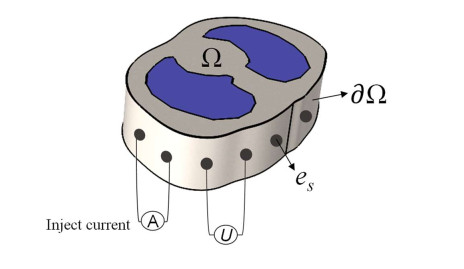
Citation: Andrey Kudryavtsev. Absolute Stock Returns and Trading Volumes: Psychological Insights[J]. Quantitative Finance and Economics, 2017, 1(2): 186-204. doi: 10.3934/QFE.2017.2.186
[1] | Tong Sun, Xin Zeng, Penghui Hao, Chien Ting Chin, Mian Chen, Jiejie Yan, Ming Dai, Haoming Lin, Siping Chen, Xin Chen . Optimization of multi-angle Magneto-Acousto-Electrical Tomography (MAET) based on a numerical method. Mathematical Biosciences and Engineering, 2020, 17(4): 2864-2880. doi: 10.3934/mbe.2020161 |
[2] | Shuaiyu Bu, Yuanyuan Li, Guoqiang Liu, Yifan Li . MAET-SAM: Magneto-Acousto-Electrical Tomography segmentation network based on the segment anything model. Mathematical Biosciences and Engineering, 2025, 22(3): 585-603. doi: 10.3934/mbe.2025022 |
[3] | Zhigao Zeng, Cheng Huang, Wenqiu Zhu, Zhiqiang Wen, Xinpan Yuan . Flower image classification based on an improved lightweight neural network with multi-scale feature fusion and attention mechanism. Mathematical Biosciences and Engineering, 2023, 20(8): 13900-13920. doi: 10.3934/mbe.2023619 |
[4] | Chaofeng Ren, Xiaodong Zhi, Yuchi Pu, Fuqiang Zhang . A multi-scale UAV image matching method applied to large-scale landslide reconstruction. Mathematical Biosciences and Engineering, 2021, 18(3): 2274-2287. doi: 10.3934/mbe.2021115 |
[5] | Hong'an Li, Man Liu, Jiangwen Fan, Qingfang Liu . Biomedical image segmentation algorithm based on dense atrous convolution. Mathematical Biosciences and Engineering, 2024, 21(3): 4351-4369. doi: 10.3934/mbe.2024192 |
[6] | Shuaiyu Bu, Yuanyuan Li, Wenting Ren, Guoqiang Liu . ARU-DGAN: A dual generative adversarial network based on attention residual U-Net for magneto-acousto-electrical image denoising. Mathematical Biosciences and Engineering, 2023, 20(11): 19661-19685. doi: 10.3934/mbe.2023871 |
[7] | Jing Wang, Jiaohua Qin, Xuyu Xiang, Yun Tan, Nan Pan . CAPTCHA recognition based on deep convolutional neural network. Mathematical Biosciences and Engineering, 2019, 16(5): 5851-5861. doi: 10.3934/mbe.2019292 |
[8] | Limin Ma, Yudong Yao, Yueyang Teng . Iterator-Net: sinogram-based CT image reconstruction. Mathematical Biosciences and Engineering, 2022, 19(12): 13050-13061. doi: 10.3934/mbe.2022609 |
[9] | Hongan Li, Qiaoxue Zheng, Wenjing Yan, Ruolin Tao, Xin Qi, Zheng Wen . Image super-resolution reconstruction for secure data transmission in Internet of Things environment. Mathematical Biosciences and Engineering, 2021, 18(5): 6652-6671. doi: 10.3934/mbe.2021330 |
[10] | Ying Xu, Jinyong Cheng . Secondary structure prediction of protein based on multi scale convolutional attention neural networks. Mathematical Biosciences and Engineering, 2021, 18(4): 3404-3422. doi: 10.3934/mbe.2021170 |
Electrical impedance tomography (EIT) is a medical functional imaging technique that images the internal conductivity distribution by measuring boundary voltage data [1]. Under different physiological and pathological conditions, such as respiration, heart beating, cancer, the human conductivity and dielectric constant will change. EIT technology has been considered as an effective solution for human functional imaging, as it can reconstruct the electrical conductivity distribution of organisms. Compared to other tomographic techniques, such as computed tomography (CT) and magnetic resonance imaging, this technique has the advantages of being non-invasive, low-cost and radiation-free. It is widely used in biomedical imaging, such as lung ventilation monitoring [2], cancer detection [3] and brain imaging [4,5,6,7]. However, the image reconstruction of EIT is an ill-posed, nonlinear inverse problem [8], which causes EIT to suffer low resolution of the reconstructed images [9]. Therefore, it is of great value to develop the EIT image reconstruction method to obtain reconstructed images with clear boundaries, fewer artifacts and higher resolution images for clinical application.
