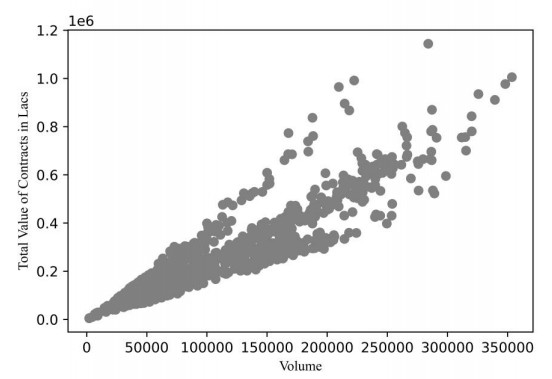
Non-linear forecasting models, including artificial neural networks, are popularly adopted in financial forecasting. These models require high computational infrastructure and resources for practical training and deployment, unlike linear forecasting models. However, this level of infrastructure and resources is not accessible to most market participants. This paper lays down a systematic approach to build a simplistic and effective forecasting model, which empowers investors to make informed decisions using a minimal computational infrastructure. Natural gas futures traded in the Multi-Commodity Exchange of India were identified as an appropriate asset for the study considering the massive, expected movement in energy consumption pattern in India. We have used data analytics and statistical techniques to identify optimal training strategies and features, which resulted in an accurate linear forecasting model. Data analytics also helped to accurately establish the context of the study with the identification of a positive shift in the sentiments of market participants of the asset, which was duly verified using the substantial change in the valuation of the asset. While formulating the forecasting model, several avenues, including identifying weekly and yearly patterns, introducing seasonality and exogenous variables were explored. The paper concludes that such attempts to reduce external dependency and segregate noise data result into better model performance with minimal computational resource and infrastructure.
Citation: Paarth Thadani. 2021: Financial forecasting using stochastic models: reference from multi-commodity exchange of India, Data Science in Finance and Economics, 1(3): 196-214. doi: 10.3934/DSFE.2021011
[1] | Alejandro Rodriguez Dominguez, Om Hari Yadav . A causal interactions indicator between two time series using extreme variations in the first eigenvalue of lagged correlation matrices. Data Science in Finance and Economics, 2024, 4(3): 422-445. doi: 10.3934/DSFE.2024018 |
[2] | Ming Li, Ying Li . Research on crude oil price forecasting based on computational intelligence. Data Science in Finance and Economics, 2023, 3(3): 251-266. doi: 10.3934/DSFE.2023015 |
[3] | Wojciech Kuryłek . Can we profit from BigTechs' time series models in predicting earnings per share? Evidence from Poland. Data Science in Finance and Economics, 2024, 4(2): 218-235. doi: 10.3934/DSFE.2024008 |
[4] | Samuel Asante Gyamerah, Collins Abaitey . Modelling and forecasting the volatility of bitcoin futures: the role of distributional assumption in GARCH models. Data Science in Finance and Economics, 2022, 2(3): 321-334. doi: 10.3934/DSFE.2022016 |
[5] | Morten Risstad, Mathias Holand . On the relevance of realized quarticity for exchange rate volatility forecasts. Data Science in Finance and Economics, 2024, 4(4): 514-530. doi: 10.3934/DSFE.2024021 |
[6] | Yunus Emre Gur . Development and application of machine learning models in US consumer price index forecasting: Analysis of a hybrid approach. Data Science in Finance and Economics, 2024, 4(4): 469-513. doi: 10.3934/DSFE.2024020 |
[7] | Wojciech Kurylek . Are Natural Language Processing methods applicable to EPS forecasting in Poland?. Data Science in Finance and Economics, 2025, 5(1): 35-52. doi: 10.3934/DSFE.2025003 |
[8] | Nitesha Dwarika . The risk-return relationship in South Africa: tail optimization of the GARCH-M approach. Data Science in Finance and Economics, 2022, 2(4): 391-415. doi: 10.3934/DSFE.2022020 |
[9] | Feite Zhou . Cross-validation research based on RBF-SVR model for stock index prediction. Data Science in Finance and Economics, 2021, 1(1): 1-20. doi: 10.3934/DSFE.2021001 |
[10] | Ezekiel NN Nortey, Edmund F. Agyemang, Enoch Sakyi-Yeboah, Obu-Amoah Ampomah, Louis Agyekum . AI meets economics: Can deep learning surpass machine learning and traditional statistical models in inflation time series forecasting?. Data Science in Finance and Economics, 2025, 5(2): 136-155. doi: 10.3934/DSFE.2025007 |
Non-linear forecasting models, including artificial neural networks, are popularly adopted in financial forecasting. These models require high computational infrastructure and resources for practical training and deployment, unlike linear forecasting models. However, this level of infrastructure and resources is not accessible to most market participants. This paper lays down a systematic approach to build a simplistic and effective forecasting model, which empowers investors to make informed decisions using a minimal computational infrastructure. Natural gas futures traded in the Multi-Commodity Exchange of India were identified as an appropriate asset for the study considering the massive, expected movement in energy consumption pattern in India. We have used data analytics and statistical techniques to identify optimal training strategies and features, which resulted in an accurate linear forecasting model. Data analytics also helped to accurately establish the context of the study with the identification of a positive shift in the sentiments of market participants of the asset, which was duly verified using the substantial change in the valuation of the asset. While formulating the forecasting model, several avenues, including identifying weekly and yearly patterns, introducing seasonality and exogenous variables were explored. The paper concludes that such attempts to reduce external dependency and segregate noise data result into better model performance with minimal computational resource and infrastructure.
