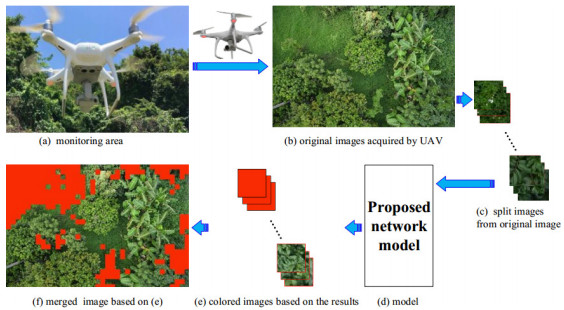
Citation: Issam Tout, Marie Marotel, Isabelle Chemin, Uzma Hasan. HBV and the importance of TLR9 on B cell responses[J]. AIMS Allergy and Immunology, 2017, 1(3): 124-137. doi: 10.3934/Allergy.2017.3.124
[1] |
Feng Chen, Xuqing Zhu, Jing Zheng, Tingting Xu, Kuan Wu, Chuhui Ru .
RHAMM regulates the growth and migration of lung adenocarcinoma A549 cell line by regulating Cdc2/CyclinB1 and MMP9 genes . Mathematical Biosciences and Engineering, 2020, 17(3): 2150-2163. doi: 10.3934/mbe.2020114 |
[2] | Yunfeng Liu, Guowei Sun, Lin Wang, Zhiming Guo . Establishing Wolbachia in the wild mosquito population: The effects of wind and critical patch size. Mathematical Biosciences and Engineering, 2019, 16(5): 4399-4414. doi: 10.3934/mbe.2019219 |
[3] | Yanmei Jiang, Mingsheng Liu, Jianhua Li, Jingyi Zhang . Reinforced MCTS for non-intrusive online load identification based on cognitive green computing in smart grid. Mathematical Biosciences and Engineering, 2022, 19(11): 11595-11627. doi: 10.3934/mbe.2022540 |
[4] | Diego Vicencio, Olga Vasilieva, Pedro Gajardo . Monotonicity properties arising in a simple model of Wolbachia invasion for wild mosquito populations. Mathematical Biosciences and Engineering, 2023, 20(1): 1148-1175. doi: 10.3934/mbe.2023053 |
[5] | Shikang Zheng, Kai Chen, Xinping Lin, Shiqian Liu, Jie Han, Guomin Wu . Quantitative analysis of facial proportions and facial attractiveness among Asians and Caucasians. Mathematical Biosciences and Engineering, 2022, 19(6): 6379-6395. doi: 10.3934/mbe.2022299 |
[6] | Martin Strugarek, Nicolas Vauchelet, Jorge P. Zubelli . Quantifying the survival uncertainty of Wolbachia-infected mosquitoes in a spatial model. Mathematical Biosciences and Engineering, 2018, 15(4): 961-991. doi: 10.3934/mbe.2018043 |
[7] | Yanfeng Shi, Shuo Qiu, Jiqiang Liu, Tinghuai Ma . Novel efficient lattice-based IBE schemes with CPK for fog computing. Mathematical Biosciences and Engineering, 2020, 17(6): 8105-8122. doi: 10.3934/mbe.2020411 |
[8] | Qian Shao, Rongrong Xuan, Yutao Wang, Jian Xu, Menglin Ouyang, Caoqian Yin, Wei Jin . Deep learning and radiomics analysis for prediction of placenta invasion based on T2WI. Mathematical Biosciences and Engineering, 2021, 18(5): 6198-6215. doi: 10.3934/mbe.2021310 |
[9] | Hui Li, Xintang Liu, Dongbao Jia, Yanyan Chen, Pengfei Hou, Haining Li . Research on chest radiography recognition model based on deep learning. Mathematical Biosciences and Engineering, 2022, 19(11): 11768-11781. doi: 10.3934/mbe.2022548 |
[10] | Yu Li, Meilong Zhu, Guangmin Sun, Jiayang Chen, Xiaorong Zhu, Jinkui Yang . Weakly supervised training for eye fundus lesion segmentation in patients with diabetic retinopathy. Mathematical Biosciences and Engineering, 2022, 19(5): 5293-5311. doi: 10.3934/mbe.2022248 |
As a perennial vine, Ipomoea cairica (L.) sweets are viewed as the noxious invasive weeds in China and are called as plant killer because of heavily covering other plants in wild lands [1]. Ipomoea cairica (L.) sweets like climbing, can rapiddly grow, propagate, and cover in its area. Consequently, Ipomoea cairica (L.) sweets can result in the serious harm to the biodiversity and stability of the ecosystem [2]. How to identify and monitor the Ipomoea cairica (L.) sweets in the wild is becoming very important since it can help the governments to take the necessary strategies and methods in the identified area to prevent and control the growth of the Ipomoea cairica (L.) sweets for biodiversity and stability of the ecosystem.
Currently, identifying and monitoring Ipomoea cairica (L.) sweets mainly depends on manual approaches of low efficiency and high cost [3]. With the quick development of image processing and artificial intelligence (AI), it is possible to help managements to make the precise efficient faster measures by efficiently identifying and monitoring Ipomoea cairica (L.) sweets [4]. Hyperspectral images have been used to identify the invasive species for monitoring and control their rapidly growth. Liu, et al. used the high-resolution imagery from Google Earth to monitor the Spartina alterniflora and showed that this approach can help governments to control Spartina alterniflora invasion [5], West et al. tested five models to identify Tamarik in satellite remote sensing data [6]. Bustamante et al. used hyperspectral sensors to detect Spartina densiflora Brongn [7]. Jomar et al. used imaging spectroscopy to detect Psidium cattleianum in Hawaiian Forests [8]. Khare et al. assess invasive plant species diversity in Pléiades 1A, RapidEye and Landsat-8 data [9]. However, it is difficult for these methods to detect the Ipomoea cairica (L.) sweets since the high-resolution hyperspectral images collected by aircraft and satellite are very high cost. For free low-resolution spectral data, some key information in these images is lost. As a result, the hyperspectral images are not suitable to monitor Ipomoea cairica (L.) sweets in the early stages.
The number of study has proved that the high-resolution digital images can be used to plants identification in various environments and shows their excellent results [10], especially the high-resolution images collected by low-altitude UAV because of retained good characteristics. Ge et al. used the aerial photographs to determine the Tamarix parviflora [11]. Jones et al. used the image analysis-based approach to detect Japanese Knotweed s.l. taxa in high-resolution, infra-red aerial images [12]. Dorigo et al. proposed an identifying method based on remote sensing to find F. japonica from low-cost images [13]. Sandino et al. used unmanned aerial vehicles (UAVs) and machine learning to detect the buffel grass and spinifex [14]. Hung et al. used feature learning to classify the invasive weed species based on the images from UAVs [15]. However, the detecting accuracy need be further improved, the efficient method with high accuracy need be developed for identifying invasive weed in the wild.
