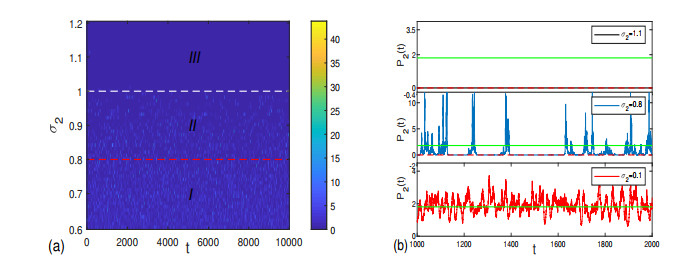
The development of tolerant rice varieties using doubled-haploid technology is necessary to speed up the release of a variety tolerant to salinity stress. However, this requires a reliable screening method and selection index model for enhancing selection effectiveness. One approach is through the development of a selection index based on factor analysis under soil salinity screening in the greenhouse. The objective of this study was to develop a selection index model based on factor analysis and select tolerant doubled-haploid lines under high salinity conditions. The experimental design used was a split-plot design with salinity stress treatments as the main plot, i.e., normal (0 mM NaCl) and saline (25 mM NaCl ~ 5.6–5.8 dS/m) and 42 genotypes as the subplot. The genotypes consisted of 36 doubled-haploid lines, four commercial varieties, and two check varieties. The results indicated that a salinity selection index model involving yield and productive tiller traits could be used for selecting rice genotypes tolerant to salinity stress in soil artificial screening. This index which was developed through a combination of factor analysis, stress tolerance index (STI), and path analyses have identified 15 doubled haploid rice lines which were considered as good tolerant lines under salinity stress in soil artificial screening.
Citation: Muhammad Fuad Anshori, Bambang Sapta Purwoko, Iswari Saraswati Dewi, Willy Bayuardi Suwarno, Sintho Wahyuning Ardie. Salinity tolerance selection of doubled-haploid rice lines based on selection index and factor analysis[J]. AIMS Agriculture and Food, 2022, 7(3): 520-535. doi: 10.3934/agrfood.2022032
[1] | Saswati Biswas, Pankaj Kumar Tiwari, Yun Kang, Samares Pal . Effects of zooplankton selectivity on phytoplankton in an ecosystem affected by free-viruses and environmental toxins. Mathematical Biosciences and Engineering, 2020, 17(2): 1272-1317. doi: 10.3934/mbe.2020065 |
[2] | Juan Li, Yongzhong Song, Hui Wan, Huaiping Zhu . Dynamical analysis of a toxin-producing phytoplankton-zooplankton model with refuge. Mathematical Biosciences and Engineering, 2017, 14(2): 529-557. doi: 10.3934/mbe.2017032 |
[3] | Zhenyao Sun, Da Song, Meng Fan . Dynamics of a stoichiometric phytoplankton-zooplankton model with season-driven light intensity. Mathematical Biosciences and Engineering, 2024, 21(8): 6870-6897. doi: 10.3934/mbe.2024301 |
[4] | Xin Zhao, Lijun Wang, Pankaj Kumar Tiwari, He Liu, Yi Wang, Jianbing Li, Min Zhao, Chuanjun Dai, Qing Guo . Investigation of a nutrient-plankton model with stochastic fluctuation and impulsive control. Mathematical Biosciences and Engineering, 2023, 20(8): 15496-15523. doi: 10.3934/mbe.2023692 |
[5] | Xinyou Meng, Jie Li . Stability and Hopf bifurcation analysis of a delayed phytoplankton-zooplankton model with Allee effect and linear harvesting. Mathematical Biosciences and Engineering, 2020, 17(3): 1973-2002. doi: 10.3934/mbe.2020105 |
[6] | Jean-Jacques Kengwoung-Keumo . Dynamics of two phytoplankton populations under predation. Mathematical Biosciences and Engineering, 2014, 11(6): 1319-1336. doi: 10.3934/mbe.2014.11.1319 |
[7] | Yuanpei Xia, Weisong Zhou, Zhichun Yang . Global analysis and optimal harvesting for a hybrid stochastic phytoplankton-zooplankton-fish model with distributed delays. Mathematical Biosciences and Engineering, 2020, 17(5): 6149-6180. doi: 10.3934/mbe.2020326 |
[8] | Ruiqing Shi, Jianing Ren, Cuihong Wang . Stability analysis and Hopf bifurcation of a fractional order mathematical model with time delay for nutrient-phytoplankton-zooplankton. Mathematical Biosciences and Engineering, 2020, 17(4): 3836-3868. doi: 10.3934/mbe.2020214 |
[9] | Jean-Jacques Kengwoung-Keumo . Competition between a nonallelopathic phytoplankton and an allelopathic phytoplankton species under predation. Mathematical Biosciences and Engineering, 2016, 13(4): 787-812. doi: 10.3934/mbe.2016018 |
[10] | Zhiwei Huang, Gang Huang . Mathematical analysis on deterministic and stochastic lake ecosystem models. Mathematical Biosciences and Engineering, 2019, 16(5): 4723-4740. doi: 10.3934/mbe.2019237 |
The development of tolerant rice varieties using doubled-haploid technology is necessary to speed up the release of a variety tolerant to salinity stress. However, this requires a reliable screening method and selection index model for enhancing selection effectiveness. One approach is through the development of a selection index based on factor analysis under soil salinity screening in the greenhouse. The objective of this study was to develop a selection index model based on factor analysis and select tolerant doubled-haploid lines under high salinity conditions. The experimental design used was a split-plot design with salinity stress treatments as the main plot, i.e., normal (0 mM NaCl) and saline (25 mM NaCl ~ 5.6–5.8 dS/m) and 42 genotypes as the subplot. The genotypes consisted of 36 doubled-haploid lines, four commercial varieties, and two check varieties. The results indicated that a salinity selection index model involving yield and productive tiller traits could be used for selecting rice genotypes tolerant to salinity stress in soil artificial screening. This index which was developed through a combination of factor analysis, stress tolerance index (STI), and path analyses have identified 15 doubled haploid rice lines which were considered as good tolerant lines under salinity stress in soil artificial screening.
Plankton, the organisms that the freely floating and weakly swimming in aquatic environments, occupy the first tropic level and the second trophic level of any aquatic food chains. Phytoplankton are the photosynthetic microorganisms and commonly unicellular and microscopic in size, and zooplankton are the heterotrophic plankton that live on phytoplankton. In addition to recognizing the importance of plankton for the wealth of the aquatic ecosystems and ultimately for the planet itself [1], the variation of plankton biomass is an important factor influencing the real aquatic environments, and understanding of plankton dynamics can be helpful to estimate the productivity of aquatic ecosystems [2,3] and regulate the balance of plankton ecosystem. However, planktonic blooms can occur under some conducive environments, which may cause seriously environmental issues and threat to human health. But the processes underlying the formation of planktonic blooms are not yet well understood. In this respect, thus, the great effort has been made towards the understanding of the complex dynamics of plankton, and then mathematical models can be acted as a useful tool to investigate the dynamics of plankton ecosystem, which can provide a deeper understanding of the dynamic mechanisms of changes in plankton populations.
Actually, many mathematical models have been constructed to study the dynamical behaviors of plankton since the pioneering work of Riley et al. [4], and many physical and biological processes underlying the mechanisms of plankton dynamics in the aquatic environments have been investigated [1,5,6,7,8,9,10]. For example, in order to study how the nutrient affects the dynamics of phytoplankton blooms, Huppert et al. [1] presented a simple nutrient-phytoplankton model and identified an important threshold effect that a bloom will only be triggered when nutrients exceed a certain defined level using mathematical model analysis. Caperon [6] concluded that the time-lag effect exists in the growth process of phytoplankton, and further suggested that models play an important role in understanding the growth dynamics of phytoplankton characterized by time delays. Lin et al. [8] used a nutrient-phytoplankton-zooplankton model to examine the patterns and consequences of adaptive changes in plankton body size and suggested that evolutionary interactions between phytoplankton and zooplankton may have contributed to observed changes in phytoplankton sizes and associated biogeochemical cycle over geological time scales.
