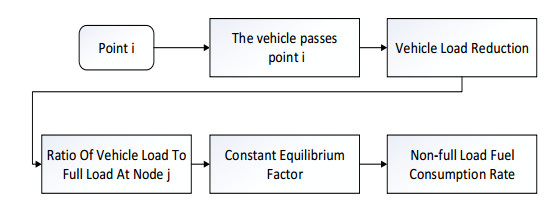
Citation: Stefania Conti, Santi A. Rizzo, Nunzio Salerno, Giuseppe M. Tina. Distribution network topology identification based on synchrophasor[J]. AIMS Energy, 2018, 6(2): 245-260. doi: 10.3934/energy.2018.2.245
[1] | Ningning Zhao, Shihao Cui . Study on 4D taxiing path planning of aircraft based on spatio-temporal network. Mathematical Biosciences and Engineering, 2023, 20(3): 4592-4608. doi: 10.3934/mbe.2023213 |
[2] | Li-zhen Du, Shanfu Ke, Zhen Wang, Jing Tao, Lianqing Yu, Hongjun Li . Research on multi-load AGV path planning of weaving workshop based on time priority. Mathematical Biosciences and Engineering, 2019, 16(4): 2277-2292. doi: 10.3934/mbe.2019113 |
[3] | Lei Jin, Sixiang Lin, Binglei Xie, Lin Liu . A vulnerability-based vehicle routing approach for solving capacitated arc routing problem in urban snow plowing operations. Mathematical Biosciences and Engineering, 2021, 18(1): 166-181. doi: 10.3934/mbe.2021009 |
[4] | Hao Yuan, Qiang Chen, Hongbing Li, Die Zeng, Tianwen Wu, Yuning Wang, Wei Zhang . Improved beluga whale optimization algorithm based cluster routing in wireless sensor networks. Mathematical Biosciences and Engineering, 2024, 21(3): 4587-4625. doi: 10.3934/mbe.2024202 |
[5] | Ping Li, Liwei Yang . Conflict-free and energy-efficient path planning for multi-robots based on priority free ant colony optimization. Mathematical Biosciences and Engineering, 2023, 20(2): 3528-3565. doi: 10.3934/mbe.2023165 |
[6] | Xiangyang Ren, Xinxin Jiang, Liyuan Ren, Lu Meng . A multi-center joint distribution optimization model considering carbon emissions and customer satisfaction. Mathematical Biosciences and Engineering, 2023, 20(1): 683-706. doi: 10.3934/mbe.2023031 |
[7] | Huawei Jiang, Shulong Zhang, Tao Guo, Zhen Yang, Like Zhao, Yan Zhou, Dexiang Zhou . Improved whale swarm algorithm for solving material emergency dispatching problem with changing road conditions. Mathematical Biosciences and Engineering, 2023, 20(8): 14414-14437. doi: 10.3934/mbe.2023645 |
[8] | Yuzhuo Shi, Huijie Zhang, Zhisheng Li, Kun Hao, Yonglei Liu, Lu Zhao . Path planning for mobile robots in complex environments based on improved ant colony algorithm. Mathematical Biosciences and Engineering, 2023, 20(9): 15568-15602. doi: 10.3934/mbe.2023695 |
[9] | Tian Xue, Liu Li, Liu Shuang, Du Zhiping, Pang Ming . Path planning of mobile robot based on improved ant colony algorithm for logistics. Mathematical Biosciences and Engineering, 2021, 18(4): 3034-3045. doi: 10.3934/mbe.2021152 |
[10] | Liwei Yang, Lixia Fu, Ping Li, Jianlin Mao, Ning Guo, Linghao Du . LF-ACO: an effective formation path planning for multi-mobile robot. Mathematical Biosciences and Engineering, 2022, 19(1): 225-252. doi: 10.3934/mbe.2022012 |
According to the data, in the whole process of a delivery of goods, the transport cost accounts for up to 40% in China. The main aspects of the transport cost include: wear and tear of vehicle, fuel consumption, driver's salary, transportation distance between multiple delivery points, the number of delivery points and so on. In these aspects, the transportation distance and the fuel consumption can be changed artificially, the driver's salary expenses, the number of distribution point etc. are not controllable factors, and the vehicle's fuel consumption mainly determined by the driving road and vehicle performance, due to vehicle performance varied, unable to be a criterion. Therefore, this paper focuses on vehicle fuel consumption and vehicle path, and strives to reduce the high cost of logistics and transportation, and provides a basis for optimizing path optimization. It turns out that through the experiments in this paper, the method can provide decision support for the cost of the logistics system and provide a better method for the operation of a more advanced logistics system.
The rapid development of e-commerce also brings about many problems. For example, a large number of cost losses lead to the waste of resources, and the complex transportation system cannot guarantee the safe driving of vehicles. Then, the reasonable planning of the vehicle's driving path, is an urgent problem. The Chinese and international scholars have carried out in-depth analysis on this problem, and the solution effect is obvious. For example, the famous VRP (Vehicle Routing Problem) is a classic interdisciplinary problem in operations research, mathematics, computer science and so on, at the same time the problem [1,2] is a combinatorial optimization problem, belongs to N-P problem, many scholars of VRP problems to mathematical modeling and algorithm design, and make it better, and the problem to be solved in this paper also falls within the scope of this problem.