The economic development of countries largely relies upon the available energy sources, which is evident when considering the case studies of advanced economies. The developing economies in the 21st century do not enjoy such un-restricted supplies. They aim to balance the nation's development and the adverse effects of traditional energy sources such as fossil fuels, whose after-effects are no secret. Considering the rapid urbanization and drastic changes in the economic conditions in India, it is evident that this decade, India will witness significant changes in the energy use pattern considering the substantial increasing demand for energy (Ahmad and Zhang, 2020).
Natural Gas has been in the energy landscape of India for a long time. However, it has never been a prominent source of energy. It possesses various benefits over traditional fossil fuels, which occupy the significant energy mix of the nation as of 2019–2020 (Ministry of Statistics and Programme Implementation, 2021). These benefits include lower SOx, NOx, mercury and particulate emission, and lower emission of greenhouse gases. Natural gas only accounts for 7.6% of energy consumption as of 2019–2020 (Ministry of Statistics and Programme Implementation, 2021). Also, it is not considered a scarce resource, unlike a decade ago. Its primary markets include fertilizer and electricity generation. The above stated factors account for the initiatives undertaken by the government to maximize the usage of the same (Petroleum and Natural Gas Regulatory Board, 2013). Thus, it can be claimed that Natural Gas will hold a prominent position in the energy vertical in the next decade.
Natural Gas being traded in the derivatives commodity exchange, Multi-Commodity Exchange of India (MCX) shall witness some major market movements in the coming decade, thus setting the context of the study. Natural gas futures contracts were launched first in July 2006 in line with New York Mercantile Exchange (NYMEX). However, there have been various new developments in this segment. An exclusive exchange was launched in 2016, the Indian Gas Exchange (IGX), allowing market participants to trade in both spot and forward markets.
With rapid technological developments in the previous decade, in order to make an informed decision, market participants employ various techniques to anticipate the movement of several quantities. Therein, forecasting is imperative to form investment decisions. These techniques have penetrated to the grassroots level, with even novice investors using several methods to keep their investments in check. There are three primary techniques to predict an asset's future in financial markets: Fundamental Analysis, Technical Analysis, and Quantitative Analysis. In this paper, we shall restrict ourselves to the quantitative analysis segment leveraging data analytics and computational intelligence to forecast the asset's state.
Data Analytics and Computational Intelligence are extensively used for deriving financial observations as well as for regulating the industry. Today, data is being generated using billions of financial events and transactions, which can be utilized to create advanced, effective pipelines that operate on these collected datasets to derive insights and detect anomaly events, specifically market manipulation. These resources are available to gigantic institutions. The majority of market participants, especially in India, consist of individual investors which don't have access to such advanced computational resources and infrastructure. Considering the above scenario, this paper focuses on using minimal computation resource, thus formulating effective model with least complexity. This will empower investors even at the grassroots level to take data-supported decisions. There are two broad categories of financial forecasting models: Linear forecasting models and Non-linear forecasting models, which will be discussed sequentially.
Linear forecasting models include Auto-regressive (AR), Moving average (MA), Seasonal auto-regressive integrated moving average with exogenous variable (SARIMAX). These models are being used since decades and they are quite effective for a particular financial asset, which was pretty evident considering the explanation derived using ARIMA-Intervention time-series analysis for the rapid decline in the values of the price index of Shanghai A shares during the world economic debacle in 2008 (Jarret and Kyper, 2011). Linear forecasting models are influenced using various characteristics of the input time-series such as seasonality and cyclicity. Seasonality has a substantial effect on the return generated by the investment, this was validated in the study conducted from 1st April 2005 to 31st March 2015 on closing price of BSE SENSEX, where existence of weekly and monthly seasonality was suspected (Chander and Kumar, 2016). This study helped in realising the effect of seasonality on natural gas futures, operating in the same political condition with similar market participants. Performance of linear forecasting models highly depend upon the incorporated features and effective estimation of parameters such as number of lags. These models tend to perform better when given data with minimal noise. However, linear forecasting models have their capabilities and don't function well once there is non-linearity in the time-series data.
