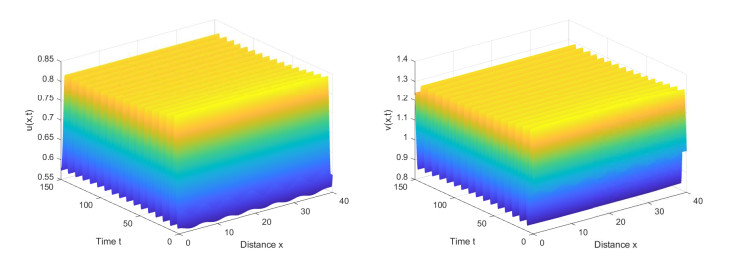
Citation: Siming Liu, Mengxin Wang, Yong Tan. Stabilizing inflation expectations in China: Does economic policy uncertainty matter?[J]. Green Finance, 2019, 1(4): 429-441. doi: 10.3934/GF.2019.4.429
[1] | Zizhuo Wu, Qingshan She, Zhelong Hou, Zhenyu Li, Kun Tian, Yuliang Ma . Multi-source online transfer algorithm based on source domain selection for EEG classification. Mathematical Biosciences and Engineering, 2023, 20(3): 4560-4573. doi: 10.3934/mbe.2023211 |
[2] | Xia Chen, Yexiong Lin, Qiang Qu, Bin Ning, Haowen Chen, Xiong Li . An epistasis and heterogeneity analysis method based on maximum correlation and maximum consistence criteria. Mathematical Biosciences and Engineering, 2021, 18(6): 7711-7726. doi: 10.3934/mbe.2021382 |
[3] | Bo Wang, Yabin Li, Xue Sui, Ming Li, Yanqing Guo . Joint statistics matching for camera model identification of recompressed images. Mathematical Biosciences and Engineering, 2019, 16(5): 5041-5061. doi: 10.3934/mbe.2019254 |
[4] | Shi Liu, Kaiyang Li, Yaoying Wang, Tianyou Zhu, Jiwei Li, Zhenyu Chen . Knowledge graph embedding by fusing multimodal content via cross-modal learning. Mathematical Biosciences and Engineering, 2023, 20(8): 14180-14200. doi: 10.3934/mbe.2023634 |
[5] | Pingping Sun, Yongbing Chen, Bo Liu, Yanxin Gao, Ye Han, Fei He, Jinchao Ji . DeepMRMP: A new predictor for multiple types of RNA modification sites using deep learning. Mathematical Biosciences and Engineering, 2019, 16(6): 6231-6241. doi: 10.3934/mbe.2019310 |
[6] | Ting-Huai Ma, Xin Yu, Huan Rong . A comprehensive transfer news headline generation method based on semantic prototype transduction. Mathematical Biosciences and Engineering, 2023, 20(1): 1195-1228. doi: 10.3934/mbe.2023055 |
[7] | Shizhen Huang, ShaoDong Zheng, Ruiqi Chen . Multi-source transfer learning with Graph Neural Network for excellent modelling the bioactivities of ligands targeting orphan G protein-coupled receptors. Mathematical Biosciences and Engineering, 2023, 20(2): 2588-2608. doi: 10.3934/mbe.2023121 |
[8] | Jun Yan, Tengsheng Jiang, Junkai Liu, Yaoyao Lu, Shixuan Guan, Haiou Li, Hongjie Wu, Yijie Ding . DNA-binding protein prediction based on deep transfer learning. Mathematical Biosciences and Engineering, 2022, 19(8): 7719-7736. doi: 10.3934/mbe.2022362 |
[9] | Sarth Kanani, Shivam Patel, Rajeev Kumar Gupta, Arti Jain, Jerry Chun-Wei Lin . An AI-Enabled ensemble method for rainfall forecasting using Long-Short term memory. Mathematical Biosciences and Engineering, 2023, 20(5): 8975-9002. doi: 10.3934/mbe.2023394 |
[10] | M Kumaresan, M Senthil Kumar, Nehal Muthukumar . Analysis of mobility based COVID-19 epidemic model using Federated Multitask Learning. Mathematical Biosciences and Engineering, 2022, 19(10): 9983-10005. doi: 10.3934/mbe.2022466 |
In nonlinear chemical reaction systems, the three-molecule autocatalytic model shows abundant dynamical behaviors, many abundant research results have been obtained [1,2,3,4,5,6,7]. In 1979, Schnakenberg proposed a typical three-molecule autocatalytic reaction-diffusion model [8]. In [9], the authors studied the one-dimensional static Turing bifurcation of Schnakenberg model. In [10,11,12], the authors introduced the relevant research background of reaction-diffusion Schnakenberg system. However, most references [13,14,15,16] focus on whether the constant equilibrium solution has Turing instability, but pay little attention to whether the periodic solutions of system may also suffer from Turing instability. Therefore, by applying the theoretical methods in [17,18], we study the Turing instability of Hopf bifurcating periodic solutions for the Schnakenberg model.
On the basis of the Schnakenberg model [8], We introduce self-diffusion and cross-diffusion coefficients, and establish reaction-diffusion Schnakenberg model with cross-diffusion and self-diffusion:
{ut−d11Δu−d12Δv=a−u+u2v,x∈Ω,t>0,vt−d21Δv−d22Δv=b−u2v,x∈Ω,t>0,u(x,0)=u∗(x),v(x,0)=v∗(x),x∈Ω,∂u∂ν=∂v∂ν=0,x∈∂Ω,t>0, | (1.1) |
where Ω is a open bounded domain in n-dimensional Euclidean space, and its boundary ∂Ω is smooth. Δ is Laplace operator. The parameters a,b,d11,d12,d21,d22 are all positive constants. u=u(x,t) and v=v(x,t) indicate the concentrations of chemicals at position x∈Ω and time t>0, respectively, and the initial concentrations u∗(x),v∗(x) are nonnegative functions. d11,d22 denote self-diffusion coefficients of u and v, respectively. d12,d21 represent cross-diffusion coefficients of u and v, respectively. Simultaneously, we suppose that d11d22−d12d21>0 holds.
