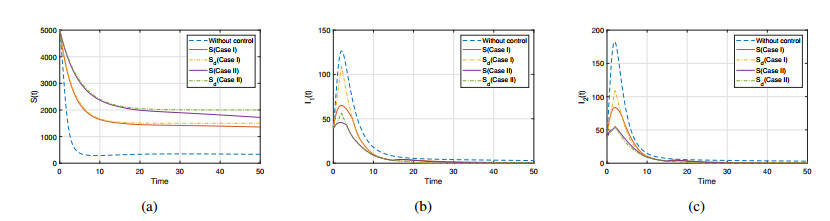
This paper aims to predict companies' financial distress situation with the use of four different models; Altman Z score, Revised Altman Z Score (Linear Discriminant Analysis) and Quadratic Discriminant Analysis, Random Forest Machine Learning Model with the use of same variables suggested by Altman. Also a review of Altman Z score model for Turkey case is assessed whether it is applicable to Turkish companies and how accurate the results are. This study differentiates itself from the previous studies by the content of the data; it includes both publicly traded companies and private companies. Additionally, there are only a few studies are applied for random forest model in bankruptcy prediction in Turkey. For this reason, it aims to fill the gap from both a rare used model and originality of the data. The data consisted of the 80 firms' financial ratio analysis between the years 2013 to 2018. There are 44 firms are listed in BIST; remaining 36 firms are private firms with the size of small and micro enterprises. Random forest model with use of Altman variables has shown 95% performance and surpassed the other three models. Moreover, the classification results for publicly traded companies was 100% for Random Forest whereas other models have shown greater performance for private firms than publicly traded firms.
Citation: Zeynep Cındık, Ismail H. Armutlulu. A revision of Altman Z-Score model and a comparative analysis of Turkish companies' financial distress prediction[J]. National Accounting Review, 2021, 3(2): 237-255. doi: 10.3934/NAR.2021012
[1] | Ya-Dong Zhang, Hai-Feng Huo, Hong Xiang . Dynamics of tuberculosis with fast and slow progression and media coverage. Mathematical Biosciences and Engineering, 2019, 16(3): 1150-1170. doi: 10.3934/mbe.2019055 |
[2] | H. M. Srivastava, Khaled M. Saad, J. F. Gómez-Aguilar, Abdulrhman A. Almadiy . Some new mathematical models of the fractional-order system of human immune against IAV infection. Mathematical Biosciences and Engineering, 2020, 17(5): 4942-4969. doi: 10.3934/mbe.2020268 |
[3] | Chaoyue Wang, Zhiyao Ma, Shaocheng Tong . Adaptive fuzzy output-feedback event-triggered control for fractional-order nonlinear system. Mathematical Biosciences and Engineering, 2022, 19(12): 12334-12352. doi: 10.3934/mbe.2022575 |
[4] | Tao-Li Kang, Hai-Feng Huo, Hong Xiang . Dynamics and optimal control of tuberculosis model with the combined effects of vaccination, treatment and contaminated environments. Mathematical Biosciences and Engineering, 2024, 21(4): 5308-5334. doi: 10.3934/mbe.2024234 |
[5] | Eduardo Ibargüen-Mondragón, M. Victoria Otero-Espinar, Miller Cerón Gómez . A within-host model on the interaction dynamics between innate immune cells and Mycobacterium tuberculosis. Mathematical Biosciences and Engineering, 2025, 22(3): 511-527. doi: 10.3934/mbe.2025019 |
[6] | Jimin Yu, Jiajun Yin, Shangbo Zhou, Saiao Huang, Xianzhong Xie . An image super-resolution reconstruction model based on fractional-order anisotropic diffusion equation. Mathematical Biosciences and Engineering, 2021, 18(5): 6581-6607. doi: 10.3934/mbe.2021326 |
[7] | Cristiana J. Silva, Helmut Maurer, Delfim F. M. Torres . Optimal control of a Tuberculosis model with state and control delays. Mathematical Biosciences and Engineering, 2017, 14(1): 321-337. doi: 10.3934/mbe.2017021 |
[8] | Shuai Zhang, Yongqing Yang, Xin Sui, Yanna Zhang . Synchronization of fractional-order memristive recurrent neural networks via aperiodically intermittent control. Mathematical Biosciences and Engineering, 2022, 19(11): 11717-11734. doi: 10.3934/mbe.2022545 |
[9] | Georgi Kapitanov . A double age-structured model of the co-infection of tuberculosis and HIV. Mathematical Biosciences and Engineering, 2015, 12(1): 23-40. doi: 10.3934/mbe.2015.12.23 |
[10] | Wenjie Qin, Jiamin Zhang, Zhengjun Dong . Media impact research: a discrete SIR epidemic model with threshold switching and nonlinear infection forces. Mathematical Biosciences and Engineering, 2023, 20(10): 17783-17802. doi: 10.3934/mbe.2023790 |
This paper aims to predict companies' financial distress situation with the use of four different models; Altman Z score, Revised Altman Z Score (Linear Discriminant Analysis) and Quadratic Discriminant Analysis, Random Forest Machine Learning Model with the use of same variables suggested by Altman. Also a review of Altman Z score model for Turkey case is assessed whether it is applicable to Turkish companies and how accurate the results are. This study differentiates itself from the previous studies by the content of the data; it includes both publicly traded companies and private companies. Additionally, there are only a few studies are applied for random forest model in bankruptcy prediction in Turkey. For this reason, it aims to fill the gap from both a rare used model and originality of the data. The data consisted of the 80 firms' financial ratio analysis between the years 2013 to 2018. There are 44 firms are listed in BIST; remaining 36 firms are private firms with the size of small and micro enterprises. Random forest model with use of Altman variables has shown 95% performance and surpassed the other three models. Moreover, the classification results for publicly traded companies was 100% for Random Forest whereas other models have shown greater performance for private firms than publicly traded firms.
