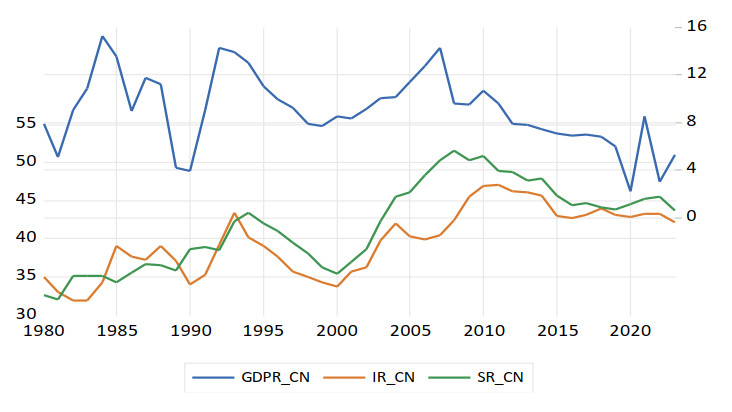
We aimed to compile the flow of funds and stock matrix based on the Who-to-Whom (W-t-W) model. Our objective was to analyze structural changes in China's flow of funds and macroeconomic regulation. To strengthen macroeconomic monitoring, we examined the characteristics of fund sources and uses across sectors from a sectoral perspective of the flow of funds. Utilizing data on fund flows and stocks, we constructed the Chinese flow-of-funds matrix and financial asset-liability matrix for the period 1998–2022. Furthermore, time series methods were introduced to track the flow of funds and conduct influence and sensitivity analysis of assets and liabilities. We also performed a multiplier analysis of the fluctuations in the flow of funds and discuss the position and role of the Financial Corporation and Government sector in the financial market.
Citation: Nan Zhang, Li Zhu. Structural changes in China's flow of funds (1992–2022): A Who-to-Whom model[J]. Quantitative Finance and Economics, 2025, 9(1): 167-201. doi: 10.3934/QFE.2025006
[1] | Md Qamruzzaman, Jianguo Wei . Do financial inclusion, stock market development attract foreign capital flows in developing economy: a panel data investigation. Quantitative Finance and Economics, 2019, 3(1): 88-108. doi: 10.3934/QFE.2019.1.88 |
[2] | Mohammad Ashraful Ferdous Chowdhury, M. Kabir Hassan, Mohammad Abdullah, Md Mofazzal Hossain . Geopolitical risk transmission dynamics to commodity, stock, and energy markets. Quantitative Finance and Economics, 2025, 9(1): 76-99. doi: 10.3934/QFE.2025003 |
[3] | Juan David Vega Baquero, Miguel Santolino . Capital flows in integrated capital markets: MILA case. Quantitative Finance and Economics, 2022, 6(4): 622-639. doi: 10.3934/QFE.2022027 |
[4] | Wenhao Chen, Kinkeung Lai, Yi Cai . Topic generation for Chinese stocks: a cognitively motivated topic modelingmethod using social media data. Quantitative Finance and Economics, 2018, 2(2): 279-293. doi: 10.3934/QFE.2018.2.279 |
[5] | Ngo Thai Hung . Equity market integration of China and Southeast Asian countries: further evidence from MGARCH-ADCC and wavelet coherence analysis. Quantitative Finance and Economics, 2019, 3(2): 201-220. doi: 10.3934/QFE.2019.2.201 |
[6] | Fangzhou Huang, Jiao Song, Nick J. Taylor . The impact of business conditions and commodity market on US stock returns: An asset pricing modelling experiment. Quantitative Finance and Economics, 2022, 6(3): 433-458. doi: 10.3934/QFE.2022019 |
[7] | Julius Nickelsen, Olaf Stotz . Do fund managers in the Chinese mutual fund market deliver positive risk-adjusted returns? Yes, but it is mainly observed for local fund managers. Quantitative Finance and Economics, 2023, 7(4): 595-621. doi: 10.3934/QFE.2023029 |
[8] | Chiao Yi Chang, Fu Shuen Shie, Shu Ling Yang . The relationship between herding behavior and firm size before and after the elimination of short-sale price restrictions. Quantitative Finance and Economics, 2019, 3(3): 526-549. doi: 10.3934/QFE.2019.3.526 |
[9] | Anton Velinov . On the importance of testing structural identification schemes and the potential consequences of incorrectly identified models. Quantitative Finance and Economics, 2018, 2(1): 591-611. doi: 10.3934/QFE.2018.1.106 |
[10] | Rubaiyat Ahsan Bhuiyan, Tanusree Chakravarty Mukherjee, Kazi Md Tarique, Changyong Zhang . Hedge asset for stock markets: Cryptocurrency, Cryptocurrency Volatility Index (CVI) or Commodity. Quantitative Finance and Economics, 2025, 9(1): 131-166. doi: 10.3934/QFE.2025005 |
We aimed to compile the flow of funds and stock matrix based on the Who-to-Whom (W-t-W) model. Our objective was to analyze structural changes in China's flow of funds and macroeconomic regulation. To strengthen macroeconomic monitoring, we examined the characteristics of fund sources and uses across sectors from a sectoral perspective of the flow of funds. Utilizing data on fund flows and stocks, we constructed the Chinese flow-of-funds matrix and financial asset-liability matrix for the period 1998–2022. Furthermore, time series methods were introduced to track the flow of funds and conduct influence and sensitivity analysis of assets and liabilities. We also performed a multiplier analysis of the fluctuations in the flow of funds and discuss the position and role of the Financial Corporation and Government sector in the financial market.
After the 2008 financial crisis, an international consensus at the G20 Finance Ministers and Central Bank Governors meeting in London was to strengthen financial statistics and promote the systematic integrity of financial statistics. In 2009 the International Monetary Fund and the Financial Stability Board (FSB) called for identifying financial crises and information gaps. One of the priorities was to strengthen the flow of funds accounts (FFA) and balance sheet approach and financial soundness1. Moreovoer, the aim was to improve data transfer methods and more detailed sectoral data and produce detailed tables of stock and flow for each institutional sector by the counterparty, that is, from-whom-to-whom (W-t-W) financial statistics. A three-dimensional structure can show counterparty data for each instrument transaction. The European Central Bank (ECB) has specially edited and published two monographs on financial crises from the perspective of the flow of funds (Winkler et al., 2013; de Rougemont and Winkler, 2014). It can be seen that detailed flow of funds data is an important basis for observing macroeconomic operations and monitoring financial risks.
1 Financial Stability Board & International Monetary Fund (2009) The Financial Crisis and Information Gaps.
We utilize flow of funds statistics to advance macroeconomic monitoring. The movement of capital within an economic system is frequently likened to the circulatory system of blood in a living organism. The development of the flow of funds accounts traces back to Copeland's seminal work, A Study of Money Flows in the United States (Copeland, 1952), which inspired central banks globally to adopt and implement systems for flow of funds accounting. The flow of funds accounts became the central account in the SNA in 1968. Its methods and applications have been popularized in many countries. Under the technical guidance of Copeland, the Federal Reserve Board studied and improved the compilation of MFA. In 1955, it published the Flow of Funds Accounts of the United States (1939–1953) for the first time. The change from "Money-flows" to "Flow of Funds" not only reflects the change of technical terms but also reflects the change of statistical connotation and scope. On June 6, 2013, the FRB changed the name of Flows of Funds Accounts to Financial Accounts of the United States - Z.1, a statistical system that inherited the basic framework of the previous Flows of Funds Accounts2. Flows of Funds Accounts include data on transactions and levels of financial assets and liabilities by sector and financial instrument full balance sheets. All institutional units are divided into five major sectors, namely, Financial Corporations (FCs), Non-Financial Corporations (NFCs), General Government (GG), Household and NPISH (HHs), and the Rest of the World (ROW).
2 Zhang (2020), 33.
Stone (1966) and Klein (1983) connected the flow of funds analysis (FFA) with the national income account and the input-output table to develop the financial matrix model, which made a pioneering contribution to the expansion of FFA analysis. In 1996, John C. Dawson3 was the chief editor and published the book Fund Flow Analysis, which discusses the calculation method, theoretical research and analysis method of capital flow from 50's to 90's mid. The major authors included Morris A. Copeland, Stephen P. Taylor, Nancy D. Ruggles, Benjamin M. Friedman, James Tobin, Kenta Marwan, and Lawrence R. Klein were among more than ten economists.
Ishida (1993) called the statistical observation and analysis, including fund flow and stock as FFA. The flow of funds in the real economy, as the exchange of capital and commodities "mutual convection"; Ishida also put forward the theoretical conception of international flow of funds analysis. Tsujiura and Mizoshita (2002) applied the principle of the Leontief inverse matrix of input-output analysis to apply the Power-of-Dispersion Index (PDI) and Sensitivity-of-Dispersion Index (SDI), which show the capital supply and demand into the asset-liability matrix. Tsujimura and Tsujimura (2018, 2020) also took the effect of quantitative easing policy in the United States as the research object and extended the above research results using serial analysis of capital flows.
Zhang (2005, 2020) discussed the relationship between the system of national accounts (SNA) and FFA account system, the preparation and analysis methods of FFA, the statistical methods of macroeconomic monitoring and financial security with FFA as the basic framework, and paid special attention to the expansion and extension of FFA to Global flow of funds (GFF). Zhang explored data sources and the establishment of a statistical matrix of GFF, financial network and measurement model, and other methodological issues.
China began to publish the Flow of Funds Accounts from 1992 in 1998, which was based on the 1993 SNA, and the flow of funds accounts is divided into two parts: non-financial transaction and financial transaction. Among them, the non-financial transaction part is compiled by the National Bureau of Statistics, and the financial transaction part is compiled by the People's Bank of China. In 2021, the People's Bank of China published balance sheet data from 2017 to 2020 for the first time. FFA is a relatively unpopular research field in China, but many Chinese scholars and statistical experts of the People's Bank of China have done meaningful research on China's flow of funds using China's FFA. Li's (1998) study on Flow of Funds in the process of China's opening up, Wen and Ruan's (2006) analysis of financial policies based on reality. Hu (2010) compiled three kinds of input-output flow of funds statements and constructed the corresponding capital correlation model to analyze the flow of funds relationship in the economic system. Gong and Bian (2010) quantified and analyzed the circulatory transmission trajectory of "economic shock" among various sectors of the national economy through the network model based on the data of the matrix table of the sector-to-sector financial financing relationship.
In addition, Li and Zhang (2020) compiled China's sovereign balance sheet (levels of financial assets and liabilities data) in an attempt to assess China's financial risks and published China's National Balance Sheet continuously from 2000 to 2019. The stock data (as opposed to flow data) represents accumulated economic outcomes and plays a crucial role in detecting financial crises and bridging data gaps. Yi (2020) used the flow and stock statistics of FFA to link financial structure, financial development, and economic growth. From the perspective of financial asset structure changes, he explained the internal logic and policy implications of China's macroeconomic operation.
In the study of the evolution of China's economic structure, a more recent perspective can be cited from Bekkers et al. (2021). Using the WTO global trade model, their speculative results indicate that as global trade relations change, China's economy will increasingly reorient its focus towards the domestic economy. China's trade surplus will transform into a trade deficit, reducing its share in global exports. This shift is also bound to bring about changes in China's external flow of funds. Moreover, Shi's (2023) paper indicates that over the past 40 years, despite state-led and market-led reform processes in China's financial system, the Chinese economy has not experienced financialization, and the state continues to play a significant role. The relational structure of China's finance and government control mechanisms has not been replaced by marketization and privatization mechanisms, which is of great reference value for understanding the financial circulation of the Chinese economy. The latest research by the ECB (2024) also suggests that structural changes are occurring in the Chinese economy, with the investment-driven growth model facing increasing pressure. This is mainly manifested in the decline in the return on investment, a significant downturn in the policy-driven real estate sector, shrinking external demand, as well as structural challenges including an aging population and sluggish productivity growth.
