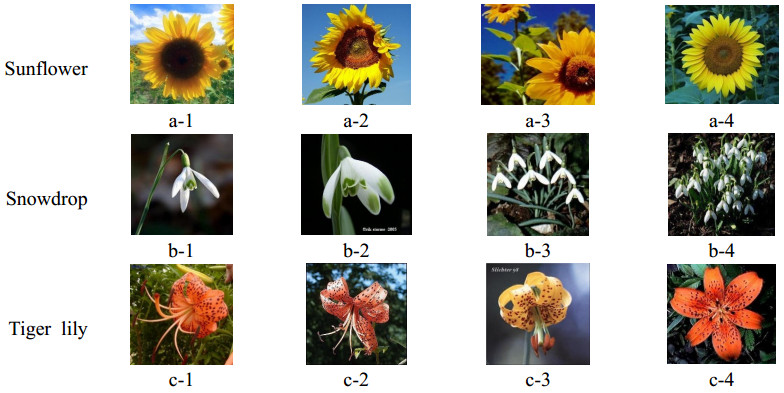
The emergence of the Internet of Things (IoT) has brought a revolution in global communication network technology. It has acquired many day-to-day applications in healthcare, education, agriculture, etc. In addition, IoT has also had a significant impact in the field of environmental monitoring.The significant factors in a healthy environment are air quality, water pollution, and waste management, where the world's population can live securely. Monitoring is necessary for us to achieve global sustainability. As monitoring technology has advanced in recent years, environmental monitoring systems have evolved from essential remote monitoring to an advanced environment monitoring (AEM) system, incorporating Internet of Things (IoT) technology and sophisticated sensor modules.The present manuscript aims to accomplish a critical review of noteworthy contributions and research studies about environmental monitoring systems, which involve monitoring air quality, water quality, and waste management.The rapid growth of the world's population and the exhaustion of natural resources, coupled with the increasing unpredictability of environmental conditions, lead to significant concerns about worldwide food security, global warming, water pollution, and waste overflowing. Automating tasks in the building environment, based on the Internet of Things (IoT) application, is meant to eliminate problems with the traditional approach. This study aims to examine and evaluate numerous studies involving monitoring air, water, waste, and overall environmental pollution, as well as their effect on the environment. This article categorizes studies based on their research purposes, techniques, and findings. This paper examines advanced environmental monitoring systems through sensor technology, IoT, and machine learning.
Citation: Suprava Ranjan Laha, Binod Kumar Pattanayak, Saumendra Pattnaik. Advancement of Environmental Monitoring System Using IoT and Sensor: A Comprehensive Analysis[J]. AIMS Environmental Science, 2022, 9(6): 771-800. doi: 10.3934/environsci.2022044
[1] | Zhigao Zeng, Cheng Huang, Wenqiu Zhu, Zhiqiang Wen, Xinpan Yuan . Flower image classification based on an improved lightweight neural network with multi-scale feature fusion and attention mechanism. Mathematical Biosciences and Engineering, 2023, 20(8): 13900-13920. doi: 10.3934/mbe.2023619 |
[2] | Huiying Zhang, Jiayan Lin, Lan Zhou, Jiahui Shen, Wenshun Sheng . Facial age recognition based on deep manifold learning. Mathematical Biosciences and Engineering, 2024, 21(3): 4485-4500. doi: 10.3934/mbe.2024198 |
[3] | Xiao Ma, Xuemei Luo . Finger vein recognition method based on ant colony optimization and improved EfficientNetV2. Mathematical Biosciences and Engineering, 2023, 20(6): 11081-11100. doi: 10.3934/mbe.2023490 |
[4] | Sakorn Mekruksavanich, Anuchit Jitpattanakul . RNN-based deep learning for physical activity recognition using smartwatch sensors: A case study of simple and complex activity recognition. Mathematical Biosciences and Engineering, 2022, 19(6): 5671-5698. doi: 10.3934/mbe.2022265 |
[5] | Yuanyao Lu, Kexin Li . Research on lip recognition algorithm based on MobileNet + attention-GRU. Mathematical Biosciences and Engineering, 2022, 19(12): 13526-13540. doi: 10.3934/mbe.2022631 |
[6] | Sakorn Mekruksavanich, Wikanda Phaphan, Anuchit Jitpattanakul . Epileptic seizure detection in EEG signals via an enhanced hybrid CNN with an integrated attention mechanism. Mathematical Biosciences and Engineering, 2025, 22(1): 73-105. doi: 10.3934/mbe.2025004 |
[7] | Long Wen, Liang Gao, Yan Dong, Zheng Zhu . A negative correlation ensemble transfer learning method for fault diagnosis based on convolutional neural network. Mathematical Biosciences and Engineering, 2019, 16(5): 3311-3330. doi: 10.3934/mbe.2019165 |
[8] | Cong Lin, Yiquan Huang, Wenling Wang, Siling Feng, Mengxing Huang . Lesion detection of chest X-Ray based on scalable attention residual CNN. Mathematical Biosciences and Engineering, 2023, 20(2): 1730-1749. doi: 10.3934/mbe.2023079 |
[9] | Junting Lin, Shan Li, Ning Qin, Shuxin Ding . Entity recognition of railway signal equipment fault information based on RoBERTa-wwm and deep learning integration. Mathematical Biosciences and Engineering, 2024, 21(1): 1228-1248. doi: 10.3934/mbe.2024052 |
[10] | Jia-Gang Qiu, Yi Li, Hao-Qi Liu, Shuang Lin, Lei Pang, Gang Sun, Ying-Zhe Song . Research on motion recognition based on multi-dimensional sensing data and deep learning algorithms. Mathematical Biosciences and Engineering, 2023, 20(8): 14578-14595. doi: 10.3934/mbe.2023652 |
The emergence of the Internet of Things (IoT) has brought a revolution in global communication network technology. It has acquired many day-to-day applications in healthcare, education, agriculture, etc. In addition, IoT has also had a significant impact in the field of environmental monitoring.The significant factors in a healthy environment are air quality, water pollution, and waste management, where the world's population can live securely. Monitoring is necessary for us to achieve global sustainability. As monitoring technology has advanced in recent years, environmental monitoring systems have evolved from essential remote monitoring to an advanced environment monitoring (AEM) system, incorporating Internet of Things (IoT) technology and sophisticated sensor modules.The present manuscript aims to accomplish a critical review of noteworthy contributions and research studies about environmental monitoring systems, which involve monitoring air quality, water quality, and waste management.The rapid growth of the world's population and the exhaustion of natural resources, coupled with the increasing unpredictability of environmental conditions, lead to significant concerns about worldwide food security, global warming, water pollution, and waste overflowing. Automating tasks in the building environment, based on the Internet of Things (IoT) application, is meant to eliminate problems with the traditional approach. This study aims to examine and evaluate numerous studies involving monitoring air, water, waste, and overall environmental pollution, as well as their effect on the environment. This article categorizes studies based on their research purposes, techniques, and findings. This paper examines advanced environmental monitoring systems through sensor technology, IoT, and machine learning.
The main purpose of flower recognition is to make judgments of flower category though some flower attributes, such as color, texture and semantics, which plays an important role in the fields of forestry informatization and plant medicine [1]. Different from classical image recognition [2,3,4], flower category recognition is a typical fine-grained image recognition task, which requires model have strong inter-class and intra class discrimination capabilities, and also is a popular research topic in the fields of computer vision, pattern recognition and forestry informatization.
In recent years, deep learning [5] has achieved great success in computer vision, multimedia signal processing and natural language processing [6]. Although there are many classification methods in the literature [7,8,9], Deep Convolutional network (DCCN), as the most outstanding representative of deep learning, has been widely used in image classification, scene recognition, semantic information extraction, and still maintains the current best results [10,11]. In the view of this, some researchers have applied DCNN to the problem of flower category recognition and achieved good performance [12,13]. Although the methods based on DCNN can improve the accuracy and speed of flower category recognition, it still has 3 main problems: 1) The number of training samples is insufficient. DCNN always contains a lot of parameters, and training deep models in a small dataset is much challenging due to the over-fitting problem. Unfortunately, there have no public dataset with sufficient types and quantities at the same time in flower category recognition task, which directly limits the performance of the model. Even if the problem can be mitigated by data augmentation or fine-tuning on ImageNet, the useful information contained in the dataset is not increased. 2) Low recognition accuracy. Flower category recognition is a fine-grained image recognition task and has the characteristics such as high similarity between heterogeneous flowers. In addition, due to the complexity and variability of the natural environment, the pose and view angle of flowers may change unpredictably, which makes model difficult to train and the performance poor. 3) The background of image is complicated. Flower images collected from nature always have complex backgrounds and contain many noise, which may limit the recognition performance of deep learning models.
To enable deep learning quickly focus on the key points of the input data, self-attention mechanism-based model has been developed and successfully applied to many tasks, such as natural language processing and human-machine dialogue [14]. Human can quickly scan the visual information and obtain the attention target area. Not only that, but people pay more attention to the target area to get more detailed information and suppress other useless things, which is a survival mechanism formed by humans over a long period of evolution. Therefore, we proposed a novel flower recognition method based on attention mechanism (Visual Attentional-driven DCNNs, VA-DCNNs), which can effectively identify flower species accurately. The model is mainly divided into four-fold. Firstly, due to deep learning method always need massive training data to guarantee the performance, we adopt data augmentation techniques to increase samples. We rotate the picture in a clockwise angle and clip along the middle, which will be fuse with original samples as the training set for the experiments. Secondly, a Visual Attentional Learning (VAL) block are constructed for the vanilla DCNNs (we use ResNet14 and ResNet50 as the baseline in this paper), which makes VA-DCNNs have strong discriminative learning ability. Thirdly, the layer weights of the model are obtained by using datasettraining. And finally, we get the recognition accuracy of the model on testing set. The experimental on the Flowers 17 public dataset prove the effectiveness of VA-DCNNs, which can achieve an accuracy of up to 85.7%. Compared with other recognition methods, VA-DCNNs can achieve better results, and has strong practicability and generalization.