TB is a deadly infectious disease and one of the world's top ten causes of death [1,2,3]. Most of the infected individuals can be cured by adopting a systematical diagnostic and using appropriate antibiotics. However, the impact of TB on human health and social development still cannot be ignored due to the lack of knowledge about TB prevention and diagnosis and the low treatment coverage in some areas. According to the global tuberculosis report 2021 published by the World Health Organization [4], about 9.9 million people worldwide suffered from TB in 2020, of which more than 1.3 million died. The massive destruction caused by TB to the economy and society forces us to understand the transmission mechanism of TB deeply.
Mathematical models play an essential role in understanding the dynamics of infectious diseases, including TB [5,6,7,8,9,10]. Exploring the transmission mechanism of tuberculosis is the first step to establishing a mathematical model, so references [11,12,13] have studied the transmission dynamics of tuberculosis. In addition, Castillo-Chavez and Feng [14] formulated one-strain and two-strain TB models to analyze the effects of fundamental epidemiological factors. Subsequently, the TB compartment model was proposed by Feng et al. [15] and is considered one of the basic frameworks of the transmission mechanism of TB. The authors in [16] established the TB models with fast and slow dynamics by considering multi-level contact structure. Castillo-Chavez and Song [17] comprehensively summarized the research trends of the tuberculosis dynamic models and control problems in the past decades, and proposed some exciting and challenging questions. It should be pointed out that the research results of [15] indicate that it is not enough to reduce the basic reproduction number to less than 1 for the prevention and control of TB. After that, the researchers further discussed the influence of various factors on the transmission of TB by proposing different dynamical models [18,19,20,21,22,23,24,25]. For example, Hattaf et al. [23] established a TB disease model with exogenous reinfection, and studied the optimal control problem to reduce the infectious group by using Pontryagin's maximum principle. The multi-drug resistance, and case detection and treatment were considered in [24] and [25], respectively. Meanwhile, based on the actual infection data of TB in the United States from 1988 to 2019, the authors in [26] established an SVEITR dynamical model by considering vaccination, incomplete treatment and relapse, and analyzed the sensitivity of basic reproduction number and solved the optimal control problem issue by using Pontryagin's maximum principle.
It should be pointed out that the models in all of the literature mentioned above are established by using the conventional integer-order calculus, which is a local concept. Fractional-order calculus, as an extension of integer-order one, is a global concept that can more effectively characterize the hereditary and memory properties of many physical processes and materials [27,28]. Therefore, some scholars try to describe the spread and outbreak of infectious disease by using the fractional dynamics model [29,30,31,32,33,34]. The results show that the fractional order model can better fit the actual data. Although the fractional-order model has the above advantages, the control of the fractional TB model is still in the ascendant due to less application of fractional calculus in biology, which is one of the motivations of the current paper.
Adaptive control is one of the important control methods in engineering [35,36]. Its characteristic is that the control target can be given in advance, and then the control variable can be designed so that the state variable or the related variable of the model can track the control target with small errors, so as to realize the control of the model. Recently, some results adopt the adaptive control method to realize the control of biology [37,38,39]. In particular, the authors in [39] studied the nonlinear adaptive control of COVID-19. However, the system parameters are estimated by the adaptive laws in [37,38,39], which are heavily dependent on the initial values of adaptive laws. Therefore, how to reduce this dependency is also one of the motivations of this paper.
Motivated by the above discussions, the problem of nonlinear neural networks control is studied for the fractional-order TB model. The main contributions of this paper are summarized as follows: (ⅰ) By analyzing the transmission mechanism of TB and considering the historical dependence on the spreading of TB, a fractional-order TB model under Caputo fractional derivative is established. Compared with the model in [33], in this paper we consider that the proportion of smear-positive and smear-negative from susceptible population is not equal to that from latent population, and the patients after rehabilitation may become susceptible ones again. (ⅱ) This is a successful attempt to apply the universal approximation principle of radial basis function neural networks to biological model, and thus the controllers for fractional-order TB model are proposed and the initial values dependency of adaptive laws are also reduced. Different from [37,38,39], where the model parameters are estimated by adaptive laws, the unknown functions that include model parameters are approximated in this paper, and thus the processes of estimations to model parameters are avoided.
This paper is organized as follows. The problem to be studied is formulated and some preliminaries are presented in Section 2. The adaptive neural networks controllers are designed and the stability of controlled model is also analyzed in Section 3. Some numerical examples are given in Section 4 to illustrate the proposed controllers. Finally, a brief summary is presented in Section 5.
Definition 1. [40] The Caputo derivative of function f(t)∈Cn+1([t0,+∞),R) with the order α is given as follows
Dαf(t)=1Γ(n−α)∫tt0f(n)(τ)(t−τ)α+1−ndτ, |
where Γ(⋅) denotes Gamma function, n∈N+ and n−1<α≤n.
Definition 2. [40] For α>0, the Mittag-Leffler function with a single parameter is defined as Eα(z)=∑∞i=0ziΓ(αi+1), where z is plural. In particular, E1(z)=ez.
Lemma 1. [40] Let the function f(t)∈C1([t0,+∞),R), then Dαf2(t)≤2f(t)Dαf(t) for t≥t0 and α∈(0,1].
