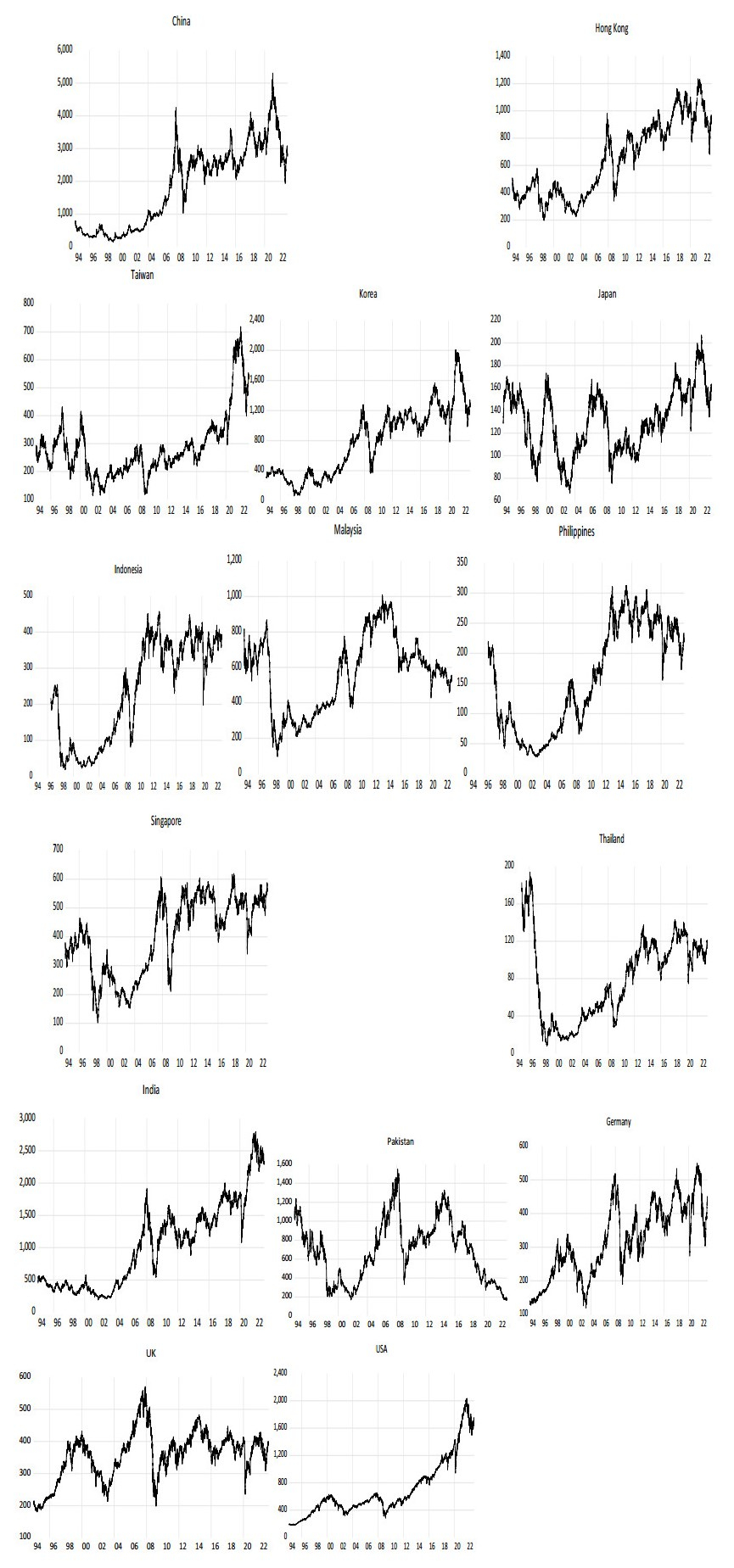
We used the quantile vector autoregressive (QVAR) dynamic connectedness framework to examine whether leading stock markets in America and Europe would have any impact on major stock markets in Asia.1 More precisely, we analyzed systematically the stock market connectedness in 15 countries, namely Germany, the UK, the USA, and 12 Asian countries, which include five major ASEAN countries, namely Indonesia, Malaysia, Philippines, Singapore, and Thailand from 1996 to 2023. The findings indicated that Hong Kong and Singaporean stocks were major transmitters of financial shocks at the extreme low price market condition, while Germany and UK were minor transmitters. By contrast, the USA could be considered the major transmitter of financial shock during the extreme high market price returns condition. In the normal market condition, these three countries in Europe and America are important transmitters of financial shock. More interestingly, the empirical findings indicated the centrality of Singapore in the stock market connectedness in Asia.
1 The authors are grateful to Professor David Gabauer who makes available the R codes for all calculations in this paper.
Citation: OlaOluwa S. Yaya, Miao Zhang, Han Xi, Fumitaka Furuoka. How do leading stock markets in America and Europe connect to Asian stock markets? Quantile dynamic connectedness[J]. Quantitative Finance and Economics, 2024, 8(3): 502-531. doi: 10.3934/QFE.2024019
[1] | Mohammad Ashraful Ferdous Chowdhury, M. Kabir Hassan, Mohammad Abdullah, Md Mofazzal Hossain . Geopolitical risk transmission dynamics to commodity, stock, and energy markets. Quantitative Finance and Economics, 2025, 9(1): 76-99. doi: 10.3934/QFE.2025003 |
[2] | Samuel Kwaku Agyei, Ahmed Bossman . Investor sentiment and the interdependence structure of GIIPS stock market returns: A multiscale approach. Quantitative Finance and Economics, 2023, 7(1): 87-116. doi: 10.3934/QFE.2023005 |
[3] | Korhan Gokmenoglu, Baris Memduh Eren, Siamand Hesami . Exchange rates and stock markets in emerging economies: new evidence using the Quantile-on-Quantile approach. Quantitative Finance and Economics, 2021, 5(1): 94-110. doi: 10.3934/QFE.2021005 |
[4] | Emmanuel Assifuah-Nunoo, Peterson Owusu Junior, Anokye Mohammed Adam, Ahmed Bossman . Assessing the safe haven properties of oil in African stock markets amid the COVID-19 pandemic: a quantile regression analysis. Quantitative Finance and Economics, 2022, 6(2): 244-269. doi: 10.3934/QFE.2022011 |
[5] | Chikashi Tsuji . The historical transition of return transmission, volatility spillovers, and dynamic conditional correlations: A fresh perspective and new evidence from the US, UK, and Japanese stock markets. Quantitative Finance and Economics, 2024, 8(2): 410-436. doi: 10.3934/QFE.2024016 |
[6] | Lorna Katusiime . Time-Frequency connectedness between developing countries in the COVID-19 pandemic: The case of East Africa. Quantitative Finance and Economics, 2022, 6(4): 722-748. doi: 10.3934/QFE.2022032 |
[7] | Isaac O. Ajao, Hammed A. Olayinka, Moruf A. Olugbode, OlaOluwa S. Yaya, Olanrewaju I. Shittu . Long memory cointegration and dynamic connectedness of volatility in US dollar exchange rates, with FOREX portfolio investment strategy. Quantitative Finance and Economics, 2023, 7(4): 646-664. doi: 10.3934/QFE.2023031 |
[8] | Kim Hiang Liow, Jeongseop Song, Xiaoxia Zhou . Volatility connectedness and market dependence across major financial markets in China economy. Quantitative Finance and Economics, 2021, 5(3): 397-420. doi: 10.3934/QFE.2021018 |
[9] | Andrey Kudryavtsev . Absolute Stock Returns and Trading Volumes: Psychological Insights. Quantitative Finance and Economics, 2017, 1(2): 186-204. doi: 10.3934/QFE.2017.2.186 |
[10] | Raéf Bahrini, Assaf Filfilan . Impact of the novel coronavirus on stock market returns: evidence from GCC countries. Quantitative Finance and Economics, 2020, 4(4): 640-652. doi: 10.3934/QFE.2020029 |
We used the quantile vector autoregressive (QVAR) dynamic connectedness framework to examine whether leading stock markets in America and Europe would have any impact on major stock markets in Asia.1 More precisely, we analyzed systematically the stock market connectedness in 15 countries, namely Germany, the UK, the USA, and 12 Asian countries, which include five major ASEAN countries, namely Indonesia, Malaysia, Philippines, Singapore, and Thailand from 1996 to 2023. The findings indicated that Hong Kong and Singaporean stocks were major transmitters of financial shocks at the extreme low price market condition, while Germany and UK were minor transmitters. By contrast, the USA could be considered the major transmitter of financial shock during the extreme high market price returns condition. In the normal market condition, these three countries in Europe and America are important transmitters of financial shock. More interestingly, the empirical findings indicated the centrality of Singapore in the stock market connectedness in Asia.
1 The authors are grateful to Professor David Gabauer who makes available the R codes for all calculations in this paper.
With rapid growth, the Asian economy has established a prominent position in the global capital market. As of April 2023, China had the largest market in Asia with a capitalization of $12.3 trillion. Japan and Hong Kong also had substantial capital markets, with market capitalizations of $5.598 trillion and $4.557 trillion, respectively. The other economies with significant market capitalizations were India ($3.321 trillion), South Korea ($1.779 trillion), Singapore (624.159 billion), Thailand ($549.157 billion), Indonesia ($633.023 billion), Malaysia ($361.157 billion), the Philippines ($301.137 billion), and Pakistan ($22.156 billion) (CEIC, 2024). Globally, China was ranked second after the U.S., which has a market capitalization of $40.7 trillion. Japan and Hong Kong's markets were the next largest, whilst the U.K. market capitalization accounted for $2.971 trillion (CEIC, 2024). With their high capitalization rates, China, Japan, Hong Kong, and South Korea are commonly identified as highly established Asian economies with significant potential for investment. Singapore, Thailand, Indonesia, and Malaysia could be considered medium-capitalized economies, while the Philippines and Pakistan are considered relatively low-capitalized ones.
Although Asian stock markets have become a powerful force in the global capital market, their self-reliance and decoupling potential from advanced economies remain to be explored. In the era of financial integration, many countries have formed close interconnections with them. From 1996 to 1998, international speculators successively attacked the stock markets in Thailand, the Philippines, Malaysia, and Hong Kong (HK). Countries including Japan and South Korea also experienced a decline in stock prices, while Russia defaulted on its debt repayments. In 2007, the global financial crisis was triggered by the subprime mortgage crisis in the United States (US), which quickly spread to Europe affecting countries and regions around the world. In March and April 2020, despite the U.S. Federal Reserve's sharp interest rate cut of 150 basis points, it failed to stop the continued plummet of the US stock market, which fell by nearly 32%. European and Asian stock markets also experienced sharp declines in their stock indices, with a drop of 12.8% in the FTSE (Financial Times Stock Exchange) 100 index in March, 10.5% in the Nikkei 225 in Japan, and approximately 4.5% in the Shanghai Composite Index (CEIC, 2024). Stock markets around the world suffered huge losses during the pandemic (Baig et al., 2021; Cepoi, 2020).
Against the background of internalization and global interdependency, research on the connectedness of stock markets is of paramount importance for formulating monetary and financial policies at the macroeconomic level and maintaining financial stability across regions and countries. Moreover, the dynamic econometric method is in its infancy stage. Generally, authors employ it to investigate the spillover effects and deepness of connectedness (via connectedness index and connectedness plot) among variables of interests. In the case of stocks, the deepness of network of connectedness at the centre imply high risk of stocks returns and volatility spillovers (Gong et al., 2019). Further, connectedness is also a function of risk perceptions of investors as it measures investors' risk perceptions in markets; as in the case of the US and global stock market integration (Serrano et al., 2023). This is of particular significance for individuals and institutional investors who look for diversifying global financial assets through risk management activities. Such academic endeavor is much needed in Asia.
The financial market structure in Asia is complex, encompassing developed countries such as Japan, South Korea, and Singapore, and emerging economies such as China and Malaysia, among others. Apart from its complexities, market size also speaks of the significance of this study. In 2021, the Asian capital market accounted for approximately 30% of the world capital market, making it the largest regional market globally.
Therefore, we aim to investigate the extent to which leading stocks from the US and Europe are connected with those in Asia. Furthermore, it endeavours to analyse the contagions of global and regional stock markets, as has been previously explored by Mensi et al. (2018). To be explicit, it adopts the Quantile Vector Autoregressive (QVAR) dynamic connectedness framework of Chatziantoniou et al. (2021) with the corrected total connectedness index, as given in Chatziantoniou and Gabauer (2021).2 In their methodological framework, their findings are based on the reduced form quantile VAR, which is a purely "econometric" model than a "theoretical" model. In other words, their findings could not be used for the economic interpretation, such as a "causal" narrative of the spillover. Schüler (2014) and Chavleishvili and Manganelli (2024) suggested to estimate the structural model by using the Cholesky decomposition method to decompose the estimated covariance matrix. For the estimation of structural model, they imposed some structural identification assumptions that endogenous variables can react contemporaneously to exogenous variables, but only lagged value of the exogenous variables could react to the endogenous variable. These identification assumptions may have some implications for the findings if the analysis of structural model is estimated (Schüler, 2014; Chavleishvili and Manganelli, 2024).3
2 Ando et al. (2022) propose a similar quantile connectedness method which is based on common factor error structure instead of the Generalized Forecasts Error Variance Decomposition (GFEVD).
3 The authors are grateful to anonymous referee' suggestion for referring to the difference between reduced form model and structural model.
We use the Time-Varying Parameter VAR (TVP-VAR) model to estimate the parameters of interest (Antonakakis et al., 2020; Furuoka et al., 2023). The TVP-VAR is a modified version of the dynamic connectedness estimation which is based on the rolling-window VAR framework (Diebold and Yılmaz, 2014). Another methodological advantage of estimation in this paper is to use the quantile connectedness approach, which is suggested in Chatziantoniou et al. (2021) for the parameter estimation. This method could be used to more nuanced estimation of parameters by taking account of different level of quantile in the price level (i.e., high price market vs low price market).
By so doing, we enable the investigation of volatility transmission across high and low volatility regimes, that is, between the market in extreme low and high market price returns conditions. Given global stock markets are increasingly integrated, the quantile dynamic connectedness offers an improvement over the original Diebold and Yilmaz (2009, 2012, 2014) general forecast variance decomposition for the normalization technique in the forecast variance decomposition. We found interesting results, relevant for stock market participants and policy makers interested in international finance. The roles of European and American stocks transmission are dominant, particularly the US stock market. Also, among the Asian stocks, Singaporean stock acted as the strongest stock market.
The econometric approach is invariant to different connectedness methods employed so far. This is a parametric approach, while many papers have combined both non-parametric and parametric methods in establishing their findings. For example, Marfatia (2017) combined the features of parametric and nonparametric methods in investigating inter-linkages of volatility spillovers across 22 leading global stocks by relying on the wavelet techniques with time-varying conditional volatility to study the comovement of risks at the country level and regional level. Other methods of connectedness are the cross-quantile dependence applied in suggested by Dai et al. (2022), the quantile cross-spectral coherence method using quantile spectral coherency model, cross-quantilogram correlation approach, windowed time-lagged cross-correlation, and windowed scalogram difference models applied in Tiwari et al. (2022).
The contribution of this paper is threefold. First, we uncover the dynamic connectedness of stock market activities between advanced economies and 12 Asian markets, covering three sub-regions of Asia, namely Southeast Asia, Northeast Asia, and South Asia. Second, the quantile dynamic connectedness approach sheds light on the behavior of markets in extremely low and high market price conditions. To the best of our knowledge, we are the first to use the quantile-VAR to investigate these aspects in a unified framework by using empirical evidence of Asian stock markets. Third, there is a little agreement on the stock market contagion (Iwanicz-Drozdowska et al., 2021). In other words, there is ongoing debate the direction of the stock market contagion among different regions. Some studies indicated that the contagion effects from the large market, such as US stock market, to the smaller market, such as Asian market (Lin et al., 1994; Yang and Zhou, 2017). Other researchers have showed the contagion from the opposite direction (Wang and Wang, 2010; Samarakoon, 2011). We contributed to this debate by offering new insights from the quantile connectedness method. More precisely, at the upper extreme quantile condition (τ=0.95), the major transmitters of financial contagion are relatively larger market, such as US market. However, at the lower extreme condition (τ=0.05), relatively smaller markets, such as Singapore and HK markets, could be considered the major transmitters.
The remainder of this study is organized as follows. In Section 2, we provide the theoretical perspective and the empirical literature on stock markets connectedness around the world and in Asia. In Section 3, we present the quantile Vector Autoregression (VAR) framework. In Section 4, we present empirical results with a robustness check using the Time-Varying Parameter VAR (TVP-VAR) model. In Section 5, we conclude the paper.
(a) The contagion effect
The most common channel of financial shock could be the stock market prices. In the highly integrated financial market, changes in prices in a stock market would be translated into changes in prices in another stock market. This channel of stock market prices could be operated through investors' reallocation of their funds to different stock markets. This means that this type of financial flow from one country to another country may not be determined by the macroeconomic foundation. The transmission of financial shock, without depending on macroeconomic factors, is often called the "contagion effect" (Agénor and da Silva, 2022).
There is no exact definition of the "contagion" effect. However, the "contagion" effect could be defined as a significant increase in the cross-country linkage caused by the transmission of financial shock from one country to another country. This concept could be better understood by comparing it with the "interdependence" effect or the "fundamental-based" effect. The "interdependence" effect could be considered a "rational" effect caused by a normal level of financial transmission from one country to another. By contrast, the "contagion" effect could be considered an "irrational" effect caused by the extremely high or excessive transmission of financial shocks that are often not based on any macroeconomic foundation (Dornbusch et al., 2000; Korkmaz, 2014; Agénor and da Silva, 2022).
