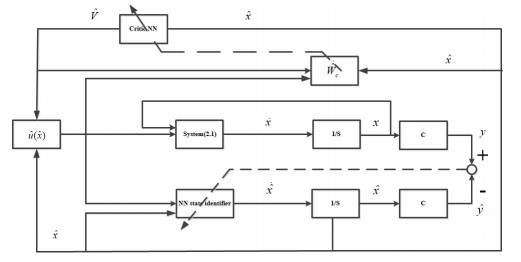
The decrease in soil productivity and quality caused by the continuous and abusive use of mineral fertilizers makes necessary to adopt more sustainable agricultural soil management strategies that help to maintain soil edaphic fertility. In light of these considerations, we have evaluated the effect of organic vs. inorganic fertilization on soil microbial communities, soil quality, and crop yield in a melon crop. The following treatments were tested: i) aerobic sewage sludge from a conventional wastewater treatment plant (WWTP) using aerobic bacteria (SS); ii) aerobic sewage sludge from a WWTP using a bacteria-microalgae consortium (B); iii) N-P-K mineral fertilizer (M); iv) a treatment in which 50% of the N was contributed by SS and 50% by mineral fertilizer (M + SS); v) a treatment in which 50% of the N was contributed by B and 50% by mineral fertilizer (M + B); and vi) a no-fertilized control soil. Melon yield and fruit quality were determined in addition to several soil physical, chemical, biochemical and microbiological parameters. Organic fertilizers (SS and B) increased the percentage of soil water-stable aggregates (52 and 60% respectively) as well as the content of organic C (18 and 31%), water soluble C (21 and 41%), N (15 and 41%) and available P content (41 and 82%) compared to inorganic fertilization. They also stimulated bacterial and fungal abundance to a greater extent than mineral fertilizers (189 and 242% vs 85%, and 57 and 122% vs 29%, respectively), as well as soil respiration, and dehydrogenase, β-glucosidase, phosphatase, urease, and glycine aminopectidase activities. The analysis of principal components with parameters linked to soil quality clearly showed that organic fertilizers cause a greater improvement in soil characteristics and microbial community than mineral fertilizers. Results demonstrate that organic and combined fertilization could be used as substitutes for nitrogen mineral fertilizers in melon crop, since these treatments led to similar melon production and quality while improving soil characteristics and microbial population size and activity.
Citation: Teresa Hernandez, José Guillermo Berlanga, Isabel Tormos, Carlos Garcia. Organic versus inorganic fertilizers: Response of soil properties and crop yield[J]. AIMS Geosciences, 2021, 7(3): 415-439. doi: 10.3934/geosci.2021024
[1] | Yuhang Yao, Jiaxin Yuan, Tao Chen, Xiaole Yang, Hui Yang . Distributed convex optimization of bipartite containment control for high-order nonlinear uncertain multi-agent systems with state constraints. Mathematical Biosciences and Engineering, 2023, 20(9): 17296-17323. doi: 10.3934/mbe.2023770 |
[2] | Zichen Wang, Xin Wang . Fault-tolerant control for nonlinear systems with a dead zone: Reinforcement learning approach. Mathematical Biosciences and Engineering, 2023, 20(4): 6334-6357. doi: 10.3934/mbe.2023274 |
[3] | Vladimir Djordjevic, Hongfeng Tao, Xiaona Song, Shuping He, Weinan Gao, Vladimir Stojanovic . Data-driven control of hydraulic servo actuator: An event-triggered adaptive dynamic programming approach. Mathematical Biosciences and Engineering, 2023, 20(5): 8561-8582. doi: 10.3934/mbe.2023376 |
[4] | Dongxiang Gao, Yujun Zhang, Libing Wu, Sihan Liu . Fixed-time command filtered output feedback control for twin-roll inclined casting system with prescribed performance. Mathematical Biosciences and Engineering, 2024, 21(2): 2282-2301. doi: 10.3934/mbe.2024100 |
[5] | Na Zhang, Jianwei Xia, Tianjiao Liu, Chengyuan Yan, Xiao Wang . Dynamic event-triggered adaptive finite-time consensus control for multi-agent systems with time-varying actuator faults. Mathematical Biosciences and Engineering, 2023, 20(5): 7761-7783. doi: 10.3934/mbe.2023335 |
[6] | Yuhan Su, Shaoping Shen . Adaptive predefined-time prescribed performance control for spacecraft systems. Mathematical Biosciences and Engineering, 2023, 20(3): 5921-5948. doi: 10.3934/mbe.2023256 |
[7] | K. Renee Fister, Jennifer Hughes Donnelly . Immunotherapy: An Optimal Control Theory Approach. Mathematical Biosciences and Engineering, 2005, 2(3): 499-510. doi: 10.3934/mbe.2005.2.499 |
[8] | Tianqi Yu, Lei Liu, Yan-Jun Liu . Observer-based adaptive fuzzy output feedback control for functional constraint systems with dead-zone input. Mathematical Biosciences and Engineering, 2023, 20(2): 2628-2650. doi: 10.3934/mbe.2023123 |
[9] | Siyu Li, Shu Li, Lei Liu . Fuzzy adaptive event-triggered distributed control for a class of nonlinear multi-agent systems. Mathematical Biosciences and Engineering, 2024, 21(1): 474-493. doi: 10.3934/mbe.2024021 |
[10] | Yong Xiong, Lin Pan, Min Xiao, Han Xiao . Motion control and path optimization of intelligent AUV using fuzzy adaptive PID and improved genetic algorithm. Mathematical Biosciences and Engineering, 2023, 20(5): 9208-9245. doi: 10.3934/mbe.2023404 |
The decrease in soil productivity and quality caused by the continuous and abusive use of mineral fertilizers makes necessary to adopt more sustainable agricultural soil management strategies that help to maintain soil edaphic fertility. In light of these considerations, we have evaluated the effect of organic vs. inorganic fertilization on soil microbial communities, soil quality, and crop yield in a melon crop. The following treatments were tested: i) aerobic sewage sludge from a conventional wastewater treatment plant (WWTP) using aerobic bacteria (SS); ii) aerobic sewage sludge from a WWTP using a bacteria-microalgae consortium (B); iii) N-P-K mineral fertilizer (M); iv) a treatment in which 50% of the N was contributed by SS and 50% by mineral fertilizer (M + SS); v) a treatment in which 50% of the N was contributed by B and 50% by mineral fertilizer (M + B); and vi) a no-fertilized control soil. Melon yield and fruit quality were determined in addition to several soil physical, chemical, biochemical and microbiological parameters. Organic fertilizers (SS and B) increased the percentage of soil water-stable aggregates (52 and 60% respectively) as well as the content of organic C (18 and 31%), water soluble C (21 and 41%), N (15 and 41%) and available P content (41 and 82%) compared to inorganic fertilization. They also stimulated bacterial and fungal abundance to a greater extent than mineral fertilizers (189 and 242% vs 85%, and 57 and 122% vs 29%, respectively), as well as soil respiration, and dehydrogenase, β-glucosidase, phosphatase, urease, and glycine aminopectidase activities. The analysis of principal components with parameters linked to soil quality clearly showed that organic fertilizers cause a greater improvement in soil characteristics and microbial community than mineral fertilizers. Results demonstrate that organic and combined fertilization could be used as substitutes for nitrogen mineral fertilizers in melon crop, since these treatments led to similar melon production and quality while improving soil characteristics and microbial population size and activity.