Lemma 2. Consider the system Dαx(t)=φ(t)−x(t) with initial value x(0)>0. If 0<α<1 and function φ(t)(t≥0) is a non-negative and bounded continuous function, then the solution x(t) of this system is positive and bounded.
Proof. This Lemma can be proven by using the similar method in [44,Lemma 5], so we omit it here.
Lemma 3. [41] For a given approximation accuracy ˜ϵ>0, any continuous function f(x) defined on a compact set can be approximated by the radial basis function neural networks W∗TΨ(x) as follows
f(x)=W∗TΨ(x)+ϵ(x),|ϵ(x)|≤˜ϵ, |
where W∗=argminW[supx|f(x)−WTΨ(x)|], x represents the input vector, W∗=(w1,⋯,wl)T∈Rl denotes the ideal weight vector, l>1 represents the nodes number, Ψ(x)=(Ψ1(x),⋯,Ψl(x))T, Ψi(x) is selected as Ψi(x)=exp[−(x−σi)T(x−σi)/κ2] for i=1,⋯,l, where σi=(σi1,⋯,σin)T and κ are the center and width of the Gaussian function, respectively.
Since it usually takes four to eight weeks from the time TB enters the body to the time it shows symptoms, and people with TB can have either smear positive or smear negative. Therefore, the people carrying mycobacterium TB is divided into latent population (E), smear positive population (I1) and smear negative population (I2). The rest of the population is classified as susceptible (S) and immune or quarantined due to cure (R). Based on the above division, literature [33] established a dynamic model of TB, discussed the global stability of disease-free equilibrium point and endemic equilibrium point, and analyzed the impact of smear-negative individuals on TB transmission. Considering that the proportion of smear-positive and smear-negative from S is not equal to that from E, and the patients after rehabilitation may become susceptible ones again, we improve the model in [33] to the following one
{˙S(t)=Π−(β1I1+β2I2)S−μS+λR,˙E(t)=(1−k)(β1I1+β2I2)S−(μ+m)E,˙I1(t)=p1k(β1I1+β2I2)S+q1mE−(γ1+δ1+μ)I1,˙I2(t)=p2k(β1I1+β2I2)S+q2mE−(γ2+δ2+μ)I2,˙R(t)=γ1I1+γ2I2−(μ+λ)R, | (2.1) |
where Π represents the input rate of the population, β1 and β2 represent the infection rates of smear positive and negative population to susceptible population respectively, μ represents the natural mortality rate, γ1 and γ2 are the cure rates of smear positive and negative population respectively, δ1 and δ2 are the disease-related death rates of smear positive and negative infected persons respectively, λ denotes the transfer rate of the immune or quarantined population that loses immunity or released from quarantine and becomes susceptible again, m represents the transfer rate from latent population to infected population, k represents the proportion of susceptible people who become infected directly after carrying TB bacteria (p1 and p2 represent the proportion of smear positive and negative respectively), q1 and q2 represent the proportion of smear positive and negative respectively in the people who enter the infected population from the latent population. All parameters in model (2.1) are positive except 0≤p1,p2,q1,q2≤1 and p1+p2=q1+q2=1. The initial values of model (2.1) are S(0)>0,E(0)>0,I1(0)>0,I2(0)>0,R(0)>0.
In practice, the infection rate of the bacteria and the transfer rate of the population among the compartments depend on the infection rate and transfer rate at each historical moment. That is, it has heritability and memory, which is completely ignored by the integer-order derivative adopted in model (2.1). It is worth noting that the fractional-order derivatives can better describe the genetic and memory characteristics of the process or materials, so the fractional-order compartmental model can be established to better describe the transmission process of mycobacterium TB bacteria in the population based on model (2.1). On the other hand, effective and feasible control measures need to be taken to control the spread of the bacteria in the population. Usually, media coverage for susceptible population to reduce the infection rate and treatment for infected population are the two most commonly used control measures, which are introduced into the fractional-order compartmental model to obtain the following fractional-order control model
{DαS(t)=Π−(β1I1+β2I2)S(1−c1u1)−μS+λR,DαE(t)=(1−k)(β1I1+β2I2)S(1−c1u1)−(μ+m)E,DαI1(t)=p1k(β1I1+β2I2)S(1−c1u1)+q1mE−(γ1+δ1+μ)I1−c2u2I11+α1I1,DαI2(t)=p2k(β1I1+β2I2)S(1−c1u1)+q2mE−(γ2+δ2+μ)I2−c3u3I21+α2I2,DαR(t)=γ1I1+γ2I2+c2u2I11+α1I1+c3u3I21+α2I2−(μ+λ)R, | (2.2) |
where α∈(0,1), c1,c2,c3∈[0,1] are the effective rate of control measures, u1,u2,u3∈[0,1] denote the intensity of control measures, α1,α2>0 are half-satiation constants. The reasons why we set u1(t),u2(t),u3(t) as control variables are summarized as follows:
(ⅰ) Media coverage for susceptible population (u1): after the media coverage to susceptible population, people can take scientific and effective protective measures to reduce the infection rate of the bacteria, which is described by (1−c1u1) in (2.2).
(ⅱ) Treatment to smear positive infected population (u2) and smear negative infected population (u3): due to the limited medical resources, the saturation treatment rate is used to characterize the treatment control measures.