In recent years, the dynamical behaviors of phytoplankton-zooplankton systems with various biological factors, such as stability, bifurcation and spatiotemporal pattern, have been explored extensively [6,10,11,12]. Nevertheless, some phytoplankton species are harmful phytoplankton that can produce potent toxic or allelopathic substances during phytoplankton blooms [13], which can affect species interaction by suppressing the growth and establishment of other phytoplankton species [14]. Moreover, some laboratory experiments [15,16], as well as field observation [17] have suggested that the toxicity may be as a strong mediator in the zooplankton feeding rate. As a result, some researchers have taken this important factor of toxic production released by TPP into account when studying the phytoplankton-zooplankton systems [18,19,20]. For example, Scotti et al. [18] indicated that a toxic phytoplankton may destabilize the spatially homogeneous coexistence and trigger the formation of spatial pattern, and further concluded that local blooms more likely occur when the strength of the toxicity is of a certain level. Additionally, some results from field observations and model analysis concluded that the toxic substances can affect the interaction between phytoplankton and zooplankton and reduce the growth of zooplankton, indicating TPP may act as a biological control way for the termination of planktonic blooms [21,22,23,24]. Sarkar et al. [25] proposed the following mathematical model consisting of two harmful phytoplankton and zooplankton species:
{dP1(t)dt=r1P1(t)(1−P1(t)K1)−a1P1(t)P2(t)−αP1(t)Z(t),dP2(t)dt=r2P2(t)(1−P2(t)K2)−a2P1(t)P2(t)−βP2(t)Z(t),dZ(t)dt=mP1(t)Z(t)+nP2(t)Z(t)−γP1(t)Z(t)a+P1(t)−δP2(t)Z(t)b+P2(t)−dZ(t), | (1) |
where P1(t),P2(t) and Z(t) are the population densities of two harmful phytoplankton and zooplankton species at time t, respectively; r1 and r2 denote the intrinsic growth rates of two TPP, respectively; K1 and K2 are their corresponding environmental carrying capacities; a1 and a2 represent be the inhibitory effects of two harmful phytoplankton species; α and β are the maximum zooplankton ingestion rates for both two TPP species, respectively; m and n are the maximum zooplankton conversion rates, respectively; d is the natural death rate of zooplankton; γ and δ are the rates of toxin liberation by two TPP species, respectively; a and b denote the half-saturation constants for two TPP species. In [25], the authors studied the asymptotic stability of the system (1) and claimed that the presence of two harmful phytoplankton has a positive impact for the termination of planktonic blooms.
In the real world, however, the unpredictability and ubiquity of environmental fluctuations in the natural aquatic ecosystems, for example, the necessary nutrient availability, water temperature, light and turbulence, can greatly cause the growths of plankton populations to experience random fluctuations. Systems with such kinds of environmental fluctuations can be described by stochastic differential equations, which play a significant role in the population dynamics as they can provide some additional degree of realism compared to their corresponding deterministic counterparts [26]. Thus, stochastic population systems, as an important application in ecological and biological systems, have attracted increasing attention [27,28,29,30,31,32,33]. Especially, stochastic plankton systems with white noise have been the common area of interest among researchers [9,23,30,34,35,36,37,38,39,40,41,42] in recent years and many interesting results have been shown. For example, Sarkar and Chattopadhayay [34] proposed a toxic phytoplankton-non-toxic phytoplankton-zooplankton with stochastic perturbation around the positive equilibrium, and they concluded that TPP and stochastic fluctuations can significantly affect the coexistence of species. Yu et al. [41] investigated a nutrient-phytoplankton system with TPP under environmental fluctuations, and they obtained some conditions for extinction, persistence and the existence of ergodic stationary distribution. All these works greatly stimulate researchers to explore the way how environmental stochasticity and toxin production affect the coexistence and survival prospect of plankton populations in the presence of harmful phytoplankton. Obviously, it is meaningful to further incorporate the environmental fluctuations into the underlying model (1). Moreover, there are few literatures to study the dynamics of the stochastic phytoplankton-zooplankton system with two harmful phytoplankton, and the dynamics of the stochastic phytoplankton-zooplankton system with two harmful phytoplankton is still not very clear currently. Hence, we mainly present the influence of the effects of environmental white noise and toxic liberation rates produced by two TPP on the dynamics of phytoplankton-zooplankton system in this paper. Motivated by the works above, we assume that the intrinsic growth rates of two harmful phytoplankton and the death rate of zooplankton are influenced by the environmental fluctuations effect, and thus introduce the white noise into underlying system (1), resulting in the following form:
{dP1(t)=P1(t)[r1(1−P1(t)K1)−a1P2(t)−αZ(t)]dt+σ1P1(t)dB1(t),dP2(t)=P2(t)[r2(1−P2(t)K2)−a2P1(t)−βZ(t)]dt+σ2P2(t)dB2(t),dZ(t)=Z(t)[mP1(t)+nP2(t)−γP1(t)a+P1(t)−δP2(t)b+P2(t)−d]dt+σ3Z(t)dB3(t), | (2) |
where Bi(t) are mutually independent standard Brownian motions with Bi(0)=0 [43], and dBi(t) are standard white noise and σ2i(t) are their intensities, i=1,2,3.
By now, we have successful introduced a stochastic phytoplankton-zooplankton system with two toxic phytoplankton focusing on the effects of environmental stochasticity and TPP, and our research questions include: (i) How does environmental stochasticity affects the dynsmics of plankton populations? (ii) What influences the peak of the outbreaks of planktonic blooms in a fluctuating environment? The rest of this paper is organized as follows: Section 2 presents the basic assumptions firstly, and then we investigate the existence and uniqueness of global positive solutions, and apply the Itˆo's formula to obtain the sufficient conditions for the extinction and persistence in the mean of system (2), and the existence of a unique ergodic stationary distribution by establishing a appropriate stochastic Lyapunov function. A series of numerical simulations are carried out to further study the dynamics of system (2) in section 3. In section 4, we summarize the results and present our conclusions.
In this section, we investigate mainly the existence and uniqueness of global positive solutions, the extinction and persistence in the mean of system (2), and discuss the positive recurrence and ergodic property of system (2) as well.
Denote R+=[0,+∞) and Rn+={(x1,⋯,xn)∈Rn:xi>0,i=1,2,⋯,n}, and |x|=√∑ni=1x2i. Throughout this paper, unless otherwise indicated, we always assume that (Ω,Ft,{Ft}t≥0,P) is a completed probability space with a filtration {Ft} satisfying the usual normal conditions (i.e., it is right-continuous and increasing while {F0} contains all P-null sets). For convenience, if φ(t) is a integrable function on R+, we define ⟨φ⟩=1T∫T0φ(s)ds,T>0.
Generally, we consider the n-dimentional stochastic differential equation:
dx(t)=f(x(t),t)dt+g(x(t),t)dB(t),t∈[t0,T] | (3) |
with initial value x(t0)=x0∈Rn, where B(t) denotes a n-dimentional standard Brownian motion defined on the completed probability space (Ω,Ft,{Ft}t≥0,P). Denote by C2,1(Rn×R+;R) the family of all non-negative functions V(x,t) defined on Rn×R+ such that they are continuously twice differentiable in x and once in t. Define a differential operator L associated with Eq (3) by [44] as follows:
L=∂∂t+n∑i=1fi(x,t)∂∂xi+12n∑i,j=1[gT(x,t)g(x,t)]ij∂2∂xi∂xj. |
Let V(x,t)∈C2,1(Rn×R,R), then
LV=Vt+Vxf(x,t)+12trace[gT(x,t)Vxxg(x,t)], |
where
Vt=∂V∂t,Vx=(∂V∂x1,⋯,∂V∂xn),Vxx=(∂2∂xi∂xj)n×n. |
By Itˆo's formula, if x(t)∈Rn, then
dV=LV(x(t),t)dt+Vx(x(t),t)g(x(t),t)dB(t). |
Next, we introduce the criterion of positive recurrent and the ergodic properties. Before it, we consider the stochastic equation:
dx(t)=f(x(t))dt+k∑r=1σr(x)dB(t), | (4) |
where x(t) is a homogeneous Markov process in n-dimentional Euclidean space Rn. The diffusion matrix is D(x)=(dij(x)) and (dij)=∑kr=1σir(x)σjr(x). Thus, a lemma which describes the criterion of stationary distribution can be given.
Lemma 2.1 (see [45]) Suppose that there exists a bounded open set E⊂Rn with a smooth regular boundary Θ satisfying the following conditions:
(i) the diffusion matrix D(x) is strictly positive definite for all x∈E;
(ii) there exists a non-negative C2-function V(x) and a positive constant M such that LV≤−M for ∀x∈Rn/E.
