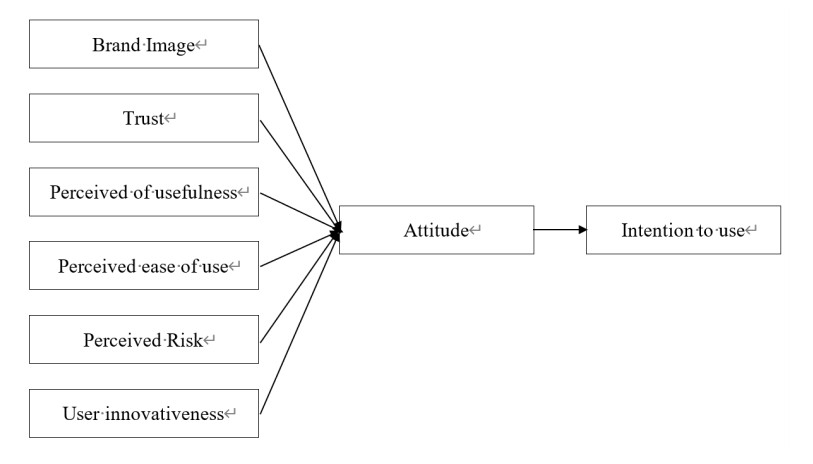
In recent years, the number of fintech startups has increased substantially, especially those that offer financial services in areas considered as traditional advantage of commercial banks such as payment, deposit or lending services. This paper examines the cooperation in the provision of financial services between banks and Fintech from consumers' perspective, which is the customers' adoption of financial services offered by the partnership between banks and Fintechs. The study employs SEM utilizing data of 234 customers using financial services in Vietnam to identify the factors influence their behaviors and intention to use financial services offered by banks and fintechs partnerships. The findings indicate that perceived usefulness, brand image, user innovativeness and trust have positive effects on customers' attitude and consequently on their intention to use. However, there is no evidence regarding the impact of perceived ease of use and perceived risks on behavior and intention to use of customers. The findings also provide more evidence on the factors that influence the intention of customers' technology acceptance in the traditional Technology Acceptance Model (TAM).
Citation: Yen H. Hoang, Duong T.T. Nguyen, Linh H.T. Tran, Nhung T.H. Nguyen, Ngoc B. Vu. Customers' adoption of financial services offered by banks and fintechs partnerships: evidence of a transitional economy[J]. Data Science in Finance and Economics, 2021, 1(1): 77-95. doi: 10.3934/DSFE.2021005
[1] | Hana Demma Wube, Sintayehu Zekarias Esubalew, Firesew Fayiso Weldesellasie, Taye Girma Debelee . Text-Based Chatbot in Financial Sector: A Systematic Literature Review. Data Science in Finance and Economics, 2022, 2(3): 232-259. doi: 10.3934/DSFE.2022011 |
[2] | Dominic Joseph . Estimating credit default probabilities using stochastic optimisation. Data Science in Finance and Economics, 2021, 1(3): 253-271. doi: 10.3934/DSFE.2021014 |
[3] | Ying Li, Keyue Yan . Prediction of bank credit customers churn based on machine learning and interpretability analysis. Data Science in Finance and Economics, 2025, 5(1): 19-34. doi: 10.3934/DSFE.2025002 |
[4] | Mesbaul Haque Sazu, Sakila Akter Jahan . Impact of blockchain-enabled analytics as a tool to revolutionize the banking industry. Data Science in Finance and Economics, 2022, 2(3): 275-293. doi: 10.3934/DSFE.2022014 |
[5] | Yuxin Li, Jizheng Yi, Huanyu Chen, Duanxiang Peng . Theory and application of artificial intelligence in financial industry. Data Science in Finance and Economics, 2021, 1(2): 96-116. doi: 10.3934/DSFE.2021006 |
[6] | Maria Paola Priola, Annalisa Molino, Giacomo Tizzanini, Lea Zicchino . The informative value of central banks talks: a topic model application to sentiment analysis. Data Science in Finance and Economics, 2022, 2(3): 181-204. doi: 10.3934/DSFE.2022009 |
[7] | Changjun Zheng, Md Mohiuddin Chowdhury, Anupam Das Gupta . Investigating the influence of ownership on the relationship between bank capital and the cost of financial intermediation. Data Science in Finance and Economics, 2024, 4(3): 388-421. doi: 10.3934/DSFE.2024017 |
[8] | Changjun Zheng, Md Abdul Mannan Khan, Mohammad Morshedur Rahman, Shahed Bin Sadeque, Rabiul Islam . The impact of monetary policy on banks' risk-taking behavior in an emerging economy: The role of Basel II. Data Science in Finance and Economics, 2023, 3(4): 427-451. doi: 10.3934/DSFE.2023024 |
[9] | Sina Gholami, Erfan Zarafshan, Reza Sheikh, Shib Sankar Sana . Using deep learning to enhance business intelligence in organizational management. Data Science in Finance and Economics, 2023, 3(4): 337-353. doi: 10.3934/DSFE.2023020 |
[10] | Sami Mestiri . Credit scoring using machine learning and deep Learning-Based models. Data Science in Finance and Economics, 2024, 4(2): 236-248. doi: 10.3934/DSFE.2024009 |
In recent years, the number of fintech startups has increased substantially, especially those that offer financial services in areas considered as traditional advantage of commercial banks such as payment, deposit or lending services. This paper examines the cooperation in the provision of financial services between banks and Fintech from consumers' perspective, which is the customers' adoption of financial services offered by the partnership between banks and Fintechs. The study employs SEM utilizing data of 234 customers using financial services in Vietnam to identify the factors influence their behaviors and intention to use financial services offered by banks and fintechs partnerships. The findings indicate that perceived usefulness, brand image, user innovativeness and trust have positive effects on customers' attitude and consequently on their intention to use. However, there is no evidence regarding the impact of perceived ease of use and perceived risks on behavior and intention to use of customers. The findings also provide more evidence on the factors that influence the intention of customers' technology acceptance in the traditional Technology Acceptance Model (TAM).
Technology is developing so speedily that it affects all facets of the business. One of these is the creative use of the internet to help companies improve their business (Meyliana et al., 2019). Technological innovation has led to the combination of information and financial technology into Financial Technology (Fintech). Fintech is a digital technology with blockchain core, big data, and smart investment consulting are widely used in the financial sector. It provides the new points of financial services that become more efficient in payment schemes in a transaction. It changes the traditional financial services to innovative services in the financial services industry in the form of Fintech. Thus, it provides a broader range in the field of financial services, starting from products created to available services and markets. In addition, the Financial Stability Board (FSB) (2019) defined FinTech as new frontier technologies, including AI (artificial intelligence), blockchain and big data that promote emerging business models, new technology applications and advanced product services. As traditional financial services, the banks understand the importance of user experience, and some have begun to improve core competitiveness and market share by acquiring or partnering with Fintech company. Fintech has brought about tremendous changes in the digital economy, especially in India, the United Kingdom and China (Chua et al., 2019; Cham et al., 2018; Kim et al., 2015). Since the end of the first decade of the 21st century, financial technology has been adopted into the digitalization of the following sectors: payment (electronic money, mobile payment, QR code payment, etc.); savings; investment and asset management; blockchain and related applications; credit, peer-to-peer lending; insurance; data analysis (Stewart & Jürjens, 2018; Wonglimpiyarat, 2017; Datta, 2011; Donner & Tellez, 2008). Moreover, Fintech also mentioned the operations of technology companies that have an advantage in re-searching and developing software solutions to support or directly provide alternative banking services with safety, speed and low costs priority (Vietnam Microfinance Working Group, 2018; Romānova & Kudinska, 2016). In recent years, Fintech have become major trends in the world and in Vietnam as banking and financial markets witnessed a steady growth of the company non-banking technology.
In general, the increase of Fintech has brought about a great impact on the banking industry, changing the banking ecosystem, even overthrowing previous business models as well as service modes (Hamidi and Safareeyeh, 2019). Furthermore, the development of Fintech in the recent years has brought positive change to Vietnam's economy. Vietnamese banks have accelerated their digital push, partnering with Fintech companies to ramp up efforts. For example, Vietnam's Tien Phong Commercial Joint Stock Bank (TPBank) recently partnered with digital banking platform Backbase to provide its customers with digital priority products and services. Non-cash payments, digital banking and green banking are the top three priorities of the industry in the period 2020–2025 in Vietnam. Besides, the development of digital banking is accelerating the combination of Fintech rapidly, the e-commerce industry is booming. Although many banks have chosen to cooperate with foreign fintechs, such as VietinBank and Opportunity Network (UK), CIMB Bank Vietnam and Toss (Korea), VPBank and BE Group (Sweden), OCB and RippleNet (USA), TPBank and Backbase (Netherlands), several other banks have partnered with local startups. Thus, Fintech companies and banks choose to cooperate with each other and how customers react to the combined products of these two partners.