(b) Financial contagion around the world
There are some major examples of financial contagion in Latin America, Southeast Asia, East Asia, Russia, and Brazil. For example, the debt crisis began in Mexico in 1982 and the crisis has spread to other Latin American countries. In 1994, the currency crisis spread over the same region. Another financial crisis began in Thailand in July 1997 and the financial shocks spread to its neighboring countries in Southeast Asian countries, such as Malaysia, the Philippines, and Indonesia. This financial shock transmitted from Southeast Asia to an East Asian country South Korea. With financial assistance from International Monetary Fund, South Korea avoided sovereign default in 1998. This financial crisis in East Asia has spread to Russia and Brazil (Ito and Krueger, 2009).
(c) Financial contagion in Asia
The financial contagion in Asia began in July 1997 when the Thai government decided to devalue its currency, baht, due to a massive speculative attack against the currency. In other words, Thailand became the "ground-zero" country of Asian financial contagion in the 1990s. The financial shock from Thailand's currency crisis quickly spread over other countries in Southeast Asia, such as Indonesia, Malaysia, and the Philippines in August 1997. The financial shocks were further transmitted to the countries in East Asia, such as South Korea and Taiwan in October 1997. The government in these Asian countries make serious efforts to contain these negative contagions but all these currencies substantially fall. This contagion effect is known as the "Asian flu".
Despite its rapid spread over the region, the financial contagion in Asia has different levels of impact on the local currencies in the region. Due to the financial crisis, the Thai baht devaluated the US dollar again by 58.9% from July 1997 to September 1998. In the case of Indonesia and the Philippines, the impacts of financial shock are amplified. The value of Indonesia's rupiah was depreciated by 354.3% while the Philippines' peso was depreciated by 66.1%. By contrast, the impact of the financial crisis was reduced in the case of Malaysia and South Korea. The value of the Korean won against the US dollar depreciated by 54.7% while the Malaysian ringgit depreciated by 47.8%. Interestingly, the financial shock from Thailand's currency crisis has had a devastating impact on the economic condition of neighbouring Asian countries. In other words, the regional economic crisis was triggered by the financial contagion in Asia. As a consequence, five countries in Asia suffered from negative economic growth in 1998, namely Indonesia, Malaysia, the Philippines, South Korea, and Thailand. These five countries, except the Philippines, are known as Asia's "miracle economy", which was celebrated as a role model for other developing countries by the World Bank. In other words, the Asian contagion transformed the Asian "miracle" into the Asian "debacle".
However, the question could be: Why did the transmission of financial shock increase its impact for some countries, such as Indonesia and the Philippines, and decrease its impact for other countries, such as Malaysia and South Korea? Mishkin (1999) asserted that the magnitude of the contagion effect could be determined by the "asymmetric information problem". In other words, the magnitude of transmission of financial shock may not be determined by economic foundations, but by the asymmetry of information. The asymmetric information may contribute to amplifying the financial shock by swinging investors' assessment from excessive optimism to excessive pessimism (Mishkin, 1999).
In this context, this financial contagion in Asia could be considered an example of the "irrational exuberance" in which investors reacted excessively to the financial crisis. This means that the excessively pessimistic assessment of investors or "financial panic" could be considered a driver of the contagion effects in the Asian financial crisis. This means that a small financial shock that began with a minor capital withdrawal escalated into a big financial shock because of imperfection in the financial market. Interestingly, the "financial panic" did not happen in some East Asian countries, such as China and Taiwan. These countries tended to maintain some kind of restriction on free capital movement. In other words, the financial panic in Asian financial contagion could be understood as a policy predicament in Obstfeld's theoretical model of "financial trilemmas" (Noble and Ravenhill, 2000).
Chatziantoniou et al. (2021) developed a quantile vector autoregression (QVAR) framework that allows measuring the connectedness and transmission of spillovers among a group of variables. The QVAR method allows for the connectedness/spillover between the variables to be investigated for different quantiles, τ (0 < τ < 1). Usually, empirical research considers the lower quantile τ at the extreme end to 0, middle quantile τ=0.5, and upper quantile τ at the other extreme ends close to 1. These three points correspond to extreme low, normal, and extreme high market price states in the network of connectedness. The QVAR setup uses a quantile VAR(p) model specified as,
Yt=μ(τ)+∑pj=1Φj(τ)Yt−j+ut(τ), | (1) |
where Yt and Yt−j are the n×1 dimensional endogenous variable vectors such that Yt = (y1t, y2t,…, ynt), μ(τ) is the n×1 dimensional conditional mean vector, Φj(τ) is the n×n dimensional coefficient matrix, ut(τ) are the n×1 dimensional error vector with a n×n dimensional variance-covariance matrix, ∑(τ). The Wold's representation of the QVAR(p) in (1) is then obtained as the Quantile Vector Moving Average [QVMA(∞)}, given as,
Yt=μ(τ)+∑∞i=0Ψi(τ)ut−i. | (2) |
This facilitates the computation of the H-step ahead Generalized Forecast Error Variance Decomposition (GFEVD) based on Rolling window VAR estimation relying on Cholesky factor orthogonalization as incorporated in Koop et al. (1996) and Pesaran and Shin (1998). In the analysis of the vector autoregression (VAR) model, the Forecast Error Variance Decomposition (FEVD) method is a prominent statistical technique to measure the impact of exogenous shocks to variables. However, in this estimation of the FEVD, the linear model, such as the Impulse response function (IRF), is too restrictive because it could not properly capture the asymmetry of shocks in the business cycle. For example, the recessionary shocks tend to be more persistent than the expansionary shocks (Koop et al., 1996; Pesaran and Shin, 1998). To overcome this methodological shortcoming, Pesaran and Shin (1998) suggested a Generalized Impulse Response Function (GIRF) which is building up the nonlinear framework suggested by Koop et al. (1996). As Pesaran and Shin (1998) suggested, we use the Generalized Forecast Error Variance Decomposition (GFEVD) method, which is based on the nonlinear GIRF framework. Thus, the H-step ahead GFEVD is
Ψτij(H)=∑(τ)−1ii∑H−1h=0(e′iΨh(τ)∑(τ)ej)2∑H−1h=0(e′iΨh(τ)∑(τ)Ψh(τ)′ei | (3) |
To show that all variables together explain 100% forecast error variance of the variables in the network of connectedness, each entry of the variance decomposition matrix is normalized as
˜Ψτij(H)=Ψτij(H)∑kj=1Ψτij(H), | (4) |
where ei is a vector containing elements 0, with only 1 on the ith position, and similarly to ej. From (4), two equalities are established as in Chatziantoniou et al. (2021). One, ∑kj=1˜Ψτij(H)=1. Two, ∑ki,j=1˜Ψτij(H)=k. Thus, ˜Ψτij(H) measures the influence of variable j on all other variables i in terms of its share of forecast error variance/shocks. This is also defined as the total directional connectedness TO others (from variable j to all other variables i), i.e. Cτi←j(H).
Cτi←j(H)=∑kj=1,i≠j˜Ψτij(H)∑kj=1˜Ψτij(H)×100 | (5) |
On the other hand, the directional volatility spillovers transmitted by all other variables i to variable j is the total directional connectedness received FROM others (to variable j from all other variables i), i.e.
Cτi→j(H)=∑kj=1,i≠j˜Ψτji(H)∑kj=1˜Ψτji(H)×100 | (6) |
The net directional connectedness is therefore the difference:
NETτi,j=Cτj→i(H)−Cτj←i(H) | (7) |
Thus, NETτi,j > 0, it implies that variables i are net transmitters of shocks since the influence variable j is more than being influenced by them. Also, if NETτi,j > 0, it means that variables i are the net receiver of shocks since variable j influences them more. The Total Connectedness Index (TCI) is given in (6) as.
TCIτ=∑ki,j=1,i≠j˜Ψτij(H)k∑ki,j=1˜Ψτij(H)×100, | (8) |
where TCI determines the strength of the network of connectedness. This also determines the overall market risk, as a high TCI implies high market risk, and vice versa.
We examine the connectedness among 12 Asian stocks in three different regions: Five countries in Southeast Asia (i.e., Indonesia, Malaysia, the Philippines, Singapore, and Thailand); five economies in East Asia (i.e., China, Hong Kong, Japan, South Korea, and Taiwan); and two countries in South Asia (India and Pakistan). We also analyze the impact of the American and European markets (Germany, the United Kingdom, and the United States) on these Asian stock markets. We use the market indices of the FTSE (Financial Times Stock Exchange) country indices for these countries spanning July 2 1996, to 21 February 2023. The source of data is the S&P (Standard & Poor) Capital IQ (S&P Global, 2023). In current studies, only two stock exchange in Europe, namely the London Stock Exchange (LSE) and the Frankfurt Stock Exchange (FSE) are chosen for the empirical analysis because these two stock exchanges could be considered as two major stock exchange in Europe (Ullah, 2015; Tuominen, 2020). Therefore, researchers have also considered to select these two stock markets as major European stock market in their empirical research (Yuce & Simga-Mugan, 2000). In this study, another major stock exchange in Europe or the Euronext is excluded from the empirical analysis because this stock exchange is pan-European stock exchange which is created in 2000 by merging of four European stock exchanges, namely the Paris, Amsterdam, Lisbon and Brussels exchanges. The data coverage of current study is the period of 1996–2023, which includes the periods which the Euronext did not exist. In other words, the FSE and the LSE are two oldest stock European exchanges in the continuous operation. The former was established in 1565 and the later was established in 1698 (Grote, 2007).
Plots of these daily price indices are given in Figure 1. The dynamics of each index is that of a random walk, as the custom of an asset price movement over time t. Thus, these series show nonstationarity properties. A closer look shows that Asian stocks move together due to regional and global market integration. In 1998, most Asian stocks experienced significant market price drops due to the Asian financial crisis of 1997/1998. Others are the 2007/2008 global financial crisis and the global COVID-19 pandemic that crashed financial markets in February–March, 2020. Asian stocks as well as European and American stocks have experienced periods of market ups and downs over the historic periods, and the historic movement is likely to indicate evidence of price movements in terms of market risks (returns or volatility). As researchers (Kao et al., 2015; Gautam and Lepone, 2024) suggested, there is a possibility that the time-zone difference may have impact on the stock market performances in different region, such as America, Europe, Asia, and Oceania. If this is the case, Asian stock market could have advantage of absorbing all information, which was dissimilated in the US stock market in the previous day. In other words, the event that may have impacted the Asian market did not happen the same day as in the US market, but it did happen the previous day.4
4 The authors are grateful to anonymous referee's suggestion for referring to time-zone difference between US market and Asian market.
Since volatility and its clustering are inherent in prices, but are more visible in the log-returns and their absolute version, we first obtain descriptive measures on the log-returns, and as well compute Pearson pairwise correlation analysis. The descriptive analysis allows one to understand the properties and distribution of the volatility series, while the correlation analysis is a preliminary test for connectedness analysis for stocks. Log-return series, yit=loge(Pit/PitPit−1Pit−1)∗100 where Pit is the current day t price of i stock index, Pit−1 is the previous day price of the stock index, and yit is the corresponding log-return series of the stock.
There are four major methods to test the spillover effects, namely the Diebold-Yilmaz (DY) methods, the Copula method, the BEKK-GARCH method, and the quantile VAR (QVAR) method. Among them, we choose the QVAR for its empirical analysis. The methodological advantage of the QVAR method could be explained by comparing it with other three methods. First, the DY method could be used to estimate the total spillover effect. By contrast, the QVAR method could be used to estimate the directional spillover effect. Second, the Copula method is very popular method to estimate spillover effect. However, there could be a misspecification problem when the marginal function and copula could be estimated (Fermanian & Scaillet, 2005; Mai & Scherer, 2013). By contrast, the QVAR method is based on the generalized connectedness framework, which could be used for linear and nonlinear specification. Third, the BEKK-GARCH method is another popular method for the spillover analysis. However, this method suffers from the identification problem because the number of parameter are often relatively large (Huang et al., 2010). By contrast, the QVAR method is based on the TVP-VAR methods in which the identification problem rarely becomes a problem for the empirical analysis.
Table 1 presents the descriptive measures and pairwise correlation estimates on the log-returns. In Malaysia, Thailand, and Pakistan, mean returns are negative, while for all other countries, these are positive; however, all are close to 0, which implies market efficiency evidence in Asian stocks. In all cases, variances of returns are higher than their corresponding mean returns values due to the volatile nature of the series. The UK and US stocks present the lowest returns variance, while quite high return variances are found in Indonesia (5.874), Thailand (4.069), China (3.483), Korea (4.880), Pakistan (3.267), and others. Distribution of returns are negatively skewed in the case of Taiwan, Indonesia, India, Pakistan, Germany, the UK, and the US stocks, and in most cases, these are significantly different from 0 according to D'Agostino (1970). This significant leptokurtosis is typical of stock returns as they are often asymmetric and leptokurtic as indicated in the excess kurtosis estimates that are quite above 3 and significant in all cases. For the stability of the log-returns, we also present the results of the unit root tests by the Elliott-Rothenberg-Stock (ERS) test by Elliot et al. (1992). The results indicate stern rejection of unit root in the returns, implying that returns series are stationary after the log-differencing. We also conduct Q tests of serial autocorrelation, and Q2 tests of ARCH/GARCH errors on returns of stocks following Fisher and Gallagher (2012). The results are found to be highly significant, indicating the possibility of investigating returns/volatility dynamic connectedness using the present datasets, that is the QVAR method. Thus, we present the correlation tests using Pearson moment correlations to uncover the extent or strength of returns dependency at the current time lag. The results, as given in the lower panel of Table 1, show positive and highly significant correlations among Asian stock pairs. The UK, US, and Germany stocks are also positively significantly correlated with Asian stocks except in the case of Indonesia, as it is found that the UK, US, and Germany stocks are not significantly correlated. Moreover, this does not preclude Indonesia since this correlation is static and is not dynamic (no consideration for lagged values dependency). Thus, the results in Table 1 support the adoption of dynamic total connectedness via the quantile method as in Balcilar et al. (2021).
