specifically designed for solving discrete problems. The details of this algorithm are described below.
PSO is known to be better suited for solving continuous problems. As a result, we approximate the rescheduled time xi for aircraft i to join the corridors-in-the-sky as a continuous real number for operational purposes, followed by rounding operations to obtain discrete values. In PSO, each particle ith is coded as Xi=(xi1,xi2,...,xij...,xim) (i=1,…,p;j=1,…,m), where p denotes the population size of PSO, and m denotes the number of aircraft entering the corridors-in-the-sky on the jet route. These particles must satisfy the constraints defined by Eqs (4) and (5).
Before solving the objective function value, we first need to decode Xi. This process involves arranging xi1,xi2,…,xim in ascending order as a new sequence xib(1),xib(2),…,xib(m) to satisfy Eq (2), followed by applying the FCFS strategy to allocate the time of entry into the corridors-in-the-sky for xib(1),xib(2),…,xib(m), satisfying Eqs (1) and (3). Finally, we substitute the decoded values of Xi back into Eqs (6) or (9) to obtain the objective function value. With this encoding and decoding process, PSO is capable of solving the two mixed-integer programming models proposed in this paper.
The efficiency of the discrete particle swarm optimization algorithm heavily depends on the size of the particle swarm. If the population size is too small, the diversity of the population decreases, making it easy to fall into local optimization. To address this issue, we conducted several experiments to determine the optimal size of the particle swarm for the aircraft scheduling optimization for on-ramp of the corridors-in-the-sky. Our findings reveal that a particle swarm size of 20 meets the required efficiency for the optimization process.
The improved discrete particle swarm algorithm incorporates the SA-based local search mechanism for better search ability in local space. Additionally, the K-OPT method is employed to hasten the convergence speed of solving combinatorial optimization problems. The following steps are taken in implementing the SA-based local search incorporating the K-OPT idea:
● Determine the initial temperature T0, termination temperature Tter, initial solution S0, chain length L, and cooling rate ξ.
● Apply the K-OPT strategy to generate a new solution S1.
● Calculate the increment of S1, Δt=f(S1)−f(S0), where f(Si) is the evaluation function.
● Apply the Metropolis criterion to determine whether to assign the new solution or maintain the current solution. If Δt<0, assign S1 to the current solution; Otherwise, if exp(−Δ/T)>rand(), S1 is assigned to the current solution, otherwise the current solution remains unchanged.
● If the termination conditions are satisfied, output the current solution as the optimal solution, otherwise, update the temperature and repeat the steps.
By incorporating the SA-based local search mechanism with the K-OPT idea, the improved algorithm achieves better performance in comprehensively solving combinatorial optimization problems.
The fitness function is used to evaluate the position of particles in particle swarm optimization. By obtaining the evaluation function value of each particle, we can guide the particle swarm to fly towards the optimal solution.
In the context of aircraft sequencing at the on-ramp of the corridors-in-the-sky, the objective is to determine the order and entering time for each aircraft to minimize the total delayed time or delayed cost. To achieve this objective, two fitness functions are proposed: a time-based objective function and a cost-based objective function.
For the time-based aircraft scheduling optimization model, the fitness function is formulated as follows:
f1(x1,x2,…,xm)=∑mi=1(xi−Tai) | (13) |
The objective function is expressed as:
min(f1(X)) | (14) |
For the cost-based aircraft scheduling optimization model, the fitness function is defined as follow:
f2(x1,x2,…,xm)=∑mi=1(Ci,fuel+Ci,pasriqiτ)(xi−Tai) | (15) |
The objective function is denoted as:
min(f2(X)) | (16) |
Overall, the fitness functions play a critical role in guiding particle swarm optimization towards the optimal solution in aircraft sequencing problems.
The FCFS approach determines the precedence between aircraft that enter the corridors-in-the-sky in sequence [29]. Therefore, the actual times at which aircraft enter the corridors-in-the-sky are obtained by applying constraints (1)–(4) directly.
As there are no implemented corridors-in-the-sky in China, a section of air route B215 from IDGIS to SUGDO in the Beijing control area was selected as an alternative route for this study. The route was divided into three sections: the jet route (blue) from waypoint NOMOV to APEXU, the on-ramp of the corridors-in-the-sky (green) from waypoint APEXU to VAGBI, and the corridors-in-the-sky (red) from waypoint IDGIS to SUGDO. Additionally, if there are more aircraft, they may need to conduct a holding procedure (yellow) before entering the on-ramp of the corridors-in-the-sky, and the waypoint APEXU is considered a holding fix. For a better understanding, please refer to Figure 5 for a structural representation of the selected route in this case study.