We consider the corresponding zero-dimensional dynamic system of system (1.1)
{dudt=a−u+u2v, t>0,dvdt=b−u2v, t>0,u(0)=u∗>0,v(0)=v∗>0. | (2.1) |
The equilibrium (u0,v0) of system (2.1) satisfies
{a−u+u2v=0,b−u2v=0. |
with u0=a+b,v0=b(a+b)2. By straightforward computation, we know (u0,v0) is the only equilibrium of system (2.1). For convenience, setting μ:=a+b, then (u0,v0)=(μ,bμ2). In the following, for the three-molecule autocatalytic Schnakenberg model, we discuss the stability of its Hopf bifurcating periodic solutions by taking μ as parameter.
Theorem 2.1. Let μH0=3√b+√b2+127+3√b−√b2+127, for the ODEs (2.1), the following statements are true:
(1) At (μ,bμ2)T, system (2.1) is unstable for μ∈(0,μH0), while locally asymptotically stable for μ∈(μH0,+∞).
(2) At λ=μH0, system has a family of periodic solutions (uT(t),vT(t))T bifurcating from (μ,bμ2)T. Supercritical Hopf bifurcation of system (2.1) occurs at (μ,bμ2)T, and the bifurcating periodic solutions are stable.
Proof. The Jacobian matrix of system (2.1) at (μ,vμ)T is J(μ)=(−1+2bμμ2−2bμ−μ2). The characteristic equation of J(μ) is
λ2−T(μ)λ+D(μ)=0, | (2.2) |
with
T(μ)=−μ2−1+2bμ,D(μ)=μ2. |
The eigenvalue λ(μ) of J(μ) is given by
λ(μ)=T(μ)±√T2(μ)−4D(μ)2. |
When μ≥2b, all the eigenvalues of J(μ) have strict negative real parts, according to the stability theory, the equilibrium (μ,bμ2)T is locally asymptotically stable. When 0<μ<2b, T′(μ)=−2μ−2bμ2<0, then T(μ) is monotonically decreasing for 0<μ<2b. Since limμ→0T(μ)=+∞,T(2b)=−4b−12b<0, then T(μ) has only zero point μH0∈(0,2b), namely, T(μH0)=0. For any μ∈(μH0,2b), we have T(μ)<0, then system (2.1) is locally asymptotically stable at (μ,bμ2)T, while for any μ∈(0,μH0), system (2.1) is unstable at (μ,bμ2)T. When μ=μH0, J(μ) has a pair of pure imaginary roots λ=±iω0 with ω0=μ. Let λ(μ)=α(μ)±iω(μ) be the roots of Eq (2.2) near μ=μH0, then we have
α(μ)=−μ22−12+bμ,dα(μ)dμ|μ=μH0<0. |
According to Poincaré-Andronov-Hopf bifurcation theorem, system (2.1) experiences a Hopf bifurcation at μ=μH0.
Next, we study the properties of Hopf bifurcating periodic solutions of system (2.1). Here, we still use the notations and computation in [20] to deduce the expression of cubic term coefficient c1(μH0) in the norm form. By [21], we can rewrite the Poincaré normal form of the abstract form of system (2.1) in the small neighborhood of p0 as follows:
dUdt=J(μH0)U+F(μ,U)|μ=μH0. | (2.3) |
Let the eigenvector of J(μH0) corresponding to the eigenvalue iω0 be q=(a0,b0)T satisfying
J(μH0)q=iω0q,q=(a0,b0)T=(−μH0,μH0−i)T. |
Define inner product in XC:
⟨U1,U2⟩=∫lπ0(ˉu1u2+ˉv1v2)dx, |
where Ui=(ui,vi)T∈XC,i=1,2. Note that ⟨λU1,U2⟩=ˉλ⟨U1,U2⟩, denote the adjoint operator of J(μH0) by J∗(μH0), then the eigenvector of J∗(μH0) corresponding to the eigenvalue −iω0 be q∗=(a∗0,b∗0)T∈XC satisfying
J∗(μH0)q∗=−iω0q∗,<q∗,q>=1,<q∗,ˉq>=0. |
Therefore, q∗=(a∗0,b∗0)T=(−1−iμH02μH0lπ,−i2lπ)T. Performing the spatial decomposition X=Xc⊕Xs, where Xc={zq+ˉzˉq|z∈C},Xs={u∈X|<q∗,u>=0}, then for any U=(u,v)T∈X, there exist z∈C and ω=(ω1,ω2)∈Xs such that (u,v)T=zq+ˉzˉq+(ω1,ω2)T. Thus, system (2.3) can be transformed into the following system with (z,ω) as the coordinate:
{dzdt=iωz+<q∗,F(p,U)|p=p0>,dωdt=L(p0)ω+H(z,ˉz,ω), | (2.4) |
with