The experimental dataset is the Flowers 17 [15], which contains 17 common flowers in the UK. The flowers including sunflowers, hyacinths, daffodils and chrysanthemums, etc., and each category have 80 images with different pose, size and perspective. Figure 1 have shown some examples in Flowers 17 dataset. So far the dataset has been widely used in flower recognition and organ segmentation, which is one of the most representative dataset in this field.
Deep convolutional neural network always composed by multiple blocks, and each block contains several convolutional layers, batch normalization layers, activation layers, and pooling layers. The data flows and gradient transfer between blocks through convolution kernels and back propagation algorithm. A DCNNs model always contains a large number of parameters need to be trained, which can make DCNNs fitting the data well. Although sufficient training samples can make the model fine-training, augmenting the training set to increase the number of training samples is one of the most common techniques used in deep learning models to further enhance the generality and robustness of the model [16]. In this paper, we augment the original flower image by using rotating and forward cropping. Specifically, for each original 224 × 224 pixel flower image, we rotate it clockwise, perform forward cropping every 30° and save it as 224 × 224 pixel size. The rotate operation totally perform 4 times (30°, 60°, 90°, 120°), and obtain a new dataset with five times than original set in quantity. The new dataset is divided into 70% training set, 20% validation set and 10% testing set, randomly. Since this data augmentation technique has been widely used in several papers, we don't repeat it here, but more details can be found in [11].
The traditional DCNNs module extracts features by using stacking convolutional, dropout, batch normalization and activation layers (as shown in Figure 2(a)). Although the effectiveness of this structure has been verified in many DCNNs models, single stack the block easily causes "gradient explosion" or "gradient disappearance" during training when network depth further increase. Deep layer blocks cannot take the input information or gradient is lost in the back-propagation process, resulting in the model cannot be trained [17]. Therefore, deep residual network (as shown in Figure 2(b)) has been proposed in [18] to improve the trainability of the DCNNs. This model adopts residual connection to connect different layer, which can ignore some unimportant blocks in training automatically. This technique can solve some problems in traditional DCNNs. In order to make the DCNNs quickly locate the focal area of the image, inspired by the human visual mechanism, we propose a Visual Attentional Learning (VAL) block (shown in Figure 2(c)) based on the attentional mechanism. Specifically, we obtain the weight of channel and spatial position of the convolution feature by performing batch normalization on the feature map. This process can be expressed as:
w=∅(O) | (1) |
where O∈RH×W×C means C-dimensional H×W feature map. In this paper, we adopt the features from last convolutional layer in each stage. The height of each feature is H and width is W; ∅(⋅)means batch normalization function. w is learned feature weight. Batch normalization function ∅(⋅) can be defined as:
∅S(O)={m|mci,j=eOci,j∑i′,j′eOci,j} | (2) |
∅C(O)={m|mci,j=eOci,j∑c′eOc′i,j} | (3) |
∅M(O)={m|mci,j=σ(Cci,j)} | (4) |
where (i,j) means the location in the feature map O; c is the channel index of feature map; ∅S(O) is the attentional-driven block on spatial, which is used to learn feature weight on the spatial position; σ(⋅) is sigmoid function. ∅C(O) is the attentional-driven block on channel, which is used to learn feature weight of different channel dimension; ∅M(O) is the attention learning block that combines ∅S(O) and ∅C(O), which considers both spatial location information and channel information. In order to retain the advantages of the residual technique, we add the output of the attention strategy and the residual strategy as the final output of the block after weighting the convolution features. The process can be written as:
outs=∅S(O)∗O+O+F(O) | (5) |
outc=∅C(O)∗O+O+F(O) | (6) |
outm=∅M(O)∗O+O+F(O) | (7) |
where F(⋅) denotes residual connection. Based on the block defined above, the model not only can actively skip some unimportant features in the training process, but also can quickly locate some important channels and spatial positions by using attention mechanism. Therefore, the model can effectively alleviate the problems of insufficient training samples and small differences between samples of the same type in the flower Recognition task
We can structure any depth DCNNs models based on Attentional-driven residual block, but consider the local hardware and the scale of the dataset, we adopt ResNet14 and ResNet50 as the basic frameworks to construct Attentional-driven residual based version. The network structure is shown in Table 1. In this paper, we propose two novel methods, named VA-ResNet14 and VA-ResNet50, respectively. The input of two different depth model are both 224×224×3 color jpg images, and then connected to the first deep learning block (convolution layer 1+), which consists by a 7 × 7 convolutional layer, a batch normalization layer, an activation layer and a maximum pooling layer. Then, we add the Attentional-driven residual block in the last layer of second (convolutional layer 2+), third (convolutional layer 3+), fourth (convolutional layer 4+) and fifth (convolutional layer 5+) stage. Not only that, but we retain the residual connection structure in the model (as shown in Figure 2 (c)). Finally, the model realizes the flower classification task though global average pooling and fully connected layer. The improved model is structurally identical to the original residual network. Since attentional-driven block has few parameters, the improved network will not increase the training burden. In addition, attentional-driven learning with residual connection can prove the performance of the model will not roll back. Even in the worst case, the residual connection can jump over the attentional learning block to make it down.
Layer | output size | ResNet14 | VA-ResNet14 | ResNet50 | VA-ResNet50 |
Conv 1+ | 112×112×64 | Conv, 7×7, stride 2 | Conv, 7×7, stride 2 | Conv, 7×7, stride 2 | Conv, 7×7, stride 2 |
Conv 2+ | 56×56×256 | Pool, 3×3, stride 2 | Pool, 3×3, stride 2 | Pool, 3×3, stride 2 | Pool, 3×3, stride 2 |
Res block | Attentional Res block | [Res block] × 3 | [Attentional Res block]×3 | ||
Conv 3+ | 28×28×512 | [Resblockstride2]×2 | [Resblockstride2Attentionconnect]×2 | [Resblockstride2]×4 | [Resblockstride2Attentionconnect]×4 |
Conv 4+ | 14×14×1024 | [Resblockstride2]×2 | [Resblockstride2Attentionconnect]×2 | [Resblockstride2]×6 | [Resblockstride2Attentionconnect]×6 |
Conv 5+ | 7×7×2048 | [Resblockstride2]×2 | [Resblockstride2Attentionconnect]×2 | [Resblockstride2]×3 | [Resblockstride2Attentionconnect]×3 |
Pool | 1×1×2048 | Global average pool | |||
Output | 17 | FC |
Based on the Attentional-driven residual network proposed above, we use Flowers 17 dataset to evaluate its performance. By randomly dividing the augmented dataset according to proportion, we have 4760 images in training set with 280 images per class; 1360 images in validation set with 80 images per class and 680 images in testing set with 40 images per class. All of the flower images are two-dimensional color image in JPG format. The data for input need normalized by subtracting the mean value. The training process adopts Stochastic Gradient Descent (SGD) algorithm [15] to optimize the hinge loss function. The batch size is set to 128. The learning rate starts with 0.01, decreases to its 1/10 every 10,000 iterations, stops at 50,000 iterations. The weight decay parameter is 0.0005.
The experiment environment is Pytorch based on Python programming language. Pytorch as one of the most widely used framework in deep learning, has good scalability, modularity and high efficiency, which is very popular in the academic and industrial circles [19]. We implement all the algorithms in Think Station P320 workstation with 4 GTX 1080 Ti GPU to speed up image processing [20].
Based on experiment setting proposed above, we training the AL-ResNet14 and AL-ResNet50, respectively. Figure 3 shows the curve of accuracy and objective loss in validation set, where the blue curve indicates the result in VA-ResNet14 and the green curve indicates the result in VA-ResNet50. We can find that the curve become placid after about 40,000 iterations, indicating that the algorithm has been converged. In addition, the accuracy on VA-ResNet50 is high that VA-ResNet14, but the objective loss is small, means VA-ResNet50 can fitting the data better. Besides, VA-ResNet14 is volatility higher that VA-ResNet50 in Figure 3, which is due to the number of VA-ResNet14 is less. In the case of same input data, the model with less parameters are hard to find the local optimal solution [21].
To show the performance of Attentional-driven residual block, we provide the focus areas of flower images obtained by the attention learning in the first layer. As shown in the Figure 4, the brightly area is the model to focus on. We can see some interesting points as follow: 1) the focus area of attention is not continuous, but scattered into several bright spots.
The brighter the area, the greater the role it plays in the classification, and the higher wright it corresponding to. It indicates that not all part of flowers plays an important role in flower recognition task. 2) Compare to original input image, the focus areas of flower are always corresponding to more colorful part in flower, indicating that the color information is the key point to discriminate the flower. Besides, since the attention mechanism puts more effort on flower, the noise in background has no effect on flower recognition task, which bring robustness to model. Further, we visualize the convolution features from first to third layers of some flowers. Figure 5 shows the features visualization results of sunflower, snowdrop and tiger lily, respectively. From Figure 5 we can see the following conclusions. Firstly, the convolutional features learned by the shallow network are mainly understandable features such as texture and color, while the features learned by deep layers are more abstract, like outline or shape. 3) The feature from shallow layers are often high-resolution information, while the deep layers are more likely to extract some semantic information. Therefore, the resolution of images is gradually decreasing with the layer deep. In the classification process, the semantic information determines the image "what is", while the shallow features determine the discriminative information "where as" in the image.
