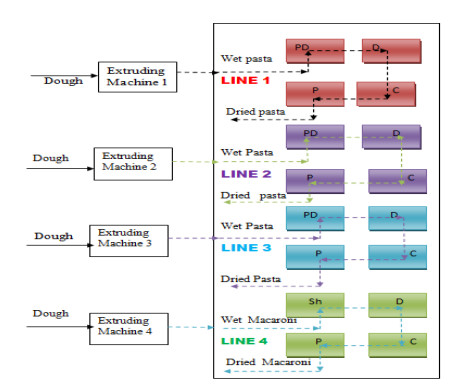
Citation: Qinglei Zhang, Wenying Feng. Detecting Coalition Attacks in Online Advertising: A hybrid data mining approach[J]. Big Data and Information Analytics, 2016, 1(2): 227-245. doi: 10.3934/bdia.2016006
[1] | Badr Ouhammou, Fatima Zohra Gargab, Samir El idrissi kaitouni, Slimane Smouh, Rachid El mrabet, Mohammed Aggour, Abdelmajid Jamil, Tarik Kousksou . Energy saving potential diagnosis for Moroccan university campuses. AIMS Energy, 2023, 11(3): 576-611. doi: 10.3934/energy.2023030 |
[2] | Ali Boharb, A. Allouhi, H. El-houari, H. El Markhi, A. Jamil, T. Kousksou . Energy audit method applied to tertiary buildings: Case study of a University campus. AIMS Energy, 2022, 10(3): 506-532. doi: 10.3934/energy.2022025 |
[3] | Tuan Le-Anh . Energy benchmark and energy saving potential in the pulp and paper industry. AIMS Energy, 2023, 11(6): 1306-1327. doi: 10.3934/energy.2023059 |
[4] | O. Corigliano, G. De Lorenzo, P. Fragiacomo . Techno-energy-economic sensitivity analysis of hybrid system Solid Oxide Fuel Cell/Gas Turbine. AIMS Energy, 2021, 9(5): 934-990. doi: 10.3934/energy.2021044 |
[5] | Alemayehu T. Eneyaw, Demiss A. Amibe . Annual performance of photovoltaic-thermal system under actual operating condition of Dire Dawa in Ethiopia. AIMS Energy, 2019, 7(5): 539-556. doi: 10.3934/energy.2019.5.539 |
[6] | Araz Alizadeh, MohammadBehshad Shafii, Alireza hajiseyed Mirzahosseini, Abtin Ataei . Experimental and simulation investigation of pulsed heat pipes in gas compressors. AIMS Energy, 2020, 8(3): 438-454. doi: 10.3934/energy.2020.3.438 |
[7] | Chris Thankan, August Winters, Jin Ho Jo, Matt Aldeman . Feasibility of applying Illinois Solar for All (ILSFA) to the Bloomington Normal Water Reclamation District. AIMS Energy, 2021, 9(1): 117-137. doi: 10.3934/energy.2021007 |
[8] | Bilal Akbar, Khuram Pervez Amber, Anila Kousar, Muhammad Waqar Aslam, Muhammad Anser Bashir, Muhammad Sajid Khan . Data-driven predictive models for daily electricity consumption of academic buildings. AIMS Energy, 2020, 8(5): 783-801. doi: 10.3934/energy.2020.5.783 |
[9] | Bertrand Tchanche . Energy consumption analysis of the transportation sector of Senegal. AIMS Energy, 2017, 5(6): 912-929. doi: 10.3934/energy.2017.6.912 |
[10] | Carla Viveiros, Rui Melicio, Victor Mendes, Jose Igreja . Adaptive and predictive controllers applied to onshore wind energy conversion system. AIMS Energy, 2018, 6(4): 615-631. doi: 10.3934/energy.2018.4.615 |
Abbreviations: RA: Theoretical air required [kg of air/kg of fuel]; AAS: Actual mass of air supplied per kg of fuel; Cp: Specific heat capacity [J/kg K]; Tf: Flue gas temperature [℃]; Ta: Ambient temperature [℃]; M: mass of moisture in fuel in kg basis [%]; Qin: Heat input [W]; Im: Measured current [A]; Ir: Rated Current [A]; Pin: Power input [W]; L: Electrical loading [%]; η: Efficiency [%]; SEC: Specific Energy Consumption; AEC: Annual Energy consumption [Wh]; AES: Annual energy saving [Wh]; ATEC: Annual thermal energy consumption [Wh]; ASME: American Society of Mechanical Engineers; GCV: Gross Calorific Value; Pf: Power factor [%]; Pr: Rated Power [W]; hy: motor [Wh]; Ee: Electrical energy consumed [Wh]; EEM: Energy efficient motor; PLC: Private Limited Company; ETB: Ethiopian Birr (currency); ASME: American Society of Mechanical Engineers; PTC: Performance Test Code; SME: Specific mechanical energy
It is known that Industries play a great role for the economic development of any country. In many African countries including Ethiopia, industrial developments are expanding which demands a large amount of electrical and thermal energy for various production processes. Since the energy sources are limited, effective and efficient way of utilizing the energy is crucial. Pasta and Macaroni factories are one among a huge energy consuming industries. Thus effective and efficient utilization of energy in such industries has large impact on countries available energy utilization.
Africa PLC Pasta and Macaroni factory is located in the city of Adama which is 100 km far from Addis Ababa, the capital city of Ethiopia and it is the biggest pasta and macaroni producing factory in the city. Since the industry is consuming a very large amount of energy which is observed to increase from time to time and no study has been done before to reason out the raise in energy consumption, a detailed energy performance study is conducted to identify the energy consumption profile of different equipment/processes and the possible energy saving potential of the industry.