The choice of algorithm. Comparing with other meta-heuristic algorithms, the experimental methods in this paper use the simulated annealing algorithm (SA) for the following reasons: First, the simulated annealing algorithm, is more general, deal with the practical problems well in this paper, secondly, SA can increase the acceptance probability of each state by controlling the temperature during the annealing process for avoiding stagnation at a localized solution; thirdly and finally, the selection space of the optimal solution can be increased by the 2-swap and 3-swap methods, so that the optimal solution can be searched in the maximum range.
The reasons for not using other meta-heuristics in this paper are as follows: For Genetic Algorithms (GA), the primary problem of genetic algorithm is encoding the problem, and also need to decode when the optimal solution is obtained, which greatly increases the complexity of the program. Especially in the case of dealing with problems with greater complexity, the disadvantage of GA will be more obvious. In addition, the choice of the parameters of the three operators of genetic algorithm will also seriously affect the quality of the solution. From this point, it requires a lot of data sets and experience can accurately grasp the choice of three operators; finally, through traversal, the result set of the GA will be infinitely close to the optimal solution, but if there is an error in each calculation, the final result will also be biased, and this algorithm will be shown in the experimental section. For the ant colony algorithm, the disadvantage is that if the parameters α and β are improper, the speed of solution is very slow and the quality of the solution is particularly poor; from the application point of view, the ant colony algorithm requires all ants to eventually choose the same route. If the number of loops is given, it is more difficult to achieve this situation; and the ant colony algorithm has a slower convergence rate and is easy to fall into local optimum, and the algorithm lacks pheromone in the initial situation, and the algorithm complexity is compared with the SA is quite big.
The disadvantage of the particle swarm optimization algorithm (PSO) is that it is prone to premature convergence (especially in dealing with complex multi-peak search problems) and poor local optimization ability. The PSO algorithm is trapped in a local minimum, mainly due to the loss of diversity of the population in the search space. The hybrid models of different PSO algorithms are mainly divided into two categories: (1) the global optimization algorithm and the local optimization algorithm are mixed; (2) the global optimization algorithm is mixed with the global optimization algorithm. Throughout the various hybrid algorithms related to the PSO algorithm, most of them basically adopt a strategy to improve them, either with other algorithms or adding mutation operations, and there are fewer hybrid algorithms using both strategies. Among the above strategies, there is a certain contradiction between the two. For the method one, although it can improve the local convergence speed, it also increases the possibility of falling into the local minimum; for the hybrid method two, the algorithm local refinement capabilities have still not improved. However, if only the mutation operation is added, the detection ability of the algorithm is improved, but the local development capability is also impaired.
The first part of this paper introduces the meaning of the topic and the choice of the algorithm, and introduces the factors that need to be considered in the article. The second part introduces some senior scholars in the path optimization problem. The third part is to solve the mathematical calculation model designed by the problems raised in this paper. The fourth part is the experimental part, and the conclusion is drawn by comparison with the traditional genetic algorithm. The fifth part is the summary of this article, focusing on the contribution of this paper and the future development direction.
At present, there are two main goals [3] for logistics transportation path optimization. First, time optimization, it is optimized according to the time window, and taken into account the service point [4] in the driving path of the vehicle, and added a time window for the customer or a penalty function to the transportation vehicle. This method improves transportation efficiency of the logistics by improving service quality of the logistics. The second optimization goal is the driving path of the vehicle. This goal is mainly to make the vehicle travel the shortest path, consume less fuel, and achieve the shortest travel distance in a two-dimensional plane. Find the shortest path by using a variety of algorithms to deliver the goods to a sorting center or warehouse center [5] in the shortest amount of time. Common typical algorithms include: Ant colony algorithm, simulated annealing algorithm, genetic algorithm and so on.
The author of the article [6] considered the safety issue in vehicle transportation. A real case was tested by an adaptive fuzzy neural network model trained by artificial bee colony algorithm, and the cost and risk of transporting dangerous goods were accurately evaluation. In the literature [7], the improved ant colony algorithm is used to solve the vehicle routing problem with soft time window. The problem is solved by updating the solution obtained by the ant colony algorithm, which improves the diversity of the problem solution and the global search ability, thus making the algorithm more capable. Solve the local optimal problem well. In the literature [8], a multi-warehouse, multi-customer, and multi-origin vehicle routing problem is studied. In order to solve the problem of total travel time of vehicles, the author proposes a fuzzy logic-guided random search algorithm, and proves its effectiveness through simulation results. Document [9] proposes a new vehicle carbon emission estimation method and four vehicle effective states. By selecting the dynamic vehicle state with the highest probability, and reconstructing the vehicle trajectory every second between consecutive sampling times, and verifying the model using the simulation data set, and comparing the linear interpolation model, the vehicle is proved. The carbon emission problem is a problem that cannot be ignored in the vehicle routing problem. Document [10] provides a linear relationship between vehicle load and fuel consumption based on actual data, and has fully demonstrated that a multi-target vehicle dry well problem model with dynamic windows with time windows and random items is established. the author of the article[11] using ANFIGS can provide the driver with actual route suggestions as needed. Obviously, this method has achieved very significant results, and the experimental results also show that the method has very good performance in the decision-making process. In the article [12], by classifying the types of vehicles, the authors used adaptive neural networks to model the re-selection of travel routes for different vehicle types and to monitor atmospheric conditions. It has played a positive role in reducing air pollution and reducing problems caused by vehicles.