This study explores customer responses through their perception of behavior and usage intentions for high-tech products combining the bank and the Fintech company. Including image awareness, trust, the usefulness of the technology, perceived ease of use, perceived risk, user innovativeness influence to the attitude of users and from that affect to customers' intention to use banking-Fintech combined high-tech products by using the Theory of Technology Acceptance Model (TAM) as the basic theory of this research.
Previous studies focused on qualitative research from the supply side (Fintechs and Banks), specifically investigating the difference between expected benefits and desired cooperation process of each party to ensure the success of the cooperation process. While banks take advantage of Fintech technology (Halilovic & Cicic, 2013), Fintechs utilize customer base of banks in the cooperation (You & Kim, 2016; Ng & Kwok, 2017). This study conducts a quantitative research from the demand side (customers) to determine the customer's perception of Fintech products distributed through banks and examinethefactors that affect the intention to choose Fintech-banks products of the Bank. Research results provide empirical evidence for both banksand Fintechs' decision makers to not only focus on the usefulness of products, user innovativeness and creating trust for customers but each parties also need to build and maintain a good brand image if they want customers to accept and choose Fintech products distributed through the banks.
A theoretical model widely applied in the research on the level of customer acceptance of Fintech services is the theory of reasoned action (TRA) model by Fishbein & Ajzen (1977). Based on the theory of TRA, the Technology Acceptance Model (TAM) was developed by Davis et al. (1989). This model is considered a fundamental theoretical model used by many researchers to build research models related to predicting or explaining individual behavior. Technology Acceptance Model (TAM) (Davis et al., 1989) has been one of the most influential models of technology acceptance, with two primary factors affecting an individual's intention to use new technology: perceived usefulness and perceived ease of use. According to Davis et al. (1989), the perception of usefulness is the degree to which a person believes using a particular system will enhance his or her productivity. Besides, perceived ease of use is as perception about ease of using technology. Based on the development, the TAM model can evaluate and identify elements that influence human behavior towards the use of a technology (Davis et al., 1989). Since the TAM model could effectively explain the difference in the consumer's information technology readiness and can be effectively improved and identified by the analytical problem, it has become into one of the most widely used models in information technology research and application. According to Lin et al. (2020) the relationship between attitude toward using and behavioral intention to use represents that a user will generate behavioral intention to use for the execution of a certain behavior when he/she has a positive attitude toward the result of the certain behavior. In addition, this model has been widely applied in academic research as well as in business practices and social fields. Advanced technologies are applied to provide products and services for individual customers (Bailey et al., 2017) includes online banking, mobile banking, or phone banking (Patel & Patel, 2018; Martins et al., 2014; Abbad, 2013; Zhou & Yang, 2011); social networks (Kim, 2012); digital library (Chen et al., 2016; Kapoor et al., 2014); and in a diversity of other business operations based on different digital platforms (Demoulin and Djelassi, 2016).
The traditional TAM holds that a positive attitude toward new technology is the premise of intentions to adopt this technology (Gupta & Arora, 2017). There is a significantly positive correlation between users' attitudes and their adoption intentions toward a certain technology (Aboelmaged & Gebba, 2013). Previous review indicated that TAM could serve as a useful theoretical framework for current research (Lee & Lehto, 2013). Furthermore, according to Shaikh et al. (2020) TAM is considered by many researchers as a model that can provide insight into complex human behavior and extend further analysis of these behavioral factors in the direction of adopting the system specific. Similarly, TAM is still successful in developing more user adoption in the Information Systems field. Several researchers use the TAM to predict FinTech adoption, such as mobile payments in Taiwan (Wu & Wang, 2005), crypto payments in the Netherlands (Jonker, 2019), e-tickets in Finland (Mallat et al., 2009), as well as e-commerce (Smith et al., 2014) among other services. Few studies have analyzed consumers' bank switching behavior (Manrai and Manrai, 2007). The study of Lee & Lehto (2013) indicates that the attitudes influence their respective intentions and behaviors toward adopting a new technology. On the other hand, in some studies, applying TAM's theoretical framework have proposed to remove the attitude factor from the model because the perceived usefulness and ease of use directly influence the intention without go through the transitional factor as initially predicted (Koufaris, 2002; Patel & Patel, 2018). There was a close relationship between Perceived Usefulness and User Behavior Intent, in which there was no effect of the attitude factor (Venkatesh et al., 2003). However, in this study, to argue more closely, the attitude factor is used as intermediate issue before other factors influence to customers intention using services from the model.
The Intention to use variable was based on the Behavioral Intent concept. According to the theory of reasoned action—TRA by Azjen & Fishbein (1980), the composition of the reason for deciding to take an action included: behavioral intention (BeI), the attitude of the subject towards the behavior (attitude-A), and the external subjective criteria affecting the behavior (subjective norm-SN). This doctrine proposed that a subject's behavioral intention depends on his or her attitude about the action and outside subjective criteria, following to the formula:
Behavioral Intention (BeI) = Attitude towards Behavior (ATT) + Subjective Norm (SN)
Behavioral intent measures the relative strength of an object of intent to perform an act. However, Fishbein & Ajzen (1977) suggested that the proportions of attitudes and subjective criteria in predicting behavior were very different. If the individual was the type of person who cared less about what those around him thought about his actions, the proportion of subjective norm (SN) would be less than the proportion of individual attitudes (A) when predicting the individual's behavior (Miller, 2005).
In the theory of "planned behavior" (TPB) (Ajzen, 2011) proposed "behavior control perception" factor to analyze the formation of attitude and behavior of performing an act. A person's attitude towards a thing or phenomenon was generally considered to be an important psychological structure, relatively permanent and stable. It was, furthermore, the factor that influences and predicts many behaviors (Olson and Zanna, 1993). In general, the more positive an attitude toward a behavior was, the stronger an individual's intention to perform the act (Ajzen, 1991).
In the Theory of Reasoned Action (TRA), a specific personal behavior was influenced by one's BeI. In turn, BeI was determined by the person's attitude (ATT), in conjunction with subjective norm (SN). TAM model inherited TRA to explain users' acceptance on information technology, eliminating the impact of SN. TAM predicated that users' intention to use a technology product was based on their affective feelings about it, which was called attitude (Yang and Yoo, 2004). There were empirical studies that have approved the positive impact of users' attitude on their intent to use Fintech products and services (Teo et al., 2009; Kim and Woo, 2016; Bailey et al., 2017).
Hypothesis H1: Customers' ATT positively affects customer's intention to use high-tech products in combination between banks and Fintech.
One characteristic of the service industry is that customer can only validate the quality after their usage of service. Therefore, it is common for customers to search for clues about service provider's quality before deciding to use it. Brand reputation is one of importance sources for customers to base their evaluation on. This factor is more critical to complex services like financial services, including banking and Fintech (Devlin, 1997). When the quality and functions of Fintech products and services were vague, reliable brands may be references for consumers (Pauline, 2003). A reputation brand, which was the result of the stability operations, long history of development and sustainability, can have positive effect on the intent of use (Wang and Li, 2012; Stocchi et al., 2019; Khodabandeh and Lindh, 2020).
Hypothesis H2: Perception of a bank's brand name positively affects the attitude of customers to use high-tech products combined between the bank and Fintech.
The trust has been identified by many studies that it has an impact on the intent to use Fintech products and services, especially due to their quality of immense and multidimensional data. Trust is an interdisciplinary concept that has been studied by many researchers in sociology, management, organizational behavior and many other disciplines (Lee and Turban, 2001; McKnight and Chervany, 2001). On research of the intention to use of banking products and services-fintech, trust refered to the overall perception of the benefits of the user. Kesharwani and Singh Bisht (2012) showed that user's trust could induce positive behaviors in the intent to use Fintech products and services, regarding its inherent characteristics (Lee and Turban, 2001). Researchers have found that trust is closely related to brand image and risk (Roy et al., 2012). User perception of banking brands and service risk perception would have significant impact on customers' confidence in the bank. In addition, many scholars have affirmed that user trust in services played an important role in deciding on using Fintech products.