The energy analysis is performed on the major energy consuming equipment and systems such as boilers, chillers, electrical motors, lighting system and air compressor, to spot the energy consumption pattern, energy saving potential, emission minimizing possibility and energy bill savings potential.
Currently the industry produces three different pasta products such as; Buona Pasta, Roma Pasta, and Rosa Pasta and three different shapes of macaroni products such as; Spiral, Big elbow, and Small elbow shaped macaroni. Wheat flour is also another product of the company. In this company currently there are two milling houses, two pasta and one macaroni plants are working.
Brunetti et al. [1] studied the energy consumption of monthly pasta production. For the monthly pasta production of 5846 kg of pasta, of which 175 kg is dry pasta the corresponding energy consumption was 1100 kWh of electricity, of which 44.77 kWh was used for dry pasta and 6231.8 kWh of thermal energy, of which 366.2 kWh was used for production of dry pasta. Every kg of monthly produced pasta requires 0.18 kWh of electricity and 1.1 kWh of thermal energy.
Wójtowicz and MoĞcicki [2] have conducted on pasta extrusion process and found the energy consumption was different for each raw material and its moisture during the extrusion and the specific mechanical energy (SME) values at the range from 0.07 to 0.13 kWh/kg during the extrusion-cooking of wheat starch. SME decreased with an increase in moisture content and extrusion temperature. The extent of reduction in SME due to an increase in moisture was lower at a higher temperature in feed materials. The observed SME values are 0.07 kWh/kg during processing of semolina pasta, with moisture content of 28% at 60 rpm and 0.45 kWh/kg when wheat flour type with moisture of 28% was processed at 120 rpm.
Oyedepo et al. [3] presented an analysis of energy use and energy saving opportunities in selected industries in southwest Nigeria and studied the annual energy consumption and production output for the food processing industry are 5,452,467.24 GJ and 197,760 tons, respectively. On the average, the food processing industry has the highest energy consumption per year at 5,039.45 TJ/yr. Food processing and the manufacture of chemicals are complex and energy intensive, often requiring large quantities of thermal energy to convert raw materials to useful products.
Carlsson-Kanyama and Faist [4] had reported a survey of data for estimating energy requirements in the food Sector. As per the study, energy consumption for oil extraction was recorded as in the range of 0.28–1.5 MJ per kg output. Pasta fabrication requires about 0.8–2.4 MJ per kg output and drinks between 2.4–6 MJ per kg output. Also the energy use for sugar extraction show a range of 2.3–26 MJ per kg output and fabrication of candy may require around 6 MJ per kg output.
Perone et al. [5] have numerically studied the possibility of installing a combined heat and power (CHP) plant in a dry pasta factory and found that a suitable energy saving could be achieved in a pasta factory with the correct installation of a medium size cogeneration plant obtained assembling in cluster an appropriate number of micro-turbines.
The industry's primary source of thermal energy is fuel oil and that of electrical energy is the national grid. Exhaustive data collection is carried out using direct observation from process monitoring instruments and physical measurements using portable test instruments like flow meter, thermocouple, digital tachometer, power analyzer and multi-meter for collecting data of flow rates, temperatures and electrical readings. Information about the past maintenance history of the appliances are collected by observation of maintenance history recording legends and interviewing the top and technical personnel of the industry. The overall electrical power consumption history of the industry is analyzed from its electricity bills. In many processes the amounts of energy consumption is variable and depends on the process stage and time elapsed after pasta is introduced for drying, in such cases many instant thermal and electrical readings are taken.
Data from electrical motors are collected for three different production plant lines. Even though there are 4 production plant lines in the factory, plant line-1 is not working. Therefore, the investigation of energy performance is done based on the data of production plant lines 2, 3 and 4 as shown in the Figure 1.
Overall, first the energy consumption data of the production processes of the industry are collected and then the performances of energy consuming units are analyzed. Finally the potential energy consuming areas are identified and the possible energy saving opportunities are analyzed and recommended.
The work flow chart shown in the Figure 2 is used for the energy analysis.
The major sections in the production process of Pasta and Macaroni in the factory is represented in block diagram as shown in Figure 3.
The boilers specifications, fuel properties, flue gas compositions and temperatures as well as the boilers surface temperatures are measured and tabulated as shown in the Tables 1 and 2.
Boiler Fuel properties (Furnace Oil) | Boiler #1 | Boiler #2 | |
C: 84.79% H2: wt 12.21% S: wt 0.5% O2: 0.5% Moisture content: 2% Specific gravity: 0.8461 Flash point: 112.3 ℉ = 44.6 ℃ GCV: 43 MJ/kg, NCV: 38.46 MJ/kg |
Tf = 184 ℃ Ta = 28 ℃ CO2 = 8.4% by volume O2 = 9.7% by volume CO = 7.8% by volume |
Tf = 180 ℃ Ta = 28 ℃ CO2 = 8.6% by volume O2 = 9.98% by volume CO = 15.4% by volume |
Boiler dimensions environmental parameters | Boiler parts | Measured Surface Temperatures | |
Boiler #1 | Boiler #2 | ||
Boiler length = 3.25 m Boiler diameter = 1.3 m Ambient temperature = 28 ℃ Wind velocity = 2.5 m/s |
Cylindrical Surface Front surface Bottom |
65 ℃ 90 ℃ 70 ℃ |
60 ℃ 97 ℃ 73 ℃ |
Thus, the input thermal energy to the factor can be calculated from:
Qin=˙mf∗GCV∗ηb | (1) |
where, Qin—Heat energy input to the boiler,
˙mf—Mass flow rate of fuel Consumed
GCV—Gross calorific value of the fuel
ηb—Combustion efficiency of the burner
In this study an indirect method is used to evaluate boiler efficiency by considering the different loses as per ASME standards for Performance Test Code for Fired Steam Generators [6].