Non-Linear Forecasting Models include machine learning algorithms, artificial neural networks such as Convolutional Neural Network (CNN), Recurrent Neural Network (RNN), Long Short-Term Memory (LSTM). In the Indian context, it has been observed that CNN tend to perform more accurately as compared to its counterparts including Multilayer Perceptron (MLP), RNN and LSTM (Hiransha et al., 2018). Algorithms in this category take into account the historical data of the asset and various external parameters such as sentiments of the market participants using social media platforms such as Twitter (Nayak et al., 2016). Cross-exchange model compatibility has also been reported in the case of New York Stock Exchange (NYSE) and National Stock Exchange (NSE) as they share the same internal dynamics (Hiransha et al., 2018). These models are much more versatile and tend to consider numerous factors that are not being explicitly considered in linear forecasting models. However, these neural networks are black-box models (Bathaee, 2018), i.e., there is no satisfactory explanation of their behaviour, making them risky to rely upon. It is evident that non-linear forecasting models tend to be more complex and require high computational infrastructure for effective training and deployment, unlike linear forecasting models. Thus, making linear forecasting models convenient to large set of market participants.
Open High Low Close (OHLC) and Volume are important indicators of market's sentiments. There are several studies that evaluate the dependency of variables on the asset's movement. This paper relies on the principle "The market discounts everything", which conveys that the market valuation of the asset accounts for all the market events occurring around the world, one need not take into account external parameter for forecasting. However, the trading volume and total value of contracts will be used extensively for data analytics. This exercise was merely conducted to analyse the variations in the underlying data and identify its intrinsic qualities, which would complement the model formulation. This study establishes that simplistic theoretical model could be adopted for data-driven decision-making in the production environment with high accuracy and robustness using minimal computational infrastructure and demonstrates systematic approach for building such model. The highlighting issues encountered while conducting the study include determination of appropriate seasonality, features which have to be incorporated into the model and manipulations for reducing external dependency, and minimizing the computational requirement of the study using simplistic models and dataset.
This section will follow a sequential approach, starting with the dataset preparation to the formulation of prospective models, equipping the investigator with the complete information to replicate and improvise the study.
Datasets are the most critical aspect of data analytics or forecasting techniques, as they predominantly rely on data being fed into the algorithm or pipeline. This study has used web scraping techniques to get the relevant Open High Low Close (OHLC), Total contracts traded (Volume) and Total value in lacs data. The OHLC data was web scrapped from Investing.Com, whereas other parameters were fetched from the official portal of Multi-Commodity Exchange of India, considering the precision of the data available.
The price of a publicly-traded asset denotes its valuation in the viewpoint of the market participants. Natural Gas being a commodity, is highly influenced by political conditions prevalent in the country (Jain and Sen, 2011). India witnessed general elections in 2014, which were the largest-ever elections globally until being surpassed by the 2019 India general elections. The elections led to significant changes in the political landscape of the country. Thus, to eradicate any political effects on the asset price, the period from 01-January-2014 to 30-April-2021 is considered for the study.
Exploratory data analytics is summarising the data and manipulating it using statistical techniques to familiarise oneself and derive meaningful observations. These observations are later incorporated into further analysis, such as identifying training techniques and parameters that can be incorporated in the forecasting model. This sub-section mainly follows a top-down approach for analytics. The parameters used for the analysis include total contracts traded in one trading day (Volume) and the traded contracts' total value.
Figure 1 provides a macroscopic view of the market data. This plot resembles the sector of a circle, which indicates that the average value for the commodity lies between a definite bracket, as the bounding lines have approximately a constant slope. This indicates the presence of a pattern in the data. The equation below displays the average asset's value for one trading day, derived using the slope of the bounding lines.
(1) |
Figure 2 shows the variation of the total number of traded contracts (Volume) with Date. On careful observation, we can say that the lower bound of the number of traded contracts is increasing, signifying the interest of market participants. These observations motivated for the further analysis of data employing statistical methods.
It is well established that there are various categories of trading days present in the data. There are a significant number of trading days that show continuous patterns. However, some lapses indicate the presence of noise in the data. The trading days were divided into three categories according to the number of contracts traded: small, medium and large volume trading days. The ideal parameter for such segmentation should change less frequently, giving the researcher space to use the exact demarcation on a rolling basis. It should also effectively classify the data for further analysis to be conducted. Considering these requirements, maximum volume till date was used for this purpose.
Figure 3 shows the distribution of trading days based on the maximum volume. The plot resembles a bell-shaped curve peaking at the (10%, 20%] Maximum Volume bracket. The most thought-provoking observation here is that more than 77% of the trading days are covered up till the 30% bracket, making them the highest contributor. Also, the (80,100%] of the maximum volume bracket constitutes around 1% of the trading days, which is very minimal. Thus, considering these conditions, classification of trading days is done employing the demarcations presented in Table 1, which confirms maximum coverage in the small volume trading days without raising the upper-bound abnormally and minimal coverage in the large volume trading days.