Many methods have been proposed for EIT reconstruction. These methods can be divided into algebraic reconstruction techniques (ARTs) [10] and artificial neural networks (ANNs) [11]. ARTs reconstruct the conductivity distribution based on iterative back-projection. The regularization and iteration methods, including Landweber iteration [12], Gauss-Newton (GN) iteration [13], Tikhonov regularization [14] and total-variation regularization [15], have been implemented to reduce the influence of ill-posedness [16]. Nguyen et al. [17] proposed a time-efficient algorithm with a self-weighted NOSER-prior method and obtained results with good accuracy and noise tolerances. Sun et al. [18] presented an improved regularization method by combining the prior information extracted from the patient with Tikhonov regularization to obtain a high-resolution image. Jin and Maass [19] introduced the regularization theory with ℓp sparsity constraints to solve the inverse problems; Margotti [20] presented a strategy that combined a gradient-like method with a Tikhonov regularization to find stable solutions to ill-posed problems. Much research on ARTs has been done, but they still have problems such as a slow solving speed, blurry boundaries and vulnerability to noise.
Differing from the ART method, ANNs reconstruct images from voltage measurements through the use of a nonlinear transform, which requires even less computation when dealing with nonlinear problems [21]. However, when using deep learning methods for EIT image reconstruction, the spatial resolution of the reconstructed image is always lower than the training size. Therefore, the image reconstructed via EIT cannot be 100% restored, and this error will never tend to zero [22].
ANNs based on deep learning techniques have attracted much attention in the recent past for reconstruction of EIT images [23]. Wang et al. [24] developed a network by fusing the hybrid particle swarm optimization algorithm and radial basis function layer to obtain a compelling image. Huuhtanen and Jung [25] proposed an ANN with multilayer perceptrons and a radial basis function layer. However, these ANNs without convolutional calculation occupy too many memory resources, reducing the computational efficiency.
Therefore, the ANNs based on multilayer convolutional neural networks (CNNs) have been introduced to EIT analysis. Tan et al. [26] proposed an improved LeNet and proved the potential of CNNs to improve image accuracy and training speed. Gao [27] offered a CNN with a convolutional denoising auto-encoder and reconstructed a robust and denoising image. Hamilton and Hauptmann [28] combined the U-Net with the D-bar method to reconstruct both circle and lung phantoms. Although the method provided refined image development relative to the traditional D-bar algorithm, the method is highly dependent on the result of the D-bar algorithm. Wei et al. [29] used the improved U-Net composed of induced contrast current as the network input. They significantly improved the speed, stability and quality of EIT imaging, especially in sharp corners and edges. de Hoop et al. [30] and Nganyu et al. [31] also applied the concept of physics to solve partial differential equations and related parameter identification problems; it is also a feasible idea to solve inverse problems. Therefore, deep neural networks may become an effective method for image reconstruction based on EIT technology.
Because the exact inverse transform may not exist a priori, especially in the presence of sensor non-idealities and noise, EIT image reconstruction is a challenging task. To solve this problem, deeper and more complex deep learning models should be proposed [32]. The image reconstruction ability of deep learning models is reinforced with the increase of network layers, as they have multiple hidden layers that enable them to learn abstractions based on inputs [33]. But, deeper networks may cause gradient vanishing. Many deep learning models, including ResNet [34] and DenseNet [35], have been proposed to solve this problem. DenseNet is a meaningful choice to solve the EIT image reconstruction problem because it uses shorter connections between each layer of the dense block to maximize the information flow and improve the fitting ability. However, using the conventional DenseNet network still cannot improve the EIT image reconstruction task.
