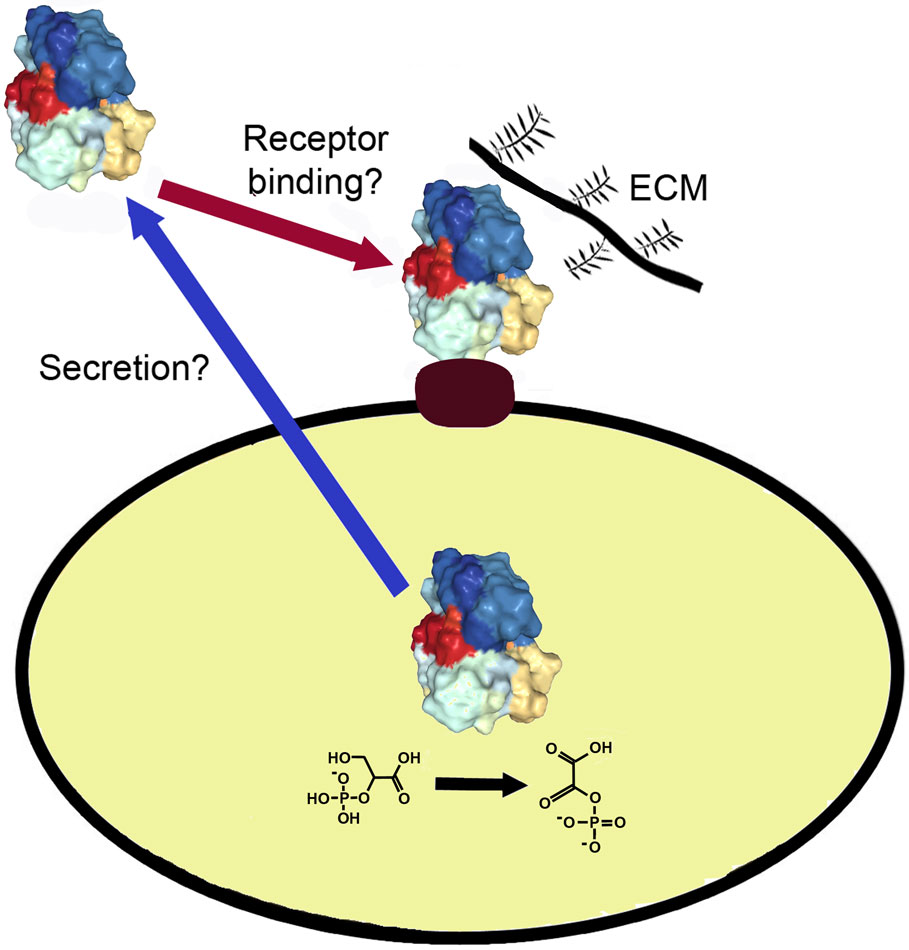
Citation: Constance J. Jeffery. Intracellular/surface moonlighting proteins that aid in the attachment of gut microbiota to the host[J]. AIMS Microbiology, 2019, 5(1): 77-86. doi: 10.3934/microbiol.2019.1.77
[1] | Constance Jeffery . Intracellular proteins moonlighting as bacterial adhesion factors. AIMS Microbiology, 2018, 4(2): 362-376. doi: 10.3934/microbiol.2018.2.362 |
[2] | Souliphone Sivixay, Gaowa Bai, Takeshi Tsuruta, Naoki Nishino . Cecum microbiota in rats fed soy, milk, meat, fish, and egg proteins with prebiotic oligosaccharides. AIMS Microbiology, 2021, 7(1): 1-12. doi: 10.3934/microbiol.2021001 |
[3] | Tatyana V. Polyudova, Daria V. Eroshenko, Vladimir P. Korobov . Plasma, serum, albumin, and divalent metal ions inhibit the adhesion and the biofilm formation of Cutibacterium (Propionibacterium) acnes. AIMS Microbiology, 2018, 4(1): 165-172. doi: 10.3934/microbiol.2018.1.165 |
[4] | Paola Brun . The profiles of dysbiotic microbial communities. AIMS Microbiology, 2019, 5(1): 87-101. doi: 10.3934/microbiol.2019.1.87 |
[5] | Mushtaq Hussain, Nusrat Jabeen, Anusha Amanullah, Ayesha Ashraf Baig, Basma Aziz, Sanya Shabbir, Fozia Raza, Nasir Uddin . Molecular docking between human TMPRSS2 and SARS-CoV-2 spike protein: conformation and intermolecular interactions. AIMS Microbiology, 2020, 6(3): 350-360. doi: 10.3934/microbiol.2020021 |
[6] | Alexandra Soares, Ana Azevedo, Luciana C. Gomes, Filipe J. Mergulhão . Recombinant protein expression in biofilms. AIMS Microbiology, 2019, 5(3): 232-250. doi: 10.3934/microbiol.2019.3.232 |
[7] | Laurent Coquet, Antoine Obry, Nabil Borghol, Julie Hardouin, Laurence Mora, Ali Othmane, Thierry Jouenne . Impact of chlorhexidine digluconate and temperature on curli production in Escherichia coli—consequence on its adhesion ability. AIMS Microbiology, 2017, 3(4): 915-937. doi: 10.3934/microbiol.2017.4.915 |
[8] | Yue Tang, Shaun Cawthraw, Mary C. Bagnall, Adriana J. Gielbert, Martin J. Woodward, Liljana Petrovska . Identification of temperature regulated factors of Campylobacter jejuni and their potential roles in virulence. AIMS Microbiology, 2017, 3(4): 885-898. doi: 10.3934/microbiol.2017.4.885 |
[9] | Esther Mezhibovsky, Yue Wu, Fiona G. Bawagan, Kevin M. Tveter, Samantha Szeto, Diana Roopchand . Impact of grape polyphenols on Akkermansia muciniphila and the gut barrier. AIMS Microbiology, 2022, 8(4): 544-565. doi: 10.3934/microbiol.2022035 |
[10] | Luciana C. Gomes, Filipe J. Mergulhão . Effect of heterologous protein expression on Escherichia coli biofilm formation and biocide susceptibility. AIMS Microbiology, 2016, 2(4): 434-446. doi: 10.3934/microbiol.2016.4.434 |
Interaction of the gut microbiota with the host requires proteins that are on the cell surface to form and maintain interactions with host cells and tissues. These interactions are important for infection by pathogens and for the symbiotic relationships between the normal gut microbiota and the host. In recent years, many of these surface proteins have been found to be identical to cytoplasmic enzymes and chaperones. They belong to a larger group of proteins with multiple functions called moonlighting proteins. Moonlighting proteins are multifunctional proteins in which a single polypeptide chain performs two or more physiologically relevant biochemical or biophysical functions [1]. Hundreds have been identified and are described further in the online MoonProt Database (moonlightingproteins.org) [2]. Over 100 are cytoplasmic proteins that have a second function on the cell surface, often as adhesins that bind to host cells and tissues [3],[4] (Figure 1). This mini-review focuses on intracellular proteins that moonlight on the cell surface of bacterial and eukaryotic gut microbiota.