However, on the same issue, most scholars mainly take the length of route into account, that is, planning the path of the vehicle [13]. There is less literature on the transportation costs of vehicles. Few scholars have analyzed the influence of road conditions on vehicle fuel consumption in logistics. According to the literature, in the logistics industry, transportation costs account for 40–50% of the total cost, and the road conditions are related to the actual fuel consumption of the vehicle. In the literature, the fuel consumption of the vehicle is also the factors are constrained, but the problems involved in the route are quite complicated [14], which cannot be enumerated one by one. This paper analyzes and calculates the factors that have greater influence: The load of the vehicle and the influence of the gradient of the road on fuel consumption. For example, if the vehicle travels from A to B, the fuel consumption of the high-gradient vehicle is very large under full load, because it mainly receives two factors, one is the load capacity of the vehicle body, and the other is the driving gradient of the vehicle. Increasing the friction coefficient, increasing the power consumption of the engine, excessive external power, etc., the transportation cost of the vehicle will be greatly improved. High-gradient sections are also extremely difficult for the vehicle to travel, because there are many uncontrollable factors, the damage to the engine during the vehicle climbing process is great, it is easy to cause damage to the vehicle itself, and it is easy to cause some safety problems [15]. However, on low-gradient sections, the vehicle has no other load-bearing load than its own load, and the possibility of a safety accident is small. The fuel consumption between the low-gradient fuel consumption and the high-gradient may be several times different. For the above situation, this paper proposes a path optimization model that takes into account the vehicle's load capacity and the vehicle's running gradient, so as to provide a basis for the choice of vehicle transportation path.
The path cost optimization model constructed in this paper is an optimization model considering the load capacity and different Road gradient.
Ordinary trains in the logistics market can be classified into four categories according to their type [16]: Heavy goods vehicles, medium goods vehicles, light goods vehicles and mini trucks. Heavy-duty trucks are generally used to transport large vehicles such as large equipment and non-removable items. Generally, the cargo load is generally more than 120 tons. The medium-sized trucks generally carry about 50 tons of cargo. But the effect of medium-sized truck is almost the same as that of heavy-duty vehicles. It is a light-duty vehicle with a load capacity of about 26–35 tons, after long-distance transportation, it will not cause loss of goods. The load of light-duty trucks is generally around 8–12 tons, which is also in line with the needs of logistics. Light vehicles and medium-sized vehicles are used as standard delivery vehicles. There are more than 30 types of fuel consumption due to various factors, but in terms of logistics, a distribution center has a total of k vehicles, r medium-sized vehicles, and 1 light-duty vehicle. Medium fuel consumption at full load Gfr, and no-load Ger. Light vehicles are fueled at full load Sfl and no-load Sel. The prerequisite for the entire experiment is that A distribution center O, and multiple delivery points Vi (i∈(1, 2, 3…m)). Perform delivery tasks, the load capacity of the vehicle k is Qk, where each vehicle of the distribution center departs from the distribution center and returns to the distribution center after performing the delivery task (one or more delivery points). All distribution nodes must be delivered once. The distance between vehicle k and the distribution center of i and j is Dijk, the average gradient between the two distribution points is Sijk, and the gradient from the distribution center to the distribution point is Sik, the fuel consumption coefficient a under different gradient. In general, the fuel consumption correction coefficient (c) of the vehicle is less than one and more than 0.5.
According to the literature, the fuel consumption and the load weight of the vehicle are linearly related. This paper proposes a method to balance the fuel consumption by the load to calculate the actual fuel consumption and describe the method in a streamlined way, as shown in Figure 1.
F(q)=(Gke+(Gkf−Gke)∗(qQ))∗c | (1) |
In this paper, the fuel consumption rate is estimated using the load condition of the vehicle, and there is a linear relationship between the actual consumption rate of fuel consumption and the load of the vehicle. In actual situations, the calculation of fuel consumption is quite complicated (road, quality, Engine power and so on). In order to be closer to reality, after the load calculation, the constant multiplication should be performed.
In the formula, F(q) represents the actual fuel consumption rate (L/100km), q represents the actual load during driving, Q represents the full load, and c is constant value (random between 0.5 and 1) and used to reduce the actual weight of the cargo, the experiment also proved that this value is more in line with the actual situation. The consumption value is a constant value of the specification, generally defined between the values 0 and 1, according to the experiment, value of c should between 0.76 and 0.91. Gek represents the empty fuel consumption of the vehicle in the distribution center, and Gfk represents the full fuel consumption of the vehicle.