Considering the problems described above, a deep learning method based on DenseNet with multi-scale convolution (MS-DenseNet) is proposed here to improve the accuracy of EIT image reconstruction. To extract the information on different scale features, the proposed network uses convolutional kernels of different sizes to perform three types of convolutional operations, forming three types of dense blocks instead of the original dense block structure. The three dense blocks were placed in parallel to extract different input features. Additionally, aiming to minimize the information loss of the pooling module during image reconstruction, a hybrid pooling structure was designed and is applied in connection blocks. To solve the problem that the parameters do not easily reach the optimal solution during the training of large networks, a learning rate setting has been reduced to two stages applied to enhance the adaptive moment estimation (Adam) algorithm fitting ability. The proposed network was trained and tested on two datasets, including a circle-phantom dataset and a lung-phantom dataset. The voltage of the phantom was fed into the MS-DenseNet, and the predicted conductivity distribution was obtained via nonlinear calculation; the result was used to verify the method's effectiveness. The root mean square error (RMSE), structural similarity (SSIM), mean absolute error (MAE) and image correlation coefficient (ICC) were adopted as the evaluation metrics. The results showed that the MS-DenseNet method has good performance in terms of generalization and robustness as compared with the conventional DenseNet and the GN method; this helps to obtain EIT reconstructed images with clear boundaries and high resolution.
The contributions of this paper can be elaborated as follows:
1) The proposed MS-DenseNet method involves obtaining the conductivity distribution from the voltage inputs directly for EIT image reconstruction. The network incorporatess three types of dense blocks, a mixed pooling structure and a modified learning rate setting to boost the performance of the network.
2) Parallel dense blocks based on various types of multi-scale convolutions are used to replace the traditional dense block. Information from data of different scales is obtained and enhances the fitting and generalization capabilities of the network.
3) A hybrid pooling structure, which includes average pooling and 2 × 2 convolutions, has been used to replace the maximum pooling or average pooling in the conventional DenseNet, thus improving the information flow of voltage measurements and effectively preventing information loss.
4) A learning rate setting that is reduced to two stages is proposed to replace the fixed learning rate setting; it can significantly improve the fitting capability and optimize the image reconstruction results in the last relatively few epochs.
The EIT image reconstruction process can be divided into forward and inverse problems. Figure 1 shows the process of EIT image reconstruction.
The solution to the forward problem aims to obtain the electrode voltage U on the boundary ∂Ω :
U=F(σ(Ω)) | (1) |
where σ(Ω) is a given distribution of conductivity in the region Ω and F(⋅):σ(Ω)→U is a forward mapping from σ(Ω) to U. Let φ(r) be the conductivity at any point r in the region Ω; the complete electrode model is used as forward mapping F(⋅); then, the forward problem can be calculated as:
∇⋅(σ(r)∇φ(r))=0,r∈Ω | (2) |
φ(r)+zsσ(r)∂φ(r)∂v=Us,r∈es,s=1,…,n | (3) |
∫esσ(r)∂φ(r)∂vdΩ=Is,s=1,…,n | (4) |
σ(r)∂φ(r)∂v=0,r∈∂Ω∖n⋃s=1es | (5) |
n∑s=1Is=0,n∑s=1Us=0 | (6) |
where es is the s-th electrodes set at ∂Ω, n is the number of electrodes, zs is the contact impedance of electrode es, Us is the electric potential of es and v is a direction vector, where the direction is perpendicular to the tangential line at r∈∂Ω. The selection and the numerical solution of r in Eqs (2)-(5) are usually completed by using the finite element method (FEM) [36].
The solution to the inverse problem aims to reconstruct the image of the inner conductivity distribution σ in the region Ω from the given boundary measurements V. The traditional inverse problem can be described as minimizing the objective Φ by finding the suitable distribution of conductivity ˆσ :
Φ=m∑i=1(V−ˆU(ˆσ))2+G(ˆσ) | (7) |
where m is the number of voltage measurements at the boundary of the object, ˆU is the measurement calculated by ˆσ and G(⋅) is a penalty function to prevent overfitting.
The deep learning-based EIT image reconstruction can be described as follows:
ˆσ=ζ(V) | (8) |
where ζ(⋅) is the nonlinear mapping between V and ˆσ, and the error between ˆσ and σ is reduced by modifying the parameters in ζ(⋅).
The overall structure of the proposed MS-DenseNet is shown in Figure 2. Based on the DenseNet, the proposed MS-DenseNet network consists of an input layer, dense blocks, transitional convolution-pooling layers and an output layer. Successive batch normalization (BN), rectified linear activation function (ReLU) and convolution (Conv) calculations are performed in dense blocks. Each dense block contains four BN-ReLU-Conv structures with the same output, interconnected by dense connections. The output of each dense block is the splicing of the input of that block with the output of the four BN-ReLU-Conv structures. Each transitional convolution-pooling layer connects two adjacent dense blocks, which contain a 1 × 1 convolution and a pooling layer. The size of the convolutional and pooling kernels varies according to the size of the weight matrix and the number of channels. The red arrow in Figure 2 indicates the direction of data flow, and the green arrow denotes the pooling layer. A set of three-dimensional vectors near each arrow represents the size of the data height, weight and number of channels. The number of final output channels "n" depends on the number of FEM elements in the conductivity distribution. Table 1 shows the details of each dense block, where "k" is the number of output channels of each BN-ReLU-Conv structure in the dense block. The number of channels of output data is equal to the number of filters in the final output module, independent of the number of channels in the input data.