Based on the above literature, we believe that there are gaps or deficiencies in the research related to flow of funds. First, there is a gap in FFA data. Researchers have not focused on the W-t-W model proposed by IMF and FSB to reveal the sectoral structure characteristics and changing trends in flow of funds from the perspectives of flow and stock, and then carry out macroeconomic monitoring and financial risk research based on stock and flow. Second, as FFA's sector classification and the composition of transaction items are complex, involving flow and stock, linking national income and balance of payments statistics, it is necessary to understand its data relationship from the perspectives of the economy as a whole and sectors, finance, and real economy, as well as domestic and overseas. Therefore, FFA is a relatively weak part in the field of economic research, so it is necessary to develop a systematic observation and analysis method. Third, the current FFA cannot reveal the transmission of financial risks among sectors from the perspective of sectors on the flow of funds and cannot answer the two key questions of "who bears the risk" and "who bears the risk better" (Yi, 2020) in a country's capital circulation, and cannot decipher how income and wealth inequalities affect the transmission of monetary policy. The risks mentioned here mainly refer to debt risk and liquidity risk.
Therefore, combined with the changes in the international economic environment caused by the US financial crisis in 2008 and COVID-19 in 2020, we attempt to take China as the observation object, compile the capital flow and stock matrix with the W-t-W model proposed by the IMF, and observe the effect and problems of China's flow of funds and macroeconomic regulation from the perspective of sectors. The basic structure of this paper is as follows: In the second part, we give the basic concept of flow of funds, and then, based on the relationship between real economy and finance, statistically describe the changes in China's real economy and flow of funds from 1992 to 2022, as well as the basic characteristics of capital operation by sectors. In the third part, we focus on the analysis of financial flow of funds and develop new data. The flow of funds statement in the form of two-dimensional accounts is deduced into a three-dimensional statistical matrix, and the W-t-W capital flow and stock matrix from 1998 to 2022 are established to meet the needs that is from who to whom with by what for running FFA. The fourth part contains the innovation analysis method. We established a W-t-W statistical matrix based on the perspective of sectors, observed the changes in capital structure from the two aspects of assets and liabilities, and evaluated the role and impact of capital operations research of each sector in the market. We triangulate the W-t-W statistical matrix established from the perspective of departments, observe the changes of the capital structure from the asset end and the liability end, and evaluate the role and influence of each sectors' capital operation in the market. The power of dispersion and sensitivity of dispersion, which were further calculated by the Stone-Formula and Klein-Formula, and we estimate the shock effect of policy authorities on capital flow, and stock of various sectors was measured. Finally, according to the structural changes in China's Flow of Funds, the objective basis and policy options of "who bears the risk" and "who bears the risk better" are given, and we try to explore how to make monetary policy based on market principles to induce the safe and efficient flow of funds in various sectors to achieve a reasonable distribution of social resources.
The flow of funds in an economy is often compared to the circulation of blood in the body. The concept refers to the flow of funds, i.e., the total amount of money flowing in the national economy over a certain period of time. The flow of funds includes physical goods and services exchanged, as well as the exchange of currency and financial assets (securities and stocks). In the real economy, the flow of funds is manifested as the "mutual convection" of money and the exchange of physical assets. In the financial economy, money is separated from physical assets, and the flow of funds is primarily manifested as capital movement caused by capital raising and use in the financial market.
From the perspective of expenditures, GDP can be divided into final consumption, investment, and net exports of goods and services. When a country's domestic savings cannot meet its investment needs, there is a shortage of funds, and it is necessary to raise capital from overseas, producing an inflow of international capital. In contrast, when savings exceed the country's domestic capital needs, excess funds will be used to provide capital to other economies, such as by purchasing bonds issued by other countries and so on, generating an outflow of domestic funds. According to the definition of the flow of funds statistics system, the real economy and the financial economy have the following ex-post equilibrium relationship with the balance of payments.
(Sp−Ip)+(T−G)=(ΔA−ΔL)=EX−IM | (1) |
where ΔA is the change in assets, ΔL is the change in liabilities, EX is the output, and IM is the input. Namely, the difference between Private Savings (Sp) and Private Investment (Ip) + the excess of Government Revenues (T, for Taxes) over Government Expenditures (G) = Financial Surplus or Deficit = Current Account. Government expenditures consist of government consumption expenditures (GC) and government investment (GI), that is, T−G=T−GC−GI. Therefore, the equilibrium for savings and investment for the government sector can be expressed as:
(T−G)=(T−GC−GI)=Sg−Ig | (2) |
In other words, Government Revenues − Government Expenditures = Government Savings − Government Investment. As seen in Equations (1) and (2), the sum of the differences between savings and investment for the private sector and for the government sector is equal to the net financial investment in the rest of the world sector, that is, the external current account.
If ΔA−ΔL in (2.1) is further decomposed into domestic and foreign financial transactions (ΔAd−ΔLd) and (ΔAf−ΔLf), the following formula is valid:
(Sp−Ip)+(Sg−Ig)=(ΔAd−ΔLd)+(ΔAf−ΔLf)=EX−IM | (3) |
Since (ΔAd−ΔLd) cancels out across domestic sectors, the following relationship can be obtained from the balance of payments statement.
EX−IM=ΔAf−ΔLf,(CurrentAccount=FinancialAccount)4 | (4) |
4 According to the Balance of Payments and International Investment Position Manual (BPM6), the Current Account + Capital account − Financial Account + error or omission = 0. To highlight the main relationship between external physical transactions and financial transactions, capital account, and error terms are omitted here.
Equations (3) and (4) indicate the theoretical equilibrium relationship between savings and investment, financial surplus, or deficit and balance of payments. According to this equilibrium relationship, the financial surplus or deficit with the rest of the world sector is consistent with the deficit or surplus of the current account and has a post-event equilibrium relationship corresponding to the balance of domestic savings and investment.
According to Equation (1), we first observe the gap between savings-investment and the reasons why China has been stuck with unusually high savings for a long time. From the 1980s to 2023, China's saving rate has been higher than the investment rate for a long time since the 1990s, which has formed structural problems in China's economic growth (see Fig. 1). The high savings rate (SR_CN) constitutes the outstanding problems on the supply side of China's economic operation, that is, the high investment and the high current account surplus. Net saving rates, in particular, were as high as 4% between 2004 and 2010 and 9.5% in 2007–2008. The current account surplus reached a record 9.6% of GDP over the same period5. However, worrying high investment may be, and however intolerable the foreign trade frictions caused by the large surplus may be, China will have to tolerate high savings to some extent to keep the national economy running while the domestic consumption rate fails to rise as hoped. Li and Yin (2007) studied the reasons for China's high savings based on the flow of funds and institutional sectors. Their analysis concluded that from 1992 to 2003, especially since 2000, the rise of China's national savings rate was mainly attributed to the government and enterprise sectors, while the household savings rate showed a long-term and stable downward trend. This conclusion is consistent with the change in China's saving rate from 1992 to 2003, but with the rise of the national saving rate from 2007 to 2023, the household saving rate has a new change.
5 See China Statistical Yearbook 2021.
We begin by discussing the reasons why the national saving rate remained elevated throughout the observation period and the impact of changes in savings rates across sectors on the national saving rate. Based on the SNA standard, China's FFA is mostly divided into five sectors, namely FCs, NFCs, GG, HHs, and ROW. According to China's FFA non-financial transaction table, for the GG and HHs, the savings account is the difference between disposable income and final consumption, but for NFCs and FCs, because there is no final consumption, their disposable income is their savings. For analysis purposes, we combine NFC and FC into a single enterprise sector (ES). In this way, HHs, ES and GG constitute the domestic sector, and the total savings of the three domestic sectors is the national savings. The ratio of national savings to disposable income of the entire domestic sector is the national savings rate, and the sectoral savings rate = sectoral savings/disposable national income. It follows that the national saving rate is also equal to the sum of the savings rates of the three sectors. Furthermore, two expressions can be used to describe the relationship between savings and disposable income in each sector. First, the sector's propensity to save = sector's saving/sector's disposable income, which reflects the consumption and saving decisions of each sector. Second, disposable income ratio = sectoral disposable income/national disposable income. Obviously, rearranging the above two relations yields the following equation:
Sectoralsavingrate=Sectoralpropensitytosave×Disposableincomeratio | (5) |
Thus, the savings rate of each sector depends not only on the change in the savings of the sector, but also on the change in the proportion of the sector in the national disposable income. As shown on the right axis of Fig. 2, the national savings rate remained above 40% throughout the observation period and peaked at 50.45% in 2010 (see Fig. 2), resulting in a high investment rate and unbalanced growth of the long-term current account surplus.
Figure 2 presents the long-run change in savings rates by each sector from 1992 to 2022, showing that the household saving rate remained at about 22% and its fluctuations were the same as the national saving rate. In 2010, the national saving rate rose to a peak of 50.45% (see Fig. 2), and the household saving rate also rose to 25.43% in 2010 and was 23.1% in 2022. Although the savings rate of the enterprise sector fluctuated, it maintained an upward trend, rising from 12.52% in 1992 to a peak of 22.4% in 2008. After that, it declined in 2012, but it recovered to 23% in 2022, which was the same as the savings rate of the household sector (23.7%). The savings rate of the government sector has been low, averaging about 3.3% during the observation period, −1.2% in 2000–2001, and even showing the largest negative value of −2.4% in 2020. Compared with other sectors, the government sector was seriously short of funds, with the fund deficit reaching a record high of RMB 8.3 trillion since the 1990s6.
6 PBC (2022) Financial Accounts
To see more clearly the reasons for the changes in the savings rates of households, enterprise and government sectors, we break down the reasons for the changes in the savings rates of each sector according to the formula of the savings rate determination. In addition, based on the change trajectory of China's GDP growth (GDPR_CN) rate from 1992 to 2022 (see Figure 1), we divided China's economic growth areas into five periods to reflect the impact of changes in the propensity to save and disposable income ratio of various sectors on the sector savings rate in each period. Table 1 shows the Propensity to save and the Disposable Income ratio of households, enterprises and government sectors calculated from the flow of funds table (Non-financial transaction) in China.
Households | Enterprises Sector | Government | ||||||
propensity to save | Disposable Income ratio | propensity to save | Disposable Income ratio | propensity to save | Disposable Income ratio | |||
1992–1999 | 30.40 | 66.80 | 100 | 20.13 | 25.52 | 13.07 | ||
2000–2006 | 35.39 | 59.46 | 100 | 23.36 | 11.00 | 17.18 | ||
2007–2012 | 42.46 | 56.34 | 100 | 23.55 | 26.85 | 20.11 | ||
2013–2017 | 38.49 | 58.77 | 100 | 16.55 | 21.10 | 20.33 | ||
2018–2022 | 36.39 | 60.47 | 100 | 22.74 | 1.60 | 16.79 | ||
Source: National Bureau of Statistics of China, Flow of Funds Account (Non-financial transaction). Notes: Since the savings of the nonfinancial enterprise sector are equivalent to its disposable income, the sector's propensity to save is 100%. Propensity to save = sector's savings/sector's disposable income; Disposable income ratio = sector's disposable income/total disposable income. |
Table 1 presents the trends in the propensity to save and the ratio of disposable income in each of the three sectors from 1992 to 2022. The propensity to save in the household sector increased from 30.4% in 1992–1999 to 36.39% in 2018–2022, an increase of almost six percentage points, but the sector's disposable income ratio declined sharply, from 66.8% in 1992–1999 to 60.47% in 2018–2022, a decline of more than 6 percentage points. The increase in the savings rate in the corporate sector was mainly due to an increase in the sector's disposable income ratio from 20.13% in 1992–1999 to over 23% in 2000–2012 and got back to 22.74% in 2018–2022. The government sector has decreased in the propensity to save, from 25.52% in 1992–1999 to 1.6% in 2018–2022, but it has risen to 26.85% in 2007–2012, while the disposable income ratio of the government sector has increased slightly, from 13.07% in 1992–1999 to 16.79% in 2018–2022.
Through this analysis, we know that the rise in China's savings rate since 1990, especially since 2000, is influenced by the increase in household propensity to save, the increase in the share of disposable income of the enterprise sector, the sharp increase in the propensity to save of the government sector from 2007 to 2012, and also a large increase in the share of government disposable income. On the other hand, although the household sector's propensity to save has increased, the decline in household proportion in the distribution pattern directly leads to the decline of the household sector's consumption, which is an important reason for the insufficient domestic demand in China.