In order to further verify the effectiveness of the methods proposed in this paper, we compare our methods to some popular image classification techniques. We ensure all the parameters are consistent with the original text to guarantee the algorithms optimization. The results on the testing set are shown in Table 2.
Method | VGGNet(16) | NIN | GoogLeNet | Inception V3 | ResNet14 | ResNet50 | VA-ResNet14 | VA-ResNet50 |
Accuacy | 63.1% | 64.2% | 65.8% | 66.9% | 67.7% | 81.3% | 69.4% | 85.7% |
Comparing our method with VGGNet [22], Network In Network [23], GoogLeNet [24], and Inception V3 [25], we can find that the method proposed in this paper has higher accuracy. Specifically, VA-ResNet14 and VA-ResNet50 have accuracy improvement of 1.7 and 3.6% than ResNet14 [18] and ResNet50 [18], respectively. This indicates that the proposed method has good universality. VA can still improve the model performance, even on the DCNN model with a strong presentation ability. Also, it can be found that ResNet with VA blocks shares very higher accuracy as compared to VGGNet [22], Network In Network [23], GoogLeNet [24], and Inception V3 [25].
In this paper, we propose a novel Attentional-driven residual network model for flower recognition. By adding an attention connection to each residual block, the model can learn from different channel features and different spatial dimensions, and at the same time, can maintain the capability of few-shot learning to compensate training samples insufficient. In order to verify the feasibility and effectiveness of the methods proposed in this paper, we take the experiments on Flowers 17 dataset. The experiments show that our method can achieve the accuracy of 85.7%, which is higher than the existing image classification methods without introducing additional training parameters. Although the methods proposed in this paper is initially designed for flower recognition, it has strong scalability and practicability that can be easily applied to other object recognition tasks, such as terrain recognition, farmland recognition, and forest recognition on remote sensing images.
In addition, our future work will focus on the following aspects. 1) Expand the flower database. Not only we expand the number of flower varieties, but also expand the number of images in each species. 2) Since our methods are supervised learning model, which need using a lot of labeled data in training process, one of our future projects is to combine with some advanced technique, like semi-supervised learning, one/few-shot learning. 3) Another project will focus on the transfer learning and data generation technique based on natural image datasets to improve the generalization ability and robustness of the model.
This work was supported by Startup Foundation for Introducing Talent of Nanjing University of Information Science and Technology (Grant No.2019r030).
The authors declared that they have no conflicts of interest to this work. We declare that we do not have any commercial or associative interest that represents a conflict of interest in connection with the work submitted
[1] | Rath M, Pattanayak B (2018) Technological improvement in modern health care applications using Internet of Things (IoT) and proposal of novel health care approach. International Journal of Human Rights in Healthcare. https://doi.org/10.1108/IJHRH-01-2018-0007 |
[2] |
Ramlowat DD, Pattanayak BK (2019) Exploring the Internet of things (IoT) in education: a review. Information systems design and intelligent applications 2019: 245–255. https://doi.org/10.1007/978-981-13-3338-5_23 doi: 10.1007/978-981-13-3338-5_23
![]() |
[3] | BiswalAK, Singh D, Pattanayak B K, et al. (2021) IoT-based smart alert system for drowsy driver detection. Wireless communications and mobile computing 2021. https://doi.org/10.1155/2021/6627217 |
[4] |
Rath M, Swain J, Pati B, et al. (2018) Network security: attacks and control in MANET. InHandbook of Research on Network Forensics and Analysis Techniques 2018: 19–37. https://doi.org/10.4018/978-1-5225-4100-4.ch002 doi: 10.4018/978-1-5225-4100-4.ch002
![]() |
[5] |
Rath M, Pati B, Pattanayak BK, et al. (2018) Relevance of soft computing techniques in the significant management of wireless sensor networks. In Soft Computing in Wireless Sensor Networks 2018: 75–94. https://doi.org/10.1201/9780429438639-4 doi: 10.1201/9780429438639-4
![]() |
[6] |
Hosenkhan MR, Pattanayak BK (2020) Security issues in Internet of things (IoT): a comprehensive review. New Paradigm in Decision Science and Management 2020: 359–369. https://doi.org/10.1007/978-981-13-9330-3_36 doi: 10.1007/978-981-13-9330-3_36
![]() |
[7] |
Biswal AK, Singh D, Pattanayak BK (2021) IoT-based voice-controlled energy-efficient intelligent traffic and street light monitoring system. In Green Technology for Smart City and Society 2021: 43–54. https://doi.org/10.1007/978-981-15-8218-9_4 doi: 10.1007/978-981-15-8218-9_4
![]() |
[8] | Biswal AK, Singh D, Pattanayak BK, et al. (2021) Adaptive fault-tolerant system and optimal power allocation for smart vehicles in smart cities using controller area network. Security and Communication Networks 2021. https://doi.org/10.1155/2021/2147958 |
[9] |
Hosenkhan R, Pattanayak BK (2019) A secured communication model for IoT. In Information Systems Design and Intelligent Applications 2019: 187–193. https://doi.org/10.1007/978-981-13-3338-5_18 doi: 10.1007/978-981-13-3338-5_18
![]() |
[10] |
Swain J, Pattanayak BK, Pati B (2021) A systematic study and analysis of security issues in mobile ad-hoc networks. In Research anthology on securing mobile technologies and applications 2021: 144–150. https://doi.org/10.4018/978-1-7998-8545-0.ch008 doi: 10.4018/978-1-7998-8545-0.ch008
![]() |
[11] |
Rath M, Darwish A, Pati B, et al. (2020) Swarm intelligence as a solution for technological problems associated with the Internet of Things. In Swarm Intelligence for Resource Management in Internet of Things 2020: 21–45. https://doi.org/10.1016/B978-0-12-818287-1.00005-X doi: 10.1016/B978-0-12-818287-1.00005-X
![]() |
[12] |
Rath M, Pati B, Pattanayak BK (2020) Manifold surveillance issues in wireless network and the secured protocol. International Journal of Information Security and Privacy (IJISP) 14: 16–26. https://doi.org/10.4018/IJISP.2020010102 doi: 10.4018/IJISP.2020010102
![]() |
[13] | Pattanayak BK, Amic S (2020) Modified lightweight aes based two-level security model for communication on IoT. TEST Eng Manage 82: 2323–2330. |
[14] |
Pattanayak BK, Nohur D, Cowlessur SK, et al. (2021) An IoT-Based System Architecture for Environmental Monitoring. In Progress in Advanced Computing and Intelligent Engineering 2021: 507–514. https://doi.org/10.1007/978-981-33-4299-6_42 doi: 10.1007/978-981-33-4299-6_42
![]() |
[15] |
Laha SR, Mahapatra SK, Pattnaik S, et al. (2021) U-INS: an android-based navigation system. In Cognitive Informatics and Soft Computing 2021: 125–132. https://doi.org/10.1007/978-981-16-1056-1_11 doi: 10.1007/978-981-16-1056-1_11
![]() |
[16] |
Pattnaik S, Banerjee S, Laha SR, et al. (2022) A Novel Intelligent Street Light Control System Using IoT. In Intelligent and Cloud Computing 2022: 145–156. https://doi.org/10.1007/978-981-16-9873-6_13 doi: 10.1007/978-981-16-9873-6_13
![]() |
[17] | Mendez-Monroy PE, Cruz May E, Jiménez Torres M, et al. (2022) IoT System for the Continuous Electrical and Environmental Monitoring into Mexican Social Housing Evaluated under Tropical Climate Conditions. Journal of Sensors 2022. https://doi.org/10.1155/2022/5508713 |
[18] |
Sharma R, Arya R (2022) UAV based long-range environment monitoring system with Industry 5.0 perspectives for smart city infrastructure. Computers & Industrial Engineering 168: 108066. https://doi.org/10.1016/j.cie.2022.108066 doi: 10.1016/j.cie.2022.108066
![]() |
[19] | Xu J, Gu B, Tian G (2022) Review of agricultural IoT technology. Artificial Intelligence in Agriculture. https://doi.org/10.1016/j.aiia.2022.01.001 |