Then there exists a solution x(t) of the system (4) which is a stationary Markov process with a stationary distribution μ(⋅) and for any integrable function g(⋅) with respect the measure μ, we have
P(limt→∞1t∫t0g(x(t))dt=∫Rng(x)μ(dx))=1. |
Before investigating the stochastic dynamics of system (2), we should first guarantee whether the solution is global and positive. Therefore, based on the biological interpretation, in this subsection, we just take the non-negative solutions into account for system (2) and discuss the existence of global positive solutions in system (2). The following result can be presented.
Theorem 2.2 For any given initial value (P1(0),P2(0),Z(0))∈R3+, system (2) exists a unique solution (P1(t),P2(t),Z(t)) on R+ and the positive solution will remain in R3+ with probability one, that is, (P1(t),P2(t),Z(t))∈R3+ for all t>0 almost surely.
Proof. From the method of the Lemma 2.1 in [46], it is obvious to find that, for any given initial value (P1(0),P2(0),Z(0))∈R3+, all coefficients of system (2) are locally Lipschitz continuous and the system admits a unique local solution (P1(t),P2(t),Z(t)) on [0,τe), where τe represents the explosion time. In the following, thus, we need to illustrate the solution is global, that is, we only need to prove τe=∞ a.s. Let n0≥1 enough large such that (P1(0),P2(0),Z(0))∈[1n0,n0], and for each integer n≥n0, we define the stopping time by the following form:
τn=inf{t∈[0,τe):max{(P1(t),P2(t),Z(t))}≤1normin{(P1(t),P2(t),Z(t))}≥n}, |
and the set inf∅=∞ (∅ denotes the empty set). Obviously, one can obtain that τn is increasing as n→∞. Thus, let τ∞=limt→+∞τn, then τ∞≤τe a.s. If we can show that τ∞=∞ a.s., then τe=∞ and (P1(0),P2(0),Z(0))∈R3+ a.s. for all t≥0. In other words, to complete the proof, we only need to proof τ∞=∞ a.s. If the statement is false, then there exist two constants T>0 and ε∈(0,1) such that P{τ∞≤T}>ε. Hence, for all n≥n1, there exists an integer n1≥n0 such that P{τ∞≤T}≥ε.
Define a C2-function ˉV:R+3→R+ by
ˉV(P1,P2,Z)=mα(P1−1−logP1)+nβ(P2−1−logP2)+(Z−1−logZ). |
Obviously, the function ˉV(P1,P2,Z,) is non-negative since the inequality x−1−logx>0 holds for all x>0. Applying Itˆo's formula to ˉV(P1,P2,Z) yields
dˉV(P1,P2,Z)=LˉV(P1,P2,Z)dt+mασ1(P1−1)dB1(t)+nβσ2(P2−1)dB2(t)+σ3(Z−1)dB3(t), |
where LˉV:R3+→R is defined by
LˉV1(P1,P2,Z)=mα(P1−1)[r1(1−P1K1)−a1P2−αZ]+mασ212+nβ(P2−1)[r2(1−P2K2)−a2P1−βZ]+nβσ222+(Z−1)(mP1+nP2−d−γP1a+P1−δP2b+P2)+σ232≤γ+δ−mα(r1−σ212)−nβ(r2−σ222)+(d+σ232)+mα[(r1+r1K1+a2−m)P1−r1K1P21]+nβ[(r2+r2K2+a1−n)P2−r2K2P22]+|m+n−d|Z≤M+|m+n−d|Z. |
where
M=γ+δ−mα(r1−σ212)−nβ(r2−σ222)+(d+σ232)+maxP1∈(0,+∞){mα[(r1+r1K1+a2−m)P1−r1K1P21]}+maxP2∈(0,+∞){nβ[(r2+r2K2+a1−n)P2−r2K2P22]}. |
Notice that
Z≤2(Z−1−lnZ)+2ln2≤2V(P1,P2,Z)+2ln2 |
for all Z>0, then one can obtain that
LV≤M+2∣m+n−d∣ln2+2∣m+n−d∣V≤Υ(1+V), |
where
Υ=max{M+2∣m+n−d∣ln2,2∣m+n−d∣}. |
The remainder of the proof follows that in the Theorem 3.3 [37], here, we omit it. This completes the proof.
Based on Theorem 2.2, we will discuss the extinction and persistence in the mean of system (2) and derive sufficient conditions for them in this subsection.
Theorem 2.3 Suppose that (P1(t),P2(t),Z(t)) is the solution of system (2) with initial value P1(0),P2(0),Z(0))∈R3+, then we have the following results:
I Let A=r1−σ212,θ1=A−a1K2r2B−αD, the species P1(t) is
(i) extinct if A<0;
(ii) persistence in the mean if θ1>0.
II Let B=r2−σ222,θ2=B−a2K1r1A−βD, the species P2(t) is
(i) extinct if B<0;
(ii) persistence in the mean if θ2>0.
III Let C=d+σ232,D=mK1r1A+nK2r2B−C, the species Z(t) is
(i) extinct if D<0;
(ii) persistence in the mean if
Γ=K1r1(m−na2K2r2)A+K2r2(n−ma1K1r1)B−C−γ−δ>0. |
Proof. I. Applying the Itˆo's formula to system (2) yields
dlnP1(t)=[r1(1−P1(t)K1)−a1P2(t)−αZ(t)−12σ21]+σ1dB1(t),dlnP2(t)=[r2(1−P2(t)K2)−a2P1(t)−βZ(t)−12σ22]+σ2dB2(t), |
and
dlnZ(t)=(mP1(t)+nP2(t)−d−γP1(t)a+P1(t)−δP2(t)b+P2(t)−12σ23)+σ3dB3(t). |
Integrating the above from 0 to t and dividing t on both sides, we have
1tlnP1(t)lnP1(0)=A−r1K1⟨P1(t)⟩−a1⟨P2(t)⟩−α⟨Z(t)⟩+M1(t)t, | (5) |
1tlnP2(t)lnP2(0)=B−r2K2⟨P2(t)⟩−a2⟨P1(t)⟩−β⟨Z(t)⟩+M2(t)t, | (6) |
and
1tlnZ(t)lnZ(0)=−C+m⟨P1(t)⟩+n⟨P2(t)⟩−⟨γP1(t)a+P1(t)⟩−⟨δP2(t)b+P2(t)⟩+M3(t)t. | (7) |
where
Mi(t)=∫t0σiPi(s)dBi(s),i=1,2,M3(t)=∫t0σ3Z(s)dB3(s). |
Moreover, the quadratic variation of Mi(t)(i=1,2,3) satisfy
⟨Mi(t),Mi(t)⟩t=∫t0σ2ids≤(σ∗i)2t,i=1,2,3. |
By the strong law of large numbers for martingales [45] yields
limt→+∞Mi(t)t=0a.s.i=1,2,3. | (8) |
Thus, according to (5), we have
lim supt→∞lnP1(t)t≤A<0a.s. |
which implies that limt→∞P1(t)=0a.s. This completes the proof of (i).
Next, we give the prove of (ii). By making some estimations of (5), we have
1tlnP1(t)lnP1(0)≥A−r1K11t∫t0P1(s)ds−a1K2r2B−αD+M1(t)t. | (9) |
where B and D will be determined later. In addition, since the fact that
limt→+∞lnP1(0)t=limt→+∞M1(t)t=0. |
Thus, from the properties of the limit, for arbitrary ϵ1, there exists a constant T1>0 such that
⟨P2(t)⟩≤K2r2B+ϵ12a1,⟨Z(t)⟩≤D+ϵ12α,lnP1(0)t≥−ϵ12,M1(t)t≥−ϵ12. |
Substituting above inequalities into (9) and for all t≥T1, we have
1tlnP1(t)≥θ1t−r1K11t∫t0P1(s)ds+M1(t)t, |
where θ1=A−a1K2r2−αD. From the Lemma 4 in [47], we have
lim inft→∞1t∫t0P1(s)ds≥K1r1θ1>0. |
This completes the proof of (ii).
II. The case II are similar to the case I, here we omit it.
III. From (I), (II), one can obtain that
lim supt→∞⟨P1(t)⟩≤K1r1A,lim supt→∞⟨P2(t)⟩≤K2r2B. | (10) |
According to Eq (7), we have
1tlnZ(t)lnZ(0)≤−C+m⟨P1(t)⟩+n⟨P2(t)⟩+M3(t)t. | (11) |
Combining with (8) and (10), and taking upper limit on both sides of (11) yields
lim supt→∞lnZ(t)t≤mK1r1A+nK2r2B−C=D<0a.s. |
which implies limt→∞Z(0)=0a.s. This completes the proof of (i).