The actual flight data used in our models was sourced from the ATC automation system and consists of ATC radar data updated every four seconds over a period between 10:00 A.M. to 12:00 A.M. on February 24th, 2019. The data was parsed and transcribed into a readable and manipulable txt format. Figure 6 provides an illustration of a sample data header and part of the trajectory data for one aircraft. The data header includes critical information such as the track number, aircraft call sign, and the number of recorded trajectory points. Each line of trajectory data represents the flight time, longitude, latitude, altitude, airspeed, and magnetic heading of the aircraft from left to right.
Based on the actual flight data, we identified 24 aircraft on the corridors-in-the-sky from waypoint IDGIS to SUGDO, and their passing times through the on-ramp point VAGBI are listed in Table 3. In addition, we found 12 aircraft on the jet route from waypoint NOMOV to APEXU, which were scheduled to join the corridors-in-the-sky. The relevant operational information discussed in Section 3.3.4 and estimated times of arrival for the aircraft on the jet route at the holding fix APEXU are given in Table 4. Furthermore, we assumed that the FCFS sequence serves as an initial order to join the corridors-in-the-sky.
S.N. | 1 | 2 | 3 | 4 | 5 | 6 | 7 | 8 |
Time at the ramp | 10:00 | 10:02 | 10:08 | 10:09 | 10:15 | 10:19 | 10:24 | 10:30 |
S.N. | 9 | 10 | 11 | 12 | 13 | 14 | 15 | 16 |
Time at the ramp | 10:31 | 10:35 | 10:39 | 10:40 | 10:44 | 10:56 | 10:57 | 11:07 |
S.N. | 17 | 18 | 19 | 20 | 21 | 22 | 23 | 24 |
Time at the ramp | 11:18 | 11:19 | 11:20 | 11:23 | 11:26 | 11:30 | 11:34 | 11:40 |
S.N. | Type | Wake turbulence category | Type of flight | Maximum passenger capacity | Estimated time when aircraft arrive at holding fix | Connecting flight |
1 | B737-700 | M | Scheduled-domestic | 130 | 10:01 | Y |
2 | A330-200 | H | Scheduled-domestic | 237 | 10:02 | N |
3 | B757-200 | H | Chartered | 200 | 10:12 | N |
4 | A320-200 | M | Scheduled-domestic | 179 | 10:14 | Y |
5 | B747-400 | H | Scheduled-domestic | 400 | 10:16 | N |
6 | B747-400 | H | Scheduled-international | 400 | 10:18 | N |
7 | B767-200 | H | Scheduled-international | 214 | 10:22 | Y |
8 | A319 | M | Scheduled-domestic | 124 | 10:24 | Y |
9 | A340-200 | H | Scheduled-international | 375 | 10:32 | N |
10 | B737-200 | M | Scheduled-domestic | 128 | 10:43 | Y |
11 | B737-700 | M | Scheduled-domestic | 130 | 10:47 | N |
12 | A310-200 | H | Scheduled-domestic | 228 | 10:49 | Y |
The computational experiments were conducted on a Windows 10 machine equipped with an Intel(R) Core(TM) i7-7820HQ CPU 2.90 GHz and 32 GB RAM processor, using MATLAB R2017a to code all the algorithms. The aim of this study is to explore the performance of the time-based optimization model and cost-based optimization model proposed in this paper, relative to FCFS, for the static aircraft scheduling problem on the on-ramp of the corridors-in-the-sky. Key metrics, including delayed time for each aircraft, cumulative delayed time for the flight sequence, delayed cost for each aircraft, and cumulative delayed cost for the flight sequence, were calculated and compared in the case study. To ensure that the experiments reflected actual operations as closely as possible, we not only used the actual data presented in Section 5.1 but also two sets of generated data that incorporated the characteristics and patterns of the actual data. By doing so, we were able to evaluate the superiority of the proposed model under realistic scenarios.
The results shown in Figure 7 clearly demonstrate the effectiveness of the two proposed optimization models and the FCFS approach in reducing delay time. Under the FCFS approach, the maximum delay time for one aircraft was 55 minutes, which led to a total delay time of 481 minutes for the entire flight sequence. On the other hand, both the time-based optimization model and the cost-based optimization model achieved a much lower maximum delay time of 23 minutes and 29 minutes, respectively, for one aircraft. As a result, the total delay time for the entire flight sequence was reduced to only 60 minutes for both optimization models, indicating a significant improvement in performance compared to the FCFS approach. Furthermore, the two proposed optimization models showed similar performance in reducing delay time.