[20] | Canning J, Guo Y, Chazcko Z (2022) Towards a bionic IoT: environmental monitoring using smartphone interrogated plant sensors. bioRxiv. |
[21] |
Osman N, Jamlos MF, Dzaharudin F, et al. (2022). Real-time and predictive analytics of air quality with iot system: A review. Recent Trends in Mechatronics Towards Industry 4.0 2022: 107–116. https://doi.org/10.1007/978-981-33-4597-3_11 doi: 10.1007/978-981-33-4597-3_11
![]() |
[22] |
Kho EP, Chua SND, Lim SF, et al. (2022) Development of young sago palm environmental monitoring system with wireless sensor networks. Computers and Electronics in Agriculture 193: 106723. https://doi.org/10.1016/j.compag.2022.106723 doi: 10.1016/j.compag.2022.106723
![]() |
[23] |
Islam MM, Kashem MA, Uddin J (2022) An internet of things framework for real-time aquatic environment monitoring using an Arduino and sensors. International Journal of Electrical and Computer Engineering 12: 826. https://doi.org/10.11591/ijece.v12i1.pp826-833 doi: 10.11591/ijece.v12i1.pp826-833
![]() |
[24] |
Quy VK, Hau NV, Anh DV, et al. (2022) IoT-Enabled Smart Agriculture: Architecture, Applications, and Challenges. Applied Sciences 12: 3396. https://doi.org/10.3390/app12073396 doi: 10.3390/app12073396
![]() |
[25] |
Zibetti GR, Wickboldt JA, de Freitas EP (2022) Context-aware environment monitoring to support LPWAN-based battlefield applications. Computer Communications 189: 18–27. https://doi.org/10.1016/j.comcom.2022.02.020 doi: 10.1016/j.comcom.2022.02.020
![]() |
[26] |
Trevathan J, Read W, Sattar A (2022) Implementation and Calibration of an IoT Light Attenuation Turbidity Sensor. Internet of Things 2022: 100576. https://doi.org/10.1016/j.iot.2022.100576 doi: 10.1016/j.iot.2022.100576
![]() |
[27] |
Dong M, Sun Z, Yu H, et al. (2022) Performance Evaluation of the Transmission Quality of the Internet of Things in Farmland Environmental Monitoring Based on ns-3. Mobile Information Systems 2022. https://doi.org/10.1155/2022/3148348 doi: 10.1155/2022/3148348
![]() |
[28] |
Sarramia D, Claude A, Ogereau F, et al. (2022) CEBA: A Data Lake for Data Sharing and Environmental Monitoring. Sensors 22: 2733. https://doi.org/10.3390/s22072733 doi: 10.3390/s22072733
![]() |
[29] |
Zhang S, Guo Y, Li S, et al. (2022) Investigation on environment monitoring system for a combination of hydroponics and aquaculture in greenhouse. Information Processing in Agriculture 9: 123–134. https://doi.org/10.1016/j.inpa.2021.06.006 doi: 10.1016/j.inpa.2021.06.006
![]() |
[30] |
Sinha BB, Dhanalakshmi R (2022) Recent advancements and challenges of Internet of Things in smart agriculture: A survey. Future Generation Computer Systems 126: 169–184. https://doi.org/10.1016/j.future.2021.08.006 doi: 10.1016/j.future.2021.08.006
![]() |
[31] |
Rehman A, Saba T, Kashif M, et al. (2022) A revisit of Internet of things technologies for monitoring and control strategies in smart agriculture. Agronomy 12: 127. https://doi.org/10.3390/agronomy12010127 doi: 10.3390/agronomy12010127
![]() |
[32] | Yan W, Wang Z, Wang H, et al. (2022) Survey on recent smart gateways for smart home: Systems, technologies, and challenges. Transactions on Emerging Telecommunications Technologies 33: e4067. https://doi.org/10.1002/ett.4067 |
[33] |
Kim D, Yoon Y, Lee J, et al. (2022) Design and Implementation of Smart Buildings: A Review of Current Research Trend. Energies 15: 4278. https://doi.org/10.3390/en15124278 doi: 10.3390/en15124278
![]() |
[34] |
Jat AS, Grønli TM (2022) Smart Watch for Smart Health Monitoring: A Literature Review. In International Work-Conference on Bioinformatics and Biomedical Engineering 2022: 256–268. https://doi.org/10.1007/978-3-031-07704-3_21 doi: 10.1007/978-3-031-07704-3_21
![]() |
[35] |
Avina-Bravo EG, Cassirame J, Escriba C, et al. (2022) Smart electrically assisted bicycles as health monitoring systems: a review. Sensors 22: 468. https://doi.org/10.3390/s22020468 doi: 10.3390/s22020468
![]() |
[36] |
Dashkevych O, Portnov BA (2022) Criteria for Smart City Identification: A Systematic Literature Review. Sustainability 14: 4448. https://doi.org/10.3390/su14084448 doi: 10.3390/su14084448
![]() |
[37] |
Schmidt DN, Pieraccini M, Evans L (2022) Marine protected areas in the context of climate change: key challenges for coastal social-ecological systems. Philosophical Transactions of the Royal Society B 377: 20210131. https://doi.org/10.1098/rstb.2021.0131 doi: 10.1098/rstb.2021.0131
![]() |
[38] |
da Costa Filho BM, Duarte AC, Santos TAR (2022) Environmental monitoring approaches for the detection of organic contaminants in marine environments: a critical review. Trends in Environmental Analytical Chemistry 2022: e00154. https://doi.org/10.1016/j.teac.2022.e00154 doi: 10.1016/j.teac.2022.e00154
![]() |
[39] | Osman N, Jamlos MF, Dzaharudin F, et al. (2022). Real-time and predictive analytics of air quality with iot system: A review. Recent Trends in Mechatronics Towards Industry 4.0 2022: 107–116. https://doi.org/10.1007/978-981-33-4597-3_11 |
[40] |
Khanna A, Kaur S (2020) Internet of things (IoT), applications and challenges: a comprehensive review. Wireless Personal Communications 114: 1687–1762. https://doi.org/10.1007/s11277-020-07446-4 doi: 10.1007/s11277-020-07446-4
![]() |
[41] |
Razmjoo A, Gandomi A, Mahlooji M, et al. (2022). An Investigation of the Policies and Crucial Sectors of Smart Cities Based on IoT Application. Applied Sciences 12: 2672. https://doi.org/10.3390/app12052672 doi: 10.3390/app12052672
![]() |
[42] |
Mori H, Kundaliya J, Naik K, et al. (2022) IoT technologies in smart environment: security issues and future enhancements. Environmental Science and Pollution Research 2022: 1–19. https://doi.org/10.1007/s11356-022-20132-1 doi: 10.1007/s11356-022-20132-1
![]() |
[43] |
Shah AAI, Fauzi SSM, Gining RAJM, et al. (2021) A review of IoT-based smart waste level monitoring system for smart cities. Indonesia J Electr Eng Comput Sci 21: 450–456. https://doi.org/10.11591/ijeecs.v21.i1.pp450-456 doi: 10.11591/ijeecs.v21.i1.pp450-456
![]() |
[44] |
Akram SV, Singh R, Gehlot A, et al. (2021) Role of wireless aided technologies in the solid waste management: A comprehensive review. Sustainability 13: 13104. https://doi.org/10.3390/su132313104 doi: 10.3390/su132313104
![]() |
[45] |
Putra RH, Kusuma FT, Damayanti TN, et al. (2019) IoT: smart garbage monitoring using android and real time database. TELKOMNIKA (Telecommunication Computing Electronics and Control) 17: 1483–1491. https://doi.org/10.12928/telkomnika.v17i3.10121 doi: 10.12928/telkomnika.v17i3.10121
![]() |
[46] |
Abuga D, Raghava NS (2021) Real-time smart garbage bin mechanism for solid waste management in smart cities. Sustainable Cities and Society 75: 103347. https://doi.org/10.1016/j.scs.2021.103347 doi: 10.1016/j.scs.2021.103347
![]() |
[47] |
Ramson SR, Moni DJ, Vishnu S, et al. (2021) An IoT-based bin level monitoring system for solid waste management. Journal of Material Cycles and Waste Management 23: 516–525. https://doi.org/10.1007/s10163-020-01137-9 doi: 10.1007/s10163-020-01137-9
![]() |
[48] |
Alobaidy HA, Nordin R, Mandeep JS, et al. (2022) Low Altitude Platform-based Airborne IoT Network (LAP-AIN) for Water Quality Monitoring in Harsh Tropical Environment. IEEE Internet of Things Journal 9: 20034–20054. https://doi.org/10.1109/JIOT.2022.3171294 doi: 10.1109/JIOT.2022.3171294
![]() |
[49] |
Yusof NM, Zulkifli MF, Yusof M, et al. (2018) Smart waste bin with real-time monitoring system. International Journal of Engineering & Technology 7: 725–729. https://doi.org/10.14419/ijet.v7i2.29.14006 doi: 10.14419/ijet.v7i2.29.14006
![]() |
[50] |
Lozano Á, Caridad J, De Paz JF, et al. (2018). Smart waste collection system with low consumption LoRaWAN nodes and route optimization. Sensors 18: 1465. https://doi.org/10.3390/s18051465 doi: 10.3390/s18051465
![]() |
[51] | Kamm M, Gau M, Schneider J, et al. (2020) Smart Waste Collection Processes-A Case Study about Smart Device Implementation. In Proceedings of the 53rd Hawaii International Conference on System Sciences. https://doi.org/10.24251/HICSS.2020.810 |