The research on optimal control for nonlinear systems plays a significant role in industry and military fields. Due to the influence of the environment and the limitations of the engineering system, it is very tough to maximize or minimize the performance index of the controlled system in practical. Therefore, optimization problem is a difficult problem in current control field, and has gradually been the focus of attention. The optimal control problems of nonlinear systems are finally transformed into the solutions of Hamilton-Jacobi-Bellman (HJB) partial differential equations.
However, because the HJB equation is a nonlinear partial differential equation, it is difficult to obtain an analytical solution. Therefore, how to obtain the analytical solution of HJB equation then realize the optimization performance index of the system, is the key points to resolve the optimization issue.
In order to avoid the problems encountered in solving HJB equation, Kalman [1] proposed the inverse-optimal-based control method for the first time. On the basis of [1], Freeman and Kokotovic [2] studied inverse optimization control of nonlinear systems. The basic idea of inverse optimization control is not to minimize the cost function by designing the controller, but to minimize the cost function by designing the appropriate control Lyapunov function(CLF). Therefore, the solution of HJB equation comes down to seeking the CLF of the controlled system, thus avoiding the shortcoming of directly solving HJB equation.
Besides, aiming at the above problems, Bellman [3] proposed the theory of dynamic programming (DP). However, the issue of "dimension disaster" would be caused in the process of DP control design, that is, the complexity of space storage and computation increases exponentially with the increase of the dimension of control vectors and states. Therefore, in order to overcome the phenomenon of "dimension disaster" in the process of optimal control design, an adaptive optimal control design method combining NNs is proposed by Werbos [4], which is called RL or adaptive/ approximate dynamic programming (ADP). In the control field, RL can effectively solve the "dimension disaster" problem in DP. In [5], Werbos retrospected the classic econometric approach and proposed a robust method. In [6], Werbos defined a more limited design called "brain-like intelligent control", it discusses the brain as a member of intelligent control, which implies a property to be sought in future research.
Since then, inspired by [4], large amounts of optimal control methods via APD have been developed, see [7,8]. Among them, for continuous-time (CT) systems, in offline situations, Abu-Khalaf and Lewis [9] presented an offline algorithm via RL to solve the optimal control issue of CT nonlinear systems. Since the offline control algorithms cannot be adopted to adjust online in real time, thus, to overcome this disadvantage, Vamvoudak and Lewis [10] proposed an online adaptive method via policy iteration. In [11], Li et al. investigated the Lyapunov stability problem for impulsive systems via event-triggered impulsive control. In [12], Li et al. considerd a class of nonlinear impulsive systems with delayed impulses, based on impulsive control theory and the ideas of average dwell-time (ADT), a set of Lyapunov-based sufficient conditions for globally exponential stability were obtained. However, we need to know the accurate knowledge of CT nonlinear systems in [9,10]. Since nonlinear systems usually contain uncertain nonlinear functions, it it difficult to acquire the analytical solution of the HJB equation.
In order to solve this problem, by choosing an appropriate cost function to reflect uncertainty regulation, the authors in [13] proposed a robust optimization controller design strategy based on an online strategy iterative algorithm for a class of continuous nonlinear systems with nonlinearities. Zhang et al. [14] designed a new data-driven robust identified optimization tracking controller via the acquired data-driven model for a kind of nonlinear CT systems.
Consider a class of affine nonlinear system as:
˙x(t)=g(x(t))u(t)+f(x(t)),x(0)=x0 | (2.1) |
where f(x) and g(x) are the uncertain smooth functions, which satisfy that f(0)=0, g(0)=0. u is the control input, x∈Rn is the state vector. For the above system, some adaptive optimization control strategies have been proposed.
In [15], for a class of affine nonlinear CT systems with unknown internal dynamics, Liu and Wang et al. developed an online method based on ADP, which constructed a critic neural network to facilitate the solution of the modified HJB equation. In [16], Liu et al. developed an online optimization control algorithm for CT affine nonlinear systems with infinite horizon cost. And in [17], Wen and Chen et al. studied an adaptive optimized tracking control method via RL algorithm and NNs.