According to Formula 1, when the vehicle is fully loaded, the fuel consumption is Gfk*c. When the vehicle is unloaded, the fuel consumption is Gek*c. In the case of load but not full load, the fuel consumption cost between the delivery point i and the delivery point j is F(q)*Dijk.
On the aspect of gradient of the road, the greater the gradient of the road, the higher the fuel consumption of the vehicle. According to the literature, there should be a difference between the fuel consumption corresponding to the gradient of each longitudinal gradient and the normal driving during the running of the vehicle. According to the actual test of the literature. The difference between the various longitudinal gradient and the fuel consumption is:
Ydiff=a+b∗i | (2) |
In this formula, i is the gradient, a and b are the coefficients and constants of the linear expression. According to the analysis of various literatures, the value of a should be between 6–10, which is in line with the conventional fuel consumption difference. The fuel consumption difference should be compared between 200 and 250, so the total fuel consumption value according to the gradient should be:
minTC=m∑i=0m∑j=0l∑k=0r∑k=0F(q)∗Dkij∗xkij+Ydiff∗(Dkij+Dki)∗ykij | (3) |
Where F(q) is the actual consumption rate of fuel consumption, and Ydiff is the difference between the fuel consumption of the longitudinal gradient and normal driving.
xkij={1 vehicle k from ito j0 other situations | (4) |
ykij={1 Fuel consumption of vehicle k from ito j 0 other situations | (5) |
Gkf>Gke>0 | (6) |
m∑i,j=0Dkij<k∗Dkij | (7) |
The earliest idea of Simulated Annealing Algorithms (SA) [17] was proposed by Metropolis et al. in 1953. After 30 years, Kirkpatrick et al. introduced annealing ideas into the field of combinatorial optimization [18]. The principle is based on solid matter. The similarity between the annealing process and the combination optimization. SA is a probability-based algorithm that warms the solid to a sufficiently high temperature and then slowly cools it. When the solid temperature rises, the internal particles of the solid become disordered due to the increase in temperature and when the temperature decreases, the activity of the particles decreases. According to principle, each temperature reaches an equilibrium state, and then reaches the ground state at the normal temperature. For the analysis of the process of this experiment, the main idea is to continuously obtain the equilibrium state of the particles through continuous iterative (cooling) process, than the current solution, and then randomize it by Metropolis [19] criterion. To find the best solution, there is a probability to receive a poor solution, and the local optimum is adjusted to the global optimality [20]. Although VRP is different from the simple TSP problem [21], the problems considered are the affected road, vehicle performance, vehicle fuel consumption [22], road condition (flatness), route congestion [23], vehicle load Capacity, vehicle scheduling [24] and more. However, as a global optimization algorithm, in many cases, the problem of converting multiple targets into single targets has been recognized by many scholars.
The implementation process of the algorithm is as follows:
(1) The initialization function, by generating the initial solution, the initialization process can objectively analyze the influence of the initialization node on the fuel consumption cost of the vehicle through the function realization. The quality of the initial solution can affect the number of iterations of the vehicle in the optimization process, in order to obtain an objective and realistic result. Initialization is achieved by a random function.
(2) The main program of the simulated annealing algorithm: The initial function value is generated by the randomly generated new solution, the Markov chain length is set internally in the temperature iterative reduction, the double-layered loop is used to globally traverse the generated solution, and the three-swap or double is selected by the random function. Exchange is a good idea to make the annealing algorithm more efficient, and to ensure global optimization.
(3) The road gradient value is extracted, and the path cost calculation is extracted. The load weight coefficient and the road gradient coefficient are added for the calculation cost when the cost distance matrix is calculated. Therefor the cost calculation is more comprehensive, and then the cost matrix considering the two is obtained.
(4) The Metropolis criterion judgement. According to the comparison of the function values of the current solution and the new solution, when the function value of the new solution is low, the new solution is accepted, and when the function value of the new solution is poor (large), the new solution is accepted with a certain probability, and the probability formula is obtained for:
P={1,df<0exp(−dft),df≥0 | (8) |
In the formula, df = f (new) – f (old), the difference between the new solution and the old solution. The result of the solution obtained by this calculation is poor when the new solution is larger than the old solution. It indicates that the new solution has a smaller value, and the solution can be directly accepted and the next step is traversed when the solution is solved.
In this paper, the initial route map is first obtained through the initial logistics node, then each route is weighted on the vehicle load, and the gradient of the road is also weighted; when passing a certain node, the vehicle is the load capacity is reduced, and the fuel consumption of the next section is reduced. At the same time, the gradient data is extracted, and the adjustment of the gradient will also affect the analysis of fuel consumption. The overall process is shown in Figure 2.
In this paper, the data of 52 data nodes on the Tsplib data platform are used for analysis. According to the initial position information of 52 points, the initial distance matrix is obtained. The initial path of the distance matrix diagram (the initial path starts from 1) is shown in Figure 3.
The gradient matrix is to calculate the average gradient between the two distribution points. The average gradient is an important indicator to measure the road condition. It is also an important factor to measure the fuel consumption of the vehicle. The Table 1 below shows the first 23 points and the distribution points 1, 2 and 3.