{H(z,ˉz,ω)=F(p,U)|p=p0−<q∗,F(p,U)|p=p0>q−<ˉq∗,F(p,U)|p=p0>ˉq,F(p,U)|p=p0=F0(zq+ˉzˉq+ω). | (2.5) |
Writing F0 as
F0(U)=12Q(U,U)+16C(U,U,U)+O(|U|4), | (2.6) |
here, Q,C is a symmetric multilinear form. For convenience, denoting QXY=Q(X,Y),CXYZ=C(X,Y,Z), we calculate Qqq, Qqˉq and Cqqˉq, where
Qqq=(c0d0),Qqˉq=(e0f0),Cqqˉq=(g0h0), |
here, denoting f(u,v)=a−u+u2v,g(u,v)=b−u2v, with
c0=fuua20+2fuva0b0+fvvb20=μH0−3μH03+4μH02i,d0=guua20+2guva0b0+gvvb20=−c0=−μH0+3μH03−4μH02i,e0=fuu|a0|2+fuv(a0¯b0+¯a0b0)+fvv|b0|2=μH0−3μH03,f0=guu |a0|2+guv (a0¯b0+¯a0b0)+gvv|b0|2=−e0=3μH03−μH0,g0=fuuu |a0|2a0+fuuv (2|a0|2b0+a20¯b0)+fuvv(2|b0|2a0+b20¯a0)a=6μH03−2μH02i,h0=guuu |a0|2a0+guuv (2|a0|2b0+a20¯b0)+guvv(2|b0|2a0+b20¯a0)=−g0=−6μH03+2μH02i, |
here, all the partial derivatives of f(u,v) and g(u,v) are evaluated at the bifurcation point (μH0,bμH02)T,
Let
H(z,ˉz,ω)=H202z2+H11zˉz+H022ˉz2+o(|z|3)+o(|z||ω|), | (2.7) |
from (2.5) and (2.6), we can obtain
{H20=Qqq−<q∗,Qqq>q−<ˉq∗,Qqq>ˉqH11=Qqˉq−<q∗,Qqˉq>q−<ˉq∗,Qqˉq>ˉq |
Because system (2.4) has normal manifold, which can be written as follows
ω=ω202z2+ω11zˉz+ω022ˉz2+o(|z|3). | (2.8) |
By (2.7), (2.8) and J(μH0)ω+H(z,ˉz,ω)=dωdt=∂ω∂zdzdt+∂ω∂ˉzdˉzdt, we have
ω20=(2iω0I−J(μH0))−1H20,ω11=−J−1(μH0)H11. |
By calculation, we have
<q∗,Qqq>=−c02μH0=−μH0+3μH03−4μH02i2μH0,<q∗,Qqˉq>=−e02μH0=−μH0+3μH032μH0,<ˉq∗,Cqqˉq>=−g02μH0=−6μH03+2μH02i2μH0,<ˉq∗,Qqq>=−c02μH0=−μH0+3μH03−4μH02i2μH0,<ˉq∗,Qqˉq>=−e02μH0=−μH0+3μH032μH0, |
we can also get H20=0,H11=0, this implies ω20=ω11=0, then
<q∗,Qω11q>=<q∗,Qω20ˉq>=0. |
Thus, we have
c1(μ)=i2ω0<q∗,Qqq>⋅<q∗,Qqˉq>+12<ˉq∗,Cqqˉq>. |
The real part and imaginary part of c1(μH0) are as follows
Rec1(μH0)=Re{i2ω0<q∗,Qqq>⋅<q∗,Qqˉq>+12<ˉq∗,Cqqˉq>}=−12,Imc1(μH0)=Im{i2ω0<q∗,Qqq>⋅<q∗,Qqˉq>+12<ˉq∗,Cqqˉq>}=18μH0−μH04+9μH038. | (2.9) |
By Rec1(μH0)<0, we know that the Hopf bifurcating periodic solutions of system (2.1) are stable at μ=μH0. Additionally, because transversality condition dα(μ)dμ|μ=μH0<0, so the direction of Hopf bifurcation is subcritical.
We introduce the following perturbed system model on the basis of ODEs (2.1)
(1+εd11εd12εd211+εd22)(dudt,dvdt)T=(a−u+u2vb−u2v), | (2.10) |
here, ε is sufficiently small such that (1+εd11εd12εd211+εd22) is reversible. System (2.10) is equivalent to the following system
(dudt,dvdt)T=1N(ε)(1+d22ε−d12ε−d21ε1+d11ε)(a−u+u2vb−u2v), | (2.11) |
where
N(ε):=|(1+d22ε−d12ε−d21ε1+d11ε)|=(d11d22−d12d21)ε2+(d11+d22)ε+1>0. |
Then at (μ,vμ), the Jacobian matrix of system (2.11) is
J(μ,ε):=1N(ε)(a11(μ,ε)a12(μ,ε)a21(μ,ε)a22(μ,ε)), | (2.12) |
with
a11(μ,ε):=(1+d22ε)(−1+2bμ)+d12ε2bμ,a12(μ,ε):=(1+d22ε)μ2+d12εμ2,a21(μ,ε):=−(1+d11ε)2bμ−d21ε(−1+2bμ),a22(μ,ε):=−μ2(1+d11ε)−d21εμ2. | (2.13) |
The characteristic equation corresponding to the jacobian matrix J(μ,ε) is
λ2−H(μ,ε)λ+D(μ,ε)=0, | (2.14) |
where
H(μ,ε)=1N(ε)[(2bμ−1−μ2)+ε(d22(2bμ−1)−μ2d11+d12⋅2bμ−d21⋅μ2)],D(μ,ε)=μ2N(ε). | (2.15) |
Notice that H(μH0,0)=T(μH0)=0 and ∂μH(μ,ε)=T′(μH0)≠0. According to the implicit function existence theorem, there exist a sufficiently small ε0>0 and a continuously differentiable function μHε=μH(ε) such that when ε∈(−ε0,ε0), H(μHε,ε)=0 and μH(0)=μH0 hold. Let λ(με)=β(με)±iω(με) be the characteristic root of Eq 2.14, then when μ→με, we have
β(με)=12H(μ,ε),ω(με)=12√4D(μ,ε)−H2(μ,ε). | (2.16) |
By [18], we have the following lemma.