The process heat in the factory is supplied by the fire tube boilers. It is observed that there are four boilers in the factory, were two of them are working and the others two are in standby. In this study the two working boilers performance tests are assessed separately to identify the possible losses and its energy saving opportunities.
In order to calculate the boiler efficiency by indirect method, all the losses that occur in the boiler shall be determined [7,8,9,10,11]. These losses are conveniently related to the amount of fuel burnt.
Theoretical (stoichiometric) air fuel ratio and excess air supplied are determined for computing the boiler losses. Theoretical and actual air required for combustion is calculated as [11]:
a) Theoretical air required for combustion
RA=[(11.6xC)+{34.8x(H2−O2/8)}+(4.35xS)]/100 | (2) |
b) Actual mass of air supplied/ kg of fuel
AAS=(1+EA/100)x theoretical air | (3) |
The various losses associated with the operation of boiler are discussed as below [8,11].
ⅰ) Heat loss due to dry flue gas (%)
Heat loss through the exhaust flue gas can be calculated by the following equation:
L1=(m∗Cp)dryfluegas∗(Tf−Ta)(GCV)fuel∗100% | (4) |
where: Tf is the flue gas temperature
Ta is the ambient temperature
The losses due to water vapor produced from Hydrogen in fuel, moisture present in fuel and air during the combustion were not been included in the dry flue gas loss, since they were separately calculated as a wet flue gas loss.
ⅱ) Heat loss due to evaporation of water formed due to H2in fuel (%)
The combustion of hydrogen causes a heat loss because of the formation of water vapour as a combustion product, which carries away heat energy in the form of latent heat.
L2=9mH2[584+(Cp)watervapour(Tf−Ta)](GCV)fuel∗100% | (5) |
where: Tf is the flue gas temperature
Ta is the ambient temperature
584 = Latent heat corresponding to partial pressure of water vapor
ⅲ) Heat loss due to moisture present in fuel (%)
Moisture entering the boiler with the fuel leaves as a superheated vapor. This moisture loss is made up of the sensible heat to bring the moisture to boiling point, the latent heat of evaporation of the moisture, and the superheat required bringing this hot water to the temperature of the exhaust gas. This loss can be calculated with the following formula.
L3=M[584+(Cp)watervapour(Tf−Ta)](GCV)fuel∗100% | (6) |
where: M is the mass of the moisture in the fuel
Tf is the flue gas temperature
Ta is the ambient temperature which is equivalent to fuel inlet temperature
584 = Latent heat corresponding to partial pressure of water vapor
ⅳ) Heat loss due to moisture present in air (%)
Vapor in the form of humidity present in the incoming air, is superheated as it passes through the boiler. Since this heat passes up the stack, it must be included as a boiler loss. The mass of vapor that air contains can be obtained from psychometric charts and typical values are given as below:
L4=AAS∗humidityfactor∗(Cp)watervapour(Tf−Ta)(GCV)fuel∗100% | (7) |
ⅴ) Heat loss due to incomplete combustion (%)
Products formed by incomplete combustion could be mixed with oxygen and burned again with a further release of energy. Such products include CO, H2 and various hydrocarbons and were generally found in the flue gas of the boilers. Carbon monoxide is the most gas whose concentration can be determined conveniently in a boiler plant test.
L5=[(%CO)∗mC(%CO+%CO2)][5744(GCV)fuel]∗100% | (8) |
where: mC is the mass of carbon atom in the fuel.
ⅵ) Heat loss due to radiation and convection (%)
The other heat losses from a boiler consist of the loss of heat by radiation and convection from the boiler surface to the surrounding boiler house. Energy is lost from the boiler surface to the surrounding by convection due to wind and by radiation due to the temperature difference between the boiler surface and surrounding room wall temperature.
The energy loss per unit area of the boiler surface exposed to the ambient temperature is estimated as:
L6=0.548∗[(Ts55.55)4−(Ta55.55)4]+1.957∗(Ts−Ta)1.25∗√[(196.85Vm+68.9)/68.9] | (9) |
where: Ts is the boiler surface temperature
Ta is the ambient temperature which is equivalent to boiler room wall temperature
In this factory it is observed that there are different motors with different rated power capacities and there are also motors with similar rated power capacities and rated current.
The current and power factors are measured and the power input, loading and efficiency of motors are summarized in the Table 3.
Pr[kW] | Qty | Ir[A] | Im[A] | Pf | % L | Pin[kW] | η |
0.2 | 2 | 0.57 | 0.41 | 0.80 | 68.3 | 0.4 | 0.63 |
0.25 | 11 | 0.72 | 0.34 | 0.76 | 44.9 | 1.9 | 0.66 |
0.5 | 2 | 0.92 | 0.72 | 0.88 | 74.3 | 0.8 | 0.89 |
0.55 | 28 | 1.5 | 0.89 | 0.78 | 56.4 | 12.8 | 0.68 |
0.75 | 94 | 2.1 | 1.3 | 0.76 | 58.8 | 61.1 | 0.68 |
1.1 | 30 | 2.6 | 1.8 | 0.80 | 65.8 | 28.4 | 0.76 |
1.5 | 64 | 3.3 | 2.5 | 0.80 | 72.0 | 84.2 | 0.82 |
2.8 | 27 | 6.1 | 4.48 | 0.82 | 69.8 | 65.3 | 0.81 |
3 | 1 | 7.6 | 5.56 | 0.84 | 69.5 | 3.1 | 0.68 |
3.5 | 4 | 8.2 | 6.86 | 0.84 | 79.5 | 15.2 | 0.73 |
6.5 | 1 | 14.65 | 12.5 | 0.83 | 64.8 | 5.5 | 0.77 |
7.5 | 1 | 19 | 15.56 | 0.76 | 77.8 | 6.0 | 0.75 |
20 | 6 | 50 | 36 | 0.80 | 68.4 | 113.7 | 0.72 |
Industrial motors account for a major part of electrical energy consumption. The amount of energy used by a motor-driven system is directly proportional to the power required by the system times the period of time it is operating. Since power is expressed in kilowatts and time in hours, the conventional unit of energy is kilowatt-hours [kWh]. Power and energy measurements are used to determine loads on equipment, energy consumption, operating costs, and to verify proper system sizing and operation. Power is proportional to the product of circuit voltage (V), amperage or current (I), and power factor (PF). For a three-phase system:
Percentageloading(%L)=Vm∗ImVr∗Ir∗100% | (10) |
Power input (Pin)=√3∗Vm∗Im∗PF | (11) |
where: Vm=380V, Vr=400V
ηmotor = Rated power power input ∗ load | (12) |
Annual energy consumption for electrical motors is estimated by using the following equation:
AEC=Pintotal∗L∗hy | (13) |
The water chiller plant layout in the factory and the actual power input can be represented as shown in the Figure 4.