Point | 1 | 2 | 3 |
1 | 0 | 0.54 | 0.48 |
2 | 0.56 | 0 | 0.55 |
3 | 0.17 | 0.04 | 0 |
4 | 0.17 | 0.28 | 0.20 |
5 | 0.32 | 0.16 | 0.34 |
6 | 0.07 | 0.29 | 0.38 |
7 | 0.02 | 0.19 | 0.46 |
8 | 0.26 | 0.02 | 0.21 |
9 | 0.21 | 0.49 | 0.54 |
10 | 0.54 | 0.11 | 0.53 |
11 | 0.25 | 0.52 | 0.10 |
12 | 0.36 | 0.17 | 0.05 |
13 | 0.56 | 0.28 | 0.02 |
14 | 0.58 | 0.44 | 0.09 |
15 | 0.16 | 0.06 | 0.30 |
16 | 0.36 | 0.32 | 0.16 |
17 | 0.13 | 0.11 | 0.07 |
18 | 0.47 | 0.35 | 0.43 |
19 | 0.33 | 0.51 | 0.55 |
20 | 0.10 | 0.42 | 0.08 |
21 | 0.45 | 0.39 | 0.59 |
22 | 0.33 | 0.58 | 0.31 |
23 | 0.40 | 0.33 | 0.34 |
24 | 0.25 | 0.36 | 0.59 |
25 | 0.20 | 0.17 | 0.29 |
In the actual logistics and transportation process, the transportation task of a vehicle is fixed, so the transportation demand for one vehicle at each demand point is fixed; at the same time, the load capacity of the vehicle during transportation is changed. The static distribution requirements between total load capacity and distribution point requirements simulate the cost of the vehicle.
The cost of a single vehicle in the above case is:
F=Dkij∗(a+b∗i) | (9) |
In the formula, the first term of the polynomial is the distance traveled by the vehicle k to between two points i and j, and the value of i is taken from the gradient value in the above table, that is, if i is 1, j is 2, then the value of i is 0.56. The above formula is the fuel consumption of the vehicle gradient cost between two points. Also considering the load of the vehicle, the transportation cost of a single vehicle between two points i and j can also be solved as follows:
F=Dkij∗(Gke+(Gkf−Gke)∗(qijQ))∗c | (10) |
Calculating the ratio of the load difference between i and j to the full load, calculating the real-time fuel consumption difference by calculating the fuel consumption difference between i and j of vehicle k, and then accurately Calculate the fuel consumption of the vehicle.
In the following, the two-point replacement method [25] (also called 2-swap exchange) adopted by the traditional SA is changed to the two-point replacement method or the three-point replacement method according to the random function by the neighborhood operation method [26]. The solution space search ability of the permutation method is not strong, and most of them are searched in the sequential neighborhood, the search range is small. It means that through the random two points in the solution space, three child node lists are generated, and the solution is searched in the child node list. However, as the scale of the problem increases, the time to search for solutions usually increases exponentially. This paper uses a three-point exchange method or a two-point exchange method. Based on the probability theory method, a specific exchange method is used to determine the neighborhood operation, which greatly improves the randomness of the new solution space, and increases the possibility for the algorithm to jump out of the local optimum; such a problem is also considered by many scholars; the literature [27] in order to overcome the classical particle swarm The shortcomings of optimization (PSO), by introducing adaptive mutation operator and selection operator into particle swarm optimization algorithm, improve the fitness of particle swarm optimization, improve the local search ability of the algorithm, and finally pass the change of the fitness of the global optimal particles adaptively adjusts the selection operator and the mutation operator; the literature [28] introduces a genetic algorithm based on diversity, which increases the diversity by injecting new orthogonal individuals in the search process. Optimality of the obtained individual.
In this paper, after completion distribution path of a certain vehicle, the total cost matrix is obtained. For the nodes in the figure, the cost matrix (i and j) two-point exchange method or three-point exchange method is performed by a random function. Random solution, by the cost of the solution to optimize, rather than the vehicle's transport distance; for example, in the transport cost matrix, the total transport cost of vehicle i is 575.4, and the transport distance has passed 12 nodes. Although the previous transportation optimization plan only passed 10 nodes, the transportation cost increased to 603.7.
Through the above method, the distance cost of the initial distance matrix is about 10240, and the order of node transportation is as follows (Figure 4).
After increasing the load factor and fuel consumption of the vehicle, every time the vehicle starts, the fuel consumption cost must be considered. The vehicle will re-measure the cost of short-distance and high-gradient sections, which will reduce the driving height during the driving process. The probability of the gradient route increases the stability of the driving and reduces the probability of traffic accidents. Compared with the vehicle routing problem considering the fuel consumption factor, only the transportation distance of the vehicle is considered during the operation of the vehicle, and it has a large cost value in terms of cost measurement. From the aspect of vehicle safety, various logistics companies have been consider the safety of the vehicle and the logistics vehicle is driving on a higher slope. However, due to the limited carrying capacity of the engine, the vehicle itself is subject to greater damage when transporting heavy cargo. If a large amount of goods or casualties are caused by the quality of the vehicle itself, this will not only lead to the destruction of the logistics chain, but also the reputation of the logistics company. The cost of consumption will be huge [29].