In other words, the more users trust the service provider, the more willing they will be to use the service (Wu and Wang, 2005; Munoz-Leiva et al., 2017; Qiu and Li, 2008), means the easier it is to promote the behavior of use.
Concisely, if the brand perception and trust are higher, the attitude toward purchasing is more positive. When consumers believe that the information provided by the services provider is reliable, they will have a positive attitude toward using. Therefore, the definition of "trust" (brand and service trust) in this study is "the degree to which the customers" belief in reputation, website quality and system security are related to guest behavior of using Fintech products and services.
Hypothesis H3: Trust has a positive impact on the attitude of use of customers for banking-Fintech products.
Consumers' attitude was determined to depend on their perception of the usefulness of technology (López-Nicolás et al., 2008; Dulcic et al., 2012; Wallace and Sheetz, 2014; Chuen, 2015). To promote willingness to use a new technology, potential users must perceive that the new technology could benefit them (Chau and Hu, 2002). The perception of Usefulness was also believed to be the perception of potential users about new technology that would increase their work efficiency and benefit them in the future (Moon and Kim, 2001). If users believe that the benefits offered by Fintech are useful (e.g. they can quickly complete their tasks) and easy to use (they can get started without someone else's guidance), their attitude towards using Fintech is also higher. Therefore, the usefulness and ease of use of Fintech Service are the cognitive factors for consumers to accept the new technology.
Hypothesis H4: Perception of usefulness positively affects customers' attitude to use bank-fintech products.
Perception of ease is another important factor in TAM model, refers to the degree to which potential users consider that new technology is easy to use (Moon and Kim, 2001). In this (Venkatesh and Davis, 2000) study, the degree of ease perceived is the one to which consumers feel comfortable during the process of learning to use services provided by Fintech companies. Fintech combined services could provide customers better experience, for instance, making customer feel more convenient or making products and services more customized to individual needs. This ability is the core determinant of user adoption.
In the field of banking, scholars have demonstrated a significant correlation between ease of use and new technology adoption. The perceived usefulness significantly affected user attitudes and willingness to adopt Fintech when users used complex information systems to conduct financial transactions via mobile devices (Riquelme and Rios, 2010). If users perceived that Fintech services were convenient, user-friendly, and easy to operate, they tended to accept them more (López-Nicolás et al., 2008; Dulcic et al., 2012; Kim and Woo, 2016). All TAM, TPB (planned behavior theory) and DTPB (decomposition theory of planned behavior) model agreed that "ease of use" had positive impact on the perceived usefulness.
Hypothesis H5: Perception of ease of use positively affects customers' attitude to use banking-Fintech products and services.
Perception of risk is a form of mistrust and most scholars believe that perception of financial risk and privacy are major factors that negatively influence customers' technology adoption. Financial risk refers to a consumer's property loss. Privacy risk refers to the risk that personal and transactional data as well as other confidential information are disclosed when consumers use financial products through the Internet. Risk awareness was the most important factor influencing the adoption of electronic services (Liebermann and Stashevsky, 2002; Forsythe and Shi, 2003; Kesharwani and Singh Bisht, 2012; Munoz-Leiva et al., 2017).
Hypothesis H6: Perception of risk adversely affects customers' attitude to use banking-fintech combined high-tech products.
User innovativeness or trendiness is defined as the degree of early acceptance of innovation; The degree to which individual users tend to try new products, new technologies or services. When individuals are highly innovative, they are less risk-averse and more receptive to technological innovation (Aldás-Manzano, et al. 2009; Ratten, 2014; Thakur and Srivastava, 2015).
Hypothesis H7: Perception of contemporary when using new products and/or services positively affects customers' attitude to use high-tech products combined between the bank and Fintech.
Motivation and the possibility of cooperation between the banks and fintech companies not only depend on their own needs but also on the customers' acceptance of their joint products. The more the intention to use the joint products, the higher the possibility of the actual consumption of the end users. The research plans to conduct a customer survey in order to analyze the collected data to identify the factors affecting the intention to use the bank-fintech high-tech products. The findings of the research provide the empirical evidence for banks and fintech companies to act on the critical factors to facilitate the intention to use the high-tech products.
The research model is built based on TAM. However, in addition to the two variables: perceived usefulness and perceived ease of usein the original TAM, this study identifies additional factors such as brand image, trust, user innovativeness, perceived risk identified from recent studies on acceptance behavior of customer in the 3.0 and 4.0 technology era; to determine the suitable financial products offered from the partnership betweenbanks and fintech, as well as consumer attitudes influenced by each factor. Furthermore, this study also investigates how customers' attitude can have an impact on their intention to use fintech products. With the expanded TAM model, the research provides more comprehensive empirical evidence to help banks and Fintechs's decision makers to promoteon the factors that can help improve the number of customers using high-tech products offered by banks and fintechs' partnerships.
The measurement of items is adopted from previous studies (Table 1). The variables of all items are measured on five-point Likert scale. The scale ranges from 1 which is strongly disagree to 5 for strongly agree.
Code | Variables | References |
Brand Image: BI | ||
BI1 | I choose these banking-Fintech products because the bank always provides good products and services. | (Ruparelia et al., 2010; Ha, 2004) |
BI2 | I choose these banking-Fintech products because the bank is familiar to me. | |
BI3 | I choose to use these banking-Fintech products because the bank has a good reputation. | |
TRUST | ||
TRU1 | Banking - Fintech products keep personal information secure. | (Chong et al., 2010; Sanchez et al., 2018) |
TRU2 | In general, Banking - Fintech products are reliable. | |
Perceived usefulness- PU | ||
PU1 | I can achieve my need using Banking-Fintech products. | (Lockett & Littler, 1997; Huh et al., 2009) |
PU2 | Using Banking-Fintech products help me save time in transactions. | |
PU3 | Banking-Fintech products increase transaction efficiency compared to traditional banking products. | |
PU4 | The association with Fintech helps banking products increase usefulness. | |
Perceived ease of use (PEU) | ||
PEU1 | Banking-Fintech products are easy to use. | (Cheng et al., 2006; Wang et al., 2003) |
PEU2 | Banking-Fintech products' interface is easy to understand. | |
PEU3 | Banking-Fintech products are easily used on available devices (smart phone, App, wifi...). | |
Perceived risk (PR) | ||
PR1 | I am afraid money is easily stolen from my bank account. | |
PR2 | I am afraid personal information is easily disclosed when using banking-Fintech products. | |
PR 3 | Overall, I feel insecure when using banking-Fintech products. | |
User innovativeness (UI) | ||
UI1 | When I heard about a new product, I would try it. | (Zhang et al., 2018) |
UI2 | I am usually the pioneer to try a new product. | |
Attitude (ATT) | ||
ATT1 | I believe using banking-Fintech products is a good idea. | (Grabner & Faullant, 2008) |
ATT2 | Using banking-Fintech products brings me a feeling of discovery. | |
ATT3 | I am interested in banking-Fintech products. | |
Intention to Use (ITU) | ||
INT1 | I will be using banking-Fintech products soon. | (Marakarkandy et al., 2017; Patel & Patel, 2018) |
INT2 | I am willing to keep using banking-Fintech products. | |
INT3 | I will recommend my friends to use banking-Fintech products. |
Field data collection is handled by sending questionnaires randomly and directly to those who agree to participate, as well via online Google document. The total number of returned questionnaires is 254 from 300 sent out. To improve the data reliability, questionnaires with only one option selected throughout or missing three consecutive answers discarded. After screening the returned questionnaires, 234 valid ones are utitlized for data analysis. Among 234 observations, there are 134 female customers and 101 male customers.
Data collected from 234 customers are used to test the measurement scales of the constructs in the research study, inspecting reliability with Cronbach alpha and their underlying relationships with exploratory factor analysis. After the tests, the data and the measurement scales will be use to test the research model and confirm the research hypotheses using AMOS 18.
Research to test scale reliability by analyzing Conbach alpha, composite reliability—ρc, and variance extracted—ρvc; convergence and discriminant of the scale of research concepts by exploratory factor analysis (EFA) and Confirmatory Factor Analysis (CFA).
