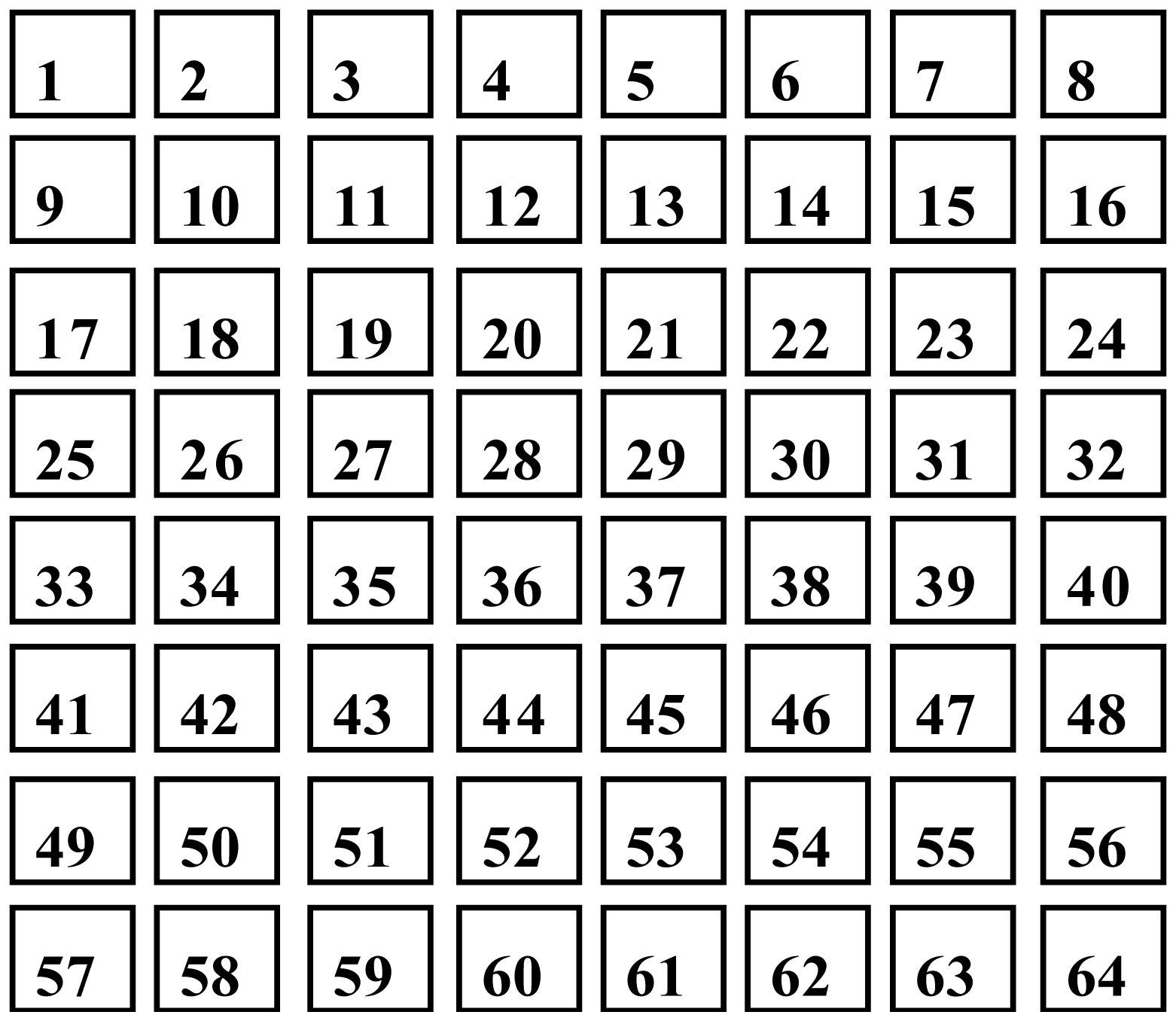
Citation: Yoshi Nishitani, Chie Hosokawa, Yuko Mizuno-Matsumoto, Tomomitsu Miyoshi, Shinichi Tamura. Effect of correlating adjacent neurons for identifying communications: Feasibility experiment in a cultured neuronal network[J]. AIMS Neuroscience, 2018, 5(1): 18-31. doi: 10.3934/Neuroscience.2018.1.18
[1] | Yoshi Nishitani, Chie Hosokawa, Yuko Mizuno-Matsumoto, Tomomitsu Miyoshi, Shinichi Tamura . Classification of Spike Wave Propagations in a Cultured Neuronal Network: Investigating a Brain Communication Mechanism. AIMS Neuroscience, 2017, 4(1): 1-13. doi: 10.3934/Neuroscience.2017.1.1 |
[2] | Yoshi Nishitani, Chie Hosokawa, Yuko Mizuno-Matsumoto, Tomomitsu Miyoshi, Shinichi Tamura . Learning process for identifying different types of communication via repetitive stimulation: feasibility study in a cultured neuronal network. AIMS Neuroscience, 2019, 6(4): 240-249. doi: 10.3934/Neuroscience.2019.4.240 |
[3] | Shinichi Tamura, Yoshi Nishitani, Chie Hosokawa . Feasibility of Multiplex Communication in a 2D Mesh Asynchronous Neural Network with Fluctuations. AIMS Neuroscience, 2016, 3(4): 385-397. doi: 10.3934/Neuroscience.2016.4.385 |
[4] | Shun Sakuma, Yuko Mizuno-Matsumoto, Yoshi Nishitani, Shinichi Tamura . Simulation of Spike Wave Propagation and Two-to-one Communication with Dynamic Time Warping. AIMS Neuroscience, 2016, 3(4): 474-486. doi: 10.3934/Neuroscience.2016.4.474 |
[5] | Shun Sakuma, Yuko Mizuno-Matsumoto, Yoshi Nishitani, Shinichi Tamura . Learning Times Required to Identify the Stimulated Position and Shortening of Propagation Path by Hebb’s Rule in Neural Network. AIMS Neuroscience, 2017, 4(4): 238-253. doi: 10.3934/Neuroscience.2017.4.238 |
[6] | Dirk Roosterman, Graeme S. Cottrell . Astrocytes and neurons communicate via a monocarboxylic acid shuttle. AIMS Neuroscience, 2020, 7(2): 94-106. doi: 10.3934/Neuroscience.2020007 |
[7] | Anastasios A. Mirisis, Anamaria Alexandrescu, Thomas J. Carew, Ashley M. Kopec . The Contribution of Spatial and Temporal Molecular Networks in the Induction of Long-term Memory and Its Underlying Synaptic Plasticity. AIMS Neuroscience, 2016, 3(3): 356-384. doi: 10.3934/Neuroscience.2016.3.356 |
[8] | Mohammad Mofatteh . mRNA localization and local translation in neurons. AIMS Neuroscience, 2020, 7(3): 299-310. doi: 10.3934/Neuroscience.2020016 |
[9] | Georgia Theocharopoulou . The ubiquitous role of mitochondria in Parkinson and other neurodegenerative diseases. AIMS Neuroscience, 2020, 7(1): 43-65. doi: 10.3934/Neuroscience.2020004 |
