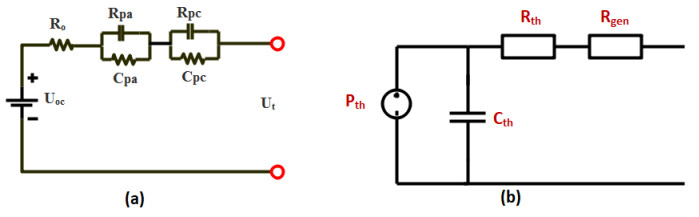
Citation: A.M.S.M.H.S.Attanayaka, J.P.Karunadasa, K.T.M.U.Hemapala. Estimation of state of charge for lithium-ion batteries - A Review[J]. AIMS Energy, 2019, 7(2): 186-210. doi: 10.3934/energy.2019.2.186
[1] | Junguang Sun, Xiaodong Zhang, Wenrui Cao, Lili Bo, Changhai Liu, Bin Wang . State of health estimation of lithium-ion batteries based on data-driven methods with a selected charging voltage interval. AIMS Energy, 2025, 13(2): 290-308. doi: 10.3934/energy.2025012 |
[2] | Xiaoyu Zheng, Dewang Chen, Yusheng Wang, Liping Zhuang . Remaining useful life indirect prediction of lithium-ion batteries using CNN-BiGRU fusion model and TPE optimization. AIMS Energy, 2023, 11(5): 896-917. doi: 10.3934/energy.2023043 |
[3] | Saad Jarid, Manohar Das . An Electro-Thermal Model based fast optimal charging strategy for Li-ion batteries. AIMS Energy, 2021, 9(5): 915-933. doi: 10.3934/energy.2021043 |
[4] | Steven B. Sherman, Zachary P. Cano, Michael Fowler, Zhongwei Chen . Range-extending Zinc-air battery for electric vehicle. AIMS Energy, 2018, 6(1): 121-145. doi: 10.3934/energy.2018.1.121 |
[5] | Eduardo Enrique Martinez Jorges, António M.N. Quintino, Diogo M.F. Santos . Economic analysis of lithium-ion battery recycling. AIMS Energy, 2023, 11(5): 960-973. doi: 10.3934/energy.2023045 |
[6] | François KREMER, Stéphane RAEL, Matthieu URBAIN . 1D electrochemical model of lithium-ion battery for a sizing methodology of thermal power plant integrated storage system. AIMS Energy, 2020, 8(5): 721-748. doi: 10.3934/energy.2020.5.721 |
[7] | Chi Van Nguyen, Thuy Nguyen Vinh . Design of energy balancing circuit for battery cells connected in series based on modifying the bidirectional CuK converter. AIMS Energy, 2022, 10(2): 219-235. doi: 10.3934/energy.2022012 |
[8] | Kritanjali Das, Santanu Sharma . Coulombic efficiency estimation technique for eco-routing in electric vehicles. AIMS Energy, 2022, 10(3): 356-374. doi: 10.3934/energy.2022019 |
[9] | Rasool M. Imran, Kadhim Hamzah Chalok . Innovative mode selective control and parameterization for charging Li-ion batteries in a PV system. AIMS Energy, 2024, 12(4): 822-839. doi: 10.3934/energy.2024039 |
[10] | K. M. S. Y. Konara, M. L. Kolhe, Arvind Sharma . Power dispatching techniques as a finite state machine for a standalone photovoltaic system with a hybrid energy storage. AIMS Energy, 2020, 8(2): 214-230. doi: 10.3934/energy.2020.2.214 |
The SOC of a battery is defined as the ratio of its current capacity Q(t) to the nominal capacity Qn which demonstrates the maximum amount of charge that can be stored in the battery [1].
SOC(t)=Q(t)/Qn | (1.1) |
Precise SOC estimation reflects some significant information such as battery performance, remaining life of battery [2] that ultimately lead to an effective management and utilization of the battery power and energy [3,4]. Furthermore SOC estimation can be used to regulate over-discharging and over-charging of the battery, which lead to a reduction in battery life, explosion or flame, accelerating aging and permanent damage to the cell structure of batteries [5]. Accurate SOC indication is thus important for the user convenience and to ensure the battery's efficiency, safety, and longevity. An accurate estimation of SOC is a fundamental consideration to eliminate failures due to thermal runaways and to regulate cell balancing [6].
Most of the SOC estimation techniques require very accurate measurements of either the battery chemical content (type of electrolyte), its operating conditions or cell variables (voltage, current) and thus are only suitable for laboratory rather than real world applications. Also a given method of SOC estimation will be more suitable or applicable for a particular application than another [7]. Furthermore the SOC of a battery is not a state that can be measured directly but estimated from available parameters such as voltage, applied current or surface temperature.
As the most improved and flexible battery technology, lithium ion batteries exhibits high power capabilities, high energy density, low self-discharge, long life cycle and high number of charge discharge cycles, low operational and maintenance requirements, satisfactory operating temperature ranges, high reliability, technological diversity as compared to other standard battery types such as lead-acid and nickel cadmium [8,9,10,11]. However, over charging or over discharging of Lithium-Ion batteries can cause permanent damage to the battery cells which may cause fire or even exploding batteries [12]. So an accurate estimation of SOC can conserve the lifetime of batteries by preventing frequent charge and discharge.
Estimating and controlling the SOC is vitally important in various power system applications such as Battery Energy Storage Systems (BESSs) and Electric Vehicles (EVs). When it comes to the high penetration of renewable energy in the distribution network, BESS is a potential solution to address the issues caused by the intermittent nature of renewable energy [13]. But the operation of the BESS is affected by dynamic disturbances such as unbalance load dynamics, the application of Electric Vehicle charging and single phase distributed generations (Solar PV). Therefore to develop a BESS incorporating such dynamic aspects and involving aspects such as modelling, simulation, development of control schemes and standard test procedures require an adequate battery model to imitate the true battery characteristics.
The most frequently employed battery models are Equivalent Circuit Battery models and Electrochemical battery models [14,15,16]. Equivalent Circuit Models (ECMs) are flexible and easy to compute compared to Electrochemical models which are complex as they consider chemical process and degradation processes in the battery employing mathematical equations (time-variant spatial partial differential equations) [15,17,18,19,20]. One of the most frequently employed Electrochemical model is Single Particle model (SPM) for which a backstepping PDE state estimator is designed. Since the SPM model captures less cell dynamics and this method requires high computational cost and time, in this paper only the ECM models are considered. Equivalent Circuit Models consists of voltage sources, resistors and capacitors to describe the electrochemical processes and dynamics of a battery [21]. Generally selected ECMs which include the Rint model, the Thevenin model (first order RC ECM), the RC model, the PNGV model and the improved Thevenin model (second order RC ECM)(see Figure. 1). Among these ECMs, the second order RC ECM consists polarization capacitors to represent the response of transient behavior (see Figure 1a).
In order to accurately estimate the SOC, battery model must be able to precisely represent the both statistic and dynamic responses. When the accuracy of the battery model increases, the computational cost and time will also increase. Therefore in regards to the compromise between the model accuracy and computational efficiency, second order RC ECM presents considerably better outcomes. Other model which are mentioned here have high computational efficiency but less accuracy. In second order Rc ECM, a voltage source represents the battery Open Circuit Voltage (OCV). Therefore to obtain an accurate battery model, it is necessary to adopt a definite SOC estimation method. There should be a compromise between the accuracy and the complexity of the SOC estimation method.
The SOC estimation algorithm is generally programmed in the Battery Management System (BMS) that regulates the energy flow in a battery pack with respect to voltages of individual cells, temperature, state of charge and state of health. The main function of BMS is to maintain a safe operating environment for the battery system, and to protect it from damages [19,23,24]. Even though the estimation of battery SOC is key function of BMS, its accuracy and online estimation is challengeable due to non-linear complex electrochemical process in the battery [25].
In this paper both traditional methods and improved methods of estimating of SOC are presented. Each method is described with its strengths and drawbacks in relation to the accuracy of the Equivalent Circuit Battery Models in imitating battery dynamic behavior.
Various experimental methods, models and algorithms, of estimating the SOC of a battery have been proposed and developed each having its own advantages and disadvantages [12]. Table 1 summarizes the various estimation methods in view point of the methodology [3,26].
Category | Method |
Direct Measurement | (ⅰ) Open Circuit Voltage (OCV) method |
(ⅱ) Terminal Voltage method | |
(ⅲ) Impedance method | |
Book keeping method | (ⅰ) Coulomb Counting method |
(ⅱ) Modified Coulomb Counting method | |
Indirect Measurement | (ⅰ) Neural Network method |
(ⅱ) Support Vector Method | |
(ⅲ) Fuzzy logic | |
(ⅳ) Kalman Filter method | |
Extended Kalman Filter method | |
Unscented Kalman Filter method | |
Sigma Point Kalman Filter | |
Hybrid methods | Coulomb Counting and Kalman filter |
Apart from these methods there are some other methods such as particle filter algorithms, non-linear observer method and reference governor method. The comparison between the methods is given by the Table 2.
