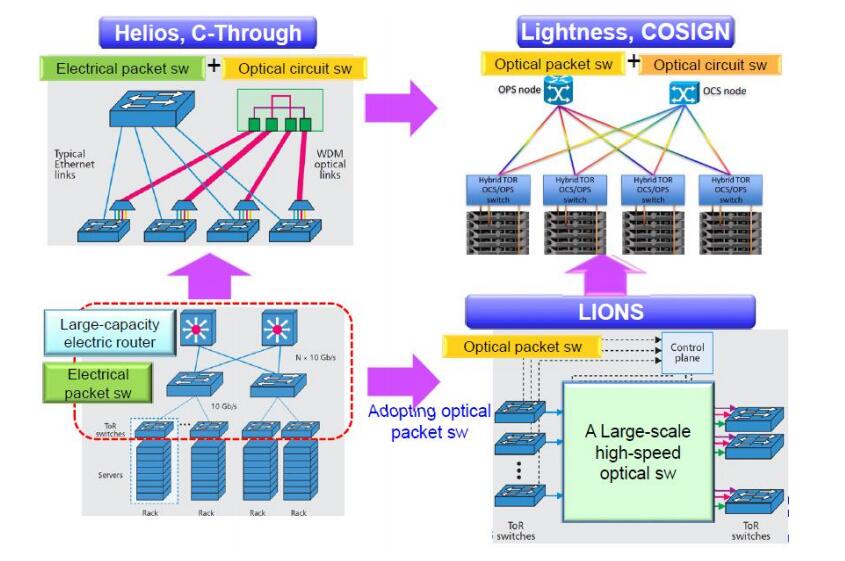
Citation: Efthymios N. Lallas. A survey on key roles of optical switching and labeling technologies on big data traffic of Data Centers and HPC environments[J]. AIMS Electronics and Electrical Engineering, 2019, 3(3): 233-256. doi: 10.3934/ElectrEng.2019.3.233
[1] | Minglong Zhang, Iek Cheong Lam, Arun Kumar, Kin Kee Chow, Peter Han Joo Chong . Optical environmental sensing in wireless smart meter network. AIMS Electronics and Electrical Engineering, 2018, 2(3): 103-116. doi: 10.3934/ElectrEng.2018.3.103 |
[2] | Hardeer Kaur, Jai Sukh Paul Singh . Software defined network implementation of multi-node adaptive novel quantum key distribution protocol. AIMS Electronics and Electrical Engineering, 2024, 8(4): 420-440. doi: 10.3934/electreng.2024020 |
[3] | Artem A. Nazarenko , Ghazanfar Ali Safdar . Survey on security and privacy issues in cyber physical systems. AIMS Electronics and Electrical Engineering, 2019, 3(2): 111-143. doi: 10.3934/ElectrEng.2019.2.111 |
[4] | B Naresh Kumar, Jai Sukh Paul Singh . Intelligence-based optimized cognitive radio routing for medical data transmission using IoT. AIMS Electronics and Electrical Engineering, 2022, 6(3): 223-246. doi: 10.3934/electreng.2022014 |
[5] | Shahzad Ashraf, Arshad Ahmad, Adnan Yahya, Tauqeer Ahmed . Underwater routing protocols: Analysis of link selection challenges. AIMS Electronics and Electrical Engineering, 2020, 4(3): 234-248. doi: 10.3934/ElectrEng.2020.3.234 |
[6] | Desh Deepak Sharma, Ramesh C Bansal . LSTM-SAC reinforcement learning based resilient energy trading for networked microgrid system. AIMS Electronics and Electrical Engineering, 2025, 9(2): 165-191. doi: 10.3934/electreng.2025009 |
[7] | Boualem Djehiche, Alain Tcheukam, Hamidou Tembine . Mean-Field-Type Games in Engineering. AIMS Electronics and Electrical Engineering, 2017, 1(1): 18-73. doi: 10.3934/ElectrEng.2017.1.18 |
[8] | Vuong Quang Phuoc, Nguyen Van Dien, Ho Duc Tam Linh, Nguyen Van Tuan, Nguyen Van Hieu, Le Thai Son, Nguyen Tan Hung . An optimized LSTM-based equalizer for 100 Gigabit/s-class short-range fiber-optic communications. AIMS Electronics and Electrical Engineering, 2024, 8(4): 404-419. doi: 10.3934/electreng.2024019 |
[9] | Suriya Priya R Asaithambi, Sitalakshmi Venkatraman, Ramanathan Venkatraman . Proposed big data architecture for facial recognition using machine learning. AIMS Electronics and Electrical Engineering, 2021, 5(1): 68-92. doi: 10.3934/electreng.2021005 |
[10] | Arebu Dejen, Jeevani Jayasinghe, Murad Ridwan, Jaume Anguera . Genetically engineered tri-band microstrip antenna with improved directivity for mm-wave wireless application. AIMS Electronics and Electrical Engineering, 2022, 6(1): 1-15. doi: 10.3934/electreng.2022001 |
The exponential growth rate of big data and IP traffic between interconnected Data Centers (DC) and High Performance Computing (HPC) systems have imposed the need for ultrahigh link capacities and ultrahigh packet switching speeds, at network nodes. In order to overcome these ultrahigh demands, and particularly packet routing and forwarding, long tested and established technologies such as optical switching and labeling technology, seem to provide adequate solutions, not only by conveying ultrahigh bit rate data streams, but also by achieving multi Tb/s cross connection throughputs, in a cost and energy efficient way. By adoption of optical switching and labeling technology, big data streams are propagating directly in optical layer, thus lessening down bottlenecks, latency issues, and multi stage hierarchy layering.
By 2020, predicted data production will be 44 times greater than that of 2009 [1]. As far as concerns cross connection switching hierarchy, the top-of-rack (ToR) switches, consisting of group of tens of server blades, has an agammaegation bandwidth of multi-Tb/s, while the interconnection of thousands of ToRs, would require a multiple total agammaegation bandwidth [2]. Adoption of optical labeling can support switching functionality, by employing optical labels attached to packets for identifying the destination ToR [3].
Conventional data center network architectures currently, have a large number of electrical switches and routers. All optical switching technology, comes at this point as a promising solution, offering high throughput, low latency, scalability, and reduced energy consumption, compared to electrical counterparts. In some cases, hybrid optical-electronic network architectures are proposed as well, but they require optical-electrical-optical (O/E/O) conversions, and multiple optical transceivers respectively, which unfortunately, consume more than 50% of the total power expenditure [4]. With all optical switching implementations, the all optical elements eliminate the use of costly and energy inefficient O/E/O conversions and vast amounts of electrical cabling required, thus improving energy and cost efficiency of the total layout. Moreover, they usually don't require multi-stage switching network implementations, as in electrical switching.
In this work, apart for the cost and energy efficiency and high bit rate throughput, many other issues and criteria of selecting the proper optical or hybrid switching architecture are examined, such as the criterion of application driven switching. Real application unpredictable traffic is normally, highly bursty with large variations, and also, bandwidth hungry streaming applications require constantly, by time to time, more and more bandwidth. In such cases, proper labeling methods must be adopted so as to provide traffic flow classification [5]. Particularly, for low volume, bursty data transmission, with large traffic fluctuations, usually optical packet switching (OPS) is the most appropriate switching type, maximizing also bandwidth utilization, while for high volume data transmission with constant latency and no packet loss, optical circuit switching (OCS) is the most suitable switching type. Hybrid switch architectures that combine both types of switching have been proposed, such as LIGHTNESS, or NTT's HOPR (hybrid optoelectronic packet routers) layout [6]. To make things more complicated, elasticity as a parameter should be considered as well, in a way of providing multiple BW flows, slicing spectrum resources dynamically, according to the running applications requirements [7].
Another criterion for adopting the proper switching architecture, concerns its implementation technology. There are several active optical component technologies that enable non blocking, spatial or wavelength-selective switching (WSS), such as micro-electromechanical systems (MEMS), liquid crystals on silicon (LCOS), semiconductor optical amplifiers (SOA), Mach-Zehnder interferometers (MZI) and micro-ring resonators (MRR) based switches, and passive component technologies, such as arrayed waveguide grating router (AWGR) based switches. Each one has its pros and cons and everything should be taken into consideration, for the finite switch layout selection.
After having decided about which switching architecture should be adopted, the next step, would be the decision for the right software based control plane to be applied, so as to enable the basic control functions of virtualization and orchestration of that large amount of heterogeneous network elements, of the switch layout. Software defined networking (SDN) paradigm, and its related protocols, such as OpenFlow, is a classic control plane solution [8].
