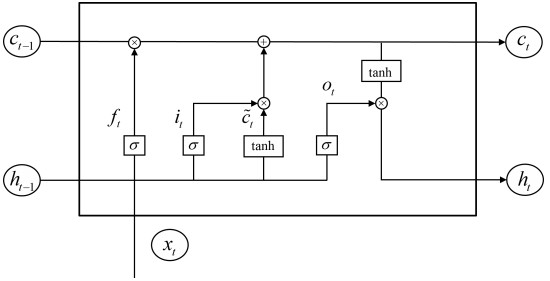
Short-term load forecasting is of great significance to the operation of power systems. Various uncertain factors, such as meteorological social data, have already been combined with historical power data to create more accurate load forecasting models. In traditional systems, data from various industries and regions are centralized for knowledge extraction. However, concerns regarding data security and privacy often prevent industries from sharing their data, limiting both the quantity and diversity of data available for forecasting models. These challenges drive the adoption of federated learning (FL) to address issues related to data silos and privacy. In this paper, a novel framework for short-term load forecasting was proposed using historical data from industries such as power, meteorology, and finance. Long short-term memory (LSTM) networks were utilized for forecasting, and federated learning (FL) was implemented to protect data privacy. FL allows clients in multiple regions to collaboratively train a shared model without exposing their data. To further enhance security, the homomorphic encryption (HE) using Paillier algorithm was introduced during the federated process. Experimental results demonstrate that the federated model, which extracts knowledge from different regions, outperforms locally trained models. Furthermore, longer HE keys have little effect on predictive performance but significantly slow down encryption and decryption, thereby increasing training time.
Citation: Mengdi Wang, Rui Xin, Mingrui Xia, Zhifeng Zuo, Yinyin Ge, Pengfei Zhang, Hongxing Ye. A federated LSTM network for load forecasting using multi-source data with homomorphic encryption[J]. AIMS Energy, 2025, 13(2): 265-289. doi: 10.3934/energy.2025011
[1] | Abdelkarim El khantach, Mohamed Hamlich, Nour eddine Belbounaguia . Short-term load forecasting using machine learning and periodicity decomposition. AIMS Energy, 2019, 7(3): 382-394. doi: 10.3934/energy.2019.3.382 |
[2] | Hassan Shirzeh, Fazel Naghdy, Philip Ciufo, Montserrat Ros . Stochastic energy balancing in substation energy management. AIMS Energy, 2015, 3(4): 810-837. doi: 10.3934/energy.2015.4.810 |
[3] | Muhammad Farhan Hanif, Muhammad Sabir Naveed, Mohamed Metwaly, Jicang Si, Xiangtao Liu, Jianchun Mi . Advancing solar energy forecasting with modified ANN and light GBM learning algorithms. AIMS Energy, 2024, 12(2): 350-386. doi: 10.3934/energy.2024017 |
[4] | Lamya Lairgi, Rachid Lagtayi, Yassir Lairgi, Abdelmajid Daya, Rabie Elotmani, Ahmed Khouya, Mohammed Touzani . Optimization of tertiary building passive parameters by forecasting energy consumption based on artificial intelligence models and using ANOVA variance analysis method. AIMS Energy, 2023, 11(5): 795-809. doi: 10.3934/energy.2023039 |
[5] | Hana Altrabalsi, Vladimir Stankovic, Jing Liao, Lina Stankovic . Low-complexity energy disaggregation using appliance load modelling. AIMS Energy, 2016, 4(1): 1-21. doi: 10.3934/energy.2016.1.1 |
[6] | K. M. S. Y. Konara, M. L. Kolhe, Arvind Sharma . Power dispatching techniques as a finite state machine for a standalone photovoltaic system with a hybrid energy storage. AIMS Energy, 2020, 8(2): 214-230. doi: 10.3934/energy.2020.2.214 |
[7] | Norman Maswanganyi, Edmore Ranganai, Caston Sigauke . Long-term peak electricity demand forecasting in South Africa: A quantile regression averaging approach. AIMS Energy, 2019, 7(6): 857-882. doi: 10.3934/energy.2019.6.857 |
[8] | Abdul Matin Ibrahimi, Mohammad Masih Sediqi, Harun Or Rashid Howlader, Mir Sayed Shah Danish, Shantanu Chakraborty, Tomonobu Senjyu . Generation expansion planning considering renewable energy integration and optimal unit commitment: A case study of Afghanistan. AIMS Energy, 2019, 7(4): 441-464. doi: 10.3934/energy.2019.4.441 |
[9] | Matteo Moncecchi, Davide Falabretti, Marco Merlo . Regional energy planning based on distribution grid hosting capacity. AIMS Energy, 2019, 7(3): 264-284. doi: 10.3934/energy.2019.3.264 |
[10] | Jinhua Tian, Yueyuan Zhang, Yanan Gao, Yu Qin, Bihan Fan, Cheng Zhang, Qiqi Zang . Distributed optimization and scheduling strategy for source load storage distribution grid based on alliance chain. AIMS Energy, 2024, 12(5): 946-967. doi: 10.3934/energy.2024044 |
Short-term load forecasting is of great significance to the operation of power systems. Various uncertain factors, such as meteorological social data, have already been combined with historical power data to create more accurate load forecasting models. In traditional systems, data from various industries and regions are centralized for knowledge extraction. However, concerns regarding data security and privacy often prevent industries from sharing their data, limiting both the quantity and diversity of data available for forecasting models. These challenges drive the adoption of federated learning (FL) to address issues related to data silos and privacy. In this paper, a novel framework for short-term load forecasting was proposed using historical data from industries such as power, meteorology, and finance. Long short-term memory (LSTM) networks were utilized for forecasting, and federated learning (FL) was implemented to protect data privacy. FL allows clients in multiple regions to collaboratively train a shared model without exposing their data. To further enhance security, the homomorphic encryption (HE) using Paillier algorithm was introduced during the federated process. Experimental results demonstrate that the federated model, which extracts knowledge from different regions, outperforms locally trained models. Furthermore, longer HE keys have little effect on predictive performance but significantly slow down encryption and decryption, thereby increasing training time.
Short-term load forecasting (STLF) [1] is one of the classic applications in smart grids, aiding various sectors in balancing power generation and consumption, thereby improving energy efficiency through management and conservation [2]. As the foundation of power system scheduling and operation, short-term load scheduling not only influences the efficient functioning of the electricity market but also directly affects the stability and sustainability of power supply. By analyzing historical load data and environmental variables, researchers can utilize various predictive models to forecast future power load trends, providing scientific decision support for power system operators [3]. Increasingly, data-driven and machine learning-based approaches are being applied for power load forecasting. However, several factors introduce uncertainties that can affect the accuracy of STLF, including historical load records [4], weather conditions, social factors, and emergencies [5].
With the rise of regulatory requirements and security measures, data sharing across different countries and sectors has become challenging, thereby limiting the applicability of machine learning approaches. Since this data originates from various business units or regional departments, concerns over data privacy often lead to data being processed in isolated systems rather than being integrated into a common system for unified machine learning objectives [6]. This fragmentation complicates data integration and utilization. Even if different departments involved in machine learning make their datasets accessible, most existing work relies on centralized training methods, where data from all clients must be collected on a central server for model training, resulting in substantial communication overhead. When models require continuous updating with new data, the communication costs of centralized learning can become prohibitive. FL is a distributed machine learning method that coordinates the collaborative training of a shared global model among participating devices under the guidance of a federated server. Each participating device trains a local model using its local data without transferring the data to the federated server. Only the model parameters are sent to the central server for updating the shared global model. Thus, the federated architecture effectively preserves data privacy. FL has already been proven effective in the domain of load forecasting. The application of FL in household load forecasting was pioneered by Taik et al. [7], who utilized data from smart meters to train a federated prediction model with successful outcomes. Gholizadeh et al. [8] analyzed six different scenarios of load forecasting using historical data from smart meters and proposed a novel consumer clustering method to reduce the convergence time of FL. Briggs et al. [9] demonstrated that centralized models struggle to capture individual household energy usage behaviors and optimize them effectively, highlighting that post-federated learning personalization steps can enhance model performance. Further investigation by Wang et al. [10] emphasized that personalized models offer greater advantages compared to local models for federated clients with limited data.
The motivation for this study stems from the need to account for as many uncertainty factors affecting load as possible and to extract knowledge from data across various sectors, such as power and meteorology to achieve short-term load forecasting. However, organizations in these sectors often refrain from sharing data due to concerns over security and privacy breaches, which impedes the development of highly accurate load forecasting models. In this context, developing a load forecasting model that strikes a balance between privacy protection and prediction efficiency poses a challenging and intricate task. FL offers a promising solution for maintaining data privacy while enabling load forecasting models to be trained on decentralized devices, making cross-industry and cross-regional data sharing possible. By training load forecasting models on local client devices without transferring raw data to a central server, FL protects data privacy and ensures compliance with regulations such as the General Data Protection Regulation (GDPR). Meanwhile, FL reduces the risk of privacy breaches while providing accuracy comparable to that of centrally trained load forecasting models.
However, FL introduces new privacy concerns related to the transmission of local model parameters between clients and the server, as these parameters could be exploited by third parties to reconstruct sensitive information. To address this issue, the homomorphic encryption (HE) mechanism [11] is introduced into FL. Homomorphic encryption enables the execution of various operations on encrypted data without the need for prior decryption. By applying homomorphic encryption to local model parameters during the federated process, data privacy can be ensured at the federated server while computational tasks are completed. The adoption of homomorphic encryption schemes necessitates consideration of a range of factors, such as the type of plaintext, the size of the ciphertext, the key size, and the mathematical operations involved. These considerations impose limitations on the applicability of homomorphic encryption schemes. Several works have already addressed the privacy of model parameters in the context of federated load forecasting. For instance, Zhang et al. [12] employed the Paillier algorithm to process model parameters during the federated process, yet completely overlooked the impact of homomorphic encryption and decryption on model training time. Wang et al. [13] utilized masking and differential privacy techniques during model gradient aggregation but acknowledged that this approach consumed significant computational and communication resources. Fang et al. [14] adopted the CAT method during the transmission of updated model parameters, updating only the sufficiently changed values in each round of model parameter updates. This approach achieved predictions with acceptable performance and approximately 90% communication bandwidth savings. However, it suffered from significant accuracy degradation after multiple rounds of training.
