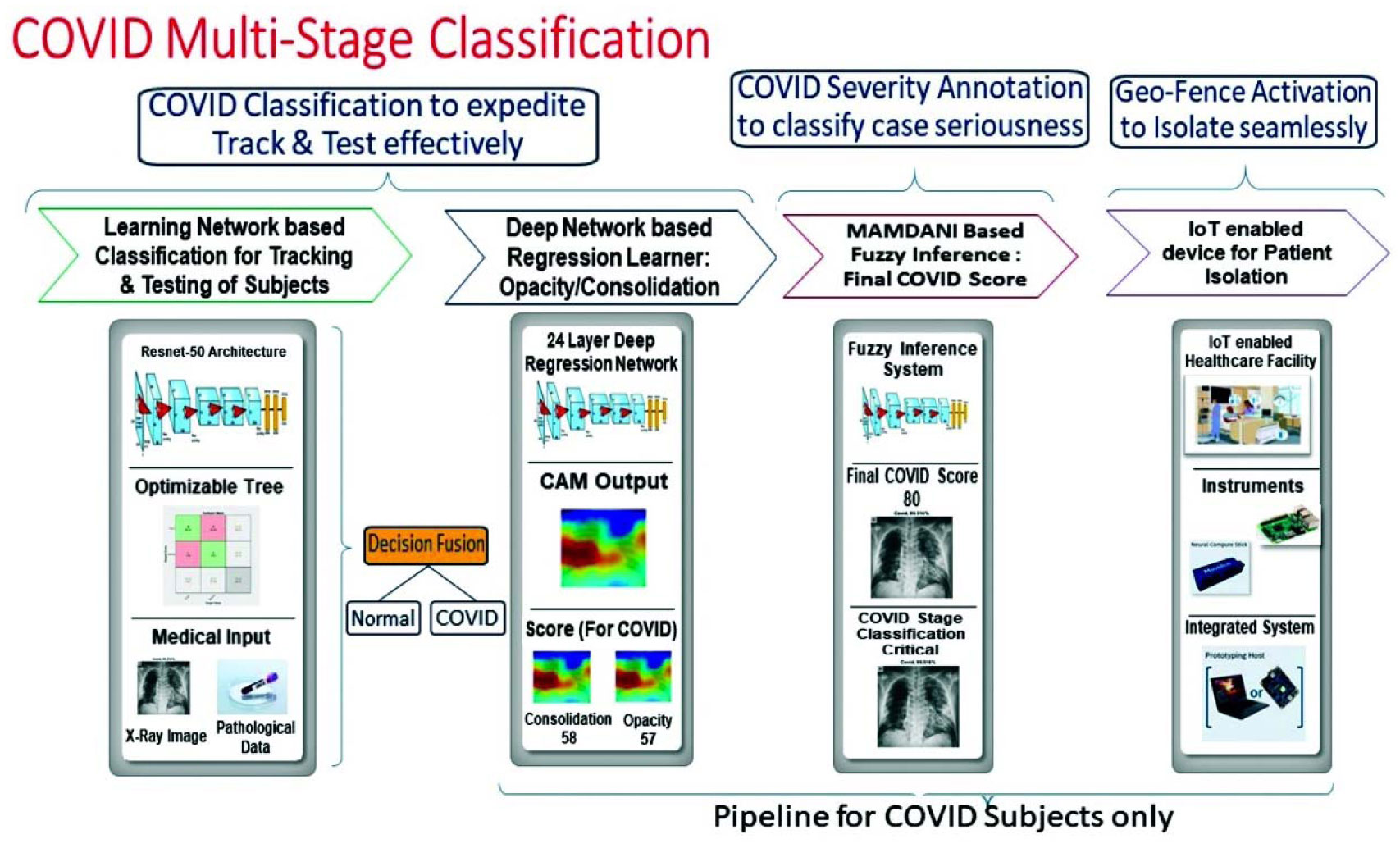
The use of Artificial Intelligence (AI) in combination with Internet of Things (IoT) drastically reduces the need to test the COVID samples manually, saving not only time but money and ultimately lives. In this paper, the authors have proposed a novel methodology to identify the COVID-19 patients with an annotated stage to enable the medical staff to manually activate a geo-fence around the subject thus ensuring early detection and isolation. The use of radiography images with pathology data used for COVID-19 identification forms the first-ever contribution by any research group globally. The novelty lies in the correct stage classification of COVID-19 subjects as well. The present analysis would bring this AI Model on the edge to make the facility an IoT-enabled unit. The developed system has been compared and extensively verified thoroughly with those of clinical observations. The significance of radiography imaging for detecting and identification of COVID-19 subjects with severity score tag for stage classification is mathematically established. In a Nutshell, this entire algorithmic workflow can be used not only for predictive analytics but also for prescriptive analytics to complete the entire pipeline from the diagnostic viewpoint of a doctor. As a matter of fact, the authors have used a supervised based learning approach aided by a multiple hypothesis based decision fusion based technique to increase the overall system's accuracy and prediction. The end to end value chain has been put under an IoT based ecosystem to leverage the combined power of AI and IoT to not only detect but also to isolate the coronavirus affected individuals. To emphasize further, the developed AI model predicts the respective categories of a coronavirus affected patients and the IoT system helps the point of care facilities to isolate and prescribe the need of hospitalization for the COVID patients.
Citation: Swarnava Biswas, Debajit Sen, Dinesh Bhatia, Pranjal Phukan, Moumita Mukherjee. Chest X-Ray image and pathological data based artificial intelligence enabled dual diagnostic method for multi-stage classification of COVID-19 patients[J]. AIMS Biophysics, 2021, 8(4): 346-371. doi: 10.3934/biophy.2021028
[1] | Soumyajit Podder, Somnath Bhattacharjee, Arijit Roy . An efficient method of detection of COVID-19 using Mask R-CNN on chest X-Ray images. AIMS Biophysics, 2021, 8(3): 281-290. doi: 10.3934/biophy.2021022 |
[2] | Dafina Xhako, Niko Hyka, Elda Spahiu, Suela Hoxhaj . Medical image analysis using deep learning algorithms (DLA). AIMS Biophysics, 2025, 12(2): 121-143. doi: 10.3934/biophy.2025008 |
[3] | Muhammad Asif Zahoor Raja, Adeeba Haider, Kottakkaran Sooppy Nisar, Muhammad Shoaib . Intelligent computing knacks for infected media and time delay impacts on dynamical behaviors and control measures of rumor-spreading model. AIMS Biophysics, 2024, 11(1): 1-17. doi: 10.3934/biophy.2024001 |
[4] | Mario Lefebvre . A Wiener process with jumps to model the logarithm of new epidemic cases. AIMS Biophysics, 2022, 9(3): 271-281. doi: 10.3934/biophy.2022023 |
[5] | Ibrahim Khater, Aaya Nassar . Looking into mucormycosis coinfections in COVID-19 patients using computational analysis. AIMS Biophysics, 2022, 9(1): 72-85. doi: 10.3934/biophy.2022007 |
[6] | Michael Worcester, Shayan Nejad, Pratyasha Mishra, Quintin Meyers, Melissa Gomez, Thomas Kuhlman . A low-cost stage-top incubation device for live human cell imaging using rapid prototyping methods. AIMS Biophysics, 2025, 12(2): 164-173. doi: 10.3934/biophy.2025010 |
[7] | Soumyajit Podder, Abhishek Mallick, Sudipta Das, Kartik Sau, Arijit Roy . Accurate diagnosis of liver diseases through the application of deep convolutional neural network on biopsy images. AIMS Biophysics, 2023, 10(4): 453-481. doi: 10.3934/biophy.2023026 |
[8] | Joshua Holcomb, Nicholas Spellmon, Yingxue Zhang, Maysaa Doughan, Chunying Li, Zhe Yang . Protein crystallization: Eluding the bottleneck of X-ray crystallography. AIMS Biophysics, 2017, 4(4): 557-575. doi: 10.3934/biophy.2017.4.557 |
[9] | Yong Zhou, Yiming Ding . Finding travel proportion under COVID-19. AIMS Biophysics, 2022, 9(3): 235-245. doi: 10.3934/biophy.2022020 |
[10] | Vittoria Raimondi, Alessandro Grinzato . A basic introduction to single particles cryo-electron microscopy. AIMS Biophysics, 2022, 9(1): 5-20. doi: 10.3934/biophy.2022002 |
The use of Artificial Intelligence (AI) in combination with Internet of Things (IoT) drastically reduces the need to test the COVID samples manually, saving not only time but money and ultimately lives. In this paper, the authors have proposed a novel methodology to identify the COVID-19 patients with an annotated stage to enable the medical staff to manually activate a geo-fence around the subject thus ensuring early detection and isolation. The use of radiography images with pathology data used for COVID-19 identification forms the first-ever contribution by any research group globally. The novelty lies in the correct stage classification of COVID-19 subjects as well. The present analysis would bring this AI Model on the edge to make the facility an IoT-enabled unit. The developed system has been compared and extensively verified thoroughly with those of clinical observations. The significance of radiography imaging for detecting and identification of COVID-19 subjects with severity score tag for stage classification is mathematically established. In a Nutshell, this entire algorithmic workflow can be used not only for predictive analytics but also for prescriptive analytics to complete the entire pipeline from the diagnostic viewpoint of a doctor. As a matter of fact, the authors have used a supervised based learning approach aided by a multiple hypothesis based decision fusion based technique to increase the overall system's accuracy and prediction. The end to end value chain has been put under an IoT based ecosystem to leverage the combined power of AI and IoT to not only detect but also to isolate the coronavirus affected individuals. To emphasize further, the developed AI model predicts the respective categories of a coronavirus affected patients and the IoT system helps the point of care facilities to isolate and prescribe the need of hospitalization for the COVID patients.