On the other hand, according to the current SDN trends, the decoupling of big data control plane from the forwarding plane is strongly encouraged. In other words, this means that the generalized multi protocol label switching/path computation element (GMPLS/PCE) control paradigm, can be used for the supervision of forwarding plane, co-operating in parallel with SDN paradigm, used for big data control plane, in a complementary way [9]. SDN can greatly facilitate big data acquisition, with big data analytics and cross layer design optimization supported by machine learning techniques, while all optical label swapping (AOLS) which is actually GMPLS descendant, applied directly onto optical network layer, can be held responsible for the big data fast switching and forwarding.
Advanced multi-level modulation formats with high spectral efficiency and throughput, such as quadrature phase-shift keying (QPSK) and quadrature amplitude modulation (QAM), and their combinations with wavelength-division-multiplexing (WDM), orthogonal frequency division multiplexing (OFDM) and polarization-division multiplexing (PDM), are promising transmission techniques to increase the link capacity [10]. Currently, optical label swapping exploits these modulation schemes with new proposed labeling techniques. Apart from classic label swapping techniques, such as bit serial or parallel or orthogonal modulation or optical code (OC) labeling, there are also some new labeling techniques, such as hybrid 2-D or 3-D labeling, and spectral amplitude coded (SAC) labeling, that seem to give adequately an effective solution to ultrahigh link capacities and packet switching speeds of scalable, big data interconnected DCs and HPC environments, when combined with advanced coherent modulation techniques.
To our knowledge, many subjects of this survey have been investigated in the past, but individually and separately, such as the unified control plane for supporting optical layer infrastructure too, or the labeling and advanced modulation techniques. This is the first time that a thorough investigation, for examining all aforementioned issues together, is provided. The rest of the paper is structured as follows: In Section 2, there is an analytical consideration of all required criteria for choosing the proper all optical or hybrid optoelectronic switch architecture for DC and HPC communication. Section 3, is dedicated on justifying all the reasons of adopting a unified SDN based control plane for management and orchestration of optical switching elements, while in Section 4, all recent optical labeling techniques, in conjunction with advanced coherent multi level constellation transmission schemes, are examined for supporting fast routing and forwarding in DCs. Section 5, is a discussion on the pros and cons over all design issues mentioned in previous sections, and finally, Section 6 comes out with a final conclusion of the outcome of this survey.
Designing and employing the proper switching architecture for handling big data traffic of DCs and HPCs is really a complicated matter, that depends on many parameters. As mentioned, big data and IP traffic growth rate between DCs and HPCs, have imposed the need for ultrahigh link capacities and ultrahigh switching speeds, at network nodes. Moreover, in nowadays, hundreds of thousands of server systems are interconnected together, forming the so called hyper scale DCs, and hence, the scalability requirements are rapidly increasing day by day, demanding multi port switching elements with ultrafast switching speeds, at the lowest cost and energy consumption. Data center interconnects (DCIs) on the other hand, have been standardized at hundreds of Gb/s, requiring transponders at equal rates. Specifically, 40 Gb/s Ethernet-based DCIs have been deployed in production data centers since 2017 [11], 100 Gb/s DCIs, have been commercially available since 2014 [12], and currently, 400Gb/s transceivers are being standardized by IEEE 802.3 bs 400 Gb/s Task Force [13].
Conventional DC networks originally were consisted of electrical switches and routers in a fat-tree or folded Clos hierarchical architecture [14]. Unfortunately, such electronic switching architectures don't have great potentials on scalability growth, and they normally consume too much power, due to the large amount of network equipment and complex wiring required. On the contrary, power consumption of optical switching (Watt/bit) is much smaller than that of electrical systems [15], and they can definitely, operate at much higher switching rates. Hence, there is a strong need for electrical switching devices to be replaced by optical ones, in order to find a drastic solution against the continuously growing needs of DC switching architectures. All optical or hybrid optoelectronic switching architectures, have been proposed by researchers for such purposes, such as HOPRs [16], or LIONS (low-latency interconnect optical network switch) [17], or HOSA (Hybrid Optical Switch Architecture) [18], or LIGHTNESS [19], or RHODA (Reconfigurable Hierarchical Optical Data Center Architecture) [20], to name but a few.
Data traffic type requirements, as determined by the running applications, they are also going to have an impact on the choice of picking the appropriate switch layout. Specifically, hybrid schemes, that combine circuit switching for handling constant and bulky traffic streams, and packet switching for handling highly variable data traffic, and even, occasionally, burst switching for handling highly bursty traffic types, would be an ideal choice. However, in practice, it is quite hard and cost inefficient to combine these three types of switching under the same layout. Hence, an optical or hybrid switching scheme capable to combine OCS and OPS, would be an approximately close to the ideal choice of DC switch architecture. There is a reasonable trend of migration from electrical switching to optical switching, combining if possible both, circuit and packet switching types, as pictured in Figure 1 [21]. The arrows of Fig. 1, point out towards the direction of that migration trend, that also reflects the switching architecture evolution, at first, from conventional electronic to hybrid electrooptical, equipped with electrical packet switching (EPS) and OCS such as HELIOS (High Energy-efficiency Locally-scheduled Input-queued Optical Switch) scheme [22], and all optical layouts, equipped exclusively with OPS such as LIONS scheme [17], and finally to all optical schemes, equipped with both OCS and OPS, such as LIGHTNESS architecture [19].
Many other all optical or hybrid switching architectures have adopted packet or circuit switching, in order to support varying traffic patterns. In ref [23], an hybrid switching architecture is proposed, that uses EPS for handling variable data traffic, and OCS for constant heavy traffic, based on an application-driven traffic model. All optical switching schemes enable varying traffic profiles as well. Specifically, E-HOSA (Extended-Hybrid Optical Switch Architecture) [24] is using fast AWGR switches for OBS, and slow MEMS switches for OCS, while LIGHTNESS [4], adopts fast and slow switches for OPS and OCS.
Most of the current DC switching architectures are considered to be hierarchical, in a way that they group sets of server racks together, forming clusters. In a common DC switching architecture, the servers are arranged into racks and be connected to ToR switches, lying on top of them, managing the inter-server communications within rack, while several ToR switches are fully interconnected by a high-capacity cluster switch (intra cluster optical switch), forming a small-size cluster network, as seen in Figure 2. Higher capacity cluster switches (inter cluster optical switches), are capable of fully interconnect many small-size cluster networks, as also seen in Fig. 2. Such an hierarchical switch layer scheme, is RHODA, a WDM-based Reconfigurable Hierarchical Optical Data Center Architecture [20], which is capable of managing two types of traffic, heavy intra-cluster traffic among racks, and varying inter-cluster traffic, with reconfigurable link capacities, via employment of optical space switches and WSSs. All optical, flat, DC switching architectures can also provide in parallel, flexible intra/inter cluster traffic via OPS switching, employing distributed fast WDM optical cross-connect (OXC) switches and RF tone labeling in packets, to identify the ToR switch of each rack [3].
The type of switch matrix and its adopted implementation technology is also a design parameter of vital importance for consideration. All optical switch architectures normally employ three types of switches, free space switch type, implemented with MEMS, or with passive AWGR technology, and photonic (Ⅲ-Ⅴ or Silicon) integrated switches, implemented with MZI and MRR SOAs technologies. MEMS switches are considered to be rather slow switches, since they are operating at 10s of milliseconds switching rates [25], while AWGR switches in conjunction with tunable lasers (TLs) or tunable wavelength converters (TWCs), are much faster, with switching operating rates within a few nanoseconds [17]. The proper choice of the optical switch in a DC, is also depended on other metrics, such is its cost-per-port and reconfiguration time. The combination of AWGR with TLs or TWCs is very commonly used, due to its fast switching rates and its low implementation cost. DOS (Datacenter Optical Switch) [26], and Petabit [27] architectures, are based on that aforementioned optical element combination. Specifically, DOS scheme consists of an array of TWCs, an AWGR, and a loopback shared buffer, while Petabit scheme consists of a three-stage AWGR based Clos network, and TWs for routing packets to their destinations. PODCA (Passive Optical Data Center Network Architecture) [28], is an architecture, entirely made of cheap passive optical devices, such as AWGR and tunable transmitters, with high scalability potentials, it lacks however, reconfigurability, as it cannot adjust its topology according to varying traffic patterns. NEST [29], is also a highly scalable designed architecture, based on high throughput AWGR elements, and also being characterized by its symmetrical topology with a reduced network diameter, so as to achieve low latency performance. Photonic Sub-Lambda (PSL) Transport architecture [30] adopts passive AWGR switching elements as well, its strong feature is however, the resource allocation flexibility, as far as concerns BW resource allocation, since it can provide only a portion of lambda bandwidth per path, on contrast to all other optical DC switch architectures that waste BW resources, as they cannot offer portions smaller than lambda capacity. Such a flexible, multi flow BW provision, or else named elasticity, is an important issue, that will be discussed in the next section.