In addition, although the disposable income of the GG sector has increased, its propensity to save has declined significantly, resulting in a low savings rate of the government sector, which is only 1.6 in 2018–2022; this means that the government's ability to regulate the market has weakened. The balance between how the GG sector raises funds and how it uses funds is also a necessary condition for the effective and rational allocation of resources. Therefore, in the future, if the policy authorities want to achieve a reasonable ratio of savings and consumption to achieve balanced economic growth, they should consider adjusting the structure of income redistribution, increasing the disposable income of the household sector to increase household consumption and improve domestic demand, and increasing the household's expectation of happiness in life. In this way, the household sector will be willing to spend, and a modest reduction in the national saving rate will help to improve the structural problems of China's economic growth. This will lead to a modest reduction in the national saving rate, which will help to address the structural problems in China's economic growth.
These statistical observations and analyses elucidate the reasons behind China's persistently high savings rate, as initially suggested in this article. At its core, high savings reflects a rational allocation of resources. Moving forward, it is essential to adopt the perspective of fund flows and utilize financial account data to explore how monetary policy can be formulated in accordance with market principles. This approach would guide capital to circulate safely and efficiently across all sectors, ultimately achieving the rational allocation of societal resources.
The difference in savings and investment of each sector in the flow of funds account is connected with the financial surplus or deficit in each sector, which becomes an important variable to observe the combination of the real economy and financial economy. Here, we observe the time changes of surplus or deficit of each sector, which are the non-financial sectors of FFA proposed in the previous section, namely, HH, NFC, GG, and ROW, to help us understand the changes in the economy and the impact of each sector on the financial market. Figure 3 uses the ratio of net financial investment to GDP of each sector to show the basic characteristics of financial surplus or deficit of each sector in China, where the causality is as follows.
Figure 3 shows that the NFC sector is the main body of investment in the real economy. In a period of economic growth, they should be in a state of strong demand for funds, that is, insufficient funds. However, the fund gap of this sector generally shows a shrinking trend, from −17.4% in 1993 to −8.24% in 2022. In the early 1990s, due to the rapid economic growth and strong investment demand, the NFC sector carried out large-scale fundraising. In 1996, the fund shortage ratio reached −16.7% and remained above −10% until 1998. After that, however, the fund demand ratio of the NFC sector decreased. By 2015 its underfunding ratio was −1.6%, the lowest since the 1990s, and in 2020, during the COVID-19, it hovered at −2%. The reason lies in the lack of investment demand caused by the NFC sector's lack of expectations on the market, which is consistent with our conclusion in the previous section that the savings rate in the corporate sector increased. The investment ratio of the NFC sector in the macro economy declines (Zhang, 2020), and the NFC sector either invests in the financial sector or holds its own funds to wait for the opportunity, resulting in a downturn in the financing activities of the enterprise sector and insufficient effective demand for credit.
In contrast, although the HH sector was in a state of financial surplus, its ratio of financial surplus to GDP tended to decline from 18.2% in 1992 to 15.7% in 2022 and reached the lowest point of 5.1% in 2017. Through Figure 2, we know that the savings of the HH sector did not decrease significantly during the analysis period; one of the major reasons is the increase in household investment in real estate through the purchase of real estate since 2005, resulting in a decrease in household net savings. On the other hand, the household saving rate was about 23%, which did not show great fluctuation. As shown in Table 1, although the propensity to save of the household sector was on the rise, the disposable income ratio of the household sector was on the decline, so the real income of the household sector was on the decline in the national disposable income. The increase in household financial surplus is simply the result of sluggish consumer spending and a shift in income to savings as a hedge against expected risks. However, in recent years, the phenomenon that M1 is too low and M2 is too high in China's deposits, and it is difficult for household savings to be converted into demand deposits of enterprises also shows the seriousness of the downward impact on the macro economy. HH sector savings is an important source of funds for enterprises and government sectors to invest and produce. Efforts to raise household income, maintain stable savings, and expand consumption and investment are necessary conditions for stable economic growth.
From the perspective of the GG sector, from 1992 to 2004, GG sectors were in a state of insufficient funds, but from 2005 to 2015, under the state of insufficient investment in the NFC, the GG sector generally became surplus sectors, showing the structural problems in the use of funds in China. During 2007–2008, due to the impact of the financial crisis in the United States, the GG sector increased public investment to expand domestic demand and stimulate the economy, which resulted in insufficient funds for the GG sector (−2.4% in 2007). From 2008 to 2015, the fund surplus ratio of the GG sector was about 2%. However, the ratio of insufficient funds for the GG sector in 2018–2022 reached the peak of −8.2% during the analysis period, and its funding gap reached 8.3 trillion yuan at 2020, the highest among all sectors. GG debt to GDP ratio has increased continuously after the jump up in 2020, this is a problem that deserves special attention and vigilance (Vitor Gaspar, 2023).
On the other hand, the financial surplus or shortage of the ROW sector is just the opposite of that of the domestic sector. Except for 1993, the ROW sector has always been underfunded, that is, the net outflow of domestic capital (including the increase of foreign exchange reserves). The financial shortage ratio of the ROW sector increased from 1.3% in 1992 to a peak of 9.8% in 2008. Since then, due to changes in the external environment, such as the U.S. financial crisis and the European debt crisis, China has maintained its external financial net surplus, but the financial surplus ratio has shown a downward trend, from 9.8% in 2008 to 2.3% in 2022. Overall, China's net foreign financial investment is in a declining trend.
There are two notable inflection points in the long-term development trend of fund flows and the direction of non-financial sectors, one in 2008 and the other in 2017. Although affected by the American financial crisis before and after 2008, the Chinese policy had to deal with it properly, and there were positive changes in the fund flows and flow of all sectors. The financial surplus of the HH sector is gradually recovering, the investment demand of the NFC sector is increasing, the financial surplus of the GG sector is stable, and China's net external financial assets are increasing. However, since 2017, the financial surplus of the GG sector has dropped to the lowest point of −8.21% in 2020, and has not seen a significant recovery so far; the capital demand of the NFC sector continues to show a shrinking trend; the funding gap of GG sector has increased in recent years; and the decrease of China's net external financial investment. It shows that China's economy is facing a new and more severe situation with the international environment upheaval caused by the China-US trade friction since 2018, COVID-19 in 2020 and the Russia-Ukraine war in 2022.
From the analysis of the time series changes in the financial surpluses or deficits of various sectors above, it is evident that since 2017, there has been a structural shift in the financial operations of different sectors in China. The decrease in expected consumption by the HH sector has led to an increase in net savings, while the NFS sector has experienced insufficient effective funding demand and a decline in the financing ratio. Correspondingly, to stimulate the economy, the GG sector has expanded public investment, further increasing its funding deficit rate to −8.2%, with a funding gap reaching 8.3 trillion yuan, the highest among all sectors. Moreover, the ROW sector has also shown a contraction trend in external capital circulation. Facing this new situation, the policy authorities have proposed a dual-circulation development strategy. To achieve balanced development in the dual-circulation strategy, it is essential to first adhere to market mechanisms and utilize monetary and fiscal policy tools to regulate the efficient and secure allocation of financial resources among various sectors. Then, in response to changes in the international trade environment, China's external capital circulation is bound to undergo shifts in the scale and direction of foreign financial investments and financing. Consequently, there is an increasing need for information on counterparties within domestic sectors as well as those involved in external financial transactions, namely W-t-W data.
Considering China's long-standing high savings rate and the intensification of financial bubbles as analyzed earlier, it is imperative to address structural issues in fund flows. A more detailed examination of the capital operations and policy impacts on both the non-financial and financial sectors, from both flow and stock perspectives, is essential.
Since the flow data sometimes have negative numbers, we adjusted the data to obtain the ratio coefficients of all positive numbers and then compile the financial matrix table. That is, the negative data of the liabilities in the flow of funds table is moved to the corresponding asset side, which makes it positive data, and the negative data of the asset side is moved to the corresponding liability side, making it become positive data. This adjustment method is both in accordance with the principle of double-entry accounting and the meaning of the actual economy, which is the data of the amount of capital flow that is positive.
As for the stock data, the People's Bank of China has been publishing the 2017–2023 financial asset-liability stock data since 2022. Li and Zhang (2020) compiled the asset-liability stock data for 2000–2019. This data is based on the basic concepts of the 2008 SNA, the framework of transaction items, and sector classification and is consistent with the accounting principles of the 2008 SNA. We use the above stock data to estimate the power-of-dispersion index (PDI) and sensitivity-of-dispersion index (SDI), and the results show that the two coefficients are distributed in the same quadrant, so it can be considered that the integration and accuracy of these data are the same. Next, based on the analytical framework of the financial flow of funds, use the above asset-liability data and flow data to prepare the flow of funds matrix and asset-liability matrix.
In the international standard flow of funds statement, the rows represent each item (i.e., m financial instruments) and the columns represent each institutional sector (i.e., n institutional sectors), within which assets and liabilities are listed separately according to double-entry bookkeeping. We focus on the method of establishing the Y matrix (sector × sector).
Two methods for converting T-type accounts into Y matrices were proposed by Stone (1966) and Klein (1983), respectively. When Stone (1966) presided over the revision of the 1968SNA, he prepared the financial matrix table reflecting the asset-liability relationship of various sectors by referring to Table U and Table V (see Table 2 for details). According to the rows and columns, the matrix is divided into four parts: Institutional sector, financial transaction items, physical assets, and total.
n sectors | m financial claims | Real asset/accumulated saving | Row totals | |
n sectors | Y | Ajk | ej | wj |
m financial claims | L'jk | X | lk | |
Real asset/ accumulated saving | z'j | ξ | ||
Column totals | x'j | a'k | ε | |
Source: R. Stone, "The Social Accounts from a Consumer's Point of View", pp.19−24. |
The n rows and n columns of the original matrix Y on the left correspond to each institutional sector, each row represents the assets of each sector, and each column represents the liabilities of each sector, reflecting the relationship from who to whom. M rows and m columns on the second block show the various financial instruments, constituting two matrices with n institutional sectors, namely, "sector × instrument" matrix (Ajk) and "instrument × sector" matrix (L'jk). Among them, the Ajk shows that the j sector held the k class financial instrument assets, and L'jk shows the j sector held the k class financial instrument liability. Next, according to the row and column embedded in real assets and accumulate savings, respectively, for ej and z'j, respectively. The last row and column describe the total of rows wj, lk,ξ and the total of columns x'j,a'k,ε. The financial matrix table reflects the basic structure of input-output table of U-V type. According to this framework, the flow of funds account of T-shaped type can be converted into Y matrix (sectors × instruments) and X matrix (instruments × instruments).
According to the definition of Stone (1966), the asset input coefficient a'jk is calculated by the vector and row sum of the asset transpose matrix, while the liability input coefficient ljk is calculated by the liability matrix and column sum. If the w and k in Table 1 are known, the two-input coefficient is as follows:
a'jk=A'jkˆwj−1 | (6) |
ljk=Ljkˆlk−1 | (7) |
Among them, the ˆwj for vector wj diagonal matrix, ˆlk for vector lk diagonal matrix, the top right corner −1 and apostrophes are the symbols for inverse matrix and transposed, respectively.
Klein (1983) also put forward the research idea of linking the fund flow statement with the national income account and the input-output table in a matrix representation. His analytical approach is similar to Stone's (1966) but with a different definition of the input coefficient. Klein calculated each proportion by dividing each item of assets and liabilities with the total of the other party, focusing on the fact that the use of funds of each subject depends on the funds raised, and then monitoring the relationship between the fundraising and use of each department.
Continuing to take Table 1 as an example, Klein (1983) defined the input coefficient of the flow of funds matrix as follows.
d'jk=L'jkˆwj−1 | (8) |
cjk=Ajkˆlk−1 | (9) |
where, d'jk represents the liability ratio of various financial instruments in the total assets held by each sector, and cjk represents the asset ratio which each sector in the total liabilities of each financial instrument.