Method | Advantages | Limitations |
Open Circuit Voltage (OCV) method [74,75,76] | Simple Cost effective Independent of battery models An effective method for measuring SOC only at the initial stage and end stage |
Off-line technology long rest time is required |
Coulomb Counting method [77,78,77,8] | Simple Cost effective Independent of battery models |
Small errors will accumulate due to the integration term(initial SOC) Accuracy depends on the battery age, discharge rate and sensor precision |
Neural Network method [45,79,80] | Higher accuracy Temperature effect is taken as an input |
High computational cost Time consuming A large set of data set is required for the training process Better outputs only for constant current input |
Fuzzy logic method [81,82,83] | Higher Accuracy | High computational cost Fuzzy rules are to be clearly defined The approach is based on the static characteristics of the battery (practically not applicable for BESSs) The aging of battery can not be considered |
Support Vector Machines [84,85,86] | Higher accuracy The size of the training data set is small compared to neural network method |
The obtained training data should covered the expected range of operation |
KF, EKF, UKF [87,88,89,90,91,92,93,94,95] | High computational cost High complexity Depends on sensor accuracy and battery models Numerical instabilities due to complex matrix operations |
Independence of initial SOC Higher accuracy Consideration of noise |
In direct measurement methods, to estimate SOC value physical measurements such as the voltage and impedance of the battery [3] are used. Frequently used direct measurement methods are Open Circuit Voltage (OCV) method, terminal voltage method, impedance method and impedance spectroscopy method.
Open circuit voltage(OCV) is the battery thermodynamic potential under no load condition that has a non linear relationship with SOC for a lithium ion battery [3,27]. The OCV is usually obtained through offline OCV test at definite ambient temperatures and aging stages [28]. Even though the open-circuit voltage (OCV) method is very accurate, it requires a rest time to estimate the SOC and hence difficulty to be utilized in real world applications.
OCV is present in Electrical Equivalent Circuit models (ECM) as an ideal but variable voltage source to which over-potential is added by the remaining resistor and capacitor elements of the ECM [27].
Furthermore the relationship of the OCV–SOC differs among batteries and therefore an unacceptable error can be occurred due to the usage of this varying OCV–SOC data for the SOC estimation algorithms [29]. The conventional relationship of the OCV–SOC is developed by measuring the OCV at each SOC state. The relationship differs with the variation in capacity among batteries and shows different results even if the batteries are composed with the same structures and materials. But estimating the OCV of each battery at each SOC for the validity of estimation process is a considerably time-consuming process [29].
SOC estimation can be significantly influenced by OCV hysteresis [32]. Hysteresis can be defined as the dissimilarity between the OCV of charging process and the OCV of discharging process [32]. Therefore it can be stated that the information of OCV alone is not sufficient to determine the SOC and the history of charge-discharge should also be taken into account [27].
Furthermore the hysteresis characteristics differ with type of the electrode in li-ion batteries (electrodes with lithium iron phosphate as the active material exhibit hysteresis phenomenon). In order to analyze the effect of hysteresis on battery SOC estimation or ECM parameters, hysteresis effect should be measured against battery SOC value or capacity. The OCV-SOC function is implemented either as an analytical expression or look-up table where there are a number of advantages in analytical method including data processing efficiency [33].
It can be stated that only a few researches have been carried out to determine SOC using terminal voltage method of Lithium ion batteries. The method is based on the fact that when the battery is discharged because of the internal resistance, terminal voltage falls and Electromotive Force (EMF) is equivalent to the terminal voltage [3].
In order to calculate the SOC using the impedance method, both voltage and current measurement are recorded at different excitation frequencies since the battery impedance depends on the frequency. The principle consists of injecting current in a certain range of frequencies to find impedance. The change of impedance is negligible for higher values of the SOC but when the SOC reaches a certain low SOC level, the impedance increases swiftly [3].
Among many methods, Electrochemical Impedance Spectroscopy (EIS) which brings significant information about the complex electrochemical processes occuring inside the battery is considered. Even though there are many methods of estimating SOC based on EIS, the complexity of using EIS directly is considerable high [35]. As one approach, an impedance Model is constructed on account of EIS data which is presented as a Nyquist plot where the measured impedance is plotted as the real part against the imaginary part [23]. The Nyquist plot impedance spectra is parted into three section: low frequency section, mid frequency section and high frequency section. The parameter identification is simplified due to this partition process and the SOC can be estimated using ECM model-based methods which are discussed in this paper [23].
The coulomb counting method and modified coulomb counting method are categorized as two kinds of book-keeping methods. The battery charging/discharging current is taken as the input to this method. It allows to add several battery internal effect; such as capacity-loss and self-discharge, for the SOC estimation, and also battery data such as nominal capacity. [3].
In the coulomb counting method (also known as Ampere-hour method), the SOC of a battery is calculated by cumulating the charge flowed in or out of the battery [36].The accuracy of the coulomb counting method is affected by the accuracy of initial SOC estimation and measurement of the battery current (accuracy of current sensors) [37]. The coulomb counting method is convenient for SOC estimation of lithium-ion batteries with high charging and discharging efficiencies [36] and required long time monitoring. Even though the method is not suitable for real-time SOC estimation, it can be used to verify the accuracy of the results obtained by other estimation methods.
SOC(t)=SOCo–1Crated∫idt | (2.1) |
Where SOC0 is the initial SOC value and i(t) is the current of the battery with a negative value at charge, Crated is the rated capacity. The initial SOC value (SOC0) can be obtained by OCV method. Even though the method is simple and inexpensive, the drawbacks of the method can be listed as follows [38].
Since the coulomb counter is an open loop estimator, errors in the current sensor is added by the estimator. The cumulative error becomes larger, when the SOC estimator operates through a longer time period. Also, an incorrect result can be generated faster, when the current sensor has massive errors.
When the battery ages in real time, the battery capacity varies, but the coulomb counter cannot detect or take measures for the issue. As a result, SOC estimation will not be accurate, if the real pattern of the battery estimation deviates from the expected pattern.
The initial SOC should be measured by the terminal voltage of the battery pack. So any error contained in the initial estimation method will be carried throughout the process and this method cannot detect or repair the initial error.
The Coulomb Counting method can be improved by considering the Coulombic efficiency (ηAh) at different temperature and charge rates. It is defined as the ratio between the number of charges extracted during the discharging process and the number of charges enter during the charging process or the ratio of the discharging capacity to the charging capacity [39,40] (given by the below equation).
ηAh=QdischargeQcharge×100% | (2.2) |
Since ηAh depends on the current rate (discharge or charge) as mentioned above, an Equivalent Coulombic Efficiency (ECE) (ηeq) is developed including the discharge and charge Coulombic Efficiency. In general According to that the modified Coulomb Counting equation can be depicted as below with ηeq and Ca representing the ECE and the present available capacity which differs from the rated capacity Crated due to temperature and age effect [41].
SOC(t)=SOCo–1Ca∫ηeqidt | (2.3) |
Among the different battery chemistries, li-ion batteries offer the highest Coulombic efficiency in the normal SOC region (exceeds 99%) [39,40]. But the estimation of Coulomb efficiency is difficult task as it requires highly accurate equipment.
The adaptive systems are consistent, because of their ability to deal with the nonlinearities of battery systems and show considerably excellent accuracy. Yet, in order to obtain good results from the adaptive systems, the specific information of battery characteristics or an accurate Equivalent Circuit Model (ECM) are required [42].
Neural Network is a mathematical model (subfield of artificial intelligence) that consists of interconnected artificial neurons stimulated by biological neural networks and to predict the output of a nonlinear system past data of that system is used [26]. Neural Network methods do not rely on any, physical, electrical, chemical or thermal model and take less time period to generate results compared to Extended Kalman Filter (EKF) [26,43]. Neural Network consists of inputs and outputs and is made of a number of processing units called neurons interconnected with each other. The accuracy of Neural Network method depends on how far the network is trained and the training process is the most important phase.
The most two common network architectures to estimate the SOC are the nonlinear input-output (NIO) feed-forward network and nonlinear autoregressive with exogenous input (NARX) feed-back network [26]. Figure 3 shows the structure of a feed-forward neural network [44]. In order to represent the input variables and output variables, the neural network consists of an input layer with nodes and output layer respectively. Additionally there are one or more hidden layers to simulate the nonlinearity between the input variables and output variable. The nodes between two adjacent layers are interconnected [45]. Only in the output layer and the hidden layers are the processing layers with activation functions at each nodes. There are several types of activation functions such as logistic tanh-hyperbolic tangent and ReLu-rectified linear units. In most scenarios hyperbolic tangent sigmoid function and the linear transfer function are used as functions of activation the hidden layer and the output layer respectively. As there are no theoretical criteria when it comes to selecting the number of hidden layers and the neurons, it will be done using the expertise knowledge. In regarding to the SOC estimation, the neural network method determines the SOC direct from the voltage and current without OCV-SOC look-up tables following steps can be considered as the constructive approach to the neural network SOC estimation.
1.Initialization (determination of the dimensions of the input layer and the number of neurons in the hidden layer)
2.Train the neural network with input variables (current, voltage, temperature) and output variable (SOC)
3.Error calculation between the estimated output and the actual inputs
4.If the error is within the expected level searching is ended; otherwise repeat the step 2
There are many factors that influence the SOC of the power battery [44,47,48]. Since the current, terminal voltage and temperature have the greatest influence on the SOC of the battery, these three parameters are chosen as the input of the network, the battery SOC value is chosen as the output, and the number of nodes in the hidden layer is set according to the experience [44].
Kalman filter (KF) is a recognized technique that is used as an optimal estimator to estimate the inner state of dynamic linear system. Basically, KF is a recursive set of equations that consists of two steps: prediction step which predicts the system output, system state and error [49] and correction step which corrects the current state estimate value based on system output value [26,49]. The block diagram of Kalman filtering process is shown in figure 4.
In order to design estimate SOC using Kalman filter, a battery state-space model is constructed using the equivalent circuit model. Considering the system noise and observation noise, the discrete state-space model is constructed [46]. Since the battery OCV (which is shown by a voltage source in a second order RC ECM) and SOC has a non-linear relationship and KF algorithm is only suitable for linear systems, a linearization method should be followed with an acceptable accuracy as a supplementary part. As a result of the linearization process, the discrete space-state model equation (output equation) is reduced to a less complex condition. The generalized error between the measurable value and system state variable (ex: SOC) is calculated the KF using the output equation. Then the Kalman gain is adapted to update the system variables (SOC)
Because of highly nonlinear characteristics of battery system and unsuitable battery model inaccurate outputs can be generated in KF method.