To address these issues, a novel architecture for short-term load forecasting is proposed, named FLSTM-HE, to tackle the aforementioned challenges. The FLSTM-HE method combines federated learning with an LSTM network. The federated learning framework in FLSTM-HE is designed to build a privacy-preserving, LSTM-based general model that effectively predicts short-term load based on multi-source historical data. The Paillier homomorphic encryption scheme, proposed by Paillier [15], is then applied to encrypt the model weights during the federated learning process. The server performs federated averaging on the encrypted weights, further enhancing data privacy. To mitigate the impact of homomorphic encryption on training time, the selective encryption of parameters is proposed, which has been demonstrated to be robust and effective in reducing model training time [16]. The main contributions of this paper are as follows:
● A federated learning framework is introduced into the smart grid system to address issues of data availability and privacy, utilizing cross-industry and cross-regional data to improve prediction performance.
● A short-term load forecasting model based on LSTM is proposed, which effectively predicts short-term electricity loads by learning the contextual information from historical data.
● To further enhance data privacy, the Paillier homomorphic encryption algorithm is applied to encrypt the model weights, and the selective encryption of model weights helps reduce the encryption and decryption times.
The rest of the paper is organized as follows: Section 2 provides a detailed explanation of the relevant technologies. Section 3 presents the proposed FLSTM-HE framework for load forecasting. Section 4 describes the experimental setup. Section 5 presents and analyzes the experimental results. Section 6 concludes this paper.
Federated learning provides a solution for analyzing, classifying, and predicting tasks across multiple datasets while considering data privacy, through distributed machine learning and local model training [17]. It enables the aggregation of data from multiple parties in environments where privacy is a concern. At the same time, FL can yield more effective results for participants with poor data quality or insufficient data volumes in distributed machine learning scenarios [9]. Typically, FL involves three main steps: 1) Construct models and initially train them using a shared dataset. 2) Participants train their local models with the initial datasets and then share their updated local models with a central server. 3) The central server aggregates the local models to create a global model, which is then shared back with the participants. As a distributed framework, FL can be seamlessly integrated into various applications through specific methodologies.
For example, in medical research, FL can facilitate the analysis of patient data from multiple hospitals without compromising patient privacy. Sheller et al. [18] showed that the data quality of the FL model across 10 institutions reached 99% of that of a centralized model. Sadilek et al. [19] conducted a series of studies, demonstrating that the performance and interpretability of FL results are comparable to those of centralized models. Ng et al. [20] showed that through FL, clients with small datasets can achieve performance comparable to that of models trained on large datasets, which benefits smaller medical institutions in building their own AI models. FL is also frequently applied in edge computing. Due to limitations in bandwidth, storage, and privacy concerns, sending all edge device data to a centralized location for training machine learning models is often impractical [21]. Recent studies have shown that edge-assisted federated learning schemes, such as FedEdge [22], can effectively address these challenges by reducing communication overhead and improving model accuracy.
Compared to traditional centralized machine learning training, federated learning offers several distinct advantages:
● Reduced training time: FL leverages multiple devices to compute gradients in parallel, significantly accelerating model training time. In a federated framework, each device maintains a local copy of the model, enabling rapid and confident predictions without the latency associated with querying a cloud server.
● Privacy protection: Storing sensitive information in the cloud poses significant privacy risks for applications like smart grid systems and medical devices. Privacy breaches in these contexts can have severe consequences; therefore, keeping data on local devices helps protect end-user privacy.
● Collaborative learning: FL is based on collaborative learning, which is both straightforward and energy-efficient, as the model is trained on edge devices. This makes edge computing an ideal environment for FL. Utilizing FL within an edge-cloud paradigm helps address issues related to communication costs, privacy, security, and compliance.
The LSTM network is an advanced type of recurrent neural network (RNN), designed to address the issues of vanishing and exploding gradients that traditional RNNs encounter when processing long sequences of data [23]. LSTMs have demonstrated outstanding performance in sequence data processing and are widely applied in time series forecasting, natural language processing, speech recognition, and other fields. Due to its superior capability in handling time series data, LSTM has gradually become a mainstream method for power load forecasting [24]. There are two primary approaches for performing electricity load forecasting: 1) models based on physical principles and 2) models based on statistical and machine learning techniques. With the advent of smart meters, it has become feasible to obtain energy consumption data at the level of individual buildings or residences. The large amounts of time series data collected by smart meters can be analyzed using time series analysis methods to summarize users' electricity consumption habits and patterns, leading to more accurate predictions of future energy usage. As smart meters are increasingly deployed, data-driven machine learning models are being increasingly employed in load forecasting.
Compared to the aggregated total electricity load, building-level and residential-level loads exhibit higher uncertainty and volatility due to more variable consumption patterns. Predicting the electricity load of a single household is particularly challenging. LSTM, with its unique gating mechanism, can effectively capture and leverage both short- and long-term dependencies within electricity load data [25]. Many studies have used LSTM networks in combination with convolutional neural networks (CNNs), where the convolutional layers are utilized to extract internal features and identify key attributes from the data, while LSTMs capture the short- and long-term dependencies [26]. For instance, Rafi et al. (2021) developed a short-term load forecasting method using this hybrid CNN-LSTM model, which showed promising results in predicting energy load [27]. Similarly, Alhussein et al. (2020) employed a hybrid CNN-LSTM approach for individual household load forecasting, highlighting the effectiveness of CNNs for feature extraction and LSTMs for modeling sequential patterns [28]. However, due to the specific requirements of the federated environment, more complex model parameters would result in increased computation and encryption/decryption costs. Therefore, this study employs only the LSTM network.
The basic unit of an LSTM network is the LSTM cell, which is designed to overcome the vanishing gradient problem encountered in traditional RNNs.
An LSTM cell consists of several key components as shown in Figure 1:
● Cell State (ct): This is a memory unit that carries information across time steps.
● Hidden State (ht): This is the output of the LSTM cell at a given time step.
● Forget Gate (ft): Decides the proportion of the cell state to forget.
● Input Gate (it): Determines how much of the new information should be added to the cell state.
● Output Gate (ot): Decides how much of the cell state should be output.
Given the input at time step t as xt, the previous hidden state ht−1, and the previous cell state ct−1, the LSTM cell computes the following:
● Forget Gate (ft):
ft=σ(Wfxt+Ufht−1+bf) | (2.1) |
● Input Gate (it) and Input Modulation Gate (˜ct):
it=σ(Wixt+Uiht−1+bi) | (2.2) |
˜ct=tanh(Wcxt+Ucht−1+bc) | (2.3) |
● Cell State Update (ct):
ct=ft⊙ct−1+it⊙˜ct | (2.4) |
● Output Gate (ot) and Hidden State Update (ht):
ot=σ(Woxt+Uoht−1+bo) | (2.5) |
ht=ot⊙tanh(ct) | (2.6) |
where Wf is the weight matrix for the input xt. Uf is the weight matrix for the previous hidden state ht−1. bf is the bias term. σ is the sigmoid activation function. Wi, Ui, bi are the weight matrix, previous hidden state matrix, and bias term for the input gate, respectively. Wc, Uc, bc are the corresponding matrices and bias term for the cell state. Wo, Uo, bo are the matrices and bias term for the output gate. ⊙ denotes element-wise multiplication. tanh is the hyperbolic tangent activation function.
Homomorphic encryption is an encryption technique that allows mathematical operations to be performed on encrypted ciphertexts, generating an encrypted result without requiring decryption first [29]. When the encrypted result is decrypted, it matches the result of performing the same mathematical operations on the plaintext. HE enables third parties to perform specific operations on a client's data without decrypting it, thereby achieving third-party application objectives while maintaining the privacy of the client's data.
Homomorphic encryption (HE) has gained increasing attention for its ability to preserve privacy during data processing. For instance, Zhang et al. (2023) applied HE in Internet of Things (IoT)-enabled healthcare systems, enabling secure federated learning for medical data analysis while safeguarding privacy [30]. Similarly, Hatip et al. (2024) focused on enhancing security and privacy in IoT-based learning systems through the use of HE, addressing growing concerns about data protection in connected environments [31]. In the realm of biomedical research, Kachouh et al. (2021) employed HE to preserve the privacy of genomic data, offering a secure method for processing sensitive genetic information [32]. HE has also been applied in blockchain technology; Basuki et al. (2021) used HE to ensure the integrity and confidentiality of images in blockchain-driven watermarking systems [33]. Moreover, Gadiwala et al. (2024) demonstrated the effectiveness of HE in federated learning for privacy-preserving machine learning, highlighting its potential for secure multi-party data collaboration [34]. Finally, Mohialden et al. (2023) proposed a homomorphic encryption model for secure federated learning, focusing on improving privacy and security in distributed machine learning environments [35].
When using HE, the client first encrypts the data before uploading it to a cloud server. The cloud server, which is unaware of the content of the encrypted data, uses HE to perform mathematical operations on the encrypted data, typically completing its application or task. The client then receives the encrypted result from the cloud server and decrypts it using their private key. Based on the scale of mathematical operations performed on encrypted messages, HE can be categorized into three types [11]: partially homomorphic encryption (PHE), somewhat homomorphic encryption (SHE), and fully homomorphic encryption (FHE).
In the proposed FL framework, PHE is considered as a promising solution to ensure the confidentiality and privacy of intelligent industrial data in an anomaly detection FL environment, while the Paillier encryption scheme is a form of PHE that allows servers to process and aggregate model parameters with homomorphic properties without requiring decryption.
The Paillier encryption consists of three main components: key generation, encryption, and decryption.
● Public Key (n,g): The public key consists of modulus n and a random integer g.
● Private Key (λ,μ): The private key is composed of λ, calculated as the least common multiple (LCM) of p−1 and q−1, and μ is an optional precomputed value.
● Key Generation: Given two large prime numbers p and q:
n=p×q | (2.7) |
Calculate λ, the least common multiple (LCM) of p−1 and q−1:
λ=LCM(p−1,q−1) | (2.8) |
Select a random integer g∈Z∗n such that:
gcd(L(gλmodn2),n)=1 | (2.9) |
Optionally, compute:
μ=(L(gλmodn2))−1modn | (2.10) |
The public key is (n,g), and the private key is λ (with optional μ).