Net block | Layer | Activation function | ||
Dense Block 1 | − | − | Dense Block type3 (k = 8) |
ReLU |
Dense Block 2 | Dense Block type1 (k = 8) |
Dense Block type2 (k = 8) |
Dense Block type3 (k = 32) |
ReLU |
Dense Block 3 | Dense Block type1 (k = 16) |
Dense Block type2 (k = 16) |
Dense Block type3 (k = 96) |
ReLU |
Final output | Fully Connect | / | / | Sigmoid |
The image reconstruction process for EIT is ill-conditioned and nonlinear. Therefore, acquiring and retaining more input features is the key to EIT image reconstruction. The design principle of MS-DenseNet is that the whole network can obtain and retain as much input feature information as possible, so as to yield higher-quality reconstruction images.
Dense blocks preserve input features through a densely connected structure. The role of multi-scale convolution in neural networks is to capture information from data at different scales. The combination of these two blocks makes the network more sensitive to input data, allowing it to obtain and preserve diverse features. The hybrid pooling can preserve more input features and effectively prevent information loss. The concatenation layer combines the features of the parallel input of the previous layer. These structures make MS-DenseNet suitable for EIT image reconstruction problems. The flowchart of the proposed MS-DenseNet is shown in Figure 3.
As shown in Figure 4, three different inception structures make up three kinds of dense blocks.
In the Convolutions type1 block, the output of the ReLU function is fed into the 1 × 1, 3 × 3 and 5 × 5 convolutional layers in parallel, and the number of channels corresponding to the filter is generated; then, the Sum layer sums the elements of the corresponding channels and corresponding positions in the output of different branches to obtain the computational results of the multi-scale convolution. Multi-scale convolution can yield feature information for different scales, which helps to improve the accuracy of reconstructed images.
In the Convolutions type2 block, the output of the ReLU function is first connected to a 1 × 1 convolutional layer to reduce the dimensions. The output of this 1 × 1 convolutional layer is then fed in parallel to a multi-scale convolutional layer. The Concatenation layer stitches together the features extracted by the three convolutional operations. Finally, the data dimensions are increased by the 1 × 1 convolutional layer. The connection layer combines the features of the parallel input of the previous layer to obtain more global information.
In the Convolutions type3 block, the output of the ReLU function is fed to the serial 1 × 1, 3 × 3 and 5 × 5 convolutional layers. The outputs of all three convolutional layers with different convolutional sizes are used as the inputs of the Sum layer. The result of the Convolutions type3 block is then obtained by the Sum layer. Various features extracted by multi-scale convolution use dense connections to reduce information loss during feature extraction.
In addition, 5 × 5 convolutions are replaced with 3 × 3 convolutions in Dense Block 3 due to the same calculation effect for the 2 × 2 input size.
In the EIT image reconstruction problem, the dimensionality of the output data is often larger than that of the input data, and any input data is valuable for the output. However, traditional pooling loses a large amount of data with unimportant features. Meanwhile, the convolutional layer can choose a proportional relationship between different inputs, allowing more detailed information to be retained.
As shown in Figure 5, the hybrid pooling structure combining the average pooling and 2 × 2 convolutions are used to replace the traditional max pooling or the average pooling structure in the connection block. To prevent information loss in the process of pooling, the feature maps of the hybrid pooling output are concatenated with the corresponding output feature maps of a 2 × 2 convolution and an average pooling layer. The average pooling layer provides 25% of the channels, and the 2 × 2 convolution provides 75% of the channels. The hybrid pooling structure can effectively improve information flow, reduce information loss and improve the quality of image reconstruction.
Two FEM phantoms, including a circle phantom and lung phantom, were used to complete the training of MS-DenseNet and DenseNet.