In Figure 8, the delay costs of each aircraft and flight sequence are illustrated, and once again, the superiority of the optimization models over the FCFS approach is evident. Specifically, the FCFS approach resulted in a maximum delayed cost of 783 CNY for one aircraft, leading to a total delayed cost of 5825 CNY for the entire flight sequence. In contrast, both the time-based optimization model and the cost-based optimization model achieved significantly lower delayed costs, with a maximum delayed cost of 425 CNY and 371 CNY for one aircraft, respectively. As a result, the total delayed cost for the entire flight sequence was substantially reduced to 837 CNY and 735 CNY for the two models, respectively. These results further highlight the significant reduction in delay costs achieved using the optimization models compared to the FCFS approach, with the cost-based optimization model showing a slight advantage over the time-based optimization model in terms of the total cost of the flight sequence.
To further validate the feasibility and superiority of the optimization models, two sets of data were generated by mimicking the actual operational data of the Beijing control area.
In the first set of data, the number of aircraft from the jet route was twice that of the aircraft on the corridors-in-the-sky, leading to 24 aircraft on the jet route joining the corridors-in-the-sky where 12 aircraft were flying in two hours. The results presented in Figures 9 and 10 show that the optimization models still outperformed the FCFS approach, although the difference was not as obvious as in the previous scenario. Compared to the previous case, the maximum delayed time and cost for one aircraft, and the total delayed time and cost for the flight sequence were greatly reduced due to the change in the percentage of aircraft on the jet route and the corridors-in-the-sky. For the FCFS strategy, the time-based optimization model and the cost-based optimization model had runtimes of 0.7 seconds, 460 seconds, and 486 seconds, respectively.
In the second set of data, the number of aircraft on the jet route and the corridors-in-the-sky were doubled compared to the original scenario. This led to 24 aircraft on the jet route joining the corridors-in-the-sky where 48 aircraft were flying in four hours. The results associated with this set of data are presented in Figures 11 and 12. It is apparent that, for a particular flight, the time-based and cost-based optimization models did not demonstrate significant advantages and were even worse than the FCFS strategy. However, for the entire flight sequence, these two models still performed far better than FCFS in terms of delay time and cost. Furthermore, for the FCFS strategy, the time-based optimization model and the cost-based optimization model had runtimes of 0.7 seconds, 514 seconds, and 569 seconds, respectively.
In recent years, the concept of "corridors-in-the-sky" has gained popularity among scholars as a solution to the problem of airspace resource scarcity. This innovative air route structure has several advantages, including ensuring safe and efficient aircraft operation, improving airspace utilization, and increasing air traffic density. The ability of an aircraft to join the corridors-in-the-sky through the on-ramp in a reasonable and smooth manner is crucial in determining the extent of these advantages. Therefore, studying aircraft scheduling for the on-ramp of the corridors-in-the-sky is particularly important.
In this paper, we propose two aircraft scheduling models for the on-ramp of the corridors-in-the-sky with the objective of minimizing the delay time and delay cost of the flight sequence. Considering the safety separation requirement in reality, the priority of the aircraft to join the corridors-in-the-sky is determined based on a comparison of the operational cost of different categories of aircraft on both the corridors-in-the-sky and the jet route. Our case study demonstrates that the applied optimization models outperform the FCFS strategy in dealing with aircraft scheduling problem scenarios. The results show that this method can significantly reduce the cumulative delay cost of a single aircraft as well as the flight sequence, maximize the advantages of the corridors-in-the-sky, and ensure the operational efficiency of airlines. However, compared with FCFS, the main limitation of the optimization models proposed in this paper is their longer running time, which only allows for solving offline problems of flight sequencing.
Regarding the possibility of implementing these models in real-world operation, several adaptations would be necessary. For example, the models would need to be integrated with existing air traffic management systems and validated through rigorous testing and evaluation. Additionally, the location and number of on-ramp and off-ramp points, as well as the connections between different corridors-in-the-sky, would need to be carefully considered to ensure optimal efficiency and safety. Furthermore, the investigation of the impact of safety risk on sequencing optimization when the aircraft merge from the ramp into the corridors-in-the-sky should be further investigated. This analysis would provide valuable insights into improving the safety and efficiency of the on-ramp of the corridors-in-the-sky.