Now, we show the proof of (ii). Computing (5)×mK1r1+(6)×nK2r2+(7), we have
mK1r11tlnP1(t)P1(0)+nK2r21tlnP2(t)P2(0)+1tlnZ(t)Z(0)≥K1r1(m−na2K2r2)A+K2r2(n−ma1K1r1)B−C+γ+δ−(mαK1r1+nβK2r2)∫t0Z(s)ds+mK1r1M1(t)t+nK2r2M2(t)t+M3(t)t. | (12) |
By the strong law of large numbers for martingales, we can derive that
limt→∞mK1r1M1(t)t=0,limt→∞nK2r2M2(t)t=0,limt→∞M3(t)t=0a.s. |
From the Lemma 2.3 in [48], we can obtain that
limt→∞mK1r11tlnP1(t)P1(0)≥0,limt→∞nK2r21tlnP2(t)P2(0)≥0,limt→∞1tlnZ(t)Z(0)≥0. |
Thus, taking the limit superior in (12) and from the lemma 4 in [49], one can see that
liminft→∞Z(t)≥(mαK1r1+nβK2r2)−1[K1r1(m−na2K2r2)A+K2r2(n−ma1K1r1)B−C−γ−δ]>0. |
which implies
liminft→∞Z(t)>0. |
This completes the proof of (ii).
In this subsection, by constructing a suitable Lyapunov function, we discuss the positive recurrence of system (2) and some sufficient conditions for the existence and uniqueness of stationary distribution are obtained.
Theorem 2.4 If the following condition
Π=mK1r1A+nK2r2B−C−γ−δ>0 |
holds. Then for any given initial value (P1(0),P2(0),Z(0))∈R3+, the solution (P1(t),P2(t),Z(t)) of system (2) admits a unique ergodic stationary distribution.
Proof. In order to prove this theorem, we only need to prove both two conditions of Lemma 2.1 one by one. Obviously, we can obtain that the diffusion matrix dij(x,K)=diag{σ21,σ22,σ23} of system (2) is positive definite, which implies that the condition (i) in Lemma 2.1 holds.
Next, we prove the condition (ii) in Lemma 2.1. We construct a C2-function ˜V:R3+→R as follows:
˜V(P1,P2,Z)=1p+1(mαP1+nβP2+Z)p+1−M(mK1r1lnP1−nK2r2lnP2−lnZ)−Z |
where p is a positive constant satisfying 0<p<d3p2[σ21∨σ22∨σ23] and M>0 will be determined later. Since the function ˜V(P1,P2,Z) is continuous, then there exists a unique point (~P1,~P2,˜Z) in R3+ which is the minimum value of ˜V(P1,P2,Z). Thus, construct a non-negative C2-function V:R3+→R by
V(P1,P2,Z)=˜V(P1,P2,Z)−˜V(~P1,~P2,˜Z)=1p+1(mαP1+nβP2+Z)p+1−M(mK1r1lnP1−nK2r2lnP2−lnZ)−Z−˜V(~P1,~P2,˜Z)=V1(P1,P2,Z)+V2(P1,P2,Z)+V3(P1,P2,Z). |
Using the generalized Itˆo's formula, one can see that
LV1(P1,P2,Z)=(mαP1+nβP2+Z)p[mr1αP1−mr1αK1P21−ma1αP1P2+nr2βP2−nr2βK2P22−na2βP1P2−dZ−γP1Za+P1−δP2Za+P2]+p2(mαP1+nβP2+Z)p−1[σ21(mαP1)2+σ22(nβP2)2+σ23Z2]≤mr1αP1(mαP1+nβP2+Z)p−m2r1α2K1Pp+21+nr2βP2(mαP1+nβP2+Z)p−n2r2β2K2Pp+22−dZp+1+p2(mαP1+nβP2+Z)p+1(σ21∨σ22∨σ23). |
Noting that the inequality |∑ni=1ci|n≤kn−1∑ni=1|ci|n, we can obtain that
LV1(P1,P2,Z)≤−m2r12α2K1Pp+21−n2r22β2K2Pp+22−d2Zp+1−d4Zp+1+3pp2(Pp+11+Pp+12+Zp+1)[σ21∨σ22∨σ23]−m2r12α2K1Pp+21−n2r22β2K2Pp+22−d4Zp+1+mr1αP1(mαP1+nβP2+Z)p+nr2βP2(mαP1+nβP2+Z)p=−m2r12α2K1Pp+21−n2r22β2K2Pp+22−d2Zp+1−(d4−3pp2(σ21∨σ22∨σ23))Zp+1+H≤−m2r12α2K1Pp+21−n2r22β2K2Pp+22−d2Zp+1+H, |
where
H=sup(P1,P2,Z)∈R3+{−m2r12α2K1Pp+21−n2r22β2K2Pp+22−d4Zp+1+mr1αP1(mαP1+nβP2+Z)p+nr2βP2(mαP1+nβP2+Z)p+3pp2(Pp+11+Pp+12)(σ21∨σ22∨σ23)}<∞. |
and
LV2(P1,P2,Z)=−mK1r1lnP1−nK2r2lnP2−lnZ=−(mK1r1A+nK2r2B−C−γ−δ)+a2nK2r2P1+a1mK1r1P2+(αmK1r1+βnK2r2)Z=−Π+a2nK2r2P1+a1mK1r1P2+(αmK1r1+βnK2r2)Z,LV3(P1,P2,Z)=−mP1Z−nP2Z+dZ+γP1Za+P1+δP2Zb+P1≤γaP1Z+δbP2Z+dZ. |
Thus,
LV(P1,P2,Z)≤−ΠM+na2K2Mr2P1+ma1K1Mr1P2+γaP1Z+δbP2Z+[(ma1K1r1+na2K2r2)M+d]Z−m2r12α2K1Pp+21−n2r22β2K2Pp+22−d2Zp+1+H. |
Considering the following compact subset E:
E={(P1,P2,Z)∈R3+:ε<P1<1ε,ε<P2<1ε,ε<Z<1ε}. |
where ε is a sufficiently small constant satisfying the following conditions:
−ΠM+(na2K2Mr2+γa)ε+S1≤−1, | (13) |
−ΠM+S2−m2r14α2K1ε−p−2≤−1, | (14) |
−ΠM+(ma1K1Mr1+δb)ε+S3≤−1, | (15) |
−ΠM+S4−n2r24β2K2ε−p−2≤−1, | (16) |
−ΠM+[(mαK1r1+nβK2r2)M+d+γ+δ]ε+S5≤−1, | (17) |
−ΠM+S6−d4ε−p−1≤−1, | (18) |
where
S1=sup(P1,P2,Z)∈R3+{ma1K1Mr1P2+δ2bP22+[(ma1K1r1+na2K2r2)M+d]Z+(γεa+δ2b)Z2+H},S2=sup(P1,P2,Z)∈R3+{na2K2Mr2P1+α2aP21+ma1K1Mr1P2+δ2bP22+[(ma1K1r1+na2K2r2)M+d]Z+(γ2a+δ2b)Z2−m2r14α2K1Pp+21−n2r22β2K2Pp+22−d2Zp+1+H},S3=sup(P1,P2,Z)∈R3+{na2K2Mr2P1+γ2aP21+[(ma1K1r1+na2K2r2)M+d]Z+(γ2a+δεb)Z2+H},S4=sup(P1,P2,Z)∈R3+{na2K2Mr2P1+α2aP21+ma1K1Mr1P2+δ2bP22+[(ma1K1r1+na2K2r2)M+d]Z+(γ2a+δ2b)Z2−m2r12α2K1Pp+21−n2r24β2K2Pp+22−d2Zp+1+H},S5=sup(P1,P2,Z)∈R3+{na2K2Mr2P1+γεaP21+ma1K1Mr1P2+δεbP22+H},S6=sup(P1,P2,Z)∈R3+{na2K2Mr2P1+α2aP21+ma1K1Mr1P2+δ2bP22+[(ma1K1r1+na2K2r2)M+d]Z+(γ2a+δ2b)Z2−m2r12α2K1Pp+21−n2r22β2K2Pp+22−d4Zp+1+H}. |
Then
R3+/E=E1∪E2∪E3∪E4∪E5∪E6, |
where
E1={(P1,P2,Z)∈R3+:0<P1<ε},E2={(P1,P2,Z)∈R3+:P1≥1ε}, |
E3={(P1,P2,Z)∈R3+:0<P2<ε},E4={(P1,P2,Z)∈R3+:P2≥1ε}, |
E5={(P1,P2,Z)∈R3+:0<Z<ε},E6={(P1,P2,Z)∈R3+:Z≥1ε}. |
Now, we need to prove the negativity of LV(P1,P2,Z) for any (P1,P2,Z)∈R3+/E.