Descriptive Statistics | Indonesia | Malaysia | Philippines | Singapore | Thailand | China | Hong Kong | Japan | Korea | Taiwan | India | Pakistan | Germany | UK | USA |
Mean | 0.008 | −0.005 | 0.000 | 0.004 | −0.006 | 0.031 | 0.011 | 0.000 | 0.020 | 0.008 | 0.024 | −0.023 | 0.013 | 0.008 | 0.026 |
Variance | 5.874 | 1.994 | 2.618 | 1.953 | 4.069 | 3.483 | 2.063 | 1.863 | 4.880 | 2.324 | 2.531 | 3.267 | 2.349 | 1.718 | 1.475 |
Skewness | −1.027*** | 0.638*** | 0.195*** | 0.222*** | 0.469*** | 0.085*** | 0.000 | 0.011 | 0.234*** | −0.086*** | −0.345*** | −0.462*** | −0.233*** | −0.389*** | −0.406*** |
Ex.Kurtosis | 31.17*** | 42.30*** | 12.66*** | 11.48*** | 13.26*** | 6.741*** | 9.578*** | 4.369*** | 14.87*** | 3.518*** | 8.708*** | 9.552*** | 6.070*** | 10.83*** | 9.932*** |
ERS | −35.40*** | −32.59*** | −17.80*** | −22.64*** | −11.44*** | −22.84*** | −30.99*** | −29.85*** | −36.90*** | −13.21*** | −11.16*** | −28.98*** | −36.74*** | −39.56*** | −33.28*** |
Q(20) | 181.8*** | 182.1*** | 151.7*** | 92.82*** | 191.9*** | 65.55*** | 31.92*** | 41.19*** | 129.4*** | 45.13*** | 74.27*** | 88.77*** | 25.50*** | 65.88*** | 73.96*** |
Q2(20) | 2824.0*** | 2778.0*** | 786.1*** | 2433.1*** | 2236.2*** | 3538.6*** | 2876.7*** | 1950.6*** | 3600.2*** | 1380.3*** | 1338.9*** | 1959.5*** | 2526.9*** | 3251.2*** | 5708.5*** |
Pearson Corelation | Indonesia | Malaysia | Philippines | Singapore | Thailand | China | Hong Kong | Japan | Korea | Taiwan | India | Pakistan | Germany | UK | USA |
Indonesia | 1.000*** | 0.118*** | 0.200*** | 0.078*** | 0.124*** | 0.046*** | 0.054*** | 0.053*** | 0.038*** | 0.062*** | 0.032*** | 0.026** | −0.006 | −0.015 | −0.001 |
Malaysia | 0.118*** | 1.000*** | 0.345*** | 0.488*** | 0.414*** | 0.307*** | 0.405*** | 0.265*** | 0.328*** | 0.314*** | 0.256*** | 0.125*** | 0.192*** | 0.223*** | 0.066*** |
Philippines | 0.200*** | 0.345*** | 1.000*** | 0.416*** | 0.364*** | 0.303*** | 0.406*** | 0.294*** | 0.320*** | 0.318*** | 0.257*** | 0.081*** | 0.168*** | 0.200*** | 0.077*** |
Singapore | 0.078*** | 0.488*** | 0.416*** | 1.000*** | 0.511*** | 0.458*** | 0.671*** | 0.412*** | 0.457*** | 0.447*** | 0.409*** | 0.110*** | 0.407*** | 0.439*** | 0.235*** |
Thailand | 0.124*** | 0.414*** | 0.364*** | 0.511*** | 1.000*** | 0.313*** | 0.441*** | 0.268*** | 0.363*** | 0.316*** | 0.300*** | 0.104*** | 0.257*** | 0.273*** | 0.143*** |
China | 0.046*** | 0.307*** | 0.303*** | 0.458*** | 0.313*** | 1.000*** | 0.637*** | 0.307*** | 0.352*** | 0.389*** | 0.346*** | 0.081*** | 0.261*** | 0.286*** | 0.165*** |
Hong Kong | 0.054*** | 0.405*** | 0.406*** | 0.671*** | 0.441*** | 0.637*** | 1.000*** | 0.422*** | 0.483*** | 0.466*** | 0.392*** | 0.111*** | 0.343*** | 0.375*** | 0.182*** |
Japan | 0.053*** | 0.265*** | 0.294*** | 0.412*** | 0.268*** | 0.307*** | 0.422*** | 1.000*** | 0.399*** | 0.364*** | 0.211*** | 0.059*** | 0.202*** | 0.224*** | 0.063*** |
Korea | 0.038*** | 0.328*** | 0.320*** | 0.457*** | 0.363*** | 0.352*** | 0.483*** | 0.399*** | 1.000*** | 0.468*** | 0.324*** | 0.079*** | 0.264*** | 0.290*** | 0.148*** |
Taiwan | 0.062*** | 0.314*** | 0.318*** | 0.447*** | 0.316*** | 0.389*** | 0.466*** | 0.364*** | 0.468*** | 1.000*** | 0.307*** | 0.106*** | 0.237*** | 0.249*** | 0.120*** |
India | 0.032*** | 0.256*** | 0.257*** | 0.409*** | 0.300*** | 0.346*** | 0.392*** | 0.211*** | 0.324*** | 0.307*** | 1.000*** | 0.116*** | 0.321*** | 0.351*** | 0.204*** |
Pakistan | 0.026** | 0.125*** | 0.081*** | 0.110*** | 0.104*** | 0.081*** | 0.111*** | 0.059*** | 0.079*** | 0.106*** | 0.116*** | 1.000*** | 0.050*** | 0.063*** | 0.023 |
Germany | −0.006 | 0.192*** | 0.168*** | 0.407*** | 0.257*** | 0.261*** | 0.343*** | 0.202*** | 0.264*** | 0.237*** | 0.321*** | 0.050*** | 1.000*** | 0.806*** | 0.540*** |
UK | −0.015 | 0.223*** | 0.200*** | 0.439*** | 0.273*** | 0.286*** | 0.375*** | 0.224*** | 0.290*** | 0.249*** | 0.351*** | 0.063*** | 0.806*** | 1.000*** | 0.502*** |
USA | −0.001 | 0.066*** | 0.077*** | 0.235*** | 0.143*** | 0.165*** | 0.182*** | 0.063*** | 0.148*** | 0.120*** | 0.204*** | 0.023 | 0.540*** | 0.502*** | 1.000*** |
Note, ***, ** indicate significance at 1% and 5% significance levels. For normality test, skewness is based on D'Agostino (1970) test, while kurtosis is based on Anscombe and Glynn (1983) test. The Elliott, Rothenberg and Stock (ERS) unit root test is detailed in Ellliot et al. (1992). The Q and Q2 portmanteau tests serial correlation in returns were based on Fisher and Gallagher (2012), and these were computed for up to 20 lags in returns. |
In what follows in Sections 4.2, 4.3, and 4.4, we present the results of dynamic connectedness based on the quantile dynamic method for lower quantile, upper, and middle quantiles, respectively.
Table 2 presents the average connectedness measures at a lower quantile (τ=0.05) as given in the connectedness table. Recall that the dynamic connectedness uses a normalization technique in obtaining the forecast variance decomposition for the connectedness measures. In Table 2, the major diagonal elements are their own contribution while off-diagonal elements are spillover effects; that is, these give either connectedness "from" or "to" others' contributions. Thus, each row of stock variables gives the contribution of the country's stock market volatility to the forecast error variance of one specific stock market. Each column gives the contribution of forecast error variance of a specific stock on all other stocks. For instance, by looking at the row in the results table, the forecast error variance for China is 16.31 which is its own contribution, and this leaves 83.69 as a contribution FROM all other variables in the network.5 By looking at the column in the results table for China, the own contribution of 16.31 gives 88.79 as contribution TO other variables in the network. This leaves the Chinese market as a net transmitter of shocks (NET = 5.1) during the extreme low price market phase. Similarly, Germany with their own shock contribution of 16.41 is influenced by up to 83.59 forecast error variance shocks from other variables in the network, and Germany can influence other variables in the network by up to 86.65 forecast error variance. This leaves Germany as a net transmitter of shocks with NET = 3.06. At this extreme low price market phase, Singaporean and Hong Kong markets are the strongest markets against shocks influenced by other markets in the network as these two markets are net transmitters of shocks with NET = 11.79 and NET = 10.76, respectively. Other markets that are net transmitters of shocks during the extreme low price market phase are Korea (NET = 6.09), China (NET = 5.1), Taiwan (NET = 2.64), and Malaysia (NET = 0.82).
Indonesia | Malaysia | Philippines | Singapore | Thailand | China | Hong Kong | Japan | Korea | Taiwan | India | Pakistan | Germany | UK | USA | FROM | |
Indonesia | 20.18 | 5.85 | 5.97 | 5.99 | 5.49 | 5.59 | 5.85 | 5.87 | 5.83 | 5.59 | 5.74 | 4.96 | 5.75 | 5.79 | 5.55 | 79.82 |
Malaysia | 4.53 | 17.07 | 6.06 | 7.57 | 6.48 | 6.2 | 6.57 | 5.74 | 6.67 | 6.45 | 5.86 | 4.52 | 5.54 | 5.68 | 5.06 | 82.93 |
Philippines | 4.99 | 6.45 | 18.27 | 6.81 | 6.28 | 6.25 | 6.55 | 5.76 | 6.22 | 6.23 | 5.75 | 4.78 | 5.17 | 5.37 | 5.13 | 81.73 |
Singapore | 4.14 | 6.73 | 5.71 | 14.97 | 6.33 | 6.61 | 7.95 | 5.92 | 6.85 | 6.41 | 6.22 | 4.22 | 6.18 | 6.47 | 5.29 | 85.03 |
Thailand | 4.41 | 6.64 | 6.0 | 7.24 | 17.46 | 6.25 | 6.77 | 5.58 | 6.23 | 5.95 | 6.17 | 4.66 | 5.74 | 5.76 | 5.13 | 82.54 |
China | 4.13 | 5.89 | 5.59 | 7.01 | 5.8 | 16.31 | 9.9 | 5.67 | 6.94 | 6.58 | 5.9 | 4.38 | 5.36 | 5.58 | 4.95 | 83.69 |
Hong Kong | 4.07 | 5.88 | 5.53 | 8.01 | 5.95 | 9.43 | 15.12 | 5.93 | 7.21 | 6.81 | 5.83 | 4.18 | 5.59 | 5.68 | 4.79 | 84.88 |
Japan | 4.82 | 5.92 | 5.58 | 6.82 | 5.68 | 6.16 | 6.82 | 17.37 | 7.18 | 6.43 | 5.52 | 4.65 | 5.76 | 5.92 | 5.36 | 82.63 |
Korea | 4.32 | 6.28 | 5.51 | 7.25 | 5.78 | 6.88 | 7.54 | 6.56 | 15.92 | 7.59 | 5.93 | 4.42 | 5.4 | 5.62 | 5.01 | 84.08 |
Taiwan | 4.26 | 6.3 | 5.71 | 7.05 | 5.7 | 6.79 | 7.42 | 6.08 | 7.91 | 16.55 | 5.76 | 4.67 | 5.33 | 5.45 | 5.02 | 83.45 |
India | 4.58 | 5.94 | 5.48 | 7.07 | 6.14 | 6.3 | 6.62 | 5.44 | 6.38 | 6.0 | 17.24 | 4.89 | 6.09 | 6.3 | 5.53 | 82.76 |
Pakistan | 4.92 | 5.64 | 5.57 | 5.86 | 5.63 | 5.64 | 5.73 | 5.58 | 5.78 | 5.9 | 5.99 | 21.38 | 5.57 | 5.51 | 5.29 | 78.62 |
Germany | 4.47 | 5.37 | 4.77 | 6.78 | 5.51 | 5.5 | 6.09 | 5.39 | 5.58 | 5.31 | 5.89 | 4.42 | 16.41 | 10.98 | 7.56 | 83.59 |
UK | 4.43 | 5.44 | 4.87 | 6.99 | 5.46 | 5.66 | 6.12 | 5.49 | 5.75 | 5.34 | 6.0 | 4.29 | 10.83 | 16.14 | 7.19 | 83.86 |
USA | 4.78 | 5.41 | 5.15 | 6.36 | 5.42 | 5.54 | 5.7 | 5.52 | 5.66 | 5.49 | 5.86 | 4.61 | 8.33 | 8.01 | 18.16 | 81.84 |
TO | 62.84 | 83.75 | 77.48 | 96.82 | 81.65 | 88.79 | 95.65 | 80.52 | 90.18 | 86.09 | 82.41 | 63.65 | 86.65 | 88.12 | 76.87 | TCI |
NET | −16.98 | 0.82 | −4.25 | 11.79 | −0.89 | 5.1 | 10.76 | −2.11 | 6.09 | 2.64 | −0.36 | −14.97 | 3.06 | 4.25 | −4.97 | 82.76 |
Note, TCI is the total connectedness index. |
5 Recall, FROM gives the average total spillovers received by a stock market from all other markets in the network, while TO is the average total spillovers transmitted to all other stocks in the market by a particular stock market. NET = TO – FROM. The dynamic evolution of TO and FROM for lower, upper and middle quantiles are given in Figures A1, A2, A3, A4, A5, and A6 in the Appendix.
The net receivers of shocks are the Indonesia, Pakistan, Philippines, Japan, Thailand and India stock markets, while Indonesian and Pakistani stock markets are deemed to be badly hit during the extreme low price market condition due to very high NET values (NET = −16.98, NET = −14.97, respectively). The stocks in the UK and Germany are both net transmitters of shocks in the network, with NET = 3.06 and NET = 4.25, respectively, while US stock is a net receiver of shocks during the extreme low price market state. (NET = −4.97). Thus, at the extreme low price market phase of Asian stocks, European markets (Germany and the UK), and other Asian notable markets such as those in Taiwan, Korea, Hong Kong, China, and Singapore are net transmitters of shocks to the Asian stock market network. The average value of the TCI is 82.76 at this extreme low market price phase, implying that 82.76% of the forecast error variance within this network of variables is linked to the interactions of cross-market innovations, while idiosyncratic effects account for the remaining 17.24% of the forecast error variance in the network. Thus, Asian stocks co-move among themselves at the extreme low market price phase, and these also co-move considerably with global stocks such as those in America and Europe.
The average measures of connectedness given in Table 2 are mere summaries of the strength of the inter-relationships among stock markets under consideration at the market state, while average measures do not point to the timings of the major events that evolve over time in the global financial market. Crises in the market can be mostly either economic or political. Over the sampled period (1996–2013), Asian markets have witnessed events that led to significant market price crashes. These include the Asian crisis of 1997/1998, the global financial crisis of 2007–2009, the COVID-19 pandemic of 2020, and the current Russian-Ukraine war that started in early 2022. These events, as they occur, make the dynamic measures of connectedness more relevant than the average measures of connectedness. The dynamic connectedness gives the evolution of the total connectedness index (TCI) over time (see Figure 2) and it gives the shock transmission nature of specific variables in the network (either the net transmitter or net receiver) as presented in Figure 3. By looking at the evolution of the TCI, there was a rapid increase in the total connectedness of the stock market among Asia, Europe, and America at the extreme low price market condition from the end of the 1990s to the beginning of the 2010s. However, there were some fluctuations in the connectedness from the middle of the 2010s to the end of the 2010s. Due to the COVID-19 pandemic, the total connectedness decreased at the beginning of the 2020s. Overall, it is clear to conclude that the nature of the connectedness among Asian stocks and other developed economies stocks in America and Europe is dynamic at the extreme low price market phase.
As the findings indicated, the "reflexive" connectedness or spillover effect from a stock exchange to same stock market relatively low. For example, it is only 20.1 percent in the case of Indonesia. These findings are in line with a previous study, which reported the low level of "reflexive" connectedness under the extreme quantile condition (Mensi et al., 2024). Furthermore, it could be counterintuitive to conceive a "negative" net spillover effects from the US stock market in the lower extreme quantile condition. These findings may indicate that US stock market may not have strong connectivity with some Asian market and average value of connectedness among 12 Asian market are less than 6. By contrast, US stock market have stronger connectivity with stock market in Germany and UK. On the other hand, the Asian major stock market, such as HK and Singapore, have relatively stronger connectivity with other Asian markets. For example, the stock market in HK has relatively strong connectivity with four Asian stock markets, namely Singapore, Chia, Korea and Taiwan. Similarly, the stock market in Singapore has relatively stronger connectivity with four Asian stock markets, namely Malaysia, Taiwan, HK, and Taiwan. As a result, the overall score of the US stock market connectivity would become negative.
Figure 3 gives the dynamic responses of each stock market, as a net transmitter or net receiver over time, having obtained the indices from values of TO minus FROM (see Appendix, Figure A1 and A2). From the figure, the plot area skewed more to the negative side of the vertical axis of Net connectedness in the cases of Indonesia, Philippine, USA, Japan, and Pakistan. By contrast, the plot area are skewed more to the positive side in the cases of Korea, Germany, Malaysia, China, Taiwan, the UK, Hong Kong, and Singapore. In the remaining countries, we cannot decide due to the fact that the net dynamic connectedness hovers between being positive and negative almost symmetrically. These are the cases of India and Malaysia with weak NET connectedness in Table 2. During the extreme low price market, quantile connectedness tends to increase from that of a normal market as correlations between stocks increase. During this period, investors attempt to move their investments out of riskier stocks into safer ones, leading to a "flight to quality" effect. This leads to stronger stock market integration, and shocks in one part of the market tend to have a higher impact on other stocks in the market. This explains why the connectedness index during the extreme low price market is likely to be above that of the normal market.
Table 3 presents the average connectedness measures at the upper quantile (τ=0.95) for the extreme high price return market condition. It is observed that own variable contributions of forecasts error variance shocks are lower than those reported for the lower quantile, and this implies the fragility of each stock market during this market phase. Thus, market spillover contributions (i.e., off-diagonal elements) increased. Forecasts error variance shocks then increased along the rows and columns at the extremely high price market phase compared to the extreme low price market phase. This leads to high total connectedness received FROM other stock markets by a stock market and high total connectedness transmitted by a stock market TO other stocks. The transmissions TO are quite higher than the transmissions received FROM in the case of the Philippines, Singapore, China, Hong Kong, India, Pakistan, Germany, the UK, and the USA, making these stock markets net transmitters of shocks during the extreme high price market. Thus, during the extreme high price market phase, the listed Asian markets and other advanced economy markets transmit shocks strongly to the international financial markets. The net receivers of shocks during the extreme high price market phase are therefore Indonesia, Malaysia, Thailand, Hong Kong, Japan, Korea, and Taiwan.