As shown in Figure 6, a circular tank with a radius of 9.5 cm was used as the imaging region for the circle phantom. The background conductivity was set to 1, two symmetrically distributed circles were selected to constitute the simulated normal conductivity region (blue region), the radius of the normal conductivity region was set to vary in the range of 3 to 4 cm, the normal conductivity region centers {x, y} were randomly distributed near the coordinates {5.5, 0} or {−5.5, 0} with a distance of less than 2.06 cm from the coordinates, the conductivity region was set to vary in the range of 0.1 to 0.5 and the step size was 0.05. Furthermore, 0-2 abnormal regions (red region) were set in the circle. The conductivity of the abnormal region was set to be 1.2 to 2, and the step size was 0.2. The radius of the abnormal region was set to 1-2.5 cm. Additionally, 20, 000 sets of data were established for network training to avoid overfitting. At the same time, it should be noted that the two abnormal conductivity regions cannot overlap.
The circle phantom datasets were taken from the EIDORS project [37]. Figure 6 shows that the process of creating the circle phantom datasets can be separated into three steps. The steps for creating the datasets are as follows:
1) Create a circle phantom, where the radius of the circle is 9.5 cm and 16 electrodes are evenly set in the circle's boundary. Divide the circle into 1650 elements via FEM generation.
2) Change the conductivity of normal region and abnormal region elements. Randomly generate 20, 000 sets of parameter data matrices, where each set of data includes center coordinates of abnormal regions, radius of abnormal regions, the number of abnormal regions, center coordinates of the normal region, and radius of the normal region. Furthermore, if an element is in both the normal and abnormal regions, its conductivity should follow the conductivity of the abnormal region.
3) Inject a current of 0.95 mA via a pair of adjacent electrodes; set the other two adjacent electrodes set as the voltage-measuring electrodes. Change the measurement electrodes until all of the electrodes (except for the injecting electrodes) are measured. Then, change the injected electrodes in sequence until all electrodes are injected. For each circle phantom with different conductivity distributions, collect 208 simulated measurements of voltage data as input data by using the above method; collect the conductivity of 1650 elements as output labels.
In order to simulate the monitoring of the lungs when the information is sufficient, a 2D lung phantom was created with actual lung and thorax boundaries. As shown in Figure 7, the lung phantom consisted of two lung regions and a background thoracic region. The conductivities of the lung and the thorax were set to 0.2 and 0.4, respectively, and the entire thorax region had the same conductivity. Three different conductivity anomaly types were applied in the lung region: Type a changes the conductivity of the whole lung region in the left or right, Type b changes the conductivity of a circle in the lung region and Type c changes the conductivity of a square in the lung region. Furthermore, the conductivity variation of the thoracic region was designed to be unaffected by these three variations. Considering the conductivity variation of the phantom in monitoring, the lung region conductivity was set to vary from 0.1 to 0.3, and the step size was set to 0.03. The thoracic region conductivity was set to vary from 0.35 to 0.45, and the step size was set to 0.02. All of the biological tissue conductivity values were obtained from a previous study about biological conductivity [38].
Chest CT images of an adult male were extracted from the EIDORS project and selected as lung and thorax segment points. The process of creating the lung phantom can be separated into the following three steps:
1) Load the sequential coordinates of different regional boundaries, including the lung and thorax. In this step, three sequential coordinates should be loaded, including a left lung, a right lung and a thorax.
2) Transfer the sequential coordinates to the FEM calculation software COMSOL Multi-physics to generate FEM meshes.
3) Use the created FEM meshes to solve the forward problem based on EIDORS data by using the same method as that for the circle phantom.
Each sample in the lung phantom dataset included 208 analog measurements of voltage data as input data and 3774 conductivity elements as output labels.
Both circle and lung data were randomly separated into two parts, including test datasets and training datasets. The test datasets included 1000 sets of data for the circular phantom and 1000 sets of data for the lung phantom. The training datasets included 18, 000 sets of data for the circular phantom and 10, 000 sets of data for the lung phantom. To further verify the stability and robustness of the proposed network structure, a 20 dB random Gaussian noise signal was added to the data of the test datasets to form datasets with 20 dB noise.
For the proposed MS-DenseNet and DenseNet training, the labels were set by applying normalized conductivity distribution, including 1650 for the circle phantom and 3774 for the lung phantom. The sigmoid and the mean squared error (MSE) were selected as the output activation function and loss function, respectively, which are suitable for multidimensional regression tasks.
The network training was run on a PC with an Intel Core i7-9700, 3.00 GHz CPU and 16 GB RAM. The operating system of the computer was a 64-bit Windows 10, and the structure of the network was implemented by using the open-source deep learning library MatConvNet with MATLAB2019b implementation.