For [16,17], value function is selected as:
V(z)=∫∞tr(z(r),u(z))dτ | (2.2) |
where r(z,u)=zT(t)Q(x)z(t)+uTu is the value function, and Q(x)=q(x)qT(x)∈Rn×n is a positive definite matrix. The HJB equation is defined as:
H(z,u,Vz)=VTz(z)z(t)+r(z,u)=VTz(f(x)+g(x)u−yd(t))+zTQ(x)z(t)+uTu | (2.3) |
where Vz∈Rn is the partial gradient of Vz, yd(t)∈Rn is the ideal tracking trajectory, z(t)=x(t)−yd(t) is the tracking error. When considering the input constraint control and saturation constraints control issue, Liu and Yang developed a robust optimal adaptive optimal control method via RL for a kind of uncertain nonlinear systems. In [18,19], there exists a symmetric definite matrix Q, and the value function is selected as:
V(x(t))=∫∞t[xTQx+ϖ(u)]ds,(s≥t) | (2.4) |
where ϖ(u) is positive. For the sake of solving the constraint control issue, define ϖ(u) as:
ϖ(u)=2κ∫u0(ψ−1(υ/κ))TRdv=2κm∑i=1∫u0(ψ−1(υi/κ))TRidv | (2.5) |
where R=diag[r1,⋯,rn] with ri>0, (i=1,⋯,m), ψ(⋅) is a bounded one-to-one function with |ψ(⋅)|≤1, ψ∈Rm, ψ−1=(ψ−1)T, ψ−1(υ/κ)=[ψ−1(υ1/κ),⋯,ψ−1(υm/κ)]T, u(x)∈Ξ, Ξ={u|u∈Rm,|ui|≤κ,i=1,2,⋯,m}, κ>0 is a constant. Define the HJB equation and the value function as:
H(x,Vx,u)=VTx(f(x)+g(x)u)+r(x,u) | (2.6) |
where Vx∈Rn is the partial derivative of V(x) with respect to x.
In the previous article, since there exist the unknown nonlinear functions f(x) and g(x), the analytic solution of the equation cannot be received when resolving the HJB equation. Because of their properties and fault tolerance, attributes of nonlinearity, adaptivity, the identified solution of the HJB equation can be obtained symmetric via NNs.
Because the system (2.1) contains unknown dynamics, we can identify the system for receiving the optimal control. In [20], Yang et al. presented identifier-actor-critic (IAC) structure, where the actor NN is carried out control actions, and critic NN is employed to estimated these actions, and then returns the evaluations to actor, and the dynamics of uncertain system robust dynamic can be approximate by NN identifiers. From system (2.1), we have that:
˙x=g(x)u+f(x)=g(x)u+Ax+ϝ(x) | (2.7) |
where A∈Rn×n is a certain constant matrix, ϝ(x)=f(x)−Ax.
A NN is applied to identify ϝ(x) as follows:
ϝ(x)=WT1σ(x)+ε1(x) | (2.8) |
where ε1(x)∈Rn is the NN function reestablishment error, σ(x) is the activation function, WT1∈Rn×n is the NN weight. By using (2.8), (2.7) can be developed by:
˙x(t)=g(x)u+ε1(x)+Ax+WT1σ(x) | (2.9) |
The NN identifier is designed as:
˙ˆx(t)=g(ˆx)u+v(t)+Aˆx+ˆWT1σ(ˆx) | (2.10) |
where ˆx∈Rn is the identifier NN state, ˆW1∈Rn×n is weight estimation, and v(t) is the robust feedback term.
The optimal value function can be expressed by NN as:
V∗(ˆx)=WTϕ(ˆx)+εv(ˆx) | (2.11) |
The optimal control can be expressed by NN as:
u∗(ˆx)=−12R−1gT(ˆx)(ϕ′(ˆx)TW+ε′v(ˆx)T) | (2.12) |
where εv(⋅)∈R is the function reestablishment error, ϕ(ˆx)=[ϕ1(ˆx),ϕ2(ˆx),⋯,ϕN(ˆx)]T∈RN, ϕ′(ˆx)=△∂ϕ(ˆx)∂ˆx and W∈RN are uncertain desired NN weights, N is the number of neurons.
The critic-actor ˆV(ˆx) and ˆu, which can learn the optimization value function and adjust the optimization control online, is expressed as:
ˆV(ˆx)=ˆWTcϕ(ˆx) | (2.13) |
ˆu(ˆx)=−12R−1gT(ˆx)ϕ′T(ˆx)^Wa | (2.14) |
where ˆWc(t)∈RN and ˆWa(t)∈RN estimate the ideal weights of the critic-actor NNs. Whereas the system dynamics are estimated online by using the identification error ˜x(t)=x(t)−ˆx(t). The overall planning diagram of the control algorithm is given in Figure 1.
Besides Bhasin et al. [21] proposed an online adaptive solution via RL for the unbounded optimization control nonlinear systems with CT uncertain problem. The advantage of using the IAC structure is that the learning of critics, actors, and identifiers is successive and simultaneous, removing the knowledge of system drift dynamics.
However, the above proposed control design algorithm for the affine nonlinear systems cannot be used to solve the optimal control issues for unmatching condition nonlinear systems, because it cannot guarantee the optimization of each subsystem.
The above research methods on affine nonlinear systems cannot be applied to nonlinear systems with unmatching conditions and the optimality of each subsystem can not be guaranteed. In order to solve the problem of unmatching conditions, we used the backstepping technology, which can also optimize each subsystem.
Consider the following strict feedback nonlinear systems as:
{˙xi=fi(¯xi)+xi+1,i=1,2,⋯,n−1˙xn=fn(¯xn)+uy=x1 | (3.1) |
where u and y are the control input and output, x is the state, ¯xi=[x1,x2,⋯,xi], is the system state vector. fi(⋅) is the uncertain nonlinear function, which satisfies f(0)=0.
In 1995, Kristic [22] firstly proposed the backstepping technology. The design idea of the backstepping algorithm is as follows: for systems that satisfy strict feedback control structures, via the backstepping algorithm, the Lyapunov function and controller are constructed in a systematic way. Then, for each subsystem, local Lyapunov function and intermediate control function are designed successively until the design of the whole controller is completed.
For the sake of solving the control issue for unmatching nonlinear systems (3.1), Wen et al. [23] first proposed an optimized backstepping control technology, under the backstepping framework, we can ensure that each subsystem can be optimized. Based on [23], for a kind of nonlinear large-scale systems with strict-feedback structure, Tong et al. [24] proposed the fuzzy decentralized adaptive optimal control, and used FLS to identify the uncertain nonlinear function of the systems. And in [25], for a quarter of the car active electric suspension systems, Li et al. addressed the output-feedback adaptive NN optimization control issue.
Because there are unknown nonlinear functions, the updating laws and learning laws designed for the above systems are very complex. In order to solve this problem, in [26], Wen et al. proposed a simplified RL algorithm, which generates a negative gradient of a simple positive function from the partial derivative of HJB equation, and derives a new law from the negative gradient.