Remark 1. In references [29,30,31,32], fractional-order TB models were established by considering different factors that influence the transmission of TB, where the fractional derivatives with nonsingular kernel were adopted, while the Caputo fractional derivative is used in model (2.2). Although the Caputo fractional derivative contains a singular kernel, this does not affect the main purpose of this paper: extend the adaptive control and neural networks approximation method widely used in the engineering fields to the biological field, and design control variables for the fractional-order TB model. It should be pointed out that the new and interesting definition of fractional derivative [27,28,42] proposed in recent years can effectively overcome some shortcomings of the traditional fractional derivative, but the stability theory related to these new definition is still relatively lacking, which may lead to difficulties in analyzing the stability of the controlled TB model.
The control objectives of this paper are as follows: The appropriate expressions of u1,u2,u3 in model (2.2) are designed so that the susceptible population S, smear positive population I1 and smear negative population I2 can track the control targets Sd, I1d and I2d with small errors, respectively. It is assumed that Sd,I1d,I2d and their fractional-order derivative with order α are all bounded and continuously differentiable. In order to achieve the control goal, the following lemma is given.
Lemma 4. Ω={(S,E,I1,I2,R)|S≥0,E≥0,I1≥0,I2≥0,R≥0,S+E+I1+I2+R≤Πμ} is a positive invariant set of models (2.1) and (2.2).
Proof. Lemma 4 can be proved by using similar methods of [43,Theorems 1 and 2], so omitted here.
In this section, the controllers are designed for the controlled model (2.2) through three steps, and then the stability of the control system is analyzed.
For convenience, the control errors are defined as follows
˜S=S−Sd,˜I1=I1−I1d,˜I2=I2−I2d. |
Step 1: The Lyapunov function V1 is constructed as
V1=12˜S2+12ξ−11˜θ21, | (3.1) |
where ξ1>0 is a design parameter, ˜θ1=ˆθ1−θ1, ˆθ1 is the estimate of θ1=‖W∗1‖2, W∗1 will be defined later. Based on lemma 1, the fractional-order derivative of the function V1 is
DαV1≤˜SDα˜S+ξ−11˜θ1Dα˜θ1≤˜S[Π−(β1I1+β2I2)S(1−c1u1)−μS+λR−DαSd]+ξ−11˜θ1Dα˜θ1=˜S[c1u1S(β1I1+β2I2)−DαSd+F1(X1)]+ξ−11˜θ1Dα˜θ1, |
where F1(X1)=Π−(β1I1+β2I2)S−μS+λR, X1=(S,I1,I2,R)T. From Lemma 4, we know that X1 belongs to a compact set, so continuous function F1(X1) can be approximated by radial basis function neural networks. According to Lemma 3, F1(X1) can be approximated as F1(X1)=W∗1Ψ1(X1)+ϵ1(X1), ϵ1(X1) is the approximation error and satisfies |ϵ1(X1)|<˜ϵ1. Using Young's inequality, we get
˜S(W∗1Ψ1(X1)+ϵ1(X1))≤|˜S|(‖W∗1‖‖Ψ1(X1)‖+˜ϵ1)≤12a21˜S2θ1ΨT1(X1)Ψ1(X1)+12a21+12˜S2+12˜ϵ21. |
Then, DαV1 can be written as
DαV1≤˜S[c1u1S(β1I1+β2I2)+12a21˜Sθ1ΨT1(X1)Ψ1(X1)+12˜S−DαSd]+12a21+12˜ϵ21+ξ−11˜θ1Dα˜θ1. | (3.2) |
The controller u1 and Dαˆθ1 are designed as
u1=1c1S(β1I1+β2I2)[−(ρ1+0.5)˜S−12a21˜Sˆθ1ΨT1(X1)Ψ1(X1)+DαSd], | (3.3) |
Dαˆθ1=ξ12a21˜S2ΨT1(X1)Ψ1(X1)−ˆθ1, | (3.4) |
where ρ1>0 is a design parameter. By substituting (3.3) and (3.4) into (3.2) and noting that ˜θ1=ˆθ1−θ1, we can obtain
DαV1≤−ρ1˜S2−ξ−11˜θ1ˆθ1+12a21+12˜ϵ21. |
Further, since −˜θ1ˆθ1=−˜θ21−˜θ1θ1≤−12˜θ21+12θ21, we get
DαV1≤−ρ1˜S2−12ξ−11˜θ21+Δ1, | (3.5) |
where Δ1=12ξ−11θ21+12a21+12˜ϵ21.