To test the scale reliability, Cronbach alpha is employed. The results indicate that the alphas of all constructs are greater than 0.7 and item-total relationships are all greater than 0.3. Hence, the measurement scales are highly reliable. With the sample of 234, all 20 measurement variables are the inputs for exploratory factor analysis. With the use of Equamax rotation, the variable BI1 used for measuring BI is loaded into three constructs and its weights at the three constructs are not much different. Similarly, the observed variables P1 and ATT1 are not converged at the intended constructs. To increase the convergence of these items, BI1, P1 and ATT1 are omitted from measuring brand image, perceived usefulness and customer attitude. EFA outputs after dropping P1, BI1 and ATT1 indicate the acceptable levels of convergence.
The measurement scales are next tested with Confirmatory Factor Analysis CFA. The results include CMIN/DF = 1.535 < 3; RMSEA = 0.048 < 0.08; TLI = 0.957 and CFI = 0.971 (both greater than 0.9 and less than 1), therefore, it indicates the fit between observed data and the conceptualized model (Bentler and Bonett, 1980; Carmines and McIver, 1983; Steiger, 1990).
Standardized regression weights (Table 2) indicate that all standardized weights of the variables are over 0.5 and statistically significant. Therefore, the measurement scales of the research constructs all satisfy convergent validity (Gerbing and Anderson, 1988).
Estimate | Estimate | ||||||
ITU2 | < --- | ITU | 0.826 | P4 | < --- | PU | 0.788 |
ITU1 | < --- | ITU | 0.827 | P2 | < --- | PU | 0.840 |
ITU3 | < --- | ITU | 0.841 | Trust1 | < --- | TRUST | 0.827 |
PR2 | < --- | PR | 0.843 | Trust2 | < --- | TRUST | 0.883 |
PR1 | < --- | PR | 0.775 | Per2 | < --- | UI | 0.900 |
PR3 | < --- | PR | 0.690 | Per1 | < --- | UI | 0.870 |
Conv1 | < --- | PEU | 0.822 | ATT2 | < --- | Attitude | 0.853 |
Conv3 | < --- | PEU | 0.778 | ATT3 | < --- | Attitude | 0.867 |
Conv2 | < --- | PEU | 0.871 | BI3 | < --- | BI | 0.632 |
P3 | < --- | PU | 0.793 | BI2 | < --- | BI | 0.708 |
The outputs of the tests of correlation between observed variables and the constructs are given in Appendix 1. The correlation coefficients among research constructs are different from 1 and the standard errors are all less than 1 and statistically significant. There fore, the discriminant validity is secured.
The test for reliability is conducted with three indicators: Cronbach alpha, composite reliability—ρc, and variance extracted—ρvc. From the CFA outpus of AMOS software of the saturated model and Cronbach alpha calculated with SPSS software, the authors use Excel to calculate ρc, ρvc and Cronbach alpha (Appendix 2). It is evident that the scales satisfy reliability when ρc, ρvc are greater than 0.5 and ρc ≥ Cronbach alpha (Hair, 1998).
The scales for ITU, Trust, Conv, ATT, BI satisfy reliability as ρc and ρvc are greater than 0.5 and composite reliability ρc ≥ Cronbach alpha. As for PR, P and Per, they have ρc close to Cronbach alpha, but the differences are not much so their scales satisfy composite reliability. All 20 observed variables measuring 8 constructs are then used to test the research model and the research hypotheses with AMOS 18 software.
The measurement scales for the research constructs fitting the content validity, convergent validity and discriminant validity as well as reliability, are used to test the theoretical model with the technique of structural equation modeling and AMOS 18.
The estimates (standardized) of the parameters of the official research model are presented in Table 2. The relationships among the constructs of the model are all statistically significant (p < 0.05).
The non-standardized results indicate that perceived usefulness, trust, and user innovativeness influence their behavior with confidence level of 95%. The brand image (BI) positively influences the behavior at 94% confidence level. There is no evidence on the effect of convenience and perceived risk on the customers Attitude on the joint products between banks and fintech companies. The results also show that attitude positively affects the intention to use the product, which is statistically significant p = 1% (see Table 3).
Estimate regression weights | S0.E0. | C0.R0. | P | ||||
Unstandardized | Standardized | ||||||
ATT | < --- | BI | 0.455 | 0.371 | 0.247 | 10.843 | 0.065 |
ATT | < --- | UI | 0.112 | 0.163 | 0.055 | 20.056 | 0.040 |
ATT | < --- | TRUST | 0.207 | 0.251 | 0.077 | 20.698 | 0.007 |
ATT | < --- | PU | 0.561 | 0.538 | 0.132 | 40.231 | *** |
ATT | < --- | PR | −0.045 | −0.063 | 0.041 | −10.098 | 0.272 |
ATT | < --- | CONV | −0.201 | −0.204 | 0.126 | −10.596 | 0.110 |
ITU | < --- | ATT | 0.835 | 0.829 | 0.071 | 110.778 | *** |
The econometric model can be summarized as following:
Y=α+βiXi+ϵ | (1) |
where: i represents the number of independent variables.
Y is the intention to use financial products and services of customers.
Xi represents specific independent variables such as brand image (BI), user innovativeness (UI), customers' trust (TRUST), perceived usefulness (PU), perceived risk (PR), convenience (CONV) and customers' attitude (ATT).
The standardized regressions (Table 2) indicate that the image of the bank influence the behavior of the customers towards the product (β = 0.371, p < 0.05). The hypothesis H1 is then accepted. So, the Vietnamese customers are more concerned about the image of the fintech service suppliers. This fits with what happen in reality in Vietnam when technology products provided by fintech companies are not yet popular, especially to those of middle age and older. As per documented in "Vietnam Fintech Report 2020", 72% of fintech companies in Vietnam have joined force with banks to provide services of payment, P2C, P2P, C2C, B2B rather than having decided to become their direct competitors. This is a sensible decision because fintech companies are new, so the customers may not be ready to use their services. When contemplating the possible usage, the customers look for information from the existing providers of similar services or from those who had collaborating relationships with fintech companies. The search words for fintech products leading to the webpage of a bank may strengthen the trust for the customers about the products. From this reasoning, when the customers are confused about the use of a new high-tech product, if it is provided by a bank with positive branding impression, behavior intention would increase and the possibility of actual use of the product would increase as well. Ratnasingham (2003) also points out that when product information has not been verified by consumers, brands would help them to make decision. The customers select the high-tech products of the bank when they had positively experienced other services that the bank supplied. There are also cases when the customers decide to use the joint products of banks in collaborating with fintech companies because of the reputation of the banks.
The findings also reveal the impact of customer trust on behavior intention (β = 0.251, p < 0.05), and consequently on usage intention (β = 0.829, p < 0.05). The hypothesis H2 is then accepted. Given the characteristics of the high-tech joint products provided by banks and fintech companies, the customers tend to have positive attitude towards the product and then increase the intention use them (Kesharwani and Singh Bisht, 2012; Malaquias and Hwang, 2016). So, for customers to accept and select to use the joint products, both banks and fintech companies have to make sure that the customers feel secure for personal information, trust the commitment of the products to the features of their products.
With the estimated β = 0.538 and significant level p < 0.05, hypothesis H3 is accepted. The usefulness of the product influences the most on behavior then positively influences the behavior intention (β = 0.829; pvalue < 0.05). The customers find a high-tech product helpful for work efficiency and of essential benefits, they in turn would have positive behavior on the product and increase intention to use it. Therefore, to attract the customers to use the high-tech products, banks and fintech companies have to focus on the benefits of the products brought to the customers. The fintech companies can take advantage of regulation on KYC that the state bank requires the commercial banks to obtain wet signatures of the customers in their initial transactions with the banks or when having special requests. The customers in these cases have to go to the branches of the banks to provide information verified by manual signatures. However, the fintech companies are not regulated by the stipulation on KYC in verifying wet signature, and even can forgo a signature. Then, when using the joint products of fintech companies and banks, the customers can have confidence in using the bank services, at the same time can benefit from the advantages of the product offered by fintech companies.
Research findings identify no evidence of the impact of convenience and perceived risks on customer behaviors (coefficients and significance levels for convenience and perceived risks are respectively β = −0.201; pvalue = 0.11 and β = −0.045; pvalue = 0.272). The consumers may have already felt that convenience improves product usefulness. For fintech products, the consumers fear the financial risks and privacy risks so the positive behavior and intention to use decrease (Bansal et al., 2010; Khedmatgozar and Shahnazi, 2018). However, for the joint products offered by banks and fintech companies, perceived risks seem not to influence behavior and intention of use as the customers tend to assume that they are using the products provided by the banks (instead of the fintech partner which they might not be aware of) with high level of security.