Dozens of intracellular proteins have been found on the surface of gut microbiota where they interact with host cell surface receptors, ECM, plasminogen or other proteins. These proteins are found to be moonlighting in many types of gut microbiota - pathogens and commensal bacteria, including both Gram negative and Gram positive species, yeast, and an amoeba.
One of the most commonly found intracellular/surface moonlighting proteins in pathogenic bacteria is Hsp60, a chaperone involved in protein folding. In the gut microbiome, it is also used on the cell surface as an adhesin in several species that cause diarrhea and other, sometimes serious or even lethal, complications. [5]–[8] (Table 1). The Gram positive spore forming Clostridium difficile (CDI or C-dif), causes tens of thousands of deaths in the US each year. Helicobacter pylori is a Gram negative bacterium that can be a normal part of the stomach biome but can also lead to chronic gastritis, ulcers and stomach cancer. The Gram negative Salmonella enterica serotype Typhimurium is an intracellular pathogen that causes food poisoning, salmonellosis, which is often spread by eating contaminated meat, eggs, or milk. Listeria monocytogenes is transmitted in contaminated unpasteurized dairy products and the reason pregnant women are recommended not to eat soft cheeses, such as brie, because it can cause meningitis in newborns. In addition to Hsp60 [9], Listeria uses another intracellular protein, alcohol acetaldehyde dehydrogenase [10], as an adhesin and Ami autolysin, a cell surface enzyme that cleaves cell wall glycopeptides, in a second role as an adhesin to mammalian cells [11].
Cytoplasmic Function | Cell Surface Function | Reference | |
Bifidobacteria | |||
Bile salt hydrolase | hydrolase | binds plasminogen | 20 |
DnaK | chaperone | binds plasminogen | 20 |
Enolase | hydratase | binds plasminogen | 19,20 |
Glutamine synthetase | synthetase | binds plasminogen | 20 |
Phosphoglycerate mutase | mutase | binds plasminogen | 20 |
Lactobacillus johnsonii | |||
Ef-Tu | elongation factor | Binds human cells and mucins | 17 |
Hsp60 | chaperone | binds mucins and epithelial cells | 16 |
Lactobacillus crispatus | |||
Enolase | hydratase | binds plasminogen and laminin | 13 |
Glucose 6-phosphate isomerase | isomerase | binds laminin, collagen | 15 |
Glutamine synthetase | synthetase | binds plasminogen, fibronectin, laminin, collagen | 15 |
Lactobacillus plantarum | |||
Enolase | hydratase | Binds fibronectin | 12 |
GAPDH | dehydrogenase | binds mucin and Caco2 cells | 18 |
Lactobacillus acidophilus | |||
GAPDH | dehydrogenase | binds mucin | 14 |
Clostridium difficile | |||
Hsp60 | chaperone | adhesin | 5 |
Helicobacter pylori | |||
Hsp60 | chaperone | adhesin | 6,8 |
Listeria monocytogenes | |||
Alcohol acetaldehyde | dehydrogenase | adhesin | 10 |
Hsp60 chaperone | chaperone | adhesin | 9 |
Ami autolysin | autolysin | adhesin | 11 |
Salmonella enterica serotype Typhimurium | |||
Hsp60 | chaperonin | adhesin | 7 |
Candida albicans | |||
Alcohol dehydrogenase (ADH1) | dehydrogenase | binds plasminogen | 21 |
Enolase | hydratase | binds plasminogen | 23 |
Fructose bisphosphate aldolase | aldolase | binds plasminogen | 21 |
GAPDH | dehydrogenase | binds plasminogen, fibronectin, laminin | 21,22 |
Peroxisomal catalase (CTA1) | catalase | binds plasminogen | 21 |
Phosphoglycerate kinase | kinase | binds plasminogen | 21 |
Phosphoglyceromutase | mutase | binds plasminogen | 21 |
Transcription elongation factor | elongation factor | binds plasminogen | 21 |
Thiol-specific antioxidant protein | antioxidant | binds plasminogen | 21 |
glycerol 3-phosphate dehydrogenase | dehydrogenase | binds plasminogen | 24 |
high-affinity glucose transporter 1 | sugar transporter | complement inhibitor | 25 |
Enteamoeba histolytica | |||
alcohol dehydrogenase (EhADH2) | dehydrogenase | Binds fibronectin, laminin, collagen | 26 |
Homo sapiens (human) | |||
Hsp90α | chaperone | binds to bacterial pathogens | 27 |
Eukaryotic gut microbiota also use intracellular proteins as cell surface adhesins. The yeast Candida albicans is a common part of the gut microbiome and an opportunistic pathogen that can cause candidiasis in immunocompromised individuals. It can also be found on biofilms on implanted medical devices. GAPDH is an enzyme in glycolysis and has many moonlighting functions in many species (Table 1). In C. albicans, it was found to bind plasminogen as well as fibronectin and laminin [21],[22]. Candida also uses several other proteins from glycolysis and gluconeogenesis to bind to plasminogen, enolase [23], fructose 1,6-bisphosphate aldolase [21], phosphoglycerate kinase [21], and phosphoglyceromutase [21]. Glycerol 3-phosphate dehydrogenase, which functions in glycerol accumulation, is also an adhesion [24]. Three proteins involved in protection from alcohol, hydrogen peroxide and antioxidants also bind to plasminogen: alcohol dehydrogenase (ADH1) [21] which protects cells from ethanol, peroxisomal catalase (CTA1) [21], which protects cells from the toxic effects of hydrogen peroxide, and a thiol-specific antioxidant protein [21]. The transcription elongation factor TEF1, which promotes the GTP-dependent binding of aminoacyl-tRNA to the A-site of the ribosome during protein biosynthesis, is also a cell surface plasminogen binding protein [21].