The heat energy balance for each boiler is represented by Sankey diagrams to understand the different losses and the useful heat energy to the process.
As it can be seen from the Sankey diagrams (Figure 5) about 149.9 kW and 100.45 kW of heat losses are observed from boiler #1 and #2 respectively. This correspondingly accounts for 18.65% and 19.47% of the input energy to the boiler. Further it is can also be estimated that 539.6 MJ and 361.4 MJ thermal energy is lost hourly from boiler #1 and #2 respectively. This indicates 12.55 kg (i.e., 14.83 liter) and 8.15 kg (i.e., 9.63 liter) of fuel is lost per hour and 1.43 million and 2.21 million ETB are lost per annum due to poor efficiency of boiler #1 and #2 respectively.
The detailed annual electrical energy consumption of 199 electrical motors is conducted for production plant lines #2 and #3 with their respective production zones (Table 4). In general 3,386 MWh is consumed by machine driving motors from the inlet to pre dryer, 7,126 MWh is consumed by pre dryer driving motors, 25,374 MWh is consumed by the dryer, 545 MWh is consumed by cooling zone and 368 MWh is consumed by the driving motors from cooling to packaging. Hence, the total annual electrical energy consumption of production plant lines #2 and #3 is 36,799 MWh.
Production process zone | Qty | Pr[kW] | AEC [kWh] | Qty | Pr[kW] | AEC [kWh] |
Inlet-pre dryer[3386010 kWh] | 4 | 20 | 1917389 | 2 | 0.2 | 4786 |
2 | 7.5 | 204458 | 2 | 2.8 | 340589 | |
8 | 0.55 | 173911 | 4 | 3 | 147588 | |
6 | 0.75 | 139074 | 2 | 3.5 | 68482 | |
Pre dryer Zone | 20 | 1.5 | 3784320 | 4 | 2.8 | 273929 |
[7126139 kWh] | 21 | 1.1 | 2796155 | 10 | 0.55 | 271735 |
Dryer Zone | 81 | 0.75 | 25346193 | 1 | 2.8 | 17121 |
[25374183 kWh] | 2 | 0.55 | 10869 | |||
Cooling Zone | 6 | 0.75 | 139074 | 4 | 0.55 | 43478 |
[544865 kWh] | 8 | 1.1 | 405791 | |||
Cooler- packaging | 2 | 0.75 | 15453 | 6 | 0.25 | 35399 |
[368258 kWh] | 4 | 2.8 | 273929 |
The electric motor energy consumption during macaroni production process of plant line #4 with respective production process zones are given in Table 5.
Production process zone | Qty | Pr[kW] | AEC [kWh] | Qty | Pr[kW] | AEC [kWh] |
From inlet—dryer [1,341,960 kWh] |
2 1 3 1 1 2 |
20 7.5 0.55 0.75 0.2 1.5 |
454181 40892 20454 3348 1197 33302 |
1 1 2 5 1 |
6.5 2.8 3 3.5 1.1 |
31221 14797 75494 661599 454181 |
Dryer zone [5,324,488 kWh] |
1 2 5 25 |
0.75 0.5 0.25 1.5 |
3348 10414 16716 5203440 |
1 5 1 |
2.8 0.55 3 |
14797 56817 18955 |
From cooling- packaging [99,081 kWh] |
2 1 |
0.25 0.2 |
2675 1192 |
6 2 |
0.55 0.75 |
81817 3392 |
Specific energy consumption (SEC) is an important factor that determines the energy situation in a typical industry. The total annual specific energy consumption of the factory is identified as 5.478 MJ/kg of the product, of this 0.778 MJ/kg of the product is thermal energy and 4.7 MJ/kg of the product is electrical energy.
In general, after detailed thermal energy consumption analysis is conducted, 26%, 36% and 38% of total annual thermal energy is consumed by driers in the production plant lines 2, 3 and 4 respectively as shown in the Figure 6.
It is observed from the analysis that the industrial driving motors electrical energy consumption of plant line 2, plant line 3 and plant line 4 are about 20%, 25%, and 8% of the total annual electrical energy consumed by the industry respectively and similarly about 47% of the total electrical energy are consumed by the pump motor, air compressor, chiller and lighting systems in the factory (Figure 7).
The zone wise annual electrical energy consumption of production plant lines 2 and 3 show that 3%, 9%, 19% and 69% of the total is consumed by machine drives of cooling to package zone, mixer to pre-dryer zone, pre-dryer zone and dryer zone respectively as shown in the Figure 8.