Category | Percentage of Maximum Volume |
Small Volume | (0, 30] |
Medium Volume | (30, 80] |
Large Volume | (80,100] |
Figures 4–6 provide the macroscopic perspective of data in each bucket. The small volume trading days follow the sector of a circle format as discussed earlier. Even the medium volume trading days display band formation having an upper bound, which can be helpful in the identification of patterns. However, the large volume trading days are random and do not represent any pattern. This category mainly represents the sudden reaction of the market participants in response to any significant event, including the decline in export/imports, increase in taxes on the commodity and even breakthrough in an alternative energy source. That being established, the trading days might occur in a pattern concerning the day of the week, month or year. The further sub-section focuses on understating the occurrences of trading days and identifying patterns in the same.
Figure 7 indicates that small volume trading days comprise the most percentage of trading days until the onset of 2020. After April 2020 specifically, small trading days are scarce, which is thought-provoking. This might be due to the SARS-CoV-2 Pandemic that has influenced the interest of the market participants and investors, which is fueled by potential spike in the consumption of natural gas. Apart from this, one primary reason for such reaction could be the expansion plans laid by the government and other Public Sector Undertakings (PSUs), which aim to attain at least 20% market share of natural gas in the energy mix of India by 2030 as compared to 7.6% in 2019–2020 (Petroleum and Natural Gas Regulatory Board, 2013).
In a broad view, the terminal and initial trading days of the week have a possibility of many contracts being traded. This parameter would be influential in developing the forecasting model and validating its effectiveness on the testing data would be interesting. Figure 8 shows the distribution of all categories of trading days according to the days of the week. The small and medium trading days were approximately equal across official working days i.e., Monday, Tuesday, Wednesday, Thursday and Friday. However, the large trading days are concentrated in the middle of the week i.e., Wednesday and Thursday. It is suspected that the distribution of large trading days across the week follow a bell-shaped curve. There are also three instances where the Multi-Commodity Exchange of India was functional on Saturday and two instances where the financial market was functional on Sunday, which was quite surprising.
Natural Gas Futures (Symbol: NATURALGAS and Exchange: MCX) have displayed growth of 40.68% from 1-January-2020 to 30-April-2021. As of 26-July-2021, the asset is being traded at 304.20, displaying a growth of 96.13% from 1-January-2020. These statistics complement the described analysis and project the asset to be a lucrative investment and a long positional trading opportunity.
This paper aims to develop simplistic, effective linear forecasting models with a progressive approach. This sub-section discusses the techniques employed to develop the potential models and psychology behind various parameters and selections. Forecasting the state of the asset is essential to get an overall perspective about the valuation of the asset on the next trading day and generate monetary benefit. The close price of the asset is the most appropriate parameter for this purpose as it provides a holistic view of the markets when compared effectively using analytical and statistical techniques.
As discussed in the previous sub-section, "Exploratory Data Analytics", small volume trading days constitute a significant chunk of the data, and medium and large volume trading days represent the sudden response of the market participants for a market event during the training period. In this paper, the models are only trained using the main-stream trading days i.e., small volume trading days, with an intent that model will perform better in general scenarios as noise and dependency on external market events are removed. Apart from this, Linear forecasting models, mainly regressive ones, tend to produce more accurate results when given data of similar nature, unlike Artificial Neural Networks, where overfitting will make the accuracy of forthcoming data much worse.
Now that being established, segmenting the data in training and test dataset is critical. The previous discussions convey that with the onset of 2020, there were significant changes in the sentiments of the market participants. According to the established demarcations, the medium and large volume trading days were the newly established mainstream after the onset of 2020. Considering the above conditions, dataset was split in the following manner into Training and Testing period (See Table 2):
Period | Start Date | End Date |
Training Period | 1-Januray-2014 | 31-December-2019 |
Testing Period | 1-January-2020 | 30-April-2021 |
In the above segmentation, the training is done using only mainstream trading data. In contrast, the model is tested on the entire period irrespective of the volume. While forecasting the price for the next trading day it is impossible to accurately predict the number of contracts traded, hence, the category of the coming trading day. Thus, the model it tested on the complete testing period, making it unbiased.
The formulated model will be trained with small volume trading days although it mainly predicts the medium and large volume trading days. This methodology might raise some queries. It should be noted that small volume trading days were mainstream during the training period. However, during the testing period, medium and large volume trading days took their position. This primarily happened due to the positive events that altered the sentiments of the market participants and the formulated model should accommodate such change in sentiments, making the approach appropriate.
Decomposition of the time-series data is essential while formulating forecasting models. It provides insights about the components of the time series, namely: Trend, Seasonality and Residual. These factors become vital, especially when dealing with data from the financial markets, because they correlate with the sentiments of the market participants. Figure 9 displays the time-series plot of close price of the asset for the entire observation period i.e., 1-January-2014 to 30-April-2021.