[52] |
Kang KD, Kang H, Ilankoon IMSK, et al. (2020) Electronic waste collection systems using Internet of Things (IoT): Household electronic waste management in Malaysia. Journal of cleaner production 252: 119801. https://doi.org/10.1016/j.jclepro.2019.119801 doi: 10.1016/j.jclepro.2019.119801
![]() |
[53] |
Shanthini E, Sangeetha V, Jagadeeswari M, et al. (2022) IoT based Smart City Garbage Bin for Waste Management. In 2022 4th International Conference on Smart Systems and Inventive Technology (ICSSIT) 2022: 105–110. https://doi.org/10.1109/ICSSIT53264.2022.9716343 doi: 10.1109/ICSSIT53264.2022.9716343
![]() |
[54] |
Wen Z, Hu S, De Clercq D, et al. (2018) Design, implementation, and evaluation of an Internet of Things (IoT) network system for restaurant food waste management. Waste management 73: 26–38. https://doi.org/10.1016/j.wasman.2017.11.054 doi: 10.1016/j.wasman.2017.11.054
![]() |
[55] |
Idwan S, Mahmood I, Zubairi JA., et al. (2020) Optimal management of solid waste in smart cities using the Internet of things. Wireless Personal Communications 110: 485–501. https://doi.org/10.1007/s11277-019-06738-8 doi: 10.1007/s11277-019-06738-8
![]() |
[56] |
Al-Masri E, Diabate I, Jain R, et al. (2018) Recycle. io: An IoT-enabled framework for urban waste management. In 2018 IEEE international conference on big data (big data) 2018: 5285–5287. https://doi.org/10.1109/BigData.2018.8622117 doi: 10.1109/BigData.2018.8622117
![]() |
[57] |
Chen WE, Wang YH, Huang PC, et al. (2018) A smart IoT system for waste management. In 2018 1st International Cognitive Cities Conference (IC3) 2018: 202–203. https://doi.org/10.1109/IC3.2018.00-24 doi: 10.1109/IC3.2018.00-24
![]() |
[58] |
Marques P, Manfroi D, Deitos E, et al. (2019) An IoT-based smart cities infrastructure architecture applied to a waste management scenario. Ad Hoc Networks 87: 200–208. https://doi.org/10.1016/j.adhoc.2018.12.009 doi: 10.1016/j.adhoc.2018.12.009
![]() |
[59] |
Thürer M, Pan YH, Qu T, et al. (2019) Internet of Things (IoT) driven kanban system for reverse logistics: solid waste collection. Journal of Intelligent Manufacturing 30: 2621–2630. https://doi.org/10.1007/s10845-016-1278-y doi: 10.1007/s10845-016-1278-y
![]() |
[60] |
Misra D, Das G, Chakrabortty T, et al. (2018) An IoT-based waste management system monitored by cloud. Journal of Material Cycles and Waste Management 20: 1574–1582. https://doi.org/10.1007/s10163-018-0720-y doi: 10.1007/s10163-018-0720-y
![]() |
[61] |
Pardini K, Rodrigues JJ, Kozlov SA, et al. (2019) IoT-based solid waste management solutions: a survey. Journal of Sensor and Actuator Networks 8: 5. https://doi.org/10.3390/jsan8010005 doi: 10.3390/jsan8010005
![]() |
[62] |
Asefa EM, Damtew YT, Barasa KB (2021) Landfill site selection using GIS-based multicriteria evaluation technique in Harar City, Eastern Ethiopia. Environmental health insights 15: 11786302211053174. https://doi.org/10.1177/11786302211053174 doi: 10.1177/11786302211053174
![]() |
[63] |
Singh A (2019) Remote sensing and GIS applications for municipal waste management. Journal of environmental management 243: 22–29. https://doi.org/10.1016/j.jenvman.2019.05.017 doi: 10.1016/j.jenvman.2019.05.017
![]() |
[64] |
Vu HL, Ng KTW, Fallah B, et al. (2020) Interactions of residential waste composition and collection truck compartment design on GIS route optimization. Waste management 102: 613–623. https://doi.org/10.1016/j.wasman.2019.11.028 doi: 10.1016/j.wasman.2019.11.028
![]() |
[65] | Tarone AV, Katgube AA, Shendre HH, et al. (2018) IoT based smart garbage monitoring system using ESP8266 with GPS link. Int Res J Eng Technol 5: 5–6. |
[66] |
Ferronato N, Preziosi G, Portillo MAG, et al. (2020) Assessment of municipal solid waste selective collection scenarios with geographic information systems in Bolivia. Waste Management 102: 919–931. https://doi.org/10.1016/j.wasman.2019.12.010 doi: 10.1016/j.wasman.2019.12.010
![]() |
[67] |
Okot P, Ogao PJ, Abandu J (2019) Site selection model for urban solid waste disposal management using GIS and remote sensing: a case of Gulu Municipality. International Journal of Environment and Waste Management 24: 405–436. https://doi.org/10.1504/IJEWM.2019.103645 doi: 10.1504/IJEWM.2019.103645
![]() |
[68] |
Ahmad S, Kim DH (2020) Quantum GIS based descriptive and predictive data analysis for effective planning of waste management. Ieee Access 8: 46193–46205. https://doi.org/10.1109/ACCESS.2020.2979015 doi: 10.1109/ACCESS.2020.2979015
![]() |
[69] |
MİHİRETİE (2020) A Suitable Solid Waste Disposal Site Selection Using Geographical Information System: A Case of Debre Markos Town, Ethiopia. International Journal of Environment and Geoinformatics 9: 1–8. https://doi.org/10.30897/ijegeo.784087 doi: 10.30897/ijegeo.784087
![]() |
[70] |
Amal L, Son LH, Chabchoub H, et al. (2020) Analysis of municipal solid waste collection using GIS and multi-criteria decision aid. Applied Geomatics 12: 193–208. https://doi.org/10.1007/s12518-019-00291-6 doi: 10.1007/s12518-019-00291-6
![]() |
[71] |
Mussa A, Suryabhagavan KV (2021) Solid waste dumping site selection using GIS-based multi-criteria spatial modeling: a case study in Logia town, Afar region, Ethiopia. Geology, Ecology, and Landscapes 5: 186–198. https://doi.org/10.1080/24749508.2019.1703311 doi: 10.1080/24749508.2019.1703311
![]() |
[72] |
Zeeshan S, Shahid Z, Khan S, et al. (2018) Solid waste management in Korangi District of Karachi using GPS and GIS: a case study. In 2018 7th International Conference on Computer and Communication Engineering (ICCCE) 2018: 1–4. https://doi.org/10.1109/ICCCE.2018.8539241 doi: 10.1109/ICCCE.2018.8539241
![]() |
[73] |
Gautam S, Brema J, Dhasarathan R (2020) Spatio-temporal estimates of solid waste disposal in an urban city of India: A remote sensing and GIS approach. Environmental Technology & Innovation 18: 100650. https://doi.org/10.1016/j.eti.2020.100650 doi: 10.1016/j.eti.2020.100650
![]() |
[74] | Ali N, Awais M, Muzammul M, et al. (2018) Intelligent System for Garbage collection: IoT technology with Ultrasonic sensor and Arduino Mega. Int J Comput Sci Netw 18: 102. |
[75] |
Sohag MU, Podder AK (2020) Smart garbage management system for a sustainable urban life: An IoT based application. Internet of Things 11: 100255. https://doi.org/10.1016/j.iot.2020.100255 doi: 10.1016/j.iot.2020.100255
![]() |
[76] |
Ramson SR, Moni DJ, Vishnu S, et al. (2021) An IoT-based bin level monitoring system for solid waste management. Journal of Material Cycles and Waste Management 23: 516–525. https://doi.org/10.1007/s10163-020-01137-9 doi: 10.1007/s10163-020-01137-9
![]() |
[77] |
Hussain A, Draz U, Ali T, et al. (2020) Waste management and Prediction of air pollutants using IoT and machine learning approach. Energies 13: 3930. https://doi.org/10.3390/en13153930 doi: 10.3390/en13153930
![]() |
[78] | Li Y, Wang X, Zhao Z, et al. (2020) Lagoon water quality monitoring based on digital image analysis and machine learning estimators. Water Research 172 115471. https://doi.org/10.1016/j.watres.2020.115471 |
[79] |
Aliyu AG, Jamil NRB, Adam MBB, et al. (2020) Spatial and seasonal changes in monitoring water quality of Savanna River system. Arabian Journal of Geosciences 13: 1–13. https://doi.org/10.1007/s12517-019-5026-4 doi: 10.1007/s12517-019-5026-4
![]() |
[80] |
Ragi NM, Holla R, Manju G (2019) Predicting water quality parameters using machine learning. In 2019 4th International Conference on Recent Trends on Electronics, Information, Communication & Technology (RTEICT) 2019: 1109–1112. https://doi.org/10.1109/RTEICT46194.2019.9016825 doi: 10.1109/RTEICT46194.2019.9016825
![]() |
[81] |
Budiarti RPN, Sukaridhoto S, Hariadi M, et al. (2019) Big data technologies using SVM (case study: surface water classification on regional water utility company in Surabaya). In 2019 International Conference on Computer Science, Information Technology, and Electrical Engineering (ICOMITEE) 2019: 94–101. https://doi.org/10.1109/ICOMITEE.2019.8920823 doi: 10.1109/ICOMITEE.2019.8920823