[10] | Ernest Greene . New encoding concepts for shape recognition are needed. AIMS Neuroscience, 2018, 5(3): 162-178. doi: 10.3934/Neuroscience.2018.3.162 |
The brain is a well-known large neuronal network assembled through spike propagation (action potentials) through synapses [1]–[5]. How can neuronal networks comprising neurons with fluctuating characteristics reliably communicate (transmit information)? Many previous studies have attempted to answer this question, using spike-coding metrics [6], spatiotemporal coding models [7]–[13], and synchronous action models [14]–[18]. Since neuronal networks are considered spatiotemporal spike propagation fields and since the spatiotemporal form of spike activity is considered the fundamental generator of intelligence in the brain, these studies primarily aimed to investigate the principles of spike propagation in detail; however, these studies could not elucidate the basic means of communication between neurons. Therefore, the mechanisms underlying communication in the brain remain unknown.
In our previous studies attempting to resolve this issue, we reported that spikes propagating from stimulated neurons are received by afferent neurons as random-like sequences in simulated and natural asynchronous neuronal networks [19]–[24]. This phenomenon is similar to radio wave propagation in artificial data communication systems; hence, this phenomenon was referred to as “spike wave propagation.” In these studies, we showed that stimulated neurons were able to identify various spatiotemporal patterns of spike wave propagation in specific areas (receiving area) of the neuronal network. From the viewpoint of communication, individual spike waves propagating from specific neurons are regarded as individual communication, thereby suggesting that distinct communications occur in multiple brain neuronal networks. In addition, certain adjacent neurons correlate to classify communications, in simulated neuronal networks [23].
On in-depth investigation, numerous neurons seem involved in communication, e.g., 3 neurons in the receiving area, and result in smooth and stable spike propagation, whereas fewer neurons make communication more difficult. This suggests a correlation between some adjacent neurons to improve the classification quality of communication in neuronal networks, similar to diversity antennae, used to improve the quality of communication in artificial data communication systems [25].