Step 2: The Lyapunov function V2 is constructed as
V2=12˜I21+12ξ−12˜θ22, | (3.6) |
where ξ2>0 is a design parameter, ˜θ2=ˆθ2−θ2, ˆθ2 is the estimate of θ2=‖W∗2‖2, W∗2 will be defined later. Applying Young's inequality and noticing that u1∈[0,1], we can obtain
˜I1p1k(β1I1+β2I2)S(1−u1)≤12˜I21+p21k2(β1I1+β2I2)2S2+12(1−u1)2≤12˜I21+p21k2(β1I1+β2I2)2S2+12. | (3.7) |
According to Lemma 1 and using (3.7), we can easily obtain
DαV2≤˜I1[−c1u2I11+α1I1−DαI1d+F2(X2)]+ξ−12˜θ2Dα˜θ2+12, |
where F2(X2)=12˜I1p21k2(β1I1+β2I2)2S2+q1mE−(γ1+δ1+μ)I1, X2=(S,E,I1,I2,I1d)T. The function F2(X2) can be approximated by radial basis function neural networks because it is continuous and X2 belongs to a compact set. According to Lemma 3, F2(X2) can be approximated as F2(X2)=W∗2Ψ2(X2)+ϵ2(X2), where |ϵ2(X2)|<˜ϵ2 is the approximation error. Using Young's inequality, we get
˜I1F2(X2)≤|˜I1|(‖W∗2‖‖Ψ2(X2)‖+˜ϵ2)≤12a22˜I21θ2ΨT2(X2)Ψ2(X2)+12a22+12˜I21+12˜ϵ22, |
where a2>0 is a constant. Then, we have
DαV2≤˜I1[−c1u2I21+α1I1−DαI1d+12a22˜I1θ2ΨT2(X2)Ψ2(X2)+12˜I1]+ξ−12˜θ2Dα˜θ2+12+12a22+12˜ϵ22. |
Design the controller u2 and adaptive law of ˆθ2 as
u2=1+α1I1c1I1[(ρ2+12)˜I1+12a22˜I1ˆθ2ΨT2(X2)Ψ2(X2)−DαI1d], | (3.8) |
Dαˆθ2=ξ22a22˜I21ΨT2(X2)Ψ2(X2)−ˆθ2, | (3.9) |
where ρ2>0 is a design parameter. Substituting (3.8), (3.9) into DαV2, and using ˜θ2=ˆθ2−θ2 and −˜θ2ˆθ2≤−12˜θ22+12θ22, we can get
DαV2≤−ρ2˜I21−12ξ−12˜θ22+Δ2, | (3.10) |
where Δ2=12ξ−12θ22+12+12a22+12˜ϵ22.
Step 3: Similar to step 2, construct the Lyapunov function V3 as
V3=12˜I22+12ξ−13˜θ23, | (3.11) |
where ξ3>0 is a designed parameter, ˜θ3=ˆθ3−θ3, ˆθ3 is the estimate of θ3=‖W∗3‖2, W∗3 is the weight vector. Similarly, we can design u3 and Dαˆθ3 as
u3=1+α2I2c2I2[(ρ3+12)˜I2+12a23˜I2ˆθ3ΨT3(X3)Ψ3(X3)−DαI2d], | (3.12) |
Dαˆθ3=ξ32a23˜I22ΨT3(X3)Ψ3(X3)−ˆθ3. | (3.13) |
where ρ3>0 is a design parameter, X3=(S,E,I1,I2,I2d)T. Using lemma 1, 3 and Young's inequality, we have
DαV3≤−ρ3˜I22−12ξ−13˜θ23+Δ3, | (3.14) |
where Δ3=12ξ−13θ23+12+12a23+12˜ϵ23.
Remark 2. In previous works [37,38,39], the vectors to be estimated by adaptive laws were constructed by using the model parameters, with the result that the initial values of adaptive laws have an important influence on the estimated value of the parameter vector. However, in this paper, the functions that contain model parameters are approximated by neural networks, and then the norms of weight vectors are estimated by adaptive laws, so it avoids the estimations to model parameters. Thus, the initial values dependency of adaptive laws are reduced.
Theorem 1. For control model (2.2), the designed controllers (3.3), (3.8), (3.12) and the adaptive laws (3.4), (3.9), (3.13) can ensure that S, I1 and I2 track the control target Sd, I1d and I2d with small errors, respectively. That is, the control goal can be achieved.
Proof. According to (3.1), (3.6) and (3.11), constructing Lyapunov function as V(t)=V1+V2+V3. Thus, based on (3.5), (3.10) and (3.14), we have
DαV≤−ρ1˜S2−ρ2˜I21−ρ3˜I22−123∑i=1ξ−1i˜θ2i+Δ≤−ρV+Δ, |
where ρ=min{2ρ1,2ρ2,2ρ3,1} and Δ=Δ1+Δ2+Δ3 are positive constants. Since there is 0≤Eα(−ρtα)<1 for any t≥0, according to [44,Lemma 5], it can be obtained
V(t)≤(V(0)−Δρ)Eα(−ρtα)+Δρ≤V(0)Eα(−ρtα)+Δρ. | (3.15) |
From the form of Lyapunov function V1,V2,V3, limt→∞Eα(−ρtα)=0 and (3.15), the control errors ˜S, ˜I1 and ˜I2 can enter into a small neighborhood of the origin by choosing appropriate parameters Δ and ρ. In other words, under the designed control variables, the control objective of TB model (2.2) can be achieved with small error.
Remark 3. According to Eqs (3.3), (3.8) and (3.12), the values of u1,u2,u3 will lie outside the interval [0,1] at some time, so, the value of u1,u2,u3 can be adjusted as follows
ui(t)={1,ui(t)>1,ui(t),0≤ui(t)≤1,0,ui(t)<0,i=1,2,3. |
The above adjustment is reasonable, which is mainly because the restriction on the range of control variables will reduce the control effect of the model to a certain extent, but will not affect the stability of the model, which is explained as follows by taking the "Step 1" in Subsection 3.1 as an example. In fact, if u1 is restrict into interval [0,1], then based on Lemma 4 and the assumptions for Sd in Subsection 2.2, we can conclude that the term c1u1S(β1I1+β2I2)+12˜Sθ1ΨT1(Z1)Ψ1(Z1)+12˜S−DαSd+ρ1˜S is bounded by a positive constant ζ1. Thus, (3.2) can be rewritten as
DαV1≤|˜S|ζ1+˜S[−12˜S˜θ1ΨT1(Z1)Ψ1(Z1)+12˜S˜θ1ΨT1(Z1)Ψ1(Z1)−ρ1˜S]+12a21+12˜ϵ21+ξ−11˜θ1Dα˜θ1. |
According to Lemma 2, we know that ˆθ1 is bounded and thus ˜θ1=ˆθ1−θ is bounded, which means that 12˜S˜θ1ΨT1(Z1)Ψ1(Z1) is bounded. Further, by using the boundedness of ˜S, DαV1 can be calculated as
DαV1≤−ρ1˜S2−12ξ−11˜θ21+ζ2, |
where ζ2>0 is a constant. Therefore, the stability of model (2.2) can be ensured by using the similar argument as in Subsection 3.2. Besides, this restriction also makes it possible to use the control measures in practice, because the control input outside the interval [0,1] is meaningless in practice.