Another protein in Candida albicans has a second function that is involved in modulation of the host's immune system. The high-affinity glucose transporter 1 is a sugar transporter that is also an inhibitor of the host's complement system [25]. It binds to the complement regulators FH and C4BP and protects the yeast from actions of the host's complement cascade.
Entamoeba histolytica is a parasitic amoeba that infects the large bowel. It is estimated to infect about 50 million people worldwide, usually asymptomatically, but it can sometimes enter the epithelial cell layer and result in a lethal infection. It kills more than 50,000 people each year. An intracellular enzyme, alcohol dehydrogenase (EhADH2), which has both alcohol dehydrogenase and acetaldehyde dehydrogenase activity, can be found on the cell surface where it binds proteins of the host's extracellular matrix (ECM), including fibronectin, laminin, and type II collagen [26].
In some cases of gut microbiota interactions with humans, it is the human cell that displays the moonlighting protein. Hsp90 on mammalian cell surfaces is involved in sensing bacterial proteins and lipopolysaccharide (LPS) and can aid in initiating an immune response. The cell surface protein JlpA from Campylobacter jejuni, a common cause of food poisoning, interacts directly and specifically with cell surface-exposed Hsp90 on human epithelial cells [27]. Binding to Hsp90 results in the activation of proinflammatory immune responses through signaling pathways involving NF-κB and p38 MAP kinase. It's not clear how the signal crosses the cell membrane because Hsp90 does not contain a transmembrane domain, but there must be at least one additional cell surface protein interacting with Hsp90 that can transduce the signal into the cell.
The moonlighting proteins described above and many other intracellular proteins have also been observed on the surface of these and other species through larger scale proteomics studies of cell surface proteins [31]. Proteomics studies of E. coli identified elongation factor Tu, D-tagatose 1,6-bisphosphate aldolase 2, and isocitrate lyase on the cell surface [32]. Proteins found on the surface of Enterococcus faecalis included elongation factors G and Tu, tyrosine—tRNA ligase, alanine—tRNA ligase, chaperone protein DnaK, phosphoglycerate mutase, pyruvate kinase, fructose 1,6-bisphosphate aldolase, enolase, GAPDH, formate acetyltransferase, and adenylate kinase [33]. Some of these proteins and many others were found on the surface of Listeria monocytogenes, including GroEL, DnaK , GAPDH, enolase, translation elongation factors tsf and G, pyruvate kinase, cysteine synthase, phosphoglycerate kinase, glutamate dehydrogenase, transketolase, branched chain amino acid aminotransferase, glucose 6-phosphate isomerase, and triosphosphate isomerase [34]. Ten proteins often found to moonlight were found on the surface of Bifidobacterium animalis ssp. Lactis KLDS 2.0603, including GroEL, GroES, EF-ts, GAPDH, transaldolase, DnaK, enolase, phosphoglucosamine mutase, bile salt hydrolase, and ribosomal protein L13, and many of the same proteins were found on the surface of Lactobacillus acidophilus NCFM [41]. Kinoshita and coworkers showed that phosphate buffer can wash over a dozen intracellular proteins from the surface of intestinal lactic acid bacteria, including GroEL, enolase, EF-Tu, phosphoglyceromutase, triosephosphate isomerase, DnaK, phosphofructokinase, and phosphoglycerate kinase, and at least some of these proteins bind to porcine intestinal mucosa [38].
The results of several proteomics studies show that changes in dietary components can cause changes in the expression or secretion of intracellular/surface moonlighting proteins and thereby might have an effect on the strength of interaction of some probiotics with host cells. Celebioglu and coworkers showed that several intracellular proteins are found on the surface of Lactobacillus acidophilus and that growth in the presence of different carbon sources, plant polyphenols, or ‘prebiotics’ (molecules like raffinose that humans cannot digest but intestinal bacteria can) affected the level of expression of several of these proteins on the cell surface, including EF-Tu and pyruvate kinase [39],[42],[43]. In addition, Montoro and coworkers showed that the levels of surface expression of phosphoglucomutase and several other proteins normally found in the cytoplasm varied in strains of the probiotic Lactobacillus pentosus that varied in their strength of adhesion to porcine mucin [44].
Overall, a wide variety of intracellular proteins have been found on the surface of gut microbiota. From the results of the proteomics studies, it was not determined if the proteins perform the same function on the cell surface as in the cell or if they perform a different function there, so additional experiments would be needed before determining if they are true moonlighting proteins. In some cases, observing a protein on the cell surface could be due to challenges in the experimental method, for example, proteins that are interacting firmly with a cytoplasmic domain of a transmembrane protein complex, might be misidentified as being on the cell surface. Further experiments will be needed to confirm if the intracellular proteins identified in the surface proteomics studies also function as adhesins.
The observation of many intracellular proteins with a second function on the cell surface raises several questions.
First, the mechanisms by which these intracellular proteins are secreted while many other highly abundant intracellular proteins remain in the cell is not known. They do not contain signal sequences for secretion through the canonical Sec secretion pathway or other known motifs required for noncanonical secretion pathways. An analysis of one hundred intracellular/surface moonlighting proteins found that the intracellular/surface moonlighting proteins have physicochemical features that are similar to other cytosolic proteins [35].
It is also not known how most of the intracellular/surface moonlighting proteins bind to the cell surface. The secretion pathways and receptors or other mechanisms for binding to the cell surface might be versions of the known pathways and receptors or might involve novel processes. Because many of the intracellular/surface proteins described above are widely conserved in evolution and play important roles in human cells, their catalytic mechanisms might not be good targets in the development of novel therapeutics to treat infections. Instead, elucidating how these proteins are secreted and bound to the bacterial cell surface might lead to the identification of processes and proteins that could serve as targets for therapeutics.