Now deep learning shows the excellent ability in image recognition fields and is widely applied to the object detection in agricultural. Pound et al. demonstrated deep approaches can offer excellent ability to identify root and shoot feature [16]. Alvaro et al. presented deep learning method to identify the tomato diseases [17]. Amanda et al. presented the transfer learning and deep CNN for pest damage and diseases detection, their results showed that their method can offer a fast, affordable method for digital disease detection [18]. Lu et al. used the deep CNN to identify the counts of maize tassels [19]. Though deep learning has widely applied to the agriculture fields, it still is difficult for identifying Ipomoea cairica (L.) sweets since it is very similar between Ipomoea cairica (L.) sweets and phorophyte.
To better address aforementioned challenges, we present a novel identifying and monitoring framework and method for Ipomoea cairica (L.) sweets based on unmanned aerial vehicle (UAV) and artificial intelligence (AI). In the proposed framework, we can low-costly collected the images with 8256 × 5504 pixels of the monitoring area by the UAV, and the collected images are split into more small sub-images with 224 × 224 pixels for identifying model. For identifying Ipomoea cairica (L.) sweets, we propose a novel deep CNN (ICSNet) which consists of 12 layers. Finally, the Ipomoea cairica (L.) sweets can be monitored by painting the area containing Ipomoea cairica (L.) sweets. Our experiment results show our method can automatically monitoring and eradicating Ipomoea cairica (L.) sweets in wilds.
In summary, the main contributions of this work are as follows:
1) A novel identifying and monitoring framework and method based on unmanned aerial vehicle (UAV) and artificial intelligence (AI) are proposed for Ipomoea cairica (L.) sweets
2) A deep CNN (ICSNet) is proposed to identifying Ipomoea cairica (L.) sweets, which can achieve the tradeoff between accuracy and time.
3) The framework and method are validated in our experiments and the experimental results show our method has the excellent performance.
The remainder is as follows. Section Ⅱ presents our methods. Section Ⅲ describes the experimental results and discussions. The conclusions are in Section Ⅳ.
For monitoring the Ipomoea cairica (L.) sweets, we propose a novel detecting framework for Ipomoea cairica (L.) sweets, which is shown in Figure 1. In our framework, monitoring the Ipomoea cairica (L.) sweets includes the following steps:
First step: The UAV is used to collect the images with 8256 × 5504 pixels of monitoring area, the whole images of monitoring area consists of sub-images with 8256 × 5504 pixels. The image sample is shown in Figure 1(b).
Second step: The collected images with 8256 × 5504 pixels are split into many more small images with 224 × 224 pixels. The samples are shown in Figure 1(c).
Third step: For each image with 224 × 224 pixels, we use the proposed deep CNN model to identify if the Ipomoea cairica (L.) sweets are contained in the images. This is corresponding to Figure 1(d).
Four step: Based on above identified results, we paint the images containing Ipomoea cairica (L.) sweets in red color. This is corresponding to Figure 1(e).
Five step: The painted images with 224 × 224 pixels is reassembled, as a result, the areas containing Ipomoea cairica (L.) sweets are covered by red color in the images with 8256 × 5504 pixels. This is corresponding to Figure 1(f). Finally, all the Ipomoea cairica (L.) sweets are identified in monitoring area.
For our framework and algorithm, in fact we can review it as a special case of object detection algorithm. For R-CNN and Fast R-CNN, they usually include three main steps: determining candidate region, feature extraction and classification. But slowly detecting speed is the lack of these algorithms. For our algorithm, we improve detection speed by in advance splitting the image into the little sub-image with fixed same size. Consequently, we can achieve the required monitoring accuracy by adjusting the size of sub-image, and detection time also can meet the actual requirements. Although, the object detection algorithms such as Yolo and SSD, which achieves the classification and positioning in one step, can improve the detection speed, their detection accuracy is lower than R-CNN and Fast R-CNN.
The multilayer neural network only consists of input, output and hidden layers. The actual needs decide the number of the hidden layers. Based on the original multilayer neural network, a more effective feature learning part is added in network. The part is the convolution layer and pooling layer which is set in front of fully connected layer. This is convolution neural network (CNN) which is shown in Figure 2. CNN deepen the number of layers in neural network and deep learning can be realized.
Here, we build a 12-layer deep CNN network model for identifying Ipomoea cairica (L.) sweets, which mainly consists of convolutional layer, pool layer, inception module, dropout layer, full connected layer. The input is the RGB image with the size of 224 × 224 × 3. The output consists of two neurons. The proposed network model is named ICSNet for short. The architecture of ICSNet is illustrated in Figure 3.
First layer: It is a convolution layer, which is with 64 3 × 3 filters and the stride is 2. The ReLU (rectified linear units) are used as the activation function for improving the convergence speed and simple gradient calculation. The ReLU can nonlinearly map output results of convolution layer. The output results of second layer is the data with the size of 112 × 112 × 64.
The pooling operator is connected after this layer for extracting the main features of a certain area, reducing the number of parameters, and preventing the model from over fitting. The maximum pooling with a 2 × 2 window and 2 strides is used in this layer. The pooling result is the data with the size of 56 × 56 × 64.
After pooling, the LRN (Local Response Normalization) is used to normalize the local response. The LRN enhances the larger response value and reduce the smaller response value. Thus, the LRN also can improve the generalization of the model. The output result of the LRN is the data with the size of 56 × 56 × 64.
Second layer: It is a convolution layer, which is with 128 3 × 3 filters and the stride is 1. The ReLU is selected as the activation function. The LRN is connected after this layer, the output results is the data with the size of 56×56×128. Then above data is processed by pooling operator with a 3 × 3 window and 2 strides. The output result of this layer is the data with the size of 28 × 28 × 128.
Third to eighth layer: These layers consist of three inception modules which consist of seven convolution modules and a pooling module. They are divided into the following four branches.
The first branch try convolution with 1 × 1 window on the output result of previous layer. The 1 × 1 convolution can efficiently enhance the expression ability, and at the same time, it can increase and reduce the dimension of the output channel. All four branches of the inception module use 1 × 1 convolution to perform low-cost (much less computation than 3 × 3 cross-channel feature transformation). Then 3 × 3 convolution is connected.
In the second branch, 1 × 1 convolution is used first, then 5 × 5 convolution is connected. The third branch is to use 3 × 3 maximum pooling with 2 strides and then connect 3 × 3 convolution. The fourth branch uses 1 × 1 convolution. Finally, the four branches are merged through an agammaegation operation at the end, and the output is the data with the size of 28 × 28 × 128.
After first inception modules, second and third inception modules are set in turn. The output is the data with the size of 28 × 28 × 480. Then, the pooling and LRN are performed, the data with the size of 14 × 14 × 480 is output.
Ninth layer: It is a convolution layer with 480 3 × 3 filters and their stride is 1. The ReLU is selected as the activation function. The result of this layer is the data with the size of 14 × 14 × 480. After convolution, the data is performed by LRN. Then, the maximum pooling with a 3 × 3 window and 2 strides is used. The output result is the data with the size of 6 × 6 × 480.
Tenth layer: This layer is dropout layer which is used to improve the generalization capability. The size of output result is 17,280.
Eleventh layer: This layer is the full connected layer with 100 neurons.
Twelfth layer: This layer is the Softmax activation function layer with 2 neurons.
In our ICSNet, we adopt the inception module from GoogLeNet, but only three inception modules are built in ICSNet. And we also add more convolution layers than AlextNet, but the number of convolution layers is less than VGGNet and ResNet. Consequently, the tradeoff between accuracy and time consuming can be achieved by our ICSNet. This is basic idea of our work.