Overall, our research highlights the potential benefits of using corridors-in-the-sky and provides a foundation for future studies to build upon. As air traffic continues to grow, the development and implementation of innovative solutions like the corridors-in-the-sky will be crucial for ensuring safe and efficient air travel.
This research was funded by the Fundamental Research Funds for the Central Universities of China under Grant No. ZXH2012L005; the National Natural Science Foundation of China under Grant No. U1633124; Civil Aviation Safety Capacity Building Fund Project of China No. [2022]156 and MOE (Ministry of Education in China) Youth Fund Project of Humanities and Social Sciences under Grant No. 21YJCZH075.
The authors declare there is no conflict of interest.
[1] | ADR in Beijing: Civil aircraft market forecast annual report 2020–2039, 2020. Available from: https://www.avic.com/c/2020-12-22/513568.shtml. |
[2] |
Y. Lin, L. Li, P. Ren, Y. Wang, W. Szeto, From aircraft tracking data to network delay model: A data-driven approach considering en-route congestion, Transp. Res. Part C Emerging Technol., 131 (2021), 103329. https://doi.org/10.1016/j.trc.2021.103329 doi: 10.1016/j.trc.2021.103329
![]() |
[3] | J. Chen, D. Andrisani, Design and analysis of a flow corridor-based traffic management initiative, in AIAA Guidance, Navigation, and Control Conference, Portland, Oregon, 2011. https://doi.org/10.2514/6.2011-6695 |
[4] |
A. Yousefi, A. N. Zadeh, Dynamic allocation and benefit assessment of NextGen flow corridors, Transp. Res. Part C Emerging Technol., 33 (2013), 297–310. https://doi.org/10.1016/j.trc.2012.04.016 doi: 10.1016/j.trc.2012.04.016
![]() |
[5] | J. Alipio, P. Castro, H. Kaing, N. Shahid, Dynamic airspace super sectors (DASS) as high-density highways in the sky for a new US air traffic management system, in IEEE Systems and Information Engineering Design Symposium, IEEE, (2003), 57–66. https://doi.org/10.1109/SIEDS.2003.158005 |
[6] | D. J. Wing, J. C. Smith, M. G. Ballin, Analysis of a dynamic multi-track airway concept for air traffic management, NASA Langley Research Center, 2008. |
[7] | Joint Planning and Development Office, Concept of operations for the next generation air transportation system, in Technical Report, Citeseer, 2007. |
[8] | A. Mundra, E. Simons, Self-Separation Corridors, in 2007 IEEE/AIAA 26th Digital Avionics Systems Conference, Dallas, IEEE, 2007. https://doi.org/10.1109/DASC.2007.4391883 |
[9] | Y. Morooka, N. Takeichi, S. Yamamoto, Feasibility study of a layered air corridor through direct operational cost evaluation, in AIAA Scitech 2019 Forum, San Diego, California, 2019. https://doi.org/10.2514/6.2019-1362 |
[10] | R. Hoffman, J. Prete, Principles of airspace tube design for dynamic airspace configuration, in The 26th Congress of ICAS and 8th AIAA ATIO, Anchorage, Alaska, 2008. https://doi.org/10.2514/6.2008-8939 |
[11] | H. Hering, Air traffic freeway system for Europe, Eurtocontrol Experimental Centre, EEC Note, 2005. |
[12] | B. Sridhar, S. Grabbe, K. Sheth, K. Bilimoria, Initial study of tube networks for flexible airspace utilization, in AIAA Guidance, Navigation, and Control Conference and Exhibit, Keystone, Colorado, 2006. https://doi.org/10.2514/6.2006-6768 |
[13] | C. Han, X. Zhang, K. Cai, Q. Pan, Improvements of tube design for trunk routes in China, in Proceedings of the 2011 IEEE International Conference on Vehicular Electronics and Safety, Amsterdam, Netherlands, 2011. https://doi.org/10.1109/ICVES.2011.5983833 |
[14] |
N. Takeichi, Y. Abumi, Benefit optimization and operational requirement of Flow Corridor in Japanese airspace, Proc. Inst. Mech. Eng., Part G: J. Aerosp. Eng., 230 (2016), 1780–1787. https://doi.org/10.1177/0954410015616412 doi: 10.1177/0954410015616412