Many of the intracellular proteins found to be adhesins on pathogens are homologues of proteins found to be adhesins on the surface of probiotic species, for example Hsp60. This might help explain why some probiotics appear to be able to compete with or crowd out pathogenic species. This use of homologous proteins by pathogens and probiotics also means that it would be important to find treatments that affect only those protein homologues in the pathogens and not in the probiotic species, or perhaps it would be possible to find a way to use these proteins to help probiotic species to displace pathogens and improve the balance of bacterial species in the gut.
It is also interesting that both pathogens and probiotics have cell surface proteins that bind to plasminogen. Plasminogen can be converted to plasmin, an active protease that can be used to degrade host extracellular matrix and basement membrane and thereby enable pathogens to invade nearby tissues [28]–[30], but it's not clear why probiotic species bind plasminogen.
Intracellular/surface moonlighting proteins are used by species described above that can cause serious intestinal infections. For example, Clostridium difficile can cause life-threatening intestinal inflammation and diarrhea and leads to tens of thousands of deaths in the US each year. An imbalance between pathogenic and probiotic species is also associated with inflammatory bowel disease (ulcerative colitis and Crohn's disease), chronic diseases that affect over 1 million people in the US alone and is increasing in prevalence worldwide [36],[37]. Understanding more about intracellular/surface moonlighting proteins, their mechanisms of secretion, their mechanisms for binding to the cell surface, their interactions with the host, and the competition with other species could be important for finding improved methods to prevent or treat many serious infections and chronic diseases of the gut for many people.
[1] |
Jeffery CJ (1999) Moonlighting proteins. Trends Biochem Sci 24: 8–11. doi: 10.1016/S0968-0004(98)01335-8
![]() |
[2] | Chen C, Zabad S, Liu H, et al. (2018) MoonProt 2.0: an expansion and update of the moonlighting proteins database. Nucleic Acids Research 46: D640–D644. |
[3] |
Kainulainen V, Korhonen TK (2014) Dancing to another tune-adhesive moonlighting proteins in bacteria. Biology 3: 178–204. doi: 10.3390/biology3010178
![]() |
[4] | Jeffery CJ (2018) Intracellular proteins moonlighting as bacterial adhesion factors. AIMS Microbiol 4: 362–376. |
[5] |
Hennequin C, Porcheray F, Waligora-Dupriet A, et al. (2001) GroEL (Hsp60) of Clostridium difficile is involved in cell adherence. Microbiology 147: 87–96. doi: 10.1099/00221287-147-1-87
![]() |
[6] | Yamaguchi H, Osaki T, Kurihara N, et al. (1997) Heat-shock protein 60 homologue of Helicobacter pylori is associated with adhesion of H. pylori to human gastric epithelial cells. J Med Microbiol 46: 825–831. |
[7] | Ensgraber M, Loos M (1992) A 66-kilodalton heat shock protein of Salmonella typhimurium is responsible for binding of the bacterium to intestinal mucus. Infect Immun 60: 3072–3078. |
[8] | Hoffman PS, Garduno RA (1999) Surface-Associated heat shock proteins of Legionella pneumophila and Helicobacter pylori: Roles in pathogenesis and immunity. Infect Dis Obstet Gynecol 7: 58–63. |
[9] |
Wampler JL, Kim KP, Jaradat Z, et al. (2004) Heat shock protein 60 acts as a receptor for the Listeria adhesion protein in Caco-2 cells. Infect Immun 72: 931–936. doi: 10.1128/IAI.72.2.931-936.2004
![]() |
[10] |
Jagadeesan B, Koo OK, Kim KP, et al. (2010) LAP, an alcohol acetaldehyde dehydrogenase enzyme in Listeria, promotes bacterial adhesion to enterocyte-like Caco-2 cells only in pathogenic species. Microbiology 156: 2782–2795. doi: 10.1099/mic.0.036509-0
![]() |
[11] |
Milohanic E, Pron B, Berche P, et al. (2000) Identification of new loci involved in adhesion of Listeria monocytogenes to eukaryotic cells. Microbiology 146: 731–739. doi: 10.1099/00221287-146-3-731
![]() |
[12] |
Castaldo C, Vastano V, Siciliano RA, et al. (2009) Surface displaced alfa-enolase of Lactobacillus plantarum is a fibronectin binding protein. Microb Cell Fact 8: 14. doi: 10.1186/1475-2859-8-14
![]() |
[13] |
Antikainen J, Kuparinen V, Lähteenmäki K, et al. (2007) pH-dependent association of enolase and glyceraldehyde-3-phosphate dehydrogenase of Lactobacillus crispatus with the cell wall and lipoteichoic acids. J Bacteriol 189: 4539–4543. doi: 10.1128/JB.00378-07
![]() |
[14] |
Patel DK, Shah KR, Pappachan A, et al. (2016) Cloning, expression and characterization of a mucin-binding GAPDH from Lactobacillus acidophilus. Int J Biol Macromol 91: 338–346. doi: 10.1016/j.ijbiomac.2016.04.041
![]() |
[15] |
Kainulainen V, Loimaranta V, Pekkala A, et al. (2012) Glutamine synthetase and glucose-6-phosphate isomerase are adhesive moonlighting proteins of Lactobacillus crispatus released by epithelial cathelicidin LL-37. J Bacteriol 194: 2509–2519. doi: 10.1128/JB.06704-11
![]() |
[16] |