We use the DJI Phantom 4 Advanced UAV to collect the color images with 8256 × 5504 pixels of Ipomoea cairica (L.) sweets in the monitoring area. The flight altitude is approximately 15 m above the ground. In our experiments, we set the distance according to the following three rules: 1) the clear images can be acquired; 2) the identifying accuracy can meet the actual requirements; 3) the UAV can be easily controlled. So we set the value of distance 15m above the ground according to our experience. The camera angle is adjusted in horizontal 45° for good front view since Ipomoea cairica (L.) sweets are a climbing weed. Finally, 100 raw images with 8256 × 5504 pixels are acquired. Figure 4 shows four samples of the scaled-down raw images.
We produce the dataset according to the following steps:
(1) The raw images are automatically divided into small images with 224 × 224 pixels by computer program. Figure 5 shows the samples of the images. Finally, 32000 samples with 224 × 224 pixels are selected, in which 24,000 samples contain Ipomoea cairica (L.) sweets and 8000 samples do not contain Ipomoea cairica (L.) sweets.
(2) The new samples are generated by horizontally and vertically rotating sample. Accordingly, 32000 samples are expanded to 96,000 samples.
(3) The new samples are generated by subtracting the mean of R, G and B from R, G, B value of sample, and Gray conversion. Finally, 288,000 samples are obtained to form the dataset in our experiments in which 216,000 samples contain Ipomoea cairica (L.) sweets and 72,000 samples do not contain Ipomoea cairica (L.) sweets.
In our experiments, the Accuracy, Precision, Recall, F1, AUC, min-batch size, computing cost and the number of layers are used to validate the performance of methods.
For test samples, TP denotes the number of correctly identified samples including Ipomoea cairica (L.) sweets, TN denotes the number of correctly identified samples without Ipomoea cairica (L.) sweets, FP denotes the number of incorrectly identified samples including Ipomoea cairica (L.) sweets, FN denotes the number of incorrectly identified samples without Ipomoea cairica (L.) sweets. The Accuracy, Precision, Recall, F1 are respectively written as follows:
Accuracy =TP+TNTP+TN+FP+FN×100% | (1) |
Precision =TPTP+TN×100% | (2) |
Recall =TP+TNTP+FN×100% | (3) |
F1=2× Precision × Recall Precision + Recall ×100% | (4) |
AUC is Area under the Curve of ROC (Receiver Operating Characteristics). The min-batch size is the number of samples in one batch. The time is the identifying time of samples. The number of layers is the number of convolutional and full connected layers, which can denote the complexity of the model. For obtaining valid results, the k-fold cross-validation (CV) is used to evaluate the algorithms and k is 10 in our experiments.
To efficiently validate the proposed method, we make comparisons with LeNet, AlexNet, GoogLeNet, VGG-19 and ResNet, all of which are deep CNNs [20].
(1) LeNet is firstly proposed by LeCun et al. in the 1990s, which includes three convolution layers, two sampling layers, two fully connected layers. At that time, it is difficult to run LeNet due to the limit of computation and memory capacity [21].
(2) AlexNet is proposed by Alex et al. and won the ILSVRC 2012 [22]. AlexNet achieves higher identifying accuracy than all the traditional machine learning algorithms. It is significant breakthrough for machine learning for classification.
(3) GoogLeNet is proposed by Christian of Google and is the winner of ILSVRC 2014 [23], in which inception layers, including different receptive areas with different kernel sizes capturing sparse correlation patterns, are integrated into CNN.
(4) VGG is proposed by Simonyan et al. in 2014, in which the convolution filter is a 3 × 3 filter and the stride is 2. VGG-11, VGG-16 and VGG-19 respectively include 11, 16 and 19 layers. The Softmax layer is the final layer for classification [24].
(5) ResNet is proposed by Kaiming et al. in 2015 to address to the vanishing gradient problem and shows the excellent ability of classification [25]. The popular ResNet consists of 49 convolution layers.
All the algorithms in our experiments are coded in Matlab R2019a. The values of initial parameters are randomly generated for networks. The parameters are set as follows: the number of epochs, learning rate drop factor, learning rate drop period, batch size are 200, 0.0004, 0.6, 30 and 128, respectively. The operating system of PC is Window 7 and the GPU is a NVIDIA with 8 GB memory.
The results of six algorithms are listed in Table 1 and Figure 6. Compared with ICSNet, ICSNet_n do not include inception modules. As we can see, the accuracy of ICSNet is 93.00%, the highest among six algorithms. The identifying time of ICSNet is 7.439 s, ranking the third shortest among six algorithms. The number of layers of ICSNet is 12, ranking the third lest among six algorithms. The accuracy of ResNet is equal to our algorithm, but their identifying time is more than that of our algorithm. The time of LeNet and AlexNet is less than that of our algorithm, but their accuracy is obviously lower than our algorithm. The Precision of our algorithm is 93.20%, only is lower than that of ResNet. The Recall of our algorithm is 93.30%, equal to that of ResNet ranking the first highest among six algorithms. The F1 of our algorithm is 93.00%, equal to that of ResNet, ranking the highest among six algorithms. The AUC of our algorithm is 94%, the second highest among six algorithms. Consequently, there is the best tradeoff between accuracy and time. Compared with LeNet, AlexNet, the number of layers of ICSNet is more, accordingly, the accuracy of ICSNet becomes higher but the time cost is only increased a little. The reason is that the size of filters is 5 × 5 in LeNet, the size of filters is 5 × 5 or 11 × 11 in AlexNet, the size of filters is 3 × 3 or 1 × 1 in ICSNet. For our algorithm, the size of filters is smaller than LeNet and AlexNet.
Algorithms | Accuracy (%) | Precision (%) | Recall (%) | F1 (%) | AUC | Min-batch size | Time(s) | The number of Layers |
ICSNet | 93.00 | 93.20 | 93.30 | 93.00 | 94.00 | 128 | 7.439 | 12 |
ICSNet_n | 80.50 | 81.60 | 80.50 | 80.50 | 81.44 | 128 | 6.451 | 7 |
LeNet | 80.50 | 81.42 | 80.64 | 80.50 | 81.00 | 128 | 6.511 | 7 |
AlexNet | 85.00 | 84.35 | 84.41 | 84.38 | 85.00 | 128 | 6.953 | 8 |
VGG-19 | 91.00 | 92.30 | 92.00 | 92.15 | 91.50 | 28 | 16.342 | 19 |
GoogLeNet | 92.50 | 92.64 | 92.50 | 93.00 | 93.00 | 80 | 13.192 | 22 |
ResNet-18 | 91.10 | 92.42 | 92.15 | 92.24 | 92.00 | 20 | 13.012 | 18 |
ResNet-50 | 92.15 | 92.50 | 92.15 | 92.50 | 92.50 | 20 | 20.160 | 50 |
ResNet152 | 93.00 | 93.45 | 93.30 | 93.00 | 94.20 | 20 | 31.879 | 152 |
We also see that, the more the number of the layers of networks, the larger the min-batch size is. But the VGG-19 is just the opposite due to its too much layers. The inception module induces the number of parameters and computing cost of the GoogLeNet and ICSNet, and remains more high identifying ability.