![]() |
[15] | M. Xue, S. Zelinski, Complexity analysis of traffic in corridors-in-the-sky, in 10th AIAA Aviation Technology, Integration, and Operations Conference, 2010. https://doi.org/10.2514/6.2010-9112 |
[16] |
B. Ye, B. Liu, A. Xue, Y. Tian, Optimal design of flow corridors to minimize environmental impact with consideration of en-route weather conditions, Discrete Dyn. Nat. Soc., 2020 (2020), 1–9. https://doi.org/10.1155/2020/2973539 doi: 10.1155/2020/2973539
![]() |
[17] |
Y. Zhang, J. Shortle, L. Sherry, Methodology for collision risk assessment of an airspace flow corridor concept, Reliab. Eng. Syst. Saf., 142 (2015), 444–455. https://doi.org/10.1016/j.ress.2015.05.015 doi: 10.1016/j.ress.2015.05.015
![]() |
[18] | R. G. Dear, The dynamic scheduling of aircraft in the near terminal area, Cambridge, Mass.: Flight Transportation Laboratory, Massachusetts Institute of Technology, 1976. |
[19] |
R. Prakash, R. Piplani, J. Desai, An optimal data-splitting algorithm for aircraft scheduling on a single runway to maximize throughput, Transp. Res. Part E Logist. Transp. Rev., 95 (2018), 570–581. https://doi.org/10.1016/j.trc.2018.07.031 doi: 10.1016/j.trc.2018.07.031
![]() |
[20] |
A. Lieder, D. Briskorn, R. Stolletz, A dynamic programming approach for the aircraft landing problem with aircraft classes, Eur. J. Oper. Res., 243 (2015), 61–69. https://doi.org/10.1016/j.ejor.2014.11.027 doi: 10.1016/j.ejor.2014.11.027
![]() |
[21] | K. K. H. Ng, C. K. M. Lee, Aircraft scheduling considering discrete airborne delay and holding pattern in the near terminal area, in Intelligent Computing Theories and Application: 13th International Conference, Liverpool, ICIC 2017, Springer International Publishing, 2017. https://doi.org/10.1007/978-3-319-63309-1_50 |
[22] |
J. A. Bennell, M. Mesgarpour, C. N. Potts, Airport runway scheduling, 4OR: Quart. J. Oper. Res., 9 (2011), 115–138. https://doi.org/10.1007/s10288-011-0172-x doi: 10.1007/s10288-011-0172-x
![]() |
[23] |
K. Ng, C. Lee, F. Chan, Y. Qin, Robust aircraft sequencing and scheduling problem with arrival/departure delay using the min-max regret approach, Transp. Res. Part E Logist. Transp. Rev., 106 (2017), 115–136. https://doi.org/10.1016/j.tre.2017.08.006 doi: 10.1016/j.tre.2017.08.006
![]() |
[24] | International Civil Aviation Organization, Procedures for Air Navigation Services: Air Traffic Management, 2001. |
[25] |
X. Li, G. Liu, The economic loss of airlines and passengers caused by flight delays, Syst. Eng., 25 (2007), 20–23. http://dx.doi.org/10.3969/j.issn.1001-4098.2007.12.004 doi: 10.3969/j.issn.1001-4098.2007.12.004
![]() |
[26] | Civil Aviation Administration of China, CAAC civil aviation industry development statistics bulletin 2021, 2021. Available from: http://www.caac.gov.cn/XXGK/XXGK/TJSJ/index_1214.html, accessed: Oct-2022. |
[27] | ICAO, Doc8168-Procedures for Air Navigation Services (PANS)-Aircraft Operations, International Civil Aviation Organization, 7th edition, Montréal, Quebec (Canada), 2020. |
[28] | J. Kennedy, R. Eberhart, Particle swarm optimization, in Proceedings of ICNN'95-International Conference on Neural Networks, IEEE, 4 (1995). http://dx.doi.org/10.1109/ICNN.1995.488968 |
[29] |
J. A. Bennell, M. Mesgarpour, C. N. Potts, Dynamic scheduling of aircraft landings, Eur. J. Oper. Res., 258 (2017), 315–327. http://dx.doi.org/10.1016/j.ejor.2016.08.015 doi: 10.1016/j.ejor.2016.08.015
![]() |
[30] | A. Yousefi, G. L. Donohue, Optimum airspace sectorization with air traffic controller workload constraints, in 1st International Conference for Research in Air Transportation, 2004. |