Buffering implementation for equipped optical or hybrid DC switching architectures, is also an important design parameter to be taken into consideration. Conventional, electronic buffered choices are considered to be power hungry, as they require multiple O-E-O conversions. All optical buffering implementation via the use of fiber delay lines (FDL), is a suggested promising solution. In ref [31], an hybrid optoelectronic architecture, which adopts OPS combined with EPS, exploits FDL optical buffering along with a deflection routing scheme, while in ref [32], three AWGR based optical packet switch designs are proposed, equipped with FDL based buffering, as well.
However, hybrid or all optical switching schemes that combine different types of switching such as OCS, OPS and OBS (optical burst switching), would normally require different hardware sets of control, thus making the management of the whole layout, quite costly and complicated. A unified control platform, for managing all heterogeneous technologies together, would be an effective solution for controlling a switching architecture. HOPRs architecture [16], which is the NTT's corporation official DC switching solution, is enabled with OPS, OCS, and virtual OCS, all managed by a single hardware platform, a unified OpenFlow controller of the SDN control paradigm. The role of a unified control plane upon heterogeneous switch entities is a critical issue, and it will be discussed in next session.
Finally, manufacturability potentials in terms of packaging and integration of all optical components, either passive or active (such as modulators, detectors, transmission sources and switching elements), composing a single switch layout, is critical for the implementation of a low-cost, energy efficient, switch package module, with ultrahigh spatial bandwidth density, enabling massive interconnections between vast data center deployments. Active components, such as VCSEL laser sources combined with WDM technology, or broadband optical modulators, such as electro-absorption modulators (EAMs) and Mach-Zehnder modulators (MZMs), used in combination with distributed feedback laser (DFB) arrays, or comb sources, or photonic (Ⅲ-Ⅴ or Silicon) integrated switches, they all, if possible, should be integrated onto the same substrate platform, so as to ensure low cost per port and high volume manufacturing integration [13]. Graphene based integrated photonics have been also considered as a promising, low cost and low power consumption solution, with ultrahigh spatial bandwidth density, for board connectivity and connectivity between DCs [33].
Large volume big data applications, such as streaming or high definition applications, normally require, not only high bit rate capacity and low latency, but also high power consumption, and flexible connectivity for efficient end to end service delivery. Current network infrastructure is mostly characterized by complexity and heterogeneity, with variable level required performance among its elements, while on the other hand, service provisioning requires flexibility, dynamic operation, and multi-vendor interoperability. Hence, there is a strong need, all these entities of different origin, to be governed and controlled by an, as much as possible, unified control plane, lying on top of layering hierarchy.
Optical networking technology, as known, seems adequate to face today's applications and big data traffic requests. GMPLS [34], which is an extension of multi protocol label switching (MPLS) [35] into broader type of switching data, along with centralized PCE [36], have been considered as established technologies for the control of optical networks, separating control and data planes, through label switching. However, they have been focused on inspecting mostly optical network elements, with distributed oriented control over them. SDN control paradigm [37] on the other hand, integrated with OpenFlow protocol platform [38], suits perfectly at this point, by offering centralized control onto dynamic, flexible optical network infrastructure. There is an urge for a unified control model, with orchestration approach across heterogeneous technologies and services, enriched with open standard interfaces, covering devices, networks, and service models. Yet, this unavoidable control platform migration, from traditional GMPLS/PCE to newly and promising SDN/OpenFlow, has a lot of unsolved issues, that have challenged many researchers interests.
As known, prior to GMPLS, there used to be MPLS, that exploited label switching mechanism, for providing separate services for the control and data planes. According to MPLS main principle, IP packets are forwarded through Label Switching Routers (LSR), forming the Label Switched Paths (LSPs), in a hop by hop basis, by exchanging labels between neighbor nodes. Labels are valid only for the current link between neighboring MPLS nodes, and can be also used to identify the forwarding equivalence class (FEC), thus supporting quality of service (QoS) provision. In GMPLS which succeeded MPLS, the switching process has been globally defined, supporting not only space or time switching, but also wavelength or waveband switching, and fiber switching, with labels to be mapped onto time slots or wavelengths, or band of wavelengths or fibers accordingly. GMPLS based optical switches, are transparent to payload data rates, modulation formats, and protocols, without the need to undergo onto O-E-O conversion. Moreover, GMPLS includes constrained based routing, that offers effective network resource management and traffic engineering (TE), thus simplifying the network operations by separating the forwarding and control operations. Basic protocols included in GMPLS for TE, are resource reservation protocol (RSVP)-TE for signaling, open shortest path first (OSPF)-TE for intra-domain routing, Intermediate System to Intermediate System (ISIS)-TE for inter-domain routing, and link management protocol (LMP) for link management. PCE protocol, as defined by its name, has been designed for path computation in optical networks. Its integration with GMPLS led to GMPLS/PCE control platform for performing on demand, dynamic optical network control and management [39]. A Network Management System (NMS) along with its interface (NMI), ensures a unified view of the GMPLS plane, consisting of connection controller modules (CC) and corresponding interfaces (CCI), and consequently of the data plane, consisting of network elements (NE) along with its physical interfaces (PI), as seen in Figure 3. AOLS paradigm, completely implemented at the optical transport layer by optical routers equipped with all optical switching elements, has succeeded GMPLS, and has been established to offer exclusively, label swapping function [40].
SDN on the other hand, is definitely a centralized control model architecture that consists of 3 layer activities: the infrastructure layer, the control layer, and the application layer. The control layer is related to SDN's key role activity, which is the control, and the dynamic and on demand provisioning of connectivity services between network entities, which communicate via proper interfaces, across various domains and vendors, assuring inter-operability between them. The other two application and infrastructure layer activities, are related to each other, since, within SDN software architecture, the network is programmable through software applications, running on top of the network operating system (NOS), which interacts with the underlying data plane devices [41]. Due to its centralized nature, SDN control plane, has a global view of the network, and the decisions are all taken globally by a single entity, the controller, the so called brain of the network. The interface, by which a controller communicates with devices, is named South Bound Interface (SBI), while the set of Application Programming Interfaces (APIs) offered to applications, is named the North Bound Interface (NBI) [42]. The whole SDN architecture and its interfaces can be seen in Figure 4. Infrastructure layer and its network elements can be controlled via OpenFlow and NETCONF protocols, fully complied to SBI standards, as also seen in Fig. 4. There are also horizontal activity interfaces, such as the West Bound Interface (WBI) for interconnecting multiple SDN domains, and the East Bound Interface (EBI) for interconnecting non-SDN domains [43].
As mentioned, SDN's basic feature is the decoupling of big data control plane from the forwarding plane, providing the chance for both planes to be controlled together, in a complementary way, via SDN/OpenFlow and GMPLS/PCE paradigms, respectively. The control plane performs tasks like scheduling, traffic matrix, routing, and switch configuration, while the data plane performs data forwarding on already set paths, by the controller. SDN can greatly facilitate big data acquisition, with big data analytics and cross layer design optimization, supported by machine learning techniques, while GMPLS or more specifically AOLS, can be held responsible for the big data rapid switching and forwarding, thus giving pace for integration potentials between the two platforms. SDN/GMPLS integration has been considered by researchers as an option, which can be simply achieved, via the addition of proper functional and signaling extensions to PCE protocol, which apart from being originally designed for serving path computation for GMPLS, it has been also used as an SDN interface, as well. Alternatively, OpenFlow protocol has been designed for SDN controller, in order to exclusively inspect optical network elements, as originally, GMPLS used to (Fig 4). Hence, control on all these heterogeneous network elements, including optical equipment, is feasible, via a unified control platform.
SDN with OpenFlow, is feasible to control a wide portfolio of optical infrastructure elements, such as optical flexible bandwidth variable transceivers (e.g. BVTs) supporting any kind of flexible modulation format (QPSK 8QAM and 16QAM), circuit, packet and burst switches of any type (e.g. WSS), optical amplifiers, and all types of multiplexing, such as WDM, TDM SDM (wavelength, time and space respectively), due to its integration with GMPLS. Nephele, is a remarkable paradigm of centralized SDN control and orchestration on managing the underlying data plane by adopting TDMA, in order to dynamically assign network resources directly in the optical layer. In ref [44], there is a remarkable approach for SDN to control reconfigurable OADM, which is the basic component for core and metro DC network deployment, with the adoption of OpenROADM software architecture.