Similar to the findings of Leicht et al. (2018), Hu et al. (2019), the standardized regression weights show that user innovativeness positively affects behavior (β = 0.163; pvalue < 0.05). Hypothesis H6 is then accepted. So, when the customers have enough understanding and can easily approach and accept technology change (higher user innovativeness), their behavior towards the high-tech products jointly offered by banks and fintech companies changes positively. Intention to use then also increases. It is an advantage for fintech companies and banks when the Global Innovation Index 2020 of Vietnam ranks 42 in the world and places 3rd among Asean countries (WIPO, 2020). Besides, by 2020 Vietnam with population of 97.4 million, has internet covering 52% of the population, and 45% of its people are using smart phones (Fintech Singapore, 2020). Therefore, with high innovation index, high percentage of internet and smart phone penetration, Vietnam is a lucrative market for fintech companies to enjoy.
The research study reveals that the customers have positive behaviors and increased intention to use for the products which are practical, time-saving and cost-saving. When the customers have confidence about security and personal information confidentiality, they feel positive about the product and increase their intention to use. Besides, comments about the images of the banks and fintech companies also influence the behavior and intention to use. The higher the customers appreciate the image of the banks and fintech companies, the more positive feelings they have towards the products offered by the banks and fintech companies, and the intention to use increases. In addition, the customers would have no concerns about trying the products if they are more adventurous and curious about the products on smartphones. Perceived risks and convenience do not influence behavior and then have no impact on intention to use the products integrated to banks' products or distributed via the banking channels. Therefore, in order to attract the customers to products jointly provided by banks and fintech companies, both need to pay attention to products' practicality, create trust, develop positive brands and images, and focus the managerial efforts on the younger customers who prefer to explore and experience.
This work was supported by The Ministry of Education and Training of Vietnam; Approved Research Proposal number 2019-KSA-01.
The authors declare no conflicts of interest in this paper.
[1] |
Abbad MM (2013) E-banking in Jordan. Behav Inform Technol 32: 681-694. doi: 10.1080/0144929X.2011.586725
![]() |
[2] | Aboelmaged MGG, Gebba TR (2013) Mobile banking adoption: an examination of technology acceptance model and theory of planned behavior. Int J Bus Res Dev 2: 35-50. |
[3] |
Ajzen I (1991) The theory of planned behaviour. Organ Behav Hum Decis Process 50: 179-211. doi: 10.1016/0749-5978(91)90020-T
![]() |
[4] |
Ajzen I (2011) The theory of planned behaviour: reactions and reflections. Psychol Health 26: 1113-1127. doi: 10.1080/08870446.2011.613995
![]() |
[5] | Alaeddin O, Altounjy R (2018) Trust, technology awareness and satisfaction effect into the intention to use cryptocurrency among generation Z in Malaysia. Int J Eng Technol 7: 8-10. |
[6] |
Aldás-Manzano J, Lassala-Navarre C, Ruiz-Mafe C, et al. (2009) The role of consumer innovativeness and perceived risk in online banking usage. Int J Bank Mark 27: 53-75. doi: 10.1108/02652320910928245
![]() |
[7] | Anderson JC, Gerbing DW (1988) Structural equation modeling in practice: A review and recommended two-step approach. Psychol Bull 103: 411. |
[8] | Azjen I, Fishbein M (1980) Understanding attitudes and predicting social behavior, Englewood Cliffs, NJ: Prentice-Hall. |
[9] |
Bailey AA, Pentina I, Mishra AS, et al. (2017) Mobile payments adoption by US consumers: an extended TAM. Int J Retail Distrib Manage 45: 626-640. doi: 10.1108/IJRDM-08-2016-0144
![]() |
[10] | Bansal S, Bansal A, Blake MB (2010) Trust-based dynamic web service composition using social network analysis, 2010 IEEE International Workshop on: Business Applications of Social Network Analysis (BASNA), 1-8. |
[11] |
Bentler PM, Bonett DG (1980) Significance tests and goodness of fit in the analysis of covariance structures. Psychol Bull 88: 588-606. doi: 10.1037/0033-2909.88.3.588
![]() |
[12] | Carmines EG, McIver JP (1983) An introduction to the analysis of models with unobserved variables. Political Methodol 9: 51-102. |
[13] | Cham TH, Low SC, Lim CS, et al. (2018) Preliminary study on consumer attitude towards fintech products and services in Malaysia. Int J Eng Technol 7: 166-169. |
[14] | Chang YP, Lan LY, Zhu DH (2018) Understanding the intention to continue use a mobile payment. Int J Bus Inform 12: 363-390. |
[15] |
Chau PY, Hu PJ (2002) Examining a model of information technology acceptance by individual professionals: an exploratory study. J Manage Inform Syst 18: 191-229. doi: 10.1080/07421222.2002.11045699
![]() |
[16] |
Chen JF, Chang JF, Kao CW, et al. (2016) Integrating ISSM into TAM to enhance digital library services. Electron Lib 34: 58-73. doi: 10.1108/EL-01-2014-0016
![]() |
[17] |
Cheng TE, Lam DY, Yeung AC (2006) Adoption of internet banking: an empirical study in Hong Kong. Decis Support Syst 42: 1558-1572. doi: 10.1016/j.dss.2006.01.002
![]() |
[18] |
Chong A, Ooi K, Lin B, et al. (2010) Online banking adoption: an empirical analysis. Int J Bank Mark 28: 267-287. doi: 10.1108/02652321011054963
![]() |
[19] |
Chua CJ, Lim CS, Aye AK (2019) Factors affecting the consumer acceptance towards fintech products and services in Malaysia. Int J Asian Soc Sci 9: 59-65. doi: 10.18488/journal.1.2019.91.59.65
![]() |
[20] | Chuang LM, Liu CC, Kao HK (2016) The adoption of fintech service: TAM perspective. Int J Manage Admin Sci 3: 1-15. |
[21] | Chuen DLK (2015) Handbook of Digital Currency: Bitcoin, Innovation, Financial Instruments, and Big Data, Academic Press. |
[22] |
Datta PA (2011) Preliminary study of ecommerce adoption in developing countries. Inform Syst J 21: 3-32. doi: 10.1111/j.1365-2575.2009.00344.x
![]() |
[23] |
Davis FD, Bagozzi RP, Warshaw PR (1989) User acceptance of computer technology: a comparison of two theoretical models. Manage Sci 35: 982-1003. doi: 10.1287/mnsc.35.8.982
![]() |
[24] |
Demoulin NTM, Djelassi S (2016) An integrated model of self-service technology (SST) usage in a retail context. Int J Retail Distrib Manage 44: 540-559. doi: 10.1108/IJRDM-08-2015-0122
![]() |
[25] |
Devlin JF (1997) Adding value to retail financial services. J Mark Pract 3: 251-267. doi: 10.1108/EUM0000000004461
![]() |
[26] |
Donner J, Tellez CA (2008) Mobile banking and economic development: Linking adoption, impact, and use. Asian J Commun 18: 318-332. doi: 10.1080/01292980802344190
![]() |
[27] |
Dulcic Z, Pavlic D, Silic I (2012) Evaluating the intended use of decision support system (DSS) by applying technology acceptance model (TAM) in business organizations in Croatia. Procedia-Soc Behav Sci 58: 1565-1575. doi: 10.1016/j.sbspro.2012.09.1143
![]() |
[28] | 2020 Fintech Vietnam Report and Startup Map: Fintech startups Tripled since 2017, 2020. Fintech Singapore, 2020. Available from: https://fintechnews.sg/45354/vietnam/2020-fintech-vietnam-report-and-startup-map/. |