Case I: If (P1,P2,Z)∈E1, then P1Z≤εZ≤ε(1+Z2), and one can obtain that
LV(P1,P2,Z)≤−ΠM+na2K2Mr2P1+ma1K1Mr1P2+γaP1Z+δbP2Z+[(ma1K1r1+na2K2r2)M+d]Z−m2r12α2K1Pp+21−n2r22β2K2Pp+22−d2Zp+1+H≤−ΠM+na2K2Mr2ε+ma1K1Mr1P2+γaε(1+Z2)+δbP22+Z22+[(ma1K1r1+na2K2r2)M+d]Z−m2r12α2K1Pp+21−n2r22β2K2Pp+22−d2Zp+1+H≤−ΠM+(na2K2Mr2+γa)ε+S1≤−1. |
By (13), we have LV(P1,P2,Z)≤−1 for any (P1,P2,Z)∈E1.
Case II: If (P1,P2,Z)∈E2, we have
LV(P1,P2,Z)≤−ΠM+na2K2Mr2P1+ma1K1Mr1P2+γaP1Z+δbP2Z+[(ma1K1r1+na2K2r2)M+d]Z−m2r12α2K1Pp+21−n2r22β2K2Pp+22−d2Zp+1+H≤−ΠM+na2K2Mr2P1+ma1K1Mr1P2+γaP22+Z22+δbP22+Z22+[(ma1K1r1+na2K2r2)M+d]Z−m2r14α2K1Pp+21−m2r14α2K1Pp+21−n2r22β2K2Pp+22−d2Zp+1+H≤−ΠM+S2−m2r14α2K1ε−p−2≤−1. |
By (14), we have LV(P1,P2,Z)≤−1 for any (P1,P2,Z)∈E2.
Case III: If (P1,P2,Z)∈E3, the similar analysis to case I, we can obtain that
LV(P1,P2,Z)≤−ΠM+(ma1K1Mr1+δb)ε+S3≤−1. |
By (15), we have LV(P1,P2,Z)≤−1 for any (P1,P2,Z)∈E3.
Case IV: If (P1,P2,Z)∈E4, the similar analysis to case II, we have
LV(P1,P2,Z)≤−ΠM+S4−n2r14β2K1ε−p−2≤−1. |
which follows from (16), then we have LV(P1,P2,Z)≤−1 for any (P1,P2,Z)∈E4.
Case V: If (P1,P2,Z)∈E5, then P1Z≤εP1≤ε(1+P21),P2Z≤εP2≤ε(1+P22), we have
LV(P1,P2,Z)≤−ΠM+na2K2Mr2P1+ma1K1Mr1P2+γaP1Z+δbP2Z+[(ma1K1r1+na2K2r2)M+d]Z−m2r12α2K1Pp+21−n2r22β2K2Pp+22−d2Zp+1+H≤−ΠM+na2K2Mr2P1+ma1K1Mr1P2+γaε(1+P21)+δbε(1+P22)+[(ma1K1r1+na2K2r2)M+d]ε−m2r12α2K1Pp+21−n2r22β2K2Pp+22−d2Zp+1+H≤−ΠM+[(mαK1r1+nβK2r2)M+d+γ+δ]ε+S5≤−1. |
According to (17), we have LV(P1,P2,Z)≤−1 for any (P1,P2,Z)∈E5.
Case VI: If (P1,P2,Z)∈E6, we have
LV(P1,P2,Z)≤−ΠM+na2K2Mr2P1+ma1K1Mr1P2+γaP1Z+δbP2Z+[(ma1K1r1+na2K2r2)M+d]Z−m2r12α2K1Pp+21−n2r22β2K2Pp+22−d2Zp+1+H≤−ΠM+na2K2Mr2P1+ma1K1Mr1P2+γaε(1+P21)+δbε(1+P22)+[(ma1K1r1+na2K2r2)M+d]Z−m2r12α2K1Pp+21−n2r22β2K2Pp+22−d4Zp+1+H≤−ΠM+S6−d4Z−p−1≤−1. |
From (18), we have LV(P1,P2,Z)≤−1 for any (P1,P2,Z)∈E6.
Hence, the condition (ii) of Lemma 2.1 is verified. Thus, system (2) admits a unique ergodic stationary distribution. This completes the proof.
In this section, based on the Milstein's Higher Order Method mentioned in [50], some numerical simulations are carried out to further study the effects of the environmental noise and toxin rate released by TPP on the dynamics of system (2). Due to in a phytoplankton-zooplankton system that takes toxic phytoplankton into consideration, the intrinsic growth rates of two toxic phytoplankton and the death rate of zooplankton are parameters that are most susceptible to environmental influences and are relatively important. Therefore, we only consider the intrinsic growth rates and death rate affected by white noise. In the following numerical simulations, unless otherwise specified, the following parameter values are always used: r1=0.55, r2=0.5, a1=0.005, a2=0.004, α=0.15, β=0.15, m=0.09, n=0.075, d=0.09, a=0.1, b=0.12, K1=2, K2=5, the initial value (P1(0),P2(0),Z(0))=(0.5,0.5,0.5), and other parameters are chosen as control parameters. In order to study how the white noise and two TPP affect the dynamics of system (2), we firstly consider system (2) does not experience the white noise, that is, system (2) becomes its corresponding deterministic system. According to [25], we can obtain that the system (1) possesses a unique positive interior equilibrium E∗(0.8178,1.8066,2.1071) which is locally asymptotically stable, depicting the coexistence of all three species.
Next, the impact of white noise on the stochastic dynamics of system (2) will be shown. We first fix σ1=0.1 and σ3=0.1, and let σ2(0≤σ2≤1.2) vary to see how the white noise influences the survival of plankton populations. According to the Theorem 2.3, we can obtain that all three species of system (2) will undergo extinction when the white noise reaches some a critical value. Obviously, we can find from Figure 1(a) that the species P2(t) of system (2) is always persistence in the mean in the area of I and persistence in the mean or extinct alternating in the area of II, but species P2(t) becomes die out rapidly in the space III when σ2 is beyond σ2=1. Figure 1(b) depicts that the stochastic dynamical behaviors of species P2(t) with respect to Figure 1 (a) for σ2=0.1,σ2=0.8, σ2=1.1 and t on [1000,2000], respectively. Moreover, we can observe from Figure 1(b) that, with the increase in the magnitude of the environmental fluctuations, the random variation of plankton density becomes more significant, which implies that white noise can accelerate the stochastic oscillation of plankton density. For example, let σ2=0.1, it is not difficult to find that the two TPP and zooplankton of system (2) can coexist at a relatively stable state and their densities exhibit oscillation around the deterministic steady state values P∗1=0.8178, P∗2=1.8066 and Z∗=2.1071, respectively (see Figure 2(a)-(c)). Actually, following the Theorem 2.3 and Theorem 2.4, system (2) is persistence in the mean and has a unique ergodic stationary distribution, which are consistent with our numerical analysis. From the stationary distribution of all three species, it can be seen clearly that they are distributed normally around the values 0.8, 1.8 and 2.1, respectively, which illustrates that the standard deviation σ1,σ2 and σ3 can keep processes P1(t), P2(t) and Z(t) moving around the solution of system (1). In other words, system (2) can preserve some stability in the random sense when the intensities of white noise are relatively weak. Now let σ2 vary within some a level, it can be concluded that the weaker the environmental fluctuations are, the closer the solutions of system (2) are to steady state E∗ (The Figures here are not given due to the similarity to Figure 2). However, when we increase the density of environmental forcing to σ2>1, for example σ2=1.1, we can easily get from Theorem 2.3 that B=−0.105<0, which implies that species P2(t) of system (2) tends to go rapid extinct, even if its corresponding deterministic system (1) still presents obvious stability, indicating a different phenomenon from its deterministic system (see Figure 3). This also shows that white noise intensity can help to control the density of toxic phytoplankton. Comparing Figures 1, 2 and 3, it is obvious to find that the intensity of white noise can not only aggravate the stochastic oscillation of plankton density, but also significantly change the dynamics of the plankton system. That is, a high white noise intensity can accelerate the extinction of the plankton populations, which implies that the white noise can help control the biomass of plankton populations and provide a guide for us to the termination of planktonic blooms. This is consistent with the results obtained by the work of Sarkar and Chattopadhayay [34], who demonstrated the controlling of plankonic blooms by artifical eutrophication or the intensity of white noise from their experimental and field observations. Thus, it is worth pointing out that the results from the Figures 1, 2 and 3 can support that the plankton systems incorporating white noise can better simulate planktonic blooms than its corresponding deterministic counterparts. Similarly, if we impose the intensities of white noise on species P1(t) and Z(t), respectively, we can easily obtain the similar results, thus here we omit it.