Multiplicative decomposition technique is most appropriate when the variation in seasonal pattern, or the variation around the trend cycle, is suspected to be proportional to the time series level, which is not visible in the pictorial representations of time-series data. This can also be validated using Figure 10, where the seasonal component is not proportional to the time-series level. Thus, in this study we have used additive decomposition technique, which can be represented as:
(2) |
here, yt is the data, St is the seasonal component, Tt is the trend-cycle component, and Rt is the residual component. Multi-Commodity Exchange of India is operational five days a week i.e., Monday to Friday, making the number of working days 20 per month on average. Thus, the seasonality was observed in multiples of 20 days. The period of 900 days displayed maximum seasonality, which is approximately 45 months. On carefully observing the above plot, it can be concluded that there is a cyclic pattern in the time-series data. A cyclic pattern exists when data exhibit rises and falls that are not of the fixed period, whereas a seasonal pattern is always of the fixed period. The time-series data may show a seasonal pattern of four years. Concrete assertions regarding the four-year seasonality cannot be made, however, the maximum seasonal component of the time series increased steadily as the period was incremented in the fashion described above. It will be interesting to investigate this aspect once appropriate data is available. However, it can be firmly stated that there exists a cyclic pattern. For now, seasonal period in multiples of 20 days, starting from 20 days are incorporated in the model. Figures 11–15 display the additive decomposition of time series with seasonal period of 20 days, 60 days, 120 days, 240 days and 900 days respectively. It can be noticed from the mentioned figures that as the time period is increased, the seasonal component registered a new peak as well as there are substantial variations even within the component.
This sub-section follows an incremental approach to formulate the models. However, before moving forward, it is essential to check the stationarity of the time-series data. A stationary time series is one whose statistical properties such as mean and variance are constant over time. For this purpose, we have used Augmented Dickey-Fuller Test. While conducting the test, Akaike Information Criteria (AIC) minimization lags determination method was used. The test was performed on three different periods, namely: entire observational period, training data and test data.
The results displayed in the Tables 3–5, clearly indicate that the complete data and training data are stationary while the testing data is non-stationary, which was again predictable. As identified in the "Exploratory Data Analytics" section, there is positive traction in the Natural Gas futures after April 2020 due to various reasons discussed earlier which accounts for the non-stationary behaviour. That being established, eradicates the need for differencing for the initial training time-series data.
Metrics | Value |
ADF Test Statistic | -3.1167 |
p-value | 0.02534 |
Metrics | Value |
ADF Test Statistic | -2.9150 |
p-value | 0.04363 |
Metrics | Value |
ADF Test Statistic | -1.5622 |
p-value | 0.5026 |
Keeping the study's objective intact, the study commenced with developing the most basic forecasting model, i.e. auto-regressive (AR), which uses the previous lagged terms to predict the future state of the variable. With an intent to improve the accuracy, the moving average (MA) terms were also introduced, making the model more versatile and complex. MA terms forecast the variable's value using the error encountered in calculating the previous lagged forecasts in a weighted manner. The ARMA models were formulated using maximization of condition sum of squares method and its values were used as starting values for the computation of the likelihood using the Kalman filter.
As discussed earlier, the latter half of the month tend to be more volatile considering the expiry of the contract being comparatively closer. The boolean parameter "Detrimental-Day", which indicated the presence of trading day in the latter half of the month, was introduced into the model as an exogenous variable. The equation below represents the generalized form of the model i.e., ARMAX:
(3) |
in the above equation, and θ are polynomial in lag operator L, and yt is the endogenous variable whereas Xt is the exogenous variable. Further, seasonality was also incorporated with periods starting from 20 days to 900 days, in the multiples of 20. This resulted into models with two new architectures: SARMA and SARMAX. In the models, where exogenous variable was incorporated, the regression coefficients for exogenous variables were part of maximum likelihood estimation. The parameters for the model were identified based on auto-correlation and partial auto-correlation plots (Figures 16 and 17). As clearly evident, the complexity of the models is kept minimal, which can be trained and deployed using a minimal computational infrastructure. A total of 143 models were developed during the study, which were later compared using the relevant metrics to identify the optimal model.
The formulated models were compared based on Mean Absolute Error (MAE), Mean Squared Error (MSE), Mean Absolute Percentage Error (MAPE), Akaike Information Criteria (AIC), Bayesian Information Criteria (BIC), Hannan-Quinn Information Criteria (HQIC). As mentioned, the testing of the models was done in an unbiased manner where the coefficients were updated after completion of each mainstream training day, these led to change in AIC, BIC, HQIC. During the testing period, the mainstream trading days include the medium volume training days, unlike the training period where the small volume trading days were mainstream. Hence, with a pursuit to make an informed decision mean, median, initial and final values of the parameters were considered in the analysis. The stationarity of the time-series after addition of each mainstream day was verified considering the nature of the models used. Table 6 displays the relevant metrics of important models which will come handy in the further discussion.