Although this observation was exclusive to simulation studies, remarkable similarities in the manner of correlation in some adjacent neurons for intelligence activity have been observed [26]. If these similarities hold true, they could provide evidence to determine the mechanism underlying communication in the brain. To accomplish this, we need to investigate whether the same phenomenon is observed physiologically as with simulation. Thus, this study aimed to investigate this phenomenon in a cultured neuronal network and determine if its physiological correlates are in line with those demonstrated in previous simulation studies.
Hippocampal neurons were dissected from Wistar rats on embryonic day 18. The procedure conformed to the protocols approved by the Institutional Animal Care and Use Committee of the National Institute of Advanced Industrial Science and Technology. Cell culturing, stimulated spike recording, and coding spike trains were performed as described previously [24]. Figure 1 depicts the deposition of an electrode in microelectrode array (MEA) dishes with 64 (8 × 8) planar microelectrodes (channels). In this study, we cultured 5 samples of neuronal networks named cultures 01, 02, 03 planted on MED-P515A (spacing between electrodes, 150 µm) and cultures 04 and 05 planted on MED-P545A (spacing between electrodes, 450 µm). Two channels in each culture were selected as stimulation channels and 5–20 recordings were obtained from them. In this study, the stimulated channels are referred to as StimA and StimB. This experiment aimed to classify the StimA and B in accordance with time sequence data based on spike trains in specific neurons (current channels) and their adjacent neurons. The difference in stimulation channel was regarded as the difference of spike wave propagation [24].
To estimate improvement in the classification quality of communication through correlation of adjacent neurons, as shown in Figure 2, we constructed time sequence data which linked the spike train interval of current channels (ch) with the time lag in spike train intervals between current and adjacent channels. Although Figure 2 shows only one adjacent channel for simplicity, several adjacent channels were actually referred. We compared the time sequence based on three types of adjacent channel locations, as shown in Figure 3. Moreover, time differences from the current channel are signs of delayed (+1) or preceding (−1) spike trains; however, this figure shows only delayed spike trains. Further, the encoded time sequence was generated from these time sequence data for the back propagation of neuronal networks (BPN) method described below in detail. These procedures were performed for all 64 channels in each culture.
On fluctuating conditions in neuronal networks, e.g., synaptic weight, refractory period, and others, spatiotemporal patterns of spike wave propagations were not the same even if they were stimulated at the same channel in the same culture. Therefore, identifying the stimulated channels by only observing wave propagation is not easy. In our previous study, we used our original simple learning algorithm based on the arithmetic mean method for classification [24]. However, the resolution was not adequate to classify spike wave propagations completely. Thus, we believe that the BPN method has moderately strong pattern recognition ability [27] and could hence be applied to show the feasibility of classifying spatiotemporal patterns of spike wave propagation in neuronal networks, in this study.
Round–Robin learning and the test procedure for the BPN method are depicted in Figure 4. This figure depicts the example of the encoded data number and stimulation channel (stim ch) number in the case of culture 01 (stim ch 13 vs 54).
To estimate the effect of correlation between current and adjacent channels and the classification quality, we calculated the rate of test data wherein the stimulation channel was detected correctly at the current channel in the BPN procedure; this was called “success rate ch” and the rate of test data wherein the stimulation channel was detected incorrectly (for example, in culture 01, the detection result was st54 when the real stimulating channel was channel 13) at current channel called fail rate ch. The equations for determining the success rate ch and fail rate ch are as follows:
In these equations, dtcablenumch is the number of test data when the stimulation channel was detected correctly at the current channel, test num is the number of test data (5 to 10), eronumch is the number of test data when the stimulation channel was detected incorrectly. In this study, we considered the success ratech > 60% as the classifiable channel. In other words, two different stimulations could correctly identify the classifiable channel. Furthermore, we regarded fail ratech > 60% as the miss-classifiable channel.
Figure 5 shows the number of classifiable channels and miss-classifiable channels in 64 channels in each culture in Single, Multi3, and Multi5 (Figure 3). Though, in some cases, the number of classifiable channels is smaller in Multi than in Single, contrary to our assumption similar to that in Culture 03, we could confirm that the mean number of classifiable channels in all experiments in Multi3 and Multi5 was significantly larger than that in Single (p < 0.05). However, the difference between Multi 5 and Multi 3 was not significant; furthermore, Multi 5 tended to be worse than Multi 3. Meanwhile, the number of miss-classifiable channels tended to be larger in Multi than in Single; however, in reality, they were fewer than the number of classifiable channels. These results show that the time lag in spike trains between adjacent neurons may effectively improve the quality of communication.