Remark 4. Although there are many TB models which were built by using different fractional derivative definitions [29,30,31,32], these studies mainly focus on the existence and uniqueness of the solution, dynamic behavior, numerical methods and so on. However, the research results on the control problem of fractional TB model using adaptive control method are relatively scarce. In this paper, we successfully designed the expressions of control variables for the model by using adaptive control method and radial basis function neural networks, and analyzed the stability of the model under control variables.
This section is devoted to illustrating the theoretical results in previous section by some numerical examples.
The values of parameters in model (2.2) are chosen as: α=0.95, Π=1.4,β1=5.07394×10−3,β2=1.01479×10−3, μ=1/66,m=0.001,k=0.001, p1=0.35,p2=0.65, q1=0.4,q2=0.6, γ1=0.2038,γ2=0.4038, δ1=δ2=0.22, c1=0.7,c2=0.8,c3=0.7, α1=1,α2=1. The designed parameters in the controllers are selected as: ρ1=250,ρ2=ρ3=20, a1=a3=1,a2=2, ξ1=ξ2=ξ3=1. The initial values of model (2.2) and adaptive laws (3.4), (3.9) and (3.13) are (S(0),E(0),I1(0),I2(0),R(0))=(5000,80,40,40,20) and (ˆθ1(0),ˆθ2(0),ˆθ3(0))=(10−4,10−5,10−5), respectively. Finally, the desired values are
Iid=Ii(0)e−bit+ri∫tt−τ[pik(β1I1(w)+β2I2(w))×S(w)(1−c1u1(w))+qimE(w)]dw,(i=1,2)Sd=b3+(S(0)−b3)e−b4t, |
where τ=3,r1=0.3,r2=0.2, b1=b2=0.4,b3=1500,b4=0.3. The parameters in radial basis function neural networks are chosen as σi=(−30,−20,−10,0,10,20,30)T,κ=10.
Remark 5. Since the number of infected people at the current time depends on the cumulative number of infected people in the recent period and the initial values, the desired values I1d and I2d are selected as the above forms. Thus, r1 (or r2) represents the proportion of people who enter I1(or I2) from t−τ to t and have not recovered at t; I1(0)e−b1t(or I2(0)e−b2t) refers to the number of patients at the initial time who have not recovered at the current time t. For susceptible populations, our goal is to try to prevent them from contracting the bacteria, that is, to keep as many people as possible in S, which can be achieve by adjusting b3 and b4 in Sd. So, b3 represents the number of people who are still susceptible in the end, and b4 means the rate from S(0) to b3.
Example 1. Tracking performance of S, I1, I2 to Sd, I1d, I2d, respectively.
As presented in Section 2, the control objective of this study is to design the controllers such that S, I1, I2 can track desired values Sd, I1d, I2d with small errors, respectively. Thus, we consider the following two cases to verify the effectiveness of the controller and the tracking performance of S,I1,I2 to Sd,I1d,I2d, respectively: Case Ⅰ, τ=2,r1=0.3,r2=0.3, b1=b2=0.4,b3=1500,b4=0.3; Case Ⅱ, τ=2,r1=0.25,r2=0.2, b1=0.5,b2=0.3,b3=2000,b4=0.2. The main reason for choosing the above parameters is that we hope that most people can change their behavior and enter the compartment R through media coverage, and most people can recover after treatment. The simulation results are presented in Figure 1. From Figure 1, we see that the trajectories of S, I1, I2 can track the desired values Sd, I1d, I2d with small errors in both Case Ⅰ and Ⅱ, respectively, which indicate the effectiveness of the proposed controllers. In addition, compared with the case of without control, the number of infected people decreased significantly in both Case Ⅰ and Ⅱ.
Example 2. The effect of the fractional-order derivative order α.
The order α is an important parameter in fractional-order model (2.2), which can be adjusted based on the actual demand. In order to exhibit the effect of α in model (2.2), we choose α=1 (i.e., integer-order model), 0.9,0.8,0.7,0.6, and run the numerical simulations. The results are shown in Figure 2. It can be seen from Figure 2 that with the decrease of α, the convergence speed of the trajectory of S, I1 and I2 are decreasing, that is, the period of TB epidemic is prolonged. This shows that the fractional-order model is more flexible than the integer-order model and has the ability to meet the needs of different situations.
Example 3. The effect of different control combinations.
The purpose of setting control variables in model (2.2) is to prevent as many people as possible from contracting TB. In order to better understand the role of each control measure, we consider the following five cases: Case Ⅰ, u1,u2,u3=0; Case Ⅱ, u1,u3=0,u2≠0; Case Ⅲ, u1,u2≠0,u3=0; Case Ⅳ, u1=0,u2,u3≠0; Case Ⅴ, u1,u2,u3≠0, where ui=0 denotes ui is in absence, and ui≠0 denotes that control measure ui is adopted (this does not mean that ui has never been equal to zero).