The COVID-19 pandemic keeps on devastatingly affecting the well-being and prosperity of the worldwide populace, brought about by the disease of people by the severe acute respiratory syndrome coronavirus 2 (SARS-CoV-2). A basic step in the battle against COVID-19 is the compelling screening of contaminated patients, with the end goal that those tainted can get prompt treatment and care, just as be secluded to alleviate the spread of the virus. COVID-19 has wreaked havoc and claimed thousands of lives across the globe. Every Government had issued public notices to maintain social distancing, wearing masks, use of sanitizers to combat the same. Sun et al. developed a model, that was trained using data from Heilongjiang province from January 23 to March 25, 2020. The model projected a smaller number of asymptomatic patients, indicating a large undiscovered asymptomatic pool. They also forecast the consequences of swift action to stop the COVID-19 spread in Heilongjiang and concluded that efforts to decrease mutual interaction could help Heilongjiang recover faster. It described the COVID-19 pandemic in Heilongjiang and recommended stringent controls for infected and asymptomatic people to decrease overall infections [1]. Melin et al. have used Self-Organizing Neural Networks (SNN) and fuzzy fractals to study COVID-19 time series data. Fuzzy fractals and self-organizing maps forecasted COVID-19 infections very accurately and developed pandemic protection methods [2]. Castillo et al. described a hybrid intelligent fuzzy fractal strategy for classifying countries based on fractal mathematical constructions which mathematically estimated the complexity of the non-linear dynamic behavior displayed by the country time series and fuzzy logic mathematical ideas. Fuzzy logic can represent and handle the categorization problem's inherent uncertainty. The hybrid intelligent technique uses a fuzzy system generated by a collection of fuzzy rules to classify countries based on their fractal dimensions. The COVID-19 data of confirmed and fatal cases are used in the hybrid technique. Using the COVID-19 time series data, the suggested hybrid technique uses fractal dimension definition and fuzzy logic concepts to accurately classify countries. The fuzzy system was built using public datasets from 11 nations, and the proposed categorization method was tested on 15 countries. The simulation results reveal a classification accuracy of over 93%, which is good for this challenging situation [3]–[5].
But it was extremely hard to spread awareness fully across especially in countries like India where the population happens to be from highly diversified backgrounds. Another issue that every Government faced was to conduct random tests, identify the patients & isolate. The principle screening strategy utilized for identifying COVID-19 cases in a reverse transcriptase-polymerase chain reaction (RT-PCR) testing [6], which can recognize SARS-CoV-2 RNA from respiratory samples (gathered through an assortment of means, for example, nasopharyngeal or oropharyngeal swabs). Needless to mention that the task in hand was a herculean one particularly given to believe the huge population that India has. The sample size also needs to be moderate across all the hotspot zones. The other important factor is to satisfy adequate infrastructure requirements is to take care of the affected individuals with proper medical care and support. While RT-PCR testing is the best quality level as it is profoundly explicit, it is a very tedious, relentless, and muddled manual procedure that is hard to come by. Besides, the affectability of RT-PCR testing is a profound factor and has not been accounted for reasonably and steadily to date [7], and introductory discoveries in China indicating moderately poor sensitivity [8].
An elective screening technique that has additionally been used for COVID-19 screening has been radiography assessment, where chest radiography imaging (e.g., chest X-Ray (CXR) or computed tomography (CT) imaging) is led and broke down by radiologists to search for visual markers related with SARS-CoV-2 viral disease. The pivot around which the proposed mathematical model revolves to give a predictive response for detailed detection and diagnosis of COVID-19 is based on radiography image i.e. X-Ray & CT Scan. It was found in early investigations that there is a distinct difference between chest radiography pictures of normal patients with those tainted with COVID-19 [9]–[11], with some proposing that radiography assessment could be utilized as an essential instrument for COVID-19 screening in plague areas [12]. For instance, Huang et al. [10] distinguished that most of the COVID-19 positive cases in their examination introduced reciprocal radiographic variations from the norm in CXR pictures. Guan et al. [11] recognized COVID-19 positive cases in their investigation, introduced by studying the radiographic anomalies, for example, ground-glass opacity, respective irregularities, and interstitial variations from the norm in CXR and CT pictures. In addition to this conventional process, the novelty of the detection algorithm lies in correctly determining the stage of COVID with a severity score tag. It would thus offer valuable information in the screening process quickly. The technique would also act as an aid to the conventional PCR based method to isolate the affected individuals quickly and efficiently.
In this article the proposed methodology involves a Deep Learning (DL) model in combination with a Classical Machine Learning (ML) Model to detect COVID-19 based on Chest Radiography & other medical & pathological vitals. The deep network architecture then acts on the radiography feed (X-Ray) and predicts an outcome at the evaluation stage. With intrinsic viewpoint of medical practitioners, we identified three key pathological parameters namely, neutrophil count, lymphocyte count, leukocyte count and the value of C-Reactive Protein (CRP) which goes through abnormal levels when coronavirus is contracted. All these parameters are duly incorporated in the machine learning model to make the analysis more realistic. The structural and pathological details of the parameters of the patients are incorporated in the machine learning model for training purpose.
All things considered, radiography assessment can be directed quicker and have more prominent accessibility in the chest radiology imaging frameworks in the present days [13]. It can be said that this imaging technique can be a decent supplement to RT-PCR testing especially since CXR imaging regularly proceeds as a major aspect of the standard method for patients with a respiratory complaint [14]. Moreover, it can be proposed that as the COVID-19 pandemic advances, there will be a more noteworthy dependence on versatile CXR due to the previously mentioned advantages [15].
Inspired by the requirement for a quicker understanding of radiography pictures, various artificial intelligence (AI) frameworks based on deep learning have been proposed [16]. The results have demonstrated to be very encouraging as far as precision in recognizing patients tainted with COVID-19 employing radiography imaging, with the attention principally on HRCT imaging [17]–[20].
Chest X-Ray is one of the common imaging modalities for envisioning and evaluating the basic results of thoracic illnesses, giving pictures of malady movement and treatment reaction. Magree et al. [21] recorded the rate of pneumonia affirmed with X-Ray imaging and exhibited a high occurrence, which guided the later anticipation and treatment of immunization. Jacobi et al. [15] depicted the most well-known appearances and examples of lung variation from the norm on chest X-Ray in COVID-19 and recommended that clinical network can every time depend on versatile chest X-Ray than CT scan. Wong et al. [22] exhibited that discoveries at chest radiography in patients with coronavirus disease 2019 habitually demonstrated bilateral lower zone consolidation, which crested at 10–12 days from the beginning of the symptoms. Borghesi et al. [23] introduced a test chest X-Ray scoring framework and applied it to hospitalized patients with COVID-19 to measure and screen the seriousness and movement of COVID-19. Distinctive from these considers the authors center around the viral pneumonia screening and expect to build up a quick and exact calculation to separate viral pneumonia from non-viral pneumonia and ordinary controls for the anticipation and control of a potential episode.