[29] | Fishbein M, Ajzen I (1977) Belief, attitude, intention, and behavior: an introduction to theory and research. Contemp Sociol 6 : 244. |
[30] |
Forsythe SM, Shi B (2003) Consumer patronage and risk perceptions in Internet shopping. J Bus Res 56: 867-875. doi: 10.1016/S0148-2963(01)00273-9
![]() |
[31] | FSB (2019) Financial Stability Implications from Fintech. Financial Stability Board. |
[32] | Global Innovation Index 2020. World Intellectual Property Organization, 2020. Available from: https://www.wipo.int/global_innovation_index/en/2020/. |
[33] |
Grabner-Kräuter S, Faullant R (2008) Consumer acceptance of Internet banking: the influence of internet trust. Int J Bank Mark 26: 483-504. doi: 10.1108/02652320810913855
![]() |
[34] | Group VMW (2018) Report "Application of Financial Technology (Fintech) in Microfinance activities towards Universal Finance in Vietnam", Vietnam. |
[35] |
Gupta A, Arora N (2017) Consumer adoption of m-banking: a behavioral reasoning theory perspective. Int J Bank Mark 35: 733-747. doi: 10.1108/IJBM-11-2016-0162
![]() |
[36] | Ha H (2004) Factors influencing consumer perceptions of brand trust online J Prod Brand Manage 13: 329-342. |
[37] | Hair JF (1998) Multivariate data analysis, Upper Saddle River, N.J., Prentice Hall. |
[38] |
Halilovic S, Cicic M (2013) Antecedents of information systems user behavior-extended expectation-confirmation model. Behav Inform Technol 32: 359-370. doi: 10.1080/0144929X.2011.554575
![]() |
[39] |
Hamidi H, Safareeyeh M (2019) A model to analyze the effect of mobile banking adoption on customer interaction and satisfaction: a case study of m-banking in Iran. Telematics Inform 38: 166-181. doi: 10.1016/j.tele.2018.09.008
![]() |
[40] |
Hanafizadeh P, Behboudi M, Koshksaray AA, et al. (2014) Mobile-banking adoption by Iranian bank clients. Telematics Inform 31: 62-78. doi: 10.1016/j.tele.2012.11.001
![]() |
[41] | Hu Z, Ding S, Li S, et al. (2019) Adoption intention of fintech services for bank users: An empirical examination with an extended technology acceptance model. Symmetry 11: 340. |
[42] |
Huh HJ, Kim TT, Law R (2009) A comparison of competing theoretical models for understanding acceptance behavior of information systems in upscale hotels. Int J Hosp Manage 28: 121-134. doi: 10.1016/j.ijhm.2008.06.004
![]() |
[43] | Jiwasiddi A, Adhikara C, Adam M, et al. (2019) Attitude toward using Fintech among Millennials. The 1st Workshop on Multimedia Education, Learning, Assessment and its Implementation in Game and Gamification in conjunction with COMDEV 2018, European Alliance for Innovation (EAI). |
[44] | Jonker N (2019) What drives the adoption of crypto-payments by online retailers? Electron Comm Res Appl 35: 100848. |
[45] |
Kapoor K, Dwivedi Y, Piercy NC, et al. (2014) RFID integrated systems in libraries: extending TAM model for empirically examining the use. J Enterp Inform Manage 27: 731-758. doi: 10.1108/JEIM-10-2013-0079
![]() |
[46] |
Kesharwani A, Singh Bisht S (2012) The impact of trust and perceived risk on internet banking adoption in India: An extension of technology acceptance model. Int J Bank Mark 30: 303-322. doi: 10.1108/02652321211236923
![]() |
[47] |
Khedmatgozar HR, Shahnazi A (2018) The role of dimensions of perceived risk in adoption of corporate internet banking by customers in Iran. Electron Comm Res 18: 389-412. doi: 10.1007/s10660-017-9253-z
![]() |
[48] | Khodabandeh A, Lindh C (2020) The importance of brands, commitment, and influencers on purchase intent in the context of online relationships. Australas Mark J. |
[49] |
Kim S (2012) Factors affecting the use of social software: TAM perspectives. Electron Lib 30: 690-706. doi: 10.1108/02640471211275729
![]() |
[50] |
Kim YG, Woo E (2016) Consumer acceptance of a quick response (QR) code for the food traceability system: application of an extended technology acceptance model (TAM). Food Res Int 85: 266-272. doi: 10.1016/j.foodres.2016.05.002
![]() |
[51] |
Kim YJ, Park YJ, Choi J, et al. (2015) An empirical study on the adoption of "fintech" service: focused on mobile payment services. Adv Sci Technol Lett 114: 136-140. doi: 10.14257/astl.2015.114.26
![]() |
[52] |
Koksal MH (2016) The intentions of Lebanese consumers to adopt mobile banking. Int J Bank Mark 34: 327-346. doi: 10.1108/IJBM-03-2015-0025
![]() |
[53] |
Koufaris M (2002) Applying the technology acceptance model and flow theory to online consumer behavior. Inform Syst Res 13: 205-223. doi: 10.1287/isre.13.2.205.83
![]() |
[54] |
Lee DY, Lehto MR (2013) User acceptance of YouTube for procedural learning: an extension of the technology acceptance model. Comput Educ 61: 193-208. doi: 10.1016/j.compedu.2012.10.001
![]() |
[55] |
Lee MKO, Turban E (2001) A trust model for consumer internet shopping. Int J Electron Comm 6: 75-91. doi: 10.1080/10864415.2001.11044227
![]() |
[56] |
Leicht T, Chtourou A, Youssef KB (2018) Consumer innovativeness and intentioned autonomous car adoption. J High Technol Manage Res 29: 1-11. doi: 10.1016/j.hitech.2018.04.001
![]() |
[57] |
Liebermann Y, Stashevsky S (2002) Perceived risks as barriers to Internet and e-commerce usage. Qual Mark Res 5: 291-300. doi: 10.1108/13522750210443245
![]() |
[58] |
Lien NTK, Thi Doan TT, Bui TN (2020) Fintech and banking: evidence from Vietnam. J Asian Financ Econ Bus 7: 419-426. doi: 10.13106/jafeb.2020.vol7.no9.419
![]() |
[59] |
Lin WR, Wang YH, Hung YM (2020) Analyzing the factors influencing adoption intention of internet banking: Applying DEMATEL-ANP-SEM approach. PlOS ONE 15: e0227852. doi: 10.1371/journal.pone.0227852
![]() |
[60] |
López-Nicolás C, Molina-Castillo FJ, Bouwman H (2008) An assessment of advanced mobile services acceptance: Contributions from TAM and diffusion theory models. Inform Manage 45: 359-364. doi: 10.1016/j.im.2008.05.001
![]() |
[61] |
Lockett A, Littler D (1997) The adoption of direct banking services. J Mark Manage 13: 791-811. doi: 10.1080/0267257X.1997.9964512
![]() |
[62] |
Malaquias RF, Hwang Y (2016) An empirical study on trust in mobile banking: A developing country perspective. Comput Hum Behav 54: 453-461. doi: 10.1016/j.chb.2015.08.039
![]() |
[63] |
Mallat N, Rossi M, Tuunainen VK (2009) The impact of use context on mobile services acceptance: The case of mobile ticketing. Inform Manage 46: 190-195. doi: 10.1016/j.im.2008.11.008
![]() |
[64] |
Manrai LA, Manrai AK (2007) A field study of customers' switching behavior for bank services. J Retailing Consum Serv 14: 208-215. doi: 10.1016/j.jretconser.2006.09.005
![]() |
[65] |
Marakarkandy B, Yajnik N, Dasgupta C (2017) Enabling internet banking adoption: an empirical examination with an augmented technology acceptance model (Tam). J Enterp Inform Manage 30: 263-294. doi: 10.1108/JEIM-10-2015-0094
![]() |
[66] |
Martins C, Oliveira T, Popovič A (2014) Understanding the Internet banking adoption: A unified theory of acceptance and use of technology and perceived risk application. Int J Inform Manage 34: 1-13. doi: 10.1016/j.ijinfomgt.2013.06.002
![]() |
[67] |
McKnight DH, Chervany NL (2001) What trust means in e-commerce customer relationships: An interdisciplinary conceptual typology. Int J Electron Comm 6: 35-59. doi: 10.1080/10864415.2001.11044235