The simulation results are presented in Figure 3. It follows from Figure 3(a) that, compared with the cases without media coverage (i.e., Cases Ⅰ, Ⅱ, Ⅳ), there are more people who can remain in compartment S when media coverage measures are taken for the susceptible people (i.e., Cases Ⅲ, Ⅳ), which shows that media coverage (u1) is an effective control measure to curb the spread of TB. Figure 3(b) shows the exposed individuals under five cases, which also indicate the significance of media coverage. From Figure 3(c), (d), we know that, when only control measures u2 or u3 (i.e., treatment to the smear positive and negative individuals) are adopted, their effect on reducing the number of TB infections is extremely limited. However, when media coverage and treatment measures are adopted at the same time, the infectious scale of TB epidemic can be greatly reduced. Therefore, we can conclude that comprehensive control measures can be more effective than single ones to prevent the large-scale spread of the bacteria when the TB outbreak occurs. Figure 3(e) presents the trajectories of R. The trajectories of control variables u1,u2 and u3 in Case Ⅴ are given in Figure 3(f).
The adaptive neural networks control problem has been investigated for the fractional-order TB model. First, based on the spreading mechanism of TB, a fractional-order compartment model of TB has been established. Then, by using the universal approximation principle of neural networks and constructing Lyapunov functions, adaptive controllers have been designed, which can ensure the control objective can be realized. Finally, some numerical simulations have been presented to illustrate the effectiveness of the proposed controllers. It should be noted that some interesting and meaningful new definitions for fractional-order derivative, including Caputo-Fabrizio, Atangana, Atangana-Baleanu, Losada-Nieto and so on [28,42], have emerged in recent years, which, compared with Caputo derivative adopted in this paper, have nonsingular kernel. Thus, we need to consider the modeling and control problem of TB under these new definition in the future. Meanwhile, some comparisons should be made to explore the advantage and disadvantage of Caputo derivative and other new definitions, which is also one of our future works.
This work was supported by the Natural Science Foundation of China (12201330).
The author declares there is no conflicts of interest.
[1] | Abbas Q, Ahmad AR (2012) Modeling Bankruptcy Prediction for Non-Financial Firms: The Case of Pakistan. SSRN Electron J. |
[2] |
Affes Z, Hentati-Kaffel R (2019) Predicting US Banks Bankruptcy: Logit Versus Canonical Discriminant Analysis. Comput Econ 54: 199–244. doi: 10.1007/s10614-017-9698-0
![]() |
[3] |
Alaka HA, Oyedele LO, Owolabi HA, et al. (2018) Systematic review of bankruptcy prediction models: Towards a framework for tool selection. Expert Syst Appl 94: 164–184. doi: 10.1016/j.eswa.2017.10.040
![]() |
[4] | Altman EI (1968) Financial Ratios, Discriminant Analysis and the Prediction of Corporate Bankruptcy. J Financ 23: 589. |
[5] | Altman EI (1983) Corporate Financial Distress, A Complete Guide to Predciting, Avoiding, and Dealing with Bankruptcy, John Wiley and Sons. |
[6] |
Altman EI, Iwanicz-Drozdowska M, Laitinen EK, et al. (2017) Financial Distress Prediction in an International Context: A Review and Empirical Analysis of Altman's Z-Score Model. J Int Financ Manage Account 28: 131–171. doi: 10.1111/jifm.12053
![]() |
[7] |
Ansari A, Ahmad IS, Bakar AA, et al. (2020) A Hybrid Metaheuristic Method in Training Artificial Neural Network for Bankruptcy Prediction. IEEE Access 8: 176640–176650. doi: 10.1109/ACCESS.2020.3026529
![]() |
[8] |
Balcaen S, Ooghe H (2006) 35 years of studies on business failure: An overview of the classic statistical methodologies and their related problems. Br Account Rev 38: 63–93. doi: 10.1016/j.bar.2005.09.001
![]() |
[9] |
Barboza F, Kimura H, Altman E (2017) Machine learning models and bankruptcy prediction. Expert Syst Appl 83: 405–417. doi: 10.1016/j.eswa.2017.04.006
![]() |
[10] |
Barreda AA, Kageyama Y, Singh D, et al. (2017) Hospitality Bankruptcy in United States of America: A Multiple Discriminant Analysis-Logit Model Comparison. J Quality Assur Hospitality Tourism 18: 86–106. doi: 10.1080/1528008X.2016.1169471
![]() |
[11] | Beaver WH (1966) Financial Ratios As Predictors of Failure. J Account Res 4: 71. |
[12] |
Breiman L (2001) Random Forests. Machine Learn 45: 5–32. doi: 10.1023/A:1010933404324
![]() |
[13] | Çolak S (2019) A New Index Score for the Assessment of Firm Financial Risks. (February), Central Bank of the Republic of Turkey Research Working Papers. Available from: https://www.tcmb.gov.tr/wps/wcm/connect/c3419f04-4318-4042-8692-d33c0d2db6de/wp1904.pdf?MOD=AJPERES&CACHEID=ROOTWORKSPACE-c3419f04-4318-4042-8692-d33c0d2db6de-mA0n3Sq. |