Wang et al. [24] proposed a feebly directed order and limitation structure, Rajpurkar et al. [25] built a 121-layer thick convolutional neural system that can play out the undertaking at a level exceeding the expectations of the radiologists, and Wang et al. [26] acquainted a consideration model which gives a spotlight on the injury zone and along these lines additionally improved the detection capabilities. Besides, numerous endeavours [27],[28] have been made to explore neural network-based grouping models for pneumonia recognition and the separation among viral and bacterial pneumonia, expecting to encourage fast referrals for kids who need immediate medications. Such arrangement models may be used to recognize viral and non-viral pneumonia since the classification of viral pneumonia depends on the cases with profoundly visual appearances in image data. The benefits of the medical Internet of Things (IoT) have been critical in the effort to avert a pandemic of COVID-19. Deep learning (DL) models may be developed in conjunction with IoT to free up medical staff and doctors to handle additional patients. According to coronavirus studies, various study publications have demonstrated that numerous probable cases of infection have been detected utilising DL models that are used to identify coronavirus using X-ray, CT scan, and ultrasound as input [29]. The authors of the mentioned article created a system for early stage detection of affected persons using IoT modalities. Previously, with 98 percent accuracy, ResNet101 in combination with Faster Region CNN (FRCR) was utilised to identify infected cases that were suspected positive [30]. Multiple ConvNet models were employed in this study framework, including CheXNet, SqueezeNet, ResNet18, ResNet101, VGG19, DenseNet201, Inceptionv3, and MobileNetv2. This methodology was validated using 423 cases of COVID virus infection, 1485 cases of viral pneumonia, and 1579 patients with non-diagnostic chest X-ray (CXR) pictures. A 3D convolutional neural network (3DCNN) was used to identify COVID-19 from other illnesses [31]. On the basis of attention-based deep 3D multiple instance learning (AD3D-MIL), an approach for fully automated screening of COVID-19 was devised [32]. The AD3D-MIL learned from labels distributed according to the Bernoulli distribution, which makes the method extremely efficient. M3 Lung-sys CT screening system is a suggested multi-task multi-slice deep learning system that aids in the detection of coronavirus-infected individuals [33]. The framework was inspired by the current advancements in deep learning models for automated identification of coronavirus illness. The suggested methodology based on ensemble learning (EL) will benefit both doctors and healthcare professionals in determining whether patients have COVID-19 (+) or healthy circumstances. The automated diagnosis of suspected COVID-19 instances is handled by a custom-built deep ensemble learning model and IoT-based architecture. This ensemble model combines InceptionResNetV2, ResNet152V2, VGG16, and DenseNet201. With medical sensors that monitor chest X-ray methods, deep ensemble learning is utilised to identify an illness. This dataset was utilised experimentally and was divided into two classes (COVID-19 (+) and healthy. In comparison to existing models, the suggested model will demonstrate improved efficiency and expediency in detecting coronavirus suspected patients. AD3D-MIL was trained and tested on 460 CT images. The following article describes how DCNN was developed using a dual-sampling approach and online attention refinement. This network was used to locate infection sites and to evenly redistribute pneumonia-infected sites. According to our testing, the DCNN was applied to 2,757 CT scan pictures of 2,057 patients. As a result, it is safe to presume that the precision of the impacted region is still lacking. DL-CRC, or DL-based chest radio classification, was developed for identifying individuals with confirmed coronavirus infection using chest X-ray [34]. The DL-CRC uses data augmentation and a generative adversarial network to construct simulated coronavirus-infected X-ray pictures. DL-CRC was used to evaluate multiple CXR datasets. The generative adversarial network (GAN) was designed as a support classifier model for the generation of synthetic CXR pictures. The created framework, CovidGAN, was invoked and used to distinguish other viral pneumonias from coronavirus infections [35]. CovidGAN was evaluated using 192 CXR pictures. It is uncertain whether any type of cross-validation was conducted on the CovidGAN design. VGG19 was utilised in the following article to construct an automatic technique for image classification. The image quality was enhanced by applying pre-processing techniques to eliminate sample bias. However, data fusion techniques can be used to improve classification accuracy. Using a ConvNet, a new transfer learning (TL) technique for categorising coronavirus suspected patients was introduced [31]. Varela-Santos et al. report a series of experiments utilising supervised learning models to accurately classify medical images from COVID-19 coronavirus patients and other lung disorders. This work uses picture texture feature descriptors, convolutional neural networks and feed-forward on freshly constructed COVID-19 databases. An automated approach that can diagnose COVID-19 disease from chest X-rays and computed tomography images of lungs was developed [36].
The traditional approach, RT-PCR is a very good technique to identify the healthy individuals with a higher accuracy. In the process, the technique also successfully identifies COVID affected individuals as well with classification accuracy varies from 65% to 95% [37]. Most of the COVID related works have been centred around CT scan and X-ray images. Individually the radiology images and the pathology data can be combined to have higher prediction accuracy for both healthy individuals and coronavirus affected individuals [13]. From radiology view point the diagnosis has a high percentage of accuracy for detecting COVID patients correctly but it sometimes fails to identify the healthy individuals. To strike a balance the authors have introduced the pathological data in combination with radiology images with a decision fusion on top of them. This approach essentially makes the prediction for COVID patients and normal ones with high accuracy in an equally likely fashion. Hence, the authors have proposed a multi hypothesis-based situation where pathological data in addition with the X-ray images make the model a balanced one. Secondly, most of the research papers have stopped at the detection level, i.e. COVID patient or Healthy patient. But the authors have extended the work a little further to again categorize the COVID patients into mild, severe and critical classes. Last but not the least, they have tried to wrap the entire architecture into an embedded architecture for easy deployment. More importantly, the activation of geo-fencing and the necessity for tracking, testing and isolating is emphasized in the finishing pipeline.
In order to detect and isolate coronavirus infected persons, the entire value chain has been placed under an IoT based ecosystem. The IoT technology allows point of care facilities isolate and prescribe the necessity for hospitalisation for COVID patients. This type of model development is for the first time reported in this article as the best of author's knowledge.
The end to end solution pipeline for this deployable architecture is given in Figure 1.
The inputs to this engine are radiography image and pathology data. Based on this a decision is made whether a person is COVID positive. Then the latter half of the pipeline is only for the COVID subjects. The stage classification would help in analysing the seriousness of the case. Moreover, the IoT enabled system would trigger an alarm, necessary for isolation of COVID subjects.
Chest X-Ray Images were collected from North Eastern Indira Gandhi Regional Institute of Health and Medical Sciences, India. The images obtained are first normalized and re-sized into [224 X 224] and shuffled, and placed under the training & test sets, respectively. To address the class imbalance issue, the authors had performed a data augmentation technique for both the radiology and pathology samples. For the radiology set of images they used orientation related transformation technique viz-a-viz rotation, reflection, translation etc. to augment the present data samples. An identical technique is performed to augment the minority data in pathological type. The technique used is SMOTE (Synthetic Minority Oversampling Technique) [38]. The training set has pre-defined labels whereas the test set has no pre-defined labels associated. The number of images and their respective classes as divided under the training and test sections is given in Table 1.
Label | Training Images | Test Images |
COVID | 1030 | 510 |
NORMAL | 2080 | 220 |
The distribution of the patients of Indian origin only is shown in the following Figure 2.
The other pre-processing technique which the authors have applied to the training data is an augmentation technique. The different augmentation methods used are rotation, reflection, and shear as discussed earlier. The augmentation technique is especially important since the developed model would be used where the images would be captured from different X-ray machines.
Model: Optimizable Tree | Optimized Hyperparameters | Optimizer Options | |||
Surrogate Decision Splits | OFF | Max No of Splits | 73 | Bayesian Optimization | |
Accuracy | 82% | Training Time | 300 S | ||
Total Misclassification Cost | 101 | Iterations | 30 | ||
Prediction Speed | ~17000 observations/sec | Split | Max Deviance Reduction | Training Time | TRUE |
Training Time | 58.164 sec |
This mathematical model for COVID detection is based on machine learning and a deep learning-driven algorithm. The machine learning algorithm takes pathological data as its input feed to identify the presence of COVID. The data set contained age, gender, leukocyte, lymphocyte & neutrophil count as parameters. The data was trained using the Classification Learner App in MATLAB, so the model with the highest accuracy could be achieved. The details of the trained model along with the different performance evaluation parameters are provided in Table 2 for better understanding.
The other important aspect of this paper emphasizes the power of a combined hypothesis, based on the pathological study as explained above, and AI-enabled radiography assisted technique for COVID detection. This AI-enabled method is powered by a powerful multi-layer convolutional neural network, also known as a deep neural network in short. Many pre-trained networks are available, but the authors have chosen the networks which had been successfully used in the field of medical imaging and related works [39]. The authors have compared the performances of different network architectures for the same dataset and the result is given in Figure 3.