The figure below shows the comparison of the fuel consumption map and the vehicle's delivery point sequence after optimization (Figure 6).
Through the comparison of the two pictures, it shows that the vehicle's driving path is optimized, Figure 4 is the vehicle transportation cost and distribution path curve optimized for the shortest path, and Figure 5 is the vehicle transportation with the minimum fuel consumption of the vehicle. Cost and distribution roadmap, as shown in the above figure, while taking into account the demand of the distribution point, the fuel consumption goal of increasing the gradient shows a superior cost in terms of cost, and the fuel consumption cost is reduced by about 10–15%.
This article lists a 30-city example in TSPLIB, and tests the performance through the traditional genetic algorithm, and obtains the following results. When the algorithm is iterated to the 454th generation (maximum time is 500), the effect is not very obvious, and the result is not obtained.
From Figures 6 and 7, it can be concluded that the results are not ideal under the premise of using traditional genetic algorithms, which further proves that the method used in this paper is more worthy of recognition.
This paper mainly introduces a model of simulated annealing algorithm successfully applied to vehicle path optimization based on cost considerations. The Studies have shown that simulated annealing algorithms are a very successful and very effective tool for this application. This paper proves that more road factors should be considered in the vehicle routing optimization problem, and should not only consider the path optimization scheme under the influence of traditional factors.
From the article's Figures 4 and 5, it can be seen that the transportation cost has been reduced by 10–15%. The experiment also proves the potential utility of the method. From Figures 5 and 7, it can also be concluded that the method in this paper is a better choice.
It can be seen from the results obtained in this paper that the gradient and load of the road will provide decision support for measuring logistics cost in the future, and better meet the needs of the logistics system.
At the same time, because the logistics system is affected by many factors, the current method can meet a certain degree of demand under the current situation. However, as far as the logistics system is concerned, there are still many influencing factors that are worth studying. In the actual logistics system, it is necessary to further extract more influencing factors (such as real-time road conditions, cargo classification, air pollution) to obtain the optimal optimization model.
The authors would like to appreciate all anonymous reviewers for their insightful comments and constructive suggestions to polish this paper in high quality. This research was supported by the National Key Research and Development Program of China (No. 2018YFC0810204, 2018YFB17026), National Natural Science Foundation of China (No. 61872242), Shanghai Science and Technology Innovation Action Plan Project (17511107203) and Shanghai key lab of modern optical system.
All authors declare no conflicts of interest in this paper.
[1] | Monticelli A (2004) Electric power system state estimation. P IEEE 88: 262–282. |
[2] |
Kumar DMV, Srivastava SC, Shah S, et al. (1996) Topology processing and static state estimation using artificial neural networks. IEE P-Gener Transm D 143: 99–105. doi: 10.1049/ip-gtd:19960050
![]() |
[3] | Clements KA, Costa AS (2002) Topology error identification using normalized Lagrange multipliers. IEEE T Power Syst 13: 347–353. |
[4] | Singh N, Glavitsch H (1991) Detection and identification of topological errors in on line power system analysis. IEEE T Power Syst 6: 324–331. |
[5] |
Costa IS, Leao JA (1993) Identification of topology errors in power system state estimation. IEEE T Power Syst 8: 1531–1538. doi: 10.1109/59.260956
![]() |
[6] |
Silva APAD, Quintana VH, Silva APAD, et al. (1995) Pattern analysis in power system state estimation. Int J Elec Power 17: 51–60. doi: 10.1016/0142-0615(95)93277-6
![]() |
[7] |