Indonesia | Malaysia | Philippines | Singapore | Thailand | China | Hong Kong | Japan | Korea | Taiwan | India | Pakistan | Germany | UK | USA | FROM | |
Indonesia | 6.5 | 6.6 | 6.72 | 6.72 | 6.57 | 6.66 | 6.92 | 6.44 | 6.49 | 6.48 | 6.66 | 6.67 | 6.78 | 6.74 | 7.03 | 93.5 |
Malaysia | 6.26 | 6.89 | 6.73 | 6.74 | 6.57 | 6.67 | 6.91 | 6.41 | 6.47 | 6.47 | 6.67 | 6.68 | 6.77 | 6.76 | 7.01 | 93.11 |
Philippines | 6.24 | 6.58 | 7.02 | 6.73 | 6.57 | 6.71 | 6.9 | 6.42 | 6.47 | 6.46 | 6.68 | 6.7 | 6.74 | 6.74 | 7.03 | 92.98 |
Singapore | 6.23 | 6.63 | 6.7 | 6.99 | 6.57 | 6.69 | 6.94 | 6.42 | 6.46 | 6.48 | 6.66 | 6.69 | 6.77 | 6.76 | 7.01 | 93.01 |
Thailand | 6.24 | 6.61 | 6.72 | 6.72 | 6.88 | 6.69 | 6.9 | 6.42 | 6.51 | 6.49 | 6.65 | 6.67 | 6.76 | 6.74 | 7.0 | 93.12 |
China | 6.21 | 6.58 | 6.7 | 6.73 | 6.57 | 6.97 | 7.0 | 6.41 | 6.48 | 6.49 | 6.67 | 6.65 | 6.76 | 6.74 | 7.03 | 93.03 |
Hong Kong | 6.24 | 6.56 | 6.69 | 6.74 | 6.56 | 6.77 | 7.15 | 6.42 | 6.5 | 6.49 | 6.64 | 6.65 | 6.78 | 6.76 | 7.05 | 92.85 |
Japan | 6.23 | 6.58 | 6.69 | 6.71 | 6.61 | 6.67 | 6.88 | 6.71 | 6.5 | 6.49 | 6.62 | 6.7 | 6.79 | 6.75 | 7.08 | 93.29 |
Korea | 6.25 | 6.58 | 6.71 | 6.71 | 6.59 | 6.7 | 6.92 | 6.43 | 6.78 | 6.5 | 6.65 | 6.67 | 6.75 | 6.73 | 7.01 | 93.22 |
Taiwan | 6.23 | 6.58 | 6.72 | 6.71 | 6.59 | 6.7 | 6.92 | 6.45 | 6.53 | 6.75 | 6.64 | 6.69 | 6.73 | 6.72 | 7.03 | 93.25 |
India | 6.26 | 6.56 | 6.68 | 6.73 | 6.59 | 6.69 | 6.92 | 6.42 | 6.48 | 6.49 | 6.95 | 6.67 | 6.77 | 6.75 | 7.04 | 93.05 |
Pakistan | 6.26 | 6.56 | 6.73 | 6.71 | 6.58 | 6.66 | 6.9 | 6.41 | 6.47 | 6.48 | 6.66 | 7.03 | 6.76 | 6.75 | 7.03 | 92.97 |
Germany | 6.25 | 6.54 | 6.69 | 6.73 | 6.58 | 6.68 | 6.88 | 6.43 | 6.47 | 6.45 | 6.65 | 6.66 | 7.05 | 6.86 | 7.06 | 92.95 |
UK | 6.23 | 6.58 | 6.67 | 6.72 | 6.57 | 6.65 | 6.89 | 6.42 | 6.48 | 6.45 | 6.66 | 6.67 | 6.88 | 7.04 | 7.08 | 92.96 |
USA | 6.23 | 6.54 | 6.71 | 6.73 | 6.58 | 6.67 | 6.89 | 6.42 | 6.49 | 6.46 | 6.65 | 6.67 | 6.81 | 6.79 | 7.37 | 92.63 |
TO | 87.36 | 92.08 | 93.88 | 94.14 | 92.1 | 93.62 | 96.77 | 89.93 | 90.82 | 90.7 | 93.16 | 93.44 | 94.84 | 94.59 | 98.5 | TCI |
NET | −6.14 | −1.04 | 0.9 | 1.13 | −1.01 | 0.59 | 3.92 | −3.36 | −2.4 | −2.55 | 0.11 | 0.47 | 1.89 | 1.63 | 5.87 | 93.06 |
The TCI value at the upper quantile is 93.06%, indicating a very high connectedness of the stock market during the market phase compared to the lower quantile's case with 82.76% for the extreme low market price state. This difference of 10.3% is large enough to warrant the applicability of the QVAR method as it uncovers time-varying market regimes. Further, by comparing the extremely low market price and extremely high market price phases, the Indonesian market is the biggest receiver of financial shocks in the Asian market connectedness during the extreme high price phase (NET = −6.14). Other Asian markets, as well as American and European markets, have experienced switching in their transmission of shocks between being net receiving or net transmitting markets in extreme high price/extreme low price market conditions. Among the Asian markets, we have Malaysia, Thailand, Japan, Korea, and Taiwan as the net receivers of shocks during the extreme high price market phase, while Malaysia, Korea, and Taiwan markets have been the net transmitters of shocks during the extreme low price market phase. Next to Indonesia, the strongest shock receivers are Japan and Taiwan stock markets that are also the strong net receivers of shocks during the market phase. Among the Asian markets, Philippines, Singapore, China, Hong Kong, India, and Pakistan are net transmitters of shocks during the extreme high price market phase, while the Philippines, India, and Pakistan have been the net receivers of shocks at the break market phase.
By focusing on the European and American stocks in the network of Asian markets, the USA market is the strongest transmitter of shocks in the network with NET = 5.87 at the extreme high price market phase. Next to it is the Hong Kong stock market (NET = 3.69) as another net transmitter of shocks among other members of the Asian stock market shock transmitter. As it is further observed based on the upper quantile results, Asian stock market transmissions switched from an extremely low market price to an extremely high market price as the connectedness transcends from the extremely low quantile value to the extremely high quantile value.
The NET measure of connectedness for the three markets, Germany, the UK, and the US, are 1.89, 1.63, and 5.87, respectively, making all three markets net transmitters of shocks to the network during the extreme high price market phase; this is unlike the case of the extreme low price market case where only the USA market was a net receiver of shocks. The stock market in the US transmits shocks to the network more strongly than the Hong Kong stock market, while Hong Kong's market strongly transmits shocks more than the stock markets in Germany and the UK. Thus, the US stock market drives Asian market dynamics more at the extreme high price phase.
Figure 4 gives the plot of the dynamic total connectedness. It is observed that the connectedness measured above 93% in most cases over the sampled period. In 1999, during 2003–2004, and in early 2005, the connectedness dropped significantly below the threshold of 93%, while in some other cases, there are marginal drops from this threshold. Figure 4 shows a series of down spikes, which are also demonstrated in the net total directional connectedness in Figure 5, and in the total directional connectedness to others in Figure A4 in the appendix. Figure A3 plots in the appendix indicate much stable directional shock spillover from a stock market to others, while there are numerous occasional spiking ups of directional spillovers to other markets in the network, as observed in plots in Figure A4 in the appendix. Thus, market turbulence as a result of volatility is higher during the extreme high price market phase. By looking at the plots of net total directional connectedness in Figure 5, we observe mixed results as the transmission of shock is short-lived. This further confirmed the fragility of markets at the extreme high price market phase. We relied on the results of average connectedness obtained in Table 3.
During the normal market condition (middle quantile, τ=0.5), we investigate the connectedness of Asian stocks with American and European stocks. At this stage, we observe weak connections among markets as indicated by a TCI of 46.06% as reported in Table 4. It is observed that forecast error variance values of own variable contributions reported in the diagonal are larger than off-diagonal elements meant for shocks spillover transmission of shocks. These are even larger than those reported during the extreme low price market case. Thus, markets are more stable at normal markets compared to extreme high price and extreme low price situations.
Indonesia | Malaysia | Philippines | Singapore | Thailand | China | Hong Kong | Japan | Korea | Taiwan | India | Pakistan | Germany | UK | USA | FROM | |
Indonesia | 52.66 | 5.11 | 3.86 | 5.82 | 3.85 | 3.72 | 4.58 | 1.85 | 3.48 | 3.06 | 3.15 | 1.14 | 2.61 | 2.67 | 2.44 | 47.34 |
Malaysia | 0.92 | 55.67 | 3.17 | 6.67 | 4.4 | 3.45 | 3.8 | 1.85 | 3.84 | 3.81 | 2.94 | 1.14 | 2.71 | 2.62 | 3.02 | 44.33 |
Philippines | 1.1 | 3.79 | 60.54 | 4.35 | 3.5 | 3.17 | 3.66 | 1.64 | 2.54 | 2.91 | 2.48 | 1.46 | 2.66 | 2.55 | 3.67 | 39.46 |
Singapore | 0.74 | 5.26 | 2.88 | 42.95 | 4.35 | 5.22 | 8.1 | 2.97 | 4.9 | 4.29 | 4.07 | 1.42 | 4.28 | 5.01 | 3.57 | 57.05 |
Thailand | 1.25 | 4.39 | 3.06 | 5.72 | 57.05 | 3.65 | 4.48 | 1.85 | 3.15 | 2.68 | 3.41 | 1.42 | 2.88 | 2.84 | 2.17 | 42.95 |
China | 0.65 | 2.88 | 2.22 | 5.35 | 2.95 | 47.93 | 15.28 | 1.97 | 4.76 | 4.23 | 2.91 | 1.3 | 2.37 | 2.64 | 2.56 | 52.07 |
Hong Kong | 0.78 | 2.92 | 2.53 | 7.88 | 3.61 | 13.98 | 40.18 | 2.44 | 5.78 | 4.85 | 2.96 | 1.15 | 3.2 | 3.52 | 4.23 | 59.82 |
Japan | 0.87 | 2.17 | 1.89 | 4.18 | 2.53 | 2.95 | 3.8 | 58.89 | 4.29 | 2.64 | 1.95 | 1.09 | 3.97 | 3.58 | 5.19 | 41.11 |
Korea | 0.77 | 3.59 | 2.1 | 5.83 | 2.79 | 5.13 | 6.47 | 3.6 | 49.35 | 7.11 | 2.86 | 1.28 | 2.78 | 3.23 | 3.1 | 50.65 |
Taiwan | 0.81 | 3.65 | 2.69 | 5.19 | 2.52 | 4.98 | 6.17 | 2.29 | 7.55 | 52.21 | 2.42 | 1.55 | 2.48 | 2.52 | 2.97 | 47.79 |
India | 1.0 | 2.93 | 2.11 | 5.07 | 3.39 | 3.58 | 4.08 | 1.74 | 3.42 | 2.65 | 58.5 | 1.68 | 3.33 | 3.69 | 2.84 | 41.5 |
Pakistan | 0.8 | 1.74 | 1.7 | 2.12 | 1.9 | 1.74 | 1.82 | 1.2 | 1.72 | 1.82 | 2.06 | 76.25 | 1.73 | 1.56 | 1.84 | 23.75 |
Germany | 0.98 | 1.9 | 1.28 | 4.41 | 2.27 | 2.18 | 2.73 | 1.67 | 1.98 | 1.6 | 2.69 | 1.24 | 48.49 | 18.95 | 7.63 | 51.51 |
UK | 0.95 | 1.83 | 1.37 | 5.02 | 2.42 | 2.42 | 2.93 | 1.7 | 2.21 | 1.73 | 3.06 | 0.99 | 18.61 | 48.37 | 6.4 | 51.63 |
USA | 0.87 | 2.05 | 1.88 | 3.34 | 2.19 | 2.1 | 2.25 | 1.7 | 1.81 | 1.74 | 2.4 | 1.19 | 8.8 | 7.63 | 60.05 | 39.95 |
TO | 12.48 | 44.2 | 32.75 | 70.96 | 42.65 | 58.26 | 70.16 | 28.47 | 51.4 | 45.11 | 39.35 | 18.06 | 62.42 | 63.0 | 51.61 | TCI |
NET | −34.86 | −0.12 | −6.71 | 13.9 | −0.29 | 6.19 | 10.35 | −12.64 | 0.76 | −2.68 | −2.15 | −5.69 | 10.9 | 11.37 | 11.67 | 46.06 |
The net receivers of shocks under the normal market condition are the stock markets in Indonesia, Malaysia, Philippines, Thailand, Japan, Taiwan, India, and Pakistan. Among these receivers of financial shocks, Indonesia received the highest amount of financial shock (NET = −34.86), followed by Japan (NET = −12.64). By contrast, the remaining four Asian countries, namely Singapore, China, Hong Kong, and Korea, and three countries in Europe and America, namely Germany, the UK, and the US are the next transmitter of shock at the normal market condition. Among these seven countries, Singapore (NET = 13.9) transmitted the highest amount of financial shock, followed by the USA (NET = 11.67), the UK (NET = 11.37), Germany (NET = 10.9), Hong Kong (NET = 10.35), and China (NET = 6.19).
Figure 6 gives the evolution of total connectedness over time at the normal stock condition while Figure 7 shows the evolution of directional shock over time.6 According to Figure 6, there was a gradual increase in the total connectedness of the stock market among Asia, Europe, and America at the normal market condition from the end of the 1990s to the middle of the 2010s. However, there were some decreases in total connectedness at the end of the 2010s. Interestingly, there is a rapid increase in total connectedness at the beginning of the 2020s.
6 Note, Figures A5 and A6 are the total directional connectedness from others, and to others, respectively, and from which the NET directional connectedness in Figure 7 are obtained.
Furthermore, as Figure 7 indicates, the plot area skewed more to the negative side of the vertical axis of net connectedness than to the positive side in the cases of seven Asian countries, namely Indonesia, Taiwan, Thailand, Philippines, India, Japan, and Pakistan. Of these, Indonesia, Thailand, and Japan have been net transmitters of shocks at the three market conditions considered (extreme low price, extreme high price, and normal markets). However, Japan played a main role in receiving a financial shock in the normal market condition after Indonesia. By contrast, the plot area skewed, consciously to the positive side in the case of Singapore, China, and Hong Kong being Asian countries, and also skewed conspicuously to the positive side in the three countries in Europe and America, namely Germany, UK, and US. These six countries could be considered the major transmitters of financial shock during the normal market phase. Thus, three countries (USA, the UK, and Germany) are crucial in transmitting shocks to Asian stocks with the three Asian's stocks (Singapore, Hong Kong, and China) during the normal market within the Asian market network.
There is a close relationship between the quantile value and the risk condition in the financial market. First, the lower extreme quantile (τ=0.05) may indicate the arrival of extremely bad news. This means that the financial market stress became high or there is high risk in the financial market. Second, the upper extreme quantile (τ=0.95) may indicate the arrival of extremely bad news. This implies that the financial market stress becomes low and there is low risk in financial market. Third, he median quantile (τ=0.50) may indicate that the normal market condition. This is indicative that financial market stress is moderate or there is moderate risk in the financial market (Zhang & Wei, 2024; Mensi et al., 2024). Considering these three quantile conditions, the pattern of stock market connectedness are separately examined in four period of financial and economic crisis, namely the Asian financial crisis from July 1997 to December 1999, the subprime mortgage crisis from July 2007 to December 2008, the European debt crisis from January 2010 to December 2012, and the COVID-19 recession from January 2020 to December 2021. In this study, the rolling window method is used to examine the total spillover effect in three different quantile condition. As Figure 6 indicated, the spillover effect would become higher during these crisis periods when the normal market condition. By contrast, as Figure 2 and Figure 4 indicates, the level of spillover effect relatively stable during these crises when the high-risk and low-risk market condition. In line with previous studies (Zhang & Wei, 2024; Mensi et al., 2024), these findings indicate that the financial risks would have impact on the level of spillover only at the normal market condition. However, these risks do not seem to matter at the extreme market conditions.
To finalize the analysis of connectedness of Asian stocks viz a vis European and American stocks, it is necessary to employ the TVP-VAR dynamic connectedness method of Antonakakis et al. (2020) on the dataset. This method allows for time-varying assessment of the connectedness as in Furuoka et al. (2023). Due to the fact that both QVAR and TVP-VAR methods are both dynamic connectedness methods, the model performances are easily compared. Table 5 presents the results of the average connectedness measures where we observe similar results to those reported for the QVAR methods, particularly the results obtained for normal market case. Indonesia and Japan remained the persistent shock receiver among the Asian markets as revealed in the TVP-VAR results, Singapore, Hong Kong, and China main their position as strong shock transmitters among Asian stock to the networks. Finally, the USA stock market emerged the strongest in terms of shock transmitting to the network as the market dominated the Singaporean market (NET = 17.2) in terms of transmitting strength (NET = 18.89).
Indonesia | Malaysia | Philippines | Singapore | Thailand | China | Hong Kong | Japan | Korea | Taiwan | India | Pakistan | Germany | UK | USA | FROM | |
Indonesia | 38.55 | 6.53 | 3.75 | 7.07 | 6.61 | 4.08 | 4.99 | 2.4 | 4.84 | 3.68 | 4.11 | 2.07 | 3.52 | 3.55 | 4.23 | 61.45 |
Malaysia | 2.54 | 42.35 | 3.19 | 7.69 | 6.48 | 4.3 | 4.53 | 2.33 | 4.94 | 3.77 | 3.54 | 2.12 | 3.71 | 3.62 | 4.9 | 57.65 |
Philippines | 2.33 | 4.92 | 51.07 | 4.9 | 4.88 | 3.37 | 3.89 | 2.28 | 3.67 | 3.26 | 3.02 | 2.03 | 3.13 | 2.99 | 4.27 | 48.93 |
Singapore | 2.06 | 6.43 | 2.9 | 35.27 | 6.21 | 5.06 | 7.89 | 2.95 | 5.82 | 4.32 | 4.12 | 2.01 | 4.73 | 4.96 | 5.28 | 64.73 |
Thailand | 2.84 | 5.73 | 3.23 | 6.51 | 45.19 | 4.07 | 4.9 | 2.67 | 4.82 | 3.17 | 3.95 | 2.32 | 3.67 | 3.25 | 3.66 | 54.81 |
China | 1.61 | 4.21 | 2.44 | 5.88 | 4.64 | 40.01 | 13.32 | 2.35 | 5.12 | 4.1 | 3.65 | 1.99 | 3.22 | 3.33 | 4.14 | 59.99 |
Hong Kong | 1.74 | 4.2 | 2.73 | 8.36 | 5.17 | 12.07 | 33.67 | 2.6 | 6.26 | 4.72 | 3.3 | 1.93 | 3.79 | 3.87 | 5.59 | 66.33 |
Japan | 1.91 | 3.26 | 2.46 | 4.58 | 4.54 | 3.05 | 3.73 | 49.74 | 4.66 | 3.24 | 2.92 | 1.67 | 4.55 | 3.93 | 5.75 | 50.26 |
Korea | 2.04 | 4.95 | 2.51 | 6.55 | 5.5 | 4.93 | 6.2 | 3.5 | 38.98 | 6.52 | 3.46 | 2.19 | 3.79 | 3.68 | 5.18 | 61.02 |
Taiwan | 1.97 | 4.38 | 2.7 | 5.63 | 4.42 | 4.85 | 5.81 | 2.76 | 7.83 | 43.24 | 3 | 2.05 | 3.27 | 3.42 | 4.67 | 56.76 |
India | 2.01 | 4.15 | 2.45 | 5.6 | 5.45 | 4.03 | 4.28 | 2.42 | 4.24 | 3.16 | 48.51 | 2.2 | 3.84 | 3.78 | 3.88 | 51.49 |
Pakistan | 2.01 | 3.45 | 2.41 | 3.69 | 3.83 | 2.57 | 2.93 | 1.79 | 3.29 | 2.73 | 2.79 | 60.71 | 2.57 | 2.18 | 3.05 | 39.29 |
Germany | 1.6 | 3.07 | 1.73 | 5.12 | 4.01 | 2.71 | 3.25 | 2.31 | 3.14 | 2.11 | 3.21 | 1.73 | 40.47 | 16.98 | 8.59 | 59.53 |
UK | 1.46 | 3.21 | 1.48 | 5.62 | 3.78 | 2.91 | 3.32 | 2.07 | 3.11 | 2.37 | 3.36 | 1.47 | 16.99 | 40.98 | 7.87 | 59.02 |
USA | 2.09 | 3.8 | 2.26 | 4.74 | 3.85 | 2.84 | 3.07 | 2.08 | 3.58 | 2.63 | 2.79 | 2.21 | 8.49 | 7.76 | 47.82 | 52.18 |
TO | 28.2 | 62.29 | 36.24 | 81.93 | 69.37 | 60.83 | 72.1 | 34.51 | 65.32 | 49.78 | 47.23 | 27.99 | 69.28 | 67.29 | 71.07 | TCI |
NET | −33.25 | 4.63 | −12.69 | 17.2 | 14.56 | 0.84 | 5.78 | −15.74 | 4.31 | −6.97 | −4.26 | −11.3 | 9.74 | 8.27 | 18.89 | 56.23 |
This is the first empirical research of its kind to employ a newly developed method, the quantile vector autoregressive (QVAR) dynamic connectedness method, for the analysis of stock market connectedness in 15 countries, namely Germany, the UK, the USA, and 12 Asian countries, which include five major ASEAN countries, namely Indonesia, Malaysia, Philippines, Singapore, and Thailand from 1996 to 2023. The methodological advantage of the quantile connectedness analysis is that it could examine separately three different stock market conditions, namely the extreme low price market condition, the extreme high price market condition, and the normal market condition.