Therefore, the Stone formula uses the right side of the T-shaped account (debt) as its basis in Y matrix, expressed as YS, and Klein formula using a T-shaped account on the left (asset) for calculating standard into a Y matrix, expressed as YK. Before calculating the input coefficient matrix, the flow of funds statement of the T-shaped account is first divided into two matrix tables by the using and raising of funds. Table E reflects the use of funds and table R reflects the raising of funds. For details, refer to Tsujimura and Tsujimura (2018) and Zhang (2020). E table and R table for m × n matrix, the matrix elements ekj and rkj respectively.
Based on Stone's formula and Klein's formula, with flow (or stock) data from the R table and E table, we find the corresponding input coefficient matrix CS (Stone method) and CK (Klein method), and then establish YS and YK matrix with W-t-W form. On this basis, we can investigate the capital ripple effect from the asset side and the liability side, calculate the total amount of economic transactions generated by the use or raising of funds in a certain sector, and deduce the limit effect of net induced economic transactions by applying the principle of Leontief inverse matrix.
Through E table and R table, we further define the diagonal matrix ^T,ˆTE, and ˆTR. ˆT as a n × n matrix, the diagonal elements are tj, everything else is 0. Similarly, ˆTE and ˆTR are m × m diagonal matrix, respectively by tEk and tRk as elements. In addition, the elements of vectors ε and ρ are εj and ρj, respectively. The details are as follows7:
7 See Tsujimura and Tsujimura (2018,161–162).
tEk=∑jekj;tRk=∑jrkj | (10) |
tj=max(∑kekj,∑krkj) | (11) |
εj=tj−∑kekj≥0;ρj=tj−∑krkj≥0 | (12) |
Formulas tEkandtRk are the kth term's total financial assets and total financial liabilities, respectively, and consider all the sectors (including foreign), we can get tEk=tRk; Formula (11) the tj shows the larger value between total assets and total liabilities in j sector; Equation (12) εj and ρj fund surplus and deficiency, respectively, in the j sector.
We use the method of table U and table V in the input-output model to deal with table E and table R, and the superscript S and K represent Stone's formula and Klein's formula, respectively, as follows:
US≡R;VS≡E' | (13) |
UK≡E;VK≡R' | (14) |
Further, the use of matrix US, VS,UK,VK each element of the divided by column sum (row sum), to define the coefficient matrix BS, DS, BK, DK:
BS=USˆT−1;DS=VS(ˆTE)−1 | (15) |
BK=UKˆT−1;DK=VK(ˆTR)−1 | (16) |
Stone formula, DS for E (assets) transposed matrix input coefficient, BS for R (debt) matrix input coefficient; Klein formula, DK for R (debt) transposed matrix input coefficient, BK for E (assets) input coefficient matrix. Then, we can get the payer–payee matrices with the W-t-W form, namely YS(seeTatel2) and YK(seeTatel3), and the corresponding coefficient matrix CSandCK in the following manner:
CS=DSBS;YS=CSˆT | (17) |
CK=DKBK;YK=CKˆT | (18) |
When the economy is in a state of unbalanced growth, the financial risks in a certain sector have different ripple effects on the use and raising of funds. By observing the Stone formula from debt as a benchmark flow of funds matrix YS, the column represents a fund-raising (debt), and the row represents fund use (assets). If a column total of YS matrix is greater than the corresponding row total, the net debt of this sector increases; thus, the possibility of debt default risk can be observed. This situation will affect the external impact of other sectors' assets and then affect the overall flow of the funds system. Similarly, the asset side is a benchmark to observe matrix YK, the column represents fund use (assets), and the row shows the raising (liabilities). Such as YK, a column total is less than the corresponding row total, the net assets reduce the sector, which can be viewed as the department's net worth falling to form a liquidity risk. This change will also affect the overall flow of the funds system. Because tEk=tRk, then YS and YK are a diagonal symmetry, namely YK=(YS)', and each sector of the asset-liability changes in the flow of the funds system is completely symmetrical transmission mechanism (Tsujimura and Tsujimura, 2018; Zhang, 2020).
Referencing formula (6)−(18), using the data of China's flow of funds and assets from 1998 to 2022 and assets and liabilities data from 2000–2022, we calculated the flow of funds matrix and assets and liabilities matrices with YSandYK form time series. Due to space limitations, only the flow matrix and stock matrix of 2022 are listed here, as shown in Table 3 and Table 4.
YS | |||||||
HHs | NFCs | FCs | GG | ROW | ε | Yi | |
HHs | 61 | 3225 | 222786 | 17909 | 1372 | 0 | 245354 |
NFCs | 1073 | 12720 | 70846 | 2220 | 12317 | 99323 | 198499 |
FCs | 54814 | 173806 | 88306 | 83265 | 33889 | 0 | 434081 |
GG | 128 | 610 | 18221 | 6 | 3907 | 83705 | 106577 |
ROW | 58 | 8137 | 12300 | 3176 | 7763 | 27813 | 59247 |
ρ | 189220 | 0 | 21621 | 0 | 0 | TVFT | |
Yj | 245354 | 198499 | 434081 | 106577 | 59247 | 832917 | |
YK | |||||||
HHs | NFCs | FCs | GG | ROW | ρ | Yi | |
HHs | 61 | 1073 | 54814 | 128 | 58 | 189220 | 245354 |
NFCs | 3225 | 12721 | 173805 | 610 | 8137 | 0 | 198499 |
FCs | 222786 | 70846 | 88306 | 18221 | 12301 | 21618 | 434079 |
GG | 17909 | 2220 | 83265 | 6 | 3176 | 0 | 106577 |
ROW | 1372 | 12317 | 33888 | 3906 | 7763 | 0 | 59246 |
YS | |||||||
HHs | NFCs | FCs | GG | ROW | ε | Yi | |
HHs | 5727 | 210443 | 1965090 | 121419 | 27158 | 0 | 2329837 |
NFCs | 1894 | 408839 | 1125760 | 7548 | 133729 | 1056509 | 2734278 |
FCs | 843316 | 1885021 | 1503634 | 683060 | 334788 | 0 | 5249819 |
GG | 219 | 22668 | 402803 | 149 | 1989 | 408844 | 836671 |
ROW | 130 | 207308 | 89433 | 24496 | 147119 | 176297 | 644783 |
ρ | 1478551 | 0 | 163099 | 0 | 0 | TVFT | |
Yj | 2329837 | 2734278 | 5249819 | 836671 | 644783 | 10153738 | |
YK | |||||||
HHs | NFCs | FCs | GG | ROW | ρ | Yi | |
HHs | 5727 | 1894 | 843316 | 219 | 130 | 1478551 | 2329837 |
NFCs | 210443 | 408839 | 1885021 | 22668 | 207308 | 0 | 2734279 |
FCs | 1965090 | 1125760 | 1503632 | 402803 | 89433 | 163099 | 5249817 |
GG | 121419 | 7548 | 683060 | 149 | 24496 | 0 | 836671 |
ROW | 27158 | 133729 | 334788 | 1989 | 147119 | 0 | 644783 |
Source: People's Bank of China (2022) Flow of Funds Statement (Financial Accounts); Financial Assets and Liabilities Statement (Financial Accounts). Note: The total value of the economic transactions (TVET) in the bottom-right corner of each table indicates the total value of economic transactions between sectors (including the rest of the world). |
Table 3 and Table 4 show the direction and flow of funds raised and used by each sector, as well as the size of assets and liabilities held by each sector to other sectors. The three-dimensional structure can display information about the counterparties of each financial instrument according to the columns of the flow matrix YS in 2022, the financial liabilities of the GG from HHs, NFCs, FCs, and ROW are respectively RMB 1790.9 billion, RMB 222 billion, RMB 8326.5 billion, and RMB 317.6 billion. The GG also holds assets of RMB 12.8 billion, RMB 61 billion, RMB 1822.1 billion, and RMB 390.7 billion in these four sectors, respectively.
The financial surplus or deficit for each sector can be calculated by finding the difference between the total of each row and the total of the corresponding column. Looking at the totals from the perspective of the GG, the column totals RMB 10657.7 billion, which represents total liabilities, while the row totals RMB10657.7 billion, including both assets and net liabilities. As a result, the net debt held by the GG is RMB 8370.5 billion in 2022 (see Table 3), the highest since the 1990s. It can be seen that Chinese government sectors are in a serious stage of capital shortage in recent years.
Moreover, the last column and row of the matrix indicate that the sum of each row is equivalent to the sum of each column. In other words, the total assets of each sector plus the net liabilities are equivalent to the total liabilities of each sector plus the net assets. Furthermore, the overall value of social assets equals that of liabilities, indicating the total magnitude of social fund flows and the assets-liabilities during a particular period. In 2022, China recorded a total fund flow of RMB 83.29 trillion (refer to Table 3), and its total assets-liabilities amounted to RMB 1015.37 trillion (refer to Table 4).
Based on the flow of funds matrix and financial asset-liability matrix prepared above, the supply and demand of capital and the dynamic changes of each sector in China's flow of funds in the past two decades are investigated to answer the questions of "who is bearing the risk" and "who is better to bear the risk".
The triangulation of matrix is a visual data processing that defines the net flow of funds by deducting the net flow of funds between various sectors and rearranging its rows and columns through the flow order of funds. Through such sorting, the maximum number of non-zero elements can be located below the diagonal line to know the mutual relationship and sorting of various sectors in the fund operation. Tsujimura and Mizoshita (2002, 63–68) first applied this method to the asset-liability matrix derived from the financial balance sheet. In the sequence of capital borrowing and lending with a strict triangular financial asset liability matrix, the sectors below the diagonal are the providers of capital, and the sectors above the diagonal are the demanders of capital. The sectors above the diagonal have influence over the sectors below when they raise funds, but the sectors below the diagonal have no influence over the sectors above when they raise funds. Similarly, the sectors above the diagonal do not influence the sectors below them when they use their funds, but the sectors below the diagonal influence the sectors above when they use their funds.
The creditor-debtor relationship between departments is usually two-way, so the triangulation offsets the creditor-debtor of sectors "i to j" and "j to I". Reference Tsujimura and Tsujimura (2020) formula and the method of the triangle first determine the net assets of sector i towards department j; then, set the elements of the net fund outflow from sector i to sector j to be positive as 1, and 0 for others, constructing a matrix consisting of 0s and 1s. The specific relations are as follows.
yij={yij−yji(i≠j;i,j=1,…,n)yij(i=j;i,j=1,…,n) | (19) |
˘yij={1 yij>00else(i,j=1,…,n) | (20) |
The elements yij, yij and ˘yij are n×n matrices Y, Y, and ˘Y, respectively. Let ZC be a vector whose elements are the number of nonzero elements in each column of ⌣Y and let ZR be a vector whose elements are the number of nonzero elements in each row of ˘Y. Furthermore, define the triangular sort Z as follows.
Z=n⋅I−ZC+ZR=n⋅I−˘Y'I+˘YI | (21) |
where I is a unit vector. The Y matrix to the rows and columns of the Zi in ascending order for triangularization can be prepared in accordance with the fund-raising for based on column vector matrix. Alternatively, a matrix based on row vectors can be created, with fund-using as the benchmark. As shown in the previous derivation, the mathematical operation results of Stone's formula and Klein's formula are symmetric. According to the above setup, the sectors below the triangle borrow from a limited number of sectors and provide loans to many sectors, which are the major providers of funds. Moreover, the sectors in the top half of the triangle lend only to a limited number of sectors but raise funds from multiple sectors, which are the major borrowers. If the triangulation ranking of a sector increases, it means that its net asset value to other sectors decreases or a large amount of debt accumulation, bringing debt default risk and liquidity risk. That is, the risk that the borrower is unable to repay the debt, and that one party in the funding credit cannot buy or sell assets at a reasonable price in a timely manner or cannot meet the funding requirements.
Based on the perspective of fund raising, the Stone-formula triangulation of the stock matrix and flow matrix between sectors are shown in Figure 4 and Figure 5.