[31] | A. Yousefi, G. L. Donohue, L. Sherry, High-volume tube shaped sectors (HTS): A network of high-capacity ribbons connecting congested city pairs, in The 23rd Digital Avionics Systems Conference, IEEE, 1 (2004). |
[32] |
M. Xue, P. Kopardekar, High-capacity tube network design using the Hough transform, J. Guid. Control Dyn., 32 (2009), 788–795. https://doi.org/10.2514/1.40386 doi: 10.2514/1.40386
![]() |
[33] | N. Takeichi, S. Yamamoto, Y. Morooka, A. Harada, Potential cost-benefit analysis for the assessment of air corridor installation into Japanese airspace, in 2018 Winter Simulation Conference (WSC), IEEE, (2018), 2297–2308. https://doi.org/10.1109/WSC.2018.8632382 |
[34] | A. Yousefi, J. Lard, J. Timmerman, NextGen flow corridors initial design, procedures, and display functionalities, in IEEE/AIAA 29th Digital Avionics Systems Conference, Salt Lake City, UT, 2010. https://doi.org/10.1109/DASC.2010.5655340 |
[35] | D. Wing, J. C. Smith, M. G. Ballin, Analysis of a dynamic multi-track airway concept for air traffic management, in NASA/tp-2008-215323, NASA Langley Research Center, 2008. |
[36] |
N. Takeichi, Y. Nakamura, K. Kageyama, High density air corridor: benefit, allocation and self-separation, Aeronaut. Space Sci. Jpn., 60 (2012), 449–454. https://doi.org/10.14822/kjsass.60.12_449 doi: 10.14822/kjsass.60.12_449
![]() |
[37] |
N. Takeichi, Y. Nakamura, K. Kageyama, Aircraft self-separation algorithm for high density air corridor operation based on flight intent, Trans. Jpn. Soc. Aeronaut. Space Sci., 57 (2014), 179–185. https://doi.org/10.2322/tjsass.57.179 doi: 10.2322/tjsass.57.179
![]() |
[38] | B. Barmore, W. J. Penhallegon, L. A. Weitz, Interval management: development and implementation of an airborne spacing concept, in AIAA Guidance, Navigation, and Control Conference, San Diego, CA, 2016. https://doi.org/10.2514/6.2016-1608 |
[39] | K. Sheth, T. Islam, P. Kopardekar, Analysis of airspace tube structures, in 2008 IEEE/AIAA 27th Digital Avionics Systems Conference, IEEE, 2008. https://doi.org/10.1109/DASC.2008.4702805 |
[40] | P. Thomas, L. Paul, Human-in-the-loop evaluation of nextgen concepts in the airspace operations laboratory, in AIAA Modeling and Simulation Technologies Conference, Toronto, Ontario, 2010. https://doi.org/10.2514/6.2010-7609 |
[41] | S. Banavar, I. Tanim, G.Gautam, Design and simulation methodology to improve the performance of airspace tube networks, in AIAA Guidance, Navigation, and Control Conference, Toronto, Ontario, 2010. https://doi.org/10.2514/6.2010-8288 |
[42] |
Y. Nakamura, N. Takeichi, A self-separation algorithm using relative speed for high density air corridor, Trans. Jpn. Soc. Aeronaut. Space Sci., 57 (2014), 336–342. https://doi.org/10.2322/tjsass.57.336 doi: 10.2322/tjsass.57.336
![]() |
[43] | B. Robertson, Fuel Conservation Strategies: cost index explained, Boeing Aero Q., 2 (2007), 26–29. |
[44] | M. Xue, Design analysis of corridors-in-the-sky, in AIAA Guidance, Navigation, and Control Conference, Chicago, Illinois, 2009. https://doi.org/10.2514/6.2009-5859 |
[45] | P. Maini, P. B. Sujit, Path Planning Algorithms for Single and Multiple Mobile Robot Systems, Ph.D thesis, Indraprastha Institute of Information Technology Delhi, New Delhi, 2020. |
[46] |
M. V. A. R. Bahubalendruni, A. Gulivindala, M. Kumar, B. B. Biswal, L. N. Annepu, A hybrid conjugated method for assembly sequence generation and explode view generation, Assem. Autom., 39 (2019), 211–225. https://doi.org/10.1108/AA-01-2018-014 doi: 10.1108/AA-01-2018-014
![]() |
[47] | G. Gupta, B. Sridhar, A. Mukherjee, Freeways in the sky: exploring tube airspace design through mixed integer programming, in Proceedings of the INFORMS Annual Meeting, Washington, 2008. https://doi.org/10.2514/6.2008-6824 |