Figure 7 shows the relation between Min-batch size and Accuracy, Epoches. As we see in Figure 7(a), when the Min-batch size is 128, the accuracy is the highest. This indicates the Min-batch size is relation to the accuracy of algorithm. In Figure 7(b), the larger is the Min-batch size, the larger is the number of epochs. When the Min-batch size is more than 256, the number of epochs is sharp large. The larger is the number of the epochs, the more is the consumed time when the algorithm converges. We also can see, when the Min-batch size is 128, the accuracy of algorithm is high, and the number of epochs is relatedly small.
The Table 2 lists the accuracy of each fold in test sets in the 10-folds CV and the confusion matrix of each fold. In Table 2, "A" denotes the image containing Ipomoea cairica (L.) sweets, "B" denotes the image without Ipomoea cairica (L.) sweets.
Fold | Class | Number | A | B | Accuracy (%) |
1 | A | 21600 | 20079 | 1521 | 93.00 |
B | 7200 | 495 | 6705 | ||
2 | A | 21600 | 20036 | 1564 | 93.00 |
B | 7200 | 452 | 6748 | ||
3 | A | 21600 | 20415 | 1185 | 94.48 |
B | 7200 | 405 | 6885 | ||
4 | A | 21600 | 20440 | 1160 | 94.41 |
B | 7200 | 539 | 6661 | ||
5 | A | 21600 | 19814 | 1786 | 90.12 |
B | 7200 | 748 | 6452 | ||
6 | A | 21600 | 20596 | 1004 | 95.42 |
B | 7200 | 315 | 6885 | ||
7 | A | 21600 | 19491 | 2109 | 89.60 |
B | 7200 | 886 | 6314 | ||
8 | A | 21600 | 19795 | 1805 | 91.22 |
B | 7200 | 729 | 6471 | ||
9 | A | 21600 | 21122 | 478 | 97.57 |
B | 7200 | 221 | 6979 | ||
10 | A | 21600 | 19966 | 1634 | 91.18 |
B | 7200 | 906 | 6294 | ||
Average | 93.00 |
Compared with LeNet, AlexNet, the number of layers of ICSNet is more, accordingly, the accuracy of ICSNet becomes higher but the time cost is only increased a little. Compared with GoogLeNet and ResNet, the time cost is obviously reduced. Since the layers of network is closely related to the time cost, our algorithm is designed considering their tradeoff.
The ICSNet aims to accurately identify Ipomoea cairica (L.) sweets and non-Ipomoea cairica (L.) sweets in the wild. Some incorrectly identified samples are shown in Figure 8. We can see that, in Figure 8(b–e) , (g), (k) and (l), the parts of Ipomoea cairica (L.) sweets are clear, but their proportions are very small. It is reason that they are incorrectly identified by our algorithms. For Figure 8 (f), (d) and (i), the bad quality of images affects the performance of our algorithm. The samples in Figure 8 (a) is too dark, so the misidentification is excusable. To solve the problem, in future work, we need consider two aspects: (1) Set the parameters of camera of UAV for good quality of acquired images. (2) Determine the right distance between UAV and Ipomoea cairica (L.) sweets for good quality of acquired images.
Based on the results of our algorithm, we can draw the area of Ipomoea cairica (L.) sweets in the raw images or map to help management to efficiently monitor the Ipomoea cairica (L.) sweets and take measures to protect the environment. The raw images are shown in Figure 9 (a) and (c), and the corresponding images, in which the area of Ipomoea cairica (L.) sweets is painted red, are shown in Figure 9 (b) and (d). In Figure 9 (a) and (c), the images in big yellow box are zoomed, which are corresponding the images in small yellow box. As we can see in Figure 9, the area of Ipomoea cairica (L.) sweets can be intuitively found.
Ipomoea cairica (L.) sweets is an invasive weed which has caused serious harm to the biodiversity and stability of the ecosystem. It is very important to accurately and rapidly identifying Ipomoea cairica (L.) sweets in the wild for management taking the necessary strategies to control the Ipomoea cairica (L.) sweets to rapidly grow in the wild. In this study, we propose an identification framework and method for Ipomoea cairica (L.) sweets based on UAV and AI.
The UAV can help us to low-costly acquire lots of high quality of images of monitoring areas. The deep CNN is designed to efficiently identify Ipomoea cairica (L.) sweets in the acquired images. To validate the efficiency of proposed method, we generated 288000 samples in our experiments, and made comparison with LeNet, AlexNet, GoogleNet, VGG and ResNet. The experimental results showed that our method was excellent, the identifying accuracy is 93.00% and the identifying time is 7.439 s. Our work can help to promote the performance of online monitoring systems for Ipomoea cairica (L.) sweets.
In our experiments, we set the distance according to the following three rules: 1) the clear images can be acquired; 2) the identifying accuracy can meet the actual requirements; 3) the UAV can be easily controlled. In addition, we also consider the sensor resolution. So we set the value of distance 15m above the ground according to our experience. When the value of distance is higher than 15 m, the collected images became more and more vague. This will affect the accuracy of model. When the value of distance is lower than 15 m, although we can collect clear images, this will consume the more time to collect the data and process data.
For our detection frame and model, the size of images can be set as the possible predefined size. The size of image affects not only the accuracy of model but also the monitoring accuracy. It is important to design an excellent model and algorithm in the special application. So, in future, we will further study the question.
Our model also can be regarded as the special GoogLeNet. The reason simplifying GoogLeNet is to achieve a tradeoff between accuracy and time. In addition, we also adopt the idea of VGGNet, that is 3 × 3 filters is used in our model to further reduce the time complexity. For our algorithm, the idea of ResNet is not adopted. The reason is that we find the performance cannot meet our requirements.
In the future, we will acquire more Ipomoea cairica (L.) sweets images to train ICSNet model for further improving the identifying accuracy of model. In addition, we also will build the dynamic monitoring system of Ipomoea cairica (L.) sweets.
The work is funded by the Scientific Research Team Project of Shenzhen Institute of Information Technology (SZIIT2019KJ022), Special Innovation Project of Guangdong Education Department (2019GKTSCX093) and National Natural Science Foundation of China (71971089).
The authors declare that there is no conflict of interests regarding the publication of article.