First, from the stock observation (see Fig. 4), we can see that the HH sector has always been at the bottom of the triangle, which indicates that the household sector is the source of capital raising in the economy. However, the HH sector borrowing rose slightly after 2009, reached a peak in 2013, and then stabilized at order 1, lasting until 2022. This is consistent with the tendency of the HHs sector surplus to shrink, as shown in Figure 3. The NFC sectors have always been at the top of the triangle and are the major borrowers, that is, the main body of investment demand. Although Figure 3 shows that the capital demand of the enterprise sector has shrunk somewhat, it still maintains the position of the main borrowers in the economy. The FC sector institutions are in the middle of the triangulation, playing a role of regulation and intermediation, with little contrast in ranking changes. Since 2006, they have ranked the third order and are in the middle position. Their financing tendency continues steadily until 2019 and drops to the second level from 2020 to 2022, with their financing ability returning to the state before 2005. In other words, the FC sectors have limited influence on the NFCs, the ROW, and the GG sectors when raising funds, and their role as financial intermediaries in the financial flow of funds needs to be further played.
The GG sector has the biggest change in the ranking of the triangle. From 2001 to 2004, it was in the fourth order, which was higher in the ranking. From 2006 to 2016, it was always in the second order, which was lower in the ranking. The rise to order 4 in 2020–2022 indicates that the scale of the GG sector borrowing has increased in recent years. As can be seen from the prepared matrix table, the financial assets and liabilities of the GG sectors at the end of 2022 are 40.88 trillion yuan and 83.67 trillion yuan, respectively (See Table 4), which are 0.42 times and 3.01 times that at the end of 2016, and the net assets in 2016 are 73.3 trillion yuan and the net liabilities in 2022 are 40.88 trillion yuan, which significantly increases the debt default risk.
The ROW sector is higher than the FCs sector in the triangulation, but the change is low, rising from the middle level in 2000 to a higher rank after 2004, but falling back to the third order after 2017–2022. Since the use and raising of funds in the ROW sector is opposite to that in the domestic sector, it indicates that China's use of foreign funds is relatively shrinking. This is consistent with the characteristics shown in Figure 3.
Then, we observe the flow matrix. The changes in the sectors' order of the flow is larger than that of the stock, which can reflect the capital flow caused by the change in economic prosperity. The frequent transposition of the top and bottom of the triangulation of each sector indicates the fluctuation of the ranking position of each sector in the market fundraising. It is worth noting that the HHs sectors are basically at the bottom during the observation period, while the NFC sectors are mostly at the top of this chart, but they have been replaced by the GG sector in the past three years. This ranking indicates that the HHs sector's financial supply plays an important role in overall financial flows, while the position of the NFC sector, which is the main body of investment demand, has continued to decline after 2015, particularly to the lowest point in the observed period (level 3) in 2019–2022, which is consistent with the phenomenon observed in Figure 3.
The position of the GG sector changes the most and fluctuates the most. According to the fund flow matrix, the net financial debt of the GG sector reached −175.2 billion yuan in 2002, and the triangulation reached the highest point. In 2007, in response to the US financial crisis, the net debt of the GG sector increased to −654 billion yuan, resulting in the triangular ranking rising to the fourth level. Due to the strong countermeasures, the GG sector turned to net financial assets of 6.1 billion yuan in 2008, and the triangular ranking fell to the second level. In 2015, the financial net assets of 1150.8 billion yuan reached their lowest point in the triangulation analysis. However, after 2016, the new net debt of the GG sector continued to rise, soaring from −808.4 billion yuan in 2016 to −40.88 trillion yuan in 2022, the highest value in the entire observation period. In the triangulation of the fund flow matrix, it exceeded other sectors and was at the highest point, affecting other sectors below it. From this, it can be seen that the capital raising of the GG sector has had a large fluctuation in recent years, even more than that of the NFCs sectors, and the debt default risk of the GG sector has increased.
The FCs sectors are typically the dominant lender, ranking roughly in the middle third tier over the period under review, moving up when lending began to increase in 2015 and down when loans were withdrawn. During the observation period, the flow triangulation of the FC sectors continued to decline, and the support role of the real economy was insufficient. In 2018–2020, the sector's lending slowed, its triangulation declined, and it did not fully play its due role as a financial intermediary, but the ranking returned to third tier in 2021–2022. The FC sectors should flexibly use market leverage to strengthen its intermediary role in serving the real economy.
Combining Figure 4 and Figure 5, it can be seen that the volatility of fund flows will not show up in the assets and liabilities until it accumulates to a certain extent. The triangulation sort inflection points of the flow matrix appeared in 2015, and since 2016, the ranking of the NFCs sectors and the FCs sectors have continued to decline, while the ranking of the GG sector and HHs have continued to rise. The triangulation sort inflection points of the inventory matrix appeared in 2017, and the ranking of the GG sector continued to rise from 2017, while other sectors did not change much. From the perspective of various sectors, the fund provider status of the HHs sector is generally stable, but after 2015, it shows a trend of increasing fund demand, and its flow matrix triangulation reaches the second order in 2022. The stock triangulation of the NFCs sectors remained at level 5, and the fund-raiser position remained stable. However, the flow rate fluctuates greatly. After 2015, the demand for funds plummeted, and its triangulated sort of the flow matrix shifted from level 5 in 2014 to level 2 in 2021. It is foreseeable that the triangulation sort of the NFC stock matrix will also begin to decline in the future, reflecting weak real investment and increasing downward pressure on the economy. In the FC sector, the triangulation sort of the flow matrix has been declining since 2015, and the triangulation of the stock matrix has been declining since 2020, indicating that financial institutions are investing funds for economic growth. Before 2016, the GG sectors were primarily the fund providers for the NFC sectors and financial institutions. However, since 2017, their fund flow has fluctuated significantly, and the triangulation sort of the flow matrix has risen rapidly, from the second level in 2016 to the highest level in 2020–2022. This indicates that the net asset value of the government has declined, resulting in liquidity risks and a large amount of debt accumulation. Government debt has a strong 'pro-cyclicality, ' especially given the current increasing economic downward pressure. If the risk of government debt default arises, banks and other financial institutions will incur a significant number of non-performing assets on their balance sheets. Therefore, the risk of government debt warrants close attention.
Here, the power of dispersion index (PDI) and sensitivity of dispersion index (SDI)8 of each sector in the flow of funds matrix and financial asset-liability matrix are observed from the sectoral perspective. PDI refers to the capital ripple effect of the whole system brought by the increase of unit capital supply of a certain sector, while SDI focuses on the capital ripple effect of a certain sector when the capital demand of the whole system increases by one unit.
8 It was Rasmussen (1956) who invented the dispersion indices for the input-output analysis. While the Power-of-Dispersion Index (PDI) is the mean-normalized column sum, the Sensitivity-of-Dispersion Index (SDI) is the mean-normalized row sum of the Leontief inverse.
The PDI of a sector is the ratio of the column sum of the Jth column of the Leontief inverse9 matrix to the column mean, and the SDI of the sector is the ratio of the row sum of the ith row to the row mean. We use dpj to define the PDI of sector j, and use dsi to define the SDI of sector i as follows:
9 Leontief (1953, 1963).
dpj=∑ni=1cij1n∑nj=1∑ni=1cij;dsi=∑nj=1cij1n∑ni=1∑nj=1cij | (22) |
Among them, the cij for the Leontief inverse matrix (I−C)−1 elements. C is the input coefficient matrix of matrix Y, corresponding to the direct consumption coefficient in the Input-Output Model. When PDI > 1, the impact of fundraising (supply) on other sectors is higher than the overall average level. When PDI < 1, the impact of fundraising (supply) on other sectors is lower than the overall average level. Obviously, the higher the PDI, the greater the impact of capital fluctuations in this sector on the capital market. Similarly, the size of SDI also reflects the differences in the perception of the overall average fundraising (supply) among different sectors.
According to different definitions of columns and rows of the matrix, C can be substituted into the input coefficient matrix with Stone type CS and Klein type CK respectively, and then solve out the Stone formula PDIS and SDIS, and Klein formula PDIK and SDIK. PDIS represents the total amount of capital supply in the economy as a whole (i.e., indirect and direct) when unit capital raising increases in a sector; PDIK represents the ripple effect on the total demand for capital in the economy when a sector increases the supply of capital per unit. SDIS represents the ripple effect of a sector that can increase the fund supply when the fundraising of all sectors increases per unit; SDIK represents the ripple effect on the amount of capital demand of a certain sector when the capital supply of all sectors increases by one unit. Both coefficients can partly reflect risk bearing and the dispersion. For example, if the influence of capital supply PDIK and the sensitivity of capital raising SDIS of a certain sector are both large, it indicates that the potential debt risks of other sectors have increased and are gathering to this sector through inter-sectoral transmission.
Using the prepared asset-liability matrix and flow of funds matrix, the time series of PDIS and SDIS of Stone's formula and PDIK and SDIK of Klein's formula were calculated, respectively, which were plotted as a Scatter diagram, as shown in Figure 6–9. Figure 6 shows the PDIs Scatter diagram of each sector in the asset-liability matrix from 2000 to 2022. The horizontal axis PDIS represents the influence coefficient of liabilities, and the vertical axis PDIK represents the influence coefficient of assets. The coordinates of the intersection are (1, 1). Starting from the upper right corner of the figure, the PDI of each sector is divided counterclockwise into four quadrants, namely Quadrants Ⅰ, Ⅱ, Ⅲ, and Ⅳ, indicating the basic ranking of the influence of each sector in the flow of funds system.
It can be seen that FCs are in Quadrant Ⅰ, HHS and GG are in Quadrant Ⅱ, and NFCs and ROW are distributed in Quadrant Ⅳ. This indicates that from the perspective of stock, the influence of the financial sector on fundraising (supply) is significantly greater than that of other sectors during 2000–2022. HHs and GG sectors PDIK > 1, PDIS < 1. This indicates that the influence of the two sectors on increasing fundraising is less than the average level, while the influence of fund supply is larger, and the influence of HHs on fund supply is slightly greater than that of GG. However, the GG sector abnormally falls in quadrant Ⅳ in 2020–2022, which means that the impact of liabilities of the GG sector increases, and the impact of assets decreases during 2020–2022. NFCs and ROW sectors, mainly in Quadrant Ⅳ, PDIK<1, PDIS>1, contrary to the HHs and GG sectors, these two sectors have a greater influence on the fund demand of the whole society when the demand for funds increases, while the influence of fund supply is lower than other sectors.
Figure 7 is a Scatter diagram of the SDIs asset-liability matrix, the horizontal axis SDIS represents the liability induction coefficient, and the vertical axis SDIK represents the asset induction coefficient. The FC sector is positioned in Quadrant Ⅰ, especially in 2020, when it jumps to the maximum value. It is the most sensitive to the economic economy. The fund raising and supply of all sectors have a large ripple effect on the FCs sector. The NFCs sectors, as the major borrowers, have a basic distribution in Quadrants Ⅰ and Ⅱ, rendering SDIS<SDIK, the liability sensitivity is lower than the asset sensitivity. When the fund supply of the whole society increases, the sensitivity of this sector is greater than the ripple effect brought by the whole society fundraising. The GG and the ROW are in Quadrant Ⅲ, far from the coordinate intersection point (1, 1), which means that the sensitivity of social financing in these two sectors is low and they are not the major beneficiaries of the financial market. The location of the HHs sector in Quadrant Ⅳ, SDIK<1, SDIS>1. When the fund raising of the whole society increases, HHs have a strong sense of the change of capital supply.