Basically, where the input is applied current, the terminal voltage is used as output, and the battery SOC is placed as the hidden state [50]. Then the hidden state is estimated by KF, EKF, Unscented Kalman Filter (UKF) or Particle Filter (PF).
But, in the EKF method, the Jacobian matrix must be constructed and if the system is highly nonlinear with non-Gaussian noise, the results may be generated with large errors [51]. But as an advantage, if there is an incorrect initial SOC value, the KF system can conquer it and could detect and represent cell aging.
Extended Kalman Filter (EKF) is the improved version KF and is used to estimate the inner state of a nonlinear dynamic system using a state-space mode [26]. Simply it predicts the future state of system based on the previous data [52]. when it comes to the estimation of SOC, it employs advanced battery cell models and requires a relatively high computation capability.
The EKF consists of two equations. One equation consists of matrices constructed using the parameters of ECM along with the system state matrices (SOC) measurable input matrices and non-measurable process noise [53,54]. The parameters will be identified performing standard test procedures. The second equation is the measurement equation which shows the output voltage in terms of system state vectors, measurable input matrices and measurement noise. Employing appropriate software tool (MATLAB simulink) SOC can be estimated.
In some EKF methods, an inner filter is established to adjust the SOC and the battery model is adjusted by an outer filter [55].
According to the SOC and the cell model, the inner filter proposes a corresponding voltage using the measured current. The SOC is adjusted by comparing the measure voltage and proposed voltage. Thus, the system feedback is voltage and its output is SOC. After monitoring the applied current and voltage over a long period of time, the outer filter gradually adjusts the parameters of the system model. In this method, the cell aging and other lifetime effects are detected and modeled in real time.
An accurate battery model must be established in order to obtain better results from the EKF method and the battery system must be treated as a nonlinear time-variant dynamic system [26]. Most common models are shepherd model, Unnewehr Model, Nernst Model, Linear Model, Thevenin model and RC Model [56].
The EKF algorithm not only can be used for online SOC estimation and track the battery state of charge parameter, but also can be employed to identify the parameter of the battery model [56].
Since SOC estimation of lithium-ion battery system inherits highly non linear characteristics [57], it can produce large errors for EKF method, because it is a first or second order Taylor series expansion to approximate the nonlinear functions [56]. Apart from the above disadvantage, EKF must compute the Jacobian matrix and if the system consists of non-Gaussian noise, the produced results may not be at an acceptable level. So Unscented Kalman Filter (UKF) has been established to improve the accuracy of KF and EKF methods. Since the UKF deals with non Gaussian noises as well and Jacobian matrix is not calculated, it more suitable for SOC estimation [51].
In this method the battery's SOC is chosen as an element of the state vector. The two sub-models in the proposed UKF methods are the process model and measurement model, which describe the relationship between the SOC and cell states such as, current, the terminal voltage and temperature.
Fuzzy logic method can be used to model, non-linear and time-varying systems without the need for mathematical models or ECMs of a battery [58]. For the estimation of SOC using Fuzzy logic method, environmental temperature, applied current and battery terminal voltage are considered as the input variable balancing the complexity and accuracy well. The higher the number of input variables (dimensions of fuzzy controller), more accurate are the results. But when the dimension is higher, the rules of fuzzy control will be much complex to implement [59,60]. As the first step, Fuzzification is the mapping from the above input with fuzzy variables using membership functions. Membership functions can be triangular-shaped or trapezoidal-shaped functions considering the memory storage and efficiency. In the second step, the relationship between the input and output is described using rule based representation of expert knowledge [61]. The third step is the reasoning mechanism that performs inference procedure. Defuzzification is the third step that converts the modified control outputs into real valued outputs. The membership functions and rule sets are defined by generating as a result of neural network algorithm or an expert. In order that the Fuzzy logic model to accurately predict the SOC of the battery without considering the initial capacity, a "training" data set are constructed [62].
In most fuzzy logic methods, the SOC value of the battery is predicted without the rated capacity or previous knowledge of the discharge history of the cell and only by measuring the imaginary component of the impedance at a few specific frequencies [63].
In most scenarios, clustering algorithms are used to construct input membership functions and rules. The output membership parameters are optimized using least square fit.
Support Vector Machines (SVM) are a set of related supervised learning methods used for regression and classification that can be generally appropriate for any multi-variable function to a higher accuracy level [56] and have been effectively applied especially in highly nonlinear systems.
In order to estimate SOC using SVM regression model temperature, current measurement and voltage are considered nonlinear input variables [64]. Using a kernel function in the SOC estimation process, a training data set of the above input variable which covers the expected range of operation should be selected [38,65]. Then the proposed SVM model is validated using the new data not used for training [66].
Particle filter (PF) is an effective nonlinear filter technique that can obtain the particles and corresponding weight values through random sampling [68]. The principle is to represent the probability densities with a set of weighted particles [69]. The accuracy of the PF algorithm depends on the experimental data and the structure of the PF. In order to estimate SOC of the battery using PF algorithm method, it is necessary to establish a discrete state-state model which consists of state equation and observation equation [70]. The state equation is derived by the SOC definition which is expressed in the coulomb-counting method. The observation equation is obtained considering the terminal voltage of the second order RC equivalent circuit as the observed valued. The parameters of the second order RC ECM is acquired through standard test procedures [71].
The collected data from charging process or discharging process data are stored as experimental data. Then to establish the state equation of the state-space model, the SOC estimation equation is discretized and considering the characteristics of collected data.
The particle filter algorithm has high accuracy for SOC estimation and the estimation error is comparatively small [72]. Using PF algorithm as an individual SOC estimation method, combination of PF and KF, PF and EKF, PF and EKF will improve the robustness of the KF algorithm while solving probability distribution function selection of PF algorithm [73].
When it comes to the BESS in microgrids with Distributed Generations (DGs), dynamic behavior (Ex: unbalance loads, unequal line impedances, phase asymmetry in branch configurations at the common coupling point of the microgrid at grid connection) of the power system has a negative impact on the battery performance [96]. So to optimize the Battery management System, the requirement of an accurate battery model for the BESS is inevitable. Due to the flexible nature and ability to represent the above mentioned dynamic situations, Equivalent Circuit Models (ECM) have been adapted by many researchers [67,96,97]. Since the battery model is the foundation for the BESS operation, the representation of ECM with appropriate model parameters will enhance the modelling of BESS. As many ECMs are established, considering the ability to represent dynamic response and less complexity of the model, the second order RC Equivalent Circuit Model can be considered as the most suitable model. Figure 1a showed the schematic diagram of second order RC ECM which consists of controllable voltage source denoting the Open Circuit Voltage (OCV) which normally varies nonlinear with SOC, RC parallel networks representing the polarization response and R0 ohmic resistance denoting the electrolyte resistance [17,21,50,98,99]. The addition of the RC network improve the representation of the dynamic behavior of the battery, but it is much complex to simulate.
Since the OCV in the second order ECM varies nonlinear with SOC, when identifying the above mentioned parameters, it is necessary to consider the battery SOC value as an input to the simulation process [22,100]. So appropriate SOC estimation method should be included for the modelling of BESS. The objective of this paper is to identify the SOC estimation approach that can be used to update the OCV (controllable voltage source) of the ECM. Also when selecting a method, the dynamic modelling of the battery must be preserved. The objective can be achieved by either adopting model-based SOC approach which calculate SOC and update OCV in the same procedure or adopting non-model based method then considering the estimated SOC as an input to the ECM. The most frequently used ECM model-based SOC techniques are Kalman Filter methods including EKF, UKF, CKF (Cubature Kalman Filter) and PF algorithm which consist of both estimator and battery model [101,102]. All other methods mentioned in this paper are non ECM-based methods which usually use physical parameters, mathematical or artificial intelligence algorithms.
The most important factor to be considered is the thermal behavior of li-ion battery and how it effects the performance of the BESS. The battery internal resistance and battery capacity are influenced by temperature distribution in terms of battery lifetime degradation or battery performance [103]. Furthermore round-trip efficiency, operation of electrochemical reactions and charge acceptance are influenced by the temperature [104]. Also higher temperature will be a cause to hazardous situations of lithium [31]. Therefore in order to maintain the battery temperature within the appropriate range, suitable cooling and heating systems whose operation depends on the battery cell surface temperature and internal temperature are required [22,105]. Since the battery SOC and ECM parameters are varied according to the temperature, those variations should be taken into account. As one approach, temperature can be considered as an input to the SOC estimation method, but the ambient temperature is not sufficient enough because it is necessary to model the heat generation and heat transfer (conductive, convective and radiation) [31] to accurately represent thermal behavior.
The most widely used thermal model is the lumped capacitor model which is shown in the Figure 1b [22,105,106]. In this model Pgen represents the heat generation inside the battery. The Rth, Rcon and Rth describe the internal resistance, the convection heat transfer and the thermal capacitance respectively. Since the thermal behaviour is represented using electrical elements, these three parameters also depends on the battery current, SOC and battery internal temperature.
Therefore coupling the thermal model that represent the above mentioned thermal phenomena with the second order RC equivalent circuit is convenient (see Figure 6).
Since the complexity of the battery model increases when coupling the ECM model and thermal model, it is insightful to acquire a less complex SOC calculation method for this objective. More importantly, typical BESS consist of buck/boost converter, DC link, inverter, AC filter and the grid [96] which are shown by the Figure 5. The analysis of different operation conditions (grid-connected or isolated) with balanced loads or unbalanced loads may be affected by the complexity of those models. Therefore a suitable SOC method must be adopted considering the simulation time, because even adopting the rint model may increase the time of computation considerably [96]. Preserving those factors, the above mentioned SOC estimation methods will be accessed.