[1] | Hepatitis B (2017) Available from: http://www.who.int/mediacentre/factsheets/fs204_Jul2014/en/. |
[2] | Lavanchy D (2005) Worldwide epidemiology of HBV infection, disease burden, and vaccine prevention. J Clin Virol 34: S1–S3. |
[3] |
McMahon BJ (2005) Epidemiology and natural history of hepatitis B. Semin Liver Dis 25: 3–8. doi: 10.1055/s-2005-915644
![]() |
[4] | Bertoletti A, Gehring AJ (2006) The immune response during hepatitis B virus infection. J Gen Viro l87: 1439–1449. |
[5] |
Dienstag JL (2008) Hepatitis B virus infection. New Engl J Med 359: 1486–1500. doi: 10.1056/NEJMra0801644
![]() |
[6] | Mason WS, Aldrich C, Summers J, et al. (1982) Asymmetric replication of duck hepatitis B virus DNA in liver cells: free minus-strand DNA. Proc Natl Acad Sci USA 79: 3997–4001. |
[7] |
Summers J, Mason WS (1982) Replication of the genome of a hepatitis B-like virus by reverse transcription of an RNA intermediate. Cell 29: 403–415. doi: 10.1016/0092-8674(82)90157-X
![]() |
[8] | Ganem D (2001) Virology. The X files--one step closer to closure. Science 294: 2299–2300. |
[9] |
European Association For The Study Of The Liver (2012) EASL clinical practice guidelines: Management of chronic hepatitis B virus infection. J Hepatol 57: 167–185. doi: 10.1016/j.jhep.2012.02.010
![]() |
[10] |
Bertoletti A, Kennedy PT (2015) The immune tolerant phase of chronic HBV infection: new perspectives on an old concept. Cell Mol Immunol 12: 258–263. doi: 10.1038/cmi.2014.79
![]() |
[11] |
Bertoletti A, Ferrari C (2013) Innate and adaptive immune responses in chronic hepatitis B virus infections: towards restoration of immune control of viral infection. Postgrad Med J 89: 294–304. doi: 10.1136/postgradmedj-2011-301073rep
![]() |
[12] |
Guidotti LG, Chisari FV (2006) Immunobiology and pathogenesis of viral hepatitis. Annu Rev Pathol 1: 23–61. doi: 10.1146/annurev.pathol.1.110304.100230
![]() |
[13] |
McMahon BJ (2009) The influence of hepatitis B virus genotype and subgenotype on the natural history of chronic hepatitis B. Hepatol Int 3: 334–342. doi: 10.1007/s12072-008-9112-z
![]() |
[14] | Buti M, Rodriguez-Frias F, Jardi R, et al. (2005) Hepatitis B virus genome variability and disease progression: the impact of pre-core mutants and HBV genotypes. J Clin Virol 34: S79–S82. |
[15] |
Sonneveld MJ, Rijckborst V, Zeuzem S, et al. (2012) Presence of precore and core promoter mutants limits the probability of response to peginterferon in hepatitis B e antigen-positive chronic hepatitis B. Hepatology 56: 67–75. doi: 10.1002/hep.25636
![]() |
[16] | Tacke F, Gehrke C, Luedde T, et al. (2004) Basal core promoter and precore mutations in the hepatitis B virus genome enhance replication efficacy of Lamivudine-resistant mutants. J Virol 78: 8524–8535. |
[17] |
Alberti A, Diana S, Sculard GH, et al. (1978) Detection of a new antibody system reacting with Dane particles in hepatitis B virus infection. BMJ-Brit Med J 2: 1056–1058. doi: 10.1136/bmj.2.6144.1056
![]() |
[18] | Loggi E, Gamal N, Bihl F, et al. (2014) Adaptive response in hepatitis B virus infection. J Viral Hepatitis 21: 305–313. |
[19] |
Hangartner L, Zinkernagel RM, Hengartner H (2006) Antiviral antibody responses: the two extremes of a wide spectrum. Nat Rev Immunol 6: 231–243. doi: 10.1038/nri1783
![]() |
[20] |
Seeger C, Mason WS (2000) Hepatitis B virus biology. Microbiol Mol Biol R 64: 51–68. doi: 10.1128/MMBR.64.1.51-68.2000
![]() |
[21] | Weber B (2005) Genetic variability of the S gene of hepatitis B virus: clinical and diagnostic impact. J Clin Virol 32: 102–112. |
[22] | Sayan M, Sentürk O, Akhan SÇ, et al. (2010) Monitoring of hepatitis B virus surface antigen escape mutations and concomitantly nucleos(t)ide analog resistance mutations in Turkish patients with chronic hepatitis B. Int J Infect Dis 14: e136–e141. |
[23] |
Mitchison NA (2004) T-cell-B-cell cooperation. Nat Rev Immunol 4: 308–312. doi: 10.1038/nri1334
![]() |
[24] |
Garraud O, Borhis G, Badr G, et al. (2012) Revisiting the B-cell compartment in mouse and humans: more than one B-cell subset exists in the marginal zone and beyond. BMC Immunol 13: 63. doi: 10.1186/1471-2172-13-63
![]() |
[25] |
LeBien TW, Tedder TF (2008) B lymphocytes: how they develop and function. Blood 112: 1570–1580. doi: 10.1182/blood-2008-02-078071
![]() |
[26] | Das A, Ellis G, Pallant C, et al. (2012) IL-10-producing regulatory B cells in the pathogenesis of chronic hepatitis B virus infection. J Immunol 189: 3925–3935. |
[27] |
Xu X, Shang Q, Chen X, et al. (2015) Reversal of B-cell hyperactivation and functional impairment is associated with HBsAg seroconversion in chronic hepatitis B patients. Cell Mol Immunol 12: 309–316. doi: 10.1038/cmi.2015.25
![]() |
[28] |
Liu Y, Cheng LS, Wu S, et al. (2016) IL-10-producing regulatory B-cells suppressed effector T-cells but enhanced regulatory T-cells in chronic HBV infection. Clin Sci 130: 907–919. doi: 10.1042/CS20160069
![]() |
[29] |
Farci P, Diaz G, Chen Z, et al. (2010) B cell gene signature with massive intrahepatic production of antibodies to hepatitis B core antigen in hepatitis B virus-associated acute liver failure. Proc Natl Acad Sci USA 107: 8766–8771. doi: 10.1073/pnas.1003854107
![]() |
[30] |
Moir S, Ho J, Malaspina A, et al. (2008) Evidence for HIV-associated B cell exhaustion in a dysfunctional memory B cell compartment in HIV-infected viremic individuals. J Exp Med 205: 1797–1805. doi: 10.1084/jem.20072683
![]() |
[31] | Weiss GE, Crompton PD, Li S, et al. (2009) Atypical memory B cells are greatly expanded in individuals living in a malaria-endemic area. J Immunol 183: 2176–2182. |
[32] |
Oliviero B, Cerino A, Varchetta S, et al. (2011) Enhanced B-cell differentiation and reduced proliferative capacity in chronic hepatitis C and chronic hepatitis B virus infections. J Hepatol 55: 53–60. doi: 10.1016/j.jhep.2010.10.016