Lemma 2.1. Assume μ is sufficiently close to μHε, T is the minimum positive period of the stable periodic solution (uT(t),vT(t)) of system (2.1) bifurcating from (μ,vμ), then there exists ε1>0 such that for any ε∈(−ε1,ε1), system (2.10) has a periodic solution (uT(t,ε),vT(t,ε)) depending on ε. Its minimum positive period is T(ε), simultaneously, it satisfies
1) When ε→0, (uT(t,ε),vT(t,ε))→(uT(t),vT(t)) and T(ε)→T.
2) T(ε)=2πω(μHε)(1+(β′(μHε)Im(c1(μHε))ω(μHε)Re(c1(μHε))−ω′(μHε)ω(μHε))(μ−μHε)+O((μ−μHε)2) with
c1(μHε)=i2ω(μHε)(g20(ε)g11(ε)−2|g11(ε)|2−13|g02(ε)|2)+g21(ε)2. |
Theorem 2.2. Suppose that μ is sufficiently close to μHε, (uT(t),vT(t)) is the stable periodic solution of system (2.1), then when ε→0, we have
T′(0)=πμH0(L1(μH0)d11−L2(μH0)d22−L3(μH0)d12−L4(μH0)d21), |
with
L1(μH0)=54−μH202+9μH404,L2(μH0)=(14μH20+9μH204−1)(2bμH0−1)−1,L3(μH0)=(14μH0+9μH304−μH0)2bμH20,L4(μH0)=(μH0−14μH0−9μH304)μH0. |
Proof. According to Lemma 2.1, we have
T′(ε)=−2πω2(μHε)dω(μHε)dε−2πω(μHε)(β′(μHε)Im(c1(μHε))ω(μHε)Re(c1(μHε))−ω′(μHε)ω(μHε))dμHεdε+O(μ−μHε). |
If μ→μHε, then O(μ−μHε)→0, so the sign of T′(ε) is mainly determined by the sign of the first two at ε=0. Next, we calculate the expressions of dμHεdε|ε=0 and dω(μHε)dε|ε=0.
At μ=μHε, by (2.15), we can derive
(2bμHε−1−μHε2)+ε(d22(−1+2bμHε)−μHε2d11+d122bμHε−d21μHε2)=0. | (2.17) |
Differentiating (2.17) with ε, we have
dμHεdε|ε=0=b(μH0)2bμH02+2μH0, | (2.18) |
with
b(μH0)=d22(−1+2bμH0)−μH02d11+d122bμH0−d21μH02. | (2.19) |
According to (2.16),
ω(μ)=12√4D(μ,ε)−H2(μ,ε). |
Differentiating it with μ, we know
ω′(μ)=∂μD(μ,ε)−12H(μ,ε)∂μH(μ,ε)√4D(μ,ε)−H2(μ,ε). |
When μ→μHε, H(μHε,ε)=0 and ∂λD(μHε,ε)=1N(ε)D′(μHε). Hence,
ω′(μH0)=∂λD(μHε,ε)2√D(μHε,ε)|ε=0=D′(μHε)2√N(ε)D(μHε)|ε=0=D′(μ)2√D(μ). | (2.20) |
From ω(μHε)=√D(μHε,ε) and D(μHε,ε)=D(μHε)N(ε), differentiating them with ε, we can obtain
dω(μHε)dε=12√D(μHε,ε)ddε(D(μHε,ε)). | (2.21) |
ddε(D(μHε,ε))=−N′(ε)N2(ε)D(μHε)+ddε(D(μHε))1N(ε). | (2.22) |
When ε=0, we get
−N′(0)N2(0)D(μH0)=−(d11+d22)D(μH0),ddε(D(μHε))1N(ε)|ε=0=D′(μ0)dμHεdε(0). | (2.23) |
Thus, according to (2.22) and (2.23), we have
ddε(D(μHε,ε))|ε=0=−(d11+d22)D(μH0)+b(μH0)2bμH02+2μH0D′(μH0), | (2.24) |
where b(μH0) is defined in (2.19). From (2.21) and (2.24), we can obtain
ddε(ω(μHε))|ε=0=−12√D(μH0)(d11+d22−b(μH0)2bμH02+2μH0D′(μH0)D(μH0)). | (2.25) |
By (2.18), (2.20) and (2.25), we can deduce
T′(0)=πD(μH0)(√D(μH0)d11+√D(μH0)d22+b(μH0)Im(c1(μH0))Re(c1(μH0))). | (2.26) |
At last, substituting (2.19) into (2.26), we can obtain
T′(0)=πμH0(L1(μH0)d11−L2(μH0)d22−L3(μH0)d12−L4(μH0)d21), |
with
L1(μH0)=54−μH202+9μH404,L2(μH0)=(14μH20+9μH204−1)(2bμH0−1)−1,L3(μH0)=(14μH0+9μH304−μH0)2bμH20,L4(μH0)=(μH0−14μH0−9μH304)μH0. |
In this section, applying the theory elaborated in [17], we study stable spatially homogeneous Hopf bifurcating periodic solutions of system (2.1) will become Turing unstable in reaction-diffusion system (1.1) with cross-diffusion. According to the previous discussion, we give the following theorem.
Theorem 3.1. Suppose that μ is sufficiently close to μ0, (uT(t),vT(t)) is stable spatially homogeneous Hopf bifurcating periodic solution of system (2.1) bifurcating from (μ,vμ). If T′(0)<0 and Ω is large enough, then (uT(t),vT(t)) will become Turing unstable in system (1.1) with cross-diffusion.