Define the Hamiltonian's approximation error as:
E=H(ˆz,u,ˆV∗ˆz)−H(z,u∗,V∗ˆz)=H(ˆz,u,ˆV∗ˆz) | (3.2) |
where V∗ˆz(ˆz) is the gradient of V∗(ˆz), u∗ is the optimal control. Since V∗ˆz(ˆz) and u∗ contain the unknown part V0ˆz(ˆz), which can be approximated on a compact set by NNs as:
V0ˆz(ˆz)=Θ∗TVφV(ˆz)+εV(ˆz) | (3.3) |
Since Θ∗V is an uncertain constant vector, it is not available in practical control, RL algorithm is implemented by both critic-actor NNs.
The learning law of critic NN is designed as:
˙ˆΘVc(t)=−kcφV(ˆz)φTV(ˆz)ˆΘVc(t) | (3.4) |
where kc is the critic network learning rate, ˆΘVc(t) is the critic NN weight.
The learning law of actor NN is designed as:
˙ˆΘVa(t)=−φV(ˆz)φTV(ˆz)(ka(ˆΘVa(t)−ˆΘVc(t))+kcˆΘVc(t)) | (3.5) |
where ka is the actor network learning rate, ˆΘVa(t) is the actor NN weight, ka>kc>0.
In accordance with the above description, the optimal solution ˆα(ˆz) is supposed to meet E(t)=H(ˆz,u,ˆV∗ˆz)→0.
If H(ˆz,u,ˆV∗ˆz) is held and exist the unique solution, then it is equivalent to the following equation holds:
∂H(ˆz,u,ˆV∗ˆz)∂ˆΘVa=φVφTV(ˆΘTVa(t)−ˆΘTVc(t))=0 | (3.6) |
The positive definite function is designed as:
P(t)=(ˆΘVa(t)−ˆΘVc(t))T(ˆΘVa(t)−ˆΘVc(t)) | (3.7) |
Clearly, the Eq (3.6) is the equivalent to P(t)=0. Since ∂P(t)∂ˆΘVa(t)=−∂P(t)∂ˆΘVc(t)=2(ˆΘVa(t)−ˆΘVc(t)), we can get
∂P(t)dt=∂P(t)∂ˆΘVc(t)⋅ˆΘVc(t)+∂P(t)∂ˆΘVa(t)⋅ˆΘVa(t)=−kc∂P(t)∂ˆΘVc(t)φVφTVˆΘTVc(t)−∂P(t)∂ˆΘVc(t)φVφTV[ka(ˆΘVa(t)−ˆΘVc(t))+kcˆΘVc(t)]=−ka2∂P(t)∂ˆΘa(t)φVφTV∂P(t)∂ˆΘa(t)≤0 | (3.8) |
In [27], for nonlinear lithium battery systems, Pei et al. addressed adaptive NN output feedback optimization control problem, and the stability of the nonlinear lithium battery is proved.
On the basis of [26], under the frame of backstepping control, some simplified-based adaptive optimization control algorithms have been proposed, which require construct all intermediate control functions and the actual control function of backstepping to be the optimization controls, hence, RL is performed in each subsystem (see Figure 2).
In [28], Wen et al. addressed optimization control method for nonlinear strict-feedback systems with unknown functions. In [29], for second-order unknown nonlinear multiagent systems, Lan et al. proposed a distributed time-varying optimization formation protocol based on an adaptive NN state observer. In [30], Xiao et al. addressed the distributed optimization containment control issue for multiple nonholonomic mobile robots differential game.
It is worth mentioning that system states usually need to be confined within some preselected compact sets due to the physical limitations of actual systems. For real systems, in [31], Jiang and Lou considered the input-to-state stability (ISS) of delayed systems with bounded-delay impulses. In [32], for a hydraulic servo actuator (HSA) with sensor faults, Vladimir and Ljubisa investigated the mechanism for the fault estimation (FE) problem. However, methods in [29,30] could not solve the actual constraint problem. To solve this problem, various state-constrained control methodologies is discussed.
Aiming at strict-feedback nonlinear systems, which contain immeasurable states and internal dynamics, Li et al. [33] proposed an output-feedback adaptive NN optimization control design. Under the backstepping control design, there will be coupling terms or cross terms at each step, which will lead to that each subsystem is not optimal. Therefore, state constraints should be introduced to make the coupling terms bounded to ensure that each subsystem is optimal. And all the states are limited in the compact sets, that is, |xi|<kci, where kci>0.
The neoteric barrier optimization performance index functions for subsystems are designed to ensure that the system state does not violate the constraint bounds and achieves the optimization control objective, which is selected as:
J(z(t))=limτ→∞1τ∫τtq(z((t),α(z)))dz | (3.9) |
where τ is the terminal time, q(z,α)=ξlog[k4b/(k4b−z4)]+r(α)2, ξ>0 is a constant, α is the intermediate control function, The following Hamiltonian can be derived as:
H(ˆz,u,ˆV∗ˆz)=ξlogk4b(k4b−z4)+r(α)2+dV∗(z)dz(α∗+g(x)−yr) | (3.10) |
According to the algorithm presented in [34], for power systems with stochastic character, Li et al. designed the adaptive NN optimal tracking control to resolve the issue of state constraints and uncertain nonlinear dynamics. In [35], Li et al. put forward an adaptive NN optimized output-feedback control method to solve the issue of unknown nonlinear dynamics and input saturation. In [36], for uncertain nonlinear systems with time-varying full state constraints, input saturation and unknown control direction, Wu and Xie employed asymmetric barrier Lyapunov functions, the auxiliary subsystem and the Nussbaum gain technique.
Based on the above published works, some adaptive optimal control methods via backstepping control have also been applied to practical systems, for example, see [37,38]. In [37], Li et al. presented an adaptive NN optimized control strategy for full vehicle active suspension system. And in [38], Li et al. studied adaptive optimal formation control approach for second-order stochastic multi-agent system, which contains unknown nonlinear dynamics.
Based on the inverse optimization control method in [1], Ezal et al. [38] proposed a new robust backstepping inverse optimal control design, which achieved both local optimization and global inverse optimization. For a class of nonlinear uncertain strict feedback systems, Li et al. [39] designed adaptive fuzzy inverse optimization control by establishing an equivalent system and an auxiliary system.