On the one hand, in order to study how the effect of one toxin liberation rate on population density dynamic evolution trend under the environmental fluctuations, we choose γ as a control parameter and all other parameters are the same as Figure 2. Clearly, we can observe from Figure 4 that the species P1(t) and P2(t) are persistence in the mean and their biomass will increase as the increasing value of γ, while species Z(t) undergoes extinction when γ beyond a certain value, here the colorbars denote the biomass of species P1(t), P2(t) and Z(t), respectively. Actually, it is easy to obtain that Π>0 under the condition of 0≤γ<0.3846 and Π=0 if and only if γ≈0.3846, which indicates that system (2) is persistence in the mean and exists the stationary distribution under the condition of γ<0.3846. In contrast, species Z(t) of system (2) will die out. Therefore, it can be asserted that TPP can significantly affect the coexistence of all the three species. For precisely, we take three different values of γ(γ=0.1,0.2,0.35), then system (2) has a unique stationary distribution. Figure 5 depicts the relative frequency density of P1(t),P2(t) and Z(t) with these different values, respectively, where the smoothed curves are the probability density functions of system (2). More importantly, we can obtain the result from the Figure 5 that with the increasing value of γ, the distributions of two TPP appear closer to the normal distribution, but the distribution of zooplankton becomes more skewness, which implies that the increase of the liberation rate γ can increase the survival chance of two harmful phytoplankton but decrease the biomass of zooplankton. Additionally, it can be seen from Figure 5 that the peak value of the probability density functions of system (2) will be higher as γ increases. All these results indicate that for the value of the toxin liberation rate γ satisfying the condition of Theorem 2.4, its enhancement will contribute to the persistence in the mean of system (2) though the termination of planktonic blooms. In addition, from the Theorems 2.3 and 2.4, we want to know what happens if Π<0? Selecting γ=0.39, we can easily check Π≈−0.0054<0 and Γ≈−0.0194<0, which imply that the conditions of the Theorems 2.3 and 2.4 are not satisfied, but system (2) has a stationary distribution, indicating all the three species are persistence in the mean (see Figure 6). However, when we choose γ=0.42, similarly, we can obtain that Π≈−0.0354<0 and Γ≈−0.0494<0. Obviously, the two toxic phytoplankton can coexist while the zooplankton tends to go extinct (see Figure 7). For the case of γ=0.06 and changing the value of δ, we can easily get the similar results, which are omitted here.
Finally, the combined effects of two toxin liberation rates on the dynamics of system (2) are studied as well. Figure 8 depicts how the combined role of γ and δ affect the dynamics of system (2), where the red smoothed curves are probability density functions of system (2). By a straightforward computation, the condition of Theorem 2.4 can be verified, which means system (2) has a unique stationary distribution (see Figure 8). Comparing Figure 5 and Figure 8, one can see that the mean values of two harmful phytoplankton are larger than the case of γ=0.2,δ=0.07, while that of zooplankton is smaller than that case, indicating the high abundance of toxic phytoplankton because of the low level or the absence of other toxic phytoplankton, while the peaks of zooplankton population come down. Thus, we can obtain that, by controlling two harmful phytoplankton, the mean values of both the harmful phytoplankton are larger than the value observed when considering in the case of any one of toxic phytoplankton, while that of zooplankton is smaller than the case of any one of toxic phytoplankton is present, which imply that a considerable decrease in the biomass accumulations of plankton as compared with the case of in the presence of a single toxic phytoplankton. Therefore, the introduction of two harmful phytoplankton is contribute to the persistence of system (2) and plays an important role in the termination of planktonic blooms.
It is now well recognized that stochastic population dynamics play a significant role in population dynamics, since environmental fluctuations can affect the growth process of species, such as the growth rate and death rate, which can be described by white noise [51]. And the Gaussian white noise can been theoretically preferred to model environmental fluctuations because of its irregularity and thus a good approximation to the phenomena of rapid fluctuations [52]. The study of stochastic population dynamics goes back to the pioneering work by Haminskii [53], who introduced two white noise to stabilize a linear system. After that, lots of attention has been paid on stochastic population dynamics studies [54,55,56]. Mao et al. [54] pointed out that stochastic noise can suppress potential explosion in population dynamics. Wang et al. [55] showed that time-periodic forcing can lead to the transitions from a spatially homogeneous stationary state to a periodic oscillation in time. Additionally, lots of stochastic plankton growth systems have been derived by numerous researchers [40,42,57], and stochastic plankton systems involve toxin-producing phytoplankton have become a hot topic in ecological studies due to harmful phytoplankton can significantly affect the dynamics of plankton systems [18,19,20,21,22,23,24,25,34,41].
In this paper, therefore, we first propose a stochastic phytoplankton-zooplankton system with two harmful phytoplankton populations, where the intrinsic growth rates of two harmful phytoplankton and the natural death rate of zooplankton are influenced by the environmental noise, and we then study the effects of TPP and white noise on the dynamics of system (2) theoretically and numerically. In order to ensure that the system is biologically meaningful, the existence and uniqueness of global positive solutions of system is discussed, and the results demonstrate that for any initial value (P1(0),P2(0),Z(0))∈R3+, the solution will remain in R3+ with probability one. Based on this situation, we derive some sufficient conditions for the extinction and persistence in the mean of the system. Obviously, those conditions are great significance to study the extinction and persistence in the mean for the phytoplankton-zooplankton system [30,34]. Significantly, when the system is persistence in the mean, we also investigate the existence and uniqueness of positive recurrent of solution for the system, which implies the system has a unique stationary distribution under some conditions. Numerical analysis illustrates our theoretical results and further indicates that both two TPP and environmental fluctuations have a significant effect on the controlling of planktonic blooms.
On the one hand, from Theorems 2.3 and 2.4, which follows that, when the low level intensity of white noise satisfies the conditions θ1>0,θ2>0, and Π>0, system (2) is persistence in the mean and exists a ergodic stationary distribution, indicating the coexistence of all those three species in the random sense for a long time (see Figure 2). However, when we increase the intensity of environmental forcing such that the condition of θ2<0 holds, the species P2(t) undergoes rapid extinction while other two species are persistence in the mean, as it is shown in Figure 3. Comparing Figures 2 and 3, it can be asserted that white noise can aggravate the stochastic oscillation of plankton density and significantly change the dynamics of phytoplankton-zooplankton system. Especially, a high intensity of white noise can accelerate the extinction of plankton populations. Consequently, these results may be more realistic than that of in [25], which implies that the controlling of rapid environmental fluctuations may be a good way in the termination of planktonic blooms. Therefore, it is great ecological significance to consider environmental noise when studying phytoplankton-zooplankton interaction in the presence of harmful phytoplankton.
On the other hand, it is investigated how the dynamics of system (2) strongly depends on TPP. By controlling one toxin liberation rate, the dynamic behaviours of system (2) can be changed. That is, when the toxin liberation rate is beyond some a critical value, two harmful phytoplankton can coexist, while zooplankton tends to extinction (see Figure 4). Moreover, when controlling any one of the two TPP, it is obvious to survey from Figure 5 that the increasing value of one toxin liberation rate can reduce the biomass of zooplankton, while increase the survival chance of two phytoplankton. In addition, in the presence of both two TPP, it can be seen from Figures 5 and 8 that the combined effects of two liberation rates on the changes in plankton populations are stronger than that of controlling any one of the two TPP. Thus, the introduction of two harmful phytoplankton is conducive to the persistence of the system (2) through the termination of planktonic blooms. Therefore, TPP has a profound impact on the dynamics of phytoplankton-zooplankton systems and may be used as a biological way to control planktonic blooms.