[48] |
Y. Tian, Y. Dong, B. Ye, L. Wan, A framework for the assessment of distributed self-separation procedures for air traffic in flow corridors, IEEE Access, 7 (2019), 123544–123557. https://doi.org/10.1109/ACCESS.2019.2937655 doi: 10.1109/ACCESS.2019.2937655
![]() |
[49] |
F. P. Moreno, Methodology of air traffic flow clustering and 3-D prediction of air traffic density in ATC sectors based on machine learning models, Exp. Syst. Appl., 223 (2023), 119897. https://doi.org/10.1016/j.eswa.2023.119897 doi: 10.1016/j.eswa.2023.119897
![]() |
[50] |
Z. Wang, D. Delahaye, J. L. Farges, S. Alam, Complexity optimal air traffic assignment in multi-layer transport network for Urban Air Mobility operations, Transp. Res. Part C Emerging Technol., 142 (2022), 103776. https://doi.org/10.1016/j.trc.2022.103776 doi: 10.1016/j.trc.2022.103776
![]() |
[51] | Z. Wang, D. Delahaye, J. L. Farges, S. Alam, Route network design in low-altitude airspace for future urban air mobility operations-A case study of urban airspace of Singapore, in International Conference on Research in Air Transportation, 2020. https://doi.org/10.13140/RG.2.2.13920.97285 |
[52] |
Z. Wang, D. Delahaye, J. L. Farges, S. Alam, Air traffic assignment for intensive urban air mobility operations, J. Aerosp. Inf. Syst., 18 (2021), 860–875. https://doi.org/10.2514/1.I010954 doi: 10.2514/1.I010954
![]() |
1. | Xu Zhan, Yang Yong, Wang Xiao, Phased mission reliability analysis of unmanned ship systems, 2023, 31, 2688-1594, 6425, 10.3934/era.2023325 | |
2. | Hongyan Dui, Huiting Xu, Haohao Zhou, Reliability analysis and resilience measure of complex systems in shock events, 2023, 31, 2688-1594, 6657, 10.3934/era.2023336 |
Notations | Explanation | Unit |
n | The number of aircraft on the corridors-in-the-sky | / |
m | The number of aircraft on the jet route | / |
Tl | The time when the aircraft l on the corridors-in-the-sky arrives at the on-ramp | / |
Tai | The time when the aircraft i on the jet route arrives at the holding fix of the on-ramp | / |
td | The required minimum safety time interval which the aircraft should maintain on the corridors-in-the-sky | Minute |
tg | The transition time of the aircraft from the holding fix to the on-ramp | Minute |
tw | The time for aircraft to conduct a standard holding procedure | Minute |
K | The maximum number of standard holding procedures | / |
C1 | The economic loss of airlines caused by delay | CNY |
Ci, fuel | The fuel consumption cost per minute of flight i | CNY/Minute |
C2 | The economic loss of passengers caused by delay | CNY |
Ci, pas | The average delay cost per person of flight i | CNY/Minute |
ri | The maximum passenger capacity of flight i | / |
qi | The delay cost coefficient of flight i | / |
τ | The flight occupancy rate | / |
Decision variables | Explanation | |
xi | Actual time of joining the corridors-in-the-sky of aircraft i | |
ki | The cumulative number of completing standard holding procedures for aircraft i | |
δij | 1, if aircraft i is scheduled to join the corridors-in-the-sky before aircraft j (i=1,…,m;j=1,…,m;i≠j) 0, otherwise |
Wake turbulence category | Symbol | Maximum takeoff weight (ton) | Operation cost (CNY/minute) |
Heavy | H | > 136 | 468 |
Medium | M | 7-136 | 258 |
Light | L | ≤ 7 | 24 |
S.N. | 1 | 2 | 3 | 4 | 5 | 6 | 7 | 8 |
Time at the ramp | 10:00 | 10:02 | 10:08 | 10:09 | 10:15 | 10:19 | 10:24 | 10:30 |
S.N. | 9 | 10 | 11 | 12 | 13 | 14 | 15 | 16 |
Time at the ramp | 10:31 | 10:35 | 10:39 | 10:40 | 10:44 | 10:56 | 10:57 | 11:07 |
S.N. | 17 | 18 | 19 | 20 | 21 | 22 | 23 | 24 |
Time at the ramp | 11:18 | 11:19 | 11:20 | 11:23 | 11:26 | 11:30 | 11:34 | 11:40 |