System (2.1) can be rewritten as the following nonlinear system:
˙x=G(x)u+F(x)+q(x) | (3.11) |
where u∈R is the control input, x is the state vector, x=0 is the equilibrium point of system. q(x) is an uncertain bounded function vector, G(x) and F(x) are smooth function vectors.
Define γ is a class K∞ function, then the derivative of γ exists and it is also a class K∞ function. An auxiliary system is constructed for the nonlinear system (2.1):
˙x=lγ(2|LΔV|R(x))×R−2(x)(LΔV)T(LΔV)2+F(x)+G(x)u | (3.12) |
where V(x) is the control Lyapunov function. L△V=∂△V/∂x, LFVn=∂Vn/∂xF(x), LGVn=∂Vn/∂xG(x).
The cost functional is selected as:
J(u)=supd∈D{limt→∞[∫t0(l(x))+uTRu−γ(d))dτ+E(x)]} | (3.13) |
where D is a set of locally bounded functions of x, R(x) is matrix-valued function, which satisfied that R(x)=R(x)T>0. E(x) and l(x) are positive definite radially unbounded functions.
The fuzzy adaptive inverse optimization control structure is shown in Figure 3.
And in [40], Li et al. studied a fuzzy inverse optimization fuzzy adaptive output feedback control method based on observer for a class of nonlinear strict feedback systems. In [41], Lu et al. addressed a fuzzy adaptive inverse optimization control issue, and a switching inverse optimization controller is constructed by using a single parameter learning mechanism, which confirmed that the method guarantees the input-to-state stability of the control systems.
Inspired by the above theory, the inverse optimization theory is also widely applied to some practical systems. In [42], for vehicle active suspension system with unknown nonlinear dynamics, Li et al. designed an adaptive fuzzy inverse optimal control method via state observer. Long et al. [43] proposed an inverse optimal fuzzy adaptive control approach for the system of flexible spacecraft system with fault-free actuator, which is subjected to input saturation, uncertain parameter and external disturbances.
In addition, people hope the practical engineering will reach the stable in finite time, and use the less control energy when achieving the satisfactory performance indicators simultaneously. Thus, how to achieve the effective balance between control quality and control energy has become a hot research issue. In [44], for nonlinear impulsive systems, Li and Ho studied the problem of finite-time stability (FTS). In [45], Li and Yang developed the Lyapunov–Razumikhin method for finite-time stability (FTS) and finite-time contractive stability (FTCS) of time-delay systems. In [46], via the power integral control approach and backstepping control method, Yang designed a semi-global real finite time controller. Then, according to the basic idea of inverse optimization, an appropriate objective functional is constructed, and the constructed objective functional is minimized by adjusting the parameters of semi-global real finite time controller.
Consider the Lyapunov function as follows:
V1=[r12v−τ]x(2v−τ)r1+12¯ω12 | (3.14) |
where ¯ω1=ω∗1−^ω1, ^ω1 is the estimation of the unknown parameter ω∗1, v=max{r1,p1r2}, j=1,2,⋯,n, pjrj+1=rj+τ, υ=max1≤j≤n{rj,pjrj+1}, j=1,2,⋯,n. rj and pj is the ratio of two positive odd numbers. r1=1, τ is the design parameter.
Based on [46], for a class of interlinked nonlinear systems with powers of positive odd rational numbers, Li et al. [47] developed a series of homogeneous controllers, which are capable of guaranteeing the local finite-time stability of the closed-loop systems by using the adding one power integrator approach and backstepping technique.
Most of the existing optimization finite-time control methods are limited by complicated design and updating process, which vastly affect the ideal property of optimization finite-time control. In order to solve this issue, in [48], Lu et al. first proposed an immediate fuzzy adaptive inverse optimization approach to receive a switching-type inverse optimization controller and a one parameter learning mechanism. The inverse optimal stabilization is solvable, and there exists a matrix-valued function P(x), which satisfied that P(x)=P(x)T>0, then the cost function is defined as:
J(u)=limt→∞∫t0[L(x)+ℏ(|P(x)12u)]dτ} | (3.15) |
where ℏ and its derivative ℏ′ are K∞ functions, L(x) is positive functions, u(x) is away from the origin in succession with u(x)=0.
For a kind of robotic manipulator system, which contains uncertain dynamics and input saturation, the authors in [49] proposed a fixed-time trajectory tracking control approach based on RL. For the sake of guaranteeing that e1 and e2 convergence to diminutive neighborhood around 0 in a uniformly bounded convergence time Ts, where Ts stands alone with the original states. A noval nonsingular fixed-time fast terminal sliding mode is proposed as:
s=K(e1e1)+sigυ1(e2) | (3.16) |
where Ke1=diag[ke11,ke12,⋯,ke1n] is a diagonal matrix. ke1i,i=1,2,⋯,n, are designed as:
ke1i=(α|e1i|p−1/(kυ1)+β|e1i|g−1/(kυ1))kυ1 | (3.17) |
where p and g are positive scalars with gk>1 and 1/υ1<pk<1, α>0, β>0, k>1, υ1>1.
Obviously, the above developed control method can effectively solve the finite/fixed-time optimal control problems and can make the minimize the cost function. Besides, in [50], Hu et al. considered the fixed-time stability of delayed neural networks with impulsive perturbations.
It can be seen from this review that optimization control design for unknown nonlinear systems via RL and ADP has been diffusely studied in control area and has achieved fruitful results. The origin and the development of optimization algorithms have been introduced, the research results of optimization control of affine nonlinear systems have been summarized. Then, under the frame of backstepping control, the adaptive optimal control, finite-time inverse optimal control, constraint control have also been described for strict-feedback nonlinear systems. At the same time, we have summarized the applications development of adaptive optimization control methods. In addition, as a novel hot issue in this field, finite/fixed-time optimal control via backstepping and RL/ADP for nonlinear systems have attracted considerable attentions, both theory and practical applications also need to be further studied in the future.
This work was supported by the National Natural Science Foundation of China under Grant No. 61822307.
The authors declare there is no conflict of interest.