● Encryption: Given a message m∈Zn, choose a random number r∈Z∗n, and compute the ciphertext c as:
c=gm×rnmodn2 | (2.11) |
● Decryption: Given the ciphertext c and private key λ, compute the decrypted message m as:
m=L(cλmodn2)L(gλmodn2)modn | (2.12) |
If μ has been precomputed, the message can also be decrypted as:
m=L(cλmodn2)×μmodn | (2.13) |
where L(x)=x−1n is the L-function used in the decryption process.
In this section, the proposed framework FLSTM-HE is introduced. This framework is designed for short-term load forecasting in smart grid systems based on federated learning, creating a distributed machine learning environment for short-term power load prediction while protecting data privacy. To further secure the transmission of weights during the federated learning process, federated averaging (FedAvg) is adopted as the federated algorithm for the central server and we introduce the Paillier homomorphic encryption algorithm to prevent the reconstruction of sensitive information from the transmitted model parameters. While the central federated server is presumed to be honest and reliable, capable of executing designated weight calculations and managing communication, there remains a concern regarding the sensitivity of model gradients. The computing nodes, which may operate with semi-honesty and have an inherent curiosity about other nodes' data, necessitate the implementation of HE. This encryption is crucial for safeguarding the transmitted model parameters during communication.
In the proposed FL framework, the central server operates in the cloud, receiving encrypted model weights from regional data units, performing federated averaging, and returning the updated model weights for the next round of training to each unit. The regional data units collect meteorological and electrical data within their respective regions and train local ML models, Furthermore, remote terminal units (RTUs) are utilized to facilitate data communication with the central server, thereby ensuring efficient data transmission, as illustrated in Figure 2. The flowchart in Figure 3 provides a visual representation of the entire process, from data collection to model updates.
Initially, the central server and regional data units negotiate a key distribution and share an initial model. These regional data units then collect and preprocess local data, performing local training using the initial model. After completing local training, model weights are encrypted using Paillier homomorphic encryption and shared with the central server. The central server, upon receiving the encrypted model weights from each data unit, runs the federated averaging function on the encrypted weights to compute the model weights for the next training round and sends them back. The model weights are then decrypted by each data unit and used for the subsequent round of model training.
In the federated learning framework, the Paillier homomorphic encryption is used, which supports additive operations on encrypted values. This encryption scheme allows us to securely aggregate encrypted model updates from various clients. The Paillier encryption scheme is defined by the following encryption function:
C=Enc(m,r,λ) | (3.1) |
where C denotes the ciphertext (encrypted value), m represents the plaintext message (e.g., model parameters), r is a random value used in the encryption process to ensure security, and λ is the public key of the Paillier encryption scheme.
Each regional data unit trains a local LSTM network and participates in federated learning as a client, with the federated server choosing the FedAvg method. Initially, the federated server uses a public dataset to initialize the model. After the initial model training is completed, the federated server distributes the model to all participating clients.
In the federated learning framework, each client j trains its local model with parameters Θj. To ensure privacy, these parameters are encrypted using the Paillier homomorphic encryption scheme before being sent to the central server. The encryption of the model parameters Θj on the client side can be represented as:
Cj=Enc(Θj,k) | (3.2) |
where Cj denotes the encrypted model parameters, and k is the public key used for encryption.
Once the server receives the encrypted parameters Cj from all participating clients, it aggregates them using the FedAvg algorithm. The homomorphic properties of the Paillier encryption scheme allow the server to compute the average of the encrypted parameters without decrypting them:
Cavg=Enc(1NN∑j=1Θj,k)=N∏j=1C1Nj | (3.3) |
where Cavg is the encrypted average of the model parameters, and N is the number of clients. The server then sends Cavg back to each client.
Finally, each client decrypts Cavg to obtain the updated global model parameters:
Θnew=Dec(Cavg,k−1) | (3.4) |
where Θnew represents the updated model parameters for the next training round, and k−1 is the private key used for decryption.
This section is dedicated to describing the dataset types used in the experimental setup and the detailed information of the proposed FLSTM-HE method. In the experiments, Python 3.9 along with its tools such as Pandas, NumPy, PyTorch, and scikit-learn are used.
Time series data collected from the Open Power System Data platform [36] are used to analyze the performance of the proposed framework. This dataset encompasses numerous European countries, aggregating electricity load sequence data and weather sequence data on a national level. Specifically, the load sequence data includes hourly electricity consumption, wind generation, solar generation, and electricity prices. These data are primarily available from the ENTSO-E Transparency Platform, although earlier records may need to be obtained through individual national sources. The weather sequence data, based on the MERRA-2 dataset provided by NASA, contains hourly geographically aggregated weather variables, such as temperature, precipitation, and wind speed. Additionally, the dataset includes simulated hourly country-aggregated heat demand and the coefficient of performance (COP) of heat pumps.
To improve the quality of the dataset, countries with high rates of missing data were excluded, ensuring consistency across the remaining datasets. This step enhanced the balance and distribution of the data, making it more suitable for training and evaluation. For feature selection, correlation analysis was performed to eliminate redundant and irrelevant variables, leaving only the most informative features. The final set of selected features includes electrical load, photovoltaic generation, direct radiation intensity, diffuse radiation intensity, and heat demand.
To maintain uniform temporal granularity, all data points were standardized to hourly intervals, ensuring alignment and consistency across the time series.
Subsequently, all features underwent min-max normalization to scale the values within the range [0, 1], as described by the formula below:
X′=X−XminXmax−Xmin | (4.1) |
where X and X′ represent the original and the normalized feature values, respectively, while Xmin and Xmax indicate the minimum and the maximum values of the feature, respectively.
The dataset was then split into two subsets: Case 1 and Case 2. Case 1 is comprised of data from 13 countries collected between January 1, 2018, and December 30, 2019, with hourly data points, while Case 2 contains data from 9 countries spanning from January 1, 2015, to December 30, 2016, also at an hourly frequency. The total size of Case 1 is approximately 14.6 MB, and Case 2 is approximately 10.1 MB. The datasets were further partitioned into training and testing sets, with 70% allocated for training and the remaining 30% for testing. The client-country mapping for both cases is presented in Table 1.
Client | Case 1 | Case 2 |
Client 1 | Austria | Austria |
Client 2 | Belgium | Belgium |
Client 3 | Bulgaria | Bulgaria |
Client 4 | Czech Republic | Estonia |
Client 5 | Germany | Spain |
Client 6 | Estonia | France |
Client 7 | Spain | United Kingdom |
Client 8 | France | Portugal |
Client 9 | United Kingdom | Slovenia |
Client 10 | Lithuania | — |
Client 11 | Portugal | — |
Client 12 | Slovenia | — |
Client 13 | Slovakia | — |
Figures 4 and 5 illustrate the comparison of load and temperature data for four countries across two randomly selected time periods. It is evident that the load across different countries exhibits similar periodicity, which can be attributed to the diurnal cycle of human activities. However, there are distinct characteristics in load variations specific to each country. Additionally, in terms of temperature changes, we observe that the temperature differences during winter are significantly greater than those during summer. Summer temperatures exhibit more regularity and consistency, while winter temperature variations are characterized by greater randomness.
The simulated federated environment is set up on a computer with the following specifications: an NVIDIA A100 GPU with 80 GB of memory, 256 GB of RAM, and an Intel Xeon Gold 6151 processor with 18 cores. Since the federated process was simulated on a single machine, the training was conducted serially. However, this did not affect the comparison of model prediction accuracy and computation time between the federated and the centralized architecture. Python 3.9 and PyTorch were used on this machine to simulate the federated server and the local networks of each client. Each client's local network consisted of 2 LSTM layers and 1 FC layer, trained with mean squared error (MSE) loss. The initial learning rate was set to 0.001 and was optimized using the Adam algorithm [37]. Additionally, the learning rate was decayed by a factor of 0.1 every 10 training epochs. The batch size was set to 32. All the hyperparameters are shown in Table 2.
Hyperparameter | Value | Description |
Learning Rate | 0.001 | Initial learning rate for the optimizer |
Batch Size | 32 | Number of samples per training batch |
Epochs | 100 | Total number of training iterations |
Optimizer | Adam | Optimization algorithm used |
Dropout Rate | 1e-4 | Dropout probability for regularization |
Weight Decay | 0.1 | Decay in every 10 training epochs |
The feature table is treated as time series data based on timestamps, with each row representing a record sampled at hourly intervals. A sliding window is implemented to look back at 24 records in order to predict the load values for the next 24 hours. Thus, the designed network provides a prediction of the load value for the following hour. A training sample includes the features from 24 records and the corresponding electricity consumption value for the next hour. The input dimension is M×L, where M is the number of feature columns in the feature table, and L represents the width of the sliding window.
In the following experiments, a single machine was used to simulate the federated learning process. In Case 1, the number of client nodes was set to 13, while in Case 2, it was set to 9. Each client performed 10 rounds of local training before conducting federated averaging.
To evaluate the predictive performance of FLSTM-HE, four baseline models were used for comparison. To evaluate the predictive performance of FLSTM-HE, four baseline models were used for comparison. To mitigate the impact of homomorphic encryption on model training time, the number of model parameters processed during encryption and decryption was reduced. Specifically, only the first effective layer and the final fully connected (FC) layer of each model were selectively encrypted, rather than encrypting all parameters. The time required for encrypting the full set of parameters can be estimated based on the ratio of the number of parameters used to the total number of parameters. A brief introduction to these models is provided below:
(1) Local LSTM: A standard artificial recurrent neural network (RNN) architecture widely used in deep learning for sequential data modeling.
(2) Federated LSTM Training (Fed-LSTM) Model: This model shares the same architecture and parameters as FLSTM-HE but does not apply homomorphic encryption to the model weights during federated learning updates.
(3) BiLSTM: A bidirectional LSTM that processes data in both forward and backward directions, capturing contextual information from past and future sequences. To evaluate its performance, multiple versions of BiLSTM are tested, including federated learning with and without homomorphic encryption, as well as different encryption key sizes.
(4) GRU: The gated recurrent Unit (GRU) simplifies the LSTM architecture by combining the input and forget gates, reducing model complexity while maintaining the ability to capture sequential dependencies. Similar to BiLSTM, GRU under various federated learning settings and homomorphic encryption configurations was evaluated, including different key sizes.