Figure 8 shows the Scatter diagram of the PDIs fund-flow-matrix 1998–2022, and the PDI of the flow data changes greatly. The FCs sector was in Quadrant Ⅰ at the beginning of this century, but after the financial crisis of 2008, especially since 2017, it has entered Quadrant Ⅱ, and the influence of capital raising has declined. The HHs sector is usually in Quadrant Ⅱ, but the PDIS of the HHs is below average, while the PDIK is in a slow decline, explaining that its influence of fundraising is low, and the asset clout is waning. The GG's PDI changes the most, with distribution across four quadrants. It was in Quadrant Ⅳ at the beginning of this century, moved up to Quadrant Ⅰ during 2003–2004, and then moved to Quadrant Ⅱ. In recent years, especially 2019–2022, it has clustered in Quadrant Ⅲ and Ⅳ, whose influence is below average and very unstable. In contrast, NFCs are mainly distributed in Quadrant Ⅳ, where liabilities have a strong influence and assets have a weak influence, but they are in Quadrant Ⅰ in 2007, 2010, 2015, and 2022. ROWs are mainly in Quadrant Ⅳ, but since 2018, they have risen to Quadrant Ⅰ, and the overall observation shows that the influence of this sector on financing from China is higher than that on the use of funds in China, showing a tendency to exert more influence on China's financial market than other domestic sectors.
Figure 9 is the Scatter diagram of the SDIs flow-of-fund-matrix 1998–2022. FCs sectors are mainly distributed in the upper right part of Quadrant Ⅰ, with the strongest sense of capital supply and demand. NFCs straddle Quadrants Ⅰ and Ⅱ, indicating that the increment of capital demand of non-financial enterprises increases with economic growth and decreases with economic stagnation, which is quite sensitive to economic prosperity. The household sector is mainly distributed in quadrants Ⅲ and Ⅳ. As the sector that holds net assets, the HHs sector has a relatively low sensitivity to capital demand, so it is not very sensitive to changes in the economic environment. The SDIs distribution of GG and ROW sectors is concentrated in the lower left corner of Quadrant Ⅲ, reflecting the slow sensitivity to the overall economic changes, which is lower than the average level of other sectors.
Fig. 8 and Fig. 9 reflect the distribution changes in the flow of funds time series, from which two significant features can be found. First, both these graphs are distributed in sequence coordinates at roughly a 45-degree Angle but with opposite slopes. Fig. 8 slopes downward from left to right at a 45-degree Angle with a negative slope, while Fig. 9 extends upward from left to right at a 45-degree Angle with a positive slope. Second, in Fig. 8, the horizontal axis shows the influence of liabilities, while the vertical axis shows the influence of assets. The changing trajectory of each sector over the past 23 years shows that there is a negative correlation between the influence of liabilities and the influence of assets. In Fig. 9, the horizontal axis is liability sensitivity, and the vertical axis is asset sensitivity. For the changes in liability sensitivity and asset sensitivity, each sector presents a strong positive correlation trend. Through these regular changes, the policy authorities can take appropriate measures to prevent financial risks.
Through the observation of Figure 6–9, it can be seen that the FCs sector has the strongest influence on China's financial market and the highest degree of sensitivity in the asset-liability matrix and the flow of funds matrix during the analysis period. The influence of the HHs sector, as the largest capital provider, is in Quadrant Ⅱ in both the stock matrix and the flow matrix, while the degree of sensitivity is listed in Quadrant Ⅳ in the stock matrix and across Quadrants Ⅲ and Ⅳ in the flow matrix. The NFC sector, which has the largest capital demand, is constantly changing its position in China's flow of funds, and its influence in both the stock and flow matrix ranks in Quadrant Ⅳ. When the capital demand of this sector increases, it has a greater impact on other sectors. In addition, the position of influence and sensitivity of GG sectors in China's flow of funds is the most unstable. The influence sequence of the stock matrix is in Quadrant Ⅱ, the influence sequence of the flow matrix is distributed in four Quadrants, respectively, and the induction sequence of the stock and flow is in Quadrant Ⅲ, which is lower than the average level. PDI of ROW sectors were mainly clustered in Quadrant Ⅳ and Ⅰ, indicating that they had a certain influence on the domestic financial market, but their SDI was distributed in Quadrant Ⅲ, and foreign funds' sensitivity to changes in the Chinese market was lower than that of other sectors.
The time series distribution of influence and sensitivity of various sectors reflects the structural changes in China's financial assets and liabilities and the results of policy intentions. The influence of money supply on the FCs' PDIK induction degree and liabilities SDI of FCs was most pronounced, particularly evident from 1998 to 2022, with certain sectors demonstrating a heightened risk of default concentration towards financial institutions. Compared with PDIKandSDIS of other sectors, the FCs have the strongest influence on capital supply and the most sensitive degree of debt. It can be seen that from 1998–2022, the potential default risks of all sectors are concentrated in FC sectors. Combined with the above observation and analysis results, it can be seen that in recent years, the debt risk has gradually concentrated in the GG sector, and once the debt default exceeds the warning line, it may have a spillover effect on FCs sectors. In practice, so far it has been the government that has assumed and absorbed the debt risk.
Then, from the perspective of macro-prudential supervision, who is better to bear financial risks? Use the flow of funds matrix, and by Stone-formula PDISandSDIS, do a multiplier calculation, and an inspection of the FCs sector and the GG sectors show that they affected the effect of management of funds
A macro index in the flow of funds analysis is the ripple effect coefficient of capital supply and demand (Tsujimura and Mizoshita, 2002). The liability ripple coefficient (liability multiplier) and asset ripple coefficient (asset multiplier) can be obtained from the liability side and the asset side, respectively, according to the sum of the coefficients of the Leontief inverse matrix calculated by the two formulas divided by the number of sectors. The larger the ripple coefficient is, the higher the multiplier effect of the fundraising and usage of the same unit is. The value has nothing to do with the amount of capital and depends on the investment portfolio of the fund operation of each institutional sector. Therefore, it is easy to observe the time change in a country's capital circulation operation effect using the capital ripple coefficient. Since the flow data can more keenly capture the changes, the asset and liability multipliers of China's flow of funds matrix for 1998−2022 are calculated here (see Figure 10).
As seen from Figure 10, the capital ripple coefficient of China changed steadily from 1998 to 2008. There were some differences in the changes of asset multiplier and liability multiplier, but they moved in the same direction. However, since the US financial crisis in 2008, the capital ripple coefficient has shown significant changes. China's GDP growth rate was 14.7 percent in 2007 but dropped to 6.4 percent in 2019 and 2.3 percent in 2020 due to the pandemic. Along with the economic development, the ripple effect of the use or raising of funds per unit is also increasing, and the multiplier of assets and liabilities was also rising from 4.01 and 3.98 in 2008 to 19.53 and 19.86 in 2016, which shows a good policy effect. However, after 2017, the multiplier effect showed a significant decline, and the two began to deviate from 2018 to 2022. The asset multiplier was smaller than the liability multiplier. In 2022, the asset multiplier was 3.28, and the liability multiplier was 4.13, the lowest during the observation period. Use the 2022 TVET, which is in the 2022 flow of funds matrix in Table 2, to compare the 2016 TVET (RMB 98359.6 billion10), where the funding ripple coefficient peaked showing a decline of 15.3% in 2022 (see Fig. 10). As mentioned, the capital ripple coefficient is calculated by the inverse matrix coefficient of Leontief, which has nothing to do with the amount of capital. It is a combination problem of capital operation, that is, a problem of policy operation. In other words, since 2018, China has experienced an economic slowdown, but the cost of capital utilization has increased, and the effect of policy operation has decreased.
10 We calculated the TVET from 1998 until 2022 with using the flow of funds accounts that released by the People's Bank of China.
According to the above analysis, the financial sector has the strongest Influence on China's financial market and the highest degree of Sensitivity, but the Influence and Sensitivity degree of the government sector is the most unstable in the four Quadrants. Therefore, it is necessary to measure the effect of the financial sector and government sector's fund operation. When FC sectors provide money, borrow money from other sectors, increase spending, or buy assets; this is a Klein-formula (YK) typical example of capital operation. If the money is absorbed by FCs sectors and other sectors or to raise money, either by selling assets to make up for the inadequacy of funds, use Stone-formula (YS) to describe the indebted operation process. In Section 3, we derived the monetary flow matrices for coefficients CS and CK. Provided that the input coefficient CX satisfies Theorem 1 of Solow (1952), under the given conditions, the series converges to (I−CX)−1. The total value of all economic transactions resulting from the provision (absorption) of capital V as the final case θ can be estimated (see Formula 23):
ξXθ=(I−CX)−1DXV | (23) |
If the initial capital is absorbed, ξXθ is negative. Since ξXθ=ξXθ−1 is a condition of convergence, the above equation can be used whether observed from the borrowing sectors or the issuing sector.
According to Formula (24), the total amount of Net economic transactions induced by fund utilization and raising of all sectors can be calculated, which is called Net induced economic transactions (NIET), namely:
tB=(I−CK)−1DKEB−(I−CS)−1DSRB | (24) |
where, DKEB corresponding to the sector's column (assets) of the matrix YK, and DSRB corresponding to the sector's column (liabilities) of matrix YS. The NIET can reflect the economic effect brought by fund operation and preparation. The larger the NIET of a certain department, the better the economic effect of financial risk borne by the department.
Figure 11 shows the NIET of FCs sector and GG sector funds during 1998–2022. It can be seen that the changes in NIET of FCs sector capital operation are consistent with the changes of capital ripple coefficient over time. The changes were relatively stable during 1998–2006 and have fluctuated since 2008. In particular, the instability increased around 2017, especially in 2015, when it dropped sharply to RMB −29.34 trillion, and then showed a strong rebound and rose sharply to RMB 40.2 trillion in 2017. However, it continued to decline in the following years, to RMB 11.8 trillion in 2022, lower than the 2014 level, showing a trend of declining net income in the FCs sector.
The NIET brought by GG sectors through fund operation and financing was relatively stable from 1998 to 2007 but later changed greatly, from the net induced income of RMB 17.1 trillion in 2014 to the maximum net induced loss of RMB 52.8 trillion in 2020. Since 2008, GG sectors have increased the use of public funds, which reached a peak of RMB 6.59 trillion in 201511. This measure can be regarded as the GG sector's countermeasure to cope with the NIET loss of the FCs sector reaching RMB 29.3 trillion in 2015. However, the increase in GG sectors borrowing affects the balance of funding of GG sectors. From 2018 to 2022, the government borrowing exceeded RMB 6 trillion in every year, which is the state of net debt. In 2022, the maximum net debt reached RMB 8.37 trillion, that's 75 times more than in 1998.
11 PBC (2016) Financial Accounts.
The above statistical deductive analysis can answer the question of "who is bearing the risk" in China's flow of funds in the past two decades. The government took on a lot of debt risk; Its financial influence and sensitivity are the most volatile. The default risk and liquidity risk of FC sectors are largely borne by GG sectors. The results shown in Figure 11 also reflect the dual changes of the FCs and GG sectors in the time series. To further investigate the fluctuation of the net supply of funds of GG sectors, the Fluctuations in the funds propagation multiplier (FFPM) is calculated, as shown in Equation (25).
FFPM=I'tBII'EBI−I'RBI | (25) |
The numerator is the NIET volume of the sector, and the denominator is the financial net assets of the sector, that is, FFPM is the ratio of NIET to the net financial assets of the sector, indicating the multiplier of the net supply of funds. The higher the multiplier, the better the economic performance.
As shown in Figure 12, the FFPM of FC sectors showed the highest value in 2000, which was a period of good utilization efficiency of financial assets. However, after 2009, FCs sectors' net capital supply ripple multiplier declined and fluctuated. Now look at the multiplier change in the GG sector. During the observation period, the multiplier of the government was within 10, but in 2008, it reached 205, which was much higher than that of financial institutions, and then continued to decline, only 5.44 in 2022. That is to say, when the US financial crisis had a great impact on China's finances in 2007, the Klein-multiplier of GG sectors' operational capital utilization was larger than the Stone-multiplier of fundraising, resulting in structural changes, which increased the multiplier of capital supply contagion and reduced the chain infection of China's economy from the US financial crisis to a certain extent. However, after that, the multiplier of the net supply of funds by the government declined all the way, and the economic benefits brought by government debt decreased. By comparing the flow of funds matrix in 2022 with that in 2016, when the capital spread coefficient reached its peak (see Table 3 and Figure 10), it can be seen that GG sectors' use of funds for HHs, NFCs, and FCs decreased by 90.67%, 96.1%, and 66.3%, respectively, while there was an increase of 163% to the ROW sector. Among them, the 163% increase in the use of ROW funds is mainly due to the purchase of US Treasuries to adjust the trade imbalance between China and the United States, and China's holdings of US Treasuries at the end of 2022 were 867.1 billion US dollars12. It can be seen that the China-US trade imbalance also impacts China's domestic flow of funds structure.