Singh D, Pandey JP, Chauhan DS (2005) Topology identification, bad data processing, and state estimation using fuzzy pattern matching. IEEE T Power Syst 20: 1570–1579. doi: 10.1109/TPWRS.2005.852086
![]() |
[8] | Deka D, Backhaus S, Chertkov M (2015) Structure learning and statistical estimation in distribution networks-Part II. Eprint Arxiv, 1–1. |
[9] | Bolognani S, Bof N, Michelotti D, et al. (2013) Identification of power distribution network topology via voltage correlation analysis. IEEE Conference on Decision and Control. IEEE, 1659–1664. |
[10] |
Moslehi K, Kumar R (2010) A reliability perspective of the smart grid. IEEE T Smart Grid 1: 57–64. doi: 10.1109/TSG.2010.2046346
![]() |
[11] | Conti S, Rizzo SA, El-Saadany EF, et al. (2014) Reliability assessment of distribution systems considering telecontrolled switches and microgrids. IEEE T Power Syst 29: 598–607. |
[12] |
Rueda JL, Guaman WH, Cepeda JC, et al. (2013) Hybrid approach for power system operational planning with smart grid and small-signal stability enhancement considerations. IEEE T Smart Grid 4: 530–539. doi: 10.1109/TSG.2012.2222678
![]() |
[13] | Moeini-Aghtaie M, Farzin H, Fotuhi-Firuzabad M, et al. (2016) Generalized analytical approach to assess reliability of renewable-based energy hubs. IEEE T Power Syst 32: 368–377. |
[14] |
Minciardi R, Sacile R (2012) Optimal control in a cooperative network of smart power grids. IEEE Syst J 6: 126–133. doi: 10.1109/JSYST.2011.2163016
![]() |
[15] | Zubo RHA, Mokryani G, Rajamani HS, et al. (2016) Operation and planning of distribution networks with integration of renewable distributed generators considering uncertainties: A review. Renew Sust Energ Rev 72: 1177–1198. |
[16] |
Soltani NY, Kim SJ, Giannakis GB (2015) Real-time load elasticity tracking and pricing for electric vehicle charging. IEEE T Smart Grid 6: 1303–1313. doi: 10.1109/TSG.2014.2363837
![]() |
[17] |
Gungor VC, Sahin D, Kocak T, et al. (2011) Smart grid technologies: communication technologies and standards. IEEE T Ind Inform 7: 529–539. doi: 10.1109/TII.2011.2166794
![]() |
[18] |
Hamilton B, Summy M (2011) Benefits of the smart grid [In My View]. IEEE Power Energy M 9: 104–112. doi: 10.1109/MPE.2010.939468
![]() |
[19] |
Ansari N (2013) CONSUMER: A novel hybrid intrusion detection system for distribution networks in smart grid. IEEE T Emerg Top Com 1: 33–44. doi: 10.1109/TETC.2013.2274043
![]() |
[20] |
Conti S, Corte AL, Nicolosi R, et al. (2016) Impact of cyber-physical system vulnerability, telecontrol system availability and islanding on distribution network reliability. Sust Energ Grid Netw 6: 143–151. doi: 10.1016/j.segan.2016.03.003
![]() |
[21] |
Davis KR, Davis CM, Zonouz SA, et al. (2015) A cyber-physical modeling and assessment framework for power grid infrastructures. IEEE T Smart Grid 6: 2464–2475. doi: 10.1109/TSG.2015.2424155
![]() |
[22] |
Hug G, Giampapa JA (2012) Vulnerability assessment of ac state estimation with respect to false data injection cyber-attacks. IEEE T Smart Grid 3: 1362–1370. doi: 10.1109/TSG.2012.2195338
![]() |
[23] | Li X, Poor HV, Scaglione A (2016) Blind topology identification for power systems. IEEE International Conference on Smart Grid Communications. IEEE, 91–96. |
[24] |
Erseghe T, Tomasin S, Vigato A (2013) Topology estimation for smart micro grids via powerline communications. IEEE T Signal Proces 61: 3368–3377. doi: 10.1109/TSP.2013.2259826
![]() |
[25] | Zhao L, Song WZ, Tong L, et al. (2014) Topology identification in smart grid with limited measurements via convex optimization. Innovative Smart Grid Technologies-Asia. IEEE, 803–808. |
[26] |
Singh R, Manitsas E, Pal BC, et al. (2010) A recursive bayesian approach for identification of network configuration changes in distribution system state estimation. IEEE T Power Syst 25: 1329–1336. doi: 10.1109/TPWRS.2010.2040294
![]() |
[27] |
Luan W, Peng J, Maras M, et al. (2015) Smart meter data analytics for distribution network connectivity verification. IEEE T Smart Grid 6: 1964–1971. doi: 10.1109/TSG.2015.2421304
![]() |
[28] |
Chatzarakis GE (2009) Nodal analysis optimization based on the use of virtual current sources: A powerful new pedagogical method. IEEE T Educ 52: 144–150. doi: 10.1109/TE.2008.921459