The evaluation results will be assessed using the distribution of the root mean squared error (RMSE) and mean absolute percentage rrror (MAPE). RMSE is utilized to measure the difference between the actual and predicted values, while MAPE is employed to evaluate the accuracy of the predictions. The definitions of RMSE and MAPE are presented in (4.2) and (4.3), respectively.
RMSE=√1nn∑i=1(yi−ˆyi)2 | (4.2) |
MAPE=1nn∑i=1|yi−ˆyiyi|×100% | (4.3) |
where yi represents the actual value, ˆyi represents the predicted value, and n is the total number of observations.
The proposed FLSTM-HE model was evaluated alongside four baseline models. Among the baseline models in Case 1, LSTM was trained using local data from each client, while Fed-LSTM was a federated learning model involving 13 local LSTM models, but without utilizing HE for processing model parameters. For FLSTM-HE, we employed two different key lengths. Figure 6 shows the training loss across different clients. The mapping between each client and its corresponding country is provided in Table 1. Each client participates in federated averaging after every 10 rounds of local training. It can be observed in Figure 6 that the training loss exhibits periodic increases. This occurs because, during the federated averaging process, each client incorporates knowledge from other models, which often temporarily increases the local training loss due to the updated model weights.
Tables S1 and S2 (Supplementary) present the MAPE and RMSE values for all clients under different models after 100 epochs. Four clients were randomly selected to illustrate the predicted values of the proposed model compared to the actual values. From the prediction set, 150 consecutive points were randomly chosen to plot the comparison between the predicted and actual values, as shown in Figure 7. It is evident that the true load values of different clients follow a roughly periodic pattern, determined by the cyclic nature of electricity usage. However, the load curves of different clients exhibit unique details. Our proposed model successfully learned the load trends specific to each client.
From Tables S1 and S2 (Supplementary), it can be observed that the LSTM models trained independently by each client using local data perform worse in terms of RMSE. The local BiLSTM model exhibits the worst performance in terms of MAPE. After applying federated learning, all models, including GRU, LSTM, and BiLSTM, show improvements in both MAPE and RMSE. Given the small fluctuations in MAPE and RMSE values as training approaches convergence, the FLSTM-HE(256) and FLSTM-HE(512) models, which incorporate homomorphic encryption, achieve prediction accuracies similar to that of Fed-LSTM. The homomorphic encryption process does not enhance or degrade the model's predictive performance. Among the various models, the Fed-GRU model yields the best prediction performance, while the BiLSTM model performs the worst. Notably, BiLSTM experiences the most significant performance improvement after the introduction of federated learning. From the results, it can be observed that clients with poor prediction performance (MAPE greater than 1% or RMSE in the three-digit range) experienced significant improvement in their prediction outcomes after participating in federated training. Although the performance of the clients with the best prediction accuracy slightly deteriorated after federation, the average prediction performance across all clients improved.
The experimental results for Case 2, presented in Tables S3 and S4 (Supplementary), lead to conclusions similar to those observed in Case 1. Specifically, all models demonstrate improvements in MAPE and RMSE after federated learning, with the homomorphic encryption process not negatively impacting the model's predictive performance. GRU continues to exhibit the best performance. A notable difference is that BiLSTM shows the worst performance both in local training and federated training. The predictive performance of FLSTM-HE models remains in the middle tier.
In the conducted experiments, it was observed that GRU outperformed LSTM, while LSTM was found to achieve better performance than BiLSTM on the provided dataset. This outcome might be considered counterintuitive, given the theoretical advantages attributed to LSTM and BiLSTM in capturing long-term dependencies and bidirectional context. However, this phenomenon can be primarily attributed to the scale of the problem and the size of the dataset. For smaller-scale prediction tasks with limited data, GRU, characterized by its fewer parameters and simpler architecture, is often more suitable and less prone to overfitting when compared to LSTM and BiLSTM. Conversely, for more complex and larger-scale problems, where the capturing of long-term dependencies and bidirectional context is critical, the use of LSTM or BiLSTM networks remains recommended for prediction.
To evaluate the impact of homomorphic encryption on training time, the runtime of networks trained using GRU or BiLSTM, as well as the FLSTM-HE model with LSTM, was recorded and compared under different key lengths in a federated environment in Table 3. Since federated training was locally simulated, the runtime divided by the number of clients provides an approximate estimate of the average training time per client. Table 3 presents the average time required for each client to complete 100 training epochs for different models. In the experiments, homomorphic encryption was selectively applied to the first effective layer of the model and the last fully connected layer. This reduced the scale of encryption and decryption operations, mitigating their impact on training time. However, the time required for encrypting the full set of parameters can be estimated based on the ratio of the number of parameters processed to the total number of parameters. For the BiLSTM and LSTM networks, approximately 50.57% of the parameters were processed, while for the GRU network, this proportion was about 50.72%.
Case | Model | Federated | HE (256-bit) | HE (512-bit) |
Case 1 | LSTM | 212.04 | 218.18 | 235.60 |
GRU | 202.13 | 213.42 | 213.22 | |
BiLSTM | 330.78 | 351.12 | 372.80 | |
Case 2 | LSTM | 207.81 | 213.43 | 230.86 |
GRU | 201.35 | 209.94 | 224.00 | |
BiLSTM | 334.54 | 350.52 | 354.08 |
It can be observed that the introduction of homomorphic encryption (which requires encrypting and decrypting model parameters) increases the training time. For a 256-bit key length, encrypting half of the parameters with homomorphic encryption resulted in a training time increase of approximately 5%–6%. The use of longer keys offers higher security but also slows down the encryption and decryption processes, further extending the training time. The training time of GRU is not highly sensitive to changes in key length, as the amount of encrypted parameters is relatively small, and the computational cost of encryption may be much smaller than that of the model training itself. In this case, the additional encryption overhead (e.g., 512-bit vs. 256-bit) is overshadowed by other operations in the training process, such as gradient computation and backpropagation, resulting in insignificant time differences. According to Table 3 and previous predictions, BiLSTM is the least recommended network architecture, as its network parameter count is approximately twice that of LSTM under the same structure. Its training and parameter encryption/decryption process is more time-consuming without a clear improvement in predictive performance. It was also found that shorter key lengths may lead to decryption conflicts. Therefore, the determination of the optimal key length still requires a comprehensive consideration of factors such as the number of model parameters, device performance, and security requirements.
This paper proposes FLSTM-HE, a secure federated learning method designed for short-term load forecasting, aimed at addressing non-independent and identically distributed (non-IID) data across different regions.FLSTM-HE integrates federated learning with LSTM networks to develop a privacy-preserving general model that accurately predicts short-term load using multi-source historical data. To further enhance data privacy, the Paillier homomorphic encryption scheme is applied to encrypt the model weights during the federated learning process, after which federated averaging is performed on the encrypted weights by the server.Experimental results demonstrate that the federated process significantly improves average performance compared to local training models. The performance and time cost differences between GRU, BiLSTM, and the selected LSTM networks are also evaluated. It is found that the GRU network excels in smaller problem scale and the LSTM network offers more balanced performance, while the performance improvement of the BiLSTM network is not justified by the significantly increased time consumption.Selective encryption of model parameters and the appropriate key length have an acceptable impact on training time, although longer keys result in increased execution time without improving predictive accuracy.
However, several limitations were identified in the study. First, although the FLSTM-HE framework provides a solid privacy-preserving mechanism, the encryption process still incurs a noticeable overhead, particularly with large models and longer key lengths, which could hinder scalability in real-world applications. Additionally, while the federated process improves average performance, its ability to generalize across regions with significantly different characteristics could be limited, requiring further tuning or region-specific adaptations.
Another limitation is the complexity of handling non-IID data in federated learning. The current model assumes equal contributions from all participating clients, but the impact of data heterogeneity between clients may lead to suboptimal performance in some regions, especially when dealing with extreme outliers or imbalanced data distributions. Moreover, while LSTM networks are effective in capturing temporal dependencies, they may not be optimal for all forecasting tasks, particularly those involving more complex patterns or longer temporal dependencies. In such cases, alternative models like Transformer-based networks may offer superior performance.
To address these limitations, future research will focus on enhancing the scalability and efficiency of the encryption process, such as exploring alternative encryption schemes or optimizing the encryption strategy to strike a balance between privacy and performance. Additionally, advanced techniques for handling non-IID data, including more advanced aggregation methods or local model adjustments, will be investigated. Finally, further evaluation of alternative neural network architectures, such as Transformer or hybrid models, will be conducted to better capture complex temporal dependencies and improve generalization across diverse regions.
The authors declare that no Artificial Intelligence (AI) tools were used in the creation of this article.
Mengdi Wang: Methodology, Writing original draft; Rui Xin: Software, Resources; Mingrui Xia: Visualization, Methodology, Writing original draft; Zhifeng Zuo: Data curation, Formal Analysis, Validation; Yinyin Ge: Supervision, Review and editing; Pengfei Zhang: Data curation, Software; Hongxing Ye: Methodology, Review and editing. All authors reviewed the results and approved the final version of the manuscripts.
This research work was supported by the project "Key Technologies and Applications of Modeling and Interaction for Multi-source Heterogeneous Energy Data" (Grant Number: kj2023-040).
The authors declare that there are no conflicts of interest related to this study.