The great challenge for SDN, in order to manage the optical network efficiently and improve the performance of big data applications, would be its capability to be continuously adapted and adequately responded to the flexible resource requirements of big data applications, as they tend to change dynamically in cloud data centers. It is very common for big data service providers and users to negotiate with each other, in order to define resource requirements of running applications, via settling down service level agreements (SLAs). SLAs define the characteristics of the provided big data services, including service level objectives, expected QoS and penalties if those objectives are not met, flexible elastic BW provision per path, optical power control, quality of transmission (QoT) guarantee via RWA/RSA (Routing Wavelength Assignment/Routing and Spectrum Allocation) algorithms [45], and other parameters, strictly related to the ultimate goal of SDN, which is the optimization of a guaranteed transmission performance of optical data plane. These targets can be all achieved, by enhancing the control plane of SDN with the functionalities of orchestration and network function virtualization (NFV). According to the Open Networking Foundation (ONF), orchestration is defined as the optimum selection of the proper resources to satisfy service demands, considering that all the available resources, the service demands and the optimization criteria can dynamically change [46]. Additionally, NFV, is responsible for the coordination of the resources and networks, needed to set up cloud-based services and applications, via the use of virtualization software platforms (e.g. OpenStack) and industry standard hardware control across multiple heterogeneous domains (could be either control and data plane domains), as depicted in Figure 5 [47].
Particularly, for the case of SDN over optical network layer, the term of NFV is referred to the virtualization of network functions, so that multiple virtual networks can operate over a given installed physical network infrastructure. Such orchestrated virtual network functions (VNF), are running on virtual machines (VM) located at distributed DCs, and controlled by a virtual machine manager. Optical network infrastructure domain, consisted of multiple heterogeneous sub-domains, such as administrative boundaries, technologies and protocols, needs to be integrated efficiently with automation, into such service and resource orchestration platforms. With the enhanced programmability of SDN control plane, or alternatively GMPLS control plane, provided by NFV, there is great potential for greater flexibility, dynamic operation, and multi-vendor compatibility for optical systems. Big data will benefit from SDN, in improving traffic engineering, cross-layer design, security architecture and other areas of network control.
Artificial intelligence (AI) subject area, based on computational efforts of deep learning (DL) and machine learning (ML) algorithms has helped a lot, DCN architectures with SDN control planes, by providing a supportive, knowledge structured network orchestration mechanism, that improves the SDN's orchestration and NFV, thus managing more efficiently all these heterogeneous network entities, seen as VMs, and consequently achieving high performance service provisioning and flexible resource utilization. Knowledge defined networking (KDN) of ref [48], is a characteristic knowledge based network control paradigm, based on a KDN controller with a global view and total control over the network, that collects remotely all the necessary information, adopts AI for data analytics and DL to compose abstract knowledge afterwards, so as to finally, make compact decisions and to achieve highly efficient network control and orchestration. A knowledge defined network orchestration mechanism (NO-M), which operates on a hybrid optical/electronic DCN (HOE-DCN), is also proposed in ref [49], adopting predictive analytics and intelligent decisions, in order to improve the performance of service provisioning and system energy efficiency.
As far as concerns optical network infrastructure, there is a strong need and motivation for adopting AI and ML mechanisms for more efficient SDN based control of network. Apart from the conventional BW hungry and ultra high switching rate requirements, also advanced optical interconnections for modern applications, impose needs for more complex performance and QoS provision, in terms of processing power, response time, and data quality, thus increasing system complexity and heterogeneity, as well. Concerning physical layer, there are critical figures of merit, such as QoT estimation, optical amplifiers control, modulation format pattern recognition (MFR), nonlinearity control via optical power equalization, optical performance monitoring (OPM), that need to be inspected, analyzed, and corrected according to predictive analyses that ML techniques normally do, as seen in Figure 6 [50]. Concerning network layer, there are also critical actions that SDN must be dealt with, via adoption of ML techniques, such as traffic prediction, virtual topology design (VTD) and reconfiguration, failure management, traffic flow classification, and path computation, as also seen in Fig. 6 [50]. Traffic engineering would become more efficient by dynamically analyzing, predicting, and regulating the behavior of data transmitted over that network, thus balancing data load and maximizing network utilization, and improving network performance. With path computation, topology reconfiguration and traffic flow classification, done by modifying flow tables within the switches, any flow format of big traffic data with arbitrary granularity can be adjusted for efficient traffic engineering. Traffic flow classification would also improve flexible QoS, end to end provision and management, and discrete classification of network users. Cloud performance would then be matched with those improvements of network performance, allowing for application triggered Automatic Cloud-Network resource coordination, as proposed in ref [51].
Elastic Optical Network (EON) paradigm is considered to be the valid result of such a flexible resource utilization of optical data plane [7], capable to respond to the increased need of elasticity, via re-allocating optical network resources. Resource allocation in EON, else named flexi-grid or mixed line rate (MLR) network, is performed according to upper layer decisions, that concern flexible resource utilization, and include various multi carrier modulation techniques (OFDM, Nyquist WDM), bandwidth variable transponder types (e.g., BVT1, S-BVT) and wavelength cross-connects (BV-WXCs), advanced modulation formats (e.g., QPSK, QAM), multi coding rates, elastic access to sliced spectrum resources within flexible frequency grids, and even label swapping mechanisms, as proposed in ref. [52]. In this ref, the optical label is swapped at the receiver node with a flex grid WSS, which is controlled by the SDN plane. The basic advantage of EON lies in is its feasibility to allocate dynamically, variable sized optical bandwidth, by contiguous concatenation of optical spectrum, to a wavelength path, according to current traffic demands. There is the flexible ability of BW provisioning, either at sub-wavelength scale for low rate application requirements, or multiple wavelength superchannel connectivity for accommodating ultra-high capacity application requirements [53]. AOLS is the mechanism to be used in conjunction with super channel switching, in order to provide flexi grid resources according to the current running application demands. Specifically in ref [54], a labeled superchannel switching for flexible tailoring of available spectrum, depended on running applications needs, is experimentally proposed. Flexible BW superchannel generation is feasible with conventional label swapping methods. Multiple or sub granularity spectrum resource provisioning combinations are proposed in ref [55] for establishing or restoring a lightpath. Various other subcarrier based spectrum slicing schemes are proposed, in which the available subcarriers are either forming a single superchannel, or they independently are routed along different paths, or forming a noncontiguous spectrum along the same path. In ref [56], a flexi-grid elastic optical path network is experimentally tested, which is controlled by an SDN/OpenFlow platform for its flexible resource provisioning. Combinations of both fixed-grid and flexible-grid optical networks, controlled by a unified, SDN/OpenFlow control plane, are also feasible, as proposed in ref [57]. Finally, flexi grid technology should also be considered in conjunction with optical power control. Refs [58] and [59], indicate that flexible spectrum allocation will be benefited from optical power control of amplifiers of the available channels, in case of highly loaded links.
AOLS has been considered for many years as an established solution for providing ultrahigh link capacities and packet switching speeds imposed by interconnected DCs and HPC environments. As known, originally, label swapping process was entirely carried out at the electronic level, at intermediate nodes via the MPLS paradigm. In order to improve the efficiency, the scalability and throughput of the network, the AOLS technique has been proposed [60], where the packet routing and forwarding functions are carried out directly in the optical layer. AOLS entirely beats the electronic bottleneck of conventional switches and diminishes the transmission delay as it completely avoids O-E-O signal conversions. AOLS critical functions include all optical payload and header generation at the ingress node, all optical label replacement at each intermediate node, and finally, separate payload and label detection at the egress node.
According to the way optical label is attached to the payload, many methods have been proposed for implementing AOLS, which they can be categorized into two basic conventional types: serial labeling techniques, where the label is placed bit serial to the payload [61], and parallel labeling techniques, where the label is processed in parallel with the payload. Concerning parallel labeling, the label could be either multiplexed on a single wavelength [62] or a wavelength band [63], or on a subcarrier frequency [64], or at different orthogonal modulation combined schemes e.g. intensity modulated (IM) payload with frequency shift keying (FSK) [65] or differential phase shift keying (DPSK) label [66], or at different code, such as optical code (OC) labeling [67]. Recently, hybrid labeling techniques have been proposed, which are combinations of the aforementioned techniques, such as 2-D labeling which exploits separate lambdas and separate codes as 2 label dimensions respectively [68], or 3-D labeling formed by 2-D labeling, with the addition of separate polarizations as 3rd label dimension [69], and some other, newcomers labeling techniques, such as spectral amplitude coded (SAC) labels with label stack [70], normally associated with advanced coherent modulation formats for enhancing data rates and spectral efficiency [71].