![]() |
[29] | Phasor Advanced FAQ-Phasor-RTDMS: Available from: http://www.phasor-rtdms.com/phaserconcepts/phasor_adv_faq.html. |
[30] | Vetter WJ (1975) Vector structures and solutions of linear matrix equations. Linear Algebra & Its Applications 10: 181–188. |
[31] | Depablos J, Centeno V, Phadke AG, et al. (2004) Comparative testing of synchronized phasor measurement units. Power Engineering Society General Meeting. IEEE 1: 948–954. |
[32] |
Baran ME, Wu FF (1989) Network reconfiguration in distribution systems for loss reduction and load balancing. IEEE T Power Deliver 4: 1401–1407. doi: 10.1109/61.25627
![]() |
1. | Design and Analysis of the Task Distribution Scheme of Express Center at the End of Modern Logistics, 2019, 8, 2079-9292, 1141, 10.3390/electronics8101141 | |
2. | Yong Tao, Yufang Wen, He Gao, Tianmiao Wang, Jiahao Wan, Jiangbo Lan, A Path-Planning Method for Wall Surface Inspection Robot Based on Improved Genetic Algorithm, 2022, 11, 2079-9292, 1192, 10.3390/electronics11081192 | |
3. | Xiaojiang Yang, Naeem Jan, Optimization Algorithm of Logistics Transportation Cost of Prefabricated Building Components for Project Management, 2022, 2022, 2314-4785, 1, 10.1155/2022/1460335 | |
4. | Luxi Chen, Jia Chen, Gengxin Sun, Path Optimization Model of Rural Red Tourist Attractions Based on Ant Colony Algorithm, 2022, 2022, 1563-5147, 1, 10.1155/2022/9403207 | |
5. | Elias K. Xidias, Paraskevi Th. Zacaharia, Andreas Nearchou, SERobWaS: a support environment for a robot-based warehousing system, 2023, 0268-3768, 10.1007/s00170-023-11349-6 | |
6. | Zhongpeng Zhang, Guibao Wang, LSTM network optimization and task network construction based on heuristic algorithm, 2024, 24, 14727978, 697, 10.3233/JCM-237124 | |
7. | Xiao Zhou, Jun Wang, Wenbing Liu, Juan Pan, Taiping Zhao, Fan Jiang, Rui Li, An Intelligent Connected Vehicle Material Distribution Route Model Based on k-Center Spatial Cellular Clustering and an Improved Cockroach Optimization Algorithm, 2024, 16, 2073-8994, 749, 10.3390/sym16060749 | |
8. | Yinghuan Liu, Chungui Zhou, 2024, Optimization Model and Algorithm Design of Rural Logistics Distribution Center Location Based on Particle Swarm Optimization, 979-8-3503-9563-1, 785, 10.1109/EDPEE61724.2024.00151 | |
9. | Qi Li, 2023, Chapter 72, 978-981-99-2920-7, 799, 10.1007/978-981-99-2921-4_72 | |
10. | Chuang Wu, Jiju Sun, Haithm Yahya Mohammed Almuaalemi, A. S. M. Muhtasim Fuad Sohan, Binfeng Yin, Structural Optimization Design of Microfluidic Chips Based on Fast Sequence Pair Algorithm, 2023, 14, 2072-666X, 1577, 10.3390/mi14081577 | |
11. | Lin Hu, Qinghai Chen, Tingting Yang, Chuanjian Yi, Jing Chen, Visualization and Analysis of Hotspots and Trends in Seafood Cold Chain Logistics Based on CiteSpace, VOSviewer, and RStudio Bibliometrix, 2024, 16, 2071-1050, 6502, 10.3390/su16156502 |
Point | 1 | 2 | 3 |
1 | 0 | 0.54 | 0.48 |
2 | 0.56 | 0 | 0.55 |
3 | 0.17 | 0.04 | 0 |
4 | 0.17 | 0.28 | 0.20 |
5 | 0.32 | 0.16 | 0.34 |
6 | 0.07 | 0.29 | 0.38 |
7 | 0.02 | 0.19 | 0.46 |
8 | 0.26 | 0.02 | 0.21 |
9 | 0.21 | 0.49 | 0.54 |
10 | 0.54 | 0.11 | 0.53 |
11 | 0.25 | 0.52 | 0.10 |
12 | 0.36 | 0.17 | 0.05 |
13 | 0.56 | 0.28 | 0.02 |
14 | 0.58 | 0.44 | 0.09 |
15 | 0.16 | 0.06 | 0.30 |
16 | 0.36 | 0.32 | 0.16 |
17 | 0.13 | 0.11 | 0.07 |
18 | 0.47 | 0.35 | 0.43 |
19 | 0.33 | 0.51 | 0.55 |
20 | 0.10 | 0.42 | 0.08 |
21 | 0.45 | 0.39 | 0.59 |
22 | 0.33 | 0.58 | 0.31 |
23 | 0.40 | 0.33 | 0.34 |
24 | 0.25 | 0.36 | 0.59 |
25 | 0.20 | 0.17 | 0.29 |
Point | 1 | 2 | 3 |
1 | 0 | 0.54 | 0.48 |
2 | 0.56 | 0 | 0.55 |
3 | 0.17 | 0.04 | 0 |
4 | 0.17 | 0.28 | 0.20 |
5 | 0.32 | 0.16 | 0.34 |
6 | 0.07 | 0.29 | 0.38 |
7 | 0.02 | 0.19 | 0.46 |
8 | 0.26 | 0.02 | 0.21 |
9 | 0.21 | 0.49 | 0.54 |
10 | 0.54 | 0.11 | 0.53 |
11 | 0.25 | 0.52 | 0.10 |
12 | 0.36 | 0.17 | 0.05 |
13 | 0.56 | 0.28 | 0.02 |
14 | 0.58 | 0.44 | 0.09 |
15 | 0.16 | 0.06 | 0.30 |
16 | 0.36 | 0.32 | 0.16 |
17 | 0.13 | 0.11 | 0.07 |
18 | 0.47 | 0.35 | 0.43 |
19 | 0.33 | 0.51 | 0.55 |
20 | 0.10 | 0.42 | 0.08 |
21 | 0.45 | 0.39 | 0.59 |
22 | 0.33 | 0.58 | 0.31 |
23 | 0.40 | 0.33 | 0.34 |
24 | 0.25 | 0.36 | 0.59 |
25 | 0.20 | 0.17 | 0.29 |