So, the deep network used by the authors is ResNet50 for further analysis for its best performance and wide use in this field among others. For this research the authors have used pre-trained ResNet50 DNN. The ResNet50 is a multi-layer Direct Acyclic Graph (DAG) Network, pre-trained to perform 1000 Image Classifications, and has been previously trained on millions of images. The concept of Transfer Learning helps to re-use a pre-trained network as per the requirements by replacing the final few layers. The next important step is to tune the network to achieve the optimum most performance. The above steps are performed to obtain the most optimum model, to be used for classification. ResNet50 architecture along with the residual units, size of the filters and the outputs of each convolutional layer is given in Figure 4. The figure also shows the extraction of deep residual features (DRF) from the last convolutional layer of this network. The representation kXk, n in the convolutional layer block describes a filter of size k and n channels. FC 1000 represents the fully connected layer with 1000 neurons. The number on the top of the convolutional layer block denotes the repetition of each unit. nClasses denotes the number of output classes.
By virtue of transfer learning, the authors have tuned the final hidden layers with respect to our data set. The weights earlier/initial hidden layers remain unaffected. The same approach was adopted since training DNN from the scratch is unrealistic in terms of time which can be in order of months even. Hence this kind of a transfer learning based approach on a pretrained network helps to achieve the desired results within a finite time. The convolutional blocks of a ResNet are different from those of the traditional networks because of the existence of a shortcut connection between the input and output of each block. Identity mappings when used as shortcut connections in ResNets [40], can provide better optimization and reduced complexity of analysis. This also allows one to use deeper ResNets for its faster for training and computationally less expensive behaviour than the conventional networks i.e., VGGnet. The objective of this part is to distinguish between “COVID” class, and “NORMAL” class subjects based on radiography images using this model. Furthermore, the authors have tried to classify the different stages of “COVID” based on the CXR images. The same has been achieved by a severity score tag and a stage annotation.
As mentioned earlier input to this system includes CXR images and pathological data. So, the authors have developed two separate models to act on those. A pre-trained ResNet50 DNN based architecture with transfer learning was used for the CXR images. An Optimized decision tree was used for the pathological data set. The individual prediction goes through a decision fusion (probabilistic approach) to make the final detection. Once this step is accomplished, the rest of the pipeline of our workflow is applicable only for COVID affected individuals. As the first step, they have trained a 24-layer series DNN to predict ground glass opacity (GGO) and lung consolidation values.
Once the correct identification of COVID is achieved, the next algorithm classifies the stage of COVID based on a COVID score. The COVID score is determined by the combined effect of opacity and consolidation score, which are used to characterize the condition of the lungs. To determine the opacity & consolidation scores, the authors have proposed a deep network-enabled layer graph-based regression model. This proposed model predicts the opacity and consolidation score to determine the severity of the disease. This 24 layered deep network with the last layer conforming to a regression node, is used to calculate the respective severity scores. Based on this severity score, the authors have proposed a rule-based mathematical model to correctly determine the stage of COVID. The rule-based mathematical model is developed on a MAMDANI based fuzzy inference system (FIS) [41]. Even for medical experts, diagnosing certain disorders is tough. Data mining was invented to solve this issue. It finds information in a database. Data mining has several subfields. Fuzzy logic is a subfield. It is used in various domains including control theory, AI, and medicine. Gayathri et al. used fuzzy logic to predict the risk of breast cancer [42]. The goal of this suggested work is to diagnose breast cancer faster by decreasing the factors. The features were retrieved using Linear Discriminant Analysis (LDA) and trained using the Mamdani Fuzzy Inference Model (MFIM). With the use of cardiovascular risk factors, A study intends to provide a medical diagnostic support system for early detection of heart disorders. This clinical data-based fuzzy cardiovascular diagnosis is validated with sensitivity and specificity [43].
The authors have developed the final module of the COVID-19 Detection and Isolation system based on the above studies. Based on the evaluated Ground Glass Opacities and Lung Consolidation Values in the previous step, a Fuzzy Inference System was developed by the authors to predict the final COVID score. The Final COVID Score led to the final categorization of stages for COVID affected individuals namely Mild, Severe, Critical. The authors have used such a model due to its popularity for medical diagnostic purposes. The fuzzy logic is a rule-based method used for classification without any learning. The methodology works around the concept of values and is very much applicable where the decision is based on the values of distribution from two or more variables as input and there could be one or more output classes. Each input or output class can be defined by a set of membership functions to determine the degree of belongingness to a group.
The authors have classified the stages of COVID as mild, severe, and critical, respectively. The basic symptoms associated with the defined stages are as follows-
(1) Mild: no symptoms, mild coughing, and fever.
(2) Severe: dyspnea, hypoxia.
(3) Critical: respiratory failure, shock, multi-organ failure.
In this case, the input classes are opacity & consolidation scores. The two input classes are individually defined by three triangular membership functions, respectively. The output class is the COVID Score defined by three triangular membership functions conforming to ‘Mild’, ‘Severe’ & ‘Critical’ Classes.
In respect to the above symptoms, the lung condition can be explained in the following manner. In addition to this radiography classification problem, the novelty that the authors added in this method is to classify the set of COVID Images as per their stages. The metric used to determine the correct stage are Ground Glass Opacity (GGO) & Lung Consolidation. GGO is a hazy increased opacity in lung parenchyma without the obscuration of the underlying vessels. At the initial stage, the virus invades the alveolar epithelium, and replicates in the epithelial cells, causing the alveolar cavity to leak, and the alveolar wall or the alveolar space to become inflamed or thickened [44],[45]. The consolidation is the increased lung opacification with the obscuration of the underlying vessels. As inflammation progresses, the body reacts and a strong inflammatory reaction that results in large exudation in the alveoli.
The airspace opacities like ground-glass opacities and consolidations are the most frequent findings in COVID, often bilateral peripheral distribution particularly at the lower zone. The central parenchymal abnormalities, pleural involvement is rare (3%) [46]–[48]. The severity score is based on the involvement of bilateral bronchopulmonary segments [49]. The 18 segments of both lungs were divided into 20 regions. Each of the segment involvement carries scores of 0, 1, and 2 if parenchymal opacification involved 0%, less than 50%, or equal or more than 50% of each region. The optimal score of 19.5 is identified as severe COVID-19 with 83.3% sensitivity and 94% specificity.
Any decision fusion algorithm plays a crucial role in the multi-hypotheses situation and hence can be seamlessly used in clinical diagnostic procedures as well. The concept of multi-hypotheses will act as a valuable second opinion to doctors and hence will be an enabler for any diagnostic-based approach. The developed system involves data fusion for individual predictions conforming to different data. These individual predictions go through a decision fusion system or algorithm for the final prediction. A smart medical system based on the Internet of Things can be defined as a technological means of transmitting remote physiological data and medical signals to a monitoring centre for analysis and diagnosis over a communication network [50]. Generally, the system consists of three components: a monitoring centre, medical sensors, and a server-based data fusion algorithm. Data fusion is an efficient method for making the best use of enormous amounts of data from many sources. Multi-sensor data fusion aims to combine data from numerous sensors and sources in order to make inferences that would be impossible with a single sensor or source [51]. On one side, the medical IoT allows for the effective capture and integration of medical information, allowing for the promotion of health. Data fusion is a vital component of the medical IoT. It cannot avoid enormous multisource diverse data integration and data exchange challenges. In the network, audio, video, data and text are all captured. Personal health promotion reports are created and various tracking interventions are undertaken after intelligent processing of acquired data [52]. Moreover, the network involves many spatiotemporal data, such as health data geographical distribution, medical data, physical findings data, health monitoring and evaluation, equipment operation, and factors. They are dispersed in heterogeneous systems with varying data specification standards and analysis methods. Because of this, it must employ data fusion methods to combine medical and health resources. Thereby boosting the total worth of people's health assets [53]. Numerous medical Internet of things technology studies have been carried out abroad [54]. A data fusion tree is designed to improve data fusion efficiency and illustrate the technology's status.
To elaborate the first of the two sub diagnostic measures in this screening processes is based on pathological data of subjects. In the second screening step, the authors have proposed the idea of combining the conventional radiography-based classification with a severity score tag to help the doctors to identify any presence of strain more correctly with an annotated stage.
Since the authors have created a dual pool hypothesis scenario from two different algorithms hence, the authors are proposing an algorithm “Whoever Scores Most” in terms of a probabilistic metric to correctly recognize the affected subject concerned in decision conflict scenarios. The algorithm finally scans through the sets of inferences and finally returns the output of the class which has a higher probability score. The equation of the methodology is described below in Equation 1. Let say X represents the single output given two different output from different algorithms for all output classes.