The empirical findings can be summarized into three interesting facts on the stock market connectedness among Asia, Europe, and America. First, Germany and UK an be considered minor transmitters of financial shocks during the extreme low price market phase. By contrast, the USA can be considered a minor receiver of financial shocks in the extreme low price market phase. Second, Germany and the UK are very minor transmitters of financial shocks during the extreme high price market phase; interestingly, the USA could play an important role in transmitting shocks in the extreme high price market condition. Third, these three countries, namely Germany, the UK, and the USA, become major transmitters of financial shocks in the Asian stock markets network during the normal market condition.
This study may offer four interesting discussions by linking its findings with previous findings which were conducted by other researchers. First, the findings seem to confirm the important role of US stock market for transmitting financial shocks to the Asian stock market (Aggarwal & Rivoli, 1989; Lin et al., 1994; Diebold & Yilmaz, 2009; Samarakoon, 2011). Our additional insight is that the US market can be emerged as a main transmitter of shocks when the stock prices are high rather than low. Second, the findings are in line with previous studies where the Asian stock market also played a role as a risk transmitter to US market (Samarakoon, 2011). The current study indicates that the Asian market becomes a risk transmitter when the stock price is low. Third, the findings in the current study are in line with previous studies where the European stock market is not an important transmitter of financial risks to the Asian stock market (Diebold & Yilmaz, 2009). Finally, current findings also indicate that there is a strong connectedness among Asian market (Yousaf et al., 2023). Additional insight from the current study is that Asian market plays an important role as a transmitter in the low price condition rather than high price condition.
More importantly, the empirical findings indicated some interesting features of stock market connectedness among Asian countries. First, Singapore has played a dominant and central role in the Asian stock market connectedness. Singapore is the most prominent transmitter of financial shocks in all three different stock market conditions, except the extreme high price market condition where the transmission is weak. Additionally, stock market performance in Singapore has major impacts on the stock market in its ASEAN neighbouring countries, namely Indonesia, Malaysia, the Philippines, and Thailand. Second, despite its economic size, Indonesia can be considered a passive player in the Asian market connectedness. Indonesia, Thailand, and Japan are the net receivers of financial shocks in all three stock market conditions. Third, the evolution of total directional connectedness revealed the increasingly important role of Hong Kong and China as the transmitters of financial shocks in the Asian market. Especially, in the extreme low-price market, the magnitudes of the shock transmissions have rapidly increased since the middle 2000s.
The findings in this paper will be of relevance to policy makers in Asia. International traders will also learn the dynamic mechanism of trades among stocks in Asia, Europe, and America. Conclusively, due to the fact that Asian markets are not traded in the same time zones as Europe and America, and we tend to check if different time zones will influence our results, our findings are mere suggestions of sequential trading or markets in America that have absolved all pertinent market information. Thus, we recommend curious authors to consider intradaily high-frequency frequency data such as hourly data to verify our findings. Furthermore, a previous study indicated that the commodity market could be considered an important cause for the financial shock in the stock market (Diebold & Yilmaz, 2012). Future research may include exact causes of the financial shock between US and Asian stock markets.
Conceptualization, OOSY, and FF; methodology, OOSY and FF; software, OOSY and FF; validation, OOSY, FF, and MZ; formal analysis, OOSY and FF; investigation, OOSY, FF, MZ and HX; resources, OOSY, and FF; data curation, OOSY, and FF; writing—original draft preparation, OOSY, and FF; writing—review and editing, OOSY, FF, MZ and HX; visualization, OOSY, FF, MZ and HX; supervision; project administration, OOSY and FF; funding acquisition, OOSY, FF, MZ and HX.
The authors declare they have not used Artificial Intelligence (AI) tools in the creation of this article.
All authors declare no conflicts of interest in this paper.
[1] |
Agénor PR, Pereira da Silva LA (2022) Financial spillovers, spillbacks, and the scope for international macroprudential policy coordination. Int Econ Econ Policy 19: 79–127. https://doi.org/10.1007/s10368-021-00522-5 doi: 10.1007/s10368-021-00522-5
![]() |
[2] | Aggarwal R, Rivoli P (1989) The relationship between the US and four Asian stock markets. ASEAN Econ Bull 6: 110–117. |
[3] |
Ando T, Greenwood-Nimmo M, Shin Y (2022) Quantile Connectedness: Modeling Tail Behavior in the Topology of Financial Networks. Manag Sci 68: 2401–2431. https://doi.org/10.1287/mnsc.2021.3984 doi: 10.1287/mnsc.2021.3984
![]() |
[4] |
Anscombe FJ, Glynn WJ (1983) Distribution of the kurtosis statistic b2 for normal samples. Biometrika 70: 227–234. https://doi.org/10.1093/biomet/70.1.227 doi: 10.1093/biomet/70.1.227
![]() |
[5] |
Antonakakis N, Chatziantoniou I, Gabauer D (2020) Refined Measures of Dynamic Connectedness based on Time-Varying Parameter Vector Autoregressions. J Risk Financ Manage 13: 84. https://doi.org/10.3390/jrfm13040084 doi: 10.3390/jrfm13040084
![]() |
[6] |
Baig AS, Butt HA, Haroon O, et al. (2021) Deaths, panic, lockdowns and US equity markets: The case of COVID-19 pandemic. Financ Res Lett 38: 101701. https://doi.org/10.1016/j.frl.2020.101701 doi: 10.1016/j.frl.2020.101701
![]() |
[7] |
Balcilar M, Gabauer D, Umar Z (2021) Crude Oil futures contracts and commodity markets: New evidence from a TVP-VAR extended joint connectedness approach. Resour Policy 73: 102219. https://doi.org/10.1016/j.resourpol.2021.102219 doi: 10.1016/j.resourpol.2021.102219
![]() |
[8] | CEIC (2024) Available from: https://www.ceicdata.com/en (accessed on 7 August 2024) |
[9] |
Cepoi CO (2020) Asymmetric dependence between stock market returns and news during COVID-19 financial turmoil. Financ Res Lett 36: 101658. https://doi.org/10.1016/j.frl.2020.101658 doi: 10.1016/j.frl.2020.101658
![]() |
[10] |
Chatziantoniou I, Gabauer D (2021) EMU risk-synchronisation and financial fragility through the prism of dynamic connectedness. Q Rev Econ Financ 79: 1–14. https://doi.org/10.1016/j.qref.2020.12.003 doi: 10.1016/j.qref.2020.12.003
![]() |
[11] | Chatziantoniou I, Gabauer D, Gupta R (2021) Integration and risk transmission in the market for crude oil: a time-varying parameter frequency connectedness approach. Working Paper, University of Portsmouth, No. 202147. |
[12] |
Chatziantoniou I, Gabauer D, Stenfors A (2021) Interest rate swaps and the transmission mechanism of monetary policy: A quantile connectedness approach. Econ Lett 204: 109891. https://doi.org/10.1016/j.econlet.2021.109891 doi: 10.1016/j.econlet.2021.109891
![]() |
[13] |
Chavleishvili S, Manganelli S (2024) Forecasting and stress testing with quantile vector autoregression. J Appl Economet 39: 66–85. https://doi.org/10.1002/jae.3009 doi: 10.1002/jae.3009
![]() |
[14] |
D'Agostino RB (1970) Transformation to Normality of the Null Distribution of g1. Biometrika 57: 679–681. https://doi.org/10.2307/2334794. doi: 10.2307/2334794
![]() |
[15] |
Dai Z, Zhu J, Zhang X (2022) Time-frequency connectedness and cross-quantile dependence between crude oil, Chinese commodity market, stock market and investor sentiment. Energ Econ 114: 106226. https://doi.org/10.1016/j.eneco.2022.106226. doi: 10.1016/j.eneco.2022.106226
![]() |
[16] |
Diebold FX, Yilmaz K (2009) Measuring financial asset return and volatility spillovers, with application to global equity markets. Econ J 119: 158–171. https://doi.org/10.1111/j.1468-0297.2008.02208.x doi: 10.1111/j.1468-0297.2008.02208.x
![]() |
[17] |
Diebold FX, Yilmaz K (2012) Better to give than to receive: Predictive directional measurement of volatility spillovers. Int J Forecast 28: 57–66. https://doi.org/10.1016/j.ijforecast.2011.02.006 doi: 10.1016/j.ijforecast.2011.02.006
![]() |
[18] |
Diebold FX, Yılmaz K (2014) On the network topology of variance decompositions: Measuring the connectedness of financial firms. J Econometrics 182: 119–134. https://doi.org/10.1016/j.jeconom.2014.04.012 doi: 10.1016/j.jeconom.2014.04.012
![]() |
[19] |
Dornbusch R, Park YC, Claessens S (2000) Contagion: Understanding How It Spreads. World Bank Res Obs 15: 177–197. https://doi.org/10.1093/wbro/15.2.177 doi: 10.1093/wbro/15.2.177
![]() |
[20] | Elliott G, Rothenberg TJ, Stock JH (1992) Efficient Tests for an Autoregressive Unit Root (Working Paper No. 130) National Bureau of Economic Research. https://doi.org/10.3386/t0130 |
[21] | Fermanian JD, Scaillet O (2005) Some statistical pitfalls in copula modeling for financial applications. FAME Research paper No.108. |
[22] | Fleming JM (1962) Domestic financial policies under fixed and under floating exchange rates. Staff Pap Int Monetary Fund 9: 369–380. |
[23] |
Fisher TJ, Gallagher CM (2012) New Weighted Portmanteau Statistics for Time Series Goodness of Fit Testing. J Am Stat Assoc 107: 777–787. https://doi.org/10.1080/01621459.2012.688465 doi: 10.1080/01621459.2012.688465
![]() |
[24] |
Furuoka F, Yaya OS, Ling PK, et al. (2023) Transmission of risks between energy and agricultural commodities: Frequency time-varying VAR, asymmetry and portfolio management. Resour Policy 81: 103339. https://doi.org/10.1016/j.resourpol.2023.103339 doi: 10.1016/j.resourpol.2023.103339
![]() |
[25] | Gautam A, Lepone G (2024) Time Zone Difference and Equity Market Price Efficiency Post Earnings Announcements. http://dx.doi.org/10.2139/ssrn.4815836 |
[26] |
Gabauer D (2021) Dynamic measures of asymmetric & pairwise connectedness within an optimal currency area: Evidence from the ERM I system. J Multinatl Financ M 60: 100680. https://doi.org/10.1016/j.mulfin.2021.100680 doi: 10.1016/j.mulfin.2021.100680
![]() |
[27] |
Grote MH (2007) Mobile marketplaces—consequences of the changing governance of European stock exchanges. Growth Change 38: 260–278. https://doi.org/10.1111/j.1468-2257.2007.00366.x doi: 10.1111/j.1468-2257.2007.00366.x
![]() |
[28] |
Gong C, Tang P, Wang Y (2019) Measuring the network connectedness of global stock markets. Phys A 535: 122351. https://doi.org/10.1016/j.physa.2019.122351 doi: 10.1016/j.physa.2019.122351
![]() |
[29] | Huang Y, Su W, Li X (2010) Comparison of BEKK GARCH and DCC GARCH models: An empirical study, In: Advanced Data Mining and Applications, Springer Berlin Heidelberg, 99–110. https://doi.org/10.1007/978-3-642-17313-4_10 |