For the training process, 600 epochs with 500 mini-batches were set. The initial learning rates for the circle and lung phantoms were 0.0005 and 0.00005, respectively. The momentum was 0.9, and the L2 weight decay was set to be 0.001 to avoid overfitting. In addition, the learning rate was attenuated to 0.00005 for the circle phantom and 0.000005 for the lung phantom in the last 10 epochs. The loss function was MSE. The corresponding activation function was the sigmoid function. Adam is suitable for solving large-scale data and parameter optimization problems [39]. Thus, the solver used the Adam algorithm to keep training stable.
The conventional DenseNet and the iterative GN method were selected for comparison. The conventional DenseNet had the same training parameters as the MS-DenseNet, but the fixed learning rates were 0.0005 and 0.00005, respectively. And, they also trained with the same training datasets. The GN method was provided by the EIDORS project, using NOSER regularization. The maximum iterations were 10, the background conductivity was set to 1 for the circle phantom and 0.275 for the lung phantom and the hyperparameters were set to 0.55.
In order to compare the performance of different methods, RMSE, SSIM, MAE and ICC metrics were adopted to evaluate the reconstruction results. The RMSE, SSIM, MAE and ICC metrics between the reconstructed image and the actual image are respectively defined as follows:
RMSE(σ,ˆσ)=√n∑i=1(σi−ˆσi)2n | (9) |
SSIM(σ,ˆσ)=(2μσμˆσ+c1)(2λσ,ˆσ+c2)(μ2σ+μ2ˆσ+c1)(λ2σ+λ2ˆσ+c2)) | (10) |
MAE(σ,ˆσ)=n∑i=1|σi−ˆσi|n | (11) |
ICC(σ,ˆσ)=n∑i=1(σi−μσ)(ˆσi−μˆσ)√n∑i=1(σi−μσ)2√n∑i=1(ˆσi−μˆσ)2 | (12) |
where σi and ˆσi denote the i-th element conductivity results of the reconstructed and the actual images, respectively, and n is the number of phantom elements. Additionally, μσ and μˆσ denote the mean of σ and ˆσ, respectively, λσ and λˆσ denote the variance of σ and ˆσ, respectively, and λσ,ˆσ denotes the covariance between σ and ˆσ. The parameters c1 and c2 are constants to maintain the stability of SSIM. L is the range of the conductivity value, k1=0.01 and k2=0.03.
Figure 8 shows the average MSE between the test and training data according to epoch number for MS-DenseNet and the conventional DenseNet. Before the last 10 epochs, the learning rate settings of the two networks were the same. Figure 8(a) shows the case of the circle phantom. The MSE of the two methods quickly dropped to less than 5 in the first 30 epochs. Then, the rate of decline of the conventional DenseNet slowed down significantly and tended to stagnate after 300 epochs. The rate of decline of the conventional DenseNet is not apparent. MS-DenseNet maintained a significant decline after 300 epochs. At the same learning rate, compared with the conventional DenseNet, MS-DenseNet exhibited a better attenuation rate of the MSE curve; and, the final MSE was smaller during training. In the last 10 epochs, the learning rate of MS-DenseNet became one-tenth of the original. This part shows the comparison of MS-DenseNet before and after modification of the learning rate. In the last 10 epochs, due to the sudden decrease of the learning rate, the MSE finally declined further. The attenuation of the learning rate means that the step size of the search for the minimum MSE decreased. This adjusts the value of MSE closer to the minimum. Figure 8(b) shows the case of the lung phantom. Both methods maintained a faster rate of decline in the first 100 epochs and then maintained a steady and slow decline in the last 500 epochs. The reduction of the two methods became more unstable, especially the conventional DenseNet. It shows that the method in this paper yielded better results in terms of both the decay rate and the convergence value of the loss MSE curve given the same number of training epochs. The training time was about 117 hours, and the size of the parameters was 31.7 Mb.
The reconstructed image metrics calculated by MS-DenseNet, the conventional DenseNet and the GN method with noiseless test datasets are shown in Table 2. Compared with the conventional DenseNet, the proposed MS-DenseNet achieved an increase of 0.8, 1.5% (circle phantom), 0.4 and 0.4% (lung phantom) for the ICC and SSIM, respectively, as well as a decrease of 24.2, 28.6% (circle phantom) and 17.6, 18.7% (lung phantom) for the MAE and RMSE, respectively. Compared with the traditional GN method, MS-DenseNet achievevd a visible increase of 20.7, 25.3% (circle phantom) and 39.6, 41.6% (lung phantom) for the ICC and SSIM, respectively. The RMSE and MAE exhibited a visible decrease of 75.6, 65.9% (circle phantom) and 85.8, 89.4% (lung phantom), respectively. To facilitate comparative analysis, eight cases were randomly selected from the test datasets for observation.