![]() |
[68] |
Meyliana M, Fernando E, Surjandy S (2019) The influence of perceived risk and trust in adoption of fintech services in Indonesia. CommIT J 13: 31-37. doi: 10.21512/commit.v13i1.5708
![]() |
[69] | Miller K (2005) Communication theories, USA: Macgraw-Hill. |
[70] |
Moon JW, Kim YG (2001) Extending the TAM for a World-Wide-Web context. Inform Manage 38: 217-230. doi: 10.1016/S0378-7206(00)00061-6
![]() |
[71] | Munoz-Leiva F, Climent-Climent S, Liébana-Cabanillas F (2017) Determinants of intention to use the mobile banking apps: An extension of the classic TAM model. Span J Mark-ESIC 21: 25-38. |
[72] |
Ng AW, Kwok BKB (2017) Emergence of Fintech and cybersecurity in a global financial centre: Strategic approach by a regulator. J Financ Regul Compliance 25: 422-434. doi: 10.1108/JFRC-01-2017-0013
![]() |
[73] |
Olson JM, Zanna MP (1993) Attitudes and attitude change. Annu Rev Psycho 44: 117-154. doi: 10.1146/annurev.ps.44.020193.001001
![]() |
[74] |
Patel KJ, Patel HJ (2018) Adoption of internet banking services in Gujarat: An extension of TAM with perceived security and social influence. Int J Bank Mark 36: 147-169. doi: 10.1108/IJBM-08-2016-0104
![]() |
[75] | Pauline R (2003) Inter-Organizational-Trust in Business to Business E-Commerce: A Case Study in Customs Clearance. J Glob Inform Manage 11: 1-19. |
[76] |
Phan DTT, Nguyen TTH, Bui TA (2019) Going beyond border? Intention to use international bank cards in Vietnam. J Asian Financ Econ Bus 6: 315-325. doi: 10.13106/jafeb.2019.vol6.no3.315
![]() |
[77] |
Qiu LY, Li D (2008) Applying TAM in B2C E-commerce research: An extended model. Tsinghua Sci Technol 13: 265-272. doi: 10.1016/S1007-0214(08)70043-9
![]() |
[78] | Ratnasingam P (2003) Inter-organizational-trust in business to business e-commerce: A case study in customs clearance. J Glob Inform Manage 11: 1-19. |
[79] | Ratten V (2014) A US-China comparative study of cloud computing adoption behavior: The role of consumer innovativeness, performance expectations and social influence. J Entrepreneurship Emerg Econ 6: 53-71. |
[80] |
Riquelme EH, Rios RE (2010) The moderating effect of gender in the adoption of mobile banking. Int J Bank Mark 28: 328-341. doi: 10.1108/02652321011064872
![]() |
[81] | Romānova I, Kudinska M (2016) Banking and fintech: A challenge or opportunity? Contemp Stud Econ Financ Anal 98: 21-35. |
[82] |
Ruparelia N, White L, Hughes K (2010) Drivers of brand trust in internet retailing. J Prod Brand Manage 19: 250-260. doi: 10.1108/10610421011059577
![]() |
[83] |
Sánchez-Torres JA, Canada FJA, Sandoval AV, et al. (2018) E-banking in Colombia: factors favouring its acceptance, online trust and government support. Int J Bank Mark 36: 170-183. doi: 10.1108/IJBM-10-2016-0145
![]() |
[84] |
Shaikh IM, Qureshi MA, Noordin K, et al. (2020) Acceptance of Islamic financial technology (FinTech) banking services by Malaysian users: an extension of technology acceptance model. Foresight 22: 367-383. doi: 10.1108/FS-12-2019-0105
![]() |
[85] | Smith AA, Synowka DP, Smith AD (2014) E-commerce quality and adoptive elements of e-ticketing for entertainment and sporting events. Int J Bus Inform Syst 15: 450-487. |
[86] |
Steiger JH (1990) Structural model evaluation and modification: an interval estimation approach. Multivar Behav Res 25: 173-180. doi: 10.1207/s15327906mbr2502_4
![]() |
[87] |
Stewart H, Jürjens J (2018) Data security and consumer trust in FinTech innovation in Germany. Inform Comput Secur 26: 109-128. doi: 10.1108/ICS-06-2017-0039
![]() |
[88] |
Stocchi L, Michaelidou N, Micevski M (2019) Drivers and outcomes of branded mobile app usage intention. J Prod Brand Manage 28: 28-49. doi: 10.1108/JPBM-02-2017-1436
![]() |
[89] |
Teo T, Lee BC, Chai CS, et al. (2009) Assessing the intention to use technology among pre-service teachers in Singapore and Malaysia: A multigroup invariance analysis of the Technology Acceptance Model (TAM). Comput Educ 53: 1000-1009. doi: 10.1016/j.compedu.2009.05.017
![]() |
[90] |
Thakur R, Srivastava M (2015) A study on the impact of consumer risk perception and innovativeness on online shopping in India. Int J Retail Distrib Manage 43: 148-166. doi: 10.1108/IJRDM-06-2013-0128
![]() |
[91] |
Venkatesh V, Davis FD (2000) A theoretical extension of the technology acceptance model: Four longitudinal field studies. Manage Sci 46: 186-204. doi: 10.1287/mnsc.46.2.186.11926
![]() |
[92] |
Venkatesh V, Morris MG, Davis GB, et al. (2003) User acceptance of information technology: toward a unified view. MIS Quart 27: 425-478. doi: 10.2307/30036540
![]() |
[93] | Vietnam Fintech Report 2020. Fintech Singapore, 2020. Available from: https://fintechnews.sg/wp-content/uploads/2020/11/Vietnam-Fintech-Report-2020.pdf. |
[94] | Vietnam Microfinance Working Group. Annual Report, 2018. Available from: https://microfinance.vn/wp-content/uploads/2019/06/gg.pdf. |
[95] |
Wallace LG, Sheetz SD (2014) The adoption of software measures: A technology acceptance model (TAM) perspective. Inform Manage 51: 249-259. doi: 10.1016/j.im.2013.12.003
![]() |
[96] |
Wang WT, Li HM (2012) Factors influencing mobile services adoption: A brand-equity perspective. Internet Res 22: 142-179. doi: 10.1108/10662241211214548
![]() |
[97] |
Wang Y, Wang Y, Lin H, et al. (2003) Determinants of user acceptance of Internet banking: An empirical study. Int J Serv Ind Manage 14: 501-519. doi: 10.1108/09564230310500192
![]() |
[98] |
Wonglimpiyarat J (2017) FinTech banking industry: a systemic approach. Foresight 19: 590-603. doi: 10.1108/FS-07-2017-0026
![]() |
[99] |
Wu JH, Wang SC (2005) What drives mobile commerce?: An empirical evaluation of the revised technology acceptance model. Inform Manage 42: 719-729. doi: 10.1016/j.im.2004.07.001
![]() |
[100] |
Yang HD, Yoo Y (2004) It's all about attitude: revisiting the technology acceptance model. Decis Support Syst 38: 19-31. doi: 10.1016/S0167-9236(03)00062-9
![]() |
[101] | Zhang T, Lu C, Kizildag M (2018) Banking "on-the-go": examining consumers' adoption of mobile banking services. Int J Qual Serv Sci 10: 279-295. |
[102] | Zhou L, Yang D (2011) An enterprise mobile e-business system model based on pushmail, 2011 IEEE 2nd International Conference on Software Engineering and Service Science, 730-733. |
![]() |
![]() |
1. | T.N. Mursitama, D.N. Utama, S.A. Abrori, The Impact of Digital Literacyon Fintech Service Usage Through Financial Literacy, 2023, 426, 2267-1242, 02003, 10.1051/e3sconf/202342602003 | |
2. | Evan Hamzah Muchtar, Budi Trianto, Irwan Maulana, Muhammad Nurul Alim, Ruslan Husen Marasabessy, Wahyu Hidayat, Edy Junaedi, , Quick response code Indonesia standard (QRIS) E-payment adoption: customers perspective, 2024, 11, 2331-1975, 10.1080/23311975.2024.2316044 | |
3. | Erick Fernando, Yulius Denny Prabowo, , 2024, The Impact of Social Media in Marketing on Brand Awareness and Customer Trust in Increasing Purchase Intention, 979-8-3503-7621-0, 335, 10.1109/ICITRI62858.2024.10698890 | |
4. | Winanti Winanti, Erick Fernando, The Role of Brand Image and Trust in the Adoption of FinTech Digital Payment for Online Transportation, 2024, 10, 2443-2555, 126, 10.20473/jisebi.10.1.126-138 | |