[1] | Singh H, Verma A, Ansari MW, et al. (2014) Physiological response of rice (Oryzasativa L.) genotypes to elevated nitrogen applied under field conditions. Plant Signal Behav 9: e29015. |
[2] |
Galloway JN, Townsend AR, Erisman W, et al. (2008) Transformation of the nitrogen cycle: recent trends, questions, and potential solutions. Science 320: 889-892. doi: 10.1126/science.1136674
![]() |
[3] |
Zhou J, Guan D, Zhou B, et al. (2015) Influence of 34-years of fertilization on bacterial communities in an intensively cultivated black soil in northeast China. Soil Biol Biochem 90: 42-51. doi: 10.1016/j.soilbio.2015.07.005
![]() |
[4] | Wang Y, Zhu Y, Zhan S, et al. (2018) What could promote farmers to replace chemical fertilizers with organic fertilizers? J Clean Prod 199: 882-890. |
[5] | Natsheh B, Mousa S (2014) Effect of organic and inorganic fertilizers application on soil and cucumber (Cucumis Sativa L.) plant productivity. Int J Agric For 4: 166-170. |
[6] | Hernandez T, Chocano C, Moreno JL, et al. (2016) Use of compost as an alternative to conventional inorganic fertilizers in intensive lettuce (Lactuca sativa L.) crops. Effects on soil and plant. Soil Tillage Res 160: 14-22. |
[7] |
Cai A, Zang W, Xu M, et al. (2018) Soil fertility and crop yield after manure addition to acidic soils in South China. Nutr Cycl Agroecosyst 11: 61-72. doi: 10.1007/s10705-018-9918-6
![]() |
[8] |
Cai A, Xu M, Wang B, et al. (2019) Manure acts as a better fertilizer for increasing crop yields than synthetic fertilizer does by improving soil fertility. Soil Tillage Res 189: 168-175. doi: 10.1016/j.still.2018.12.022
![]() |
[9] |
Hernandez T, Chocano C, Coll MD, et al. (2018) Composts as alternative to inorganic fertilization for cereal crops. Environ Sci Pollut Res 26: 35340-35352. doi: 10.1007/s11356-018-3898-6
![]() |
[10] |
De Souza JRM, Artur AG, Taniguchi CAK, et al. (2018) Yellow melon yield in response to mineral or organic fertilization. J Plant Nutr 41: 1197-1204. doi: 10.1080/01904167.2018.1434543
![]() |
[11] |
Rezácová V, Czakó A, Stehlik M, et al. (2021) Organic fertilization improves soil aggregation through increases in abundance of eubacteria and products of arbuscular mycorrhizalfungi. Sci Rep 11: 12548. doi: 10.1038/s41598-021-91653-x
![]() |
[12] |
Xin X, Zhang J, Zhu A, et al. (2016) Effects of long-term (23 years) mineral fertilizer and compost application on physical properties of fluvo-aquic soil in the North China plain. Soil Tillage Res 156: 166-172 doi: 10.1016/j.still.2015.10.012
![]() |
[13] | Hernandez T, Garcia E, García C (2015) A strategy for marginal semiarid degraded soil restoration: A sole addition of compost at a high rate. A five-year field experiment. Soil Biol Biochem 89: 61-71. |
[14] |
Ochoa-Hueso R, Delgado-Baquerizo M, King PTA, et al. (2019) Ecosystem type and resource quality are more important than global change drivers in regulating early stages of litter decomposition. Soil Biol Biochem 129: 144-152. doi: 10.1016/j.soilbio.2018.11.009
![]() |
[15] |
Van der Wal A, Geydan TD, Kuyper TW, et al. (2013) A thread affair: linking fungal diversity and community dynamics to terrestrial decomposition processes. FEMS Microbiol Rev 37: 477-494. doi: 10.1111/1574-6976.12001
![]() |
[16] |
Fierer N, Lauber CL, Ramirez KS, et al. (2012) Comparative gradients. ISME J 6:1007-1017. doi: 10.1038/ismej.2011.159
![]() |
[17] |
Smalla, Wachtendorf U, Heuer H, et al. (1998) Analysis of Biolog GN substrate utilization patterns by microbial communities. Appl Environ Microbiol 64: 1220-1225. doi: 10.1128/AEM.64.4.1220-1225.1998
![]() |
[18] | Preston-Mafham J, Boddy L, Randerson PF (2002) Analysis of microbial community functional diversity using sole-carbon-source utilization profiles- a critique. FEMS Microbiol Ecol 42: 1-14. |
[19] |
Braun S, Thomas V, Quiring R, et al. (2010) Does nitrogen deposition increase forest production? The role of phosphorus. Environ Pollut 158: 2043-2052. doi: 10.1016/j.envpol.2009.11.030
![]() |
[20] |
Ros M, Pascual JA, Garcia C, et al. (2006) Hydrolase activities, microbial biomass and bacterial community in a soil after long-term amendment with different composts. Soil Biol Biochem 38: 3443-3452. doi: 10.1016/j.soilbio.2006.05.017
![]() |
[21] | Directive 86/278/EEC of 12 June, Relative to the environment protection, in particular, soils in the utilization of sewage sludges in agriculture, 1986. Available from: https://op.europa.eu/en/publication-detail/-/publication/f76faa39-2b27-42f2-be1e-9332f795e324. |
[22] |
Rehman RA, Rizwan M, QayyumMF, et al. (2018) Efficiency of various sewage sludges and their biochars in improving selected soil properties and growth of wheat (Triticum aestivum). J Environ Manag 223: 607-613. doi: 10.1016/j.jenvman.2018.06.081
![]() |
[23] |
Koutroubas SD, Antoniadis V, Fotiadis S, et al. (2014) Growth, grain yield and nitrogen use efficiency of Mediterranean wheat in soils amended with municipal sewage sludge. Nutr Cycl Agroecosyst 100: 227-243. doi: 10.1007/s10705-014-9641-x
![]() |
[24] |
Lax A, Díaz E, Castillo V, et al. (1994) Reclamation of physical and chemical properties of salinized soil by organic amendment. Arid Land Res Manag 8: 9-17. doi: 10.1080/15324989309381374
![]() |