Proof. Assume that the stable periodic solution of system (2.1) is (uT(t),vT(t)) with minimum positive period T, then the linearized system of (1.1) evaluated at (uT(t),vT(t)) is
(∂ϕ∂t,∂φ∂t)T=diag(DΔϕ,D Δ φ)+JT(t)(ϕ,φ)T, | (3.1) |
where D:=(d11d12d21d22), Δ is Laplace operator, the Jacobian matrix of system (2.1) at (uT(t),vT(t)) is JT(t):=(−1+2uT(t)vT(t)uT2(t)−2uT(t)vT(t)−uT2(t)). Setting βn and ηn(x) be the eigenvalue and eigenfunction of −Δ in Ω with Neumann boundary condition. Let (ϕ,φ)T=(h(t),g(t))T∑∞n=0knηn(x), then
(dhdt,dgdt)T=−τD(h(t)g(t))+JT(t)(h(t)g(t)), | (3.2) |
in which, τ:=βn≥0,n∈N0:={0,1,2⋯}. Assume d11=d12=d21=d22=0, then system (3.2) can be rewritten as
(dhdt,dgdt)T=JT(t)(h(t),g(t))T. | (3.3) |
Let Φ(t) be the fundamental solution matrix of system (3.3) satisfying Φ(0)=I2. Denote λi,i=1,2 as the eigenvalue of Φ(T), the corresponding characteristic function is (Ni,Mi)T, i.e.,
Φ(T)(Ni,Mi)T=λi(Ni,Mi)T, |
then λi is the Floquet multiplier corresponding to the periodic solution (uT(t),vT(t)) of system (3.2). Define
(ϕi(t),ψi(t))T:=Φ(t)(Ni,Mi)T, |
apparently,
(ϕi(0),ψi(0))T=(Ni,Mi)T,Φ(T)(ϕi(0),ψi(0))T=λi(ϕi(0),ψi(0))T. |
In system (2.1), differentiating with t, we have
(du′dt,dv′dt)T=(−1+2uvu2−2uv−u2)(u′v′). |
Then 1 is the eigenvalue of Φ(T), the corresponding eigenvector is (u′T(0),v′T(0))T. We might as well assume λ1=1, (ϕ1(t),ψ1(t))T=(u′T(t),v′T(t))T. Since (uT(t),vT(t)) is stable, then |λ2|<1. Let Φ(t,τ) be the fundamental solution matrix of system (3.2), and Φ(0,τ)=I, namely,
∂Φ(t,τ)∂t=−τDΦ(t,τ)+JT(t)Φ(t,τ). |
For sufficiently small τ, Φ(t,τ) is continuously differentiable with respect to t and τ, and Φ(t,0)=Φ(t). Define mapping L:[0,+∞)×C×C2→C2, where C:=R⊕iR, we have
L(τ,δi,(nimi)):=Φ(T,τ)(nimi)−δi(nimi), |
clearly, L(0,λi,(Ni,Mi)T)=(0,0)T and
Lδi(0,λi,(Ni,Mi)T)=−(Ni,Mi)T,L(ni,mi)T(0,λi,(Ni,Mi)T)=Φ(T)−λiI, |
here, Lδi is the Fréchet derivative of L with respect to δi, and L(ni,mi)T is the Fréchet derivative of L with respect to (ni,mi)T. Setting λi is the single eigenvalue of Φ(T), then we have
Ker(λiI−Φ(T))=span{(Ni,Mi)T},(Ni,Mi)T∉Rank(λiI−Φ(T)). |
where Ker represents the kernel of λiI−Φ(T), and Rank represents the range of λiI−Φ(T), then L(δi,(ni,mi)T)(0,λi,(Ni,Mi)T),i=1,2 is an isomorphic mapping. By the implicit function theorem, there exist τ1>0, τ∈(−τ1,τ1) and continuously differentiable functions δi(τ),ni(τ),mi(τ) such that
Φ(T,τ)(ni(τ),mi(τ))T=δi(τ)(ni(τ),mi(τ))T, | (3.4) |
where δi(τ),i=1,2 are the Floquet multipliers corresponding to (uT(t),vT(t)). Define
(ϕi(t,τ),ψi(t,τ))T:=Φ(t,τ)(ni(τ),mi(τ))T, | (3.5) |
by Φ(0,τ)=I and (3.5), we can obtain
(ϕi(0,τ),ψi(0,τ))T=(ni(τ),mi(τ))T. | (3.6) |
From (3.4) and (3.6), we have
Φ(T,τ)(ϕi(0,τ),ψi(0,τ))T=δi(τ)(ϕi(0,τ),ψi(0,τ))T. |
Specifically, by (3.5), we have
(ϕi(t,0),ψi(t,0))T=Φ(t,0)(ni(0),mi(0))T=Φ(t)(Ni,Mi)T, =Φ(t)(ϕi(0),ψi(0))T=(ϕi(t),ψi(t))T. | (3.7) |
By the definition of (ϕ1(t,τ),ψ1(t,τ))T in (3.5), we can obtain
(∂ϕ1(t,τ)∂t,∂ψ1(t,τ)∂t)T=−τD(ϕ1(t,τ),ψ1(t,τ))T+JT(t)(ϕ1(t,τ),ψ1(t,τ))T. | (3.8) |
Differentiating (3.8) with respect to τ and setting τ=0, we have
(∂ϕ1τ(t,0)∂t,∂ψ1τ(t,0)∂t)T=−D(ϕ1(t),ψ1(t))T+JT(t)(ϕ1τ(t,0),ψ1τ(t,0))T, | (3.9) |
here, ϕ1τ:=∂τϕ1,ψ1τ:=∂τψ1. On the other hand, from (3.4) and (3.5), we can derive
(ϕ1(T,τ),ψ1(T,τ))T=δ1(τ)(ϕ1(0,τ),ψ1(0,τ))T. | (3.10) |
Differentiating (3.10) with τ, we get
(ϕ1τ(T,τ),ψ1τ(T,τ))T=δ′1(τ)(ϕ1(0,τ),ψ1(0,τ))T+δ1(τ)(ϕ1τ(0,τ),ψ1τ(0,τ))T. | (3.11) |
In (3.11), setting τ=0, from (3.6)and δ1(0)=λ1=1, we have
(ϕ1τ(T,0),ψ1τ(T,0))T=δ′1(0)(ϕ1(0),ψ1(0))T+(ϕ1τ(0,0),ψ1τ(0,0))T. | (3.12) |
According to Lemma 2.1, (uT(t,ε),vT(t,ε)) is the periodic solution of system (2.10), that is,
(I+εD)(∂uT(t,ε)∂t,∂uT(t,ε)∂t)T=(a−uT(t,ε)+uT2(t,ε)vT(t,ε)b−uT2(t,ε)vT(t,ε)). | (3.13) |