As KF is suitable for linear system, EKF, UKF and CKF can be considered as an optimum state estimator for nonlinear systems such as li-ion battery systems [107]. The advantages of KF based method is that it is not sensitive to the initial SOC error [108], but there are disadvantages such high computational cost and complexity. Since these methods involves complex matrix operations, it is difficult to implement the algorithms in ordinary micro-controllers. Also they exhibits limitations such as linearization inaccuracy and uncertainties due to measurement noise. Even though the temperature is taken as an input while considering the ECM for the estimation, KF methods do not consider the above mentioned heat generation and heat transfer. So applying KF methods with coupled electro-thermal battery model to calculate SOC and update OCV (of ECM) will require considerable time and computational cost.
The neural network method exhibits limitations such as errors due to the over-training, effects of the previous sample data set on the present data set and the presence of too many neurons. The risk of over-fitting increases due to the presence of excessive neurons while limited neurons will under-fit the data [45]. Neural network method requires high computation as well as a large number of data set to trained. Fuzzy logic method requires high computational cost, expertise knowledge as well as clearly defined fuzzy rules. The support vector machines method demonstrates the superiority over the neural network method including no requirement to select the number of neurons, no requirement to identify the network topology and less problems regarding overfitting [109]. Additionally both neural network method and Support vector method present better results only for constant current situations and for dynamic situation the error is not in an acceptable level.
Hence non model based (direct measurement) are more suitable and among them OCV method and coulomb counting method are widely used. But adopting one of these methods alone may generate inaccuracy results. Therefore combination of the OCV method and coulomb counting method is suggested as the SOC estimation method for this purpose. The limitation of coulomb counting method can be overcome by adopting high accuracy current sensor and calculating the initial SOC value using the OCV-SOC method. The Figure 7 show the block diagram for the proposed SOC estimation method. The inputs are ambient temperature, current and OCV. The initial SOC is estimated using OCV-SOC method where the SOC values are stored using three dimensional table.The calculated SOC values is an input to the electro-thermal model.
In the electro-thermal model in Figure 6 the current, SOC, OCV and internal temperature are taken as inputs to the second order RC ECM and internal resistance, current, thermal parameters and entropy coefficients are considered as thermal model inputs.
The results from the previous research work were investigated for the quantitative analysis where the error of the estimated SOC value using indirect methods was calculated taking coulomb counting method as the reference. When calculating the SOC value using the coulomb counting method, in order to eliminate the integration error a high sensitive calibrated current sensor was used. In order to perform the quantitative analysis, in previous research work LiFePO4 batteries were tested under different temperature (0 ℃–60 ℃) and current profiles.
In neural network method it emphasizes that the RMS errors are within 4%, but the maximum error at some temperature (10 ℃–50 ℃) are larger than 10%. The errors are presented in the middle range of SOC (30%–80%). The most important fact is the inability of solving this problem by increasing the number of neurons or hidden layers because of over-fitting of neural network [45]. As a different approach, the estimated SOC curve using neural network method is compared with the reference SOC curve estimated using coulomb counting method in terms of current disturbance response [110]. In the normal situation without a current disturbance, the RMS error is less than 0.006% which is acceptable, but for a dynamic situation with a current disturbance, even though the estimated SOC converges to the reference value after some time, the deviation at the moment of the disturbance is at a considerable level.
In support vector method, for a normal condition the RMS error is over 5% where the maximum error is about 15%. For a dynamic situation error is about 2.5% where the maximum error is recorded as 13% [38]. For the fuzzy logic method the RMS error is roughly about 5% [63].
As for extended Kalman filter, the RMS error varies according to the adopted battery model [55]. As for an example for Thevenin model, the error is less than 0.6% which is acceptable [94]. for the second order RC ECM the RMS value is close to 0.75% where the maximum error is close to 2% [111].
This paper presented a review of methods of estimating of SOC of lithium-ion batteries. The estimation methods have been reviewed and evaluated in both qualitative and quantitative manner to encounter the suitability for Battery Energy Storage Systems dynamic modelling. In order to represent the dynamic behavior, the second order RC ECM is proposed and to encounter thermal effect thermal model is suggested to couple with the proposed ECM model. Since the OCV source of the second order RC ECM is nonlinear function of SOC, appropriate SOC estimation method should be adopted to achieve the objective of dynamic modelling of battery. Due to the fact of the additional complexity with the electro-thermal model, indirect measurement SOC estimation methods (model based methods) exhibits high computational cost and time. Furthermore those methods give better results only for the constant current situations. Therefore combination of two direct measurement SOC methods namely Coulomb counting method and OCV method is suggested. The primary part of the SOC estimation method consists of coulomb counting method where the initial SOC value is taken from the OCV method. As the BESS compromise of complex control schemes to regulate power, voltage and current, adopting this combination of SOC estimation method will give appreciable balance to the whole process.
The authors gratefully acknowledge the support provided by the Senate Research Council, University of Moratuwa (SRC/LT/2018/15).
The author declares that there is no conflict of interests in this paper.
[1] | Chen B, Ma H, Fang H, et al. (2014) An approach for state of charge estimation of li- ion battery based on thevenin equivalent circuit model. Prognostics and System Health Management Conference (PHM-2014 Hunan) 647–652. |
[2] | Ng KS, Moo CS, Chen YP, et al. (2008) State-of-charge estimation for lead-acid batteries based on dynamic open-circuit voltage. Power and Energy Conference, 2008. PECon 2008. IEEE 2nd International 972–976. |
[3] | Chang WY (2013) The state of charge estimating methods for battery: A review. ISRN Appl Math 2013. |
[4] | Xiong R, Cao J, Yu Q, et al.(2018) Critical review on the battery state of charge estimation methods for electric vehicles. Ieee Access 6: 1832–1843. |
[5] |
Ren H, Zhao Y, Chen S, et al. (2019) Design and implementation of a battery management system with active charge balance based on the soc and soh online estimation. Energy 166: 908–917. doi: 10.1016/j.energy.2018.10.133
![]() |
[6] | Gundogdu B, Gladwin D, Foster M, et al. (2018) A forecasting battery state of charge management strategy for frequency response in the uk system. 2018 IEEE International Conference on Industrial Technology (ICIT) 1726–1731. |
[7] |
Coleman M, Lee CK, Zhu C, et al. (2007) State-of-charge determination from emf voltage estimation: Using impedance, terminal voltage, and current for lead-acid and lithium-ion batteries. IEEE Trans Ind Electron 54: 2550–2557. doi: 10.1109/TIE.2007.899926
![]() |
[8] |
Awadallah MA, Venkatesh B (2016) Accuracy improvement of soc estimation in lithium-ion batteries. J Energy Storage 6: 95–104. doi: 10.1016/j.est.2016.03.003
![]() |
[9] |
Chun CY, Baek J, Seo GS, et al. (2015) Current sensor-less state-of-charge estimation algorithm for lithium-ion batteries utilizing filtered terminal voltage. J Power Sources 273: 255–263. doi: 10.1016/j.jpowsour.2014.08.121
![]() |
[10] |
Larsson F, Andersson P, Blomqvist P, et al. (2014) Characteristics of lithium-ion batteries during fire tests. J Power Sources 271: 414–420. doi: 10.1016/j.jpowsour.2014.08.027
![]() |
[11] | Zubi G, Dufo-López R, Carvalho M, et al. (2018) The lithium-ion battery: State of the art and future perspectives Renew Sust Energy Rev 89: 292–308. |
[12] |
He H, Xiong R, Zhang X, et al. (2011) State-of-charge estimation of the lithium-ion battery using an adaptive extended kalman filter based on an improved thevenin model.IEEE Trans Veh Technol 60: 1461–1469. doi: 10.1109/TVT.2011.2132812
![]() |