5. | Erwin Halim, Nadia Salsabila, Zilvian Zidane, Placide Poba-Nzaou, 2023, Exploring the Success Factors Digital Lending: An Approach from Consumer's Perspective, 979-8-3503-2776-2, 460, 10.1109/ICE3IS59323.2023.10335330 | |
6. | Xiaoxiao Liu, Ka Yin Chau, Xiaoyun Liu, Fei Huang, The Determinants of Customer Intentions to Use Fintech Services in a Commercial Chinese Bank, 2023, 30, 1051-712X, 257, 10.1080/1051712X.2023.2248970 | |
7. | Pranay Khatiwara, Anindita Adhikary, Ajeya Jha, 2025, Chapter 25, 978-981-97-6464-8, 311, 10.1007/978-981-97-6465-5_25 |
Code | Variables | References |
Brand Image: BI | ||
BI1 | I choose these banking-Fintech products because the bank always provides good products and services. | (Ruparelia et al., 2010; Ha, 2004) |
BI2 | I choose these banking-Fintech products because the bank is familiar to me. | |
BI3 | I choose to use these banking-Fintech products because the bank has a good reputation. | |
TRUST | ||
TRU1 | Banking - Fintech products keep personal information secure. | (Chong et al., 2010; Sanchez et al., 2018) |
TRU2 | In general, Banking - Fintech products are reliable. | |
Perceived usefulness- PU | ||
PU1 | I can achieve my need using Banking-Fintech products. | (Lockett & Littler, 1997; Huh et al., 2009) |
PU2 | Using Banking-Fintech products help me save time in transactions. | |
PU3 | Banking-Fintech products increase transaction efficiency compared to traditional banking products. | |
PU4 | The association with Fintech helps banking products increase usefulness. | |
Perceived ease of use (PEU) | ||
PEU1 | Banking-Fintech products are easy to use. | (Cheng et al., 2006; Wang et al., 2003) |
PEU2 | Banking-Fintech products' interface is easy to understand. | |
PEU3 | Banking-Fintech products are easily used on available devices (smart phone, App, wifi...). | |
Perceived risk (PR) | ||
PR1 | I am afraid money is easily stolen from my bank account. | |
PR2 | I am afraid personal information is easily disclosed when using banking-Fintech products. | |
PR 3 | Overall, I feel insecure when using banking-Fintech products. | |
User innovativeness (UI) | ||
UI1 | When I heard about a new product, I would try it. | (Zhang et al., 2018) |
UI2 | I am usually the pioneer to try a new product. | |
Attitude (ATT) | ||
ATT1 | I believe using banking-Fintech products is a good idea. | (Grabner & Faullant, 2008) |
ATT2 | Using banking-Fintech products brings me a feeling of discovery. | |
ATT3 | I am interested in banking-Fintech products. | |
Intention to Use (ITU) | ||
INT1 | I will be using banking-Fintech products soon. | (Marakarkandy et al., 2017; Patel & Patel, 2018) |
INT2 | I am willing to keep using banking-Fintech products. | |
INT3 | I will recommend my friends to use banking-Fintech products. |
Estimate | Estimate | ||||||
ITU2 | < --- | ITU | 0.826 | P4 | < --- | PU | 0.788 |
ITU1 | < --- | ITU | 0.827 | P2 | < --- | PU | 0.840 |
ITU3 | < --- | ITU | 0.841 | Trust1 | < --- | TRUST | 0.827 |
PR2 | < --- | PR | 0.843 | Trust2 | < --- | TRUST | 0.883 |
PR1 | < --- | PR | 0.775 | Per2 | < --- | UI | 0.900 |
PR3 | < --- | PR | 0.690 | Per1 | < --- | UI | 0.870 |
Conv1 | < --- | PEU | 0.822 | ATT2 | < --- | Attitude | 0.853 |
Conv3 | < --- | PEU | 0.778 | ATT3 | < --- | Attitude | 0.867 |
Conv2 | < --- | PEU | 0.871 | BI3 | < --- | BI | 0.632 |
P3 | < --- | PU | 0.793 | BI2 | < --- | BI | 0.708 |
Estimate regression weights | S0.E0. | C0.R0. | P | ||||
Unstandardized | Standardized | ||||||
ATT | < --- | BI | 0.455 | 0.371 | 0.247 | 10.843 | 0.065 |
ATT | < --- | UI | 0.112 | 0.163 | 0.055 | 20.056 | 0.040 |
ATT | < --- | TRUST | 0.207 | 0.251 | 0.077 | 20.698 | 0.007 |
ATT | < --- | PU | 0.561 | 0.538 | 0.132 | 40.231 | *** |
ATT | < --- | PR | −0.045 | −0.063 | 0.041 | −10.098 | 0.272 |
ATT | < --- | CONV | −0.201 | −0.204 | 0.126 | −10.596 | 0.110 |
ITU | < --- | ATT | 0.835 | 0.829 | 0.071 | 110.778 | *** |
Code | Variables | References |
Brand Image: BI | ||
BI1 | I choose these banking-Fintech products because the bank always provides good products and services. | (Ruparelia et al., 2010; Ha, 2004) |
BI2 | I choose these banking-Fintech products because the bank is familiar to me. | |
BI3 | I choose to use these banking-Fintech products because the bank has a good reputation. | |
TRUST | ||
TRU1 | Banking - Fintech products keep personal information secure. | (Chong et al., 2010; Sanchez et al., 2018) |
TRU2 | In general, Banking - Fintech products are reliable. | |
Perceived usefulness- PU | ||
PU1 | I can achieve my need using Banking-Fintech products. | (Lockett & Littler, 1997; Huh et al., 2009) |
PU2 | Using Banking-Fintech products help me save time in transactions. | |
PU3 | Banking-Fintech products increase transaction efficiency compared to traditional banking products. | |
PU4 | The association with Fintech helps banking products increase usefulness. | |
Perceived ease of use (PEU) | ||
PEU1 | Banking-Fintech products are easy to use. | (Cheng et al., 2006; Wang et al., 2003) |
PEU2 | Banking-Fintech products' interface is easy to understand. | |
PEU3 | Banking-Fintech products are easily used on available devices (smart phone, App, wifi...). | |
Perceived risk (PR) | ||
PR1 | I am afraid money is easily stolen from my bank account. | |
PR2 | I am afraid personal information is easily disclosed when using banking-Fintech products. | |
PR 3 | Overall, I feel insecure when using banking-Fintech products. | |
User innovativeness (UI) | ||
UI1 | When I heard about a new product, I would try it. | (Zhang et al., 2018) |
UI2 | I am usually the pioneer to try a new product. | |
Attitude (ATT) | ||
ATT1 | I believe using banking-Fintech products is a good idea. | (Grabner & Faullant, 2008) |
ATT2 | Using banking-Fintech products brings me a feeling of discovery. | |
ATT3 | I am interested in banking-Fintech products. | |
Intention to Use (ITU) | ||
INT1 | I will be using banking-Fintech products soon. | (Marakarkandy et al., 2017; Patel & Patel, 2018) |
INT2 | I am willing to keep using banking-Fintech products. | |
INT3 | I will recommend my friends to use banking-Fintech products. |
Estimate | Estimate | ||||||
ITU2 | < --- | ITU | 0.826 | P4 | < --- | PU | 0.788 |
ITU1 | < --- | ITU | 0.827 | P2 | < --- | PU | 0.840 |
ITU3 | < --- | ITU | 0.841 | Trust1 | < --- | TRUST | 0.827 |
PR2 | < --- | PR | 0.843 | Trust2 | < --- | TRUST | 0.883 |
PR1 | < --- | PR | 0.775 | Per2 | < --- | UI | 0.900 |
PR3 | < --- | PR | 0.690 | Per1 | < --- | UI | 0.870 |
Conv1 | < --- | PEU | 0.822 | ATT2 | < --- | Attitude | 0.853 |
Conv3 | < --- | PEU | 0.778 | ATT3 | < --- | Attitude | 0.867 |
Conv2 | < --- | PEU | 0.871 | BI3 | < --- | BI | 0.632 |
P3 | < --- | PU | 0.793 | BI2 | < --- | BI | 0.708 |
Estimate regression weights | S0.E0. | C0.R0. | P | ||||
Unstandardized | Standardized | ||||||
ATT | < --- | BI | 0.455 | 0.371 | 0.247 | 10.843 | 0.065 |
ATT | < --- | UI | 0.112 | 0.163 | 0.055 | 20.056 | 0.040 |
ATT | < --- | TRUST | 0.207 | 0.251 | 0.077 | 20.698 | 0.007 |
ATT | < --- | PU | 0.561 | 0.538 | 0.132 | 40.231 | *** |
ATT | < --- | PR | −0.045 | −0.063 | 0.041 | −10.098 | 0.272 |
ATT | < --- | CONV | −0.201 | −0.204 | 0.126 | −10.596 | 0.110 |
ITU | < --- | ATT | 0.835 | 0.829 | 0.071 | 110.778 | *** |