Phantom | Method | RMSE | SSIM | MAE | ICC |
Circle Phantom | GN | 0.1905 | 0.7856 | 0.1193 | 0.8160 |
DenseNet | 0.0650 | 0.9702 | 0.0256 | 0.9711 | |
MS-DenseNet | 0.0464 | 0.9846 | 0.0194 | 0.9851 | |
Lung Phantom | GN | 0.0704 | 0.7017 | 0.0578 | 0.7115 |
DenseNet | 0.0123 | 0.9899 | 0.0074 | 0.9900 | |
MS-DenseNet | 0.0100 | 0.9937 | 0.0061 | 0.9931 |
Figure 9 shows the reconstructed images of eight cases extracted from the circle phantom test datasets. Table 3 shows the metrics of eight circle phantom cases on noiseless datasets. The reconstructed image of the GN method had an obscure edge and wrong estimates of the size and conductivity in the normal and abnormal conductivity regions. The implication of NOSER regularization in the GN method limited the sharp variety of conductivity distribution, which made the image edge smooth. The boundaries of different conductivity regions were difficult to distinguish. Furthermore, the region near the electrode was more prone to false predictions. The images reconstructed by the proposed MS-DenseNet and the conventional DenseNet revealed stable results. A clear and sharp edge was created between the background and the normal conductivity region in Case 2. The changes in the position and the size of the normal conductivity region were relatively small, so it was easier to reconstruct. The conventional DenseNet was unable to produce accurate images even in small abnormal regions (Cases 5 and 6); and, it yielded the worse reconstruction image in the central region (Cases 3-8). This is because the image reconstruction process of EIT is ill-posed and nonlinear, and because abnormal regions that are small or close to the center of the field will be more difficult to detect and reconstruct. Still, MS-DenseNet tended to predict two kinds of abnormal regions with a higher probability than the other methods. In Case 6, neither DenseNet methods could accurately predict the conductivity value in the region of abnormal conductivity. Compared with the DenseNet, MS-DenseNet predicted the presence of abnormal regions and yielded conductivity predictions that were closer to the truth.
Metric | Method | Case 1 | Case 2 | Case 3 | Case 4 | Case 5 | Case 6 | Case 7 | Case 8 |
RMSE | GN | 0.2246 | 0.2322 | 0.2073 | 0.2960 | 0.1281 | 0.2833 | 0.2856 | 0.2689 |
DenseNet | 0.0903 | 0.0111 | 0.0904 | 0.0718 | 0.0652 | 0.1700 | 0.2496 | 0.2274 | |
MS-DenseNet | 0.0538 | 0.0055 | 0.0473 | 0.0391 | 0.0322 | 0.1216 | 0.0745 | 0.1510 | |
SSIM | GN | 0.7797 | 0.8224 | 0.7721 | 0.7161 | 0.7932 | 0.7720 | 0.6923 | 0.7190 |
DenseNet | 0.9722 | 0.9997 | 0.9649 | 0.9871 | 0.9531 | 0.9185 | 0.8208 | 0.8351 | |
MS-DenseNet | 0.9906 | 0.9999 | 0.9907 | 0.9963 | 0.9891 | 0.9585 | 0.9840 | 0.9330 | |
MAE | GN | 0.1470 | 0.1606 | 0.1147 | 0.1804 | 0.0764 | 0.1797 | 0.1774 | 0.1637 |
DenseNet | 0.0343 | 0.0069 | 0.0351 | 0.0305 | 0.0209 | 0.0430 | 0.0688 | 0.1183 | |
MS-DenseNet | 0.0227 | 0.0032 | 0.0173 | 0.0153 | 0.0114 | 0.0319 | 0.0322 | 0.0543 | |
ICC | GN | 0.8080 | 0.8429 | 0.8146 | 0.7671 | 0.8147 | 0.7773 | 0.7335 | 0.7927 |
DenseNet | 0.9723 | 0.9997 | 0.9669 | 0.9882 | 0.9552 | 0.9201 | 0.8206 | 0.8451 | |
MS-DenseNet | 0.9926 | 0.9999 | 0.9910 | 0.9966 | 0.9892 | 0.9596 | 0.9841 | 0.9347 |
Figure 10 shows the reconstructed images of eight cases extracted from the lung phantom test datasets. Table 4 shows the metrics of eight lung phantom cases on noiseless datasets. The GN method was unable to reconstruct the lung edge, and high conductivity artifacts were produced in the thoracic region. The two DenseNet-based methods accurately restored the boundaries of the lung conductivity region in Case 2. In Cases 1, 3, 4, 7 and 8, the conductivity of the anomaly region had a high variation. Both DenseNet-based methods were able to predict a change in lung conductivity; but, MS-DenseNet yielded a more accurate and more evident edge, while the conventional DenseNet underestimated the region of conductivity change or incorrectly estimated the conductivity value, as can be observed for the left lung in Cases 3 and 7. In Cases 5 and 6, the conductivity of the anomaly region had a small size, which is more difficult to predict. The conventional DenseNet could not recognize a conductivity change in the small region. However, the two DenseNet-based methods incorrectly estimated the conductivity value at the center of the variation region, which can be observed in the prediction of the left lung in Case 3.