Spectral amplitude codes with optical label stacking is considered to be a sub category of OC labeling, and it is based on the encoding of incoherent broadband light sources in the frequency domain. Specifically, WDM and OC labeling have been used as identifying labels, with the labels to be permutated among the wavelengths or code dimensions, forming a variable label stack. There are two ways of forming SAC optical packets, one based on separable SAC labels and the other based on SAC-encoded payloads. In the first approach, the SAC label is a collection of spectral tones modulated at the packet rate and lasts for the entire packet time frame, with the payload carried on a separate wavelength modulated at the data rate (Figure 7a). In the second approach, the payload data modulates a band of wavelengths that constitute the code of the SAC label, and the label now is implicit in payload bits, as seen in Figure 7b [72].
Advanced coherent modulation techniques such as QAM or differential QPSK (DQPSK) combined with PDM, are used, to improve the optical communication and spectral system efficiency. Figure 8 shows the evolution and increase of switching speeds through the years up to present and the combination of coherent modulation techniques with PDM, so as to increase the optical communication system efficiency [73]. Coherent modulation is characterized by its flexibility in modulation formats, as information can be encoded in amplitude and phase, forming the in-phase (I) and quadrature (Q) components of a carrier. Employing DQPSK differential modulation, 4 bits per symbol can be used, while additionally by combining PDM, the transmission capacity will be multiplied, as the original signal can be transmitted over two orthogonal states of polarization on the same carrier. Hence, via combination of both PDM with DQPSK techniques, the transmission capacity of the data signal will be multiplied at rates reaching even tens of Tb/s.
However, there is always a trade off to be taken into consideration, as far as concerns the employment of intensity modulation-direct detection (IM-DD) schemes or coherent schemes in nowadays communication systems. Specifically, IM-DD schemes have simpler implementation requirements, consume less power, and cost less, while coherent modulation schemes, require more sophisticated digital signal processing (e. g. local oscillator for detection), and hence they are more expensive and consume more power [74]. Modulation levels with a larger number of bits per symbol consume more power than those with a lower number of bits per symbol, but they are more efficient, since the ratio of power consumed per bit is higher [75]. IM-DD has advantages in terms of equipment cost and simplicity and power consumption, while coherent advanced schemes yield a higher spectral and transmission efficiency, which as mentioned, is an urged need to be prioritized, pressed by scalable DCs and HPC environments. Currently, researchers are focused on providing simple and cost effective coherent PDM-QPSK schemes, such as the one of ref [76], which proposes a 32 Gb/s PDM-QPSK payload with a 2.5 Gb/s On Off keying (OOK) label, based on a simple Mach–Zehnder configuration.
Transmission performance of a DC architecture, can be further enhanced, by adopting advanced coding schemes, such as space-time coding with the aforementioned 4 dimensional (4D) combined formats of PDM and quandrature coherent modulation schemes [77]. Normally, such orthogonal space-time codes (STC) are considered as linear space-time block codes (STBC) which have a lower implementation complexity than trellis codes [78], and hence they are preferred for transmission combinations with 4D constellation schemes for enhanced transmission performances. Such a coded combination of a FEC code with 4D multi level constellations is also proposed in ref [79].
Labeling techniques would also have to be combined with advanced coherent modulation formats, as the latter are preferred for achieving higher communication efficiency. Hence, new labeling techniques such as hybrid 2-D or 3-D labeling, and SAC labeling, with the support of advanced coherent modulation techniques, seem to give adequately an effective solution to ultrahigh link capacities and packet switching speeds of scalable DCs and HPC environments. In [80] and [71], a simulation setup of a PDM-DQPSK modulated SAC labeling scheme for a 100 GHz channel spacing WDM system at 80 and 112 Gb/s rates is presented respectively. In this method, a polarization tracker, is used to recover the orthogonal polarization state of the payload signal in order to eliminate the effects of polarization mode dispersion (PMD) and polarization dependent loss (PDL) effects, which is the main drawback of these methods. Apart from SAC based labeling, other novel labeling schemes are proposed, such as the 100Mb/s polarization shift keying (PolSK) labels for 100 Gb/s dual-polarization quadrature phase-shift keying (DP-QPSK) signals of ref [81], or the two, orthogonal duobinary (DB) label and pulse position modulation (PPM) label switching schemes, which are based on polarization multiplexing-differential quadrature phase shift keying (POLMUX-DQPSK or PDQ) modulated payload of ref [82].
AOLS labeling would also have to be complied to the EON paradigm, that supports elastic resource and flexible QoS provisioning, via a unified SDN control plane, as discussed in the previous section. For future DCs and HPC environments, flexible and dynamic AOLS for hundreds of Gb/s flows (100–400 Gb/s) would be required, along with OPS technology, so as to enable ultra high bit rate packet routing and forwarding directly in the optical layer, and higher layer software functions for control purposes. The system should be able to provide the full lightpath bandwidth via grooming multicast labeling, or use sub wavelength granularity for unicast purposes, since most of the traffic needs require a portion of wavelength resources. To enable dynamic and on-demand AOLS, a unified SDN based control on all optical switching elements (e.g. WSS) of data plane is required, in conjunction with an OpenFlow API. In ref [52] a dual Nyquist-shaped carrier for generating a 400-Gb/s PM-16QAM payload with 75 GHz spacing and a 10 Gb/s Ethernet label, is proposed, all controlled by an SDN controller. By dynamically shifting the center frequency and WSS pass band width, 10 Gb/s Ethernet label spectra, can be placed on either side of a 400 Gb/s payload signal that occupies 75 GHz bandwidth, so that the agammaegate bandwidth to be kept less than 100 GHz, thus complying with a 100 GHz WDM grid. Also in ref [83], a novel multicast grooming labeling scheme is proposed, based on wavelength BW resource provision and FDL, to simultaneously address unicast and multicast transfer, traffic grooming and QoS provision, with respect to the optical resource utilization and resource availability, in the presence of several traffic types and user requirements. Specifically, two level labeling is adopted for handling the resource requests and resource allocation. AOLS as mentioned in previous section, can be used in conjunction with super channel switching in order to provide flexi grid resources as in ref [54], where a labeled superchannel switching for flexible tailoring of available spectrum is proposed, or in ref [55] where multiple or sub granularity spectrum resource provisioning combinations are proposed via agammaegated or independently routed subcarriers.
It is a certain fact that, as scalability requirements are rapidly increasing day by day, and DC interconnects, have already been standardized at hundreds of Gb/s, all optical switching technology, comes as a promising solution to replace conventional electronic DC network architectures, by offering high throughput and scalability potential, low latency, and reduced costs and energy consumption. Optical switch architectures with their multi port switching elements and ultrafast switching speeds, don't require multi-stage switch layouts, while electronic switching architectures require quite large amount of network equipment and complex wiring, and thus have no potential for scalability growth.
Currently, running applications require specific data traffic patterns, that switching layouts will have to comply with. Specifically, real application traffic can be unpredictable, highly bursty with large data flow variations, while streaming applications are constantly bandwidth hungry, requiring large amounts of bandwidth. Hybrid optical-electronic network architectures that combine circuit switching for handling constant and bulky traffic streams, and packet switching for handling highly variable data traffic, would be an ideal choice, but they are less energy efficient than all optical counterparts, since they require considerable energy for their O/E/O conversions. Hence, all optical or hybrid switching schemes that combine both all optical switching types (OCS and OPS) would be an approximate to ideal choice for DC switching architecture deployment. Moreover, these switching architectures will have to be hierarchical, in order to be able to manage these two types of traffic, heavy intra-cluster traffic among racks, and varying inter-cluster traffic patterns, with reconfigurable link capacities, by using appropriate optical switching elements (e.g. WSSs). Elasticity as a means of providing multiple BW flows, and slicing spectrum resources in variable ways, according to the running applications requirements, should be taken into consideration as well.
Implementation of the total switch layout is also a critical design issue, since it is directly related to cost and energy consumption. There are several technologies for building active, non blocking components such as, WSS, MEMS, SOAs, MZIs and MRR based switches, and passive components technologies as well, such as AWGR based switches, which they are naturally much more energy and cost efficient than their former active competitors. The proper choice of the optical switch in a datacenter is also depended on metrics such as cost-per-port and reconfiguration time. All optical switch types could be either free space switches, implemented with MEMS or alternatively with AWGR technology, or could be photonic (Ⅲ-Ⅴ or Silicon) integrated switches, implemented with MZI and MRR SOAs technologies. MEMS switches are slow switches while AWGR based switches in conjunction with TLs or TWCs, are much faster, and they are commonly used for core and metro DCs due to their low cost implementation. Moreover, conventional electronic switching architectures with buffering, are considered to be power hungry as they require multiple O-E-O conversions, and hence, all optical buffering implementation is suggested via the use of FDLs.