In these experiments, we could confirm the increase in the rate of classification through the schematic correlation of adjacent neurons. However, it remained unclear which neurons are classifiable by correlating adjacent neurons. Hence, we compared the distribution of classifiable channels in neuronal networks in Single and mul3 in Culture 1 and 3, as examples (Figure 6). We found that in culture 1, some unclassifiable channels in Single were classifiable in Multi3 (indicated by yellow lattices in Figure 6). Moreover, these channels tended to concentrate in specific areas of the neuronal network. These tendencies were also observed in culture 3 despite the number of classifiable channels in Multi3 being no more than that in Single, as shown in Figure 5(d). However, channels that classified only in Single (indicated by sky blue lattices in Figure 6) were also observed at similar numbers to that of the classifiable channels only in Multi3 in culture 03. In contrast, in culture 01, the number of channels which classified only in Single was lower than those that became classifiable in Multi3. The difference between these results in Figure 5(a) and (d) corresponded to this. However, spacing between electrodes did not affect the experimental results (150 µm: culture 01, 02, and 03; 450 µm: culture 04 and 05).
We considered the following reasons for these results. If various spike interval trains of trials are large even in the same stimulation channel, it is difficult to detect the stimulation channel of only the spike interval train in the current channel. However, if the time lag in the spike trains between the current channel and the adjacent channel does not display variety, stimulated neurons could only be detected from the train of the time lag. The image so obtained is shown in Figure 7. In this case, the stimulation channel is detectable from the difference in spike timing between the current channel and the adjacent channel. When spike interval trains in all experiments in the current channel did not display variety, only the spike train intervals in the current channel were adequate to detect the stimulation channel. The image so obtained is shown in Figure 8. In this case, it is possible that the rate of classification is worse based on the variety of spike trains in the adjacent channel.
To substantiate these assumptions, we decorrelated the current channel and adjacent channel by channel shuffling for all cultured samples; the procedure followed is shown in Figure 9. Thereafter, we performed the same experiments for the shuffled data, as for the original data.
Figure 10 shows the number of classifiable channels and miss-classifiable channels, and Figure 11 depicts the example of the distribution of classifiable channels in neuronal networks in Single and Multi3 for channel shuffled data of culture 01. Although data from other cultures are not shown owing to lack of space, results from these cultures were similar to those from culture 01, as described below. In this experiment, the detection of classifiable channels was performed only with Multi3, since it is adequate to determine the effect of neurons if this effect is observable in Multi3 or Multi5; however, it is considered that the results after channel shuffling remain the same as before channel shuffling in Single.
Multi3 mean values were remarkably lower than those of Single; however, there was no significant difference between their mean values in all cultures.
Based on the results from all cultures in this experiment, we could confirm that the number of classifiable channels in the shuffled data was significantly (p < 0.05) lower than that in the original data in Multi3 (Cf. Figure 10 vs Figure 5(a), and Figure 11 vs Figure 6(a)). However, the difference between Multi3 and Single was not significant in the shuffled data, although the values in Multi3 were lower than those in Single in several cultures including culture 01 (Figure 10), against those in the original data.
In summary, we could confirm the effect of spike timing lag between the current and their adjacent neurons. The higher number of classifiable channels in Multi3,5 than in Single was not a chance event. Considering that individual stimulation channels correspond to individual communication, in this study, although the classification quality of communications was not always improved, various communications could be characterized by the correlating spike trains of adjacent neurons even when they could not be characterized by only the current neuron.
In our recent study, we showed that stimulated neurons could identify various spatiotemporal patterns of spike wave propagation in particular areas of neuronal networks. From the viewpoint of communication, this essentially suggests that distinct communications occur via multiple communicating links in the brain. Here, we showed that the quality of communication classification tends to improve via correlation of spike trains in current and their adjacent neurons. This shows that neighboring neurons work in harmony to identify communication. Assuming a communication path is a type of memory, it seems that the present results are concurrent with previous ones, indicating that some adjacent neurons work in harmony; however, this requires more detailed investigation.