C1 represents the output class predicted by the machine learning algorithm for pathological data.
S1 represents the probability score for class C1.
C2 represents the output class predicted by the deep learning model for radiographic images.
S2 represents the probability score for output class C2.
To make this composite system available to the diagnostic facilities, the authors have used the concept of the internet of things (IoT), a technology extremely popular in telemediation. Internet of Things (IoT) has been a trusted friend of various medical facilities ever since its inception. The concept of telemedicine or remote patient monitoring system has evolved in many ways with the use of IoT based devices. The authors have used the concept of IoT to complement this AI-based patient diagnostic system to increase reliability and performance. The Intel® Movidius™ Neural Compute Stick (NCS) is a new piece of hardware used for enhancing the inference process of computer vision models on low-powered edge devices. The Intel Movidius™ product is a USB appliance that can be plugged into any device such as laptops, Raspberry Pi, or even tablets. The authors have deployed the trained models into the Intel Movidius™ Neural Compute Stick for a classification of COVID affected subjects. The power of the device lies in running parallel ML models, hence useful in the multi-hypothesis scenario. The device is chosen by the authors for its popularity in different medical imaging activities by other peer groups.
These models were initially designed and trained on a capable host machine (a development computer in this case). Then one of the supported Application Programming Interface (API) was used to profile, tune, and compile to convert the model to the format supported by the VPU hardware. With the newly formatted model, the model was validated, and the working prototype was generated on the same host machine. The hardware is physically connected to the machine and was accessed using available methods in the API. The development process using NCS is explained in Figure 5.
The philosophy of the AI software architecture to be used for deployment is described in Figure 6.
The steps followed for the inference on the edge are explained in Figure 7.
The basic steps for drawing inference from the developed models are as follows.
The authors have proposed a network architecture for making the medical facility an IoT enabled unit. The same is being proposed for faster detection and isolation of COVID-19 subjects. The network architecture is prototyped by an IoT enabled alarm system using a Raspberry Pi device. The Raspberry Pi is virtually connected with the server so that for any COVID-19 positive subject, the server can trigger specific actions on the IoT network for communicating with the Raspberry Pi device. The results that are captured from the classification of images in the server are sent to the Raspberry Pi device where the actions like turning on a red LED and a buzzer when COVID-19 is detected and turn on a blue LED when the classification results when “Normal” being detected have been programmed and activated. This is an amazingly simple Proof of Concept (POC) which shows a possibility of its powerful applications that can save time for medical staff and could help save lives through early and accurate detection. A flow diagram of the entire value chain of this intelligent framework is given in Figure 8.
The different performance evaluation criteria were used to validate the mathematical model based on pathological data is given in Figure 9 and Figure 10.
The model evaluation parameters have been given in Table 3.
Evaluation Parameter | Score |
Sensitivity (COVID) | 95.89% |
Specificity (Normal) | 0.0% |
Specificity (COVID) | 98.72% |
The area under the curve (AUC) of ROC | 0.77 |
Misclassification Error | 0.18 |
From Table 3, the authors have deduced that the two main factors that play a pivotal role in selecting the correct model are sensitivity & specificity. In simple terms and as an explanation for the above observations the authors find that “COVID” (Target Class) detected as “COVID” (Output Class) defines the sensitivity. It is observed that the sensitivity test for “COVID” has a higher accuracy return. In addition to it, the false negatives concerning the candidate being predicted as “Normal” are ‘0’. The other false negative conforming to the pneumonia aspect is of little importance since the same requires addressable medical measures.
The other part analogous to sensitivity is specificity. The authors have defined the same in two ways. One is defined by “Normal” (Target Class) detected as “Normal” (Output Class). The authors find that the sensor data are not overly specific in nature. The “Normal” detected as “COVID” (‘0’ in number) is important but not to a greater extent since they do not risk spreading the disease. The number of false positives may bring the accuracy of the model down but from medical criticality, it is of low severity over the false-negative aspect.
The basic step that the authors have employed for the implementation of the AI-based radiography assisted technique is the concept of transfer learning. The concept is useful when the output classes do not match the classes in the pre-trained model. It also helps in the reduction of training time and does not require the model to be developed from scratch. The entire flowchart of the process is explained in Figure 11.
The following figures elaborately illustrate the method to choose the optimum model. The evaluation criterion that the authors have kept is the confusion matrix & validation accuracy.
To choose the right methodology for data splitting, the authors have directly compared the hold-out & one of the cross-validation training data and its performance on a new test set which was not a part of the training or validation samples. The test data was also previously labelled. The comparison between k-fold and hold-out for model validation is given in Table 4.
Validation Type | Evaluation Parameter | Scores in % |
K-fold | Sensitivity | 98.0 |
Specificity | 22.7 | |
Precision | 74.6 | |
Accuracy | 75.3 | |
Hold out | Sensitivity | 92.2 |
Specificity | 22.7 | |
Precision | 73.4 | |
Accuracy | 71.2 |
To obtain the right model, the authors have framed the design methodology in the following manner:
(1) For the first type, the authors have divided the model in training & hold-out sets in the split percentage of 80 to 20. the authors have tuned the hyperparameters as well to make the most out of this method.
(2) In the second type, the authors have used the K-fold cross-validation concept, to reduce the misclassification error and increase the model accuracy in return. The k-fold validation shuffles the training set randomly & iteratively and deploys K different algorithms to automatically return 5 models which can individually be evaluated on some performance criteria to obtain the best out of the lot. the authors have chosen the value of K as ‘5’ in this case.
Hence in this case, the authors find that the deep network model for K-fold validation exhibits higher accuracy in terms of detecting COVID for the test set. The other part analogous to it is Specificity being defined by “Normal” (target class) detected as “Normal” (output class). the authors find that the radiography images are overly sensitive in nature and hence can be the ideal tool for detecting these kinds of cases. The other two important factors which help us to know the right model are false negatives & false positives. “COVID” Detected as “Normal” accounts for the false-negative part. The K-fold validation model again stands out for this case as well. The “Normal” detected as COVID is important but not to a greater extent since they do not risk spreading the disease. The precision rate then also is observed to be under reasonable limits of around 74.3%.
Similarly, the immediate next objective was to choose the correct K-fold Validation model from the 5 Models as returned by the proposed methodology. The comparison table for the selection of the right model based on a performance evaluation metric is given below in Table 5.
Different K Fold ResNet50 Models | Evaluation Parameter | Scores in % |
K = 1 type | Sensitivity | 98.0 |
Specificity | 22.7 | |
Precision | 74.6 | |
Accuracy | 75.3 | |
K = 2 type | Sensitivity | 82.4 |
Specificity | 31.8 | |
Precision | 73.7 | |
Accuracy | 67.1 | |
K = 3 type | Sensitivity | 82.4 |
Specificity | 18.2 | |
Precision | 70.0 | |
Accuracy | 63.9 | |
K = 4 type | Sensitivity | 84.3 |
Specificity | 45.5 | |
Precision | 78.2 | |
Accuracy | 72.6 | |
K = 5 type | Sensitivity | 82.4 |
Specificity | 18.2 | |
Precision | 70.0 | |
Accuracy | 63.0 |
The indicative inference that the authors may draw from the above comparison chart is as follows:
(1) ResNet with K= 1 Deep Network has outperformed the other models in terms of overall accuracy (75.3%). The sensitivity rate (~ 98%) is also higher in the case of ResNet-1. The false-negative rate (~2%) is also within acceptable limits. The only drawback of the model lies in the number of false positives & hence the specificity as well. A little compromise on this point is still acceptable since the true objective of the model is met. In line with this, the precision rate is hence considered to be under reasonable limits around 74.6%.
The proposed multi-hypothesis based model was assessed using the test data and shown in Figure 12. The COVID-19 class has an overall accuracy of 99.31 percent. Precision 99.80 percent, specificity 99.54 percent, sensitivity or recall 99.18 percent, and F-1 score 0.99 were demonstrated for the suggested architecture for test dataset. Further validation of the model's performance is accomplished through the use of a indicative visualisation technique called Class Activation Mapping (CAM). Using a gradient map or score, this method enables us to visualise the reason behind a classification. The red-colored zones in our example enabled the model to classify the image as belonging to any class. Some sample observations/ classifications on images along with the CAM zones are given in the following Figure 13.
One of the novelties of the proposed methodology lies in the calculation of the opacity & consolidation to identify the severity of the disease. The data set was split into training and hold-out validation set for model development.
The deep neural network-based regression learning algorithm that the authors have selected is done iteratively. the authors had initially developed two machine learning model based on statistical features from the Regression Learner App and then compared the same with the deep learning. Needless to mention that the deep learning model for calculation of opacity & consolidation outperformed the machine learning model.