Table S1 (A): Local LSTM and Fed-LSTM | ||||
Client | LSTM | Fed-LSTM | ||
MAPE | RMSE | MAPE | RMSE | |
Client 1 | 0.2325% | 23.1387 | 0.2233% | 23.3625 |
Client 2 | 0.1844% | 31.1696 | 0.1760% | 31.8089 |
Client 3 | 2.8383% | 150.2692 | 2.3123% | 121.4179 |
Client 4 | 0.1485% | 18.0169 | 0.5966% | 59.1193 |
Client 5 | 0.5717% | 396.6378 | 0.2366% | 202.2870 |
Client 6 | 0.2860% | 16.5528 | 0.3580% | 10.9219 |
Client 7 | 0.1448% | 117.4673 | 0.2547% | 127.5440 |
Client 8 | 1.5898% | 1041.2959 | 0.9762% | 696.1238 |
Client 9 | 0.6613% | 396.3458 | 0.5523% | 334.2896 |
Client 10 | 0.4472% | 33.0383 | 0.4237% | 22.2868 |
Client 11 | 0.2019% | 16.7447 | 0.3278% | 24.8086 |
Client 12 | 1.1186% | 28.4903 | 0.5314% | 15.1418 |
Client 13 | 1.4506% | 77.1825 | 0.4860% | 25.5178 |
Average | 0.7596% | 180.4885 | 0.5735% | 130.3562 |
Table S1 (B): FLSTM-HE(256) and FLSTM-HE(512) | ||||
Client | FLSTM-HE(256) | FLSTM-HE(512) | ||
MAPE | RMSE | MAPE | RMSE | |
Client 1 | 0.1667% | 19.6133 | 0.1748% | 19.4319 |
Client 2 | 0.2730% | 40.3732 | 0.2329% | 35.4805 |
Client 3 | 2.8659% | 142.7374 | 2.8026% | 142.6028 |
Client 4 | 0.1577% | 19.3684 | 0.1749% | 20.5443 |
Client 5 | 0.1643% | 147.4042 | 0.2818% | 211.1265 |
Client 6 | 0.4122% | 10.4988 | 0.3562% | 11.9746 |
Client 7 | 0.5353% | 223.7397 | 0.2470% | 127.4703 |
Client 8 | 0.7879% | 565.5097 | 0.5473% | 478.6953 |
Client 9 | 0.5073% | 302.9005 | 0.6878% | 422.2732 |
Client 10 | 0.4932% | 23.6366 | 0.7066% | 24.7226 |
Client 11 | 0.2357% | 20.1453 | 0.2482% | 20.0405 |
Client 12 | 0.4467% | 13.7034 | 0.6752% | 15.9437 |
Client 13 | 0.6742% | 31.6844 | 0.8555% | 40.3021 |
Average | 0.5939% | 120.1012 | 0.6147% | 120.8160 |
Table S2 (A): GRU and Fed-GRU | ||||
Client | GRU | Fed-GRU | ||
MAPE | RMSE | MAPE | RMSE | |
Client 1 | 0.1751% | 18.6925 | 0.3272% | 29.8447% |
Client 2 | 0.1344% | 26.8069 | 0.1584% | 29.5466% |
Client 3 | 0.7282% | 58.5978 | 0.4634% | 34.9118% |
Client 4 | 0.1850% | 19.6758 | 0.2239% | 22.8825% |
Client 5 | 0.1819% | 153.2923 | 0.2381% | 185.6571% |
Client 6 | 0.5318% | 11.9436 | 0.2685% | 7.5703% |
Client 7 | 0.1512% | 109.1369 | 0.2668% | 125.0506% |
Client 8 | 0.8736% | 604.7980 | 0.5238% | 344.1825% |
Client 9 | 0.6193% | 386.2367 | 0.4033% | 298.7554% |
Client 10 | 0.3977% | 22.7749 | 0.3100% | 17.8434% |
Client 11 | 0.1854% | 16.9627 | 0.1409% | 14.6296% |
Client 12 | 0.5906% | 15.2100 | 0.3815% | 10.8727% |
Client 13 | 0.9364% | 43.0627 | 0.5568% | 28.4600% |
Average | 0.4377% | 114.3993 | 0.3278% | 88.4775 |
Table S2 (B): BiLSTM and Fed-BiLSTM | ||||
Client | BiLSTM | Fed-BiLSTM | ||
MAPE | RMSE | MAPE | RMSE | |
Client 1 | 0.1716% | 19.8646 | 0.4930% | 47.1430 |
Client 2 | 0.2310% | 35.9470 | 0.1951% | 32.7464 |
Client 3 | 4.2433% | 236.3445 | 0.6401% | 43.4335 |
Client 4 | 0.1435% | 17.2590 | 0.3029% | 30.5922 |
Client 5 | 0.5037% | 346.2471 | 0.1726% | 150.2722 |
Client 6 | 0.3334% | 14.4913 | 0.2774% | 10.6495 |
Client 7 | 0.1471% | 124.3472 | 0.3177% | 153.2703 |
Client 8 | 0.9644% | 759.4873 | 0.7425% | 510.4865 |
Client 9 | 0.6829% | 395.2840 | 0.7730% | 392.5124 |
Client 10 | 0.6289% | 29.8619 | 0.6500% | 22.0328 |
Client 11 | 0.1554% | 14.3481 | 0.1968% | 17.6014 |
Client 12 | 0.9305% | 24.2320 | 0.5372% | 12.5902 |
Client 13 | 2.6246% | 175.3640 | 0.4328% | 23.0195 |
Average | 0.9046% | 168.6984 | 0.4409% | 111.2577 |
Table S3 (A): Local LSTM and Fed-LSTM | ||||
Client | LSTM | Fed-LSTM | ||
MAPE | RMSE | MAPE | RMSE | |
Client 1 | 0.8933% | 91.8592 | 0.5582% | 66.5811 |
Client 2 | 0.1983% | 28.9979 | 0.1831% | 28.6004 |
Client 3 | 0.4333% | 29.4944 | 0.3372% | 26.5155 |
Client 4 | 0.5612% | 16.1806 | 0.4890% | 13.7488 |
Client 5 | 0.6090% | 497.3193 | 0.8028% | 478.8105 |
Client 6 | 0.4780% | 323.2274 | 0.3391% | 275.4304 |
Client 7 | 0.3737% | 322.2883 | 0.4444% | 309.5906 |
Client 8 | 0.8951% | 69.5754 | 0.5822% | 52.9961 |
Client 9 | 0.6573% | 18.3372 | 0.8381% | 18.3289 |
Average | 0.5666% | 155.2533 | 0.5082% | 141.1718 |
Table S3 (B): FLSTM-HE(256) and FLSTM-HE(512) | ||||
Client | FLSTM-HE(256) | FLSTM-HE(512) | ||
MAPE | RMSE | MAPE | RMSE | |
Client 1 | 0.4849% | 62.2806 | 0.6437% | 68.8114 |
Client 2 | 0.1354% | 24.2656 | 0.1417% | 26.8485 |
Client 3 | 0.8521% | 49.5982 | 0.7063% | 39.2113 |
Client 4 | 0.6226% | 13.4930 | 0.5155% | 12.9916 |
Client 5 | 0.8147% | 488.0237 | 0.7119% | 450.2046 |
Client 6 | 0.3078% | 251.3012 | 0.3839% | 299.5142 |
Client 7 | 0.3420% | 283.5113 | 0.3898% | 294.3805 |
Client 8 | 0.6578% | 54.6524 | 0.4650% | 43.6366 |
Client 9 | 0.4621% | 15.6251 | 0.9675% | 20.0858 |
Average | 0.5199% | 138.0835 | 0.5473% | 139.5205 |
Table S4 (A): GRU and Fed-GRU | ||||
Client | GRU | Fed-GRU | ||
MAPE | RMSE | MAPE | RMSE | |
Client 1 | 0.4791% | 67.0230 | 0.3144% | 54.9000 |
Client 2 | 0.1769% | 27.3447 | 0.1085% | 22.2134 |
Client 3 | 0.5130% | 28.8636 | 0.2384% | 19.1360 |
Client 4 | 0.5403% | 15.4172 | 0.3154% | 11.7407 |
Client 5 | 0.7499% | 493.1271 | 0.6723% | 423.8349 |
Client 6 | 0.2514% | 210.8726 | 0.3331% | 268.8265 |
Client 7 | 0.3728% | 306.9759 | 0.4727% | 317.6952 |
Client 8 | 0.6045% | 50.3738 | 0.4756% | 39.0038 |
Client 9 | 0.7527% | 18.0678 | 0.5325% | 16.5049 |
Average | 0.4934% | 135.3407 | 0.3848% | 130.4284 |
Table S4 (B): BiLSTM and Fed-BiLSTM | ||||
Client | BiLSTM | Fed-BiLSTM | ||
MAPE | RMSE | MAPE | RMSE | |
Client 1 | 0.7849% | 83.8100 | 0.3769% | 57.2107 |
Client 2 | 0.1727% | 26.8629 | 0.1410% | 25.9918 |
Client 3 | 0.7483% | 42.8635 | 1.022% | 59.4328 |
Client 4 | 0.4853% | 16.4762 | 0.6795% | 14.7664 |
Client 5 | 0.8518% | 538.8270 | 0.6514% | 412.0954 |
Client 6 | 0.5811% | 431.4168 | 0.5684% | 402.5654 |
Client 7 | 0.4173% | 330.0225 | 0.3749% | 303.6855 |
Client 8 | 0.7536% | 56.1534 | 0.7581% | 56.2989 |
Client 9 | 0.6407% | 16.2917 | 0.5149% | 14.9171 |
Average | 0.6040% | 171.4138 | 0.5652% | 149.6627 |
[1] |
Akhtar S, Shahzad S, Zaheer A, et al. (2023) Short-Term load forecasting models: A review of challenges, progress, and the road ahead. Energies 16: 4060. https://doi.org/10.3390/en16104060 doi: 10.3390/en16104060
![]() |
[2] | ENTSO-E (2021) Enhanced load forecasting. Available from: https://www.entsoe.eu/Technopedia/techsheets/enhanced-load-forecasting. |
[3] | Lis R, Vanin A, Kotelnikova A (2017) Methods of training of neural networks for short term load forecasting in smart grids. Comput Collect Intell, 433–441. https://doi.org/10.1007/978-3-319-67074-4_42 |
[4] |
Hsiao Y-H (2015) Household electricity demand forecast based on context information and user daily schedule analysis from meter data. IEEE Trans Ind Inf 11: 33–43. https://doi.org/10.1109/TII.2014.2363584 doi: 10.1109/TII.2014.2363584
![]() |
[5] |
Lusis P, Khalilpour KR, Andrew L, et al. (2017) Short-term residential load forecasting: Impact of calendar effects and forecast granularity. Appl Energy 205: 654–669. https://doi.org/10.1016/j.apenergy.2017.07.114 doi: 10.1016/j.apenergy.2017.07.114
![]() |
[6] |
Li L, Fan Y, Tse M, et al. (2020) A review of applications in federated learning. Comput Ind Eng 149: 106854. https://doi.org/10.1016/j.cie.2020.106854 doi: 10.1016/j.cie.2020.106854