Bergonzelli GE, Granato D, Pridmore RD, et al. (2006) GroEL of Lactobacillus johnsonii La1 (NCC 533) is cell surface associated: potential role in interactions with the host and the gastric pathogen Helicobacter pylori. Infect Immun 74: 425–434. doi: 10.1128/IAI.74.1.425-434.2006
![]() |
[17] |
Granato D, Bergonzelli GE, Pridmore RD, et al. (2004) Cell surface-associated elongation factor Tu mediates the attachment of Lactobacillus johnsonii NCC533 (La1) to human intestinal cells and mucins. Infect Immun 72:2160–2169. doi: 10.1128/IAI.72.4.2160-2169.2004
![]() |
[18] |
Kinoshita H, Uchida H, Kawai Y, et al. (2008) Cell surface Lactobacillus plantarum LA 318 glyceraldehyde-3-phosphate dehydrogenase (GAPDH) adheres to human colonic mucin. J Appl Microbiol 104: 1667–1674. doi: 10.1111/j.1365-2672.2007.03679.x
![]() |
[19] |
Candela M, Biagi E, Centanni M, et al. (2009) Bifidobacterial enolase, a cell surface receptor for human plasminogen involved in the interaction with the host. Microbiology 155: 3294–3303. doi: 10.1099/mic.0.028795-0
![]() |
[20] |
Candela M, Bergmann S, Vici M, et al. (2007) Binding of human plasminogen to Bifidobacterium. J Bacteriol 189: 5929–5936. doi: 10.1128/JB.00159-07
![]() |
[21] |
Crowe JD, Sievwright IK, Auld GC, et al. (2003) Candida albicans binds human plasminogen: identification of eight plasminogen-binding proteins. Mol Microbiol 47: 1637–1651. doi: 10.1046/j.1365-2958.2003.03390.x
![]() |
[22] | Gozalbo D, Gil-Navarro I, Azorin I, et al. (1998) The cell wall-associated glyceraldehyde-3-phosphate dehydrogenase of Candida albicans is also a fibronectin and laminin binding protein. Infect Immun 66: 2052–2059. |
[23] |
Jong AY, Chen SH, Stins MF, et al. (2003) Binding of Candida albicans enolase to plasmin(ogen) results in enhanced invasion of human brain microvascular endothelial cells. J Med Microbiol 52: 615–622. doi: 10.1099/jmm.0.05060-0
![]() |
[24] |
Luo S, Hoffmann R, Skerka C, et al. (2013) Glycerol-3-phosphate dehydrogenase 2 is a novel factor H-, factor H-like protein 1-,and plasminogen binding surface protein of Candida albicans. J Infect Dis 207: 594–603. doi: 10.1093/infdis/jis718
![]() |
[25] |
Lesiak-Markowicz I, Vogl G, Schwarzmuller T, et al. (2011) Candida albicans Hgt1p, a multifunctional evasion molecule: complement inhibitor, CR3 analogue,and human immunodeficiency virus-binding molecule. J Infect Dis 204: 802–809. doi: 10.1093/infdis/jir455
![]() |
[26] |
Yang W, Li E, Kairong T, et al. (1994) Entamoeba histolytica has an alcohol dehydrogenase homologous to themultifunctional adhE gene product of Escherichia coli. Mol Biochem Parasitol 64: 253–60. doi: 10.1016/0166-6851(93)00020-A
![]() |
[27] |
Jin S, Song YC, Emili A, et al. (2003) JlpA of Campylobacter jejuni interacts with surface-exposed heat shock protein 90-alpha and triggers signaling pathways leading to the activation of NF-kappaB and p38 MAP kinase in epithelial cells. Cell Microbiol 5: 165–74. doi: 10.1046/j.1462-5822.2003.00265.x
![]() |
[28] |
Raymond BB, Djordjevic S (2015) Exploitation of plasmin(ogen) by bacterial pathogens of veterinary significance. Vet Microbiol 178: 1–13. doi: 10.1016/j.vetmic.2015.04.008
![]() |
[29] | Collen D, Verstraete M (1975) Molecular biology of human plasminogen II Metabolism in physiological and some pathological conditions in man. Thromb Diath Haemorrh 34: 403–408. |
[30] |
Dano K, Andreasen PA, Grondahl-Hansen J, et al. (1985) Plasminogen activators, tissue degradation, and cancer. Adv Cancer Res 44: 139–266. doi: 10.1016/S0065-230X(08)60028-7
![]() |
[31] |
Wang W, Jeffery CJ (2016) An analysis of surface proteomics results reveals novel candidates for intracellular/surface moonlighting proteins in bacteria. Mol Biosyst 12: 1420–1431. doi: 10.1039/C5MB00550G
![]() |
[32] |
Han MJ, Lee SY, Hong SH (2012) Comparative analysis of envelope proteomes in Escherichia coli B and K-12 strains. J Microbiol Biotechnol 22: 470–478. doi: 10.4014/jmb.1110.10080
![]() |
[33] |
Bøhle LA, Riaz T, Egge-Jacobsen W, et al. (2011) Identification of surface proteins in Enterococcus faecalis V583. BMC Genomics 12: 135. doi: 10.1186/1471-2164-12-135
![]() |
[34] |
Garcia-del Portillo F, Calvo E, D'Orazio V, et al. (2011) Association of ActA to peptidoglycan revealed by cell wall prteomics of intracellular Listeria monocytogenes. J Biol Chem 286: 34675–34689. doi: 10.1074/jbc.M111.230441
![]() |
[35] |
Amblee V, Jeffery CJ (2015) Physical features of intracellular proteins that moonlight on the cell surface. PLoS One 10: e0130575. doi: 10.1371/journal.pone.0130575
![]() |
[36] |
Matsuoka K, Kanai T (2015) The gut microbiota and inflammatory bowel disease. Semin Immunopathol 37: 47–55. doi: 10.1007/s00281-014-0454-4
![]() |
[37] | Dahlhamer JM, Zammitti EP, Ward BW, et al. (2016) Prevalence of inflammatory bowel disease among adults aged ≥18 years-United States. MMWR 65:1166–1169. |
[38] |
Kinoshita H, Ohuchi S, Arakawa K, et al. (2016) Isolation of lactic acid bacteria bound to the porcine intestinal mucosa and an analysis of their moonlighting adhesins. Biosci Microbiota Food Health 35:185–196. doi: 10.12938/bmfh.16-012