Differentiating (3.13) with respect to ε and setting ε=0, we have
(d(∂tuT(t,0))dε,d(∂tvT(t,0))dε)T=−D(ϕ1(t),ψ1(t))T+JT(t)(duT(t,0)dε,dvT(t,0)dε)T, | (3.14) |
where ∂tuT(t,0)=ϕ1(t),∂tvT(t,0)=ψ1(t). Since (uT(t,ε),vT(t,ε)) is the periodic solution with period T(ε), thus,
(uT(t,ε),vT(t,ε))T=(uT(t+T(ε),ε),vT(t+T(ε),ε))T. | (3.15) |
In (3.15), differentiating with respect to ε and setting ε=0,t=0, then
(duT(T,0)dε,dvT(T,0)dε)T=−T′(0)(ϕ1(0),ψ1(0))T+(duT(0,0)dε,dvT(0,0)dε)T, | (3.16) |
here, uT(t,0)=uT(t),vT(t,0)=vT(t),T(0)=T. Define
Γ(t):=(ϕ1τ(t,0),ψ1τ(t,0))T−(duT(t,0)dε,dvT(t,0)dε)T. |
From (3.9), (3.12), (3.14) and (3.16), we have
ddtΓ(t)=JT(t)Γ(t), | (3.17) |
Γ(T)−Γ(0)=(δ′1(0)+T′(0))(ϕ1(0),ψ1(0))T. | (3.18) |
Let Γ(t)=Φ(t)(Y1,Y2)T be the general solution of (3.17), where any vector (Y1,Y2)T∈R2. Since (ϕ1(0),ψ1(0))T and (ϕ2(0),ψ2(0))T are linearly independent, then there exit constants γ1 and γ2 so that
(Y1,Y2)T=γ1(ϕ1(0),ψ1(0))T+γ2(ϕ2(0),ψ2(0))T. | (3.19) |
Substituting (3.19) into (3.18), we can obtain δ′1(0)+T′(0)=0. Assume that T′(0)<0, which is equivalent to δ′1(0)>0, if Ω is large enough so that the minimum positive eigenvalue of −Δ is small enough, then there exists at least one eigenvalue βn of −Δ such that δ1(τ)=δ1(βn)>1. Thus, (uT(t),vT(t)) becomes Turing unstable in reaction-diffusion system (1.1) with cross-diffusion.
In this section, we shall select several groups of data for numerical simulations to support theoretical analysis. In system (1.1), Fix parameters a=0.1823,b=0.5, initial values u0=0.6+0.01cosx,v0=1+0.01sinx, then the equilibrium is (0.6823,1.074), μH0=0.6823278, Rec1(μH0)=−0.5<0, Imc1(μH0)=−0.0270945<0. According to Theorem 2.1, system (2.1) produces a spatially homogeneous Hopf bifurcating periodic solution (uT(t),vT(t))T at the equilibrium, which is stable and subcritical. By calculation, we can obtain
L1(μH0)=1.50492,L2(μH0)=−0.72787,L3(μH0)=0.85664,L4(μH0)=−0.27213. |
For different diffusion coefficients, Turing instability of system (1.1) at the periodic solution (uT(t),vT(t))T is different. Hence, we give diffusion coefficients in four cases and carry out corresponding numerical simulations.
(1) If we select d11=1,d22=1,d12=d21=0, at this moment, Turing instability of (uT(t),vT(t))T does not exist in system (1.1) (Figure 1). That is, the same diffusion rates will not cause Turing instability of periodic solution ([19]).
(2) If we select d11=0.05,d22=2,d12=d21=0, then
L1(μH0)d11+L2(μH0)d22−L3(μH0)d12−L4(μH0)d21<0. |
According to Theorem 3.1, system (1.1) is Turing unstable at periodic solution (uT(t),vT(t))T. Through simulation simulation, it can be observed that the stable periodic solution produces Turing bifurcation (Figure 2), which is consistent with the theoretical analysis.
(3) If we choose d11=d22=1,d21=0.2,d12=1.05, from Theorem 3.1, we have
L1(μH0)d11+L2(μH0)d22−L3(μH0)d12−L4(μH0)d21<0, |
then system (1.1) is Turing unstable at periodic solution (uT(t),vT(t))T. Through simulation simulation, it can be verified that the stable periodic solution generates Turing bifurcation (Figure 3). Therefore, cross-diffusion causes the stable periodic solution of system (1.1) to become Turing unstable. self-diffusion induces Turing instability of periodic solution.
(4) If we choose d11=0.5,d12=1.5,d21=0.6,d22=4, then
L1(μH0)d11+L2(μH0)d22−L3(μH0)d12−L4(μH0)d21<0. |
By Theorem 3.1, we can derive that diffusion causes the stable periodic solution of system (1.1) to become Turing unstable (Figure 4). This conforms to the theoretical analysis.
In this paper, a three-molecule autocatalytic Schnakenberg model with cross-diffusion is considered. From both theoretical and numerical perspectives, we investigated how cross-diffusion causes the Turing instability of spatially homogeneous Hopf bifurcating periodic solutions.