Similarly the zone wise annual electrical energy consumption of production plant line 4 shows that 1%, 20% and 79% of the total is consumed by machine drives of cooling to package zone, inlet to dryer zone, and dryer zone respectively as shown in the Figure 9.
The overall annual thermal and electrical energy consumption of the factory is summarized in the Figure 10 below.
The total annual electrical energy loss of driving electrical motors of production plant lines 2, 3 and 4 are estimated to be 40.78 TJ/year at an average motors efficiencies of 74% and the total annual electrical energy consumption of 156.84 TJ/year.
The thermal energy loss of the factory conducted through detailed thermal energy loss from the boilers and production lines in kWh per day is summarized in Figure 11.
Overall it is observed that 34.45 TJ/year of thermal energy and 40.78 TJ/year of electrical energy are lost annually from the factory.
There are a lot of energy-efficient technologies and practices that are proven, cost-effective, and available for implementation today. These opportunities are applicable at the component, process, facility, and organizational levels. The following major energy saving potentials are analyzed.
ⅰ. Heat recovery from exhaust dry flue gas to preheat combustion air
ⅱ. Excess combustion air control
ⅲ. Replacing standard electrical motors with energy efficient motors
Combustion air preheating is the most potent way to enhance the boiler efficiency and hot water generation. The temperature of exhaust gas stream can also be used as the source of the heat energy and a heat exchanger can be used to transfer the heat energy to the incoming combustion air [12]. Thus combustion air can gains a large portion of sensible heat that is required to take part in the combustion process. Utilization of recuperators can decreases flue gas temperature and thus, cooling expenses of flue gas, while increasing the temperature of combustion air and saving fuel.
The energy saving analysis of the proposed opportunities is as follows:
ⅰ. Heat recovery from exhaust dry flue gas to preheat combustion air
The amount of heat transfer between flue gas and combustion air is calculated as:
Q=(mCp)air∗(Tairout−Tairin)=(mCp)fluegas∗(Tfin−Tfout) | (14) |
One shell-two tube pass of shell and tube heat exchanger with cold combustion air in the tube side and hot exhaust flue gas in the shell side is used as per the recommendation of [12]. For the purposes of in-tube cleaning larger tube diameters are often required for boilers to be 20 mm [13]. Shell-diameter-to-tube-length ratio is selected to be within limits of 1/5 to 1/15 as per [14] and also the shell diameter is selected to be within the range 150 mm to 1067 mm as per [13].
With application of the heat exchanger and considering the temperature of flue gas to be decreased from 184 ℃ to 140 ℃, which is above the dew point temperature of the dry exhaust flue gas, 30.81 kW of heat recovery potential is obtained. This recovered heat will heat up the combustion air from 20 ℃ to 81.4 ℃.
The increment of combustion air temperature by 20 ℃ leads an increment of 1% boiler efficiency. Thus, the annual thermal energy saved due to the heat recovered from the dry flue gas is estimated as:
AES˙=mf∗GCV[ηnew−ηold]=782.6GJ/year |
Thus the annual thermal energy saved (AES) is 782.6 GJ/year corresponding to 18,200 kg of fuel/year and the estimated saving cost is 387,188 ETB. Also it is possible to use renewable energy sources like solar energy to preheat the air by using solar collectors like back pass non-perforated unglazed solar air collector (BPSC) [17].
ⅱ. Excess combustion air control
Excess air is required in all practical cases to ensure complete combustion. The optimum excess air level for boiler efficiency which uses diesel fuel oil is recommended to be 15–20%. Controlling excess air to an optimum level always results in reduction in flue gas losses; for every 1% reduction in excess air there is approximately 0.6% rise in boiler efficiency [8,15].
Thus, the possible annual thermal energy save due to reduction of excess air to a minimum of 20% is, AES = 1,221 GJ/year. This corresponds to about 33,560 liter fuels and thus 604,085 ETB can be saved annually.
ⅲ. Replacing the conventional electric motors by energy efficient motors
The recommended operating hours of standard electrical motors is less than 5000 hrs annually [15]. Motors operating at the above stated conditions should be replaced with the energy efficient motors in order to avoid the major power loss. Accordingly, the normal motors used in this factory shall be replaced with the energy efficient motors (EEM). Thus the annual electrical energy saving potential due to replacing of normal motors with energy efficient motors is estimated by using the following equation:
AES=hp∗L∗0.746∗hy∗Nm∗{1ηnm−1ηee}∗100 | (15) |
The efficiency of standard and high efficiency motors at different loads is provided by [16]. Considering 75% load, the annual electrical energy saving potential by replacing the normal standard motors that are equipped in the production plant lines 2, 3 and 4 with an energy efficient motors is obtained to be AES = 262,681.4 kWh. This corresponds to an annual bill savings of 685,598 ETB.
Finally, a total of 2,003.6 GJ/year of thermal energy and 2,301 GJ/year of electrical energy can be saved respectively.
The overall energy savings are summarized as in Table 6.
Energy saving opportunity | Annual energy saving | Annual bill saving [ETB] | Additional investment to implement energy saving opportunities [ETB] | Payback period | |
1. | Heat recovery from dry exhaust flue gas | 782.6 GJ/year | 387,188 | 355,928.6 | 336 days |
2. | Excess air controlling using oxygen analyzer | 1,221 GJ/year | 604,085 | 71,256 | 43 days |
3. | Replacement of normal motors by energy efficient motors | 2,301 GJ/year | 685,598 | 423,683 | 226 days |
Adopting energy saving opportunities in manufacturing industries is needed for ensuring a sustainable industrial development. In this study the Africa PLC factory's energy consumption performance and possible energy saving opportunities are investigated.