![]() |
[39] |
Celebioglu HU, Olesen SV, Prehn K, et al. (2017) Mucin-and carbohydrate-stimulated adhesion and subproteome changes of the probiotic bacterium Lactobacillus acidophilus NCFM. J Proteomics. 163: 102–110. doi: 10.1016/j.jprot.2017.05.015
![]() |
[40] | Zhu D, Sun Y, Liu F, et al. (2016) Identification of surface-associated proteins of Bifidobacterium animalis ssp. Lactis KLDS 2.0603 by enzymatic shaving. J Dairy Sci. 99: 5155–5172. |
[41] | Celebioglu HU, Svensson B (2017) Exo-and surface proteomes of the probiotic bacterium Lactobacillus acidophilus NCFM. Proteomics. 17: 11. |
[42] |
Celebioglu HU, Delsoglio M, Brix S, et al. (2018) Plant polyphenols stimulate adhesion to intestinal mucosa and induce proteome changes in the probiotic Lactobacillus acidophilus NCFM. Mol Nutr Food Res. 62: 1700638. doi: 10.1002/mnfr.201700638
![]() |
[43] |
Celebioglu HU, Ejby M, Majumder A, et al. (2016) Differential proteome and cellular adhesion analyses of the probiotic bacterium Lactobacillus acidophilus NCFM grown on raffinose - an emerging prebiotic. Proteomics. 16: 1361–1375. doi: 10.1002/pmic.201500212
![]() |
[44] |
Pérez Montoro B, Benomar N, Caballero Gómez N, et al. (2018) Proteomic analysis of Lactobacillus pentosus for the identification of potential markers of adhesion and other probiotic features. Food Res Int. 111: 58–66. doi: 10.1016/j.foodres.2018.04.072
![]() |
1. | Ernesto Palma, Bruno Tilocca, Paola Roncada, Antimicrobial Resistance in Veterinary Medicine: An Overview, 2020, 21, 1422-0067, 1914, 10.3390/ijms21061914 | |
2. | Christine Bäuerl, José M. Coll-Marqués, Carmen Tarazona-González, Gaspar Pérez-Martínez, Lactobacillus casei extracellular vesicles stimulate EGFR pathway likely due to the presence of proteins P40 and P75 bound to their surface, 2020, 10, 2045-2322, 10.1038/s41598-020-75930-9 | |
3. | Thomas Cafiero, Alvaro Toledo, Borrelia burgdorferi Surface Exposed GroEL Is a Multifunctional Protein, 2021, 10, 2076-0817, 226, 10.3390/pathogens10020226 | |
4. | Joana Dias Ho, Luiz Eduardo Massao Takara, Denize Monaris, Aline Patrícia Gonçalves, Antonio Francisco Souza-Filho, Gisele Oliveira de Souza, Marcos Bryan Heinemann, Paulo Lee Ho, Patrícia Antonia Estima Abreu, GroEL protein of the Leptospira spp. interacts with host proteins and induces cytokines secretion on macrophages, 2021, 21, 1471-2180, 10.1186/s12866-021-02162-w | |
5. | Giacomo Braschi, Margherita D’Alessandro, Davide Gottardi, Lorenzo Siroli, Francesca Patrignani, Rosalba Lanciotti, Effects of Sub-Lethal High Pressure Homogenization Treatment on the Adhesion Mechanisms and Stress Response Genes in Lactobacillus acidophilus 08, 2021, 12, 1664-302X, 10.3389/fmicb.2021.651711 | |
6. | Olga S. Savinova, Olga A. Glazunova, Konstantin V. Moiseenko, Anna V. Begunova, Irina V. Rozhkova, Tatyana V. Fedorova, Exoproteome Analysis of Antagonistic Interactions between the Probiotic Bacteria Limosilactobacillus reuteri LR1 and Lacticaseibacillus rhamnosus F and Multidrug Resistant Strain of Klebsiella pneumonia, 2021, 22, 1422-0067, 10999, 10.3390/ijms222010999 | |
7. | Giulia Alessandri, Federico Fontana, Chiara Tarracchini, Sonia Mirjam Rizzo, Massimiliano G. Bianchi, Giuseppe Taurino, Martina Chiu, Gabriele Andrea Lugli, Leonardo Mancabelli, Chiara Argentini, Giulia Longhi, Rosaria Anzalone, Alice Viappiani, Christian Milani, Francesca Turroni, Ovidio Bussolati, Douwe van Sinderen, Marco Ventura, Identification of a prototype human gut Bifidobacterium longum subsp. longum strain based on comparative and functional genomic approaches, 2023, 14, 1664-302X, 10.3389/fmicb.2023.1130592 | |
8. | E. U. Poluektova, D. A. Mavletova, M. V. Odorskaya, M. V. Marsova, K. M. Klimina, T. A. Koshenko, R. A. Yunes, V. N. Danilenko, Comparative Genomic, Transcriptomic, and Proteomic Analysis of the Limosilactobacillus fermentum U-21 Strain Promising for the Creation of a Pharmabiotic, 2022, 58, 1022-7954, 1079, 10.1134/S1022795422090125 | |
9. | Maria Fiorella Mazzeo, Anna Reale, Tiziana Di Renzo, Rosa Anna Siciliano, Surface Layer Protein Pattern of Levilactobacillus brevis Strains Investigated by Proteomics, 2022, 14, 2072-6643, 3679, 10.3390/nu14183679 | |
10. | Basavaprabhu Haranahalli Nataraj, Chette Ramesh, Rashmi Hogarehalli Mallappa, Extractable surface proteins of indigenous probiotic strains confer anti-adhesion knack and protect against methicillin-resistant Staphylococcus aureus induced epithelial hyperpermeability in HT-29 cell line, 2021, 158, 08824010, 104974, 10.1016/j.micpath.2021.104974 | |
11. | A. N. MOROZOVA, A. E. AKHREMCHUK, N. A. GOLOVNEVA, FEATURES OF THE GENOME BIFIDOBACTERIUM LONGUM BIM B-813D, REFLECTING THE ADAPTATION OF BACTERIA TO THE ENVIRONMENT, 2021, 13, 2226-3136, 66, 10.47612/2226-3136-2021-13-66-76 | |
12. | Keita Nishiyama, Cheng-Chung Yong, Nobuko Moritoki, Haruki Kitazawa, Toshitaka Odamaki, Jin-Zhong Xiao, Takao Mukai, Danilo Ercolini, Sharing of Moonlighting Proteins Mediates the Symbiotic Relationship among Intestinal Commensals, 2023, 0099-2240, 10.1128/aem.02190-22 | |