12 U.S. DEPARTMENT OF THE TREASURY, https://home.treasury.gov/data/treasury-international-capital-tic-system.
On the other hand, the funds raised by the GG sector from HHs, NFCs, FCs, and ROW sectors increased by 131%, 212%, 18.3%, and 67.1%, respectively, indicating the increasing risk of the GG sector's indebtedness to various sectors and the capital structure problems caused by excessive reliance on debt. Since the ripple multiplier of funds reflects the spillover effect of the supply and demand of funds, it should be closely watched, considering the risk structure of the asset side and the liability side. Faced with the changes in economic fundamentals since 2018, the impact of the pandemic in 2020, and the drastic changes in the international environment, the policy effect should not only consider the scale of government-led capital supply but also depend on the size of the multiplier effect of capital supply.
We conduct a long-term statistical analysis of the flow of funds within China's economy. By examining the trends and structural relationships in capital utilization and financing, we employ statistical inference through triangular sorting, calculate PDI and SDI indices, conduct multiplier analysis of fund supply effects, and evaluate the net induced economic transactions from fund utilization and raising so we can know that the greatest risk to China's economy remains within the financial sector. It is essential to mitigate financial risks by addressing issues in both the real economy and the financial sector while adjusting the economic growth model.
Descriptive statistical analysis reveals the characteristics of capital flow across sectors during various historical stages from 1992 to 2022. Although the US financial crisis around 2008 had an impact, capital flows across all sectors demonstrated positive changes due to appropriate policy responses. The capital surplus of the HHs sector rebounded, the investment demand of the NFCs sector increased, and the capital surplus of the GG sector stabilized. Additionally, China's net external financial assets showed a consistent upward trend. However, since 2017, the capital demand of the NFC sector has exhibited a continuous decline. In recent years, the widening government funding gap and the reduction in China's external financial investments indicate that, amid significant changes in the international environment, China's economy is facing a new and more challenging situation.
The major challenges are as follows. Based on the analysis of the industrial flow of funds, it is evident that after 2017, the gap in HH's disposable income widened, and the consumption growth slowed. This has undermined the foundation for expanding domestic demand, leading to a structural imbalance between savings and investment. These trends highlight the limitations of an investment-driven growth model accompanied by high savings. From observing the financial flow of funds, the greatest risk facing China's economy lies in the financial sector. Since 2017, the decline of the triangulation between FCs and NFCs, coupled with the rapid rise in the triangulation involving part of the GG, indicates that FCs have allocated a significant amount of funds to the GG. This reflects structural issues in the allocation and mobilization of funds.
The financial crisis stems from the accumulation of debt and liquidity risks. By constructing a W-t-W matrix table, the temporal sequence and causal relationship of each sector's role in the fund operations are examined. From a stock perspective, a stable hierarchical relationship exists among the sectors. HHs have consistently been at the base of the triangle, serving as the primary providers of capital, while NFCs occupy the top of the triangle, acting as the main users of capital. After 2017, the ROW exhibited new changes, with China's external flow of funds experiencing a relative contraction. FCs are positioned in the middle of the triangulation, but the role of financial intermediation in the financial flow of funds remains underdeveloped. The GG has shown the most volatile in the triangulation and has risen to the highest level since 2017, accumulating significant amounts of debt. This indicates that the GG is increasingly taking on and pricing in debt default risk within financial markets.
During the economic boom period from 2006 to 2016, the government served as the primary capital provider. However, since 2017, as the economy faced downward pressure, the government has significantly increased its debt levels. It has raised funds from banks through new project financing mechanisms, such as government procurement of service and special construction funds, including Public-Private Partnership (PPP) projects. As a result, the stocks of local government debt have expanded rapidly, further escalating the risk of implicit government debt. According to the asset-liability matrix, over 90% of the GG's debts are held by FCs. This concentration creates the potential for mutual spillover effects between government debt risks and financial sector risks. The growing government debt problem not only undermines the government's ability to deliver public services but also contributes to the accumulation of systemic financial risks.
The time series of PDI and SDI for each sector reflects the structural changes in China's financial assets and liabilities, as well as the outcomes of policy intentions. In both the flow matrix and the stock matrix, the FC sector demonstrates the strongest influence and sensitivity. In contrast, the PDI and SDI of the GG sector are the most unstable, consistently falling below the average level. Since 2015, SDI has decreased, indicating a reduced sensitivity to the overall capital supply in society. When combined with the effect of fund operation and financing, it becomes evident that the GG sector's fund operations and financing have played a positive role in stabilizing the financial market. However, the multiplier of the GG sector's net fund supply has been declining since 2017, suggesting that the economic benefits derived from the government's fiscal measures have diminished. Consequently, the contribution of government debt to the economy has declined since 2017, even as the associated risks have increased.
The government's role in a market economy is not to frequently intervene and stimulate the economy but to continuously improve the system and mechanisms of the market economy. This includes developing market infrastructure, addressing weaknesses, eliminating bottlenecks, and facilitating smooth transitions. The GG sector should be the planners of the development direction, the makers of policies, the stabilizer, and regulators of macroeconomic operations than a direct risk taker. The essence of finance lies in resource optimization and risk diversification. Therefore, the conclusion of the analysis should be that financial institutions are responsible for bearing risks. However, based on the statistical test and analysis above, the FCs sector has not fully fulfilled its role in mediating, regulating, and controlling the market in accordance with this responsibility. According to the flow of funds statistics from 1992 to 2022, the FC sector consistently holds the largest share of financial assets, exceeding 50% in most years, with the exception of approximately 40% in 1998 and 2000, and even reaching 60% in 2014–2016. Furthermore, the assets and liabilities of the FC sector exhibit the strongest degree of influence, and both the PDIKandSDIS of the FCs have continued to rise in recent years. This indicates that the influence of capital supply and the sensitivity of capital raising have increased, with the potential default risk of various sectors becoming notably concentrated in financial institutions. As a result, the FCs sectors should possess the capability to independently manage risks. FCs must regulate the rational allocation of financial resources through market mechanisms, strictly adhering to relevant laws and regulations.
Several challenges remain in studying financial risk and security using the W-t-W table. First, there is a need to enrich the data source. Currently, the People's Bank of China has published the flow data from 1992 to 2022 and the stock data from 2017 to 2022. However, compared to the statistics of advanced economies, China's capital flow statistics require further refinement. At present, the sector classifications and transaction items are overly simplistic, limiting their usefulness for in-depth analysis. If financial institutions could be further categorized—for example, into central banks, deposit-taking institutions, insurance and social security funds, securities investment trusts, and public financial institutions—structural issues could be examined at a deeper level, significantly enhancing the ability to monitor financial risks. Additionally, the time lag in data release is excessive (with a 3-year delay for physical transaction data and a 1-year delay for financial transaction data). Notably, the quarterly statistical reports for flow data have yet to be released, which hinders the timely reflection of market fluctuations.
Second, theories and methods from statistics, economics, econometrics, data science, financial engineering, and other relevant disciplines should be integrated to study the mechanisms driving changes in both the domestic and global flow of funds. This integration will help establish a systematic financial risk monitoring system. In this study, financial transactions and asset-liability positions across sectors are primarily used as the basis for monitoring financial risks. However, the emergence of financial risks is not solely attributable to financial transactions but is also closely linked to the changes in the real economy and international capital flows. Therefore, it is essential to accelerate and enhance the data collection system, including the integration of AI technologies, establish a statistical monitoring system that aligns with the level of risk management, and develop the capability to strengthen the prevention and resolution of financial risks.
The authors declare they have not used Artificial Intelligence (AI) tools in the creation of this article.
The authors are grateful to the anonymous referees for their valuable comments and helpful suggestions. An earlier version of this paper was presented at the Society for Economic Statistics 2023 Annual Research Conference, the Macroeconomic Seminar at the National School of Development, Peking University (September 15, 2023), the Kobe University Finance Research Institute (October 21, 2023), and the 81st Annual Meeting of the Japan Association for International Economics (October 2, 2022). The authors would like to express their sincere gratitude to Mr. Hagino Matoko (Director of the Statistics Commission Office, Ministry of Internal Affairs and Communications; Senior Fellow at the Cabinet Office of Japan), Professor Takayama Ozuo (Fukuyama University), Professor Harry Wu (Peking University), Professor Changhua Yu (Peking University), Professor Yoichi Matubayashi, and Professor Takekazu Iwamoto (Kyoto University) for their insightful and constructive feedback. The authors also extend their thanks to the conference hosts and discussants for their valuable suggestions and advice. All remaining errors are solely the responsibility of the authors.
This work was supported by the grants-in-aid for scientific research (Japan): [Grant Number Scientific Research (C), 20K01701], and the National Social Science Foundation of China (No. 21BTJ015).
All authors declare no conflicts of interest in this paper.