![]() |
[82] |
Sharmila RS, Sushma R, Mahanth MU, et al. (2022) Water Pollution Monitoring System Using IoT. In ICDSMLA 2020: 1163–1170. https://doi.org/10.1007/978-981-16-3690-5_111 doi: 10.1007/978-981-16-3690-5_111
![]() |
[83] |
Porwal A, Mishra SK, Kela A, et al. (2022) Prediction and Prevention of Water Pollution by Industries using IoT based Monitoring System. In 2022 IEEE 11th International Conference on Communication Systems and Network Technologies (CSNT) 2022: 504–509. https://doi.org/10.1109/CSNT54456.2022.9787664 doi: 10.1109/CSNT54456.2022.9787664
![]() |
[84] | Buhion J, Buhion J, Ocon J (2022) The development of a real time, interactive water level monitoring system through SMS with AC loads control. https://doi.org/10.47577/technium.v4i5.6698 |
[85] |
Chen Q, Cheng G, Fang Y, et al. (2018) Real-time learning-based monitoring system for water contamination. In 2018 4th International Conference on Universal Village (UV) 2018: 1–5. https://doi.org/10.1109/UV.2018.8642146 doi: 10.1109/UV.2018.8642146
![]() |
[86] |
Islam MA, Khan RH, Syeed M (2020) A smart and integrated surface water monitor system architecture: Bangladesh perspective. In Proceedings of the International Conference on Computing Advancements 2020: 1–6. https://doi.org/10.1145/3377049.3377103 doi: 10.1145/3377049.3377103
![]() |
[87] | Nasto I, Zanaj E, Bakaj A, et al. (2022) Smart Water Quality Monitoring in Vlora: Design of an expert system for real-time monitoring of drinking water and detection of contamination in the aqueduct of the city of Vlora, Albania. In 2022 11th Mediterranean Conference on Embedded Computing (MECO) 2022: 1–8. https://doi.org/10.1109/MECO55406.2022.9797157 |
[88] |
Jan F, Min-Allah N, Düştegör D (2021) IoT based smart water quality monitoring: Recent techniques, trends and challenges for domestic applications. Water 13: 1729. https://doi.org/10.3390/w13131729 doi: 10.3390/w13131729
![]() |
[89] |
Rao KR, Srinija S, Bindu KH, et al. (2018) IOT based water level and quality monitoring system in overhead tanks. International Journal of Engineering & Technology 7: 379–383. https://doi.org/10.14419/ijet.v7i2.7.10747 doi: 10.14419/ijet.v7i2.7.10747
![]() |
[90] | Shrutika AD, Suhas K, Swapnil K (2020) Smart Tank Water Monitoring System using IOT. In International Journal of Scientific & Engineering Research 6. |
[91] |
Pasika S, Gandla ST (2020) Smart water quality monitoring system with cost-effective using IoT. Heliyon 6: e04096. https://doi.org/10.1016/j.heliyon.2020.e04096 doi: 10.1016/j.heliyon.2020.e04096
![]() |
[92] |
Srivastava S, Vaddadi S, Sadistap S (2018) Smartphone-based System for water quality analysis. Applied Water Science 8: 1–13. https://doi.org/10.1007/s13201-018-0780-0 doi: 10.1007/s13201-018-0780-0
![]() |
[93] |
Alam AU, Clyne D, Deen MJ (2021) A low-cost multi-parameter water quality monitoring system. Sensors 21: 3775. https://doi.org/10.3390/s21113775 doi: 10.3390/s21113775
![]() |
[94] | Jha MK, Sah RK, Rashmitha MS, et al. (2018) Smart water monitoring system for real-time water quality and usage monitoring. In 2018 International Conference on Inventive Research in Computing Applications (CIRCA) 2018: 617–621. |
[95] |
Sui H, Zheng G, Zhou J, et al. (2020) Application of NB-IoT Technology in City Open Water Monitoring. 2020 6th International Symposium on System and Software Reliability (ISSSR) 2020: 95–98. https://doi.org/10.1109/ISSSR51244.2020.00023 doi: 10.1109/ISSSR51244.2020.00023
![]() |
[96] |
Amado TM, Cruz JCD (2018) Development of machine learning-based predictive models for air quality monitoring and characterization. In TENCON 2018-2018 IEEE Region 10 Conference 2018: 0668–0672. https://doi.org/10.1109/TENCON.2018.8650518 doi: 10.1109/TENCON.2018.8650518
![]() |
[97] |
Mihăiţă AS, Dupont L, Chery O, et al. (2019) Evaluating air quality by combining stationary, smart mobile pollution monitoring and data-driven modelling. Journal of cleaner production 221: 398–418. https://doi.org/10.1016/j.jclepro.2019.02.179 doi: 10.1016/j.jclepro.2019.02.179
![]() |
[98] |
Shetty C, Sowmya BJ, Seema S, et al. (2020) Air pollution control model using machine learning and IoT techniques. In Advances in Computers 117: 187–218. https://doi.org/10.1016/bs.adcom.2019.10.006 doi: 10.1016/bs.adcom.2019.10.006
![]() |
[99] | Mohan Kalyan C, Deepthi N, Harshitha D (2022) Air And Noise Cognizer using IoT And Machine Learning. |
[100] |
Yang H, Zhao J, Li G (2022) A new hybrid prediction model of PM2.5 concentration based on secondary decomposition and optimized extreme learning machine. Environmental Science and Pollution Research 2022: 1–28. https://doi.org/10.1007/s11356-022-20375-y doi: 10.1007/s11356-022-20375-y
![]() |
[101] |
Ayele TW, Mehta R (2018) Air pollution monitoring and Prediction using IoT. In second international conference on inventive communication and computational technologies (ICICCT) 2018: 1741–1745. https://doi.org/10.1109/ICICCT.2018.8473272 doi: 10.1109/ICICCT.2018.8473272
![]() |
[102] |
Thu MY, Htun W, Aung YL, et al. (2018) Smart air quality monitoring system with LoRaWAN. In IEEE International Conference on Internet of Things and Intelligence System (IOTAIS) 2018: 10–15. https://doi.org/10.1109/IOTAIS.2018.8600904 doi: 10.1109/IOTAIS.2018.8600904
![]() |
[103] |
Ou CH, Chen YA, Huang TW, et al. (2020) Design and implementation of anomaly condition detection in agricultural IoT platform system. In 2020 International Conference on Information Networking (ICOIN) 2020: 184–189. https://doi.org/10.1109/ICOIN48656.2020.9016618 doi: 10.1109/ICOIN48656.2020.9016618
![]() |
[104] |
Deng F, Zuo P, Wen K, et al. (2020) Novel soil environment monitoring system based on RFID sensor and LoRa. Computers and Electronics in Agriculture 169: 105169. https://doi.org/10.1016/j.compag.2019.105169 doi: 10.1016/j.compag.2019.105169
![]() |
[105] |
Rosero-Montalvo PD, Caraguay-Procel JA, Jaramillo ED, et al. (2018) Air quality monitoring intelligent system using machine learning techniques. In International Conference on Information Systems and Computer Science (INCISCOS) 2018: 75–80. https://doi.org/10.1109/INCISCOS.2018.00019 doi: 10.1109/INCISCOS.2018.00019
![]() |
[106] |
Cheng M, Fang F, Navon IM, et al. (2022) Spatio-Temporal Hourly and Daily Ozone Forecasting in China Using a Hybrid Machine Learning Model: Autoencoder and Generative Adversarial Networks. Journal of Advances in Modeling Earth Systems 14: e2021MS002806. https://doi.org/10.1029/2021MS002806 doi: 10.1029/2021MS002806
![]() |
[107] |
Ali A, Zhu Y, Zakarya M (2021) A data aggregation based approach to exploit dynamic Spatio-temporal correlations for citywide crowd flows Prediction in fog computing. Multimedia Tools and Applications 80: 31401–31433. https://doi.org/10.1007/s11042-020-10486-4 doi: 10.1007/s11042-020-10486-4
![]() |
[108] |
Wong MS, Wang T, Ho HC, et al. (2018) Towards a smart city: Development and application of an improved integrated environmental monitoring system. Sustainability 10: 623. https://doi.org/10.3390/su10030623 doi: 10.3390/su10030623
![]() |
[109] |
Siddique AB, Kazmi R, Khan HU, et al. (2022) An Intelligent and Secure Air Quality Monitoring System Using Neural Network Algorithm and Blockchain. IETE Journal of Research 2022: 1–14. https://doi.org/10.1080/03772063.2022.2052984 doi: 10.1080/03772063.2022.2052984
![]() |
[110] |
Arroyo P, Gómez-Suárez J, Suárez JI, et al. (2021) Low-Cost Air Quality Measurement System Based on Electrochemical and PM Sensors with Cloud Connection. Sensors 21: 6228. https://doi.org/10.3390/s21186228 doi: 10.3390/s21186228
![]() |
[111] |
Radovanovic I, Popovic I (2021) Identification of Degrading Effects in the Operation of Neighboring Photovoltaic Systems in Urban Environments. Electronics 10: 762. https://doi.org/10.3390/electronics10070762 doi: 10.3390/electronics10070762
![]() |
[112] |
Saini J, Dutta M, Marques G (2021) Indoor air quality monitoring systems and COVID-19. In Emerging Technologies During the Era of COVID-19 Pandemic 2021: 133–147. https://doi.org/10.1007/978-3-030-67716-9_9 doi: 10.1007/978-3-030-67716-9_9