[30] | Ito T, Krueger AO (2009) Regional and Global Capital Flows: Macroeconomic Causes and Consequences, University of Chicago Press. |
[31] |
Iwanicz-Drozdowska M, Rogowicz K, Kurowski Ł, et al. (2021) Two decades of contagion effect on stock markets: Which events are more contagious? J Financ Stabil 55: 100907. https://doi.org/10.1016/j.jfs.2021.100907 doi: 10.1016/j.jfs.2021.100907
![]() |
[32] |
Kao EH, Ho TW, Fung HG (2015) Price linkage between the US and Japanese futures across different time zones: An analysis of the minute-by-minute data. J Int Financ Mark I 34: 321–336. https://doi.org/10.1016/j.intfin.2014.12.002 doi: 10.1016/j.intfin.2014.12.002
![]() |
[33] |
Koop G, Pesaran MH, Potter SM (1996) Impulse response analysis in nonlinear multivariate models. J Econometrics 74: 119–147. https://doi.org/10.1016/0304-4076(95)01753-4 doi: 10.1016/0304-4076(95)01753-4
![]() |
[34] | Korkmaz A (2014) Can the Interdependence Effect and the Contagion Phenomena be Related with One Another? J Empirl Stud 1: 38–47. |
[35] |
Lin WL, Engle RF, Ito T (1994) Do bulls and bears move across borders? International transmission of stock returns and volatility. Rev Financ Stud 7: 507–538. https://doi.org/10.1093/rfs/7.3.507 doi: 10.1093/rfs/7.3.507
![]() |
[36] |
Mai JF, Scherer M (2013) What makes dependence modeling challenging? Pitfalls and ways to circumvent them. Statist Risk Model 30: 287–306. https://doi.org/10.1524/strm.2013.2001 doi: 10.1524/strm.2013.2001
![]() |
[37] |
Marfatia HA (2017) A Fresh Look at Integration of Risks in the International Stock Markets: A Wavelet Approach. Rev Financ Econ 34: 33–49. https://doi.org/10.1016/j.rfe.2017.07.003 doi: 10.1016/j.rfe.2017.07.003
![]() |
[38] |
Mensi W, Boubaker FZ, Al-Yahyaee KH, et al. (2018) Dynamic volatility spillovers and connectedness between global, regional, and GIPSI stock markets. Financ Res Lett 25: 230–238. https://doi.org/10.1016/j.frl.2017.10.032 doi: 10.1016/j.frl.2017.10.032
![]() |
[39] |
Mensi W, Ziadat SA, Vo XV, et al. (2024) Extreme quantile connectedness and spillovers between oil and Vietnamese stock markets: a sectoral analysis. Int J Emerg Mark 19: 1586–1625. https://doi.org/10.1108/IJOEM-03-2022-0513 doi: 10.1108/IJOEM-03-2022-0513
![]() |
[40] |
Mishkin FS (1999) Lessons from the Asian crisis. J Int Money Financ 18: 709–723. https://doi.org/10.1016/S0261-5606(99)00020-0 doi: 10.1016/S0261-5606(99)00020-0
![]() |
[41] | Noble GW, Ravenhill J (2000) Causes and Consequences of the Asian Financial Crisis, In: G. W. Noble & J. Ravenhill (Eds.), The Asian Financial Crisis and the Architecture of Global Finance Cambridge University Press, 1st ed., 1–35. https://doi.org/10.1017/CBO9781139171328.002 |
[42] |
Pesaran HH, Shin Y (1998) Generalized impulse response analysis in linear multivariate models. Econ Lett 58: 17–29. https://doi.org/10.1016/S0165-1765(97)00214-0 doi: 10.1016/S0165-1765(97)00214-0
![]() |
[43] | S&P Global (2023) S&Q Capital IQ. https://www.spglobal.com/marketintelligence/. [accessed on 25 February 2023]. |
[44] |
Samarakoon LP (2011) Stock market interdependence, contagion, and the US financial crisis: The case of emerging and frontier markets. J Int Financ Mark I 21: 724–742. https://doi.org/10.1016/j.intfin.2011.05.001 doi: 10.1016/j.intfin.2011.05.001
![]() |
[45] |
Serrano P, Vaello-Sebastià A, Vich-Llompart MM (2023) The international linkages of market risk perception. J Multinatl Financ M 72: 100826. https://doi.org/10.1016/j.mulfin.2023.100826 doi: 10.1016/j.mulfin.2023.100826
![]() |
[46] | Schüler YS (2014) Asymmetric effects of uncertainty over the business cycle: A quantile structural vector autoregressive approach. Universi ty of Konstanz, Department of Economics, Working Paper No. 2014-0. |
[47] |
Tiwari AK, Abakah EJA, Yaya OS, et al. (2022) Tail risk dependence, co-movement and predictability between green bond and green stocks. Appl Econ 55: 201–222. https://doi.org/10.1080/00036846.2022.2085869 doi: 10.1080/00036846.2022.2085869
![]() |
[48] | Tuominen DR (2020) Investigation on how Brexit has impacted IPO listings with Deutsche Börse and the London Stock Exchange. Degree Thesis, Arcada University of Applied Sciences (Finland). Available from: https://www.theseus.fi/handle/10024/353824 (accessed on 6 April 2024) |
[49] | Ullah S (2015) The Impact of Internal Corporate Governance Mechanisms on the Performance of Firms: Evidence from the UK and Germany. Open University (United Kingdom). Available from: https://www.proquest.com/docview/2171038131?pq-origsite = gscholar & fromopenview = true & sourcetype = Dissertations%20 & %20Theses(accessed on 7 February 2024) |
[50] | Wang P, Wang P (2010) Price and volatility spillovers between the Greater China Markets and the developed markets of US and Japan. Global Financ J 21: 304–317. https://doi.org/10.1016/j.gfj.2010.09.007 |
[51] |
Yang Z, Zhou Y (2017) Quantitative easing and volatility spillovers across countries and asset classes. Manage Sci 63: 333–354. https://doi.org/10.1287/mnsc.2015.2305 doi: 10.1287/mnsc.2015.2305
![]() |
[52] |
Yousaf I, Mensi W, Vo XV, et al. (2023) Spillovers and connectedness between Chinese and ASEAN stock markets during bearish and bullish market statuses. Int J Emerg Mark. https://doi.org/10.1108/IJOEM-07-2022-1194 doi: 10.1108/IJOEM-07-2022-1194
![]() |
[53] | Yuce A, Simga-Mugan C (2000) Linkages among Eastern European stock markets and the major stock exchanges. Russian East Eur Financ Trade 36: 54–69. |
[54] |
Zhang Q, Wei R (2024) Carbon reduction attention and financial market stress: A network spillover analysis based on quantile VAR modeling. J Environ Manage 356: 120640. https://doi.org/10.1016/j.jenvman.2024.120640 doi: 10.1016/j.jenvman.2024.120640
![]() |
![]() |
![]() |
1. | Naveed Khan, OlaOluwa S. Yaya, Xuan Vinh Vo, Hassan Zada, Quantile time-frequency connectedness and spillovers among financial stress, cryptocurrencies and commodities, 2025, 103, 03014207, 105527, 10.1016/j.resourpol.2025.105527 | |
2. | Naveed Khan, Ozair Siddiqui, OlaOluwa S. Yaya, Xuan Vinh Vo, Ripple Effects of the US-China Tension on Asian Emerging and Frontier Markets with Portfolio Implications, 2025, 1081-1826, 10.1515/snde-2024-0116 | |
3. | Xuewei Zhou, Zisheng Ouyang, Min Lu, Global volatility connectedness and the determinants: evidence from multilayer networks, 2025, 1351-847X, 1, 10.1080/1351847X.2025.2482829 |
Descriptive Statistics | Indonesia | Malaysia | Philippines | Singapore | Thailand | China | Hong Kong | Japan | Korea | Taiwan | India | Pakistan | Germany | UK | USA |
Mean | 0.008 | −0.005 | 0.000 | 0.004 | −0.006 | 0.031 | 0.011 | 0.000 | 0.020 | 0.008 | 0.024 | −0.023 | 0.013 | 0.008 | 0.026 |
Variance | 5.874 | 1.994 | 2.618 | 1.953 | 4.069 | 3.483 | 2.063 | 1.863 | 4.880 | 2.324 | 2.531 | 3.267 | 2.349 | 1.718 | 1.475 |
Skewness | −1.027*** | 0.638*** | 0.195*** | 0.222*** | 0.469*** | 0.085*** | 0.000 | 0.011 | 0.234*** | −0.086*** | −0.345*** | −0.462*** | −0.233*** | −0.389*** | −0.406*** |
Ex.Kurtosis | 31.17*** | 42.30*** | 12.66*** | 11.48*** | 13.26*** | 6.741*** | 9.578*** | 4.369*** | 14.87*** | 3.518*** | 8.708*** | 9.552*** | 6.070*** | 10.83*** | 9.932*** |
ERS | −35.40*** | −32.59*** | −17.80*** | −22.64*** | −11.44*** | −22.84*** | −30.99*** | −29.85*** | −36.90*** | −13.21*** | −11.16*** | −28.98*** | −36.74*** | −39.56*** | −33.28*** |
Q(20) | 181.8*** | 182.1*** | 151.7*** | 92.82*** | 191.9*** | 65.55*** | 31.92*** | 41.19*** | 129.4*** | 45.13*** | 74.27*** | 88.77*** | 25.50*** | 65.88*** | 73.96*** |
Q2(20) | 2824.0*** | 2778.0*** | 786.1*** | 2433.1*** | 2236.2*** | 3538.6*** | 2876.7*** | 1950.6*** | 3600.2*** | 1380.3*** | 1338.9*** | 1959.5*** | 2526.9*** | 3251.2*** | 5708.5*** |
Pearson Corelation | Indonesia | Malaysia | Philippines | Singapore | Thailand | China | Hong Kong | Japan | Korea | Taiwan | India | Pakistan | Germany | UK | USA |
Indonesia | 1.000*** | 0.118*** | 0.200*** | 0.078*** | 0.124*** | 0.046*** | 0.054*** | 0.053*** | 0.038*** | 0.062*** | 0.032*** | 0.026** | −0.006 | −0.015 | −0.001 |
Malaysia | 0.118*** | 1.000*** | 0.345*** | 0.488*** | 0.414*** | 0.307*** | 0.405*** | 0.265*** | 0.328*** | 0.314*** | 0.256*** | 0.125*** | 0.192*** | 0.223*** | 0.066*** |
Philippines | 0.200*** | 0.345*** | 1.000*** | 0.416*** | 0.364*** | 0.303*** | 0.406*** | 0.294*** | 0.320*** | 0.318*** | 0.257*** | 0.081*** | 0.168*** | 0.200*** | 0.077*** |
Singapore | 0.078*** | 0.488*** | 0.416*** | 1.000*** | 0.511*** | 0.458*** | 0.671*** | 0.412*** | 0.457*** | 0.447*** | 0.409*** | 0.110*** | 0.407*** | 0.439*** | 0.235*** |
Thailand | 0.124*** | 0.414*** | 0.364*** | 0.511*** | 1.000*** | 0.313*** | 0.441*** | 0.268*** | 0.363*** | 0.316*** | 0.300*** | 0.104*** | 0.257*** | 0.273*** | 0.143*** |
China | 0.046*** | 0.307*** | 0.303*** | 0.458*** | 0.313*** | 1.000*** | 0.637*** | 0.307*** | 0.352*** | 0.389*** | 0.346*** | 0.081*** | 0.261*** | 0.286*** | 0.165*** |
Hong Kong | 0.054*** | 0.405*** | 0.406*** | 0.671*** | 0.441*** | 0.637*** | 1.000*** | 0.422*** | 0.483*** | 0.466*** | 0.392*** | 0.111*** | 0.343*** | 0.375*** | 0.182*** |
Japan | 0.053*** | 0.265*** | 0.294*** | 0.412*** | 0.268*** | 0.307*** | 0.422*** | 1.000*** | 0.399*** | 0.364*** | 0.211*** | 0.059*** | 0.202*** | 0.224*** | 0.063*** |
Korea | 0.038*** | 0.328*** | 0.320*** | 0.457*** | 0.363*** | 0.352*** | 0.483*** | 0.399*** | 1.000*** | 0.468*** | 0.324*** | 0.079*** | 0.264*** | 0.290*** | 0.148*** |
Taiwan | 0.062*** | 0.314*** | 0.318*** | 0.447*** | 0.316*** | 0.389*** | 0.466*** | 0.364*** | 0.468*** | 1.000*** | 0.307*** | 0.106*** | 0.237*** | 0.249*** | 0.120*** |
India | 0.032*** | 0.256*** | 0.257*** | 0.409*** | 0.300*** | 0.346*** | 0.392*** | 0.211*** | 0.324*** | 0.307*** | 1.000*** | 0.116*** | 0.321*** | 0.351*** | 0.204*** |
Pakistan | 0.026** | 0.125*** | 0.081*** | 0.110*** | 0.104*** | 0.081*** | 0.111*** | 0.059*** | 0.079*** | 0.106*** | 0.116*** | 1.000*** | 0.050*** | 0.063*** | 0.023 |
Germany | −0.006 | 0.192*** | 0.168*** | 0.407*** | 0.257*** | 0.261*** | 0.343*** | 0.202*** | 0.264*** | 0.237*** | 0.321*** | 0.050*** | 1.000*** | 0.806*** | 0.540*** |
UK | −0.015 | 0.223*** | 0.200*** | 0.439*** | 0.273*** | 0.286*** | 0.375*** | 0.224*** | 0.290*** | 0.249*** | 0.351*** | 0.063*** | 0.806*** | 1.000*** | 0.502*** |
USA | −0.001 | 0.066*** | 0.077*** | 0.235*** | 0.143*** | 0.165*** | 0.182*** | 0.063*** | 0.148*** | 0.120*** | 0.204*** | 0.023 | 0.540*** | 0.502*** | 1.000*** |
Note, ***, ** indicate significance at 1% and 5% significance levels. For normality test, skewness is based on D'Agostino (1970) test, while kurtosis is based on Anscombe and Glynn (1983) test. The Elliott, Rothenberg and Stock (ERS) unit root test is detailed in Ellliot et al. (1992). The Q and Q2 portmanteau tests serial correlation in returns were based on Fisher and Gallagher (2012), and these were computed for up to 20 lags in returns. |
Indonesia | Malaysia | Philippines | Singapore | Thailand | China | Hong Kong | Japan | Korea | Taiwan | India | Pakistan | Germany | UK | USA | FROM | |
Indonesia | 20.18 | 5.85 | 5.97 | 5.99 | 5.49 | 5.59 | 5.85 | 5.87 | 5.83 | 5.59 | 5.74 | 4.96 | 5.75 | 5.79 | 5.55 | 79.82 |