![]() |
[33] |
Moir S, Fauci AS (2009) B cells in HIV infection and disease. Nat Rev Immunol 9: 235–245. doi: 10.1038/nri2524
![]() |
[34] |
Suarez F, Lortholary O, Hermine O, et al. (2006) Infection-associated lymphomas derived from marginal zone B cells: a model of antigen-driven lymphoproliferation. Blood 107: 3034–3044. doi: 10.1182/blood-2005-09-3679
![]() |
[35] |
Maruyama T, McLachlan A, Iino S, et al. (1993) The serology of chronic hepatitis B infection revisited. J Clin Invest 91: 2586–2595. doi: 10.1172/JCI116497
![]() |
[36] |
Böcher WO, Herzog-Hauff S, Herr W, et al. (1996) Regulation of the neutralizing anti-hepatitis B surface (HBs) antibody response in vitro in HBs vaccine recipients and patients with acute or chronic hepatitis B virus (HBV) infection. Clin Exp Immunol 105: 52–58. doi: 10.1046/j.1365-2249.1996.d01-732.x
![]() |
[37] |
Dusheiko GM, Hoofnagle JH, Cooksley WG, et al. (1983) Synthesis of antibodies to hepatitis B virus by cultured lymphocytes from chronic hepatitis B surface antigen carriers. J Clin Invest 71: 1104–1113. doi: 10.1172/JCI110860
![]() |
[38] | Barnaba V, Valesini G, Levrero M, et al. (1985) Immunoregulation of the in vitro anti-HBs antibody synthesis in chronic HBsAg carriers and in recently boosted anti-hepatitis B vaccine recipients. Clin Exp Immunol 60: 259–266. |
[39] | Barnaba V, Levrero M, Ruberti G, et al. (1987) In vitro anti-HBs antibody synthesis from anti-hepatitis B vaccine recipients. Clin Exp Immunol 70: 283–288. |
[40] | Amu S, Ruffin N, Rethi B, et al. (2013) Impairment of B-cell functions during HIV-1 infection. Aids 27: 2323–2334. |
[41] | Pensieroso S, Galli L, Nozza S, et al. (2013) B-cell subset alterations and correlated factors in HIV-1 infection. Aids 27: 1209–1217. |
[42] | Ni J, Hembrador E, Di BA, et al. (2003) Accumulation of B lymphocytes with a naive, resting phenotype in a subset of hepatitis C patients. J Immunol 170: 3429–3439. |
[43] |
Racanelli V, Frassanito MA, Leone P, et al. (2006) Antibody production and in vitro behavior of CD27-defined B-cell subsets: persistent hepatitis C virus infection changes the rules. J Virol 80: 3923–3934. doi: 10.1128/JVI.80.8.3923-3934.2006
![]() |
[44] |
Rosa D, Saletti G, Gregorio ED, et al. (2005) Activation of naïve B lymphocytes via CD81, a pathogenetic mechanism for hepatitis C virus-associated B lymphocyte disorders. Proc Natl Acad Sci USA 102: 18544–18549. doi: 10.1073/pnas.0509402102
![]() |
[45] |
Wang XD, Wang L, Ji FJ, et al. (2012) Decreased CD27 on B lymphocytes in patients with primary hepatocellular carcinoma. J Int Med Res 40: 307–316. doi: 10.1177/147323001204000131
![]() |
[46] | Li WY, Jiang YF, Jin QL, et al. (2010) Immunologic characterization of posthepatitis cirrhosis caused by HBV and HCV infection. J Biomed Biotechnol 2010: 138237. |
[47] |
Lund FE (2008) Cytokine-producing B lymphocytes-key regulators of immunity. Curr Opin Immunol 20: 332–338. doi: 10.1016/j.coi.2008.03.003
![]() |
[48] |
Llorente L, Zou W, Levy Y, et al. (1995) Role of interleukin 10 in the B lymphocyte hyperactivity and autoantibody production of human systemic lupus erythematosus. J Exp Med 181: 839–844. doi: 10.1084/jem.181.3.839
![]() |
[49] | Kakumu S, Shinagawa T, Ishikawa T, et al. (1991) Serum interleukin 6 levels in patients with chronic hepatitis B. Am J Gastroenterol 86: 1804–1808. |
[50] |
Aspord C, Bruder CJ, Jacob MC, et al. (2016) Remodeling of B-cell subsets in blood during pegylated IFNα-2a therapy in patients with chronic Hepatitis B infection. PloS One 11: e0156200. doi: 10.1371/journal.pone.0156200
![]() |
[51] |
Chang JJ, Wightman F, Bartholomeusz A, et al. (2005) Reduced hepatitis B virus (HBV)-specific CD4+ T-cell responses in human immunodeficiency virus type 1-HBV-coinfected individuals receiving HBV-active antiretroviral therapy. J Virol 79: 3038–3051. doi: 10.1128/JVI.79.5.3038-3051.2005
![]() |
[52] |
Maini MK, Boni C, Lee CK, et al. (2000) The role of virus-specific CD8 (+) cells in liver damage and viral control during persistent hepatitis B virus infection. J Exp Med 191: 1269–1280. doi: 10.1084/jem.191.8.1269
![]() |
[53] | Boni C, Penna A, Ogg GS, et al. (2001) Lamivudine treatment can overcome cytotoxic T-cell hyporesponsiveness in chronic hepatitis B: new perspectives for immune therapy. Hepatology 33: 963–971. |
[54] | Chen L, Zhang Z, Chen W, et al. (2007) B7-H1 up-regulation on myeloid dendritic cells significantly suppresses T cell immune function in patients with chronic hepatitis B. J Immunol 178: 6634–6641. |
[55] |
Takeuchi O, Akira S (2010) Pattern recognition receptors and inflammation. Cell 140: 805–820. doi: 10.1016/j.cell.2010.01.022
![]() |
[56] |
Hua Z, Hou B (2013) TLR signaling in B-cell development and activation. Cell Mol Immunol 10: 103–106. doi: 10.1038/cmi.2012.61
![]() |
[57] |
Bernasconi NL, Onai N, Lanzavecchia A (2003) A role for Toll-like receptors in acquired immunity: up-regulation of TLR9 by BCR triggering in naive B cells and constitutive expression in memory B cells. Blood 101: 4500–4504. doi: 10.1182/blood-2002-11-3569
![]() |
[58] | Ruprecht CR, Lanzavecchia A (2006) Toll-like receptor stimulation as a third signal required for activation of human naive B cells. Eur J Immunol 36: 810–816. |
[59] |
Hirsch I, Caux C, Hasan U, et al. (2010) Impaired Toll-like receptor 7 and 9 signaling: from chronic viral infections to cancer. Trends Immunol 31: 391–397. doi: 10.1016/j.it.2010.07.004
![]() |
[60] | Chen Z, Cheng Y, Xu Y, et al. (2008) Expression profiles and function of Toll-like receptors 2 and 4 in peripheral blood mononuclear cells of chronic hepatitis B patients. Clin Immunol 128: 400–408. |