[13] | Sarasua AE, Molina MG, Mercado PE (2013) Dynamic modelling of advanced battery energy storage system for grid-tied ac microgrid applications. Energy Storage: Technol Appl. |
[14] |
Moura SJ, Argomedo FB, Klein R, et al. (2017) Battery state estimation for a single particle model with electrolyte dynamics. IEEE Trans Control Syst Technol 25: 453–468. doi: 10.1109/TCST.2016.2571663
![]() |
[15] | Moura SJ, Krstic M, Chaturvedi NA (2012) Adaptive pde observer for battery soc/soh estimation. ASME 2012 5th Annual Dynamic Systems and Control Conference joint with the JSME 2012 11th Motion and Vibration Conference 101–110. |
[16] | Tsang K, Chan W, Wong Y, et al. (2010) Lithium-ion battery models for computer simulation. 2010 IEEE International Conference on Automation and Logistics 98–102. |
[17] | Chen M, Rincon-Mora GA (2006) Accurate electrical battery model capable of predicting runtime and iv performance IEEE Trans Energy Convers 21: 504–511. |
[18] | Dey S, Ayalew B, Pisu B (2014) Adaptive observer design for a li-ion cell based on coupled electrochemical-thermal model. ASME 2014 Dynamic Systems and Control Conference V002T23A001–V002T23A001. |
[19] | Satadru D, Beshah A, Pierluigi P(2015) Nonlinear robust observers for state-of-charge estimation of lithium-ion cells based on a reduced electrochemical model. IEEE Trans Control Syst Technol 23: 1935–1942. |
[20] |
Plett GL (2004) Extended kalman filtering for battery management systems of lipb-based hev battery packs: Part 3. state and parameter estimation. J Power sources 134: 277–292. doi: 10.1016/j.jpowsour.2004.02.033
![]() |
[21] | Zhang L, Peng H, Ning Z, et al. (2017)Comparative research on rc equivalent circuit models for lithium-ion batteries of electric vehicles. Appl Sci 7: 1002. |
[22] | Van den Bossche P, Omar N, Al Sakka M, et al. (2014) The challenge of phev battery design and the opportunities of electrothermal modeling. Lithium-Ion Batteries 2014: 249–271. |
[23] |
Rivera-Barrera JP, Muñoz-Galeano N, Sarmiento-Maldonado HO (2017) Soc estimation for lithium-ion batteries: review and future challenges. Electronics 6: 102. doi: 10.3390/electronics6040102
![]() |
[24] |
Xie B, Liu Y, Ji Y, et al. (2018) Two-stage battery energy storage system (bess) in ac microgrids with balanced state-of-charge and guaranteed small-signal stability. Energies 11: 322. doi: 10.3390/en11020322
![]() |
[25] |
Tang X, Liu B, Gao F (2017) State of charge estimation of lifepo4 battery based on a gain-classifier observer. Energy Procedia 105: 2071–2076. doi: 10.1016/j.egypro.2017.03.585
![]() |
[26] |
Hussein AA (2014) Kalman filters versus neural networks in battery state-of-charge estimation: A comparative study. Int J Mod Nonlinear Theory Appl 3: 199. doi: 10.4236/ijmnta.2014.35022
![]() |
[27] |
Barai A, Widanage WD, Marco J, et al. (2015) A study of the open circuit voltage characterization technique and hysteresis assessment of lithium-ion cells. J Power Sources 295: 99–107. doi: 10.1016/j.jpowsour.2015.06.140
![]() |
[28] |
Xiong R, Yu Q, Wang LY (2017) Open circuit voltage and state of charge online estimation for lithium ion batteries. Energy Procedia 142: 1902–1907. doi: 10.1016/j.egypro.2017.12.388
![]() |
[29] |
Lee S, Kim J, Lee J, et al. (2008) State-of-charge and capacity estimation of lithium- ion battery using a new open-circuit voltage versus state-of-charge. J power sources 185: 1367–1373. doi: 10.1016/j.jpowsour.2008.08.103
![]() |
[30] |
Meng J, Luo G, Ricco M, et al. (2018) Overview of lithium-ion battery modeling methods for state-of-charge estimation in electrical vehicles. Appl Sci 8: 659. doi: 10.3390/app8050659
![]() |
[31] | Tan Y, Mao J, Tseng K (2011) Modelling of battery temperature effect on electrical characteristics of li-ion battery in hybrid electric vehicle. 2011 IEEE Ninth International Conference on Power Electronics and Drive Systems 637–642. |
[32] | Cheng Z, Wang L, Liu J, et al. (2016) Estimation of state of charge of lithium-ion battery based on photovoltaic generation energy storage system. Tehnički vjesnik 23: 695–700. |
[33] | Weng C, Sun J, Peng F (2013) An open-circuit-voltage model of lithium-ion batteries for effective incremental capacity analysis. ASME 2013 dynamic systems and controlconference. 2013: V001T05A002–V001T05A002. |
[34] |
Charkhgard M, Farrokhi M (2010) State-of-charge estimation for lithium-ion batteries using neural networks and ekf. IEEE Trans Ind electron 57: 4178–4187. doi: 10.1109/TIE.2010.2043035
![]() |
[35] |
Ma Y, Zhou X, Li B, et al. (2016) Fractional modeling and soc estimation of lithium-ion battery. IEEE/CAA Journal of Auto Sin 3: 281–287. doi: 10.1109/JAS.2016.7508803
![]() |
[36] |
Ng KS, Moo CS, Chen YP, et al. (2009) Enhanced coulomb counting method for estimating state-of-charge and state-of-health of lithium-ion batteries. Appl energy 86: 1506–1511. doi: 10.1016/j.apenergy.2008.11.021
![]() |
[37] | Lipu MH, Hannan MA, Hussain A, et al. (2018) State of charge estimation for lithium-ion battery using recurrent narx neural network model based lighting search algorithm. IEEE Access 2018. |
[38] |
Hansen T, Wang CJ (2005) Support vector based battery state of charge estimator. J Power Sources 141: 351–358. doi: 10.1016/j.jpowsour.2004.09.020
![]() |
[39] |
Chang MH, Huang HP, Chang SW (2013) A new state of charge estimation method for lifepo4 battery packs used in robots. Energies 6: 2007–2030. doi: 10.3390/en6042007
![]() |
[40] |
Tudoroiu RE, Zaheeruddin M, Radu SM, et al. (2018) Real-time implementation of an extended kalman filter and a pi observer for state estimation of rechargeable li-ion batteries in hybrid electric vehicle applications-a case study. Batteries 4: 19. doi: 10.3390/batteries4020019
![]() |
[41] | Linghu J, Kang L, Liu M, et al. (2018) State of charge estimation for ternary battery in electric vehicles using spherical simplex-radial cubature kalman filter. 2018 International Conference on Power System Technology 1586–1592. |
[42] |
Wu TH, Moo CS (2017) State-of-charge estimation with state-of-health calibration for lithium-ion batteries. Energies 10: 987. doi: 10.3390/en10070987
![]() |
[43] | Li Y, Zou C, Berecibar M, et al. (2018) Random forest regression for online capacity estimation of lithium-ion batteries. Appl Energy 23: 197–210. |
[44] | Yan Q, Wang Y (2017) Predicting for power battery soc based on neural network. Control Conference (CCC), 2017 36th Chinese 4140–4143. |
[45] |
He W, Williard N, Chen C, et al. (2014) State of charge estimation for li-ion batteries using neural network modeling and unscented kalman filter-based error cancellation. Int J Electr Power Energy Syst 62: 783–791. doi: 10.1016/j.ijepes.2014.04.059
![]() |
[46] |
Yu Z, Huai R, Xiao L (2015) State-of-charge estimation for lithium-ion batteries using a kalman filter based on local linearization. Energies 8: 7854–7873. doi: 10.3390/en8087854
![]() |
[47] | Lipu MH, Hussain A, Saad M, et al. (2018) Improved recurrent narx neural network model for state of charge estimation of lithium-ion battery using pso algorithm. 2018 IEEE Symposium on Computer Applications & Industrial Electronics 354–359. |
[48] | Lv J, Yuan H, Lv Y (2012) Battery state-of-charge estimation based on fuzzy neural network and improved particle swarm optimization algorithm. 2012 Second International Conference on Instrumentation, Measurement, Computer, Communication and Control 22–27. |
[49] | Yu DX, Gao YX(2013) Soc estimation of lithium-ion battery based on kalman filter algorithm. Appl Mech Mater 347: 1852–1855. |
[50] | Tingting D, Jun L, Fuquan Z, et al. (2011) Analysis on the influence of measurement error on state of charge estimation of lifepo4 power battery. 2011 International Conference on Materials for Renewable Energy Environment 1: 644–649. |
[51] |
He Z, Gao M, Wang C, et al. (2013) Adaptive state of charge estimation for li-ion batteries based on an unscented kalman filter with an enhanced battery model. Energies 6: 4134– 4151. doi: 10.3390/en6084134
![]() |
[52] | Di¸ s¸ ci FN, El-Kahlout Y, Balık¸ cı A (2017) Li-ion battery modeling and soc estimation using extended kalman filter. 2017 10th International Conference on Electrical and Electronics Engineering 166–169. |
[53] | Huria T, Ceraolo M, Gazzarri J, et al. (2012) High fidelity electrical model with thermal dependence for characterization and simulation of high power lithium battery cells. 2012 IEEE International Electric Vehicle Conference 1–8. |
[54] | Huria T, Ceraolo M, Gazzarri J, et al. (2013) Simplified extended kalman filter observer for soc estimation of commercial power-oriented lfp lithium battery cells. SAE Technical Paper, Tech. Rep., 2013. |
[55] | Plett GL (2003) Advances in ekf soc estimation for lipb hev battery packs. Consultant to Compact Power, Inc. |
[56] | Lu J, Chen Z, Yang Y, et al. (2018) Online estimation of state of power for lithium-ion batteries in electric vehicles using genetic algorithm. IEEE Access 6: 868–880. |