Different instruments are used to measure actual values of current, power factor, flue gas characteristics, and flow rates, surface temperature of the boiler, temperature of hot water and different laboratory measurements of the industry are used. For the case where direct measurement is difficult data are taken from reading boards. The data are then analyzed to investigate the energy performance of energy consuming equipment and conducted the annual energy consumption patterns in the factory.
Fire tube boilers are significant portion of the factory to facilitate the drying process. To evaluate the efficiency of the boiler and the amount of heat that is wasted from the boiler, performance analysis of boiler is performed and obtained that a typical boiler efficiency of 81% for boiler #1 and 80% for boiler #2. The remaining part of the energy is lost in different forms and the amounts of heat losses are quantified and the potential sources of waste heat are identified.
The results of the energy performance analysis in the factory has showed that 26%, 36% and 38% of total annual thermal energy is consumed by production plant lines 2, 3 and 4 respectively. Similarly, It is observed that 20%, 25%, 8% of the total annual electrical energies are consumed the driving motors of plant lines 2, 3 and 4 respectively and about 47% of the total electrical energy is consumed by the pump motors, air compressors, chillers and lighting systems in the factory.
Further it is observed that 34.45 TJ/year of thermal energy and 40.78 TJ/year of electrical energy are lost annually from the industry which is a significant amount and need to be recovered.
Using different energy saving opportunities 2,003 GJ/year of total thermal energy 2,301 GJ/year of total electrical energy can be saved which corresponds to an annual saving of 1,676,871 ETB.
All authors declare no conflict of interest in this paper.
[1] | [ Zenithoptimedia, Available from http://techcrunch.com/2014/04/07/. |
[2] | [ Doubleclick by Google, Available from:http://www.google.ca/doubleclick/. |
[3] | [ Rightmedia from Yahoo!, Available from:https://www.rightmedia.com/. |
[4] | [ Disco MapReduce, Available from http://discoproject.org/. |
[5] | [ Hadoop MapReduce, Available from:https://hadoop.apache.org. |
[6] | [ L. Adamic and E. Adar, Friends and neighbors on the web, Social Networks, 25(2003), 211-230. |
[7] | [ V. Anupam, A. Mayer, K. Nissim and B. Pinkas, On the security of pay-per-click and other web advertising schemes, in The 8th International Conference on World Wide Web, 31(1999), 1091-1100. |
[8] | [ M. S. Charikar, Similarity estimation techniques from rounding algorithms, in Proceedings of the Thiry-fourth Annual ACM Symposium on Theory of Computing, (2002), 380-388. |
[9] | [ N. Daswani, C. Mysen, V. Rao, S. Weis, K. Gharachorloo and S. Ghosemajumde, Online advertising fraud, in Crimeware:Understanding New Attacks and Defenses, Addison-Wesley Professional, Reading, 2008. |
[10] | [ M. A. Hasan, A survey of link prediction in social networks, Social Network Data Analytics, (2011), 243-275. |
[11] | [ B. Kitts, J. Y. Zhang, A. Roux and R. Mills, Click fraud detection with bot signatures, in Proceedings of ISI 2013, Seattle, Washington, USA, (2013), 146-150. |
[12] | [ C. Kim, H. Miao and K. Shim, CATCH:A detecting algorithm for coalition attacks of hit inflation in internet advertising, Information Systems, 36(2011), 1105-1123. |
[13] | [ C. K. S. Leung, Anti-monotone constraints, in Encyclopedia of Database Systems (eds. Ling Liu and M. Tamer Özsu), Springer, (2009), 98-98. |
[14] | [ K. Lee, H. Choi and B. Moon, Parallel data processing with MapReduce:a survey, in The ACM Special Interest Group on Management of Data Record, 40(2011), 11-20. |
[15] | [ A. Metwally, D. Agrawal and A. EI Abbadi, Duplicate detection in click streams, in International World Wide Web Conference, (2005), 12-21. |
[16] | [ A. Metwally, D. Agrawal and A. EI Abbadi, Using association rules for fraud detection in web advertising networks, in Proceedings of the 31st international conference on very large data bases, (2005), 169-180. |