Malaysia | 4.53 | 17.07 | 6.06 | 7.57 | 6.48 | 6.2 | 6.57 | 5.74 | 6.67 | 6.45 | 5.86 | 4.52 | 5.54 | 5.68 | 5.06 | 82.93 |
Philippines | 4.99 | 6.45 | 18.27 | 6.81 | 6.28 | 6.25 | 6.55 | 5.76 | 6.22 | 6.23 | 5.75 | 4.78 | 5.17 | 5.37 | 5.13 | 81.73 |
Singapore | 4.14 | 6.73 | 5.71 | 14.97 | 6.33 | 6.61 | 7.95 | 5.92 | 6.85 | 6.41 | 6.22 | 4.22 | 6.18 | 6.47 | 5.29 | 85.03 |
Thailand | 4.41 | 6.64 | 6.0 | 7.24 | 17.46 | 6.25 | 6.77 | 5.58 | 6.23 | 5.95 | 6.17 | 4.66 | 5.74 | 5.76 | 5.13 | 82.54 |
China | 4.13 | 5.89 | 5.59 | 7.01 | 5.8 | 16.31 | 9.9 | 5.67 | 6.94 | 6.58 | 5.9 | 4.38 | 5.36 | 5.58 | 4.95 | 83.69 |
Hong Kong | 4.07 | 5.88 | 5.53 | 8.01 | 5.95 | 9.43 | 15.12 | 5.93 | 7.21 | 6.81 | 5.83 | 4.18 | 5.59 | 5.68 | 4.79 | 84.88 |
Japan | 4.82 | 5.92 | 5.58 | 6.82 | 5.68 | 6.16 | 6.82 | 17.37 | 7.18 | 6.43 | 5.52 | 4.65 | 5.76 | 5.92 | 5.36 | 82.63 |
Korea | 4.32 | 6.28 | 5.51 | 7.25 | 5.78 | 6.88 | 7.54 | 6.56 | 15.92 | 7.59 | 5.93 | 4.42 | 5.4 | 5.62 | 5.01 | 84.08 |
Taiwan | 4.26 | 6.3 | 5.71 | 7.05 | 5.7 | 6.79 | 7.42 | 6.08 | 7.91 | 16.55 | 5.76 | 4.67 | 5.33 | 5.45 | 5.02 | 83.45 |
India | 4.58 | 5.94 | 5.48 | 7.07 | 6.14 | 6.3 | 6.62 | 5.44 | 6.38 | 6.0 | 17.24 | 4.89 | 6.09 | 6.3 | 5.53 | 82.76 |
Pakistan | 4.92 | 5.64 | 5.57 | 5.86 | 5.63 | 5.64 | 5.73 | 5.58 | 5.78 | 5.9 | 5.99 | 21.38 | 5.57 | 5.51 | 5.29 | 78.62 |
Germany | 4.47 | 5.37 | 4.77 | 6.78 | 5.51 | 5.5 | 6.09 | 5.39 | 5.58 | 5.31 | 5.89 | 4.42 | 16.41 | 10.98 | 7.56 | 83.59 |
UK | 4.43 | 5.44 | 4.87 | 6.99 | 5.46 | 5.66 | 6.12 | 5.49 | 5.75 | 5.34 | 6.0 | 4.29 | 10.83 | 16.14 | 7.19 | 83.86 |
USA | 4.78 | 5.41 | 5.15 | 6.36 | 5.42 | 5.54 | 5.7 | 5.52 | 5.66 | 5.49 | 5.86 | 4.61 | 8.33 | 8.01 | 18.16 | 81.84 |
TO | 62.84 | 83.75 | 77.48 | 96.82 | 81.65 | 88.79 | 95.65 | 80.52 | 90.18 | 86.09 | 82.41 | 63.65 | 86.65 | 88.12 | 76.87 | TCI |
NET | −16.98 | 0.82 | −4.25 | 11.79 | −0.89 | 5.1 | 10.76 | −2.11 | 6.09 | 2.64 | −0.36 | −14.97 | 3.06 | 4.25 | −4.97 | 82.76 |
Note, TCI is the total connectedness index. |
Indonesia | Malaysia | Philippines | Singapore | Thailand | China | Hong Kong | Japan | Korea | Taiwan | India | Pakistan | Germany | UK | USA | FROM | |
Indonesia | 6.5 | 6.6 | 6.72 | 6.72 | 6.57 | 6.66 | 6.92 | 6.44 | 6.49 | 6.48 | 6.66 | 6.67 | 6.78 | 6.74 | 7.03 | 93.5 |
Malaysia | 6.26 | 6.89 | 6.73 | 6.74 | 6.57 | 6.67 | 6.91 | 6.41 | 6.47 | 6.47 | 6.67 | 6.68 | 6.77 | 6.76 | 7.01 | 93.11 |
Philippines | 6.24 | 6.58 | 7.02 | 6.73 | 6.57 | 6.71 | 6.9 | 6.42 | 6.47 | 6.46 | 6.68 | 6.7 | 6.74 | 6.74 | 7.03 | 92.98 |
Singapore | 6.23 | 6.63 | 6.7 | 6.99 | 6.57 | 6.69 | 6.94 | 6.42 | 6.46 | 6.48 | 6.66 | 6.69 | 6.77 | 6.76 | 7.01 | 93.01 |
Thailand | 6.24 | 6.61 | 6.72 | 6.72 | 6.88 | 6.69 | 6.9 | 6.42 | 6.51 | 6.49 | 6.65 | 6.67 | 6.76 | 6.74 | 7.0 | 93.12 |
China | 6.21 | 6.58 | 6.7 | 6.73 | 6.57 | 6.97 | 7.0 | 6.41 | 6.48 | 6.49 | 6.67 | 6.65 | 6.76 | 6.74 | 7.03 | 93.03 |
Hong Kong | 6.24 | 6.56 | 6.69 | 6.74 | 6.56 | 6.77 | 7.15 | 6.42 | 6.5 | 6.49 | 6.64 | 6.65 | 6.78 | 6.76 | 7.05 | 92.85 |
Japan | 6.23 | 6.58 | 6.69 | 6.71 | 6.61 | 6.67 | 6.88 | 6.71 | 6.5 | 6.49 | 6.62 | 6.7 | 6.79 | 6.75 | 7.08 | 93.29 |
Korea | 6.25 | 6.58 | 6.71 | 6.71 | 6.59 | 6.7 | 6.92 | 6.43 | 6.78 | 6.5 | 6.65 | 6.67 | 6.75 | 6.73 | 7.01 | 93.22 |
Taiwan | 6.23 | 6.58 | 6.72 | 6.71 | 6.59 | 6.7 | 6.92 | 6.45 | 6.53 | 6.75 | 6.64 | 6.69 | 6.73 | 6.72 | 7.03 | 93.25 |
India | 6.26 | 6.56 | 6.68 | 6.73 | 6.59 | 6.69 | 6.92 | 6.42 | 6.48 | 6.49 | 6.95 | 6.67 | 6.77 | 6.75 | 7.04 | 93.05 |
Pakistan | 6.26 | 6.56 | 6.73 | 6.71 | 6.58 | 6.66 | 6.9 | 6.41 | 6.47 | 6.48 | 6.66 | 7.03 | 6.76 | 6.75 | 7.03 | 92.97 |
Germany | 6.25 | 6.54 | 6.69 | 6.73 | 6.58 | 6.68 | 6.88 | 6.43 | 6.47 | 6.45 | 6.65 | 6.66 | 7.05 | 6.86 | 7.06 | 92.95 |
UK | 6.23 | 6.58 | 6.67 | 6.72 | 6.57 | 6.65 | 6.89 | 6.42 | 6.48 | 6.45 | 6.66 | 6.67 | 6.88 | 7.04 | 7.08 | 92.96 |
USA | 6.23 | 6.54 | 6.71 | 6.73 | 6.58 | 6.67 | 6.89 | 6.42 | 6.49 | 6.46 | 6.65 | 6.67 | 6.81 | 6.79 | 7.37 | 92.63 |
TO | 87.36 | 92.08 | 93.88 | 94.14 | 92.1 | 93.62 | 96.77 | 89.93 | 90.82 | 90.7 | 93.16 | 93.44 | 94.84 | 94.59 | 98.5 | TCI |
NET | −6.14 | −1.04 | 0.9 | 1.13 | −1.01 | 0.59 | 3.92 | −3.36 | −2.4 | −2.55 | 0.11 | 0.47 | 1.89 | 1.63 | 5.87 | 93.06 |
Indonesia | Malaysia | Philippines | Singapore | Thailand | China | Hong Kong | Japan | Korea | Taiwan | India | Pakistan | Germany | UK | USA | FROM | |
Indonesia | 52.66 | 5.11 | 3.86 | 5.82 | 3.85 | 3.72 | 4.58 | 1.85 | 3.48 | 3.06 | 3.15 | 1.14 | 2.61 | 2.67 | 2.44 | 47.34 |
Malaysia | 0.92 | 55.67 | 3.17 | 6.67 | 4.4 | 3.45 | 3.8 | 1.85 | 3.84 | 3.81 | 2.94 | 1.14 | 2.71 | 2.62 | 3.02 | 44.33 |
Philippines | 1.1 | 3.79 | 60.54 | 4.35 | 3.5 | 3.17 | 3.66 | 1.64 | 2.54 | 2.91 | 2.48 | 1.46 | 2.66 | 2.55 | 3.67 | 39.46 |
Singapore | 0.74 | 5.26 | 2.88 | 42.95 | 4.35 | 5.22 | 8.1 | 2.97 | 4.9 | 4.29 | 4.07 | 1.42 | 4.28 | 5.01 | 3.57 | 57.05 |
Thailand | 1.25 | 4.39 | 3.06 | 5.72 | 57.05 | 3.65 | 4.48 | 1.85 | 3.15 | 2.68 | 3.41 | 1.42 | 2.88 | 2.84 | 2.17 | 42.95 |
China | 0.65 | 2.88 | 2.22 | 5.35 | 2.95 | 47.93 | 15.28 | 1.97 | 4.76 | 4.23 | 2.91 | 1.3 | 2.37 | 2.64 | 2.56 | 52.07 |
Hong Kong | 0.78 | 2.92 | 2.53 | 7.88 | 3.61 | 13.98 | 40.18 | 2.44 | 5.78 | 4.85 | 2.96 | 1.15 | 3.2 | 3.52 | 4.23 | 59.82 |
Japan | 0.87 | 2.17 | 1.89 | 4.18 | 2.53 | 2.95 | 3.8 | 58.89 | 4.29 | 2.64 | 1.95 | 1.09 | 3.97 | 3.58 | 5.19 | 41.11 |
Korea | 0.77 | 3.59 | 2.1 | 5.83 | 2.79 | 5.13 | 6.47 | 3.6 | 49.35 | 7.11 | 2.86 | 1.28 | 2.78 | 3.23 | 3.1 | 50.65 |
Taiwan | 0.81 | 3.65 | 2.69 | 5.19 | 2.52 | 4.98 | 6.17 | 2.29 | 7.55 | 52.21 | 2.42 | 1.55 | 2.48 | 2.52 | 2.97 | 47.79 |
India | 1.0 | 2.93 | 2.11 | 5.07 | 3.39 | 3.58 | 4.08 | 1.74 | 3.42 | 2.65 | 58.5 | 1.68 | 3.33 | 3.69 | 2.84 | 41.5 |
Pakistan | 0.8 | 1.74 | 1.7 | 2.12 | 1.9 | 1.74 | 1.82 | 1.2 | 1.72 | 1.82 | 2.06 | 76.25 | 1.73 | 1.56 | 1.84 | 23.75 |
Germany | 0.98 | 1.9 | 1.28 | 4.41 | 2.27 | 2.18 | 2.73 | 1.67 | 1.98 | 1.6 | 2.69 | 1.24 | 48.49 | 18.95 | 7.63 | 51.51 |
UK | 0.95 | 1.83 | 1.37 | 5.02 | 2.42 | 2.42 | 2.93 | 1.7 | 2.21 | 1.73 | 3.06 | 0.99 | 18.61 | 48.37 | 6.4 | 51.63 |
USA | 0.87 | 2.05 | 1.88 | 3.34 | 2.19 | 2.1 | 2.25 | 1.7 | 1.81 | 1.74 | 2.4 | 1.19 | 8.8 | 7.63 | 60.05 | 39.95 |
TO | 12.48 | 44.2 | 32.75 | 70.96 | 42.65 | 58.26 | 70.16 | 28.47 | 51.4 | 45.11 | 39.35 | 18.06 | 62.42 | 63.0 | 51.61 | TCI |
NET | −34.86 | −0.12 | −6.71 | 13.9 | −0.29 | 6.19 | 10.35 | −12.64 | 0.76 | −2.68 | −2.15 | −5.69 | 10.9 | 11.37 | 11.67 | 46.06 |
Indonesia | Malaysia | Philippines | Singapore | Thailand | China | Hong Kong | Japan | Korea | Taiwan | India | Pakistan | Germany | UK | USA | FROM | |
Indonesia | 38.55 | 6.53 | 3.75 | 7.07 | 6.61 | 4.08 | 4.99 | 2.4 | 4.84 | 3.68 | 4.11 | 2.07 | 3.52 | 3.55 | 4.23 | 61.45 |
Malaysia | 2.54 | 42.35 | 3.19 | 7.69 | 6.48 | 4.3 | 4.53 | 2.33 | 4.94 | 3.77 | 3.54 | 2.12 | 3.71 | 3.62 | 4.9 | 57.65 |
Philippines | 2.33 | 4.92 | 51.07 | 4.9 | 4.88 | 3.37 | 3.89 | 2.28 | 3.67 | 3.26 | 3.02 | 2.03 | 3.13 | 2.99 | 4.27 | 48.93 |
Singapore | 2.06 | 6.43 | 2.9 | 35.27 | 6.21 | 5.06 | 7.89 | 2.95 | 5.82 | 4.32 | 4.12 | 2.01 | 4.73 | 4.96 | 5.28 | 64.73 |
Thailand | 2.84 | 5.73 | 3.23 | 6.51 | 45.19 | 4.07 | 4.9 | 2.67 | 4.82 | 3.17 | 3.95 | 2.32 | 3.67 | 3.25 | 3.66 | 54.81 |
China | 1.61 | 4.21 | 2.44 | 5.88 | 4.64 | 40.01 | 13.32 | 2.35 | 5.12 | 4.1 | 3.65 | 1.99 | 3.22 | 3.33 | 4.14 | 59.99 |
Hong Kong | 1.74 | 4.2 | 2.73 | 8.36 | 5.17 | 12.07 | 33.67 | 2.6 | 6.26 | 4.72 | 3.3 | 1.93 | 3.79 | 3.87 | 5.59 | 66.33 |
Japan | 1.91 | 3.26 | 2.46 | 4.58 | 4.54 | 3.05 | 3.73 | 49.74 | 4.66 | 3.24 | 2.92 | 1.67 | 4.55 | 3.93 | 5.75 | 50.26 |
Korea | 2.04 | 4.95 | 2.51 | 6.55 | 5.5 | 4.93 | 6.2 | 3.5 | 38.98 | 6.52 | 3.46 | 2.19 | 3.79 | 3.68 | 5.18 | 61.02 |
Taiwan | 1.97 | 4.38 | 2.7 | 5.63 | 4.42 | 4.85 | 5.81 | 2.76 | 7.83 | 43.24 | 3 | 2.05 | 3.27 | 3.42 | 4.67 | 56.76 |
India | 2.01 | 4.15 | 2.45 | 5.6 | 5.45 | 4.03 | 4.28 | 2.42 | 4.24 | 3.16 | 48.51 | 2.2 | 3.84 | 3.78 | 3.88 | 51.49 |
Pakistan | 2.01 | 3.45 | 2.41 | 3.69 | 3.83 | 2.57 | 2.93 | 1.79 | 3.29 | 2.73 | 2.79 | 60.71 | 2.57 | 2.18 | 3.05 | 39.29 |
Germany | 1.6 | 3.07 | 1.73 | 5.12 | 4.01 | 2.71 | 3.25 | 2.31 | 3.14 | 2.11 | 3.21 | 1.73 | 40.47 | 16.98 | 8.59 | 59.53 |
UK | 1.46 | 3.21 | 1.48 | 5.62 | 3.78 | 2.91 | 3.32 | 2.07 | 3.11 | 2.37 | 3.36 | 1.47 | 16.99 | 40.98 | 7.87 | 59.02 |
USA | 2.09 | 3.8 | 2.26 | 4.74 | 3.85 | 2.84 | 3.07 | 2.08 | 3.58 | 2.63 | 2.79 | 2.21 | 8.49 | 7.76 | 47.82 | 52.18 |
TO | 28.2 | 62.29 | 36.24 | 81.93 | 69.37 | 60.83 | 72.1 | 34.51 | 65.32 | 49.78 | 47.23 | 27.99 | 69.28 | 67.29 | 71.07 | TCI |
NET | −33.25 | 4.63 | −12.69 | 17.2 | 14.56 | 0.84 | 5.78 | −15.74 | 4.31 | −6.97 | −4.26 | −11.3 | 9.74 | 8.27 | 18.89 | 56.23 |
Descriptive Statistics | Indonesia | Malaysia | Philippines | Singapore | Thailand | China | Hong Kong | Japan | Korea | Taiwan | India | Pakistan | Germany | UK | USA |
Mean | 0.008 | −0.005 | 0.000 | 0.004 | −0.006 | 0.031 | 0.011 | 0.000 | 0.020 | 0.008 | 0.024 | −0.023 | 0.013 | 0.008 | 0.026 |
Variance | 5.874 | 1.994 | 2.618 | 1.953 | 4.069 | 3.483 | 2.063 | 1.863 | 4.880 | 2.324 | 2.531 | 3.267 | 2.349 | 1.718 | 1.475 |
Skewness | −1.027*** | 0.638*** | 0.195*** | 0.222*** | 0.469*** | 0.085*** | 0.000 | 0.011 | 0.234*** | −0.086*** | −0.345*** | −0.462*** | −0.233*** | −0.389*** | −0.406*** |
Ex.Kurtosis | 31.17*** | 42.30*** | 12.66*** | 11.48*** | 13.26*** | 6.741*** | 9.578*** | 4.369*** | 14.87*** | 3.518*** | 8.708*** | 9.552*** | 6.070*** | 10.83*** | 9.932*** |
ERS | −35.40*** | −32.59*** | −17.80*** | −22.64*** | −11.44*** | −22.84*** | −30.99*** | −29.85*** | −36.90*** | −13.21*** | −11.16*** | −28.98*** | −36.74*** | −39.56*** | −33.28*** |
Q(20) | 181.8*** | 182.1*** | 151.7*** | 92.82*** | 191.9*** | 65.55*** | 31.92*** | 41.19*** | 129.4*** | 45.13*** | 74.27*** | 88.77*** | 25.50*** | 65.88*** | 73.96*** |
Q2(20) | 2824.0*** | 2778.0*** | 786.1*** | 2433.1*** | 2236.2*** | 3538.6*** | 2876.7*** | 1950.6*** | 3600.2*** | 1380.3*** | 1338.9*** | 1959.5*** | 2526.9*** | 3251.2*** | 5708.5*** |
Pearson Corelation | Indonesia | Malaysia | Philippines | Singapore | Thailand | China | Hong Kong | Japan | Korea | Taiwan | India | Pakistan | Germany | UK | USA |
Indonesia | 1.000*** | 0.118*** | 0.200*** | 0.078*** | 0.124*** | 0.046*** | 0.054*** | 0.053*** | 0.038*** | 0.062*** | 0.032*** | 0.026** | −0.006 | −0.015 | −0.001 |
Malaysia | 0.118*** | 1.000*** | 0.345*** | 0.488*** | 0.414*** | 0.307*** | 0.405*** | 0.265*** | 0.328*** | 0.314*** | 0.256*** | 0.125*** | 0.192*** | 0.223*** | 0.066*** |
Philippines | 0.200*** | 0.345*** | 1.000*** | 0.416*** | 0.364*** | 0.303*** | 0.406*** | 0.294*** | 0.320*** | 0.318*** | 0.257*** | 0.081*** | 0.168*** | 0.200*** | 0.077*** |
Singapore | 0.078*** | 0.488*** | 0.416*** | 1.000*** | 0.511*** | 0.458*** | 0.671*** | 0.412*** | 0.457*** | 0.447*** | 0.409*** | 0.110*** | 0.407*** | 0.439*** | 0.235*** |
Thailand | 0.124*** | 0.414*** | 0.364*** | 0.511*** | 1.000*** | 0.313*** | 0.441*** | 0.268*** | 0.363*** | 0.316*** | 0.300*** | 0.104*** | 0.257*** | 0.273*** | 0.143*** |