[57] |
He W, Williard N, Chen C, et al. (2013) State of charge estimation for electric vehicle batteries using unscented kalman filtering. Microelectron Reliab 53: 840–847. doi: 10.1016/j.microrel.2012.11.010
![]() |
[58] |
Ma Y, Duan P, Sun Y, et al. (2018) Equalization of lithium-ion battery pack based on fuzzy logic control in electric vehicle. IEEE Trans Ind Electron 65: 6762–6771. doi: 10.1109/TIE.2018.2795578
![]() |
[59] |
Gan L, Yang F, Shi Y, et al. (2017) Lithium-ion battery state of function estimation based on fuzzy logic algorithm with associated variables. IOP Conference Series: Earth and Environmental Science 94: 012133. doi: 10.1088/1755-1315/94/1/012133
![]() |
[60] |
Wu T, Wang M, Xiao Q, et al. (2012) The soc estimation of power li-ion battery based on anfis model. Smart Grid Renewable Energy 3: 51. doi: 10.4236/sgre.2012.31007
![]() |
[61] | Singh P, Reisner D (2002) Fuzzy logic-based state-of-health determination of lead acid batteries. 24th Annual International Telecommunications Energy Conference 2002: 583– 590. |
[62] |
Singh P, Fennie C, Reisner D (2004) Fuzzy logic modelling of state-of-charge and available capacity of nickel/metal hydride batteries. J Power Sources 136: 322–333. doi: 10.1016/j.jpowsour.2004.03.035
![]() |
[63] |
Salkind AJ, Fennie C, Singh P, et al. (1999) Determination of state-of-charge and state-of- health of batteries by fuzzy logic methodology. J Power sources 80: 293–300. doi: 10.1016/S0378-7753(99)00079-8
![]() |
[64] |
Anton JA, Nieto PG, Viejo CB, et al. (2013) Support vector machines used to estimate the battery state of charge. IEEE Trans. Power Electron 28: 5919–5926. doi: 10.1109/TPEL.2013.2243918
![]() |
[65] |
Guo GF, Shui L, Wu XL, et al. (2014) Soc estimation for li-ion battery using svm based on particle swarm optimization. Adv Mater Res 1051: 1004–1008. doi: 10.4028/www.scientific.net/AMR.1051.1004
![]() |
[66] |
Pattipati B, Sankavaram C, Pattipati K (2011) System identification and estimation framework for pivotal automotive battery management system characteristics. IEEE Trans Syst Man Cybern Part C Appl Rev 41: 869–884. doi: 10.1109/TSMCC.2010.2089979
![]() |
[67] |
de Matos JG, e Silva FS, Ribeiro LAdS (2015) Power control in ac isolated microgrids with renewable energy sources and energy storage systems. IEEE Trans Ind Electron 62: 3490–3498. doi: 10.1109/TED.2015.2439958
![]() |
[68] | Jun B, Wang YX, Zhao XM (2017) State of charge estimation for electric vehicle batteries based on a particle filter algorithm. DEStech Trans Compu Sci Eng. |
[69] |
Li B, Peng K, Li G (2018) State-of-charge estimation for lithium-ion battery using the gauss-hermite particle filter technique. J Renewable Sustainable Energy 10: 014105. doi: 10.1063/1.5020028
![]() |
[70] |
Bi J, Gao H, Wang Y, et al. (2017) Estimation of state-of-charge of li-ion batteries in ev using the genetic particle filter. IOP Conference Series: Earth and Environmental Science 81: 012183. doi: 10.1088/1755-1315/81/1/012183
![]() |
[71] |
Xia B, Sun Z, Zhang R, et al. (2017) A cubature particle filter algorithm to estimate the state of the charge of lithium-ion batteries based on a second-order equivalent circuit model. Energies 10: 457. doi: 10.3390/en10040457
![]() |
[72] | Jiani D, Youyi W, Changyun W (2013) Li-ion battery soc estimation using particle filter based on an equivalent circuit model. 2013 10th IEEE International Conference on Control and Automation (ICCA) 2013: 580–585. |
[73] |
Xia B, Sun Z, Zhang R, et al. (2017) A comparative study of three improved algorithms based on particle filter algorithms in soc estimation of lithium ion batteries. Energies 10: 1149. doi: 10.3390/en10081149
![]() |
[74] | Lipu MH, Hannan M, Hussain A, et al. (2018) A review of state of health and remaining useful life estimation methods for lithium-ion battery in electric vehicles: Challenges and recommendations. J Cleaner Prod 2018. |
[75] | Deng Y, Hu Y, Cao Y (2014) An improved algorithm of soc testing based on open- circuit voltage-ampere hour method. International Conference on Life System Modeling and Simulation and International Conference on Intelligent Computing for Sustainable Energy and Environment 258–267. |
[76] | Murnane M, Ghazel A (2017) A closer look at state of charge (soc) and state of health (soh) estimation techniques for batteries. Available from: http://www. analog. com/media/en/technical-documentation/technicalarticles/A-Closer-Look-at-State- Of-Charge-and-State-Health-Estimation-Techniques-.... pdf. |
[77] | Leksono E, Haq IN, Iqbal M, et al. (2013) State of charge (soc) estimation on lifepo4 battery module using coulomb counting methods with modified peukert. 2013 Joint International Conference on Rural Information Communication Technology and Electric- Vehicle Technology (rICT & ICeV-T) 2013. |
[78] | Jeong YM, Cho YK, Ahn JH, et al. (2014) Enhanced coulomb counting method with adaptive soc reset time for estimating ocv. 2014 IEEE Energy Conversion Congress and Exposition 1313–1318. |
[79] |
Wu J, Zhang C, Chen Z (2016) An online method for lithium-ion battery remaining useful life estimation using importance sampling and neural networks. Appl energy 173: 134–140. doi: 10.1016/j.apenergy.2016.04.057
![]() |
[80] | Liu F, Liu T, Fu Y (2015) An improved soc estimation algorithm based on artificial neural network. 2015 8th International Symposium on Computational Intelligence and Design(ISCID) 2: 152–155. |
[81] | Wang L, Savvaris A, Tsourdos A (2018) Online battery pack state of charge estimation via ekf-fuzzy logic joint method. 2018 5th International Conference on Control, Decision and Information Technologies 899–904. |
[82] | Zenati A, Desprez P, Razik H, et al. (2012) A methodology to assess the state of health of lithium-ion batteries based on the battery's parameters and a fuzzy logic system. 2012 IEEE International Electric Vehicle Conference (IEVC). 2012: 1–6. |
[83] | Khayat N, Karami N (2016) Adaptive techniques used for lifetime estimation of lithium- ion batteries. 2016 Third International Conference on Electrical, Electronics, Computer Engineering and their Applications (EECEA). 2016: 98–103. |
[84] | Zhang N, Liu K (2011) The prediction of soc based on multiple dimensioned support vector machine. 2011 Second International Conference on Mechanic Automation and Control Engineering (MACE). 2011: 1786–1788. |
[85] |
Zhao Q, Qin X, Zhao H, et al. (2018) A novel prediction method based on the support vector regression for the remaining useful life of lithium-ion batteries. Microelectron Reliab 85: 99–108. doi: 10.1016/j.microrel.2018.04.007
![]() |
[86] |
Deng Z, Yang L, Cai Y, et al. (2017) Maximum available capacity and energy estimation based on support vector machine regression for lithium-ion battery. Energy Procedia 107: 68–75. doi: 10.1016/j.egypro.2016.12.131
![]() |
[87] | Rahul K, Ramprabhakar J, Shankar S (2017) Comparative study on modeling and estimation of state of charge in battery. 2017 International Conference On Smart Technologies For Smart Nation (SmartTechCon). 2017: 1610–1615. |
[88] |
Xing Y, He W, Pecht M, et al. (2014) State of charge estimation of lithium-ion batteries using the open-circuit voltage at various ambient temperatures. Appl Energy 113: 106–115. doi: 10.1016/j.apenergy.2013.07.008
![]() |
[89] | Yang D, Liu J, Wang Y, et al. (2014) State-of-charge estimation using a self-adaptive noise extended kalman filter for lithium batteries. 2014 IEEE PES Asia-Pacific Power and Energy Engineering Conference (APPEEC). 2014: 1–5. |
[90] | Liu Z, Wang Y, Du J, et al. (2012) Rbf network-aided adaptive unscented kalman filter for lithium-ion battery soc estimation in electric vehicles. 2012 7th IEEE Conference on Industrial Electronics and Applications (ICIEA). 2012: 1673–1677. |
[91] | Yang Y, Cui N, Wang C,et al. (2017) Soc estimation of lithium-ion battery based on new adaptive fading extended kalman filter. Chinese Automation Congress (CAC). 2017: 5630– 5634. |
[92] | Sangwan V, Kumar R, Rathore AK (2017) State-of-charge estimation for li-ion battery using extended kalman filter (ekf) and central difference kalman filter (cdkf). 2017 IEEE Industry Applications Society Annual Meeting 2017: 1–6. |
[93] | Zhang S, Sun H, Lyu C (2018) A method of soc estimation for power li-ion batteries based on equivalent circuit model and extended kalman filter. 2018 13th IEEE Conference on Industrial Electronics and Applications (ICIEA) 2018: 2683–2687. |
[94] | Topan PA, Ramadan MN, Fathoni G, et al. (2016) State of charge (soc) and state of health (soh) estimation on lithium polymer battery via kalman filter. International Conference on Science and Technology-Computer (ICST) 2016: 93–96. |
[95] | Jiang C, Taylor A, Duan C, et al. (2013) Extended kalman filter based battery state of charge (soc) estimation for electric vehicles. 2013 IEEE Transportation Electrification Conference and Expo (ITEC) 2013: 1–5. |
[96] |