![]() |
[7] | Taïk A, Cherkaoui S (2020) Electrical load forecasting using edge computing and federated learning. ICC 2020—2020 IEEE International Conference on Communications (ICC), 1–6. https://doi.org/10.1109/ICC40277.2020.9148937 |
[8] |
Gholizadeh N, Musilek P (2022) Federated learning with hyperparameter-based clustering for electrical load forecasting. Int Things 17: 100470. https://doi.org/10.1016/j.iot.2021.100470 doi: 10.1016/j.iot.2021.100470
![]() |
[9] |
Briggs C, Fan Z, Andras P (2022) Federated learning for short-term residential load forecasting. IEEE Open Access J Power Energy 9: 573–583. https://doi.org/10.1109/OAJPE.2022.32062200 doi: 10.1109/OAJPE.2022.32062200
![]() |
[10] |
Wang Y, Gao N, Hug G (2023) Personalized federated learning for individual consumer load forecasting. CSEE J Power Energy Syst 9: 326–330. https://doi.org/10.17775/CSEEJPES.2021.07350 doi: 10.17775/CSEEJPES.2021.07350
![]() |
[11] |
Acar A, Aksu H, Uluagac AS, et al. (2018) A survey on homomorphic encryption schemes: Theory and implementation. ACM Comput Surv (CSUR) 51: 79. https://doi.org/10.1145/3214303 doi: 10.1145/3214303
![]() |
[12] | Fan Y, Chen Z, Zhai Z, et al. (2024) Distributed power load federated prediction for high-energy-consuming enterprises based on homomorphic encryption. 43rd Chinese Control Conference (CCC), 2876–2883. https://doi.org/10.23919/CCC63176.2024.10662589 |
[13] |
Wang H, Zhao Y, He S, et al. (2024) Federated learning-based privacy-preserving electricity load forecasting scheme in edge computing scenario. Int J Commun Syst 37: e5670. https://doi.org/10.1002/dac.5670 doi: 10.1002/dac.5670
![]() |
[14] |
Fang C, Guo Y, Ma J, et al. (2022) A privacy-preserving and verifiable federated learning method based on blockchain. Comput Commun 186: 1–11. https://doi.org/10.1016/j.comcom.2022.01.002 doi: 10.1016/j.comcom.2022.01.002
![]() |
[15] |
Paillier P (1999) Public-Key cryptosystems based on composite degree residuosity classes. Lect Notes Comput Sci 1592: 223–238. https://doi.org/10.1007/3-540-48910-X_16 doi: 10.1007/3-540-48910-X_16
![]() |
[16] | Jin W, Yao Y, Han S, et al. (2023) FedML-HE: An efficient homomorphic-encryption-based privacy-preserving federated learning system. Comput Sci. https://doi.org/10.48550/arXiv.2303.10837 |
[17] | Reddy GP, Pavan Kumar YV (2023) A beginner's guide to federated learning. 2023 Intell Methods, Syst, Appl (IMSA), 557–562. https://doi.org/10.1109/IMSA58542.2023.10217383 |
[18] |
Sheller MJ, Edwards B, Reina GA, et al. (2020) Federated learning in medicine: Facilitating multi-institutional collaborations without sharing patient data. Sci Rep 10: 12598. https://doi.org/10.1038/s41598-020-69250-1 doi: 10.1038/s41598-020-69250-1
![]() |
[19] |
Sadilek A, Liu L, Nguyen D, et al. (2021) Privacy-first health research with federated learning. npj Digital Med 4: 132. https://doi.org/10.1038/s41746-021-00489-2 doi: 10.1038/s41746-021-00489-2
![]() |
[20] |
Ng D, Lan X, Yao MMS, et al. (2021) Federated learning: A collaborative effort to achieve better medical imaging models for individual sites that have small labelled datasets. Quant Imaging Med Surg 11: 852–857. https://doi.org/10.21037/qims-20-595 doi: 10.21037/qims-20-595
![]() |
[21] |
Wang S, Tuor T, Salonidis T, et al. (2019) Adaptive federated learning in resource constrained edge computing systems. IEEE J Sel Areas Commun 37: 1205–1221. https://doi.org/10.1109/JSAC.2019.2904348 doi: 10.1109/JSAC.2019.2904348
![]() |
[22] | Wang K, He Q, Chen F, et al. (2023) FedEdge: Accelerating edge-assisted federated learning. Proceedings of the ACM Web Conference 2023, 2895–2904. https://doi.org/10.1145/3543507.3583264 |
[23] |
Al-Selwi SM, Hassan MF, Abdulkadir SJ, et al. (2024) RNN-LSTM: From applications to modeling techniques and beyond—Systematic review. J King Saud Univ—Comput Inf Sci 36: 102068. https://doi.org/10.1016/j.jksuci.2024.102068 doi: 10.1016/j.jksuci.2024.102068
![]() |
[24] |
Lindemann B, Müller T, Vietz H, et al. (2021) A survey on long short-term memory networks for time series prediction. Procedia CIRP 99: 650–655. https://doi.org/10.1016/j.procir.2021.03.088 doi: 10.1016/j.procir.2021.03.088
![]() |
[25] |
Kong W, Dong ZY, Jia Y, et al. (2017) Short-term residential load forecasting based on LSTM recurrent neural network. IEEE Trans Smart Grid 10: 841–851. https://doi.org/10.1109/TSG.2017.2753802 doi: 10.1109/TSG.2017.2753802
![]() |
[26] |
Yu Y, Si X, Hu C, et al. (2019) A review of recurrent neural networks: LSTM cells and network architectures. Neural Comput 31: 1235–1270. https://doi.org/10.1162/neco_a_01199 doi: 10.1162/neco_a_01199
![]() |
[27] |
Rafi SH, Nahid-Al-Masood, Deeba SR, et al. (2021) A short-term load forecasting method using integrated CNN and LSTM network. IEEE Access 9: 32436–32448. https://doi.org/10.1109/ACCESS.2021.3060654 doi: 10.1109/ACCESS.2021.3060654
![]() |
[28] |
Alhussein M, Aurangzeb K, Haider SI (2020) Hybrid CNN-LSTM model for short-term individual household load forecasting. IEEE Access 8: 180544–180557. https://doi.org/10.1109/ACCESS.2020.3028281 doi: 10.1109/ACCESS.2020.3028281
![]() |
[29] | Cheon JH, Costache A, Moreno RC, et al. (2021) Introduction to homomorphic encryption and schemes. In: Lauter K, Dai W, Laine K, Editors Prot Privacy Homomorphic Encryption, 3–28. https://doi.org/10.1007/978-3-030-77287-1_1 |
[30] |
Zhang L, Xu J, Vijayakumar P, et al. (2022) Homomorphic encryption-based privacy-preserving federated learning in IoT-Enabled healthcare system. IEEE Trans Network Sci Eng 10: 2864–2880. https://doi.org/10.1109/TNSE.2022.3185327 doi: 10.1109/TNSE.2022.3185327
![]() |
[31] |
Hatip A, Zayood K, Scharif R, et al. (2024) Enhancing security and privacy in IoT-based learning with homomorphic encryption. Int J Wireless Ad Hoc Commun 8: 08–08. https://doi.org/10.54216/IJWAC.080101 doi: 10.54216/IJWAC.080101
![]() |
[32] |
Kachouh B, Hariss K, Sliman L, et al. (2021) Privacy preservation of genome data analysis using homomorphic encryption. Serv Oriented Comput Appl 15: 273–287. https://doi.org/10.1007/s11761-021-00326-0 doi: 10.1007/s11761-021-00326-0
![]() |
[33] |
Basuki AI, Setiawan I, Rosiyadi D (2022) Preserving privacy for blockchain-driven image watermarking using fully homomorphic encryption. Proceedings of the 2021 International Conference on Computer, Control, Informatics and Its Applications 21: 51–155. https://doi.org/10.1145/3489088.3489130 doi: 10.1145/3489088.3489130
![]() |
[34] | Gadiwala H, Bavani R, Panchal R, et al. (2024) Enabling privacy-preserving machine learning: Federated learning with homomorphic encryption. Proceedings of the 2024 10th International Conference on Smart Computing and Communication (ICSCC), 311–317. https://doi.org/10.1109/ICSCC62041.2024.10690391 |
[35] |
Mohialden YM, Hussien NM, Salman SA, et al. (2023) Secure federated learning with a homomorphic encryption model. Int J Papier Adv Sci Rev 4: 1–7. https://doi.org/10.47667/ijpasr.v4i3.235 doi: 10.47667/ijpasr.v4i3.235
![]() |
[36] |