[1] | Bonifazi P, Goldin M, Picardo MA, et al. (2009) GABAergic hub neurons orchestrate synchrony in developing hippocampal networks.Science 326: 1419-1424. |
[2] | Lecerf C (1998) The double loop as a model of a learning neural system. Proceedings World Multiconference on Systemics.Cybernetics Informatics 1: 587-594. |
[3] | Choe Y (2002) Analogical Cascade: A theory on the role of the thalamo-cortical loop in brain function.Neurocomputing 52: 713-719. |
[4] | Tamura S, Mizuno-Matsumoto Y, Chen YW, et al. (2009) Association and abstraction on neural circuit loop and coding.IIHMSP 10-07: 546-549 (appears in IEEE Xplore). |
[5] | Thorpre S, Fize D, Marlot C (1996) Speed of processing in the human visual system.Nature 381: 520. |
[6] | Cessac B, Paugam-Moisy H, Viéville T (2010) Overview of facts and issues about neural coding by spike.J Physiol-Paris 104: 5-18. |
[7] | Kliper O, Horn D, Quenet B, et al. (2004) Analysis of spatiotemporal patterns in a model of olfaction.Neurocomputing 58: 1027-1032. |
[8] | Fujita K, Kashimori Y, Kambara T (2007) Spatiotemporal burst coding for extracting features of spatiotemporally varying stimuli.Biol Cybern 97: 293-305. |
[9] | Tyukin I, Tyukina T, Van LC (2009) Invariant template matching in systems with spatiotemporal coding: A matter of instability.Neural Networks 22: 425-449. |
[10] | Mohemmed A, Schliebs S, Matsuda S, et al. (2013) Training spiking neural networks to associate spatio-temporal input–output spike patterns.Neurocomputing 107: 3-10. |
[11] | Olshausen BA, Field DJ (1996) Emergence of simple-cell receptive field properties by learning a sparse code for natural images.Nature 381: 607-609. |
[12] | Bell AJ, Sejnowski TJ (1997) The “independent components” of natural scenes are edge filters.Vision Res 37: 3327-3338. |
[13] | Aviel Y, Horn D, Abeles M (2004) Synfire waves in small balanced networks.Neural Computation 58-60: 123-127. |
[14] | Abeles M (1982) Local Cortical Circuits: An Electrophysiological study Berlin: Springer. |
[15] | Abeles M (2009) Synfire chains.Scholarpedia 4: 1441Available from: http://www.scholarpedia.org/article/Synfire_chains. |
[16] | Izhikevich EM (2014) Polychronization: Computation with spikes.Neural Comput 18: 245-282. |
[17] | Perc M (2007) Fluctuating excitability: A mechanism for self-sustained information flow in excite arrays.Chaos Soliton Fract 32: 1118-1124. |
[18] | Zhang H, Wang Q, Perc M, et al. (2013) Synaptic plasticity induced transition of spike propagation in neuronal networks.Commun Nonlinear Sci 18: 601-615. |
[19] | Mizuno-Matsumoto Y, Okazaki K, Kato A, et al. (1999) Visualization of epileptogenic phenomena using crosscorrelation analysis: Localization of epileptic foci and propagation of epileptiform discharges.IEEE Trans Biomed Eng 46: 271-279. |
[20] | Mizuno-Matsumoto Y, Ishijima M, Shinosaki K, et al. (2001) Transient global amnesia (TGA) in an MEG study.Brain Topogr 13: 269-274. |
[21] | Nishitani Y, Hosokawa C, Mizuno-Matsumoto Y, et al. (2012) Detection of M-sequences from spike sequence in neuronal networks.Comput Intell Neurosci 2012: 167-185. |
[22] | Nishitani Y, Hosokawa C, Mizuno-Matsumoto Y, et al. (2014) Synchronized code sequences from spike trains in cultured neuronal networks.Int J Eng Ind 5: 13-24. |
[23] | Tamura S, Nishitani Y, Hosokawa C, et al. (2016) Simulation of code spectrum and code flow of cultured neuronal networks.Comput Intell Neurosci 2016: 1-12. |
[24] | Nishitani Y, Hosokawa C, Mizuno-Matsumoto Y, et al. (2016) Classification of spike wave propagations in a cultured neuronal network: Investigating a brain communication mechanism.AIMS Neurosci 4: 1-13. |
[25] | Hourani H (2004) Overview of diversity techniques in wireless communication systems, postgraduate course in radio communications. |
[26] | Buzsáki G (2010) Neural syntax: cell assemblies, synapsembles, and readers.Neuron 68: 362-385. |
[27] | Rumelhart DE, Hinton GE, Williams RJ (1986) Learning representations by back-propagating errors.Nature 323: 533-536. |
1. | Shinichi Tamura, Yoshi Nishitani, Chie Hosokawa, Yuko Mizuno-Matsumoto, Asynchronous Multiplex Communication Channels in 2-D Neural Network With Fluctuating Characteristics, 2019, 30, 2162-237X, 2336, 10.1109/TNNLS.2018.2880565 |