Order | Mean Square Error | Mean Absolute Error | Mean Absolute Percentage Error | AIC (Mean) | BIC (Mean) | HQIC (Mean) |
AR (1) | 44.4035 | 4.8275 | 0.02892 | 10,111.72 | 10,127.86 | 10,117.72 |
AR (2) MA (1) MA (2) MA (3) MA (4) MA (5) MA (11) MA (15) MA (16) ARMA (1, 1) ARMA (1, 2) ARMA (1, 5) ARMA (1, 10) ARMA (1, 12) ARMA (1, 15) ARMA (2, 1) ARMA (2, 2) SARMA (1, 1) × (1, 1)20 SARMA (1, 1) × (1, 1)40 SARMA (1, 1) × (1, 1)60 SARMAX (1, 1) × (1, 1)20 |
44.4084 555.1785 251.5511 174.7944 116.8921 94.0904 59.7297 54.6836 54.4054 44.4065 44.4775 44.3819 44.6482 44.4567 44.53 44.6109 44.5359 44.8296 44.5288 44.7506 44.7806 |
4.8364 20.0723 13.2839 10.7331 8.8762 7.5895 5.9598 5.6389 5.6222 4.8358 4.8427 4.8218 4.8166 4.8089 4.8027 4.8474 4.8409 4.8518 4.8317 4.8507 4.8252 |
0.02896 0.1349 0.0882 0.069 0.0567 0.0482 0.03669 0.0345 0.0343 0.02895 0.029 0.0288 0.0288 0.0288 0.02872 0.029 0.0289 0.02897 0.0289 0.02899 0.02887 |
10,111.3 14,513.75 13,233.39 12,436.16 11,878.78 11,487 10,654.73 10,454.69 10,432.01 10,111.37 10,112.98 10,116.78 10,121.6 10,124.03 10,126 10,112.6 10,114.5 10,119 10,121.94 10,121.49 10,128.33 |
10,132.82 14,529.88 13,254.91 12,463.06 11,911.05 11,524.65 10,724.66 10,546.13 10,528.82 10,132.89 10,139.88 10,159.81 10,191.52 10,204.71 10,222.81 10,139.5 10,146.8 10,145.9 10,148.83 10,148.39 10,171.36 |
10,119.29 14,519.74 13,241.38 12,446.15 11,890.76 11,500.98 10,680.7 10,488.64 10,467.95 10,119.36 10,122.97 10,132.75 10,147.56 10,153.99 10,161.94 10,122.6 10,126.5 10,128.99 10,131.92 10,131.48 10,144.31 |
While identifying the optimal model, the main objective is to maximize the accuracy without using a very complex model i.e., minimizing AIC, BIC and HQIC. The metrics reveal on the first glance that Mean Absolute Percentage Error (MAPE) cannot be used independently to evaluate the performance of the forecasting model. There are minor variations in its value even with large deviation in the mean absolute error, which signifies the deviation of the forecasted value from the actual valuation of the asset. Taking this into consideration, a combination of Mean Square Error (MSE), Mean Absolute Error (MAE) and MAPE will be used to evaluate further.
The results of auto-regressive models indicate that the accuracy of the model peak at order 1 and decrease thereafter. However, there were two abnormal spikes in the accuracy: AR (10) and AR (15). AR (15) displayed maximum accuracy with mean absolute error of 4.7965 followed by 4.8201. This basically provides a validation of estimating the model parameters using the partial auto-correlation plot. The partial auto-correlation plot shows substantial value only at order 0 and 1, thereafter it abruptly falls to 0. This validation also stands true for the moving average models, whose accuracy increases as we increase the order of the model, i.e. incorporate more and more moving average terms, which is displayed in the exponentially decreasing auto-correlation plot. The initial moving average models up to order 20, are not effective when compared with its counter parts.
The ARMA, ARMAX, SARMA and SARMA model, do not depict a straight forward relation with their order, they in fact show anomaly spikes in the accuracy, which can be realised using the ARMA (1, 5) model. However, this an appropriate moment to mention that introduction of the exogenous variable is not necessarily positive in every case, as in this one. The introduction of the exogenous variable "Detrimental-Day", was not effective and damaged the accuracy of the model in mostly every case. This introduction is also against the assumption the study was conducted i.e., "The market discounts everything", which is validated considering this case. The seasonal introduction of 60 days proved to be more effective than the 20 days. The complexity of model is also not substantially higher than the latter. However, it is not as effective as the introduction of the 40 days seasonality. This clearly signify that monotonous trend in the seasonal model do not exists, techniques like grid search are effective for identification of the optimal model. This lays the future scope of the study, introduction of four-year seasonal order will be an interesting introduction to evaluate once the appropriate data is available. Currently, the aspect appears perplexing.
Comparing the metrics, ARMA (1, 15) was identified as the optimal model. Table 1 in the Appendix, list the actual and forecasted values for the testing period and Figure 18 illustrates the variation in a pictorial manner. In descriptive terms, the model conveys that closing price of the previous trading day and forecast errors of the previous three weeks are enough to predict the state of the asset. The introduction of seasonality and exogenous variable is not required. The simplistic model, ARMA (1, 15), will be sufficient for the investor to make informed decisions. This is pretty evident when considered the anomaly spike in the asset's closing price on 28-Septmber-2020, where it displayed substantial growth of 24.24% in one single trading day. Once this data was incorporated into the training dataset, the performance of the model was commendable as illustrated using the supported forecasted values.