[25] | Hernández T, García C (2003) Estimación de respiración microbiana del suelo (Stimation of soil respiration). Técnicas de Análisis de Parámetros Bioquímicos en Suelos. Actividades Enzimáticas y Biomasa Microbiana (Techniques of analysis of Biochemical parameters in soils. Enzymatic activities and microbial biomass), Mundi-Prensa. Madrid, 311-346. |
[26] |
García C, Hernandez MT, Costa F (1997) Potential use of dehydrogenase activity as an index of microbial activity in degraded soils. Comm Soil Sci Plant Anal 28: 123-134. doi: 10.1080/00103629709369777
![]() |
[27] |
Eivazi F, Tabatabai MA (1988) Glucosidase and galactosidase in soils. Soil Biol Biochem 20: 601-606. doi: 10.1016/0038-0717(88)90141-1
![]() |
[28] |
Kandeler E, Gerber H (1988) Short-term assay of soil urease activity using colorimetric determination of ammonium. Biol Fert Soils 6: 68-72. doi: 10.1007/BF00257924
![]() |
[29] |
Sinsabaugh RL, Antibus RK, Linkins AE, et al. (1993) Wood decomposition: nitrogen and phosphorus dynamics in relation to extracellular enzyme activity. Ecology 74: 1586-1593. doi: 10.2307/1940086
![]() |
[30] |
Garland JL, Mills AI (1991) Classification and characterization of heterotrophic microbial communities on the basis of patterns of community-level sole-carbon-source utilization. Appl Environ Microbiol 57: 2351-2359. doi: 10.1128/aem.57.8.2351-2359.1991
![]() |
[31] |
Weber KP, Legge RL (2009) One-dimensional metric for tracking bacterial community divergence using sole carbon source utilization patterns. J Microbiol Methods 79: 55-61. doi: 10.1016/j.mimet.2009.07.020
![]() |
[32] |
Kaufmann K, Christophersen M, Buttler A, et al. (2004) Microbial community response to petroleum hydrocarbon contamination in the unsaturated zone at the experimental field site Vaerlose, Denmark. FEMS Microbiol Ecol 48: 387-399. doi: 10.1016/j.femsec.2004.02.011
![]() |
[33] |
Bligh EG, Dyer WJ (1959) A rapid method for total lipid extraction and purification. Can J Biochem Physiol 37: 911-917. doi: 10.1139/o59-099
![]() |
[34] |
Frostegard Å, Tunlid A, Bååth E (1993) Phospholipid fatty acid composition, biomass, and activity of microbial communities from two soil types experimentally exposed to different heavy metals. Appl Environ Microbiol 59: 3605-3617. doi: 10.1128/aem.59.11.3605-3617.1993
![]() |
[35] |
Bardgett RD, Hobbs PJ, Frostegard A (1996) Changes in soil fungal: bacterial biomass ratios following reductions in the intensity of management of an upland grassland. Biol Fertil Soils 22: 261-264. doi: 10.1007/BF00382522
![]() |
[36] |
Demelash N, Bayu W, Tesfaye S, et al. (2014) Current and residual effects of composts and inorganic fertilizers on wheat and soil chemical properties. Nutr Cycl Agroecosyst 100: 357-367. doi: 10.1007/s10705-014-9654-5
![]() |
[37] |
Oades JM (1984) Soil organic matter and structural stability: mechanisms and implications for management. Plant Soil 76: 319-337. doi: 10.1007/BF02205590
![]() |
[38] |
Tisdall JM, Oades JM (1982) Organic matter and water-stable aggregates in soils. Soil Sci 62: 141-163. doi: 10.1111/j.1365-2389.1982.tb01755.x
![]() |
[39] | Hernandez T, Hernandez MC, Garcia C (2017) The effects on soil aggregation and carbon fixation of different organic amendments for restoring degraded.soils in semiarid areas. Eur J Soil Sci 68: 941-950. |
[40] |
Zou C, Li Y, Huang W, et al. (2018) Rotation and manure amendment increase soil macro-aggregates and associated carbon and nitrogen stocks in flue-cured tobacco production. Geoderma 325: 49-58. doi: 10.1016/j.geoderma.2018.03.017
![]() |
[41] |
Mangalassery D, Kalaivanan S, Philip PS (2019) Effect of inorganic fertilisers and organic amendments on soil aggregation and biochemical characteristics in a weathered tropical soil. Soil Tillage Res 187: 144-151. doi: 10.1016/j.still.2018.12.008
![]() |
[42] |
Long P, Sui P, Gao WS, et al. (2015) Aggregate stability and associated C and N in a silty loam soil as affected by organic material inputs. J Integr Agric 14: 774-787. doi: 10.1016/S2095-3119(14)60796-6
![]() |
[43] |
Heijboer A, ten Berge HFM, de Ruiter PC, et al. (2016) Plant biomass, soil microbial community structure and nitrogen cycle under different organic amendment regimes: a 15N tracer-based approach. Appl Soil Ecol 107: 251-260. doi: 10.1016/j.apsoil.2016.06.009
![]() |
[44] |
Zhao L, Li L, Cai H et al. (2019) Organic amendments improve wheat root growth and yield through regulating soil properties. Agron J 111: 482-495. doi: 10.2134/agronj2018.04.0247
![]() |
[45] |
Toor RR, Savage GP, Heeb A (2006) Influence of different types of fertilizers on the major antioxidant components of tomatoes. J Food Composition Anal 19: 20-27. doi: 10.1016/j.jfca.2005.03.003
![]() |
[46] |
Hu J, Lin X, Wang J, et al. (2011) Microbial functional diversity, metabolic quotient and invertase activity of a sandy loam soil as affected by long-term application of organic amendment and mineral fertilizer. J Soils Sediments 11: 271-280. doi: 10.1007/s11368-010-0308-1
![]() |
[47] | Zhong Y, Zou S, Lin L, et al. (2010) Effects of pyrene and fluoranthene on the degradation characteristics of phenanthrene in the cometabolism process by Sphingomonas sp. strain PheB4 isolated from mangrove sediments. Mar Pollut Bull 60: 2043-2049. |
[48] | Albiach R, Canet R, Pomares F, et al. (2000) Microbial biomass content and enzymatic activities after the application of organic amendments to a horticultural soil. Bioresour Technol 75: 43-48. |