13. | María J. Lorite, Ariana Casas-Román, Lourdes Girard, Sergio Encarnación, Natalia Díaz-Garrido, Josefa Badía, Laura Baldomá, Daniel Pérez-Mendoza, Juan Sanjuán, Impact of c-di-GMP on the Extracellular Proteome of Rhizobium etli, 2022, 12, 2079-7737, 44, 10.3390/biology12010044 | |
14. | Sébastien Massier, Brandon Robin, Marianne Mégroz, Amy Wright, Marina Harper, Brooke Hayes, Pascal Cosette, Isabelle Broutin, John D. Boyce, Emmanuelle Dé, Julie Hardouin, Phosphorylation of Extracellular Proteins in Acinetobacter baumannii in Sessile Mode of Growth, 2021, 12, 1664-302X, 10.3389/fmicb.2021.738780 | |
15. | Kairuo Wang, Yixuan Guo, Yuanyuan Liu, Xiao Cui, Xiang Gu, Lixiang Li, Yanqing Li, Ming Li, Pyruvate: Ferredoxin oxidoreductase is involved in IgA-related microbiota dysbiosis and intestinal inflammation, 2022, 13, 1664-3224, 10.3389/fimmu.2022.1040774 | |
16. | Despoina Eugenia Kiousi, Christos Efstathiou, Konstantinos Tegopoulos, Ioanna Mantzourani, Athanasios Alexopoulos, Stavros Plessas, Petros Kolovos, Maria Koffa, Alex Galanis, Genomic Insight Into Lacticaseibacillus paracasei SP5, Reveals Genes and Gene Clusters of Probiotic Interest and Biotechnological Potential, 2022, 13, 1664-302X, 10.3389/fmicb.2022.922689 | |
17. | Sergio Serrano-Villar, Camilla Tincati, Sajan C. Raju, Johan S. Sáenz, Elena Moreno, Rafael Bargiela, Alfonso Cabello-Ubeda, Elena Sendagorta, Alina Kurz, Jose A. Perez Molina, Amparo de Benito, Johannes R. Hov, Laura Fernandez-Lopez, Alfonso Muriel, Rosa del Campo, Santiago Moreno, Marius Trøseid, Jana Seifert, Manuel Ferrer, Microbiome-derived cobalamin and succinyl-CoA as biomarkers for improved screening of anal cancer, 2023, 29, 1078-8956, 1738, 10.1038/s41591-023-02407-3 | |
18. | Arghyadeep Bhattacharjee, Om Saswat Sahoo, Ahana Sarkar, Saurabh Bhattacharya, Rukhsana Chowdhury, Samarjit Kar, Oindrilla Mukherjee, Infiltration to infection: key virulence players of Helicobacter pylori pathogenicity, 2024, 52, 0300-8126, 345, 10.1007/s15010-023-02159-9 | |
19. | Miroslava Stastna, The Role of Proteomics in Identification of Key Proteins of Bacterial Cells with Focus on Probiotic Bacteria, 2024, 25, 1422-0067, 8564, 10.3390/ijms25168564 | |
20. | Xiao Liang, Nini Dai, Fan Yang, Haimei Zhu, Guanghui Zhang, Yongzhong Wang, Molecular identification and safety assessment of the potential probiotic strain Bacillus paralicheniformis HMPM220325 isolated from artisanal fruit dairy products, 2024, 15, 2042-6496, 747, 10.1039/D3FO04625G | |
21. | Rosa Estela Quiroz-Castañeda, Hugo Aguilar-Díaz, Itzel Amaro-Estrada, An alternative vaccine target for bovine Anaplasmosis based on enolase, a moonlighting protein, 2023, 10, 2297-1769, 10.3389/fvets.2023.1225873 | |
22. | Eucolona M. Toci, Ananya Majumdar, Caren L. Freel Meyers, Aldehyde‐based Activation of C2α‐lactylthiamin Diphosphate Decarboxylation on Bacterial 1‐deoxy‐d‐xylulose 5‐phosphate Synthase, 2024, 1439-4227, 10.1002/cbic.202400558 | |
23. | Dawei Chen, Congcong Guo, Chenyu Ren, Zihan Xia, Haiyan Xu, Hengxian Qu, Yunchao Wa, Chengran Guan, Chenchen Zhang, Jianya Qian, Ruixia Gu, Screening of Lactiplantibacillus plantarum 67 with Strong Adhesion to Caco-2 Cells and the Effects of Protective Agents on Its Adhesion Ability during Vacuum Freeze Drying, 2023, 12, 2304-8158, 3604, 10.3390/foods12193604 | |
24. | Munishwar Nath Gupta, Vladimir N. Uversky, Moonlighting enzymes: when cellular context defines specificity, 2023, 80, 1420-682X, 10.1007/s00018-023-04781-0 | |
25. | Nicole J. Curtis, Krupa J. Patel, Amina Rizwan, Constance J. Jeffery, Moonlighting Proteins: Diverse Functions Found in Fungi, 2023, 9, 2309-608X, 1107, 10.3390/jof9111107 | |
26. | Giovanna Monticelli, Joseph H. Bisesi, Jason T. Magnuson, Daniel Schlenk, Carlos Zarza, David Peggs, Daniela M. Pampanin, Effect of florfenicol administered through feed on Atlantic salmon (Salmo salar) gut and its microbiome, 2024, 580, 00448486, 740310, 10.1016/j.aquaculture.2023.740310 | |
27. | Timo M. Takala, Samira Mokhtari, Susanna L. Ahonen, Xing Wan, Per E. J. Saris, Wild-type Lactococcus lactis producing bacteriocin-like prophage lysins, 2023, 14, 1664-302X, 10.3389/fmicb.2023.1219723 | |
28. | Maria Fiorella Mazzeo, Alida Sorrentino, Stefano Morandi, Houssam Abouloifa, Abdeslam Asehraou, Milena Brasca, Rosa Anna Siciliano, Proteomics for depicting the secreted protein patterns of Lactiplantibacillus plantarum strains isolated from different food matrices, 2024, 60, 22124292, 104474, 10.1016/j.fbio.2024.104474 | |
29. | Despoina Eugenia Kiousi, Maria Panopoulou, Aglaia Pappa, Alex Galanis, Lactobacilli-host interactions inhibit Staphylococcus aureus and Escherichia coli-induced cell death and invasion in a cellular model of infection, 2024, 15, 1664-302X, 10.3389/fmicb.2024.1501119 | |
30. | Feray Gençer Bingöl, Duygu Ağagündüz, Ferenc Budán, Probiotic Bacterium-Derived p40, p75, and HM0539 Proteins as Novel Postbiotics and Gut-Associated Immune System (GAIS) Modulation: Postbiotic-Gut-Health Axis, 2024, 13, 2076-2607, 23, 10.3390/microorganisms13010023 | |