There are some interesting topics waiting for us to further explore. For example, the zooplankton mortality will occur after some time lapse due to the bloom of toxic phytoplankton [58], it seems to more reasonable to study a stochastic toxic-producing phytoplankton-zooplankton system with time delay. Another problem of interest is to consider impulsive perturbations or regime switching into the system. We leave those for our future research goals.
This work was supported by the National Natural Science Foundation of China (Grant No.61871293), the National Key Research and Development Program of China (Grant No. 2018YFE0103700) and the National Natural Science Foundation of China (Grant No. 61901303).
The author declares that there are no conflicts of interest.
[1] |
Rumanti IA, Hairmansis A, Nugraha Y, et al. (2018) Development of tolerant rice varieties for stress-prone ecosystems in the coastal deltas of Indonesia. Field Crops Res 223: 75–82. https://doi.org/10.1016/j.fcr.2018.04.006 doi: 10.1016/j.fcr.2018.04.006
![]() |
[2] | Rhaman MS, Tahjib-Ul-Arif, Kibria MG, et al. (2021) Climate change and its adverse impacts on plant growth in South Asia: Current status and upcoming challenges. Phyton Int J Exp Bot 91: 695–711. |
[3] |
Martínez ML, Intralawan A, Vázquez G, et al. (2007) The costs of our world: Ecological, economic and social importance. Field Crops Res 63: 254–272. https://doi.org/10.1016/j.ecolecon.2006.10.022 doi: 10.1016/j.ecolecon.2006.10.022
![]() |
[4] | Sopandie D (2014) Fisiologi Adaptasi Tanaman terhadap Cekaman Abiotik pada Agroekosistem Tropika. IPB Press, Bogor, Indonesia, 69–74 (In Indonesian). |
[5] |
İbrahimova U, Kumari P, Yadav S, et al. (2021) Progress in understanding salt stress response in plants using biotechnological tools. J Biotechnol 329:180–191. https://doi.org/10.1016/j.jbiotec.2021.02.007 doi: 10.1016/j.jbiotec.2021.02.007
![]() |
[6] |
Tavakkoli E, Fatehi F, Rengasamy P, et al. (2012) A comparison of hydroponic and soil-based screening methods to identify salt tolerance in the field in barley. J Exp Bot 63: 3853–3868. https://doi.org/10.1093/jxb/ers085 doi: 10.1093/jxb/ers085
![]() |
[7] | Rad HE, Aref F, Rezaei M (2012) Response of rice to different salinity levels during different growth stage. Res J Appl Sci Eng Technol 4: 3040–3047. |
[8] | Purwoko BS, Dewi IS, Khumaida N (2010) Rice anther culture to obtain doubled-haploids with multiple tolerances. Asia Pacific J Mol Biol Biotechnol 18: 55–57. |
[9] | Wolko J, Dobrzycka A, Bocianowski J, et al. (2020) Genetic variation of traits affecting meal quality in black × yellow seeded doubled haploid population of winter oilseed rape. Agron Res 18: 2259–2270. |
[10] |
Dewi IS, Purwoko BS (2012) Kultur antera untuk percepatan perakitan varietas padi di Indonesia. Jurnal AgroBiogen 8: 78–88. (In Indonesian). https://doi.org/10.21082/jbio.v8n2.2012.p78-88 doi: 10.21082/jbio.v8n2.2012.p78-88
![]() |
[11] | Hidayatullah A, Purwoko BS, Dewi IS, et al. (2018) Agronomic performance and yield of doubled haploid rice lines in advanced yield trial. SABRAO J Breed Genet 50: 242–253. |
[12] | Ismail AM, Platten JD, Miro B (2013) Physiological bases of tolerance of abiotic stresses in rice and mechanisms of adaptation. Oryza 50: 91–99. |
[13] |
Ghosh B, Ali Md N, Saikat G (2016) Response of rice under salinity stress: A review update. J Res Rice 4: 1–8. https://doi.org/10.4172/2375-4338.1000167 doi: 10.4172/2375-4338.1000167
![]() |
[14] |
Hariadi YC, Nurhayati AY, Soeparjono S, et al. (2014) Screening six varieties of rice (Oryza sativa L.) for salinity tolerance. Proc Environ Sci 28: 78–87. https://doi.org/10.1016/j.proenv.2015.07.012 doi: 10.1016/j.proenv.2015.07.012
![]() |
[15] | Safitri H, Purwoko BS, Dewi IS et al. (2016a) Morpho-physiological response of rice genotypes grown under saline conditions. J Int Soc Southeast Asian Agric Sci 22: 52–63. |
[16] |
Souleymane O, Nartey E, Manneh B, et al. (2016) Phenotypic variability of 20 rice genotypes under salt stress. Int J Plant Breed Genet 10: 45–51. https://doi.org/10.3923/ijpbg.2016.45.51 doi: 10.3923/ijpbg.2016.45.51
![]() |
[17] |
Kopittke PM, Blamey FPC, Kinraide TB, et al. (2011) Separating multiple, short-term, deleterious effects of saline solutions on the growth of cowpea seedlings. New Phytol 189: 1110–1121. https://doi.org/10.1111/j.1469-8137.2010.03551.x doi: 10.1111/j.1469-8137.2010.03551.x
![]() |
[18] |
Mansuri SM, Jelodar NB, Bagheri N (2012) Evaluation of rice genotypes to salt stress in different growth stages via phenotypic and random amplified polymorphic DNA (RAPD) marker assisted selection. Afr J Biotechnol 11: 9362–9372. https://doi.org/10.5897/AJB11.149 doi: 10.5897/AJB11.149
![]() |
[19] |
Akbar MR, Purwoko BS, Dewi IS, et al. (2021) Agronomic and yield selection of doubled haploid lines of rainfed lowland rice in advanced yield trials. Biodiversitas 22: 3006–3012. https://doi.org/10.13057/biodiv/d220754 doi: 10.13057/biodiv/d220754
![]() |
[20] | Anshori MF, Purwoko BS, Dewi IS, et al. (2019) Selection index based on multivariate analysis for selecting doubled-haploid rice lines in lowland saline prone area. SABRAO J Breed Genet 51: 161–174. |
[21] |
Anshori MF, Purwoko BS, Dewi IS, et al. (2021) A new approach to select doubled haploid rice lines under salinity stress using indirect selection index. Rice Sci 28: 368–378. https://doi.org/10.1016/j.rsci.2021.05.007 doi: 10.1016/j.rsci.2021.05.007
![]() |
[22] | Acquaah G (2007) Principles of Plant Genetics and Breeding. Blackwell Publishing, Oxford, United Kingdom, 716–718. |
[23] |
Dormann CF, Elith J, Bacher S, et al. (2013) Collinearity: A review of methods to deal with it and a simulation study evaluating their performance. Ecography 36: 27–46. https://doi.org/10.1111/j.1600-0587.2012.07348.x doi: 10.1111/j.1600-0587.2012.07348.x
![]() |
[24] |
Farid M, Nasaruddin, Musa Y, et al. (2020) Genetic parameters and multivariate analysis to determine secondary traits in selecting wheat mutant adaptive on tropical lowlands. Plant Breed Biotechnol 8: 368–377. https://doi.org/10.9787/PBB.2020.8.4.368 doi: 10.9787/PBB.2020.8.4.368
![]() |
[25] | Mattjik AA, Sumertajaya IM (2011) Multivariate Analysis Using SAS. FMIPA IPB, Bogor, 119–134 (In Indonesian). |
[26] |
Godshalk EB, Timothy EB (1988) Factor and principal component analyses as alternatives to index selection. Theor Appl Genet 76: 352–360. https://doi.org/10.1007/BF00265334 doi: 10.1007/BF00265334
![]() |
[27] |
Rocha JRDADC, Machado JC, Carneiro FCS (2018) Multitraits index based on factor analysis and ideotype-design: Proposal and application on elephant grass breeding for bioenergy. Bioenergy 10: 52–60. https://doi.org/10.1111/gcbb.12443 doi: 10.1111/gcbb.12443
![]() |
[28] | Safitri H, Purwoko BS, Dewi IS, et al. (2016b) Anther culture to obtain rice lines tolerant to salinity. J Agron Indones 44: 221–227. |
[29] | Anshori MF, Purwoko BS, Dewi IS, et al. (2018) Determination of selection criteria for screening of rice genotypes for salinity tolerance. SABRAO J Breed Genet 50: 279–294. |