Packaging and integration of all optical components, of a switch layout, is also of vital importance for the implementation of a low-cost, energy efficient single switch package, with ultrahigh spatial bandwidth density. Active components such as VCSEL lasers, EAMs and MZMs modulators, DFB laser arrays, and photonic (Ⅲ-Ⅴ or Silicon) switches, they all together should be integrated onto the same substrate platform, so as to ensure low cost per port and high-volume manufacturing integration. Graphene based integrated photonics is considered as a promising, low cost and low power consumption solution with ultrahigh spatial bandwidth density, for board connectivity and connectivity between DCs.
On the other hand, there is an urged need for all current dynamically evolving, network switch infrastructure, which is mostly characterized by complexity and heterogeneity among its components, flexible service provisioning, dynamic operation, and multi-vendor interoperability, to be controlled by a unified control plane, enhanced with the capabilities of virtualization and orchestration, such as the SDN/OpenFlow control paradigm. Due to its centralized nature, SDN control plane, has a global view of the network, and hence all the decisions are taken globally by a single entity, the controller of the network. Historically, GMPLS/PCE control platform used to be exclusively applied onto optical network layer, and held responsible for the big data rapid switching and forwarding, which has later on, been evolved to an integration with SDN/OpenFlow platform, via proper functional and signaling extensions, that added to PCE protocol. Currently, SDN/OpenFlow is feasible to control a wide portfolio of optical elements, such as optical flexible transceivers supporting any kind of flexible modulation format (BPSK, QPSK 8QAM and 16QAM) and BVTs, circuit packet and burst switches of any type (e.g. WSS), optical amplifiers, and all types of multiplexing, such as WDM, TDM and SDM.
AI domain, and particularly DL and ML algorithms have provided a supportive, knowledge structured network orchestration mechanism that improves SDN orchestration and network function virtualization functions, thus managing more efficiently all these heterogeneous network entities, seen as VMs, and consequently achieving high performance service provisioning and flexible resource utilization. Critical parameters for control, of the lower physical layer are the QoT estimation, optical amplifiers control, optical power equalization, and OPM. As far as concerns network layer, critical control functions are the traffic prediction, VTD, topology reconfiguration, failure management, traffic flow classification, flexible resource utilization and path computation. Traffic flow classification would mean proper QoS, end to end provisioning and management, and discrete classification of network users. Flexible resource utilization is achieved in optical data plane under the umbrella of EON paradigm. Resource allocation in EON is performed according to SDN control layer decisions, that concern flexible resource utilization, via various multi-carrier modulation techniques (OFDM, Nyquist WDM), BVT types, advanced modulation formats (e.g., QPSK, QAM), multi coding rates, elastic access to sliced spectrum resources within flexible frequency grids and appropriate label swapping combinations.
Recently, hybrid 2-D and 3-D labeling techniques, and also SAC labeling with label stack have been proposed, associated with advanced coherent modulation formats, and multi constellation schemes for enhancing data rates and spectral efficiency. However, there is a trade off to be considered, concerning the employment of either IM-DD schemes, which are simply implemented, consume less power and cost less or the employment of coherent schemes, which require more complicated processing and hence, they are more expensive and consume more power, at the expense of ultra high communication and spectral efficiency, that nowadays DC architectures require. Transmission performance of a DC architecture, can be further enhanced by adopting advanced coding schemes such as space-time coding with the aforementioned 4 dimensional (4D) combined formats of PDM and quandrature coherent modulation schemes.
Finally, in accordance to SDN control paradigm, AOLS would also have to be complied to the elastic resource and flexible QoS provisioning. A proper labeling technique choice, should be capable to use the full wavelength bandwidth via grooming multicast labeling, or use sub wavelength granularity for unicast purposes, according to the traffic requests of running applications.
This survey investigates in great depth and detail the key roles of optical switching technology for improving big data traffic performance between DCs, defining at the same time, the design rules for implementing such switching layouts, based on a vast reference amount of research milestones throughout all this long time era. Multi angled, important issues are presented with some of them to be considered as new and promising research directions as well, such as the 4D combined formats of PDM and quandrature coherent modulation schemes equipped transponders, or the low cost and power consumption, graphene based integrated implementation solutions for board connectivity between DCs, or the design rules of an open unified control plane capable to control not only conventional electrical components but also a wide portfolio of optical elements, with elastic resource and flexible QoS provisioning, so as to give researchers motivation for extended investigation. A survey among integration methods and power consuming processes within and between DCs could be the scope of another interesting scientific research. Naturally, it's hard for a research group to focus equivalently and at the same time, on all these aforementioned subject domain directions. It is more reasonable for researchers to pick up peak technologies from each direction and to compose the closest possible to ideal layout solution. That is indeed the scope of this survey, to present analytically milestones and peak technologies of a wide variety of DC layout design issues and get researchers inspired to proceed with a total competitive implementation plan.
This paper is a survey which gives an updated and thorough investigation on optical switching and labeling potentials and their key roles on current DC and HPC architectures. Apart from it, other critical issues, strictly related to the proper choice of a switching architecture layout, are examined, such as energy, cost, implementation technology, manufacturing, packaging and integration issues, and elasticity potentials for flexible resource provisioning. Control plane definition rules for SDN/OpenFlow platform are set, for providing cognition and controlling and orchestrating optical network elements and functions at physical and network layer respectively. New competitive optical labeling techniques with certain criteria that determine the effectiveness of them, are also examined and discussed, in conjunction with advanced coherent multi level modulation formats and low level multi coded schemes, for ultrahigh link capacities and packet switching speeds of scalable, big data interconnected DCNs and HPC environments.
The author declares that there is no conflict of interest.
[1] | Khan N, Yaqoob I, Hashem IAT, et al. (2014) Big Data: Survey, Technologies, Opportunities, and Challenges. The Scientific World Journal 2014: 712826. |
[2] | Vahdat A, Al-Fares M, Farrington N, et al. (2010) Scale-Out Networking in the Data Center. IEEE Micro 30: 29–41. |
[3] |
Miao WW, Yan FF and Calabretta NN (2016) Towards Petabit/s All-Optical Flat Data Center Networks Based on WDM Optical Cross-Connect Switches with Flow Control. IEEE Journal of Lightwave Technology 34: 4066–4075. doi: 10.1109/JLT.2016.2593040
![]() |
[4] | Saridis GM, Aguado A, Yan Y, et al. (2018) LIGHTNESS: All-Optical SDN-enabled Intra-DCN with Optical Circuit and Packet Switching. Optical Switching in Next Generation Data Centers: 147–165. |