[61] | Fathallah I, Parroche P, Gruffat H, et al. (2010) EBV latent membrane protein 1 is a negative regulator of TLR9. J Immunol 185: 6439–6447. |
[62] |
Hasan UA, Zannetti C, Parroche P, et al. (2013) The human papillomavirus type 16 E7 oncoprotein induces a transcriptional repressor complex on the Toll-like receptor 9 promoter. J Exp Med 210: 1369–1387. doi: 10.1084/jem.20122394
![]() |
[63] |
Wieland S, Thimme R, Purcell RH, et al. (2004) Genomic analysis of the host response to hepatitis B virus infection. Proc Natl Acad Sci USA 101: 6669–6674. doi: 10.1073/pnas.0401771101
![]() |
[64] |
Dunn C, Peppa D, Khanna P, et al. (2009) Temporal analysis of early immune responses in patients with acute hepatitis B virus infection. Gastroenterology 137: 1289–1300. doi: 10.1053/j.gastro.2009.06.054
![]() |
[65] |
Cheng J, Imanishi H, Morisaki H, et al. (2005) Recombinant HBsAg inhibits LPS-induced COX-2 expression and IL-18 production by interfering with the NFkappaB pathway in a human monocytic cell line, THP-1. J Hepatol 43: 465–471. doi: 10.1016/j.jhep.2005.02.033
![]() |
[66] | Müller C, Zielinski CC (1990) Impaired lipopolysaccharide-inducible tumor necrosis factor production in vitro by peripheral blood monocytes of patients with viral hepatitis. Hepatology 12: 1118–1124. |
[67] | Wang S, Chen Z, Hu C, et al. (2013) Hepatitis B virus surface antigen selectively inhibits TLR2 ligand-induced IL-12 production in monocytes/macrophages by interfering with JNK activation. J Immunol 190: 5142–5151. |
[68] |
Ml ODB, Binda RS, van Roosmalen MH, et al. (2009) Hepatitis B virus surface antigen impairs myeloid dendritic cell function: a possible immune escape mechanism of hepatitis B virus. Immunology 126: 280–289. doi: 10.1111/j.1365-2567.2008.02896.x
![]() |
[69] |
Vincent IE, Zannetti C, Lucifora J, et al. (2011) Hepatitis B virus impairs TLR9 expression and function in plasmacytoid dendritic cells. PloS One 6: e26315. doi: 10.1371/journal.pone.0026315
![]() |
[70] |
Xu Y, Hu Y, Shi B, et al. (2009) HBsAg inhibits TLR9-mediated activation and IFN-alpha production in plasmacytoid dendritic cells. Mol Immunol 46: 2640–2646. doi: 10.1016/j.molimm.2009.04.031
![]() |
[71] |
Han Q, Zhang C, Zhang J, et al. (2013) The role of innate immunity in HBV infection. Semin Immunopathol 35: 23–38. doi: 10.1007/s00281-012-0331-y
![]() |
[72] |
Lang T, Lo C, Skinner N, et al. (2011) The hepatitis B e antigen (HBeAg) targets and suppresses activation of the toll-like receptor signaling pathway. J Hepatol 55: 762–769. doi: 10.1016/j.jhep.2010.12.042
![]() |
[73] |
Visvanathan K, Skinner NA, Thompson AJV, et al. (2007) Regulation of Toll-like receptor-2 expression in chronic hepatitis B by the precore protein. Hepatology 45: 102–110. doi: 10.1002/hep.21482
![]() |
[74] | Wu J, Meng Z, Jiang M, et al. (2009) Hepatitis B virus suppresses toll-like receptor-mediated innate immune responses in murine parenchymal and nonparenchymal liver cells. Hepatology 49: 1132–1140. |
[75] |
Luangsay S, Gruffaz M, Isorce N, et al. (2015) Early inhibition of hepatocyte innate responses by hepatitis B virus. J Hepatol 63: 1314–1322. doi: 10.1016/j.jhep.2015.07.014
![]() |
[76] |
Jegaskanda S, Ahn SH, Skinner N, et al. (2014) Downregulation of interleukin-18-mediated cell signaling and interferon gamma expression by the hepatitis B virus e antigen. J Virol 88: 10412–10420. doi: 10.1128/JVI.00111-14
![]() |
[77] |
Yu S, Chen J, Wu M, et al. (2010) Hepatitis B virus polymerase inhibits RIG-I- and Toll-like receptor 3-mediated beta interferon induction in human hepatocytes through interference with interferon regulatory factor 3 activation and dampening of the interaction between TBK1/IKKepsilon and DDX3. J Gen Virol 91: 2080–2090. doi: 10.1099/vir.0.020552-0
![]() |
[78] | Hepatitis B virus polymerase suppresses NF-κB signaling by inhibiting the activity of IKKs via interaction with Hsp90β. Available from: https://www-ncbi-nlm-nih-gov.gate2.inist.fr/pubmed?term=Hepatitis%20B%20virus%20polymerase%20suppresses%20NF-kappaB%20signaling%20by%20inhibiting%20the%20activity%20of%20IKKs%20via%20interaction%20with%20Hsp90beta%5BTitle%5D. |
[79] |
Wang H, Ryu WS (2010) Hepatitis B virus polymerase blocks pattern recognition receptor signaling via interaction with DDX3: implications for immune evasion. PLoS Pathog 6: e1000986. doi: 10.1371/journal.ppat.1000986
![]() |
[80] |
Martinet J, Dufeu-Duchesne T, Bruder CJ, et al. (2012) Altered functions of plasmacytoid dendritic cells and reduced cytolytic activity of natural killer cells in patients with chronic HBV infection. Gastroenterology 143: 1586–1596. doi: 10.1053/j.gastro.2012.08.046
![]() |
[81] | Xu N, Yao HP, Lv GC, et al. (2012) Downregulation of TLR7/9 leads to deficient production of IFN-α from plasmacytoid dendritic cells in chronic hepatitis B. Inflamm Res 61: 997–1004. |
[82] |
Ratnam DT, Sievert W, Visvanathan K (2012) Natural killer cells display impaired responses to toll like receptor 9 that support viral persistence in chronic hepatitis B. Cell Immunol 279: 109–115. doi: 10.1016/j.cellimm.2012.09.005
![]() |
[83] |
Wang K, Liu H, He Y, et al. (2010) Correlation of TLR1-10 expression in peripheral blood mononuclear cells with chronic hepatitis B and chronic hepatitis B-related liver failure. Hum Immunol 71: 950–956. doi: 10.1016/j.humimm.2010.07.013
![]() |
[84] |
Zhou J, Huang Y, Tian D, et al. (2009) Expression of toll-like receptor 9 in peripheral blood mononuclear cells from patients with different hepatitis B and C viral loads. J Huazhong University Sci Technol Med Sci 29: 313–317. doi: 10.1007/s11596-009-0310-2
![]() |
[85] | Xie Q, Shen HC, Jia NN, et al. (2009) Patients with chronic hepatitis B infection display deficiency of plasmacytoid dendritic cells with reduced expression of TLR9. Microbes Infect 11: 515–523. |