![]() |
[113] |
Kristiani E, Yang CT, Huang CY, et al. (2021) The implementation of a cloud-edge computing architecture using Open Stack and Kubernetes for air quality monitoring application. Mobile Networks and Applications 26: 1070–1092. https://doi.org/10.1007/s11036-020-01620-5 doi: 10.1007/s11036-020-01620-5
![]() |
[114] |
Chaudhary V, Ashraf N, Khalid M, et al. (2022) Emergence of MXene–Polymer Hybrid Nanocomposites as High-Performance Next-Generation Chemiresistors for Efficient Air Quality Monitoring. Advanced Functional Materials 2022: 2112913. https://doi.org/10.1002/adfm.202112913 doi: 10.1002/adfm.202112913
![]() |
[115] |
Amuthadevi C, Vijayan DS, Ramachandran V (2021) Development of air quality monitoring (QAM) models using different machine learning approaches. Journal of Ambient Intelligence and Humanized Computing 2021: 1–13. https://doi.org/10.1007/s12652-020-02724-2 doi: 10.1007/s12652-020-02724-2
![]() |
[116] |
Popović I, Radovanovic I, Vajs I, et al. (2022) Building Low-Cost Sensing Infrastructure for Air Quality Monitoring in Urban Areas Based on Fog Computing. Sensors 22: 1026. https://doi.org/10.3390/s22031026 doi: 10.3390/s22031026
![]() |
[117] |
Lambey V, Prasad AD (2021) A review on air quality measurement using an unmanned aerial vehicle. Water, Air, & Soil Pollution 232: 1–32. https://doi.org/10.1007/s11270-020-04973-5 doi: 10.1007/s11270-020-04973-5
![]() |
[118] |
Saini J, Dutta M, Marques G (2020) A comprehensive review on indoor air quality monitoring systems for enhanced public health. Sustainable environment research 30: 1–12. https://doi.org/10.1186/s42834-020-0047-y doi: 10.1186/s42834-020-0047-y
![]() |
[119] |
Chojer H, Branco PTBS, Martins FG, et al. (2020) Development of low-cost indoor air quality monitoring devices: Recent advancements. Science of The Total Environment 727: 138385. https://doi.org/10.1016/j.scitotenv.2020.138385 doi: 10.1016/j.scitotenv.2020.138385
![]() |
[120] |
Ameer S, Shah MA, Khan A, et al. (2019) Comparative analysis of machine learning techniques for predicting air quality in smart cities. IEEE Access 7: 128325–128338. https://doi.org/10.1109/ACCESS.2019.2925082 doi: 10.1109/ACCESS.2019.2925082
![]() |
[121] |
Barot V, Kapadia V, Pandya S (2020) QoS enabled IoT based low cost air quality monitoring system with power consumption optimization. Cybernetics and Information Technologies 20: 122–140. https://doi.org/10.2478/cait-2020-0021 doi: 10.2478/cait-2020-0021
![]() |
[122] | Okokpujie K, Noma-Osaghae E, Modupe O, et al. (2018) A smart air pollution monitoring system. International Journal of Civil Engineering and Technology (IJCIET) 9: 799–809. |
[123] |
Liu L, Liu W, Zheng Y, et al. (2018) Third-eye: A mobile phone-enabled crowdsensing system for air quality monitoring. Proceedings of the ACM on Interactive, Mobile, Wearable and Ubiquitous Technologies 2: 1–26. https://doi.org/10.1145/3191752 doi: 10.1145/3191752
![]() |
[124] |
Anand P, Sekhar C, Cheong D, et al. (2019) Occupancy-based zone-level VAV system control implications on thermal comfort, ventilation, indoor air quality and building energy efficiency. Energy and Buildings 204: 109473. https://doi.org/10.1016/j.enbuild.2019.109473 doi: 10.1016/j.enbuild.2019.109473
![]() |
[125] |
Jo J, Jo B, Kim J, et al. (2020) Development of an IoT-based indoor air quality monitoring platform. Journal of Sensors 2020. https://doi.org/10.1155/2020/8749764 doi: 10.1155/2020/8749764
![]() |
[126] |
Goyal S (2022) IoT-Based Smart Air Quality Control System: Prevention to COVID-19. In IoT and Cloud Computing for Societal Good 2022: 15–23. https://doi.org/10.1007/978-3-030-73885-3_2 doi: 10.1007/978-3-030-73885-3_2
![]() |
[127] |
Kenarkoohi A, Noorimotlagh Z, Falahi S, et al. (2020) Hospital indoor air quality monitoring for the detection of SARS-CoV-2 (COVID-19) virus. Science of the Total Environment 748: 141324. https://doi.org/10.1016/j.scitotenv.2020.141324 doi: 10.1016/j.scitotenv.2020.141324
![]() |
[128] |
Ha QP, Metia S, Phung MD (2020) Sensing data fusion for enhanced indoor air quality monitoring. IEEE Sensors Journal 20: 4430–4441. https://doi.org/10.1109/JSEN.2020.2964396 doi: 10.1109/JSEN.2020.2964396
![]() |
[129] |
Benammar M, Abdaoui A, Ahmad SH, et al. (2018) A modular IoT platform for real-time indoor air quality monitoring. Sensors 18: 581. https://doi.org/10.3390/s18020581 doi: 10.3390/s18020581
![]() |
[130] |
Lee J, Jung Y, Sung SH, et al. (2021) High-performance gas sensor array for indoor air quality monitoring: The role of Au nanoparticles on WO 3, SnO 2, and NiO-based gas sensors. Journal of Materials Chemistry A 9: 1159–1167. https://doi.org/10.1039/D0TA08743B doi: 10.1039/D0TA08743B
![]() |
[131] |
Singer BC, Delp WW (2018) Response of consumer and research grade indoor air quality monitors to residential sources of fine particles. Indoor Air 28: 624–639. https://doi.org/10.1111/ina.12463 doi: 10.1111/ina.12463
![]() |
[132] |
Agarwal N, Meena CS, Raj BP, et al. (2021) Indoor air quality improvement in COVID-19 pandemic. Sustainable Cities and Society 70: 102942. https://doi.org/10.1016/j.scs.2021.102942 doi: 10.1016/j.scs.2021.102942
![]() |
[133] |
Saini J, Dutta M, Marques G (2021) Indoor air quality monitoring systems and COVID-19. In Emerging Technologies During the Era of COVID-19 Pandemic 2021: 133–147. https://doi.org/10.1007/978-3-030-67716-9_9 doi: 10.1007/978-3-030-67716-9_9
![]() |
[134] |
Pietrogrande MC, Casari L, Demaria G, et al. (2021) Indoor air quality in domestic environments during periods close to Italian COVID-19 lockdown. International journal of environmental research and public health 18: 4060. https://doi.org/10.3390/ijerph18084060 doi: 10.3390/ijerph18084060
![]() |
[135] |
Chang HS, Capuozzo B, Okumus B, et al. (2021) Why cleaning the invisible in a restaurant is important during COVID-19: A case study of indoor air quality of an open-kitchen restaurant. International Journal of Hospitality Management 94: 102854. https://doi.org/10.1016/j.ijhm.2020.102854 doi: 10.1016/j.ijhm.2020.102854
![]() |
[136] |
Megahed NA, Ghoneim EM (2021) Indoor Air Quality: Rethinking rules of building design strategies in post-pandemic architecture. Environmental Research 193: 110471. https://doi.org/10.1016/j.envres.2020.110471 doi: 10.1016/j.envres.2020.110471
![]() |
[137] |
Alonso A, Llanos J, Escandón R, et al. (2021) Effects of the Covid-19 pandemic on indoor air quality and thermal comfort of primary schools in winter in a Mediterranean climate. Sustainability 13: 2699. https://doi.org/10.3390/su13052699 doi: 10.3390/su13052699
![]() |
[138] |
Elsaid AM, Ahmed MS (2021) Indoor air quality strategies for air-conditioning and ventilation systems with the spread of the global coronavirus (COVID-19) epidemic: Improvements and recommendations. Environmental Research 199: 111314. https://doi.org/10.1016/j.envres.2021.111314 doi: 10.1016/j.envres.2021.111314
![]() |
[139] |
Meiss A, Jimeno-Merino H, Poza-Casado I, et al. (2021) Indoor air quality in naturally ventilated classrooms. Lessons learned from a case study in a COVID-19 scenario. Sustainability 13: 8446. https://doi.org/10.3390/su13158446 doi: 10.3390/su13158446
![]() |
[140] |
Anil I, Alagha O (2021) The impact of COVID-19 lockdown on the air quality of Eastern Province, Saudi Arabia. Air Quality, Atmosphere & Health 14: 117–128. https://doi.org/10.1007/s11869-020-00918-3 doi: 10.1007/s11869-020-00918-3
![]() |
[141] |
Nandan A, Siddiqui NA, Singh C, et al. (2021) COVID-19 pandemic in Uttarakhand, India: Environmental recovery or degradation? Journal of environmental chemical engineering 9: 106595. https://doi.org/10.1016/j.jece.2021.106595 doi: 10.1016/j.jece.2021.106595
![]() |
[142] |
Mousavi A, Wu J (2021) Indoor-generated PM2. 5 during COVID-19 shutdowns across California: application of the PurpleAir indoor–outdoor low-cost sensor network. Environmental science & technology 55: 5648–5656. https://doi.org/10.1021/acs.est.0c06937 doi: 10.1021/acs.est.0c06937