[17] | [ A. Metwally, D. Agrawal and A. EI Abbadi, Detectives:detecting coalition hit inflation attacks in advertising networks streams, in Proceedings of the 16th International Conference on World Wide Web, (2007), 241-250. |
[18] | [ A. Metwally, D. Agrawal, A. EI Abbadi and Q. Zheng, On hit inflation techniques and detection in streams of web advertising networks, in Proceedings of the 27th International Conference on Distributed Computing Systems, (2007), 52-52. |
[19] | [ C. Phua, E. Y. Cheu, G. E. Yap, K. Sim and M. N. Nguyen, Feature engineering for click fraud detection, in ACML Workshop on Fraud Detection in Mobile Advertising, 2012. |
[20] | [ Y. Peng, L. Zhang, J. M. Chang and Y. Guan, An effective method for combating malicious scripts clickbots, in Computer Security, the Series Lecture Notes in Computer Science, 5789(2009), 523-538. |
[21] | [ B. K. Perera, A Class Imbalance Learning Approach to Fraud Detection in Online Advertising, M.Sc. thesis, Masdar Institute of Science and Technology, 2013. |
[22] | [ K. Springborn and P. Barford, Impression fraud in online advertising via pay-per-view networks, in Proceedings of the 22nd USENIX Security Symposium, (2013), 211-226. |
[23] | [ F. Soldo and A. Metwally, Traffic anomaly detection based on the IP size distribution, in Proceedings of the INFOCOM International Conference on Computer Communications, Joint Conference of the IEEE Computer and Communications Societies, (2012), 2005-2013. |
[24] | [ C. Walgampaya and M. Kantardzic, Cracking the smart clickbot, in Proceedings of the 13th IEEE International Symposium on Web Systems Evolution, (2011), 125-134. |
[25] | [ C. Walgampaya and M. Kantardzic and R. Yampolskiy, Evidence fusion for real time click fraud detection and prevention, in Intelligent Automation and Systems Engineering, Lecture Notes in Electrical Engineering, Springer Science+Business Media, 103(2011), 1-14. |
[26] | [ Q. Zhang and W. Feng, Detecting coalition frauds in online-advertising, in Mathematical and Computational Approaches in Advancing Modern Science and Engineering, (eds. Jacques Bélair, Ian A. Frigaard, Herb Kunze, Roman Makarov, Roderick Melnik and Raymond J. Spiteri) Springer, (2016), 595-605. |
[27] | [ L. Zhang and Y. Guan, Detecting click fraud in pay-per-click streams of online advertising networks, in The 28th International Conference on Distributed Computing Systems, (2008), 77-84. |
Boiler Fuel properties (Furnace Oil) | Boiler #1 | Boiler #2 | |
C: 84.79% H2: wt 12.21% S: wt 0.5% O2: 0.5% Moisture content: 2% Specific gravity: 0.8461 Flash point: 112.3 ℉ = 44.6 ℃ GCV: 43 MJ/kg, NCV: 38.46 MJ/kg |
Tf = 184 ℃ Ta = 28 ℃ CO2 = 8.4% by volume O2 = 9.7% by volume CO = 7.8% by volume |
Tf = 180 ℃ Ta = 28 ℃ CO2 = 8.6% by volume O2 = 9.98% by volume CO = 15.4% by volume |
Boiler dimensions environmental parameters | Boiler parts | Measured Surface Temperatures | |
Boiler #1 | Boiler #2 | ||
Boiler length = 3.25 m Boiler diameter = 1.3 m Ambient temperature = 28 ℃ Wind velocity = 2.5 m/s |
Cylindrical Surface Front surface Bottom |
65 ℃ 90 ℃ 70 ℃ |
60 ℃ 97 ℃ 73 ℃ |
Pr[kW] | Qty | Ir[A] | Im[A] | Pf | % L | Pin[kW] | η |
0.2 | 2 | 0.57 | 0.41 | 0.80 | 68.3 | 0.4 | 0.63 |
0.25 | 11 | 0.72 | 0.34 | 0.76 | 44.9 | 1.9 | 0.66 |
0.5 | 2 | 0.92 | 0.72 | 0.88 | 74.3 | 0.8 | 0.89 |
0.55 | 28 | 1.5 | 0.89 | 0.78 | 56.4 | 12.8 | 0.68 |
0.75 | 94 | 2.1 | 1.3 | 0.76 | 58.8 | 61.1 | 0.68 |
1.1 | 30 | 2.6 | 1.8 | 0.80 | 65.8 | 28.4 | 0.76 |
1.5 | 64 | 3.3 | 2.5 | 0.80 | 72.0 | 84.2 | 0.82 |
2.8 | 27 | 6.1 | 4.48 | 0.82 | 69.8 | 65.3 | 0.81 |
3 | 1 | 7.6 | 5.56 | 0.84 | 69.5 | 3.1 | 0.68 |
3.5 | 4 | 8.2 | 6.86 | 0.84 | 79.5 | 15.2 | 0.73 |
6.5 | 1 | 14.65 | 12.5 | 0.83 | 64.8 | 5.5 | 0.77 |
7.5 | 1 | 19 | 15.56 | 0.76 | 77.8 | 6.0 | 0.75 |
20 | 6 | 50 | 36 | 0.80 | 68.4 | 113.7 | 0.72 |
Production process zone | Qty | Pr[kW] | AEC [kWh] | Qty | Pr[kW] | AEC [kWh] |
Inlet-pre dryer[3386010 kWh] | 4 | 20 | 1917389 | 2 | 0.2 | 4786 |
2 | 7.5 | 204458 | 2 | 2.8 | 340589 | |
8 | 0.55 | 173911 | 4 | 3 | 147588 | |
6 | 0.75 | 139074 | 2 | 3.5 | 68482 | |
Pre dryer Zone | 20 | 1.5 | 3784320 | 4 | 2.8 | 273929 |
[7126139 kWh] | 21 | 1.1 | 2796155 | 10 | 0.55 | 271735 |
Dryer Zone | 81 | 0.75 | 25346193 | 1 | 2.8 | 17121 |