[86] |
Huang YW, Hsu CK, Lin SC, et al. (2014) Reduced toll-like receptor 9 expression on peripheral CD14+ monocytes of chronic hepatitis B patients and its restoration by effective therapy. Antivir Ther 19: 637–643. doi: 10.3851/IMP2762
![]() |
[87] |
Xu N, Yao H, Sun Z, et al. (2008) Toll-like receptor 7 and 9 expression in peripheral blood mononuclear cells from patients with chronic hepatitis B and related hepatocellular carcinoma. Acta Pharmacol Sin 29: 239–244. doi: 10.1111/j.1745-7254.2008.00711.x
![]() |
[88] | Zhao PW, Ma L, Ji HF, et al. (2015) The expression of TLR-9, CD86, and CD95 phenotypes in circulating B cells of patients with chronic viral hepatitis B or C before and after antiviral therapy. Mediat Inflamm 2015: 762709. |
[89] | Wang G, Liu Y, Huang R, et al. (2017) Characteristics of regulatory B cells in patients with chronic hepatitis B virus infection in different immune phases. Discov Med 23: 295–304. |
[90] | Takeshita F, Suzuki K, Sasaki S, et al. (2004) Transcriptional regulation of the human TLR9 gene. J Immunol 173: 2552–2561. |
[91] | Aoyama T, Paik YH, Seki E (2010) Toll-like receptor signaling and liver fibrosis. Gastroenterol Res Pract 2010: E1–E8. |
1. | Nur Adibah Mohidem, Nik Norasma Che’Ya, Abdul Shukor Juraimi, Wan Fazilah Fazlil Ilahi, Muhammad Huzaifah Mohd Roslim, Nursyazyla Sulaiman, Mohammadmehdi Saberioon, Nisfariza Mohd Noor, How Can Unmanned Aerial Vehicles Be Used for Detecting Weeds in Agricultural Fields?, 2021, 11, 2077-0472, 1004, 10.3390/agriculture11101004 | |
2. | M. Shyamala Devi, J. Arun Pandian, Mahesh Baburao Lonare, Yeluri Praveen, 2022, Efficient Net Transfer Learning based Early Prediction of Monkey Pox Lesion, 978-1-6654-9764-0, 1297, 10.1109/ICOSEC54921.2022.9952099 | |
3. | Pu Zhang, Research on intelligent elevation control method of shearer drum based on deep learning, 2023, 1751-8806, 10.1049/sfw2.12089 | |
4. | Tieying Huang, Rahim Khan, Facility Layout Optimization of Urban Public Sports Services under the Background of Deep Learning, 2022, 2022, 1687-5273, 1, 10.1155/2022/1748319 | |
5. | Fei Luo, Jackrit Suthakorn, Robot Fault Detection Based on Big Data, 2023, 2023, 1687-5257, 1, 10.1155/2023/8375382 | |
6. | Waqas Ahmed, Vamsi Krishna Kommineni, Birgitta König-Ries, Jitendra Gaikwad, Luiz Gadelha, Sheeba Samuel, Evaluating the method reproducibility of deep learning models in biodiversity research, 2025, 11, 2376-5992, e2618, 10.7717/peerj-cs.2618 | |
7. | Fei Xing, Ru An, Xulin Guo, Xiaoji Shen, Mapping invasive noxious weed species in the alpine grassland ecosystems using very high spatial resolution UAV hyperspectral imagery and a novel deep learning model, 2024, 61, 1548-1603, 10.1080/15481603.2024.2327146 |
Algorithms | Accuracy (%) | Precision (%) | Recall (%) | F1 (%) | AUC | Min-batch size | Time(s) | The number of Layers |
ICSNet | 93.00 | 93.20 | 93.30 | 93.00 | 94.00 | 128 | 7.439 | 12 |
ICSNet_n | 80.50 | 81.60 | 80.50 | 80.50 | 81.44 | 128 | 6.451 | 7 |
LeNet | 80.50 | 81.42 | 80.64 | 80.50 | 81.00 | 128 | 6.511 | 7 |
AlexNet | 85.00 | 84.35 | 84.41 | 84.38 | 85.00 | 128 | 6.953 | 8 |
VGG-19 | 91.00 | 92.30 | 92.00 | 92.15 | 91.50 | 28 | 16.342 | 19 |
GoogLeNet | 92.50 | 92.64 | 92.50 | 93.00 | 93.00 | 80 | 13.192 | 22 |
ResNet-18 | 91.10 | 92.42 | 92.15 | 92.24 | 92.00 | 20 | 13.012 | 18 |
ResNet-50 | 92.15 | 92.50 | 92.15 | 92.50 | 92.50 | 20 | 20.160 | 50 |
ResNet152 | 93.00 | 93.45 | 93.30 | 93.00 | 94.20 | 20 | 31.879 | 152 |
Fold | Class | Number | A | B | Accuracy (%) |
1 | A | 21600 | 20079 | 1521 | 93.00 |
B | 7200 | 495 | 6705 | ||
2 | A | 21600 | 20036 | 1564 | 93.00 |
B | 7200 | 452 | 6748 | ||
3 | A | 21600 | 20415 | 1185 | 94.48 |
B | 7200 | 405 | 6885 | ||
4 | A | 21600 | 20440 | 1160 | 94.41 |
B | 7200 | 539 | 6661 | ||
5 | A | 21600 | 19814 | 1786 | 90.12 |
B | 7200 | 748 | 6452 | ||
6 | A | 21600 | 20596 | 1004 | 95.42 |
B | 7200 | 315 | 6885 | ||
7 | A | 21600 | 19491 | 2109 | 89.60 |
B | 7200 | 886 | 6314 | ||
8 | A | 21600 | 19795 | 1805 | 91.22 |
B | 7200 | 729 | 6471 | ||
9 | A | 21600 | 21122 | 478 | 97.57 |
B | 7200 | 221 | 6979 | ||
10 | A | 21600 | 19966 | 1634 | 91.18 |
B | 7200 | 906 | 6294 | ||
Average | 93.00 |
Algorithms | Accuracy (%) | Precision (%) | Recall (%) | F1 (%) | AUC | Min-batch size | Time(s) | The number of Layers |
ICSNet | 93.00 | 93.20 | 93.30 | 93.00 | 94.00 | 128 | 7.439 | 12 |
ICSNet_n | 80.50 | 81.60 | 80.50 | 80.50 | 81.44 | 128 | 6.451 | 7 |
LeNet | 80.50 | 81.42 | 80.64 | 80.50 | 81.00 | 128 | 6.511 | 7 |
AlexNet | 85.00 | 84.35 | 84.41 | 84.38 | 85.00 | 128 | 6.953 | 8 |
VGG-19 | 91.00 | 92.30 | 92.00 | 92.15 | 91.50 | 28 | 16.342 | 19 |
GoogLeNet | 92.50 | 92.64 | 92.50 | 93.00 | 93.00 | 80 | 13.192 | 22 |
ResNet-18 | 91.10 | 92.42 | 92.15 | 92.24 | 92.00 | 20 | 13.012 | 18 |
ResNet-50 | 92.15 | 92.50 | 92.15 | 92.50 | 92.50 | 20 | 20.160 | 50 |
ResNet152 | 93.00 | 93.45 | 93.30 | 93.00 | 94.20 | 20 | 31.879 | 152 |
Fold | Class | Number | A | B | Accuracy (%) |
1 | A | 21600 | 20079 | 1521 | 93.00 |
B | 7200 | 495 | 6705 | ||
2 | A | 21600 | 20036 | 1564 | 93.00 |
B | 7200 | 452 | 6748 | ||
3 | A | 21600 | 20415 | 1185 | 94.48 |
B | 7200 | 405 | 6885 | ||
4 | A | 21600 | 20440 | 1160 | 94.41 |
B | 7200 | 539 | 6661 | ||
5 | A | 21600 | 19814 | 1786 | 90.12 |
B | 7200 | 748 | 6452 | ||
6 | A | 21600 | 20596 | 1004 | 95.42 |
B | 7200 | 315 | 6885 | ||
7 | A | 21600 | 19491 | 2109 | 89.60 |
B | 7200 | 886 | 6314 | ||
8 | A | 21600 | 19795 | 1805 | 91.22 |
B | 7200 | 729 | 6471 | ||
9 | A | 21600 | 21122 | 478 | 97.57 |
B | 7200 | 221 | 6979 | ||
10 | A | 21600 | 19966 | 1634 | 91.18 |
B | 7200 | 906 | 6294 | ||
Average | 93.00 |