S.N. | Type | Wake turbulence category | Type of flight | Maximum passenger capacity | Estimated time when aircraft arrive at holding fix | Connecting flight |
1 | B737-700 | M | Scheduled-domestic | 130 | 10:01 | Y |
2 | A330-200 | H | Scheduled-domestic | 237 | 10:02 | N |
3 | B757-200 | H | Chartered | 200 | 10:12 | N |
4 | A320-200 | M | Scheduled-domestic | 179 | 10:14 | Y |
5 | B747-400 | H | Scheduled-domestic | 400 | 10:16 | N |
6 | B747-400 | H | Scheduled-international | 400 | 10:18 | N |
7 | B767-200 | H | Scheduled-international | 214 | 10:22 | Y |
8 | A319 | M | Scheduled-domestic | 124 | 10:24 | Y |
9 | A340-200 | H | Scheduled-international | 375 | 10:32 | N |
10 | B737-200 | M | Scheduled-domestic | 128 | 10:43 | Y |
11 | B737-700 | M | Scheduled-domestic | 130 | 10:47 | N |
12 | A310-200 | H | Scheduled-domestic | 228 | 10:49 | Y |
Notations | Explanation | Unit |
n | The number of aircraft on the corridors-in-the-sky | / |
m | The number of aircraft on the jet route | / |
Tl | The time when the aircraft l on the corridors-in-the-sky arrives at the on-ramp | / |
Tai | The time when the aircraft i on the jet route arrives at the holding fix of the on-ramp | / |
td | The required minimum safety time interval which the aircraft should maintain on the corridors-in-the-sky | Minute |
tg | The transition time of the aircraft from the holding fix to the on-ramp | Minute |
tw | The time for aircraft to conduct a standard holding procedure | Minute |
K | The maximum number of standard holding procedures | / |
C1 | The economic loss of airlines caused by delay | CNY |
Ci, fuel | The fuel consumption cost per minute of flight i | CNY/Minute |
C2 | The economic loss of passengers caused by delay | CNY |
Ci, pas | The average delay cost per person of flight i | CNY/Minute |
ri | The maximum passenger capacity of flight i | / |
qi | The delay cost coefficient of flight i | / |
τ | The flight occupancy rate | / |
Decision variables | Explanation | |
xi | Actual time of joining the corridors-in-the-sky of aircraft i | |
ki | The cumulative number of completing standard holding procedures for aircraft i | |
δij | 1, if aircraft i is scheduled to join the corridors-in-the-sky before aircraft j (i=1,…,m;j=1,…,m;i≠j) 0, otherwise |
Wake turbulence category | Symbol | Maximum takeoff weight (ton) | Operation cost (CNY/minute) |
Heavy | H | > 136 | 468 |
Medium | M | 7-136 | 258 |
Light | L | ≤ 7 | 24 |
S.N. | 1 | 2 | 3 | 4 | 5 | 6 | 7 | 8 |
Time at the ramp | 10:00 | 10:02 | 10:08 | 10:09 | 10:15 | 10:19 | 10:24 | 10:30 |
S.N. | 9 | 10 | 11 | 12 | 13 | 14 | 15 | 16 |
Time at the ramp | 10:31 | 10:35 | 10:39 | 10:40 | 10:44 | 10:56 | 10:57 | 11:07 |
S.N. | 17 | 18 | 19 | 20 | 21 | 22 | 23 | 24 |
Time at the ramp | 11:18 | 11:19 | 11:20 | 11:23 | 11:26 | 11:30 | 11:34 | 11:40 |
S.N. | Type | Wake turbulence category | Type of flight | Maximum passenger capacity | Estimated time when aircraft arrive at holding fix | Connecting flight |
1 | B737-700 | M | Scheduled-domestic | 130 | 10:01 | Y |
2 | A330-200 | H | Scheduled-domestic | 237 | 10:02 | N |
3 | B757-200 | H | Chartered | 200 | 10:12 | N |
4 | A320-200 | M | Scheduled-domestic | 179 | 10:14 | Y |
5 | B747-400 | H | Scheduled-domestic | 400 | 10:16 | N |
6 | B747-400 | H | Scheduled-international | 400 | 10:18 | N |
7 | B767-200 | H | Scheduled-international | 214 | 10:22 | Y |
8 | A319 | M | Scheduled-domestic | 124 | 10:24 | Y |
9 | A340-200 | H | Scheduled-international | 375 | 10:32 | N |
10 | B737-200 | M | Scheduled-domestic | 128 | 10:43 | Y |
11 | B737-700 | M | Scheduled-domestic | 130 | 10:47 | N |
12 | A310-200 | H | Scheduled-domestic | 228 | 10:49 | Y |