[30] | Kassahun BM, Alemaw G, Tesfaye B (2013) Correlation studies and path coefficient analysis for seed yield and yield components in Ethiopian coriander accessions. Afr Crop Sci J 21: 51–59. |
[31] | Manjunatha GA, Kumar MS, Jayashree M (2017) Character association and path analysis in rice (Oryza sativa L.) genotypes evaluated under organic management. J Pharmacogn Phytochem 6: 1053–1058. |
[32] | Fernandez GCJ (1992) Effective selection criteria for assessing plant stress tolerance. In: Proceeding of the international symposium on adaptation of vegetables and other food crops in temperature and water stress. Asian Vegetable Research and Development Center, Taiwan, 257–270. |
[33] | Rawlings JO, Pantula SG, Dickey DA (1998) Applied Regression Analysis: a Research Tool, Second Edition. Springer-Verlag New York Inc, New York, United State, 213–220. https://doi.org/10.1007/b98890 |
[34] | Singh RK, Chaundhary B (2007) Biometrical Methods in Quantitative Genetic Analysis. Kalyani Publisher, New Delhi, India, 69–78. |
[35] | Alvarado G, López M, Vargas M, et al. (2015) META-R (multi environment trial analysis with R for windows) version 6.04. Available from: https://hdl.handle.net/11529/10201. |
[36] | Mendiburu F, Yaseen M (2020) Agricolae: Statistical Procedures for Agricultural Research. R package version 1.4.0. Available from: https://myaseen208.github.io/agricolae/https://cran.rproject.org/package=agricolae. |
[37] |
Al-Amin M, Islam MM, Begum SN, et al. (2013) Evaluation of rice germplasm under salt stress at the seedling stage through SSR markers. Int J Agric Res, Innovation Technol 3: 52–59. https://doi.org/10.3329/ijarit.v3i1.16093 doi: 10.3329/ijarit.v3i1.16093
![]() |
[38] |
Al-Naggar AMM, Sabry SRS, Atta MMM, et al. (2015) Effects of salinity on performance, heritability, selection gain and correlations in wheat (Triticum aestivum L.) doubled haploids. Sci Agric 10: 70–83. https://doi.org/10.15192/PSCP.SA.2015.10.2.7083 doi: 10.15192/PSCP.SA.2015.10.2.7083
![]() |
[39] | Horváth É, Gombos B, Széles A (2021) Evaluation phenology, yield and quality of maize genotypes in drought stress and non-stress environments. Agron Res 19: 408–422. |
[40] | Tobi G, Bahloul YE, Oumouss S, et al. (2021) Productivity, heritability and stability analysis of a Moroccan sugar beet germplasm. Agron Res 19: 612–628. |
[41] |
Krishnamurthy SL, Sharma SK, Gautama RK, et al. (2014) Path and association analysis and stress indices for salinity tolerance traits in promising rice (Oryza sativa L.) genotypes. Cereal Res Commun 42: 474–483. https://doi.org/10.1556/CRC.2013.0067 doi: 10.1556/CRC.2013.0067
![]() |
[42] |
Mohammadi R, Mendioro MS, Diaz GQ, et al. (2014) Genetic analysis of salt tolerance at seedling and reproductive stages in rice (Oryza sativa). Plant Breed 133: 548–559. https://doi.org/10.1111/pbr.12210 doi: 10.1111/pbr.12210
![]() |
[43] | Yadav P, Singh P, Harishcandra, et al. (2018) Estimation of genetic variability, heritability and genetic advance of thirty rice (Oryza sativa L.) genotypes in saline and normal condition. Int J Curr Microbiol Appl Sci 7: 1531–1539. |
[44] | Garg HS, Kumar R, Kumar B, et al. (2017) Screening and identification of rice genotypes with drought tolerance under stress and non-stress condition. Int J Chem Stud 5: 1031–1042. |
[45] |
Georgieva N, Nikolova I, Kosev V (2015) Stability analysis for seed yield in vetch cultivars. Emirates J Food Agric 27: 903–910. https://doi.org/10.9755/ejfa.2015-04-172 doi: 10.9755/ejfa.2015-04-172
![]() |
[46] | Manjunatha GA, Kumar MS, Jayashree M (2017) Character association and path analysis in rice (Oryza sativa L.) genotypes evaluated under organic management. J Pharmacogn Phytochem 6: 1053–1058. |
[47] |
Petil MK, Lokesha R (2018) Estimation of genetic variability, heritability, genetic advance, correlations and path analysis in advanced mutant breeding lines of sesame (Sesamum indicum L.). J Pharmacogn Nat Prod 4: 1000151. https://doi.org/10.4172/2472-0992.1000151 doi: 10.4172/2472-0992.1000151
![]() |
[48] | Hosseini SJ, Sarvestani ZT, Pirdashti H (2012) Analysis of tolerance indices in some rice (Oryza sativa L.) genotypes at salt stress condition. Int Res J Appl Basic Sci 3: 1–10. |
[49] |
Singh S, Sengar RS, Kulshreshtha N, et al. (2015) Assessment of multiple tolerance indices for salinity stress in bread wheat (Triticum aesticum L.). J Agric Sci 7: 49–57. https://doi.org/10.5539/jas.v7n3p49 doi: 10.5539/jas.v7n3p49
![]() |
[50] |
Hakam M, Sudarno S, Hoyyi A (2015) Analisis jalur terhadap faktor-faktor yang mempengaruhi indeks prestasi kumulatif (IPK) mahasiswa statistika UNDIP. J Gaussian 4: 61–70. https://doi.org/10.14710/j.gauss.v4i1.8146 doi: 10.14710/j.gauss.v4i1.8146
![]() |
[51] | Wang K, Chen Z (2016) Stepwise regression and all possible subsets regression in education. Elec Int J Eng Educ 2: 60–81. |
[52] |
Fadhli N, Farid M, Rafiuddin, et al. (2020) Multivariate analysis to determine secondary trait in selecting adaptive hybrid corn lines under drought stress. Biodiversitas 21: 3617–3624. https://doi.org/10.13057/biodiv/d210826 doi: 10.13057/biodiv/d210826
![]() |
[53] |
Farid M, Nasaruddin, Musa Y, et al. (2021) Effective screening of tropical wheat mutant lines under hydroponically induced drought stress using multivariate analysis approach. Asian J Plant Sci 20: 172–182. https://doi.org/10.3923/ajps.2021.172.182 doi: 10.3923/ajps.2021.172.182
![]() |
[54] |
Yong AG, Pearce S (2013) A beginner's guide to factor analysis: focusing on exploratory factor analysis. Tutorials Quant Methods Psychol 9: 79–94. https://doi.org/10.20982/tqmp.09.2.p079 doi: 10.20982/tqmp.09.2.p079
![]() |
[55] |
Sabouri H, Rabiei B, Fazlalipour M (2008) Use of selection indices based on multivariate analysis for improving grain yield in rice. Rice Sci 15: 303–310. https://doi.org/10.1016/S1672-6308(09)60008-1 doi: 10.1016/S1672-6308(09)60008-1
![]() |
[56] |
Jahufer MZZ, Casler MD (2015) Application of the Smith-Hazel selection index for improving biomass yield and quality of switchgrass. Crop Sci 55: 1212–1222. https://doi.org/10.2135/cropsci2014.08.0575 doi: 10.2135/cropsci2014.08.0575
![]() |
1. | Jun Kikuchi, Shunji Yamada, The exposome paradigm to predict environmental health in terms of systemic homeostasis and resource balance based on NMR data science, 2021, 11, 2046-2069, 30426, 10.1039/D1RA03008F | |
2. | Sarita Kumari, Satish Kumar Tiwari, Ranjit Kumar Upadhyay, Cross diffusion induced spatiotemporal pattern in diffusive nutrient–plankton model with nutrient recycling, 2022, 202, 03784754, 246, 10.1016/j.matcom.2022.05.027 | |
3. | Anal Chatterjee, Samares Pal, 2022, Chapter 5, 978-3-031-12514-0, 99, 10.1007/978-3-031-12515-7_5 | |
4. | Xin Zhao, Lijun Wang, Pankaj Kumar Tiwari, He Liu, Yi Wang, Jianbing Li, Min Zhao, Chuanjun Dai, Qing Guo, Investigation of a nutrient-plankton model with stochastic fluctuation and impulsive control, 2023, 20, 1551-0018, 15496, 10.3934/mbe.2023692 | |
5. | Xiaojie Mu, Qimin Zhang, Optimal strategy for non-zero cost inhibiting in a stochastic microorganism flocculation model under environmental noise, 2025, 195, 09600779, 116306, 10.1016/j.chaos.2025.116306 |