Farrokhabadi M, König S, Cañizares CA, et al. (2018) Battery energy storage system models for microgrid stability analysis and dynamic simulation. IEEE Trans Power Syst 33: 2301– 2312. doi: 10.1109/TPWRS.2017.2740163
![]() |
[97] |
Thale SS, Wandhare RG, Agarwal V (2015) A novel reconfigurable microgrid architecture with renewable energy sources and storage. IEEE Trans Ind Appl 51: 1805–1816. doi: 10.1109/TIA.2014.2350083
![]() |
[98] |
Weng C, Sun J, Peng H (2014) A unified open-circuit-voltage model of lithium-ion batteries for state-of-charge estimation and state-of-health monitoring. J power Sources 258: 228– 237. doi: 10.1016/j.jpowsour.2014.02.026
![]() |
[99] |
Zhao S, Duncan SR, Howey DA (2017) Observability analysis and state estimation of lithium-ion batteries in the presence of sensor biases. IEEE Trans Control Syst Technol 25: 326–333. doi: 10.1109/TCST.2016.2542115
![]() |
[100] | Samba A (2015) Battery electrical vehicles-analysis of thermal modelling and thermal management. LUSAC (Laboratoire Universitaire des Sciences Appliquées de Cherbourg), Université de caen Basse Normandie; MOBI (the Mobility, Logistics and Automotive Technology Research Centre), Vrije Universiteit Brussel. |
[101] |
Tang X, Gao F, Zou C, et al. (2019) Load-responsive model switching estimation for state of charge of lithium-ion batteries. Appl Energy 238: 423–434. doi: 10.1016/j.apenergy.2019.01.057
![]() |
[102] |
Zou C, Manzie C, Neˇ si´ c D, et al. (2016) Multi-time-scale observer design for state-of-charge and state-of-health of a lithium-ion battery. J Power Sources 335: 121–130. doi: 10.1016/j.jpowsour.2016.10.040
![]() |
[103] | Baghdadi I, Briat O, Eddahech A, et al. (2015) Electro-thermal model of lithium-ion batteries for electrified vehicles applications. 2015 IEEE 24th International Symposium on Industrial Electronics (ISIE). 2015: 1248–1252. |
[104] |
Pesaran AA (2002) Battery thermal models for hybrid vehicle simulations. J power sources 110: 377–382. doi: 10.1016/S0378-7753(02)00200-8
![]() |
[105] |
Forgez C, Do DV, Friedrich G, et al. (2010) Thermal modeling of a cylindrical lifepo4/graphite lithium-ion battery. J Power Sources 195: 2961–2968. doi: 10.1016/j.jpowsour.2009.10.105
![]() |
[106] |
Gao Z, Chin CS, Woo WL, et al. (2017) Integrated equivalent circuit and thermal model for simulation of temperature-dependent lifepo4 battery in actual embedded application. Energies 10: 85. doi: 10.3390/en10010085
![]() |
[107] | Peng S, Chen C, Shi H, et al. (2017) State of charge estimation of battery energy storage systems based on adaptive unscented kalman filter with a noise statistics estimator. IEEE Access 5: 202–212. |
[108] | Zhou Y, Li X (2015) Overview of lithium-ion battery soc estimation. 2015 IEEE International Conference on Information and Automation 2015: 2454–2459. |
[109] | Fayazi A, Arabloo M, Shokrollahi A, et al. (2013) State-of-the-art least square support vector machine application for accurate determination of natural gas viscosity. Ind Eng Chem Res 53: 945–958. |
[110] |
Xu J, Li S, Cao B (2017) A novel current disturbance estimation method for battery management systems in electric vehicle. Energy Procedia 105: 2837–2842. doi: 10.1016/j.egypro.2017.03.621
![]() |
[111] | Ciortea F, Rusu C, Nemes M, et al. (2017) Extended kalman filter for state-of-charge estimation in electric vehicles battery packs. Optimization of Electrical and Electronic Equipment (OPTIM) & 2017 Intl Aegean Conference on Electrical Machines and Power Electronics (ACEMP), 2017 International Conference on. 2017: 611–616. |
1. | Ariel Jimenez, Natalia Morales, Carlos Paez, Arturo Fajardo, Gabriel Perilla, 2020, Low-complexity SPICE Analog Behavioral Modeling of the ideal battery for system level design, 978-1-7281-9365-6, 1, 10.1109/ANDESCON50619.2020.9272055 | |
2. | Adrienn Dineva, Bence Csomós, Szabolcs Kocsis Sz., István Vajda, Investigation of the performance of direct forecasting strategy using machine learning in State-of-Charge prediction of Li-ion batteries exposed to dynamic loads, 2021, 36, 2352152X, 102351, 10.1016/j.est.2021.102351 | |
3. | Giuliano Rancilio, Alexandre Lucas, Evangelos Kotsakis, Gianluca Fulli, Marco Merlo, Maurizio Delfanti, Marcelo Masera, Modeling a Large-Scale Battery Energy Storage System for Power Grid Application Analysis, 2019, 12, 1996-1073, 3312, 10.3390/en12173312 | |
4. | Kiran B. Kore, Pramod U. Tandale, Sachin R. Rondiya, Sagar B. Jathar, Bharat R. Bade, Mamta P. Nasane, Sunil V. Barma, Dhanaraj S. Nilegave, Niranjan V. Kurhe, Sandesh R. Jadkar, Adinath M. Funde, 2021, 2335, 0094-243X, 040002, 10.1063/5.0043346 | |
5. | Angelo Raciti, Santi Agatino Rizzo, Giovanni Susinni, Circuit model of LED light bulb suitable for typical voltage THD on LV distribution networks, 2020, 212, 03787788, 109665, 10.1016/j.enbuild.2019.109665 | |
6. | Adelina Ioana Ilies, Gabriel Chindris, Dan Pitica, 2020, A Comparison between State of Charge Estimation Methods: Extended Kalman Filter and Unscented Kalman Filter, 978-1-7281-7506-5, 376, 10.1109/SIITME50350.2020.9292232 | |
7. | Attanayaka M. S. M. H. S Attanayaka, Jayawardena P. Karunadasa, Kullappu T. M. U. Hemapala, Comprehensive electro‐thermal battery‐model for Li‐ion batteries in microgrid applications, 2021, 2578-4862, 10.1002/est2.230 | |
8. | Ja'Far Madani, Bobby Rian Dewangga, Adha Imam Cahyadi, Samiadji Herdjunanto, 2022, Parameter Optimization of Current Estimator for Lithium Polymer Battery (LiFePO4), 978-1-6654-6077-4, 13, 10.1109/ICITEE56407.2022.9954077 | |
9. | Mahyar J. Koshkouei, Erik Kampert, Andrew D. Moore, Matthew D. Higgins, Evaluation of an in situ QAM‐based Power Line Communication system for lithium‐ion batteries, 2022, 12, 2042-9738, 15, 10.1049/els2.12033 | |
10. | Di Zhu, Gyouho Cho, Jeffrey Joseph Campbell, 2021, Neural Networks Battery Applications: A Review, 978-1-6654-1846-1, 1, 10.1109/EIT51626.2021.9491835 | |
11. | Kamala Kumari Duru, Praneash Venkatachalam, Chanakya Karra, Asha Anish Madhavan, Sangaraju Sambasivam, Sujith Kalluri, Equivalent Circuit Model Parameters Estimation of Lithium-Ion Batteries Using Cuckoo Search Algorithm, 2022, 169, 0013-4651, 120503, 10.1149/1945-7111/aca6a5 | |
12. | Zhenxiao Yi, Zhenhua Zhao, Lingjun Li, Honghong Wang, Hongtao Wu, Kai Wang, Estimation methods for States of Charge and Health of Lithium-ion battery, 2022, 2221, 1742-6588, 012036, 10.1088/1742-6596/2221/1/012036 | |
13. | Rajbala Purnima Priya, Shivam Mishra, Aryan Priyadarshi, , 2023, Chapter 7, 978-981-19-7727-5, 93, 10.1007/978-981-19-7728-2_7 | |
14. | Nitika G. Panwar, Surinder Singh, Akhil Garg, Abhishek Kumar Gupta, Liang Gao, Recent Advancements in Battery Management System for Li‐Ion Batteries of Electric Vehicles: Future Role of Digital Twin, Cyber‐Physical Systems, Battery Swapping Technology, and Nondestructive Testing, 2021, 9, 2194-4288, 2000984, 10.1002/ente.202000984 | |
15. | Peng Nian, Zhang Shuzhi, Zhang Xiongwen, Co-estimation for capacity and state of charge for lithium-ion batteries using improved adaptive extended Kalman filter, 2021, 40, 2352152X, 102559, 10.1016/j.est.2021.102559 | |
16. | J.M.D.S. Jeewandara, J.P. Karunadasa, K.T.M.U. Hemapala, 2021, Comprehensive Study of Kalman Filter Based State of Charge Estimation Method for Battery Energy Management System in Microgrid, 978-1-6654-1262-9, 01, 10.1109/ICECCME52200.2021.9590949 | |
17. | Chaitali Mehta, Paawan Sharma, Amit V. Sant, 2021, A Comprehensive study of Machine Learning Techniques used for estimating State of Charge for Li-ion Battery, 978-1-6654-4211-4, 1, 10.1109/AIMV53313.2021.9671010 | |
18. | Rajbala Purnima Priya, Sanjay R, Rajakumar Sakile, State of charge estimation of lithium‐ion battery based on extended Kalman filter and unscented Kalman filter techniques, 2022, 2578-4862, 10.1002/est2.408 | |
19. | Weng Cheong Tan, Lip Huat Saw, Asrin Selan, Ming Chian Yew, Dongyang Sun, Yann Yee Koh, Wen Tong Chong, Wei-Hsin Chen, Modelling of polypropylene-based aluminum-air battery, 2023, 22147853, 10.1016/j.matpr.2023.01.293 | |
20. | Hind Elouazzani, Ibtissam Elhassani, Mohammed Ouazzani-Jamil, Tawfik Masrour, 2023, Chapter 69, 978-3-031-26851-9, 747, 10.1007/978-3-031-26852-6_69 | |
21. | Long Ling, Daoming Sun, Xiaoli Yu, Rui Huang, State of charge estimation of Lithium-ion batteries based on the probabilistic fusion of two kinds of cubature Kalman filters, 2021, 43, 2352152X, 103070, 10.1016/j.est.2021.103070 | |
22. | Asadullah Khalid, Alexander Stevenson, Arif I. Sarwat, Performance Analysis of Commercial Passive Balancing Battery Management System Operation Using a Hardware-in-the-Loop Testbed, 2021, 14, 1996-1073, 8037, 10.3390/en14238037 | |
23. | Dewang Chen, Xiaoyu Zheng, Ciyang Chen, Wendi Zhao, Remaining useful life prediction of the lithium-ion battery based on CNN-LSTM fusion model and grey relational analysis, 2023, 31, 2688-1594, 633, 10.3934/era.2023031 | |