[14] | Diakomihalis MN (2012) The Accuracy of Altman's Models in Predicting Hotel Bankruptcy. Int J Account Financ Report 2: 96. |
[15] |
Dimitras AI, Slowinski R, Susmaga R, et al. (1999) Business failure prediction using rough sets. Eur J Oper Res 114: 263–280. doi: 10.1016/S0377-2217(98)00255-0
![]() |
[16] |
Dixon WJ (1980) Efficient analysis of experimental observations. Annu Rev Pharmacol Toxicol 20: 441–462. doi: 10.1146/annurev.pa.20.040180.002301
![]() |
[17] | Georgiev V, Petrova R (2015) Testing the usefulness and predictive power of the adapted Altman Z-score model for Bulgarian public companies. (Altman 1968), 19–28. |
[18] |
Gordini N (2014) A genetic algorithm approach for SMEs bankruptcy prediction: Empirical evidence from Italy. Expert Syst Appl 41: 6433–6445. doi: 10.1016/j.eswa.2014.04.026
![]() |
[19] | Grammatikos T, Gloubos G (1984) Predicting Bankruptcy of Industrial. J Econ Bus Stat Oper Res 3: 421–423. |
[20] | Huang YP, Yen MF (2019) A new perspective of performance comparison among machine learning algorithms for financial distress prediction. Appl Soft Comput J 83: 105663. |
[21] |
Karas M, Srbová P (2019) Predicting bankruptcy in construction business: Traditional model validation and formulation of a new model. J Int Stud 12: 283–296. doi: 10.14254/2071-8330.2019/12-1/19
![]() |
[22] | Lev B (1973) Decomposition Measures for Financial Analysis. Financ Manage 2: 56. |
[23] |
Luque A, Carrasco A, Martín A, et al. (2019) The impact of class imbalance in classification performance metrics based on the binary confusion matrix. Pattern Recognit 91: 216–231. doi: 10.1016/j.patcog.2019.02.023
![]() |
[24] | Merton RC (1974) On the Pricing of Corporate Debt: The Risk Structure of Interest Rates. J Financ 29: 449. |
[25] | Muzır E, Çağlar N (2009) Finansal Başarısızlık Tahmin Modellerinin Türkiye'de Geçerliliği: Basit Model Önerileriyle Karşılaştırılmalı Bir Araştırma. Anadolu Üniversitesi Sosyal Bilimler Dergisi 9: 15–48. |
[26] | Odom MD, Sharda R (1990) A neural network model for bankruptcy prediction. IJCNN. International Joint Conference on Neural Networks, 163–168. |
[27] | Ohlson JA (1980) Financial Ratios and the Probabilistic Prediction of Bankruptcy. J Account Res 18: 109. |
[28] |
Prabowo SCB (2019) Analysis on the Prediction of Bankruptcy of Cigarette Companies Listed in the Indonesia Stock Exchange Using Altman (Z-Score) Model and Zmijewski (X-Score) Model. J Aplikasi Manaje 17: 254–260. doi: 10.21776/ub.jam.2019.017.02.08
![]() |
[29] | Restianti T, Agustina L (2018) Accounting Analysis Journal The Effect of Financial Ratios on Financial Distress Conditions in Sub Industrial Sector Company. Account Anal J 7: 25–33. |
[30] |
Ruuska S, Hämäläinen W, Kajava S, et al. (2018) Evaluation of the confusion matrix method in the validation of an automated system for measuring feeding behaviour of cattle. Behav Processes 148: 56–62. doi: 10.1016/j.beproc.2018.01.004
![]() |
[31] | Singh G, Singla R (n.d.) Corporate Bankruptcy Prediction Using Altman' S Z -Score Model : the Effect of Time and Methodology on Accuracy of the Model. Ceeol Com 11: 58–72. |
[32] |
Svabova L, Michalkova L, Durica M, et al. (2020) Business failure prediction for Slovak small and medium-sized companies. Sustainability 12: 1–14. doi: 10.3390/su12114572
![]() |
[33] | Theil H (1969) On the use of Information Theory Concepts in the Analysis of Financial Statements. Manage Sci 15: 459–480. |
[34] | Ufo A (2015) Impact of Financial Distress on the Leverage of Selected Manufacturing Firms of Ethiopia. J Proverty Invest Dev 5: 8–13. |
[35] |
Varetto F (1998) Genetic algorithms applications in the analysis of insolvency risk. J Bank Financ 22: 1421–1439. doi: 10.1016/S0378-4266(98)00059-4
![]() |
[36] |
Wang M, Chen H, Li H, et al. (2017) Grey wolf optimization evolving kernel extreme learning machine: Application to bankruptcy prediction. Eng Appl Artif Intell 63: 54–68. doi: 10.1016/j.engappai.2017.05.003
![]() |
[37] | Wang Y, Campbell M (2010) Business failure prediction for publicly listed companies in China. J Bus Manage 16: 75–88. |
[38] | Yıldız B, Ağdeniz Ş (2019) A comparative Study of Machine Learning Algorithms as an Audit Tool in Financial Failure Prediction. Tide Acade Res 1: 11–31. |
[39] | Yılmaz H, Yıldıran M (2015) Borsada İşlem Gören İşletmelerde Mali Başarısızlık Tahmini: Altman Modeli'nin BIST Uygulaması. Aksaray Üniversitesi İktisadi ve İdari Bilimler Fakültesi Dergisi 7: 43–49. |
[40] |
Zelenkov Y, Fedorova E, Chekrizov D (2017) Two-step classification method based on genetic algorithm for bankruptcy forecasting. Expert Syst Appl 88: 393–401. doi: 10.1016/j.eswa.2017.07.025
![]() |
1. | Ariyanatchi M., Vijayalakshmi G.M., Nonlinear Robust Adaptive Sliding Mode Control Strategies Involve a Fractional Ordered Approach to Reducing Dengue Vectors, 2024, 14, 26667207, 100406, 10.1016/j.rico.2024.100406 |