The machine learning model was developed on Statistical Features like skewness, kurtosis, variance, smoothness, energy, entropy, homogeneity, contrast, etc. The model was obtained by training on these features. The machine learning models obtained for both consolidation & opacity are of compact regression tree type where ‘Bayesian Optimization’ was employed to get the best model from the entire set. The root means square error (RMSE) obtained for both opacity and consolidation are 1.5023 and 2.4975, respectively. The deep network that the authors have proposed to perform such regression related tasks was compared to the machine learning models and the observations are framed below:
(1) Firstly, the deep network for both opacity & consolidation has a considerable low RMSE of around 1.7416 and 2.1394, respectively.
(2) The severity degree for both opacity & consolidation is given in terms of a score. Based on the score, the criticality condition for the subject is determined.
The fuzzy inference system, rules, and surface viewer to visualize the system architecture, the output decision on different input set & the output score distribution is defined as follows in Figure 14, 15 and 16. The detailed mathematics corresponding to the membership functions and fuzzy rules, which can be found in the refereed research article [43].
Image Name | Opacity | Consolidation | COVID Score | Class |
Image 1 | 49 | 45 | 43 | Severity |
Image 2 | 48 | 45 | 43 | Severity |
Image 3 | 58 | 47 | 80 | Critical |
Image 4 | 30 | 46 | 43 | Severity |
Image 5 | 37 | 35 | 42 | Severity |
The observations related to the severity scores (in %) with respective classes or COVID stages related to different X-Rays and the two model comparisons are given in Table 6. The observations as tabulated the authors find that how the deep learning model outperforms the machine learning one. The class prediction is done using the fuzzy inference system as explained in the previous part and explained in Table 7 and Figure 17.
Image | Actual |
Machine Learning Predicted |
Deep Learning Predicted |
Results | |||
Opacity | Consolidation | Opacity | Consolidation | Opacity | Consolidation | ||
Image 1 | 55 | 50 | 37 | 34 | 49 | 45 | Severe |
Image 2 | 50 | 50 | 37 | 35 | 48 | 45 | Severe |
Image 3 | 34 | 62 | 37 | 37 | 37 | 58 | Critical |
Image 4 | 54 | 47 | 35 | 35 | 47 | 46 | Severe |
Image 5 | 29 | 34 | 35 | 37 | 35 | 30 | Severe |
The suggested model's validation analysis is depicted in Figure 18. The proposed framework outperforms existing deep learning models, according to the results. The suggested framework surpasses existing models in terms of sensitivity, area under the curve (AUC), specificity, F-measure, and accuracy.
Moreover, from existing literature survey on CXR images, FRCR has demonstrated a 98 percent accuracy rate. The classification accuracy of the DL-CRC was 93.94 percent. 3DCNN had an accuracy of 87.5 percent, a sensitivity of 86.9 percent, a specificity of 90.1 percent, and a sensitivity of 86.9 percent. CheXNet attained a classification accuracy of 97.9 percent, a specificity of 97.9 percent, and a sensitivity of 98.8 percent, respectively. Another architecture, VGG19, achieved a sensitivity of 93 percent in total. CovidGAN had a 95 percent accuracy rate. The categorization accuracy of the ACCA was 91.1 percent. AD3D-MIL had a classification accuracy of 97.9 percent, an AUC of 99 percent, and a kappa score of 95.7 percent. As a result, the developed framework beats these methodologies, with an accuracy of 99.31 percent and an F-measure of 0.99. Additionally, the suggested model obtains sensitivity, specificity, and AUC values of 99.18 percent, 99.54 percent, and 0.9921, respectively. Thus, when compared to existing coronavirus diagnosis methods, the proposed model exhibits a better degree of performance reliability.
Our present set-up is a deployed solution based on Intel® Neural Compute stick and Intel® AI Dev cloud. The presence of cloud is of utmost importance in any IoT ecosystem-based implementation. We mainly address two objectives-(i) Raising an alarm inside the point of care facility to isolate COVID affected individuals, (ii) Requirement for hospitalization. The present set-up is piloted using Intel® AI dev cloud which is a free cloud. No other free cloud can give us the benefits in comparison to this one. The commercial versions are expensive to be used for research purpose.
The reasons we have chosen Intel AI computing stick as our embedded deployment option are as follows, the Intel® neural Compute stick platform provides reduced computational and time complexity when compared with the Raspberry pi 4 GB module, NVIDIA Jetson Nano and NVIDIA Xavier CUDA. The Figure 19 and 20 elucidates the learning time and prediction time complexity in different Single-Board Computer (SBC) platforms.
This paper for the first time reports the development of an AI enabled smart software framework based on IoT for the stage specific detection of COVID-19 patients. The solution pipeline as discussed here is a more composite and robust one since it includes a two-step screening process. The algorithm is developed into a deployable form and hence would act as an aid to the health practitioners & other medical staffs that can provide a valuable second opinion in the existing diagnostic process. The state-of-the-art system is currently deployed in an intel-based computing platform and uses intel AI dev cloud (free). This algorithm is not intended to replace the more acceptable PCR process but act as an aid to the same. In addition to being an aid, it is also a highly cost-effective solution for the Prediction of COVID-19 Cases. The developed system has been verified through a large-scale clinical testing as well. The PCR-test is specific but has a lower sensitivity of 65–95%, which means that the test can be negative even when the patient is infected. The duration of such an examination is also long. The reported method is a more effective one economically and technically as well. As part of the future scope a stand-alone application with only the models can be integrated with enterprise systems such as Hospital Information system for healthcare institutes, radiology information system and laboratory information systems for diagnostic centers or point of care facilities.
[1] |
Sun T, Wang Y (2020) Modeling COVID-19 epidemic in Heilongjiang province, China. Chaos, Soliton Fract 138: 109949. doi: 10.1016/j.chaos.2020.109949
![]() |
[2] |
Melin P, Castillo O (2021) Spatial and temporal spread of the COVID-19 pandemic using self organizing neural networks and a fuzzy fractal approach. Sustainability 13: 8295. doi: 10.3390/su13158295
![]() |
[3] |
Castillo O, Melin P (2021) A novel method for a covid-19 classification of countries based on an intelligent fuzzy fractal approach. Healthcare 9: 196. doi: 10.3390/healthcare9020196
![]() |
[4] |
Castillo O, Melin P (2020) Forecasting of COVID-19 time series for countries in the world based on a hybrid approach combining the fractal dimension and fuzzy logic. Chaos, Soliton Fract 140: 110242. doi: 10.1016/j.chaos.2020.110242
![]() |
[5] |
Boccaletti S, Ditto W, Mindlin G, et al. (2020) Modeling and forecasting of epidemic spreading: The case of Covid-19 and beyond. Chaos, Soliton Fract 135: 109794. doi: 10.1016/j.chaos.2020.109794
![]() |