[49] |
Hoogmoed M, Cunningham SC, Baker P, et al. (2014) N-fixing trees in restoration plantings: effects on nitrogen supply and soil microbial communities. Soil Biol Biochem 77: 203-213. doi: 10.1016/j.soilbio.2014.06.008
![]() |
[50] |
Shrestha P, Gautam R, Ashwath N (2019) Effects of agronomic treatments on functional diversity of soil microbial community and microbial activity in a revegetated coal mine spoil. Geoderma 338: 40-47. doi: 10.1016/j.geoderma.2018.11.038
![]() |
[51] |
Chakraborty A, Chakraborti K, Chakraborty A, et al. (2011) Effect of long-term fertilizers and manure application on microbial biomass and microbial activity of a tropical agricultural soil. Biol Fertil Soils 47: 227-233. doi: 10.1007/s00374-010-0509-1
![]() |
[52] |
Rashedul I, Puneet SC, Yoohak K, et al. (2011) Community level functional diversity and enzyme activities in paddy soils under different long-term fertilizer management practices. Biol Fertil Soils 47: 599-604. doi: 10.1007/s00374-010-0524-2
![]() |
[53] |
Yang XY, Ren WD, Sun BH, et al. (2012) Effects of contrasting soil management regimes on total and labile soil organic carbon fractions in a loess soil in China. Geoderma 177-178: 49-56. doi: 10.1016/j.geoderma.2012.01.033
![]() |
[54] |
Bray SR, Kitajima K, Mack MC (2012) Temporal dynamic of microbial communities on decomposing leaf litter of 10 plant species in relation to decomposition rate. Soil Biol Biochem 49: 30-37. doi: 10.1016/j.soilbio.2012.02.009
![]() |
[55] |
Kramer C, Gleixner G (2008) Soil organic matter in soil depth profiles: distinct carbon preferences of microbial groups during carbon transformation. Soil Biol Biochem 40: 425-433. doi: 10.1016/j.soilbio.2007.09.016
![]() |
[56] |
Börjesson G, Menichetti L, Kirchmann H (2012) Soil microbial community structure affected by 53 years of nitrogen fertilisation and different organic amendments. Biol Fertil Soils 48: 245-257. doi: 10.1007/s00374-011-0623-8
![]() |
[57] |
Tscherko D, Hammesfahr U, Marx MC, et al. (2004) Shits in rhizosphere microbial communities and enzyme activity of Poa alpine across an alpine chronosequence. Soil Biol Biochem 36: 1685-1698. doi: 10.1016/j.soilbio.2004.07.004
![]() |
[58] |
Hueso S, Garcia C, Hernandez T (2012) Severe drought conditions modify the microbial community structure, size and activity in amended and unamended soils. Soil Biol Biochem 50: 167-173. doi: 10.1016/j.soilbio.2012.03.026
![]() |
[59] |
Bossio DA, Scow KM, Gunapala N, et al. (1998) Determinants of soil microbial communities: Effects of agricultural management, season, and soil type on phospholipids fatty acid profiles. Microbial Ecol 36: 1-12. doi: 10.1007/s002489900087
![]() |
[60] |
Vorisková J, Baldrian P (2012) Fungal community on decomposing leaf litter undergoes rapid successional changes. ISME J 7: 477-486. doi: 10.1038/ismej.2012.116
![]() |
[61] |
Trivedi P, Delgado-Baquerizo M, Jeffries TC, et al. (2017) Soil aggregation and associated microbial communities modify the impact of agricultural management on carbon content. Environ Microbiol 19: 3070-3086. doi: 10.1111/1462-2920.13779
![]() |
[62] |
Lehmann J, Kleber M (2015) The contentious nature of soil organic matter. Nature 528: 60-68. doi: 10.1038/nature16069
![]() |
[63] |
Kadri T, Rouissi T, Kaur-Brar S, et al. (2017) Biodegradation of polycyclic aromatic hydrocarbons (PAHs) by fungal enzymes: a review. J Environ Sci 51: 52-74. doi: 10.1016/j.jes.2016.08.023
![]() |
[64] |
Hu P, Wu L, Hollister EB, et al. (2019) Fungal community structural and microbial functional pattern changes after soil amendments by oilseed meals of Jatropha curcas and Camelina sativa: a microcosm study. Front Microbiol 10: 537. doi: 10.3389/fmicb.2019.00537
![]() |
[65] |
Banerjee S, Kirbby CA, Schmutter DA, et al. (2016) Network analysis reveals functional redundancy and keystone taxa amongst bacterial and fungal communities during organic matter decomposition in an arable soil. Soil Biol Biochem 97: 188-198. doi: 10.1016/j.soilbio.2016.03.017
![]() |
[66] |
Broeckling CD, Broz AK, Bergelson J, et al. (2008) Root exudates regulate soil fungal community composition and diversity. Appl Environ Microbiol 74: 738-744. doi: 10.1128/AEM.02188-07
![]() |
[67] |
Sapp M, Harrison M, Hany U, et al. (2015) Comparing the effect of digestate and chemical fertiliser on soil bacteria. Appl Soil Ecol 86: 1-9. doi: 10.1016/j.apsoil.2014.10.004
![]() |
[68] |
Montiel-Rozas MM, Dominguez MT, Madejon E, et al. (2018) Long-term effect of organic amendments on bacterial and fungal communities in a degraded Mediterranean soil. Geoderma 332: 20-28. doi: 10.1016/j.geoderma.2018.06.022
![]() |
[69] |
Lopedota O, Leogrande R, Fiore A, et al. (2013) Yield and soil responses of melon grown with different organic fertilizers. J Plant Nutr 36: 415-428. doi: 10.1080/01904167.2012.748062
![]() |
[70] |
Dungait JAJ, Kemmit SJ, Michallon M, et al. (2011) Variable responses of the soil microbial biomass to trace concentrations of 13C-labelled glucose, using 13C-PLFA analysis. Eur J Soil Sci 62: 117-126. doi: 10.1111/j.1365-2389.2010.01321.x
![]() |
[71] |
Brant JB, Sulzman EW, Myrold DD (2006) Microbial community utilization of added carbon substrates in response to long-term carbon input manipulation. Soil Biol Biochem 38: 2219-2232. doi: 10.1016/j.soilbio.2006.01.022
![]() |