The theoretical results indicate that in the Schnakenberg model, when the parameters satisfy certain conditions and Ω is sufficiently large, once the instability of periodic solutions induced by diffusion occurs, new and rich spatiotemporal patterns may emerge. For the reaction-diffusion Schnakenberg model, we can derive the precise conditions of diffusion rates, under which the periodic solutions may experience the instability caused by diffusion.
By numerical simulations, the Turing instability of periodic solution (uT(t),vT(t))T can be observed. Figure 1 shows that without cross-diffusion coefficients, the identical self-diffusion coefficients will not cause the stable periodic solution to produce Turing bifurcation. Figures 2 and 4 illustrate that with appropriate diffusion coefficients, the stable periodic solution of system (1.1) generates Turing instability. Figure 3 indicates that if we select appropriate cross-diffusion coefficients, even for the models with identical self-diffusion rates, cross-diffusion can also cause stable periodic solution to produce Turing bifurcation. Thus, the Turing instability of stable periodic solution (uT(t),vT(t))T is actually induced by cross-diffusion.
The authors declare there is no conflicts of interest.
[1] |
Ang A, Bekaert G, Wei M (2007) Do macro variables, asset markets, or surveys forecast inflation better? J Monetary Econ 54: 1163-1212. Doi: 10.1016/j.jmoneco.2006.04.006 doi: 10.1016/j.jmoneco.2006.04.006
![]() |
[2] | Ankargren S, Unosson M, Yang Y (2018) A mixed-frequency Bayesian vector autoregression with a steady-state prior, Department of Statistics, Uppsala University. |
[3] |
Baker SR, Bloom N, Davis SJ (2016) Measuring economic policy uncertainty. Q J Econ 131: 1593-1636. Doi:10.1093/qje/qjw024 doi: 10.1093/qje/qjw024
![]() |
[4] |
Caggiano G, Castelnuovo E, Groshenny N (2014) Uncertainty shocks and unemployment dynamics in U.S. recessions. J Monetary Econ 67: 78-92. Doi: 10.1016/j.jmoneco.2014.07.006 doi: 10.1016/j.jmoneco.2014.07.006
![]() |
[5] |
Caggiano G, Castelnuovo E, Figueres JM (2017) Economic policy uncertainty and unemployment in the United States: A nonlinear approach. Econ Lett 151: 31-34. Doi: 10.1016/j.econlet.2016.12.002 doi: 10.1016/j.econlet.2016.12.002
![]() |
[6] |
Carlson JA, Parkin M (1975) Inflation expectations. Economica 42: 123-138. Doi: 10.2307/2553588 doi: 10.2307/2553588
![]() |
[7] | Cerisola M, Gelos G (2009) What drives inflation expectations in Brazil?, an empirical analysis. Appl Econ 41: 1215-1227. Doi: 10.1080/00036840601166892 |
[8] |
Chen L, Du Z, Tan Y (2019) Sustainable exchange rates in China: Is there the heterogeneous effect of economic policy uncertainty? Green Financ 1: 346-363.Doi:10.3934/GF.2019.4.346 doi: 10.3934/GF.2019.4.346
![]() |
[9] | Coibion O, Gorodnichenko Y, Kamdar R (2018a) The formation of expectations, inflation, and the Phillips Curve. J Econ Lit 56: 1447-1491. Doi: 10.1257/jel.20171300 |
[10] | Coibion O, Gorodnichenko Y, Kumar S, et al. (2018b) Inflation expectations as a policy tool? NBER Working paper No. w24788. Doi: 10.3386/w24788 |
[11] | Davis SJ (2016) An index of global economic policy uncertainty. NBER Working paper No. w22740. Doi: 10.3386/w22740 |
[12] |
Ferrario A, Guidolin M, Pedio M (2018) Comparing in- and out-of-sample approaches to variance decomposition-based estimates of network connectedness an application to the Italian banking system. Quant Financ Econ 2: 661-701. Doi: 10.3934/QFE.2018.3.661 doi: 10.3934/QFE.2018.3.661
![]() |
[13] |
Fontaine I, Didier L, Razafindravaosolonirina J (2017) Foreign policy uncertainty shocks and US macroeconomic activity: Evidence from China. Econ Lett 155: 121-125. Doi: 10.1016/j.econlet.2017.03.034 doi: 10.1016/j.econlet.2017.03.034
![]() |
[14] |
Fukuda S, Teruyama H, Toda HY (1991) Inflation and price-wage dispersions in Japan. J Jpn Int Econ 5: 160-188. Doi: 10.1016/0889-1583(91)90020-Q doi: 10.1016/0889-1583(91)90020-Q
![]() |
[15] | Gauvin L, McLoughlin C, Reinhardt D (2013) Policy Uncertainty Spillovers to Emerging Markets-Evidence from Capital Flows. SSRN Electron J. Doi:10.2139/ssrn.2273452 |
[16] | Ghosh T, Sahu S, Chattopadhyay S (2017) Households' inflation expectations in India: Role of economic policy uncertainty and global financial uncertainty spill-over. Indira Gandhi Institute of Development Research, Mumbai, India. |
[17] |
Ghysels E (2016) Macroeconomics and the reality of mixed frequency data. J Econometrics 193: 294-314. Doi: 10.1016/j.jeconom.2016.04.008 doi: 10.1016/j.jeconom.2016.04.008
![]() |
[18] |
Globan T, Arčabić V, Sorić P (2016) Inflation in new EU member states: a domestically or externally driven phenomenon? Emerg Mark Financ Trade 52: 154-168. Doi: 10.1080/1540496X.2014.998547 doi: 10.1080/1540496X.2014.998547