China | 0.046*** | 0.307*** | 0.303*** | 0.458*** | 0.313*** | 1.000*** | 0.637*** | 0.307*** | 0.352*** | 0.389*** | 0.346*** | 0.081*** | 0.261*** | 0.286*** | 0.165*** |
Hong Kong | 0.054*** | 0.405*** | 0.406*** | 0.671*** | 0.441*** | 0.637*** | 1.000*** | 0.422*** | 0.483*** | 0.466*** | 0.392*** | 0.111*** | 0.343*** | 0.375*** | 0.182*** |
Japan | 0.053*** | 0.265*** | 0.294*** | 0.412*** | 0.268*** | 0.307*** | 0.422*** | 1.000*** | 0.399*** | 0.364*** | 0.211*** | 0.059*** | 0.202*** | 0.224*** | 0.063*** |
Korea | 0.038*** | 0.328*** | 0.320*** | 0.457*** | 0.363*** | 0.352*** | 0.483*** | 0.399*** | 1.000*** | 0.468*** | 0.324*** | 0.079*** | 0.264*** | 0.290*** | 0.148*** |
Taiwan | 0.062*** | 0.314*** | 0.318*** | 0.447*** | 0.316*** | 0.389*** | 0.466*** | 0.364*** | 0.468*** | 1.000*** | 0.307*** | 0.106*** | 0.237*** | 0.249*** | 0.120*** |
India | 0.032*** | 0.256*** | 0.257*** | 0.409*** | 0.300*** | 0.346*** | 0.392*** | 0.211*** | 0.324*** | 0.307*** | 1.000*** | 0.116*** | 0.321*** | 0.351*** | 0.204*** |
Pakistan | 0.026** | 0.125*** | 0.081*** | 0.110*** | 0.104*** | 0.081*** | 0.111*** | 0.059*** | 0.079*** | 0.106*** | 0.116*** | 1.000*** | 0.050*** | 0.063*** | 0.023 |
Germany | −0.006 | 0.192*** | 0.168*** | 0.407*** | 0.257*** | 0.261*** | 0.343*** | 0.202*** | 0.264*** | 0.237*** | 0.321*** | 0.050*** | 1.000*** | 0.806*** | 0.540*** |
UK | −0.015 | 0.223*** | 0.200*** | 0.439*** | 0.273*** | 0.286*** | 0.375*** | 0.224*** | 0.290*** | 0.249*** | 0.351*** | 0.063*** | 0.806*** | 1.000*** | 0.502*** |
USA | −0.001 | 0.066*** | 0.077*** | 0.235*** | 0.143*** | 0.165*** | 0.182*** | 0.063*** | 0.148*** | 0.120*** | 0.204*** | 0.023 | 0.540*** | 0.502*** | 1.000*** |
Note, ***, ** indicate significance at 1% and 5% significance levels. For normality test, skewness is based on D'Agostino (1970) test, while kurtosis is based on Anscombe and Glynn (1983) test. The Elliott, Rothenberg and Stock (ERS) unit root test is detailed in Ellliot et al. (1992). The Q and Q2 portmanteau tests serial correlation in returns were based on Fisher and Gallagher (2012), and these were computed for up to 20 lags in returns. |
Indonesia | Malaysia | Philippines | Singapore | Thailand | China | Hong Kong | Japan | Korea | Taiwan | India | Pakistan | Germany | UK | USA | FROM | |
Indonesia | 20.18 | 5.85 | 5.97 | 5.99 | 5.49 | 5.59 | 5.85 | 5.87 | 5.83 | 5.59 | 5.74 | 4.96 | 5.75 | 5.79 | 5.55 | 79.82 |
Malaysia | 4.53 | 17.07 | 6.06 | 7.57 | 6.48 | 6.2 | 6.57 | 5.74 | 6.67 | 6.45 | 5.86 | 4.52 | 5.54 | 5.68 | 5.06 | 82.93 |
Philippines | 4.99 | 6.45 | 18.27 | 6.81 | 6.28 | 6.25 | 6.55 | 5.76 | 6.22 | 6.23 | 5.75 | 4.78 | 5.17 | 5.37 | 5.13 | 81.73 |
Singapore | 4.14 | 6.73 | 5.71 | 14.97 | 6.33 | 6.61 | 7.95 | 5.92 | 6.85 | 6.41 | 6.22 | 4.22 | 6.18 | 6.47 | 5.29 | 85.03 |
Thailand | 4.41 | 6.64 | 6.0 | 7.24 | 17.46 | 6.25 | 6.77 | 5.58 | 6.23 | 5.95 | 6.17 | 4.66 | 5.74 | 5.76 | 5.13 | 82.54 |
China | 4.13 | 5.89 | 5.59 | 7.01 | 5.8 | 16.31 | 9.9 | 5.67 | 6.94 | 6.58 | 5.9 | 4.38 | 5.36 | 5.58 | 4.95 | 83.69 |
Hong Kong | 4.07 | 5.88 | 5.53 | 8.01 | 5.95 | 9.43 | 15.12 | 5.93 | 7.21 | 6.81 | 5.83 | 4.18 | 5.59 | 5.68 | 4.79 | 84.88 |
Japan | 4.82 | 5.92 | 5.58 | 6.82 | 5.68 | 6.16 | 6.82 | 17.37 | 7.18 | 6.43 | 5.52 | 4.65 | 5.76 | 5.92 | 5.36 | 82.63 |
Korea | 4.32 | 6.28 | 5.51 | 7.25 | 5.78 | 6.88 | 7.54 | 6.56 | 15.92 | 7.59 | 5.93 | 4.42 | 5.4 | 5.62 | 5.01 | 84.08 |
Taiwan | 4.26 | 6.3 | 5.71 | 7.05 | 5.7 | 6.79 | 7.42 | 6.08 | 7.91 | 16.55 | 5.76 | 4.67 | 5.33 | 5.45 | 5.02 | 83.45 |
India | 4.58 | 5.94 | 5.48 | 7.07 | 6.14 | 6.3 | 6.62 | 5.44 | 6.38 | 6.0 | 17.24 | 4.89 | 6.09 | 6.3 | 5.53 | 82.76 |
Pakistan | 4.92 | 5.64 | 5.57 | 5.86 | 5.63 | 5.64 | 5.73 | 5.58 | 5.78 | 5.9 | 5.99 | 21.38 | 5.57 | 5.51 | 5.29 | 78.62 |
Germany | 4.47 | 5.37 | 4.77 | 6.78 | 5.51 | 5.5 | 6.09 | 5.39 | 5.58 | 5.31 | 5.89 | 4.42 | 16.41 | 10.98 | 7.56 | 83.59 |
UK | 4.43 | 5.44 | 4.87 | 6.99 | 5.46 | 5.66 | 6.12 | 5.49 | 5.75 | 5.34 | 6.0 | 4.29 | 10.83 | 16.14 | 7.19 | 83.86 |
USA | 4.78 | 5.41 | 5.15 | 6.36 | 5.42 | 5.54 | 5.7 | 5.52 | 5.66 | 5.49 | 5.86 | 4.61 | 8.33 | 8.01 | 18.16 | 81.84 |
TO | 62.84 | 83.75 | 77.48 | 96.82 | 81.65 | 88.79 | 95.65 | 80.52 | 90.18 | 86.09 | 82.41 | 63.65 | 86.65 | 88.12 | 76.87 | TCI |
NET | −16.98 | 0.82 | −4.25 | 11.79 | −0.89 | 5.1 | 10.76 | −2.11 | 6.09 | 2.64 | −0.36 | −14.97 | 3.06 | 4.25 | −4.97 | 82.76 |
Note, TCI is the total connectedness index. |
Indonesia | Malaysia | Philippines | Singapore | Thailand | China | Hong Kong | Japan | Korea | Taiwan | India | Pakistan | Germany | UK | USA | FROM | |
Indonesia | 6.5 | 6.6 | 6.72 | 6.72 | 6.57 | 6.66 | 6.92 | 6.44 | 6.49 | 6.48 | 6.66 | 6.67 | 6.78 | 6.74 | 7.03 | 93.5 |
Malaysia | 6.26 | 6.89 | 6.73 | 6.74 | 6.57 | 6.67 | 6.91 | 6.41 | 6.47 | 6.47 | 6.67 | 6.68 | 6.77 | 6.76 | 7.01 | 93.11 |
Philippines | 6.24 | 6.58 | 7.02 | 6.73 | 6.57 | 6.71 | 6.9 | 6.42 | 6.47 | 6.46 | 6.68 | 6.7 | 6.74 | 6.74 | 7.03 | 92.98 |
Singapore | 6.23 | 6.63 | 6.7 | 6.99 | 6.57 | 6.69 | 6.94 | 6.42 | 6.46 | 6.48 | 6.66 | 6.69 | 6.77 | 6.76 | 7.01 | 93.01 |
Thailand | 6.24 | 6.61 | 6.72 | 6.72 | 6.88 | 6.69 | 6.9 | 6.42 | 6.51 | 6.49 | 6.65 | 6.67 | 6.76 | 6.74 | 7.0 | 93.12 |
China | 6.21 | 6.58 | 6.7 | 6.73 | 6.57 | 6.97 | 7.0 | 6.41 | 6.48 | 6.49 | 6.67 | 6.65 | 6.76 | 6.74 | 7.03 | 93.03 |
Hong Kong | 6.24 | 6.56 | 6.69 | 6.74 | 6.56 | 6.77 | 7.15 | 6.42 | 6.5 | 6.49 | 6.64 | 6.65 | 6.78 | 6.76 | 7.05 | 92.85 |
Japan | 6.23 | 6.58 | 6.69 | 6.71 | 6.61 | 6.67 | 6.88 | 6.71 | 6.5 | 6.49 | 6.62 | 6.7 | 6.79 | 6.75 | 7.08 | 93.29 |
Korea | 6.25 | 6.58 | 6.71 | 6.71 | 6.59 | 6.7 | 6.92 | 6.43 | 6.78 | 6.5 | 6.65 | 6.67 | 6.75 | 6.73 | 7.01 | 93.22 |
Taiwan | 6.23 | 6.58 | 6.72 | 6.71 | 6.59 | 6.7 | 6.92 | 6.45 | 6.53 | 6.75 | 6.64 | 6.69 | 6.73 | 6.72 | 7.03 | 93.25 |
India | 6.26 | 6.56 | 6.68 | 6.73 | 6.59 | 6.69 | 6.92 | 6.42 | 6.48 | 6.49 | 6.95 | 6.67 | 6.77 | 6.75 | 7.04 | 93.05 |
Pakistan | 6.26 | 6.56 | 6.73 | 6.71 | 6.58 | 6.66 | 6.9 | 6.41 | 6.47 | 6.48 | 6.66 | 7.03 | 6.76 | 6.75 | 7.03 | 92.97 |
Germany | 6.25 | 6.54 | 6.69 | 6.73 | 6.58 | 6.68 | 6.88 | 6.43 | 6.47 | 6.45 | 6.65 | 6.66 | 7.05 | 6.86 | 7.06 | 92.95 |
UK | 6.23 | 6.58 | 6.67 | 6.72 | 6.57 | 6.65 | 6.89 | 6.42 | 6.48 | 6.45 | 6.66 | 6.67 | 6.88 | 7.04 | 7.08 | 92.96 |
USA | 6.23 | 6.54 | 6.71 | 6.73 | 6.58 | 6.67 | 6.89 | 6.42 | 6.49 | 6.46 | 6.65 | 6.67 | 6.81 | 6.79 | 7.37 | 92.63 |
TO | 87.36 | 92.08 | 93.88 | 94.14 | 92.1 | 93.62 | 96.77 | 89.93 | 90.82 | 90.7 | 93.16 | 93.44 | 94.84 | 94.59 | 98.5 | TCI |
NET | −6.14 | −1.04 | 0.9 | 1.13 | −1.01 | 0.59 | 3.92 | −3.36 | −2.4 | −2.55 | 0.11 | 0.47 | 1.89 | 1.63 | 5.87 | 93.06 |
Indonesia | Malaysia | Philippines | Singapore | Thailand | China | Hong Kong | Japan | Korea | Taiwan | India | Pakistan | Germany | UK | USA | FROM | |
Indonesia | 52.66 | 5.11 | 3.86 | 5.82 | 3.85 | 3.72 | 4.58 | 1.85 | 3.48 | 3.06 | 3.15 | 1.14 | 2.61 | 2.67 | 2.44 | 47.34 |
Malaysia | 0.92 | 55.67 | 3.17 | 6.67 | 4.4 | 3.45 | 3.8 | 1.85 | 3.84 | 3.81 | 2.94 | 1.14 | 2.71 | 2.62 | 3.02 | 44.33 |
Philippines | 1.1 | 3.79 | 60.54 | 4.35 | 3.5 | 3.17 | 3.66 | 1.64 | 2.54 | 2.91 | 2.48 | 1.46 | 2.66 | 2.55 | 3.67 | 39.46 |
Singapore | 0.74 | 5.26 | 2.88 | 42.95 | 4.35 | 5.22 | 8.1 | 2.97 | 4.9 | 4.29 | 4.07 | 1.42 | 4.28 | 5.01 | 3.57 | 57.05 |
Thailand | 1.25 | 4.39 | 3.06 | 5.72 | 57.05 | 3.65 | 4.48 | 1.85 | 3.15 | 2.68 | 3.41 | 1.42 | 2.88 | 2.84 | 2.17 | 42.95 |
China | 0.65 | 2.88 | 2.22 | 5.35 | 2.95 | 47.93 | 15.28 | 1.97 | 4.76 | 4.23 | 2.91 | 1.3 | 2.37 | 2.64 | 2.56 | 52.07 |
Hong Kong | 0.78 | 2.92 | 2.53 | 7.88 | 3.61 | 13.98 | 40.18 | 2.44 | 5.78 | 4.85 | 2.96 | 1.15 | 3.2 | 3.52 | 4.23 | 59.82 |
Japan | 0.87 | 2.17 | 1.89 | 4.18 | 2.53 | 2.95 | 3.8 | 58.89 | 4.29 | 2.64 | 1.95 | 1.09 | 3.97 | 3.58 | 5.19 | 41.11 |
Korea | 0.77 | 3.59 | 2.1 | 5.83 | 2.79 | 5.13 | 6.47 | 3.6 | 49.35 | 7.11 | 2.86 | 1.28 | 2.78 | 3.23 | 3.1 | 50.65 |
Taiwan | 0.81 | 3.65 | 2.69 | 5.19 | 2.52 | 4.98 | 6.17 | 2.29 | 7.55 | 52.21 | 2.42 | 1.55 | 2.48 | 2.52 | 2.97 | 47.79 |
India | 1.0 | 2.93 | 2.11 | 5.07 | 3.39 | 3.58 | 4.08 | 1.74 | 3.42 | 2.65 | 58.5 | 1.68 | 3.33 | 3.69 | 2.84 | 41.5 |
Pakistan | 0.8 | 1.74 | 1.7 | 2.12 | 1.9 | 1.74 | 1.82 | 1.2 | 1.72 | 1.82 | 2.06 | 76.25 | 1.73 | 1.56 | 1.84 | 23.75 |
Germany | 0.98 | 1.9 | 1.28 | 4.41 | 2.27 | 2.18 | 2.73 | 1.67 | 1.98 | 1.6 | 2.69 | 1.24 | 48.49 | 18.95 | 7.63 | 51.51 |
UK | 0.95 | 1.83 | 1.37 | 5.02 | 2.42 | 2.42 | 2.93 | 1.7 | 2.21 | 1.73 | 3.06 | 0.99 | 18.61 | 48.37 | 6.4 | 51.63 |
USA | 0.87 | 2.05 | 1.88 | 3.34 | 2.19 | 2.1 | 2.25 | 1.7 | 1.81 | 1.74 | 2.4 | 1.19 | 8.8 | 7.63 | 60.05 | 39.95 |
TO | 12.48 | 44.2 | 32.75 | 70.96 | 42.65 | 58.26 | 70.16 | 28.47 | 51.4 | 45.11 | 39.35 | 18.06 | 62.42 | 63.0 | 51.61 | TCI |
NET | −34.86 | −0.12 | −6.71 | 13.9 | −0.29 | 6.19 | 10.35 | −12.64 | 0.76 | −2.68 | −2.15 | −5.69 | 10.9 | 11.37 | 11.67 | 46.06 |
Indonesia | Malaysia | Philippines | Singapore | Thailand | China | Hong Kong | Japan | Korea | Taiwan | India | Pakistan | Germany | UK | USA | FROM | |
Indonesia | 38.55 | 6.53 | 3.75 | 7.07 | 6.61 | 4.08 | 4.99 | 2.4 | 4.84 | 3.68 | 4.11 | 2.07 | 3.52 | 3.55 | 4.23 | 61.45 |
Malaysia | 2.54 | 42.35 | 3.19 | 7.69 | 6.48 | 4.3 | 4.53 | 2.33 | 4.94 | 3.77 | 3.54 | 2.12 | 3.71 | 3.62 | 4.9 | 57.65 |
Philippines | 2.33 | 4.92 | 51.07 | 4.9 | 4.88 | 3.37 | 3.89 | 2.28 | 3.67 | 3.26 | 3.02 | 2.03 | 3.13 | 2.99 | 4.27 | 48.93 |
Singapore | 2.06 | 6.43 | 2.9 | 35.27 | 6.21 | 5.06 | 7.89 | 2.95 | 5.82 | 4.32 | 4.12 | 2.01 | 4.73 | 4.96 | 5.28 | 64.73 |
Thailand | 2.84 | 5.73 | 3.23 | 6.51 | 45.19 | 4.07 | 4.9 | 2.67 | 4.82 | 3.17 | 3.95 | 2.32 | 3.67 | 3.25 | 3.66 | 54.81 |
China | 1.61 | 4.21 | 2.44 | 5.88 | 4.64 | 40.01 | 13.32 | 2.35 | 5.12 | 4.1 | 3.65 | 1.99 | 3.22 | 3.33 | 4.14 | 59.99 |
Hong Kong | 1.74 | 4.2 | 2.73 | 8.36 | 5.17 | 12.07 | 33.67 | 2.6 | 6.26 | 4.72 | 3.3 | 1.93 | 3.79 | 3.87 | 5.59 | 66.33 |
Japan | 1.91 | 3.26 | 2.46 | 4.58 | 4.54 | 3.05 | 3.73 | 49.74 | 4.66 | 3.24 | 2.92 | 1.67 | 4.55 | 3.93 | 5.75 | 50.26 |
Korea | 2.04 | 4.95 | 2.51 | 6.55 | 5.5 | 4.93 | 6.2 | 3.5 | 38.98 | 6.52 | 3.46 | 2.19 | 3.79 | 3.68 | 5.18 | 61.02 |
Taiwan | 1.97 | 4.38 | 2.7 | 5.63 | 4.42 | 4.85 | 5.81 | 2.76 | 7.83 | 43.24 | 3 | 2.05 | 3.27 | 3.42 | 4.67 | 56.76 |
India | 2.01 | 4.15 | 2.45 | 5.6 | 5.45 | 4.03 | 4.28 | 2.42 | 4.24 | 3.16 | 48.51 | 2.2 | 3.84 | 3.78 | 3.88 | 51.49 |
Pakistan | 2.01 | 3.45 | 2.41 | 3.69 | 3.83 | 2.57 | 2.93 | 1.79 | 3.29 | 2.73 | 2.79 | 60.71 | 2.57 | 2.18 | 3.05 | 39.29 |
Germany | 1.6 | 3.07 | 1.73 | 5.12 | 4.01 | 2.71 | 3.25 | 2.31 | 3.14 | 2.11 | 3.21 | 1.73 | 40.47 | 16.98 | 8.59 | 59.53 |
UK | 1.46 | 3.21 | 1.48 | 5.62 | 3.78 | 2.91 | 3.32 | 2.07 | 3.11 | 2.37 | 3.36 | 1.47 | 16.99 | 40.98 | 7.87 | 59.02 |
USA | 2.09 | 3.8 | 2.26 | 4.74 | 3.85 | 2.84 | 3.07 | 2.08 | 3.58 | 2.63 | 2.79 | 2.21 | 8.49 | 7.76 | 47.82 | 52.18 |
TO | 28.2 | 62.29 | 36.24 | 81.93 | 69.37 | 60.83 | 72.1 | 34.51 | 65.32 | 49.78 | 47.23 | 27.99 | 69.28 | 67.29 | 71.07 | TCI |
NET | −33.25 | 4.63 | −12.69 | 17.2 | 14.56 | 0.84 | 5.78 | −15.74 | 4.31 | −6.97 | −4.26 | −11.3 | 9.74 | 8.27 | 18.89 | 56.23 |