In this paper, we have explored an alternative approach to develop effective forecasting model which can be trained and deployed using minimal computational infrastructure and resource. The study focuses on removing malicious and noise data from the training dataset, and training the model only using the mainstream data, thus eradicating the malicious data to large extent. Several financial assets are highly influenced by the government norms and regulations, which tend to reflect in the asset's market valuation. Thus, such attempts to minimise external factors can turn out to be an effective approach as demonstrated. Exploratory data analytics helps the investor to get acquainted with the data and derive its intrinsic qualities. The approach followed in this paper highly relies upon such intrinsic qualities which tend to ease the computational requirement. Linear forecasting models perform well when trained using appropriate strategy and incorporation of correct features. There are numerous possible seasonal patterns projected by the data, finding the right period is tough nut to crack. However, techniques such as grid search are useful in such scenarios. Natural Gas Future (Symbol: NATURALGAS, Exchange: MCX) has displayed a positive shift in the sentiments of market participants, which has been reflected in the asset's valuation with 96.13% growth from 1-January-2020 to 26-July-2021. The suspected reasons for such sudden positive traction include the SARS-CoV-2 pandemic and expansion plans laid by the government to mutate the energy consumption pattern in India. This study clearly validates the notion that commodities are highly influenced by the political conditions prevalent in the geographical arena. The further scope of the study includes exploration of the suspected four-year seasonal pattern, which can add tremendous value to the model considering the seasonal component in the decomposed time-series using the nearest period (900 Days).
We thank Dr. Omkar D. Palsule–Desai for his guidance, encouragement and useful critiques of this research work. We also thank the editor and two anonymous reviewers for their time and effort to provide valuable feedback on the previous versions of the manuscript.
The author declares no conflicts of interest in this paper.
[1] | Ahmad T, Zhang D (2020) A critical review of comparative global historical energy consumption and future demand: The story told so far. Energy Rep 6: 1973–1991. |
[2] | Bathaee Y (2018) The Artificial Intelligence Black Box and The Failure of Intent and Causation. Harv J L Tech 31: 889–938. |
[3] | Chander R, Kumar V (2016) An Analytical Study of Seasonality Effect in BSE SENSEX. Adv Econ Bus Manage 3: 691–694. |
[4] | Hiransha M, Gopalakrishnan EA, Menon VK, et al. (2018) NSE Stock Market Prediction Using Deep-Learning Models. Proc Comput Sci 132: 1351–1362. |
[5] | Jarret JE, Kyper E (2011) ARIMA Modeling with Intervention to Forecast and Analyze Chinese Stock Prices. Int J Eng Bus Manag 3: 53–58. |
[6] | Jain A, Sen A (2011) Natural Gas in India: An Analysis of Policy, The Oxford Institute for Energy Studies. |
[7] | Ministry of Statistics and Programme Implementation (2021) Energy Statistics India 2021. Available from: http://mospi.nic.in/publication/energy-statistics-india-2021. |
[8] | Nayak A, Pai MMM, Pai RM (2016) Prediction Models for Indian Stock Market. Proc Comput Sci 89: 441–449. |
[9] | Petroleum and Natural Gas Regulatory Board (2013) Vision 2030. Available from: https://www.pngrb.gov.in/pdf/vision/vision-NGPV-2030-06092013.pdf. |
![]() |
![]() |
![]() |
![]() |
Category | Percentage of Maximum Volume |
Small Volume | (0, 30] |
Medium Volume | (30, 80] |
Large Volume | (80,100] |
Period | Start Date | End Date |
Training Period | 1-Januray-2014 | 31-December-2019 |