24. | Ahmed Gaga, Adil Tannouche, Youness Mehdaoui, Benachir El Hadadi, Methods for estimating lithium-ion battery state of charge for use in electric vehicles: a review, 2022, 9, 2329-8774, 211, 10.1515/ehs-2021-0039 | |
25. | Oluwole Olalekan Solomon, Wei Zheng, Junxiong Chen, Zhu Qiao, State of charge estimation of Lithium-ion battery using an improved fractional-order extended Kalman filter, 2022, 49, 2352152X, 104007, 10.1016/j.est.2022.104007 | |
26. | Mehmet Korkmaz, A novel method for SoC estimation of lithium-ion batteries based on previous covariance matrices and variable ECM parameters, 2022, 0948-7921, 10.1007/s00202-022-01692-4 | |
27. | Minkyu Kwak, Bataa Lkhagvasuren, Hong Sung Jin, Gyuwon Seo, Sungyool Bong, Jaeyoung Lee, A Variable-length scale Parameter Dependent State of Charge Estimation of Lithium Ion Batteries by Kalman Filters, 2022, 17, 14523981, 220218, 10.20964/2022.02.18 | |
28. | G. Jagadeesh, B. Arundhati, K. Ravindra, 2024, 9780443189999, 83, 10.1016/B978-0-443-18999-9.00023-5 | |
29. | Arun Mozhi S, Charles Raja S, Nishanthy J, Sivakumar N, 2023, Deep Learning based EV Battery Management System, 979-8-3503-9337-8, 1, 10.1109/ICEMCE57940.2023.10434186 | |
30. | Nikolay Matanov, Plamen Nankinsky, Petar Stoev, 2023, Medium and Low Voltage Distribution Network Model, 979-8-3503-2653-6, 1, 10.1109/BulEF59783.2023.10406286 | |
31. | Chaitali Mehta, Amit V Sant, Paawan Sharma, SVM-assisted ANN model with principal component analysis based dimensionality reduction for enhancing state-of-charge estimation in LiFePO4 batteries, 2024, 8, 27726711, 100596, 10.1016/j.prime.2024.100596 | |
32. | Haya Binsalim, Salma Badaam, Arbaz Ahmed, Mohammed Abdulmajid, 2024, Battery Management System for Enhancing the Performance and Safety of Lithium-Ion Batteries, 979-8-3503-9356-9, 215, 10.1109/LT60077.2024.10468566 | |
33. | Jiabin Wang, Jianhua Du, Birong Tan, Xin Cao, Chang Qu, Yingjie Ou, Xingfeng He, Leji Xiong, Ran Tu, Establishment of a Lithium-Ion Battery Model Considering Environmental Temperature for Battery State of Charge Estimation, 2023, 170, 0013-4651, 120507, 10.1149/1945-7111/ad11af | |
34. | Chaitali Mehta, Amit V. Sant, Paawan Sharma, Optimized ANN for LiFePO4 battery charge estimation using principal components based feature generation, 2024, 3, 27731537, 100175, 10.1016/j.geits.2024.100175 | |
35. | Sudnya Vaidya, Daniel Depernet, Daniela Chrenko, Salah Laghrouche, 2024, Chapter 5, 978-3-031-55695-1, 67, 10.1007/978-3-031-55696-8_5 | |
36. | See Fung Lee, Jeevan Kanesalingam, Hock Guan Ho, Suhanya Jayaprakasam, 2023, Use of Deep Neural Networks to Predict Lithium-Ion Cell Voltages During Charging and Discharging, 978-1-6654-8258-5, 1, 10.1109/TENSYMP55890.2023.10223633 | |
37. | Zhaoliang Dou, Jiaxin Li, Hongjuan Yan, Chunlin Zhang, Fengbin Liu, Real-Time Online Estimation Technology and Implementation of State of Charge State of Uncrewed Aerial Vehicle Lithium Battery, 2024, 17, 1996-1073, 803, 10.3390/en17040803 | |
38. | Uzair Khan, Sheeraz Kirmani, Yasser Rafat, Mohd Umar Rehman, M. Saad Alam, Improved deep learning based state of charge estimation of lithium ion battery for electrified transportation, 2024, 91, 2352152X, 111877, 10.1016/j.est.2024.111877 | |
39. | Endah Retno Dyartanti, Anif Jamaluddin, Muhammad Farrel Akshya, Dimas Zuda Fathul Akhir, Himmah Sekar Eka Ayu Gustiana, Agus Purwanto, Aficena Himdani Ilmam Abharan, Muhammad Nizam, The State of Charge Estimation of LiFePO4 Batteries Performance Using Feed Forward Neural Network Model, 2024, 918, 1662-7482, 85, 10.4028/p-iiDzS6 | |
40. | Qiao Lin, Donglei Liu, Shunlin Wang, Weijia Xiao, 2023, SOC Online Estimation Error Correction Algorithm Based on Particle Swarm Optimization Particle Filter Algorithm, 979-8-3503-0934-8, 174, 10.1109/NEESSC59976.2023.10349317 | |
41. | Yanwei Sun, Yingai Jin, Zhipeng Jiang, Liang Li, A review of mitigation strategies for li-ion battery thermal runaway, 2023, 149, 13506307, 107259, 10.1016/j.engfailanal.2023.107259 | |
42. | Xinyue Liu, Yang Gao, Kyamra Marma, Yu Miao, Lin Liu, Advances in the Study of Techniques to Determine the Lithium-Ion Battery’s State of Charge, 2024, 17, 1996-1073, 1643, 10.3390/en17071643 | |
43. | Mostafa M. Shibl, Loay S. Ismail, Ahmed M. Massoud, A machine learning-based battery management system for state-of-charge prediction and state-of-health estimation for unmanned aerial vehicles, 2023, 66, 2352152X, 107380, 10.1016/j.est.2023.107380 | |
44. | Weihan Wang, Yanyun Zhang, Bin Xie, Lang Huang, Shanmu Dong, Gaojie Xu, Guanglei Cui, Deciphering Advanced Sensors for Life and Safety Monitoring of Lithium Batteries, 2024, 14, 1614-6832, 10.1002/aenm.202304173 | |
45. | Anbazhagan Geetha, S. Usha, J. Santhakumar, Surender Reddy Salkuti, Forecasting of energy consumption rate and battery stress under real-world traffic conditions using ANN model with different learning algorithms, 2025, 13, 2333-8334, 125, 10.3934/energy.2025005 | |
46. | Tanish Patel, Harshvardhan Gaikwad, 2025, Chapter 42, 978-981-97-6793-9, 667, 10.1007/978-981-97-6794-6_42 |
Category | Method |
Direct Measurement | (ⅰ) Open Circuit Voltage (OCV) method |
(ⅱ) Terminal Voltage method | |
(ⅲ) Impedance method | |
Book keeping method | (ⅰ) Coulomb Counting method |
(ⅱ) Modified Coulomb Counting method | |
Indirect Measurement | (ⅰ) Neural Network method |
(ⅱ) Support Vector Method | |
(ⅲ) Fuzzy logic | |
(ⅳ) Kalman Filter method | |
Extended Kalman Filter method | |
Unscented Kalman Filter method | |
Sigma Point Kalman Filter | |
Hybrid methods | Coulomb Counting and Kalman filter |
Method | Advantages | Limitations |
Open Circuit Voltage (OCV) method [74,75,76] | Simple Cost effective Independent of battery models An effective method for measuring SOC only at the initial stage and end stage |
Off-line technology long rest time is required |
Coulomb Counting method [77,78,77,8] | Simple Cost effective Independent of battery models |
Small errors will accumulate due to the integration term(initial SOC) Accuracy depends on the battery age, discharge rate and sensor precision |
Neural Network method [45,79,80] | Higher accuracy Temperature effect is taken as an input |
High computational cost Time consuming A large set of data set is required for the training process Better outputs only for constant current input |
Fuzzy logic method [81,82,83] | Higher Accuracy | High computational cost Fuzzy rules are to be clearly defined The approach is based on the static characteristics of the battery (practically not applicable for BESSs) The aging of battery can not be considered |
Support Vector Machines [84,85,86] | Higher accuracy The size of the training data set is small compared to neural network method |
The obtained training data should covered the expected range of operation |
KF, EKF, UKF [87,88,89,90,91,92,93,94,95] | High computational cost High complexity Depends on sensor accuracy and battery models Numerical instabilities due to complex matrix operations |
Independence of initial SOC Higher accuracy Consideration of noise |
Category | Method |
Direct Measurement | (ⅰ) Open Circuit Voltage (OCV) method |
(ⅱ) Terminal Voltage method | |
(ⅲ) Impedance method | |
Book keeping method | (ⅰ) Coulomb Counting method |
(ⅱ) Modified Coulomb Counting method | |
Indirect Measurement | (ⅰ) Neural Network method |
(ⅱ) Support Vector Method | |
(ⅲ) Fuzzy logic | |
(ⅳ) Kalman Filter method | |
Extended Kalman Filter method | |
Unscented Kalman Filter method | |
Sigma Point Kalman Filter | |
Hybrid methods | Coulomb Counting and Kalman filter |
Method | Advantages | Limitations |
Open Circuit Voltage (OCV) method [74,75,76] | Simple Cost effective Independent of battery models An effective method for measuring SOC only at the initial stage and end stage |
Off-line technology long rest time is required |
Coulomb Counting method [77,78,77,8] | Simple Cost effective Independent of battery models |
Small errors will accumulate due to the integration term(initial SOC) Accuracy depends on the battery age, discharge rate and sensor precision |
Neural Network method [45,79,80] | Higher accuracy Temperature effect is taken as an input |
High computational cost Time consuming A large set of data set is required for the training process Better outputs only for constant current input |
Fuzzy logic method [81,82,83] | Higher Accuracy | High computational cost Fuzzy rules are to be clearly defined The approach is based on the static characteristics of the battery (practically not applicable for BESSs) The aging of battery can not be considered |
Support Vector Machines [84,85,86] | Higher accuracy The size of the training data set is small compared to neural network method |
The obtained training data should covered the expected range of operation |
KF, EKF, UKF [87,88,89,90,91,92,93,94,95] | High computational cost High complexity Depends on sensor accuracy and battery models Numerical instabilities due to complex matrix operations |
Independence of initial SOC Higher accuracy Consideration of noise |