[5] | Lallas EN, Xenakis A, Stamoulis G, et al. (2018) QoS and MPLS design issues in NoCs. In: 2018 South-Eastern European Design Automation, Computer Engineering, Computer Networks and Society Media Conference (SEEDA-CECNSM ). |
[6] | Kitayama K, Huang YC, Yoshida Y, et al. (2014) Optical packet and path switching intra-data center network: Enabling technologies and network performance with intelligent flow control. The European Conference on Optical Communication, ECOC 2014, 21–25. |
[7] | Gerstel O, Jinno M, Lord A, et al. (2012) Elastic optical networking: A new dawn for the optical layer?" IEEE Commun Mag 50: 12–20. |
[8] |
Lara A, Kolasani A and Ramamurthy B (2014) Network innovation using OpenFlow: A survey. IEEE Commun Surv Tut 16: 493–512. doi: 10.1109/SURV.2013.081313.00105
![]() |
[9] |
Kreutz D, Ramos FMV, Verissimo PJE, et al. (2015) Software-defined networking: A comprehensive survey. P IEEE 103: 14–76. doi: 10.1109/JPROC.2014.2371999
![]() |
[10] | Qian D, Huang MF, lp E, et al. (2011) 101.7-Tb/s (370×294-Gb/s) PDM-128QAM-OFDM transmission over 3×55-km SSMF using pilot-based phase noise mitigation. In: 2011 Optical Fiber Communication Conference and Exposition and the National Fiber Optic Engineers Conference, 1–3. |
[11] | Singh A, Ong J, Agarwal A, et al. (2016) Jupiter rising: a decade of Clos topologies and centralized control in Google's datacenter network. Communications of the ACM 59: 88–97. |
[12] |
Zhou X, Liu H, and Urata R (2017) Datacenter optics: requirements, technologies, and trends (Invited Paper). Chin Opt Lett 15: 120008–120011. doi: 10.3788/COL201715.120008
![]() |
[13] |
Cheng Q, Bahadori M, Glick M, et al. (2018) Recent advances in optical technologies for data centers: a review. Optica 5: 1354–1370. doi: 10.1364/OPTICA.5.001354
![]() |
[14] | Kerravala Z (2015) A Data Center Fabric Is Critical to a Next-Generation Unified Data Center. Yankee Group White Paper. |
[15] |
Tucker RS (2011) Green optical communications-Part II: Energy limitations in networks. IEEE J Sel Top Quant 17: 261–274. doi: 10.1109/JSTQE.2010.2051217
![]() |
[16] |
Kitayama KI, Huang YC, Yoshida Y, et al. (2015) Torus-Topology Data Center Network Based on Optical Packet/Agile Circuit switching with Intelligent Flow Management. J Lightwave Technol 33: 1063–1071. doi: 10.1109/JLT.2015.2394384
![]() |
[17] |
Yin Y, Proietti R, Ye X, et al. (2013) LIONS: An AWGR-Based Low-Latency Optical Switch for High-Performance Computing and Data Centers. IEEE J Sel Top Quant 19: 3600409–3600409. doi: 10.1109/JSTQE.2012.2209174
![]() |
[18] | Imran M, Collier M, Landais P, et al. (2015) HOSA: Hybrid Optical Switch Architecture for Data Center Networks. In: Proceedings of the 12th ACM International Conference on Computing Frontiers, p. 27. |
[19] | Peng S, Simeonidou D, Zervas G, et al. (2014) A novel SDN enabled hybrid optical packet/circuit switched data centre network: The LIGHTNESS approach. In: 2014 European Conference on Networks and Communications (EuCNC), pp. 1–5. |
[20] | Xu M, Diakonikolas J, Modiano E, et al. (2019) A Hierarchical WDM-based Scalable Data Center Network Architecture. ArXiv:1901.06450. |
[21] | Kitayama K (2016) Optical Packet Switching: Myth, Fact, and Promise. In: 21st OptoElectronics and Communications Conference (OECC) held jointly with 2016 International Conference on Photonics in Switching (PS), pp. 1–2. |
[22] | Ye S, Shen Y, and Panwar SS(2010) HELIOS: A High Energy-efficiency Locally-scheduled Input-queued Optical Switch. In: Proceedings of the 6th ACM/IEEE Symposium on Architectures for Networking and Communications Systems (ANCS), IEEE. |
[23] | Balanici M and Pachnicke S (2018) Intra-Data Center Network Optimization by Means of Application-Based Traffic Modeling and Optical Circuit Switching. In: 20th International Conference on Transparent Optical Networks (ICTON), Bucharest, Romania. |
[24] | Lone S, Usman M, Imran M (2018) E-HOSA: Extended-Hybrid Optical Switch Architecture for Cloud Computing Data Centers. In: International Conference on Frontiers of Information Technology (FIT), pp. 53–58. |
[25] | Cisco Nexus 56128P Switch-Cisco. Available from: https://www.cisco.com/c/en/us/products/switches/nexus-56128p-switch/index.html. |
[26] | Ye X, Mejia P, Yin Y, et al. (2010) DOS: a scalable optical switch for datacenters. In: Proceedings of the 6th ACM/IEEE Symposium on Architectures for Networking and Communications Systems, ACM. |
[27] | Xi K, Kao YH and Chao HJ (2013) A petabit bufferless optical switch for data center networks. Optical Interconnects for Future Data Center Networks, pp. 135–154, Springer, New York, NY. |
[28] |
Xu M, Liu C and Subramaniam S (2018) PODCA: A Passive Optical Data Center Network Architecture. Journal of Optical Communications and Networking 10: 409–420. doi: 10.1364/JOCN.10.000409
![]() |
[29] | Lu Y, Gu H, Yu X, et al. (2018) NEST: Towards Extreme Scale Computing Systems. IEICE T Inf Syst E101–D: 2827–2830. |
[30] | Nakagawa M, Masumoto K, Onda H, et al. (2018) Photonic Sub-Lambda Transport: An Energy-Efficient and Reliable Solution for Metro Networks. In: 2018 International Conference on Optical Network Design and Modeling (ONDM), pp. 166–171. |
[31] | Wang J, Basu S, McArdle C, et al. (2015) Large-scale hybrid electronic/optical switching networks for datacenters and HPC systems. In: 2015 IEEE 4th International Conference on Cloud Networking (CloudNet), pp. 87–93. |
[32] | Shukla V and Jain A (2018) Design and analysis of high speed optical routers for next generation data centre network. Journal of Engineering Research 6: 122–137. |
[33] |
Romagnoli M, Sorianello V, Midrio M, et al. (2018) Graphene-based integrated photonics for next-generation datacom and telecom. Nature Reviews Materials 3: 392–414. doi: 10.1038/s41578-018-0040-9
![]() |
[34] | Oki E, Shiomoto K, Shimazaki D, et al. (2005) Dynamic multilayer routing schemes in GMPLS-based IP+ optical networks. IEEE Commun Mag 43: 108–114. |
[35] |
Viswanathan A, Feldman N, Wang Z, et al. (1998) Evolution of multiprotocol label switching. IEEE Commun Mag 36: 165–173. doi: 10.1109/35.668287
![]() |
[36] |
Munoz R, Casellas R, Martinez R, et al. (2014) PCE: What is it, how does it work and what are its limitations? J Lightwave Technol 32: 528–543. doi: 10.1109/JLT.2013.2276911
![]() |
[37] |
Kreutz D, Ramos FMV, Verissimo PJE, et al. (2015) Software-defined networking: A comprehensive survey. P IEEE 103: 14–76. doi: 10.1109/JPROC.2014.2371999
![]() |
[38] | Openflow1.4. [Online]. Available from: https://www.opennetworking.org/images/stories/downloads/sdn-resources/onf-specifications/op enflow/openflow-spec-v1.4.0.pdf. |
[39] |
Li Y and Kilper DC (2018) Optical Physical Layer SDN [Invited]. Journal of Optical Communications and Networking 10: A110–A121. doi: 10.1364/JOCN.10.00A110
![]() |
[40] |
Lallas EN (2019) A Survey on All Optical Label Swapping Techniques: Comparison and Trends. Opt Switch Netw 31: 22–38. doi: 10.1016/j.osn.2018.08.002
![]() |
[41] |
Cui L, Yu FR and Yan Q (2016) When big data meets software-defined networking: SDN for big data and big data for SDN. IEEE Network 30: 58–65. doi: 10.1109/MNET.2016.7389832
![]() |
[42] |
Casellas R, Martinez R, Vilalta R, et al. (2018) Control, Management, and Orchestration of Optical Networks: Evolution, Trends, and Challenges. J Lightwave Technol 36: 1390–1402. doi: 10.1109/JLT.2018.2793464
![]() |
[43] |
Thyagaturu AS, Mercian A, McGarry MP, et al. (2016) Software Defined Optical Networks (SDONs): A Comprehensive Survey. IEEE Commun Surv Tut 18: 2738–2786. doi: 10.1109/COMST.2016.2586999
![]() |
[44] | OpenROADM. [Online]. Available from: http://www.openroadm.org. |
[45] |
Zhao J, Subramaniam S and Brandt-Pearce M (2014) Intradomain and interdomain QoT-aware RWA for translucent optical networks. J Opt Commun Netw 6: 536–548. doi: 10.1364/JOCN.6.000536
![]() |
[46] | SDN Architecture, Issue 1.1, ONF TR-521, Open Networking Foundation 2016. |
[47] | Network functions virtualisation (NFV), Architectural framework, ETSI GS NFV 002 (V1.2.1), Dec. 2014. |
[48] | Mestres A, Rodriguez-Natal A, Carner J, et al. (2017) Knowledge-defined networking. ACM SIGCOMM Comp Com 47: 2–10. |