Testing Period | 1-January-2020 | 30-April-2021 |
Metrics | Value |
ADF Test Statistic | -3.1167 |
p-value | 0.02534 |
Metrics | Value |
ADF Test Statistic | -2.9150 |
p-value | 0.04363 |
Metrics | Value |
ADF Test Statistic | -1.5622 |
p-value | 0.5026 |
Order | Mean Square Error | Mean Absolute Error | Mean Absolute Percentage Error | AIC (Mean) | BIC (Mean) | HQIC (Mean) |
AR (1) | 44.4035 | 4.8275 | 0.02892 | 10,111.72 | 10,127.86 | 10,117.72 |
AR (2) MA (1) MA (2) MA (3) MA (4) MA (5) MA (11) MA (15) MA (16) ARMA (1, 1) ARMA (1, 2) ARMA (1, 5) ARMA (1, 10) ARMA (1, 12) ARMA (1, 15) ARMA (2, 1) ARMA (2, 2) SARMA (1, 1) × (1, 1)20 SARMA (1, 1) × (1, 1)40 SARMA (1, 1) × (1, 1)60 SARMAX (1, 1) × (1, 1)20 |
44.4084 555.1785 251.5511 174.7944 116.8921 94.0904 59.7297 54.6836 54.4054 44.4065 44.4775 44.3819 44.6482 44.4567 44.53 44.6109 44.5359 44.8296 44.5288 44.7506 44.7806 |
4.8364 20.0723 13.2839 10.7331 8.8762 7.5895 5.9598 5.6389 5.6222 4.8358 4.8427 4.8218 4.8166 4.8089 4.8027 4.8474 4.8409 4.8518 4.8317 4.8507 4.8252 |
0.02896 0.1349 0.0882 0.069 0.0567 0.0482 0.03669 0.0345 0.0343 0.02895 0.029 0.0288 0.0288 0.0288 0.02872 0.029 0.0289 0.02897 0.0289 0.02899 0.02887 |
10,111.3 14,513.75 13,233.39 12,436.16 11,878.78 11,487 10,654.73 10,454.69 10,432.01 10,111.37 10,112.98 10,116.78 10,121.6 10,124.03 10,126 10,112.6 10,114.5 10,119 10,121.94 10,121.49 10,128.33 |
10,132.82 14,529.88 13,254.91 12,463.06 11,911.05 11,524.65 10,724.66 10,546.13 10,528.82 10,132.89 10,139.88 10,159.81 10,191.52 10,204.71 10,222.81 10,139.5 10,146.8 10,145.9 10,148.83 10,148.39 10,171.36 |
10,119.29 14,519.74 13,241.38 12,446.15 11,890.76 11,500.98 10,680.7 10,488.64 10,467.95 10,119.36 10,122.97 10,132.75 10,147.56 10,153.99 10,161.94 10,122.6 10,126.5 10,128.99 10,131.92 10,131.48 10,144.31 |
Category | Percentage of Maximum Volume |
Small Volume | (0, 30] |
Medium Volume | (30, 80] |
Large Volume | (80,100] |
Period | Start Date | End Date |
Training Period | 1-Januray-2014 | 31-December-2019 |
Testing Period | 1-January-2020 | 30-April-2021 |
Metrics | Value |
ADF Test Statistic | -3.1167 |
p-value | 0.02534 |
Metrics | Value |
ADF Test Statistic | -2.9150 |
p-value | 0.04363 |
Metrics | Value |
ADF Test Statistic | -1.5622 |
p-value | 0.5026 |
Order | Mean Square Error | Mean Absolute Error | Mean Absolute Percentage Error | AIC (Mean) | BIC (Mean) | HQIC (Mean) |
AR (1) | 44.4035 | 4.8275 | 0.02892 | 10,111.72 | 10,127.86 | 10,117.72 |
AR (2) MA (1) MA (2) MA (3) MA (4) MA (5) MA (11) MA (15) MA (16) ARMA (1, 1) ARMA (1, 2) ARMA (1, 5) ARMA (1, 10) ARMA (1, 12) ARMA (1, 15) ARMA (2, 1) ARMA (2, 2) SARMA (1, 1) × (1, 1)20 SARMA (1, 1) × (1, 1)40 SARMA (1, 1) × (1, 1)60 SARMAX (1, 1) × (1, 1)20 |
44.4084 555.1785 251.5511 174.7944 116.8921 94.0904 59.7297 54.6836 54.4054 44.4065 44.4775 44.3819 44.6482 44.4567 44.53 44.6109 44.5359 44.8296 44.5288 44.7506 44.7806 |
4.8364 20.0723 13.2839 10.7331 8.8762 7.5895 5.9598 5.6389 5.6222 4.8358 4.8427 4.8218 4.8166 4.8089 4.8027 4.8474 4.8409 4.8518 4.8317 4.8507 4.8252 |
0.02896 0.1349 0.0882 0.069 0.0567 0.0482 0.03669 0.0345 0.0343 0.02895 0.029 0.0288 0.0288 0.0288 0.02872 0.029 0.0289 0.02897 0.0289 0.02899 0.02887 |
10,111.3 14,513.75 13,233.39 12,436.16 11,878.78 11,487 10,654.73 10,454.69 10,432.01 10,111.37 10,112.98 10,116.78 10,121.6 10,124.03 10,126 10,112.6 10,114.5 10,119 10,121.94 10,121.49 10,128.33 |
10,132.82 14,529.88 13,254.91 12,463.06 11,911.05 11,524.65 10,724.66 10,546.13 10,528.82 10,132.89 10,139.88 10,159.81 10,191.52 10,204.71 10,222.81 10,139.5 10,146.8 10,145.9 10,148.83 10,148.39 10,171.36 |
10,119.29 14,519.74 13,241.38 12,446.15 11,890.76 11,500.98 10,680.7 10,488.64 10,467.95 10,119.36 10,122.97 10,132.75 10,147.56 10,153.99 10,161.94 10,122.6 10,126.5 10,128.99 10,131.92 10,131.48 10,144.31 |