[25374183 kWh] | 2 | 0.55 | 10869 | |||
Cooling Zone | 6 | 0.75 | 139074 | 4 | 0.55 | 43478 |
[544865 kWh] | 8 | 1.1 | 405791 | |||
Cooler- packaging | 2 | 0.75 | 15453 | 6 | 0.25 | 35399 |
[368258 kWh] | 4 | 2.8 | 273929 |
Production process zone | Qty | Pr[kW] | AEC [kWh] | Qty | Pr[kW] | AEC [kWh] |
From inlet—dryer [1,341,960 kWh] |
2 1 3 1 1 2 |
20 7.5 0.55 0.75 0.2 1.5 |
454181 40892 20454 3348 1197 33302 |
1 1 2 5 1 |
6.5 2.8 3 3.5 1.1 |
31221 14797 75494 661599 454181 |
Dryer zone [5,324,488 kWh] |
1 2 5 25 |
0.75 0.5 0.25 1.5 |
3348 10414 16716 5203440 |
1 5 1 |
2.8 0.55 3 |
14797 56817 18955 |
From cooling- packaging [99,081 kWh] |
2 1 |
0.25 0.2 |
2675 1192 |
6 2 |
0.55 0.75 |
81817 3392 |
Energy saving opportunity | Annual energy saving | Annual bill saving [ETB] | Additional investment to implement energy saving opportunities [ETB] | Payback period | |
1. | Heat recovery from dry exhaust flue gas | 782.6 GJ/year | 387,188 | 355,928.6 | 336 days |
2. | Excess air controlling using oxygen analyzer | 1,221 GJ/year | 604,085 | 71,256 | 43 days |
3. | Replacement of normal motors by energy efficient motors | 2,301 GJ/year | 685,598 | 423,683 | 226 days |
Boiler Fuel properties (Furnace Oil) | Boiler #1 | Boiler #2 | |
C: 84.79% H2: wt 12.21% S: wt 0.5% O2: 0.5% Moisture content: 2% Specific gravity: 0.8461 Flash point: 112.3 ℉ = 44.6 ℃ GCV: 43 MJ/kg, NCV: 38.46 MJ/kg |
Tf = 184 ℃ Ta = 28 ℃ CO2 = 8.4% by volume O2 = 9.7% by volume CO = 7.8% by volume |
Tf = 180 ℃ Ta = 28 ℃ CO2 = 8.6% by volume O2 = 9.98% by volume CO = 15.4% by volume |
Boiler dimensions environmental parameters | Boiler parts | Measured Surface Temperatures | |
Boiler #1 | Boiler #2 | ||
Boiler length = 3.25 m Boiler diameter = 1.3 m Ambient temperature = 28 ℃ Wind velocity = 2.5 m/s |
Cylindrical Surface Front surface Bottom |
65 ℃ 90 ℃ 70 ℃ |
60 ℃ 97 ℃ 73 ℃ |
Pr[kW] | Qty | Ir[A] | Im[A] | Pf | % L | Pin[kW] | η |
0.2 | 2 | 0.57 | 0.41 | 0.80 | 68.3 | 0.4 | 0.63 |
0.25 | 11 | 0.72 | 0.34 | 0.76 | 44.9 | 1.9 | 0.66 |
0.5 | 2 | 0.92 | 0.72 | 0.88 | 74.3 | 0.8 | 0.89 |
0.55 | 28 | 1.5 | 0.89 | 0.78 | 56.4 | 12.8 | 0.68 |
0.75 | 94 | 2.1 | 1.3 | 0.76 | 58.8 | 61.1 | 0.68 |
1.1 | 30 | 2.6 | 1.8 | 0.80 | 65.8 | 28.4 | 0.76 |
1.5 | 64 | 3.3 | 2.5 | 0.80 | 72.0 | 84.2 | 0.82 |
2.8 | 27 | 6.1 | 4.48 | 0.82 | 69.8 | 65.3 | 0.81 |
3 | 1 | 7.6 | 5.56 | 0.84 | 69.5 | 3.1 | 0.68 |
3.5 | 4 | 8.2 | 6.86 | 0.84 | 79.5 | 15.2 | 0.73 |
6.5 | 1 | 14.65 | 12.5 | 0.83 | 64.8 | 5.5 | 0.77 |
7.5 | 1 | 19 | 15.56 | 0.76 | 77.8 | 6.0 | 0.75 |
20 | 6 | 50 | 36 | 0.80 | 68.4 | 113.7 | 0.72 |
Production process zone | Qty | Pr[kW] | AEC [kWh] | Qty | Pr[kW] | AEC [kWh] |
Inlet-pre dryer[3386010 kWh] | 4 | 20 | 1917389 | 2 | 0.2 | 4786 |
2 | 7.5 | 204458 | 2 | 2.8 | 340589 | |
8 | 0.55 | 173911 | 4 | 3 | 147588 | |
6 | 0.75 | 139074 | 2 | 3.5 | 68482 | |
Pre dryer Zone | 20 | 1.5 | 3784320 | 4 | 2.8 | 273929 |
[7126139 kWh] | 21 | 1.1 | 2796155 | 10 | 0.55 | 271735 |
Dryer Zone | 81 | 0.75 | 25346193 | 1 | 2.8 | 17121 |
[25374183 kWh] | 2 | 0.55 | 10869 | |||
Cooling Zone | 6 | 0.75 | 139074 | 4 | 0.55 | 43478 |
[544865 kWh] | 8 | 1.1 | 405791 | |||
Cooler- packaging | 2 | 0.75 | 15453 | 6 | 0.25 | 35399 |
[368258 kWh] | 4 | 2.8 | 273929 |
Production process zone | Qty | Pr[kW] | AEC [kWh] | Qty | Pr[kW] | AEC [kWh] |
From inlet—dryer [1,341,960 kWh] |
2 1 3 1 1 2 |
20 7.5 0.55 0.75 0.2 1.5 |
454181 40892 20454 3348 1197 33302 |
1 1 2 5 1 |
6.5 2.8 3 3.5 1.1 |
31221 14797 75494 661599 454181 |
Dryer zone [5,324,488 kWh] |
1 2 5 25 |
0.75 0.5 0.25 1.5 |
3348 10414 16716 5203440 |
1 5 1 |
2.8 0.55 3 |
14797 56817 18955 |
From cooling- packaging [99,081 kWh] |
2 1 |
0.25 0.2 |
2675 1192 |
6 2 |
0.55 0.75 |
81817 3392 |
Energy saving opportunity | Annual energy saving | Annual bill saving [ETB] | Additional investment to implement energy saving opportunities [ETB] | Payback period | |
1. | Heat recovery from dry exhaust flue gas | 782.6 GJ/year | 387,188 | 355,928.6 | 336 days |
2. | Excess air controlling using oxygen analyzer | 1,221 GJ/year | 604,085 | 71,256 | 43 days |
3. | Replacement of normal motors by energy efficient motors | 2,301 GJ/year | 685,598 | 423,683 | 226 days |