31. | Aya Osama, Ali Mostafa Anwar, Shahd Ezzeldin, Eman Ali Ahmed, Sebaey Mahgoub, Omneya Ibrahim, Sherif Abdelaziz Ibrahim, Ismail Abdelshafy Abdelhamid, Usama Bakry, Aya A. Diab, Ahmed A.Sayed, Sameh Magdeldin, Integrative multi-omics analysis of autism spectrum disorder reveals unique microbial macromolecules interactions, 2025, 20901232, 10.1016/j.jare.2025.01.036 |
Cytoplasmic Function | Cell Surface Function | Reference | |
Bifidobacteria | |||
Bile salt hydrolase | hydrolase | binds plasminogen | 20 |
DnaK | chaperone | binds plasminogen | 20 |
Enolase | hydratase | binds plasminogen | 19,20 |
Glutamine synthetase | synthetase | binds plasminogen | 20 |
Phosphoglycerate mutase | mutase | binds plasminogen | 20 |
Lactobacillus johnsonii | |||
Ef-Tu | elongation factor | Binds human cells and mucins | 17 |
Hsp60 | chaperone | binds mucins and epithelial cells | 16 |
Lactobacillus crispatus | |||
Enolase | hydratase | binds plasminogen and laminin | 13 |
Glucose 6-phosphate isomerase | isomerase | binds laminin, collagen | 15 |
Glutamine synthetase | synthetase | binds plasminogen, fibronectin, laminin, collagen | 15 |
Lactobacillus plantarum | |||
Enolase | hydratase | Binds fibronectin | 12 |
GAPDH | dehydrogenase | binds mucin and Caco2 cells | 18 |
Lactobacillus acidophilus | |||
GAPDH | dehydrogenase | binds mucin | 14 |
Clostridium difficile | |||
Hsp60 | chaperone | adhesin | 5 |
Helicobacter pylori | |||
Hsp60 | chaperone | adhesin | 6,8 |
Listeria monocytogenes | |||
Alcohol acetaldehyde | dehydrogenase | adhesin | 10 |
Hsp60 chaperone | chaperone | adhesin | 9 |
Ami autolysin | autolysin | adhesin | 11 |
Salmonella enterica serotype Typhimurium | |||
Hsp60 | chaperonin | adhesin | 7 |
Candida albicans | |||
Alcohol dehydrogenase (ADH1) | dehydrogenase | binds plasminogen | 21 |
Enolase | hydratase | binds plasminogen | 23 |
Fructose bisphosphate aldolase | aldolase | binds plasminogen | 21 |
GAPDH | dehydrogenase | binds plasminogen, fibronectin, laminin | 21,22 |
Peroxisomal catalase (CTA1) | catalase | binds plasminogen | 21 |
Phosphoglycerate kinase | kinase | binds plasminogen | 21 |
Phosphoglyceromutase | mutase | binds plasminogen | 21 |
Transcription elongation factor | elongation factor | binds plasminogen | 21 |
Thiol-specific antioxidant protein | antioxidant | binds plasminogen | 21 |
glycerol 3-phosphate dehydrogenase | dehydrogenase | binds plasminogen | 24 |
high-affinity glucose transporter 1 | sugar transporter | complement inhibitor | 25 |
Enteamoeba histolytica | |||
alcohol dehydrogenase (EhADH2) | dehydrogenase | Binds fibronectin, laminin, collagen | 26 |
Homo sapiens (human) | |||
Hsp90α | chaperone | binds to bacterial pathogens | 27 |
Cytoplasmic Function | Cell Surface Function | Reference | |
Bifidobacteria | |||
Bile salt hydrolase | hydrolase | binds plasminogen | 20 |
DnaK | chaperone | binds plasminogen | 20 |
Enolase | hydratase | binds plasminogen | 19,20 |
Glutamine synthetase | synthetase | binds plasminogen | 20 |
Phosphoglycerate mutase | mutase | binds plasminogen | 20 |
Lactobacillus johnsonii | |||
Ef-Tu | elongation factor | Binds human cells and mucins | 17 |
Hsp60 | chaperone | binds mucins and epithelial cells | 16 |
Lactobacillus crispatus | |||
Enolase | hydratase | binds plasminogen and laminin | 13 |
Glucose 6-phosphate isomerase | isomerase | binds laminin, collagen | 15 |
Glutamine synthetase | synthetase | binds plasminogen, fibronectin, laminin, collagen | 15 |
Lactobacillus plantarum | |||
Enolase | hydratase | Binds fibronectin | 12 |
GAPDH | dehydrogenase | binds mucin and Caco2 cells | 18 |
Lactobacillus acidophilus | |||
GAPDH | dehydrogenase | binds mucin | 14 |
Clostridium difficile | |||
Hsp60 | chaperone | adhesin | 5 |
Helicobacter pylori | |||
Hsp60 | chaperone | adhesin | 6,8 |
Listeria monocytogenes | |||
Alcohol acetaldehyde | dehydrogenase | adhesin | 10 |
Hsp60 chaperone | chaperone | adhesin | 9 |
Ami autolysin | autolysin | adhesin | 11 |
Salmonella enterica serotype Typhimurium | |||
Hsp60 | chaperonin | adhesin | 7 |
Candida albicans | |||
Alcohol dehydrogenase (ADH1) | dehydrogenase | binds plasminogen | 21 |
Enolase | hydratase | binds plasminogen | 23 |
Fructose bisphosphate aldolase | aldolase | binds plasminogen | 21 |
GAPDH | dehydrogenase | binds plasminogen, fibronectin, laminin | 21,22 |
Peroxisomal catalase (CTA1) | catalase | binds plasminogen | 21 |
Phosphoglycerate kinase | kinase | binds plasminogen | 21 |
Phosphoglyceromutase | mutase | binds plasminogen | 21 |
Transcription elongation factor | elongation factor | binds plasminogen | 21 |
Thiol-specific antioxidant protein | antioxidant | binds plasminogen | 21 |
glycerol 3-phosphate dehydrogenase | dehydrogenase | binds plasminogen | 24 |
high-affinity glucose transporter 1 | sugar transporter | complement inhibitor | 25 |
Enteamoeba histolytica | |||
alcohol dehydrogenase (EhADH2) | dehydrogenase | Binds fibronectin, laminin, collagen | 26 |
Homo sapiens (human) | |||
Hsp90α | chaperone | binds to bacterial pathogens | 27 |