[6] | Wang W, Xu Y, Gao R, et al. (2020) Detection of SARS-CoV-2 in different types of clinical specimens. Jama 323: 1843-1844. |
[7] | West CP, Montori VM, Sampathkumar P (2020) COVID-19 testing: the threat of false-negative results. Elsevier 95: 1127-1129. |
[8] |
Fang Y, Zhang H, Xie J, et al. (2020) Sensitivity of chest CT for COVID-19: comparison to RT-PCR. Radiology 296: E115-E117. doi: 10.1148/radiol.2020200432
![]() |
[9] |
Ng M-Y, Lee EY, Yang J, et al. (2020) Imaging profile of the COVID-19 infection: radiologic findings and literature review. Radiolo: Cardiothorac Imag 2: e200034. doi: 10.1148/ryct.2020200034
![]() |
[10] |
Huang C, Wang Y, Li X, et al. (2020) Clinical features of patients infected with 2019 novel coronavirus in Wuhan, China. The lancet 395: 497-506. doi: 10.1016/S0140-6736(20)30183-5
![]() |
[11] |
Guan WJ, Ni ZY, Hu Y, et al. (2020) Clinical characteristics of coronavirus disease 2019 in China. New Engl J Med 382: 1708-1720. doi: 10.1056/NEJMoa2002032
![]() |
[12] |
Ai T, Yang Z, Hou H, et al. (2020) Correlation of chest CT and RT-PCR testing for coronavirus disease 2019 (COVID-19) in China: a report of 1014 cases. Radiology 296: E32-E40. doi: 10.1148/radiol.2020200642
![]() |
[13] |
Khatami F, Saatchi M, Zadeh SST, et al. (2020) A meta-analysis of accuracy and sensitivity of chest CT and RT-PCR in COVID-19 diagnosis. Sci Rep 10: 22402. doi: 10.1038/s41598-020-80061-2
![]() |
[14] |
Nair A, Rodrigues JCL, Hare S, et al. (2020) A British society of thoracic imaging statement: considerations in designing local imaging diagnostic algorithms for the COVID-19 pandemic. Clin Radiol 75: 329-334. doi: 10.1016/j.crad.2020.03.008
![]() |
[15] |
Jacobi A, Chung M, Bernheim A, et al. (2020) Portable chest X-ray in coronavirus disease-19 (COVID-19): A pictorial review. Clin Imag 64: 35-42. doi: 10.1016/j.clinimag.2020.04.001
![]() |
[16] | LeCun Y, Bengio Y, Hinton G (2015) Deep learning. Nature . |
[17] | Gozes O, Frid-Adar M, Greenspan H, et al. Rapid ai development cycle for the coronavirus (covid-19) pandemic: Initial results for automated detection & patient monitoring using deep learning ct image analysis (2020) .arXiv preprint arXiv:2003.05037. |
[18] | Lessmann N, Sánchez CI, Beenen L, et al. (2020) Automated assessment of CO-RADS and chest CT severity scores in patients with suspected COVID-19 using artificial intelligence. Radiology . |
[19] | Li L, Qin L, Xu Z, et al. (2020) Artificial intelligence distinguishes COVID-19 from community acquired pneumonia on chest CT. Radiology . |
[20] |
Shi F, Xia L, Shan F, et al. (2021) Large-scale screening to distinguish between COVID-19 and community-acquired pneumonia using infection size-aware classification. Phys Med Biol 66: 065031. doi: 10.1088/1361-6560/abe838
![]() |
[21] | Magree H, Russell F, Sa'Aga R, et al. (2005) Chest X-ray-confirmed pneumonia in children in Fiji. B World Health Organ 83: 427-433. |
[22] |
Wong HYF, Lam HYS, Fong AHT, et al. (2020) Frequency and distribution of chest radiographic findings in patients positive for COVID-19. Radiology 296: E72-E78. doi: 10.1148/radiol.2020201160
![]() |
[23] |
Borghesi A, Maroldi R (2020) COVID-19 outbreak in Italy: experimental chest X-ray scoring system for quantifying and monitoring disease progression. La radiologia medica 125: 509-513. doi: 10.1007/s11547-020-01200-3
![]() |
[24] | Wang X, Peng Y, Lu L, et al. (2017) Chestx-ray8: Hospital-scale chest x-ray database and benchmarks on weakly-supervised classification and localization of common thorax diseases. Proceedings of the IEEE conference on computer vision and pattern recognition 2097-2106. |
[25] | Rajpurkar P, Irvin J, Zhu K, et al. Chexnet: Radiologist-level pneumonia detection on chest x-rays with deep learning (2017) .arXiv preprint arXiv:1711.05225. |
[26] |
Wang H, Jia H, Lu L, et al. (2019) Thorax-Net: An attention regularized deep neural network for classification of thoracic diseases on chest radiography. IEEE J Biomed Health 24: 475-485. doi: 10.1109/JBHI.2019.2928369
![]() |
[27] |
Rajaraman S, Candemir S, Kim I, et al. (2018) Visualization and interpretation of convolutional neural network predictions in detecting pneumonia in pediatric chest radiographs. Appl Sci 8: 1715. doi: 10.3390/app8101715
![]() |
[28] |
Kermany DS, Goldbaum M, Cai W, et al. (2018) Identifying medical diagnoses and treatable diseases by image-based deep learning. Cell 172: 1122-1131. doi: 10.1016/j.cell.2018.02.010
![]() |
[29] |
Horry MJ, Chakraborty S, Paul M, et al. (2020) COVID-19 detection through transfer learning using multimodal imaging data. IEEE Access 8: 149808-149824. doi: 10.1109/ACCESS.2020.3016780
![]() |
[30] | Ahmed I, Ahmad A, Jeon G (2020) An iot based deep learning framework for early assessment of covid-19. IEEE Internet Things J . |
[31] |
Chowdhury MEH, Rahman T, Khandakar A, et al. (2020) Can AI help in screening viral and COVID-19 pneumonia? IEEE Access 8: 132665-132676. doi: 10.1109/ACCESS.2020.3010287
![]() |
[32] |
Han Z, Wei B, Hong Y, et al. (2020) Accurate screening of COVID-19 using attention-based deep 3D multiple instance learning. IEEE T Med Imaging 39: 2584-2594. doi: 10.1109/TMI.2020.2996256
![]() |
[33] |
Qian X, Fu H, Shi W, et al. (2020) M Lung-Sys: A deep learning system for multi-class lung pneumonia screening from CT imaging. IEEE J Biomed Health 24: 3539-3550. doi: 10.1109/JBHI.2020.3030853
![]() |
[34] |
Sakib S, Tazrin T, Fouda MM, et al. (2020) DL-CRC: deep learning-based chest radiograph classification for COVID-19 detection: a novel approach. IEEE Access 8: 171575-171589. doi: 10.1109/ACCESS.2020.3025010
![]() |
[35] |
Waheed A, Goyal M, Gupta D, et al. (2020) Covidgan: data augmentation using auxiliary classifier gan for improved covid-19 detection. Ieee Access 8: 91916-91923. doi: 10.1109/ACCESS.2020.2994762
![]() |
[36] |
Varela-Santos S, Melin P (2021) A new approach for classifying coronavirus COVID-19 based on its manifestation on chest X-rays using texture features and neural networks. Inform Sci 545: 403-414. doi: 10.1016/j.ins.2020.09.041
![]() |
[37] |
Brihn A, Chang J, OYong K, et al. (2021) Diagnostic performance of an antigen test with RT-PCR for the detection of SARS-CoV-2 in a hospital setting—Los Angeles county, California, June–August 2020. Morbid Mortal W Rep 70: 702. doi: 10.15585/mmwr.mm7019a3
![]() |
[38] |
Chawla NV, Bowyer KW, Hall LO, et al. (2002) SMOTE: synthetic minority over-sampling technique. J Artif Intell Res 16: 321-357. doi: 10.1613/jair.953
![]() |
[39] |
Hassan M, Ali S, Alquhayz H, et al. (2020) Developing intelligent medical image modality classification system using deep transfer learning and LDA. Sci Rep 10: 1-14. doi: 10.1038/s41598-019-56847-4
![]() |
[40] | He K, Zhang X, Ren S, et al. (2016) Identity mappings in deep residual networks Springer, 630-645. |
[41] |
Prakash C, Rajkumar S, Mouli PC (2012) Medical image fusion based on redundancy DWT and Mamdani type min-sum mean-of-max techniques with quantitative analysis. 2012 International conference on recent advances in computing and software systems IEEE, 54-59. doi: 10.1109/RACSS.2012.6212697
![]() |
[42] | Gayathri BM, Sumathi CP (2015) Mamdani fuzzy inference system for breast cancer risk detection. 2015 IEEE International Conference on Computational Intelligence and Computing Research (ICCIC) IEEE, 1-6. |
[43] | Terrada O, Raihani A, Bouattane O, et al. (2018) Fuzzy cardiovascular diagnosis system using clinical data. 2018 4th International Conference on Optimization and Applications (ICOA) IEEE, 1-4. |