[1] | Al-Haschimi A, Spita T (2024) The evolution of China's growth model: challenges and long-term growth prospects. Economic Bulletin Articles. |
[2] | Copeland MA (1947) Tracing Moneyflows through the United States Economy. Am Econ Rev 37: 31-49. |
[3] | Copeland MA (1949) Social Accounting for Moneyflows. Account Rev 24: 254-264. |
[4] | Copeland MA (1952) A Study of Moneyflows in the United States, Newyork: NBER Books. |
[5] | Dawson JC (1996) Flow-of-funds Analysis: A Handbook for Practitioners, Landon: M.E. Sharpe. |
[6] |
Bekkers E, Koopman RB, Rêgo CL (2021) Structural change in the Chinese economy and changing trade relations with the world. China Econ Rev 65: 101573. https://doi.org/10.1016/j.chieco.2020.101573 doi: 10.1016/j.chieco.2020.101573
![]() |
[7] | Financial Stability Board, International Monetary Fund (2009) The Financial Crisis and Information Gaps. Report to the G-20 Finance Ministers and Central Bank Governors. Available from: http://www.imf.org/external/np/g20/pdf/102909.pdf. |
[8] | Gong X, Bian J (2010) The mechanism of inter-sectoral contagion in China's macro-finance. Econ Res J 45: 79-89. |
[9] | Hu QY (2010) Input-output capital flow statement and capital correlation model. J Quant Tech Econ 27: 133–146. |
[10] | Klein LR (1983) Lectures in Econometrics, Amsterdam: North-Holland, 35–44. |
[11] | Leontief WW (1953) Structural change, In: Leontief, W. (Ed.), Studies in the structure of the American economy (pp. 17–52). New York: Oxford University Press, Chapter 2. Domestic Production and Foreign Trade; The American Capital Position Re-Examined. |
[12] |
Leontief WW (1963) The structure of development. Sci Am 209: 148–166. https://doi.org/10.1038/scientificamerican0963-148 doi: 10.1038/scientificamerican0963-148
![]() |
[13] | Li Y (1998) Flow of Funds in China's opening up process. Econ Res J 33: 14-24. |
[14] | Li Y, Yin J (2007) Anatomy of High Savings Rate of China: Analysis Based upon Flow of Funds Account of China from 1992 to 2003. Econ Res J 42: 14-26. |
[15] | Li Y, Zhang YJ (2020) China's National Balance Sheet 2020, China Social Sciences Press. |
[16] | National Bureau of Statistics of China (2023) China Statistical Yearbook 2022, China statistics press. |
[17] | Rasmussen PN (1956) Studies in inter-sectoral relations, EinarHarcks Forlag. |
[18] | Shi Y (2023) Is China financialised? The significance of two historic transformations of Chinese finance. New Polit Econ 29: 305–320. |
[19] | Solow RM (1952) On the Structure of Linear Models. Econometrica 20: 29–46. |
[20] |
Stone R (1966) The Social Accounts from a Consumer's Point of View. Review Income Wealth 12: 1–33. https://doi.org/10.1111/j.1475-4991.1966.tb00709.x doi: 10.1111/j.1475-4991.1966.tb00709.x
![]() |
[21] | Taylor SP (1996) From Money-flows Accounts to Flow-of-funds Accounts, A paper prepared for the annual meeting of the American Statistical Association, 1991. Printed in: Dawson, J.C. (Ed.), Flow-of-funds analysis: A handbook for practitioners. M. E. Sharp, pp. 101–108. |
[22] | Tsujimura K, Mizoshita M (2002) Flow of funds Analysis: Fundamental Technique and Policy Evaluation, Keio University Press (in Japanese): 30–49, 63–98. |
[23] |
Tsujimura K, Tsujimura M (2018) A Flow of Funds Analysis of the US Quantitative Easing. Econ Syst Res 30: 137–177. https://doi.org/10.1080/09535314.2018.1443908 doi: 10.1080/09535314.2018.1443908
![]() |
[24] |
Tsujimura M, Tsujimura K (2020) Flow-of-Funds Structure of the U.S. Economy 2001–2018. Econ Syst Res 33: 385–416. https://doi.org/10.1080/09535314.2020.1795629 doi: 10.1080/09535314.2020.1795629
![]() |
[25] | Vitor Gaspar (2023) Transcript of the 2023 Fiscal Monitor Press Briefing. Available from: https://www.imf.org/en/News/Articles/2023/04/13/tr041223-fm-press-briefing-transcript. |
[26] | Wen J, Ruan J (2006) The report of China's Flow of Funds Analysis. China finance, 31–33. |
[27] | Winkler B, Riet AV, Bull P (2013a) Financial Intermediary Balance Sheet Management, A Flow of Funds Perspective on the Financial Crisis: Volume Ⅰ: Money, Credit and Sectors Balance Sheets, London: Palgrave Macmillan, 177–202. |
[28] | de Rougemont P, Winkler B (2014) The Financial Crisis in the Light of the Euro Area Accounts: Selected Issues, In: Winkler, B., Riet, A.V., Bull, P., A Flow of Funds Perspective on the Financial Crisis: Volume Ⅱ: Macroeconomic Imbalances and Risks to Financial Stability, London: Palgrave Macmillan, 155-198. https://doi.org/10.1057/9781137353016_6 |
[29] | Yi G (2020) Revisiting China's financial asset structure and policy implications. Econ Res J 55: 4-17. |
[30] | Zhang N (2005) The theory and practice of global flow of funds (in Japanese), Minerva Shobo Inc. (in Japanese), 75-99. |
[31] |
Zhang N, Zhao X (2019) Measuring Global Flow of Funds: A Case Study on China, Japan and the United States. Econ Syst Res 31: 520–550. https://doi.org/10.1080/09535314.2019.1574719 doi: 10.1080/09535314.2019.1574719
![]() |
[32] | Zhang N (2020) Statistical Observations on the Flow of Funds of China: 1992–2017. In: Flow of Funds Analysis: Innovation and Development, Springer, Singapore, 61-88. https://doi.org/10.1007/978-981-15-7720-8_2 |
[33] |
Zhang N, Zhu L (2021) Global Flow of Funds as a Network: The Case Study of the G20. Jpn J Monetary Financ Econ 9: 21-56. 2021. https://doi.org/10.32184/jjmfe.9.0_21 doi: 10.32184/jjmfe.9.0_21
![]() |
[34] | Zhang N (2022) Measuring Global Flow of Funds: who-to-whom matrix and financial network, Jpn J Stat Data Sci 5: 899-942. https://link.springer.com/article/10.1007/s42081-022-00175-x |
Households | Enterprises Sector | Government | ||||||
propensity to save | Disposable Income ratio | propensity to save | Disposable Income ratio | propensity to save | Disposable Income ratio | |||
1992–1999 | 30.40 | 66.80 | 100 | 20.13 | 25.52 | 13.07 | ||
2000–2006 | 35.39 | 59.46 | 100 | 23.36 | 11.00 | 17.18 | ||
2007–2012 | 42.46 | 56.34 | 100 | 23.55 | 26.85 | 20.11 | ||
2013–2017 | 38.49 | 58.77 | 100 | 16.55 | 21.10 | 20.33 | ||
2018–2022 | 36.39 | 60.47 | 100 | 22.74 | 1.60 | 16.79 | ||
Source: National Bureau of Statistics of China, Flow of Funds Account (Non-financial transaction). Notes: Since the savings of the nonfinancial enterprise sector are equivalent to its disposable income, the sector's propensity to save is 100%. Propensity to save = sector's savings/sector's disposable income; Disposable income ratio = sector's disposable income/total disposable income. |
n sectors | m financial claims | Real asset/accumulated saving | Row totals | |
n sectors | Y | Ajk | ej | wj |
m financial claims | L'jk | X | lk | |
Real asset/ accumulated saving | z'j | ξ | ||
Column totals | x'j | a'k | ε | |
Source: R. Stone, "The Social Accounts from a Consumer's Point of View", pp.19−24. |
YS | |||||||
HHs | NFCs | FCs | GG | ROW | ε | Yi | |
HHs | 61 | 3225 | 222786 | 17909 | 1372 | 0 | 245354 |
NFCs | 1073 | 12720 | 70846 | 2220 | 12317 | 99323 | 198499 |
FCs | 54814 | 173806 | 88306 | 83265 | 33889 | 0 | 434081 |
GG | 128 | 610 | 18221 | 6 | 3907 | 83705 | 106577 |
ROW | 58 | 8137 | 12300 | 3176 | 7763 | 27813 | 59247 |
ρ | 189220 | 0 | 21621 | 0 | 0 | TVFT | |
Yj | 245354 | 198499 | 434081 | 106577 | 59247 | 832917 | |
YK | |||||||
HHs | NFCs | FCs | GG | ROW | ρ | Yi | |
HHs | 61 | 1073 | 54814 | 128 | 58 | 189220 | 245354 |
NFCs | 3225 | 12721 | 173805 | 610 | 8137 | 0 | 198499 |
FCs | 222786 | 70846 | 88306 | 18221 | 12301 | 21618 | 434079 |
GG | 17909 | 2220 | 83265 | 6 | 3176 | 0 | 106577 |
ROW | 1372 | 12317 | 33888 | 3906 | 7763 | 0 | 59246 |
YS | |||||||
HHs | NFCs | FCs | GG | ROW | ε | Yi | |
HHs | 5727 | 210443 | 1965090 | 121419 | 27158 | 0 | 2329837 |
NFCs | 1894 | 408839 | 1125760 | 7548 | 133729 | 1056509 | 2734278 |
FCs | 843316 | 1885021 | 1503634 | 683060 | 334788 | 0 | 5249819 |
GG | 219 | 22668 | 402803 | 149 | 1989 | 408844 | 836671 |
ROW | 130 | 207308 | 89433 | 24496 | 147119 | 176297 | 644783 |
ρ | 1478551 | 0 | 163099 | 0 | 0 | TVFT | |
Yj | 2329837 | 2734278 | 5249819 | 836671 | 644783 | 10153738 | |
YK | |||||||
HHs | NFCs | FCs | GG | ROW | ρ | Yi | |
HHs | 5727 | 1894 | 843316 | 219 | 130 | 1478551 | 2329837 |
NFCs | 210443 | 408839 | 1885021 | 22668 | 207308 | 0 | 2734279 |
FCs | 1965090 | 1125760 | 1503632 | 402803 | 89433 | 163099 | 5249817 |
GG | 121419 | 7548 | 683060 | 149 | 24496 | 0 | 836671 |
ROW | 27158 | 133729 | 334788 | 1989 | 147119 | 0 | 644783 |
Source: People's Bank of China (2022) Flow of Funds Statement (Financial Accounts); Financial Assets and Liabilities Statement (Financial Accounts). Note: The total value of the economic transactions (TVET) in the bottom-right corner of each table indicates the total value of economic transactions between sectors (including the rest of the world). |
Households | Enterprises Sector | Government | ||||||
propensity to save | Disposable Income ratio | propensity to save | Disposable Income ratio | propensity to save | Disposable Income ratio | |||
1992–1999 | 30.40 | 66.80 | 100 | 20.13 | 25.52 | 13.07 | ||
2000–2006 | 35.39 | 59.46 | 100 | 23.36 | 11.00 | 17.18 | ||
2007–2012 | 42.46 | 56.34 | 100 | 23.55 | 26.85 | 20.11 | ||
2013–2017 | 38.49 | 58.77 | 100 | 16.55 | 21.10 | 20.33 | ||
2018–2022 | 36.39 | 60.47 | 100 | 22.74 | 1.60 | 16.79 | ||
Source: National Bureau of Statistics of China, Flow of Funds Account (Non-financial transaction). Notes: Since the savings of the nonfinancial enterprise sector are equivalent to its disposable income, the sector's propensity to save is 100%. Propensity to save = sector's savings/sector's disposable income; Disposable income ratio = sector's disposable income/total disposable income. |
n sectors | m financial claims | Real asset/accumulated saving | Row totals | |
n sectors | Y | Ajk | ej | wj |
m financial claims | L'jk | X | lk | |
Real asset/ accumulated saving | z'j | ξ | ||
Column totals | x'j | a'k | ε | |
Source: R. Stone, "The Social Accounts from a Consumer's Point of View", pp.19−24. |
YS | |||||||
HHs | NFCs | FCs | GG | ROW | ε | Yi | |
HHs | 61 | 3225 | 222786 | 17909 | 1372 | 0 | 245354 |
NFCs | 1073 | 12720 | 70846 | 2220 | 12317 | 99323 | 198499 |
FCs | 54814 | 173806 | 88306 | 83265 | 33889 | 0 | 434081 |
GG | 128 | 610 | 18221 | 6 | 3907 | 83705 | 106577 |
ROW | 58 | 8137 | 12300 | 3176 | 7763 | 27813 | 59247 |
ρ | 189220 | 0 | 21621 | 0 | 0 | TVFT | |
Yj | 245354 | 198499 | 434081 | 106577 | 59247 | 832917 | |
YK | |||||||
HHs | NFCs | FCs | GG | ROW | ρ | Yi | |
HHs | 61 | 1073 | 54814 | 128 | 58 | 189220 | 245354 |
NFCs | 3225 | 12721 | 173805 | 610 | 8137 | 0 | 198499 |
FCs | 222786 | 70846 | 88306 | 18221 | 12301 | 21618 | 434079 |
GG | 17909 | 2220 | 83265 | 6 | 3176 | 0 | 106577 |
ROW | 1372 | 12317 | 33888 | 3906 | 7763 | 0 | 59246 |
YS | |||||||
HHs | NFCs | FCs | GG | ROW | ε | Yi | |
HHs | 5727 | 210443 | 1965090 | 121419 | 27158 | 0 | 2329837 |
NFCs | 1894 | 408839 | 1125760 | 7548 | 133729 | 1056509 | 2734278 |
FCs | 843316 | 1885021 | 1503634 | 683060 | 334788 | 0 | 5249819 |
GG | 219 | 22668 | 402803 | 149 | 1989 | 408844 | 836671 |
ROW | 130 | 207308 | 89433 | 24496 | 147119 | 176297 | 644783 |
ρ | 1478551 | 0 | 163099 | 0 | 0 | TVFT | |
Yj | 2329837 | 2734278 | 5249819 | 836671 | 644783 | 10153738 | |
YK | |||||||
HHs | NFCs | FCs | GG | ROW | ρ | Yi | |
HHs | 5727 | 1894 | 843316 | 219 | 130 | 1478551 | 2329837 |
NFCs | 210443 | 408839 | 1885021 | 22668 | 207308 | 0 | 2734279 |
FCs | 1965090 | 1125760 | 1503632 | 402803 | 89433 | 163099 | 5249817 |
GG | 121419 | 7548 | 683060 | 149 | 24496 | 0 | 836671 |
ROW | 27158 | 133729 | 334788 | 1989 | 147119 | 0 | 644783 |
Source: People's Bank of China (2022) Flow of Funds Statement (Financial Accounts); Financial Assets and Liabilities Statement (Financial Accounts). Note: The total value of the economic transactions (TVET) in the bottom-right corner of each table indicates the total value of economic transactions between sectors (including the rest of the world). |