![]() |
[143] |
Filonchyk M, Hurynovich V, Yan H (2021) Impact of COVID-19 pandemic on air pollution in Poland based on surface measurements and satellite data. Aerosol and Air Quality Research 21: 200472. https://doi.org/10.4209/aaqr.200472 doi: 10.4209/aaqr.200472
![]() |
[144] |
Sangkham S, Thongtip S, Vongruang P (2021) Influence of air pollution and meteorological factors on the spread of COVID-19 in the Bangkok Metropolitan Region and air quality during the outbreak. Environmental Research 197: 111104. https://doi.org/10.1016/j.envres.2021.111104 doi: 10.1016/j.envres.2021.111104
![]() |
[145] |
Horb EC, Wentworth GR, Makar PA, et al. (2022) A decadal synthesis of atmospheric emissions, ambient air quality, and deposition in the oil sands region. Integrated environmental assessment and management 18: 333–360. https://doi.org/10.1002/ieam.4539 doi: 10.1002/ieam.4539
![]() |
[146] |
Lolli S (2021) Is the air too polluted for outdoor activities? Check by using your photovoltaic system as an air-quality monitoring device. Sensors 21: 6342. https://doi.org/10.3390/s21196342 doi: 10.3390/s21196342
![]() |
[147] |
Wang Z, Ma P, Zhang L, Chen H, et al. (2021) Systematics of atmospheric environment monitoring in China via satellite remote sensing. Air Quality, Atmosphere & Health 14: 157–169. https://doi.org/10.1007/s11869-020-00922-7 doi: 10.1007/s11869-020-00922-7
![]() |
[148] |
Assanov D, Zapasnyi V, Kerimray A (2021) Air quality and industrial emissions in the cities of Kazakhstan. Atmosphere 12: 314. https://doi.org/10.3390/atmos12030314 doi: 10.3390/atmos12030314
![]() |
[149] |
Danesi N, Jain M, Lee YH, et al. (2021) Monitoring atmospheric pollutants from ground-based observations. In 2021 IEEE USNC-URSI Radio Science Meeting (Joint with AP-S Symposium) 2021: 98–99. https://doi.org/10.23919/USNC-URSI51813.2021.9703595 doi: 10.23919/USNC-URSI51813.2021.9703595
![]() |
[150] |
Ascenso A, Augusto B, Silveira C, et al. (2021) Impacts of nature-based solutions on the urban atmospheric environment: a case study for Eindhoven, The Netherlands. Urban Forestry & Urban Greening 57: 126870. https://doi.org/10.1016/j.ufug.2020.126870 doi: 10.1016/j.ufug.2020.126870
![]() |
[151] |
Saravanan K, Anusuya E, Kumar R, et al. (2018) Real-time water quality monitoring using Internet of Things in SCADA. Environmental monitoring and assessment 190: 1–16. https://doi.org/10.1007/s10661-018-6914-x doi: 10.1007/s10661-018-6914-x
![]() |
[152] | George G, Menon NN, Abdulaziz A, et al. (2021) Citizen scientists contribute to real-time monitoring of lake water quality using 3D printed mini Secchi disks. Frontiers in Water 40. https://doi.org/10.3389/frwa.2021.662142 |
[153] |
Wu Z, Wang J, Bian C, et al. (2020) A MEMS-based multi-parameter integrated chip and its portable system for water quality detection. Micromachines 11: 63. https://doi.org/10.3390/mi11010063 doi: 10.3390/mi11010063
![]() |
[154] |
Schima R, Krüger S, Bumberger J, et al. (2019) Mobile monitoring—open-source based optical sensor system for service-oriented turbidity and dissolved organic matter monitoring. Frontiers in Earth Science 7: 184. https://doi.org/10.3389/feart.2019.00184 doi: 10.3389/feart.2019.00184
![]() |
[155] |
Cui L, Qiu M, Fan H, et al. (2018) Design and Research of Water Quality Monitoring System Based on nRF24L01 Wireless Communication. In IOP Conference Series: Earth and Environmental Science 208: 012074. https://doi.org/10.1088/1755-1315/208/1/012074 doi: 10.1088/1755-1315/208/1/012074
![]() |
[156] |
Alam, AU, Clyne D, Lush W, et al. (2021) A reusable, reagent-less free chlorine sensor using gold thin film electrode. Analyst 146: 2626–2631. https://doi.org/10.1039/D1AN00038A doi: 10.1039/D1AN00038A
![]() |
1. | Lifa Fang, Yanqiang Wu, Yuhua Li, Hongen Guo, Hua Zhang, Xiaoyu Wang, Rui Xi, Jialin Hou, Using Channel and Network Layer Pruning Based on Deep Learning for Real-Time Detection of Ginger Images, 2021, 11, 2077-0472, 1190, 10.3390/agriculture11121190 | |
2. | Xuanhong Wang, Kun Wu, Ying Zhang, Yun Xiao, Pengfei Xu, A GAN-based Denoising Method for Chinese Stele and Rubbing Calligraphic Image, 2022, 0178-2789, 10.1007/s00371-022-02410-8 | |
3. | Mattapally Sai Nithin, Avesha Shaik, A Balasundaram, Kovvuri Uday Surya Deveswar Reddy, Lakshmi Sai Ram kakarla, A Selvakumar, 2022, Transfer Learning Based Effective Approach for Classification of Flowers, 978-1-6654-7655-3, 316, 10.1109/ICCST55948.2022.10040338 | |
4. | Peichen Li, Huiqin Wang, Zhan Wang, Ke Wang, Chong Wang, Swin Routiformer: Moss Classification Algorithm Based on Swin Transformer With Bi-Level Routing Attention, 2024, 12, 2169-3536, 53396, 10.1109/ACCESS.2024.3387541 | |
5. | Gautam Yadav, Nishant Kumar, Rohit Rastogi, Aditi Arora, 2024, Knowledge Extraction through Floweret Recognition using ML and DS: A Sustainable Approach for 21st century Agriculture and Smart Cities, 979-8-3503-2820-2, 1, 10.1109/ic-ETITE58242.2024.10493426 | |
6. | Xionghua Huang, Tiaojun Zeng, MinSong Li, Yunfei Pan, Rohit Salgotra, Flower Recognition Algorithm Based on Nonlinear Regression of Pixel Value, 2024, 2024, 1563-5147, 1, 10.1155/2024/8868837 | |
7. | Aigou Li, Chen Yang, AGMG-Net: Leveraging multiscale and fine-grained features for improved cargo recognition, 2023, 20, 1551-0018, 16744, 10.3934/mbe.2023746 | |
8. | Ji Eun Kim, Yoon Ho Choi, Yeong Chan Lee, Gyeol Seong, Joo Hye Song, Tae Jun Kim, Eun Ran Kim, Sung Noh Hong, Dong Kyung Chang, Young-Ho Kim, Soo-Yong Shin, Deep learning model for distinguishing Mayo endoscopic subscore 0 and 1 in patients with ulcerative colitis, 2023, 13, 2045-2322, 10.1038/s41598-023-38206-6 | |
9. | Zhigao Zeng, Cheng Huang, Wenqiu Zhu, Zhiqiang Wen, Xinpan Yuan, Flower image classification based on an improved lightweight neural network with multi-scale feature fusion and attention mechanism, 2023, 20, 1551-0018, 13900, 10.3934/mbe.2023619 |
Layer | output size | ResNet14 | VA-ResNet14 | ResNet50 | VA-ResNet50 |
Conv 1+ | 112×112×64 | Conv, 7×7, stride 2 | Conv, 7×7, stride 2 | Conv, 7×7, stride 2 | Conv, 7×7, stride 2 |
Conv 2+ | 56×56×256 | Pool, 3×3, stride 2 | Pool, 3×3, stride 2 | Pool, 3×3, stride 2 | Pool, 3×3, stride 2 |
Res block | Attentional Res block | [Res block] × 3 | [Attentional Res block]×3 | ||
Conv 3+ | 28×28×512 | [Resblockstride2]×2 | [Resblockstride2Attentionconnect]×2 | [Resblockstride2]×4 | [Resblockstride2Attentionconnect]×4 |
Conv 4+ | 14×14×1024 | [Resblockstride2]×2 | [Resblockstride2Attentionconnect]×2 | [Resblockstride2]×6 | [Resblockstride2Attentionconnect]×6 |
Conv 5+ | 7×7×2048 | [Resblockstride2]×2 | [Resblockstride2Attentionconnect]×2 | [Resblockstride2]×3 | [Resblockstride2Attentionconnect]×3 |
Pool | 1×1×2048 | Global average pool | |||
Output | 17 | FC |
Method | VGGNet(16) | NIN | GoogLeNet | Inception V3 | ResNet14 | ResNet50 | VA-ResNet14 | VA-ResNet50 |
Accuacy | 63.1% | 64.2% | 65.8% | 66.9% | 67.7% | 81.3% | 69.4% | 85.7% |
Layer | output size | ResNet14 | VA-ResNet14 | ResNet50 | VA-ResNet50 |
Conv 1+ | 112×112×64 | Conv, 7×7, stride 2 | Conv, 7×7, stride 2 | Conv, 7×7, stride 2 | Conv, 7×7, stride 2 |
Conv 2+ | 56×56×256 | Pool, 3×3, stride 2 | Pool, 3×3, stride 2 | Pool, 3×3, stride 2 | Pool, 3×3, stride 2 |
Res block | Attentional Res block | [Res block] × 3 | [Attentional Res block]×3 | ||
Conv 3+ | 28×28×512 | [Resblockstride2]×2 | [Resblockstride2Attentionconnect]×2 | [Resblockstride2]×4 | [Resblockstride2Attentionconnect]×4 |
Conv 4+ | 14×14×1024 | [Resblockstride2]×2 | [Resblockstride2Attentionconnect]×2 | [Resblockstride2]×6 | [Resblockstride2Attentionconnect]×6 |
Conv 5+ | 7×7×2048 | [Resblockstride2]×2 | [Resblockstride2Attentionconnect]×2 | [Resblockstride2]×3 | [Resblockstride2Attentionconnect]×3 |
Pool | 1×1×2048 | Global average pool | |||
Output | 17 | FC |
Method | VGGNet(16) | NIN | GoogLeNet | Inception V3 | ResNet14 | ResNet50 | VA-ResNet14 | VA-ResNet50 |
Accuacy | 63.1% | 64.2% | 65.8% | 66.9% | 67.7% | 81.3% | 69.4% | 85.7% |