Wiese F, Schlecht I, Bunke W-D, et al. (2018) Open power system data—Frictionless data for electricity system modelling. Appl Energy 236: 401–409. https://doi.org/10.1016/j.apenergy.2018.11.097 doi: 10.1016/j.apenergy.2018.11.097
![]() |
[37] | Kingma DP, Ba J (2014) Adam: A method for stochastic optimization. Comput Sci. https://doi.org/10.48550/arXiv.1412.6980 |
Client | Case 1 | Case 2 |
Client 1 | Austria | Austria |
Client 2 | Belgium | Belgium |
Client 3 | Bulgaria | Bulgaria |
Client 4 | Czech Republic | Estonia |
Client 5 | Germany | Spain |
Client 6 | Estonia | France |
Client 7 | Spain | United Kingdom |
Client 8 | France | Portugal |
Client 9 | United Kingdom | Slovenia |
Client 10 | Lithuania | — |
Client 11 | Portugal | — |
Client 12 | Slovenia | — |
Client 13 | Slovakia | — |
Hyperparameter | Value | Description |
Learning Rate | 0.001 | Initial learning rate for the optimizer |
Batch Size | 32 | Number of samples per training batch |
Epochs | 100 | Total number of training iterations |
Optimizer | Adam | Optimization algorithm used |
Dropout Rate | 1e-4 | Dropout probability for regularization |
Weight Decay | 0.1 | Decay in every 10 training epochs |
Case | Model | Federated | HE (256-bit) | HE (512-bit) |
Case 1 | LSTM | 212.04 | 218.18 | 235.60 |
GRU | 202.13 | 213.42 | 213.22 | |
BiLSTM | 330.78 | 351.12 | 372.80 | |
Case 2 | LSTM | 207.81 | 213.43 | 230.86 |
GRU | 201.35 | 209.94 | 224.00 | |
BiLSTM | 334.54 | 350.52 | 354.08 |
Table S1 (A): Local LSTM and Fed-LSTM | ||||
Client | LSTM | Fed-LSTM | ||
MAPE | RMSE | MAPE | RMSE | |
Client 1 | 0.2325% | 23.1387 | 0.2233% | 23.3625 |
Client 2 | 0.1844% | 31.1696 | 0.1760% | 31.8089 |
Client 3 | 2.8383% | 150.2692 | 2.3123% | 121.4179 |
Client 4 | 0.1485% | 18.0169 | 0.5966% | 59.1193 |
Client 5 | 0.5717% | 396.6378 | 0.2366% | 202.2870 |
Client 6 | 0.2860% | 16.5528 | 0.3580% | 10.9219 |
Client 7 | 0.1448% | 117.4673 | 0.2547% | 127.5440 |
Client 8 | 1.5898% | 1041.2959 | 0.9762% | 696.1238 |
Client 9 | 0.6613% | 396.3458 | 0.5523% | 334.2896 |
Client 10 | 0.4472% | 33.0383 | 0.4237% | 22.2868 |
Client 11 | 0.2019% | 16.7447 | 0.3278% | 24.8086 |
Client 12 | 1.1186% | 28.4903 | 0.5314% | 15.1418 |
Client 13 | 1.4506% | 77.1825 | 0.4860% | 25.5178 |
Average | 0.7596% | 180.4885 | 0.5735% | 130.3562 |
Table S1 (B): FLSTM-HE(256) and FLSTM-HE(512) | ||||
Client | FLSTM-HE(256) | FLSTM-HE(512) | ||
MAPE | RMSE | MAPE | RMSE | |
Client 1 | 0.1667% | 19.6133 | 0.1748% | 19.4319 |
Client 2 | 0.2730% | 40.3732 | 0.2329% | 35.4805 |
Client 3 | 2.8659% | 142.7374 | 2.8026% | 142.6028 |
Client 4 | 0.1577% | 19.3684 | 0.1749% | 20.5443 |
Client 5 | 0.1643% | 147.4042 | 0.2818% | 211.1265 |
Client 6 | 0.4122% | 10.4988 | 0.3562% | 11.9746 |
Client 7 | 0.5353% | 223.7397 | 0.2470% | 127.4703 |
Client 8 | 0.7879% | 565.5097 | 0.5473% | 478.6953 |
Client 9 | 0.5073% | 302.9005 | 0.6878% | 422.2732 |
Client 10 | 0.4932% | 23.6366 | 0.7066% | 24.7226 |
Client 11 | 0.2357% | 20.1453 | 0.2482% | 20.0405 |
Client 12 | 0.4467% | 13.7034 | 0.6752% | 15.9437 |
Client 13 | 0.6742% | 31.6844 | 0.8555% | 40.3021 |
Average | 0.5939% | 120.1012 | 0.6147% | 120.8160 |
Table S2 (A): GRU and Fed-GRU | ||||
Client | GRU | Fed-GRU | ||
MAPE | RMSE | MAPE | RMSE | |
Client 1 | 0.1751% | 18.6925 | 0.3272% | 29.8447% |
Client 2 | 0.1344% | 26.8069 | 0.1584% | 29.5466% |
Client 3 | 0.7282% | 58.5978 | 0.4634% | 34.9118% |
Client 4 | 0.1850% | 19.6758 | 0.2239% | 22.8825% |
Client 5 | 0.1819% | 153.2923 | 0.2381% | 185.6571% |
Client 6 | 0.5318% | 11.9436 | 0.2685% | 7.5703% |
Client 7 | 0.1512% | 109.1369 | 0.2668% | 125.0506% |
Client 8 | 0.8736% | 604.7980 | 0.5238% | 344.1825% |
Client 9 | 0.6193% | 386.2367 | 0.4033% | 298.7554% |
Client 10 | 0.3977% | 22.7749 | 0.3100% | 17.8434% |
Client 11 | 0.1854% | 16.9627 | 0.1409% | 14.6296% |
Client 12 | 0.5906% | 15.2100 | 0.3815% | 10.8727% |
Client 13 | 0.9364% | 43.0627 | 0.5568% | 28.4600% |
Average | 0.4377% | 114.3993 | 0.3278% | 88.4775 |
Table S2 (B): BiLSTM and Fed-BiLSTM | ||||
Client | BiLSTM | Fed-BiLSTM | ||
MAPE | RMSE | MAPE | RMSE | |
Client 1 | 0.1716% | 19.8646 | 0.4930% | 47.1430 |
Client 2 | 0.2310% | 35.9470 | 0.1951% | 32.7464 |
Client 3 | 4.2433% | 236.3445 | 0.6401% | 43.4335 |
Client 4 | 0.1435% | 17.2590 | 0.3029% | 30.5922 |
Client 5 | 0.5037% | 346.2471 | 0.1726% | 150.2722 |
Client 6 | 0.3334% | 14.4913 | 0.2774% | 10.6495 |
Client 7 | 0.1471% | 124.3472 | 0.3177% | 153.2703 |
Client 8 | 0.9644% | 759.4873 | 0.7425% | 510.4865 |
Client 9 | 0.6829% | 395.2840 | 0.7730% | 392.5124 |
Client 10 | 0.6289% | 29.8619 | 0.6500% | 22.0328 |
Client 11 | 0.1554% | 14.3481 | 0.1968% | 17.6014 |
Client 12 | 0.9305% | 24.2320 | 0.5372% | 12.5902 |
Client 13 | 2.6246% | 175.3640 | 0.4328% | 23.0195 |
Average | 0.9046% | 168.6984 | 0.4409% | 111.2577 |
Table S3 (A): Local LSTM and Fed-LSTM | ||||
Client | LSTM | Fed-LSTM | ||
MAPE | RMSE | MAPE | RMSE | |
Client 1 | 0.8933% | 91.8592 | 0.5582% | 66.5811 |
Client 2 | 0.1983% | 28.9979 | 0.1831% | 28.6004 |
Client 3 | 0.4333% | 29.4944 | 0.3372% | 26.5155 |
Client 4 | 0.5612% | 16.1806 | 0.4890% | 13.7488 |
Client 5 | 0.6090% | 497.3193 | 0.8028% | 478.8105 |
Client 6 | 0.4780% | 323.2274 | 0.3391% | 275.4304 |
Client 7 | 0.3737% | 322.2883 | 0.4444% | 309.5906 |
Client 8 | 0.8951% | 69.5754 | 0.5822% | 52.9961 |
Client 9 | 0.6573% | 18.3372 | 0.8381% | 18.3289 |
Average | 0.5666% | 155.2533 | 0.5082% | 141.1718 |
Table S3 (B): FLSTM-HE(256) and FLSTM-HE(512) | ||||
Client | FLSTM-HE(256) | FLSTM-HE(512) | ||
MAPE | RMSE | MAPE | RMSE | |
Client 1 | 0.4849% | 62.2806 | 0.6437% | 68.8114 |
Client 2 | 0.1354% | 24.2656 | 0.1417% | 26.8485 |
Client 3 | 0.8521% | 49.5982 | 0.7063% | 39.2113 |
Client 4 | 0.6226% | 13.4930 | 0.5155% | 12.9916 |
Client 5 | 0.8147% | 488.0237 | 0.7119% | 450.2046 |
Client 6 | 0.3078% | 251.3012 | 0.3839% | 299.5142 |
Client 7 | 0.3420% | 283.5113 | 0.3898% | 294.3805 |
Client 8 | 0.6578% | 54.6524 | 0.4650% | 43.6366 |
Client 9 | 0.4621% | 15.6251 | 0.9675% | 20.0858 |
Average | 0.5199% | 138.0835 | 0.5473% | 139.5205 |
Table S4 (A): GRU and Fed-GRU | ||||
Client | GRU | Fed-GRU | ||
MAPE | RMSE | MAPE | RMSE | |
Client 1 | 0.4791% | 67.0230 | 0.3144% | 54.9000 |
Client 2 | 0.1769% | 27.3447 | 0.1085% | 22.2134 |
Client 3 | 0.5130% | 28.8636 | 0.2384% | 19.1360 |
Client 4 | 0.5403% | 15.4172 | 0.3154% | 11.7407 |
Client 5 | 0.7499% | 493.1271 | 0.6723% | 423.8349 |
Client 6 | 0.2514% | 210.8726 | 0.3331% | 268.8265 |
Client 7 | 0.3728% | 306.9759 | 0.4727% | 317.6952 |
Client 8 | 0.6045% | 50.3738 | 0.4756% | 39.0038 |
Client 9 | 0.7527% | 18.0678 | 0.5325% | 16.5049 |
Average | 0.4934% | 135.3407 | 0.3848% | 130.4284 |
Table S4 (B): BiLSTM and Fed-BiLSTM | ||||
Client | BiLSTM | Fed-BiLSTM | ||
MAPE | RMSE | MAPE | RMSE | |
Client 1 | 0.7849% | 83.8100 | 0.3769% | 57.2107 |
Client 2 | 0.1727% | 26.8629 | 0.1410% | 25.9918 |
Client 3 | 0.7483% | 42.8635 | 1.022% | 59.4328 |
Client 4 | 0.4853% | 16.4762 | 0.6795% | 14.7664 |
Client 5 | 0.8518% | 538.8270 | 0.6514% | 412.0954 |
Client 6 | 0.5811% | 431.4168 | 0.5684% | 402.5654 |
Client 7 | 0.4173% | 330.0225 | 0.3749% | 303.6855 |