[44] |
Yang W, Cao Q, Qin LE, et al. (2020) Clinical characteristics and imaging manifestations of the 2019 novel coronavirus disease (COVID-19): a multi-center study in Wenzhou city, Zhejiang, China. J Infection 80: 388-393. doi: 10.1016/j.jinf.2020.02.016
![]() |
[45] |
Yoon SH, Lee KH, Kim JY, et al. (2020) Chest radiographic and CT findings of the 2019 novel coronavirus disease (COVID-19): analysis of nine patients treated in Korea. Korean J Radiol 21: 494-500. doi: 10.3348/kjr.2020.0132
![]() |
[46] |
Rodrigues JCL, Hare SS, Edey A, et al. (2020) An update on COVID-19 for the radiologist-A British society of thoracic imaging statement. Clin Radiol 75: 323-325. doi: 10.1016/j.crad.2020.03.003
![]() |
[47] |
Ludvigsson JF (2020) Systematic review of COVID-19 in children shows milder cases and a better prognosis than adults. Acta Paediatr 109: 1088-1095. doi: 10.1111/apa.15270
![]() |
[48] |
Holshue ML, DeBolt C, Lindquist S, et al. (2020) First case of 2019 novel coronavirus in the United States. N Engl J Med 382: 929-936. doi: 10.1056/NEJMoa2001191
![]() |
[49] |
Yang R, Li X, Liu H, et al. (2020) Chest CT severity score: an imaging tool for assessing severe COVID-19. Radiol: Cardiothorac Imag 2: e200047. doi: 10.1148/ryct.2020200047
![]() |
[50] |
Zhang W, Thurow K, Stoll R (2014) A knowledge-based telemonitoring platform for application in remote healthcare. Int J Comput Commun 9: 644-654. doi: 10.15837/ijccc.2014.5.661
![]() |
[51] |
Dong J, Zhuang D, Huang Y, et al. (2009) Advances in multi-sensor data fusion: Algorithms and applications. Sensors 9: 7771-7784. doi: 10.3390/s91007771
![]() |
[52] |
Gevaert CM, García-Haro FJ (2015) A comparison of STARFM and an unmixing-based algorithm for Landsat and MODIS data fusion. Remote Sens Environ 156: 34-44. doi: 10.1016/j.rse.2014.09.012
![]() |
[53] |
Fourati H (2014) Heterogeneous data fusion algorithm for pedestrian navigation via foot-mounted inertial measurement unit and complementary filter. IEEE T Instrum Meas 64: 221-229. doi: 10.1109/TIM.2014.2335912
![]() |
[54] |
Ambühl L, Menendez M (2016) Data fusion algorithm for macroscopic fundamental diagram estimation. Transport Res Part C: Emer Technol 71: 184-197. doi: 10.1016/j.trc.2016.07.013
![]() |
1. | Anastasia-Maria Leventi-Peetz, Kai Weber, Probabilistic machine learning for breast cancer classification, 2022, 20, 1551-0018, 624, 10.3934/mbe.2023029 | |
2. | Swarnava Biswas, Saikat Adhikari, Riddhi Chawla, Niladri Maiti, Dinesh Bhatia, Pranjal Phukan, Moumita Mukherjee, Artificial intelligence enabled non-invasive T-ray imaging technique for early detection of coronavirus infected patients, 2022, 32, 23529148, 101025, 10.1016/j.imu.2022.101025 | |
3. | Kitsakorn Locharoenrat, Impact of the Internet of Medical Things on Artificial Intelligence-enhanced medical imaging systems from 2019 to 2023, 2024, 1368-2199, 1, 10.1080/13682199.2024.2400631 | |
4. | Rashmi Jha, Gaurav Kunwar, 2023, Predicting the Patient’s Severity Using Machine Learning Applied to Lungs MRI Images, 979-8-3503-4696-1, 1, 10.1109/ISCON57294.2023.10111986 |
Label | Training Images | Test Images |
COVID | 1030 | 510 |
NORMAL | 2080 | 220 |
Model: Optimizable Tree | Optimized Hyperparameters | Optimizer Options | |||
Surrogate Decision Splits | OFF | Max No of Splits | 73 | Bayesian Optimization | |
Accuracy | 82% | Training Time | 300 S | ||
Total Misclassification Cost | 101 | Iterations | 30 | ||
Prediction Speed | ~17000 observations/sec | Split | Max Deviance Reduction | Training Time | TRUE |
Training Time | 58.164 sec |
Evaluation Parameter | Score |
Sensitivity (COVID) | 95.89% |
Specificity (Normal) | 0.0% |
Specificity (COVID) | 98.72% |
The area under the curve (AUC) of ROC | 0.77 |
Misclassification Error | 0.18 |
Validation Type | Evaluation Parameter | Scores in % |
K-fold | Sensitivity | 98.0 |
Specificity | 22.7 | |
Precision | 74.6 | |
Accuracy | 75.3 | |
Hold out | Sensitivity | 92.2 |
Specificity | 22.7 | |
Precision | 73.4 | |
Accuracy | 71.2 |
Different K Fold ResNet50 Models | Evaluation Parameter | Scores in % |
K = 1 type | Sensitivity | 98.0 |
Specificity | 22.7 | |
Precision | 74.6 | |
Accuracy | 75.3 | |
K = 2 type | Sensitivity | 82.4 |
Specificity | 31.8 | |
Precision | 73.7 | |
Accuracy | 67.1 | |
K = 3 type | Sensitivity | 82.4 |
Specificity | 18.2 | |
Precision | 70.0 | |
Accuracy | 63.9 | |
K = 4 type | Sensitivity | 84.3 |
Specificity | 45.5 | |
Precision | 78.2 | |
Accuracy | 72.6 | |
K = 5 type | Sensitivity | 82.4 |
Specificity | 18.2 | |
Precision | 70.0 | |
Accuracy | 63.0 |
Image Name | Opacity | Consolidation | COVID Score | Class |
Image 1 | 49 | 45 | 43 | Severity |
Image 2 | 48 | 45 | 43 | Severity |
Image 3 | 58 | 47 | 80 | Critical |
Image 4 | 30 | 46 | 43 | Severity |
Image 5 | 37 | 35 | 42 | Severity |
Image | Actual |
Machine Learning Predicted |
Deep Learning Predicted |
Results | |||
Opacity | Consolidation | Opacity | Consolidation | Opacity | Consolidation | ||
Image 1 | 55 | 50 | 37 | 34 | 49 | 45 | Severe |
Image 2 | 50 | 50 | 37 | 35 | 48 | 45 | Severe |
Image 3 | 34 | 62 | 37 | 37 | 37 | 58 | Critical |
Image 4 | 54 | 47 | 35 | 35 | 47 | 46 | Severe |
Image 5 | 29 | 34 | 35 | 37 | 35 | 30 | Severe |
Label | Training Images | Test Images |
COVID | 1030 | 510 |
NORMAL | 2080 | 220 |
Model: Optimizable Tree | Optimized Hyperparameters | Optimizer Options | |||
Surrogate Decision Splits | OFF | Max No of Splits | 73 | Bayesian Optimization | |
Accuracy | 82% | Training Time | 300 S | ||
Total Misclassification Cost | 101 | Iterations | 30 | ||
Prediction Speed | ~17000 observations/sec | Split | Max Deviance Reduction | Training Time | TRUE |
Training Time | 58.164 sec |
Evaluation Parameter | Score |
Sensitivity (COVID) | 95.89% |
Specificity (Normal) | 0.0% |
Specificity (COVID) | 98.72% |
The area under the curve (AUC) of ROC | 0.77 |
Misclassification Error | 0.18 |
Validation Type | Evaluation Parameter | Scores in % |
K-fold | Sensitivity | 98.0 |
Specificity | 22.7 | |
Precision | 74.6 | |
Accuracy | 75.3 | |
Hold out | Sensitivity | 92.2 |
Specificity | 22.7 | |
Precision | 73.4 | |
Accuracy | 71.2 |
Different K Fold ResNet50 Models | Evaluation Parameter | Scores in % |
K = 1 type | Sensitivity | 98.0 |
Specificity | 22.7 | |
Precision | 74.6 | |
Accuracy | 75.3 | |
K = 2 type | Sensitivity | 82.4 |
Specificity | 31.8 | |
Precision | 73.7 | |
Accuracy | 67.1 | |
K = 3 type | Sensitivity | 82.4 |
Specificity | 18.2 | |
Precision | 70.0 | |
Accuracy | 63.9 | |
K = 4 type | Sensitivity | 84.3 |
Specificity | 45.5 | |
Precision | 78.2 | |
Accuracy | 72.6 | |
K = 5 type | Sensitivity | 82.4 |
Specificity | 18.2 | |
Precision | 70.0 | |
Accuracy | 63.0 |
Image Name | Opacity | Consolidation | COVID Score | Class |
Image 1 | 49 | 45 | 43 | Severity |
Image 2 | 48 | 45 | 43 | Severity |
Image 3 | 58 | 47 | 80 | Critical |
Image 4 | 30 | 46 | 43 | Severity |
Image 5 | 37 | 35 | 42 | Severity |
Image | Actual |
Machine Learning Predicted |
Deep Learning Predicted |
Results | |||
Opacity | Consolidation | Opacity | Consolidation | Opacity | Consolidation | ||
Image 1 | 55 | 50 | 37 | 34 | 49 | 45 | Severe |
Image 2 | 50 | 50 | 37 | 35 | 48 | 45 | Severe |
Image 3 | 34 | 62 | 37 | 37 | 37 | 58 | Critical |
Image 4 | 54 | 47 | 35 | 35 | 47 | 46 | Severe |
Image 5 | 29 | 34 | 35 | 37 | 35 | 30 | Severe |