![]() |
[19] | Gürkaynak RS, Swanson E, Levin A (2010) Does inflation targeting anchor long-run inflation expectations? evidence from the U.S., UK, and Sweden. J Eur Econ Assoc 8: 1208-1242. Doi: 10.1162/jeea_a_00023 |
[20] |
Hachula M, Nautz D (2018) The dynamic impact of macroeconomic news on long-term inflation expectations. Econ Lett 165: 39-43. Doi: 10.1016/j.econlet.2018.01.015 doi: 10.1016/j.econlet.2018.01.015
![]() |
[21] |
Hammoudeh S, Reboredo JC (2018) Oil price dynamics and market-based inflation expectations. Energy Econ 75: 484-491. Doi: 10.1016/j.eneco.2018.09.011 doi: 10.1016/j.eneco.2018.09.011
![]() |
[22] |
Han L, Qi M, Yin L (2016) Macroeconomic policy uncertainty shocks on the Chinese economy: a GVAR analysis. Appl Econ 48: 4907-4921. Doi:10.1080/00036846.2016.1167828 doi: 10.1080/00036846.2016.1167828
![]() |
[23] | Istrefi K, Piloiu P (2014) Economic policy uncertainty and inflation expectations. Banque de France Working Paper. No. 511. Doi: 10.2139/ssrn.2510829 |
[24] |
Kang WS, de Gracia FP, Ratti RA (2017) Oil price shocks, policy uncertainty and stock returns of oil and gas corporations. J Int Money Financ 70: 344-359. Doi: 10.1016/j.jimonfin.2016.10.003 doi: 10.1016/j.jimonfin.2016.10.003
![]() |
[25] |
Lee YM, Wang KM (2017) How do economic growth asymmetry and inflation expectations affect Fisher hypothesis and Fama's proxy hypothesis? Quant Financ Econ 1: 428-453. Doi: 10.3934/QFE.2017.4.428 doi: 10.3934/QFE.2017.4.428
![]() |
[26] |
Lei CY, Lu Z, Zhang CS (2015) News on inflation and the epidemiology of inflation expectations in China? Econ Syst 39: 644-453. Doi: 10.1016/j.ecosys.2015.04.006 doi: 10.1016/j.ecosys.2015.04.006
![]() |
[27] | Li Z, Zhong J (2019) Impact of economic policy uncertainty shocks on China's financial conditions. Financ Res Lett, [in press]. Doi: 10.1016/j.frl.2019.101303 |
[28] |
Malmendier U, Nagel S (2016) Learning from inflation experiences. Q J Econ 131: 53-87. Doi:10.1093/qje/qjw037 doi: 10.1093/qje/qjv037
![]() |
[29] | Mullineaux DJ (1980) Inflation expectations and money growth in the United States. Am Econ Rev 70: 149-161. URL:https://www.jstor.org/stable/1814744 |
[30] |
Pearce DK (1987) Short-term inflation expectations: Evidence from a monthly survey: note. J Money Credit Bank 19: 388-395. Doi: 10.2307/1992084 doi: 10.2307/1992084
![]() |
[31] |
Pesaran MH (1985) Formation of inflation expectations in British manufacturing industries. Econ J 95: 948-975. Doi: 10.2307/2233258 doi: 10.2307/2233258
![]() |
[32] |
Schorfheide F, Song D (2015) Real-time forecasting with a mixed-frequency VAR. J Bus Econ Stat 33: 366-380. Doi: 10.1080/07350015.2014.954707 doi: 10.1080/07350015.2014.954707
![]() |
[33] |
Serdar O, Ismet G (2019) Re-considering the Fisher equation for South Korea in the application of nonlinear and linear ARDL models. Quant Financ Econ 3: 75-87. Doi: 10.3934/QFE.2019.1.75 doi: 10.3934/QFE.2019.1.75
![]() |
[34] |
Stockhammar P, Österholm P (2015) Effects of US policy uncertainty on Swedish GDP growth. Empir Econ 50: 443-462. Doi:10.1007/s00181-015-0934-y doi: 10.1007/s00181-015-0934-y
![]() |
[35] |
Szyszko M, Płuciennik P (2018) Factors driving consumers' inflation expectations: does the central bank's inflation forecast really matter? Eastern Eur Econ 56: 307-328. Doi: 10.1080/00128775.2018.1467781 doi: 10.1080/00128775.2018.1467781
![]() |
1. | Deepalakshmi J, M. Chandran, 2022, An optimized clustering model for heterogeneous cross-project defect prediction using Quantum Crow search, 978-1-6654-7303-3, 30, 10.1109/ICoSEIT55604.2022.10030011 | |
2. | Dong-Gun Lee, Yeong-Seok Seo, Identification of propagated defects to reduce software testing cost via mutation testing, 2022, 19, 1551-0018, 6124, 10.3934/mbe.2022286 | |
3. | Rashmi Arora, Arvinder Kaur, Heterogeneous Fault Prediction Using Feature Selection and Supervised Learning Algorithms, 2022, 09, 2196-8888, 261, 10.1142/S2196888822500142 | |
4. | Lipika Goel, Neha Nandal, Sonam Gupta, An optimized approach for class imbalance problem in heterogeneous cross project defect prediction, 2022, 11, 2046-1402, 1060, 10.12688/f1000research.123616.1 | |
5. | Ruchika Malhotra, Shweta Meena, Empirical validation of machine learning techniques for heterogeneous cross-project change prediction and within-project change prediction, 2024, 76, 18777503, 102230, 10.1016/j.jocs.2024.102230 | |
6. | Radowanul Haque, Aftab Ali, Sally McClean, Ian Cleland, Joost Noppen, Heterogeneous Cross-Project Defect Prediction Using Encoder Networks and Transfer Learning, 2024, 12, 2169-3536, 409, 10.1109/ACCESS.2023.3343329 |