[49] | Lu W, Liang L, Kong B, et al. (2018) AI-Assisted Knowledge-Defined Network Orchestration for Energy-Efficient Datacenter Networks. IEEE Commun Mag. |
[50] |
Musumeci F, Rottondi C, Nag A, et al. (2019) An Overview on Application of Machine Learning Techniques in Optical Networks. IEEE Commun Surv Tut 21: 1383–1408. doi: 10.1109/COMST.2018.2880039
![]() |
[51] |
Yamanaka N, Okamoto S, Hirono M, et al. (2018) Application-Triggered Automatic Distributed Cloud/Network Resource Coordination by Optically Networked Inter/Intra Data Center [invited]. J Opt Commun Netw 10: B15–B24. doi: 10.1364/JOCN.10.000B15
![]() |
[52] | Huang MF (2017) Architecture of OpenFlow based Software Defined Optical Label Swapping. US PATENT No. 9.537.598. |
[53] |
Walkowiak K, Wozniak M, Klinkowski M, et al. (2015) Optical Networks for Cost-Efficient and Scalable Provisioning of Big Data Traffic. International Journal of Parallel, Emergent and Distributed Systems 30: 15–28. doi: 10.1080/17445760.2014.924516
![]() |
[54] | Wu Z, Li J, Zhu P, et al. (2015) Experimental Demonstration of Optical Labeled Superchannel Switching for Elastic Optical Network. Optical Fiber Communication Conference, Optical Society of America. |
[55] |
Dallaglio M, Giorgetti A, Sambo N, et al. (2015) Provisioning and Restoration With Sliceability in GMPLS-Based Elastic Optical Networks. J Opt Commun Netw 7: A309–A317. doi: 10.1364/JOCN.7.00A309
![]() |
[56] |
Yoshida Y, Maruta A, Kitayama K, et al. (2015) SDN-Based Network Orchestration of Variable-Capacity Optical Packet Switching Network Over Programmable Flexi-Grid Elastic Optical Path Network. J Lightwave Technol 33: 609–617. doi: 10.1109/JLT.2014.2351852
![]() |
[57] |
Yin Y, Liu L, Proietti R, et al. (2017) Software Defined Elastic Optical Networks for Cloud Computing. IEEE Network 31: 4–10. doi: 10.1109/MNET.2017.8120233
![]() |
[58] |
Kanj M, Rouzic EL, Meuric J, et al. (2016) Optical power control in GMPLS control plane. IEEE/OSA Journal of Optical Communications and Networking 8: 553–568. doi: 10.1364/JOCN.8.000553
![]() |
[59] |
Kanj M, Rouzic EL, Meuric J, et al. (2018) Optical power control in translucent flexible optical networks with GMPLS control plane. IEEE/OSA Journal of Optical Communications and Networking 10: 760–772. doi: 10.1364/JOCN.10.000760
![]() |
[60] |
Blumenthal DJ, Bowers JE, Rau L, et al. (2003) Optical signal processing for optical packet switching networks. IEEE Commun Mag 41: S23–S29. doi: 10.1109/MCOM.2003.1179494
![]() |
[61] |
Guillemot C, Renaud M, Gambini P, et al. (1998) Transparent optical packet switching: The European ACTS KEOPS project approach. J Lightwave Technol 16: 2117–2134. doi: 10.1109/50.736580
![]() |
[62] | Okada A (2002) All-optical packet routing in AWG-based wavelength routing networks using an out-of-band optical label. In: Optical Fiber Communication Conference and Exhibit, pp. 213–215. |
[63] |
Skoufis C, Sygletos S, Leligou N, et al. (2003) Data-Centric Networking Using Multiwavelength Headers/Labels in Packet-Over-WDM Networks:A Comparative Study. J Lightwave Technol 21: 2110–2122. doi: 10.1109/JLT.2003.816897
![]() |
[64] |
Zhu Z, Hernandez VJ, Jeon MY, et al. (2003) RF Photonics Signal Processing in Subcarrier Multiplexed Optical-Label Switching Communication Systems. J Lightwave Technol 21: 3155–3166. doi: 10.1109/JLT.2003.822237
![]() |
[65] |
Lallas EN, Skarmoutsos N and Syvridis D (2005) Coherent encoding of optical FSK header for All optical Label Swapping Systems. J Lightwave Technol 23: 1199–1209. doi: 10.1109/JLT.2005.843490
![]() |
[66] |
Chow CW, Wong CS and Tsang HK (2004) All-Optical ASK/DPSK Label-Swapping and Buffering Using Fabry–Perot Laser Diodes. IEEE J Sel Top Quant 10: 363–370. doi: 10.1109/JSTQE.2004.825955
![]() |
[67] | Fouli K and Maier M (2007) OCDMA and optical coding: Principles, applications, and challenges. IEEE Commun Mag 45: 27–34. |
[68] |
Fakih A, Panbude S and Jagtap S (2014) Performance Analysis of Two Dimensional Wavelength/Time Encoding System for Optical CDMA Networks. International Journal of Computer and Communication Engineering 3: 424–428. doi: 10.7763/IJCCE.2014.V3.362
![]() |
[69] |
Matsumoto R, Kodama T, Morita K, et al. (2015) Scalable two- and three-dimensional optical labels generated by 128-port encoder/decoder for optical packet switching. Opt Express 23: 25747–25761. doi: 10.1364/OE.23.025747
![]() |
[70] |
Habib C, Baby V, Chen LR, et al. (2008) All-Optical Swapping of Spectral Amplitude Code Labels Using Nonlinear Media and Semiconductor Fiber Ring Lasers. IEEE J Sel Top Quant 14: 879–888. doi: 10.1109/JSTQE.2008.918047
![]() |
[71] |
Aboagye IA, Chen F and Cao Y (2017) Performance Analysis of 112 Gb/s×4-Channel WDM PDM-DQPSK Optical Label Switching System With Spectral Amplitude Code Labels. Photonic Sens 7: 88–96. doi: 10.1007/s13320-016-0345-5
![]() |
[72] |
Seddighian P, Ayotte S, Rosas-Fernández JB, et al. (2007) Label Stacking in Photonic Packet-Switched Networks With Spectral Amplitude Code Labels. J Lightwave Technol 25: 463–471. doi: 10.1109/JLT.2006.888265
![]() |
[73] |
Mendinueta JMD, Shinada S, Furukawa H, et al. (2017) Ultra-High-Capacity Optical Packet Switching Networks with Coherent Polarization Division Multiplexing QPSK/16QAM Modulation Formats. Photonics 4: 27. doi: 10.3390/photonics4020027
![]() |
[74] | Eiselt M, Dochhan A, Elbers JP (2018) Data Center Interconnects at 400G and Beyond. ArXiv:1807.11861. |
[75] | Moura PM, Scaraficci RA and da Fonseca NLS (2015) Algorithm for energy efficient routing, modulation and spectrum assignment. In: 2015 IEEE International Conference on Communications (ICC), pp. 5961–5966. |
[76] |
Xu Y, Li X and Yu J (2016) Simple scheme for PDM-QPSK payload generation in an optical label switching network. J Opt Commun Netw 8: 53–57. doi: 10.1364/JOCN.8.000053
![]() |
[77] |
Okonkwo CM, van Uden RGH, Chen H, et al. (2015) Advanced coding techniques for few mode transmission systems. Opt Express 23: 1411–1420. doi: 10.1364/OE.23.001411
![]() |
[78] |
Tarokh V, Jafarkhani H and Calderbank AR (1999) Space-Time Block Codes from Orthogonal Designs. IEEE T Inform Theory 45: 1456–1467. doi: 10.1109/18.771146
![]() |
[79] |
Alvarado A and Agrell E (2015) Four-Dimensional Coded Modulation with Bit-Wise Decoders for Future Optical Communications. J Lightwave Technol 33: 1993–2003. doi: 10.1109/JLT.2015.2396118
![]() |
[80] | Isaac AA, Chen F, Cao Y, et al. (2017) Effects of Polarization Tracker on 80 and 112Gb/s PDM-DQPSK with Spectral Amplitude Code Labels. Proceeding of the World Congress on Engineering 1. |
[81] | Nakagawa G, Feuer MD, Mikhailov V, et al. (2015) High-speed Polarization Shift Keying Lightpath Labeling of 100 Gb/s DP-QPSK for Programmable Photonic Networks. Optical Fiber Communication Conference, pp. Tu3D-2. Optical Society of America. |
[82] |
Xu M, Li Y, Kang TZ, et al. (2016) Performance evaluations of hybrid modulation with different optical labels over PDQ in high bit-rate OLS network systems. Opt Express 24: 26228–26240. doi: 10.1364/OE.24.026228
![]() |
[83] | Khlifi Y and Alotaibi M (2018) A novel multicast grooming scheme for dynamic QoS provision over OLS networks. IEEE 8th Annual Computing and Communication Workshop and Conference (CCWC), pp. 911–917. |
1. | Konstantinos G. Tsiknas, Paraskevas I. Aidinidis, Kyriakos E. Zoiros, Performance evaluation of transport protocols in cloud data center networks, 2021, 42, 1387-974X, 105, 10.1007/s11107-021-00946-8 | |
2. | Simranjit Singh, Amit Wason, Performance Evaluation of Bandwidth Allocating Algorithms in Generalized Multi Protocol Label Switched Optical Networks to Enhance Quality of Service, 2022, 10, 2169-3536, 40731, 10.1109/ACCESS.2022.3166912 | |
3. | Dariush Jafari, Mohammad Danaie, Pejman Rezaei, Tofiq Nurmohammadi, A novel variable-length header extraction scheme based on ring laser for all-optical packet switching network, 2021, 53, 0306-8919, 10.1007/s11082-021-02973-1 | |
4. | Amer Kotb, Kyriakos E. Zoiros, Eng Hwa Yap, Numerical simulation of all-optical header processor using carrier reservoir semiconductor optical amplifiers, 2024, 0972-8821, 10.1007/s12596-023-01613-z |