Client 8 | 0.7536% | 56.1534 | 0.7581% | 56.2989 |
Client 9 | 0.6407% | 16.2917 | 0.5149% | 14.9171 |
Average | 0.6040% | 171.4138 | 0.5652% | 149.6627 |
Client | Case 1 | Case 2 |
Client 1 | Austria | Austria |
Client 2 | Belgium | Belgium |
Client 3 | Bulgaria | Bulgaria |
Client 4 | Czech Republic | Estonia |
Client 5 | Germany | Spain |
Client 6 | Estonia | France |
Client 7 | Spain | United Kingdom |
Client 8 | France | Portugal |
Client 9 | United Kingdom | Slovenia |
Client 10 | Lithuania | — |
Client 11 | Portugal | — |
Client 12 | Slovenia | — |
Client 13 | Slovakia | — |
Hyperparameter | Value | Description |
Learning Rate | 0.001 | Initial learning rate for the optimizer |
Batch Size | 32 | Number of samples per training batch |
Epochs | 100 | Total number of training iterations |
Optimizer | Adam | Optimization algorithm used |
Dropout Rate | 1e-4 | Dropout probability for regularization |
Weight Decay | 0.1 | Decay in every 10 training epochs |
Case | Model | Federated | HE (256-bit) | HE (512-bit) |
Case 1 | LSTM | 212.04 | 218.18 | 235.60 |
GRU | 202.13 | 213.42 | 213.22 | |
BiLSTM | 330.78 | 351.12 | 372.80 | |
Case 2 | LSTM | 207.81 | 213.43 | 230.86 |
GRU | 201.35 | 209.94 | 224.00 | |
BiLSTM | 334.54 | 350.52 | 354.08 |
Table S1 (A): Local LSTM and Fed-LSTM | ||||
Client | LSTM | Fed-LSTM | ||
MAPE | RMSE | MAPE | RMSE | |
Client 1 | 0.2325% | 23.1387 | 0.2233% | 23.3625 |
Client 2 | 0.1844% | 31.1696 | 0.1760% | 31.8089 |
Client 3 | 2.8383% | 150.2692 | 2.3123% | 121.4179 |
Client 4 | 0.1485% | 18.0169 | 0.5966% | 59.1193 |
Client 5 | 0.5717% | 396.6378 | 0.2366% | 202.2870 |
Client 6 | 0.2860% | 16.5528 | 0.3580% | 10.9219 |
Client 7 | 0.1448% | 117.4673 | 0.2547% | 127.5440 |
Client 8 | 1.5898% | 1041.2959 | 0.9762% | 696.1238 |
Client 9 | 0.6613% | 396.3458 | 0.5523% | 334.2896 |
Client 10 | 0.4472% | 33.0383 | 0.4237% | 22.2868 |
Client 11 | 0.2019% | 16.7447 | 0.3278% | 24.8086 |
Client 12 | 1.1186% | 28.4903 | 0.5314% | 15.1418 |
Client 13 | 1.4506% | 77.1825 | 0.4860% | 25.5178 |
Average | 0.7596% | 180.4885 | 0.5735% | 130.3562 |
Table S1 (B): FLSTM-HE(256) and FLSTM-HE(512) | ||||
Client | FLSTM-HE(256) | FLSTM-HE(512) | ||
MAPE | RMSE | MAPE | RMSE | |
Client 1 | 0.1667% | 19.6133 | 0.1748% | 19.4319 |
Client 2 | 0.2730% | 40.3732 | 0.2329% | 35.4805 |
Client 3 | 2.8659% | 142.7374 | 2.8026% | 142.6028 |
Client 4 | 0.1577% | 19.3684 | 0.1749% | 20.5443 |
Client 5 | 0.1643% | 147.4042 | 0.2818% | 211.1265 |
Client 6 | 0.4122% | 10.4988 | 0.3562% | 11.9746 |
Client 7 | 0.5353% | 223.7397 | 0.2470% | 127.4703 |
Client 8 | 0.7879% | 565.5097 | 0.5473% | 478.6953 |
Client 9 | 0.5073% | 302.9005 | 0.6878% | 422.2732 |
Client 10 | 0.4932% | 23.6366 | 0.7066% | 24.7226 |
Client 11 | 0.2357% | 20.1453 | 0.2482% | 20.0405 |
Client 12 | 0.4467% | 13.7034 | 0.6752% | 15.9437 |
Client 13 | 0.6742% | 31.6844 | 0.8555% | 40.3021 |
Average | 0.5939% | 120.1012 | 0.6147% | 120.8160 |
Table S2 (A): GRU and Fed-GRU | ||||
Client | GRU | Fed-GRU | ||
MAPE | RMSE | MAPE | RMSE | |
Client 1 | 0.1751% | 18.6925 | 0.3272% | 29.8447% |
Client 2 | 0.1344% | 26.8069 | 0.1584% | 29.5466% |
Client 3 | 0.7282% | 58.5978 | 0.4634% | 34.9118% |
Client 4 | 0.1850% | 19.6758 | 0.2239% | 22.8825% |
Client 5 | 0.1819% | 153.2923 | 0.2381% | 185.6571% |
Client 6 | 0.5318% | 11.9436 | 0.2685% | 7.5703% |
Client 7 | 0.1512% | 109.1369 | 0.2668% | 125.0506% |
Client 8 | 0.8736% | 604.7980 | 0.5238% | 344.1825% |
Client 9 | 0.6193% | 386.2367 | 0.4033% | 298.7554% |
Client 10 | 0.3977% | 22.7749 | 0.3100% | 17.8434% |
Client 11 | 0.1854% | 16.9627 | 0.1409% | 14.6296% |
Client 12 | 0.5906% | 15.2100 | 0.3815% | 10.8727% |
Client 13 | 0.9364% | 43.0627 | 0.5568% | 28.4600% |
Average | 0.4377% | 114.3993 | 0.3278% | 88.4775 |
Table S2 (B): BiLSTM and Fed-BiLSTM | ||||
Client | BiLSTM | Fed-BiLSTM | ||
MAPE | RMSE | MAPE | RMSE | |
Client 1 | 0.1716% | 19.8646 | 0.4930% | 47.1430 |
Client 2 | 0.2310% | 35.9470 | 0.1951% | 32.7464 |
Client 3 | 4.2433% | 236.3445 | 0.6401% | 43.4335 |
Client 4 | 0.1435% | 17.2590 | 0.3029% | 30.5922 |
Client 5 | 0.5037% | 346.2471 | 0.1726% | 150.2722 |
Client 6 | 0.3334% | 14.4913 | 0.2774% | 10.6495 |
Client 7 | 0.1471% | 124.3472 | 0.3177% | 153.2703 |
Client 8 | 0.9644% | 759.4873 | 0.7425% | 510.4865 |
Client 9 | 0.6829% | 395.2840 | 0.7730% | 392.5124 |
Client 10 | 0.6289% | 29.8619 | 0.6500% | 22.0328 |
Client 11 | 0.1554% | 14.3481 | 0.1968% | 17.6014 |
Client 12 | 0.9305% | 24.2320 | 0.5372% | 12.5902 |
Client 13 | 2.6246% | 175.3640 | 0.4328% | 23.0195 |
Average | 0.9046% | 168.6984 | 0.4409% | 111.2577 |
Table S3 (A): Local LSTM and Fed-LSTM | ||||
Client | LSTM | Fed-LSTM | ||
MAPE | RMSE | MAPE | RMSE | |
Client 1 | 0.8933% | 91.8592 | 0.5582% | 66.5811 |
Client 2 | 0.1983% | 28.9979 | 0.1831% | 28.6004 |
Client 3 | 0.4333% | 29.4944 | 0.3372% | 26.5155 |
Client 4 | 0.5612% | 16.1806 | 0.4890% | 13.7488 |
Client 5 | 0.6090% | 497.3193 | 0.8028% | 478.8105 |
Client 6 | 0.4780% | 323.2274 | 0.3391% | 275.4304 |
Client 7 | 0.3737% | 322.2883 | 0.4444% | 309.5906 |
Client 8 | 0.8951% | 69.5754 | 0.5822% | 52.9961 |
Client 9 | 0.6573% | 18.3372 | 0.8381% | 18.3289 |
Average | 0.5666% | 155.2533 | 0.5082% | 141.1718 |
Table S3 (B): FLSTM-HE(256) and FLSTM-HE(512) | ||||
Client | FLSTM-HE(256) | FLSTM-HE(512) | ||
MAPE | RMSE | MAPE | RMSE | |
Client 1 | 0.4849% | 62.2806 | 0.6437% | 68.8114 |
Client 2 | 0.1354% | 24.2656 | 0.1417% | 26.8485 |
Client 3 | 0.8521% | 49.5982 | 0.7063% | 39.2113 |
Client 4 | 0.6226% | 13.4930 | 0.5155% | 12.9916 |
Client 5 | 0.8147% | 488.0237 | 0.7119% | 450.2046 |
Client 6 | 0.3078% | 251.3012 | 0.3839% | 299.5142 |
Client 7 | 0.3420% | 283.5113 | 0.3898% | 294.3805 |
Client 8 | 0.6578% | 54.6524 | 0.4650% | 43.6366 |
Client 9 | 0.4621% | 15.6251 | 0.9675% | 20.0858 |
Average | 0.5199% | 138.0835 | 0.5473% | 139.5205 |
Table S4 (A): GRU and Fed-GRU | ||||
Client | GRU | Fed-GRU | ||
MAPE | RMSE | MAPE | RMSE | |
Client 1 | 0.4791% | 67.0230 | 0.3144% | 54.9000 |
Client 2 | 0.1769% | 27.3447 | 0.1085% | 22.2134 |
Client 3 | 0.5130% | 28.8636 | 0.2384% | 19.1360 |
Client 4 | 0.5403% | 15.4172 | 0.3154% | 11.7407 |
Client 5 | 0.7499% | 493.1271 | 0.6723% | 423.8349 |
Client 6 | 0.2514% | 210.8726 | 0.3331% | 268.8265 |
Client 7 | 0.3728% | 306.9759 | 0.4727% | 317.6952 |
Client 8 | 0.6045% | 50.3738 | 0.4756% | 39.0038 |
Client 9 | 0.7527% | 18.0678 | 0.5325% | 16.5049 |
Average | 0.4934% | 135.3407 | 0.3848% | 130.4284 |
Table S4 (B): BiLSTM and Fed-BiLSTM | ||||
Client | BiLSTM | Fed-BiLSTM | ||
MAPE | RMSE | MAPE | RMSE | |
Client 1 | 0.7849% | 83.8100 | 0.3769% | 57.2107 |
Client 2 | 0.1727% | 26.8629 | 0.1410% | 25.9918 |
Client 3 | 0.7483% | 42.8635 | 1.022% | 59.4328 |
Client 4 | 0.4853% | 16.4762 | 0.6795% | 14.7664 |
Client 5 | 0.8518% | 538.8270 | 0.6514% | 412.0954 |
Client 6 | 0.5811% | 431.4168 | 0.5684% | 402.5654 |
Client 7 | 0.4173% | 330.0225 | 0.3749% | 303.6855 |
Client 8 | 0.7536% | 56.1534 | 0.7581% | 56.2989 |
Client 9 | 0.6407% | 16.2917 | 0.5149% | 14.9171 |
Average | 0.6040% | 171.4138 | 0.5652% | 149.6627 |