Metric | Method | Case 1 | Case 2 | Case 3 | Case 4 | Case 5 | Case 6 | Case 7 | Case 8 |
RMSE | GN | 0.0471 | 0.0838 | 0.0629 | 0.0427 | 0.0543 | 0.0557 | 0.0504 | 0.0540 |
DenseNet | 0.0194 | 0.0073 | 0.0203 | 0.0170 | 0.0178 | 0.0122 | 0.0143 | 0.0172 | |
MS-DenseNet | 0.0147 | 0.0042 | 0.0156 | 0.0121 | 0.0113 | 0.0082 | 0.0106 | 0.0138 | |
SSIM | GN | 0.6785 | 0.6888 | 0.6371 | 0.7696 | 0.7331 | 0.7386 | 0.7761 | 0.7234 |
DenseNet | 0.9511 | 0.9980 | 0.9684 | 0.9681 | 0.9747 | 0.9891 | 0.9846 | 0.9750 | |
MS-DenseNet | 0.9720 | 0.9994 | 0.9809 | 0.9829 | 0.9897 | 0.9952 | 0.9917 | 0.9844 | |
MAE | GN | 0.0386 | 0.0691 | 0.0509 | 0.0340 | 0.0443 | 0.0459 | 0.0417 | 0.0445 |
DenseNet | 0.0111 | 0.0045 | 0.0126 | 0.0112 | 0.0099 | 0.0050 | 0.0096 | 0.0094 | |
MS-DenseNet | 0.0089 | 0.0034 | 0.0090 | 0.0069 | 0.0066 | 0.0044 | 0.0071 | 0.0079 | |
ICC | GN | 0.6949 | 0.7099 | 0.6564 | 0.7782 | 0.7466 | 0.7552 | 0.7901 | 0.7376 |
DenseNet | 0.9521 | 0.9980 | 0.9684 | 0.9686 | 0.9747 | 0.9892 | 0.9850 | 0.9753 | |
MS-DenseNet | 0.9725 | 0.9995 | 0.9809 | 0.9831 | 0.9897 | 0.9952 | 0.9918 | 0.9844 |
In order to further verify the stability and robustness of different methods, test datasets with a 20-dB random Gaussian noise signal were used in the experiment. Table 5 shows the average metric results for 2000 datasets with 20-dB Gaussian noise. Compared with the noiseless datasets, the conventional DenseNet had a decrease in ICC and SSIM of 2.2 and 2.3% for the circle phantom and 0.9 and 1.2% for the lung phantom, respectively, as well as an increase in MAE and RMSE of 51.1 and 38.9% for the circle phantom and 45.9 and 53.6% for the lung phantom, respectively. The decline of four metrics in MS-DenseNet achieved 2.1, 2.1, 67.0 and 39.7% for the circle phantom and 0.6, 0.6, 59.0 and 40.0% for the lung phantom.
Phantom | Method | RMSE | SSIM | MAE | ICC |
Circle Phantom | GN | 0.1905 | 0.7856 | 0.1193 | 0.8160 |
DenseNet | 0.0903 | 0.9481 | 0.0387 | 0.9497 | |
MS-DenseNet | 0.0770 | 0.9630 | 0.0324 | 0.9640 | |
Lung Phantom | GN | 0.0704 | 0.7017 | 0.0578 | 0.7115 |
DenseNet | 0.0189 | 0.9774 | 0.0137 | 0.9809 | |
MS-DenseNet | 0.0140 | 0.9880 | 0.0097 | 0.9881 |
Figure 11 shows the same reconstructed images as Figure 9, calculated with the 20-dB signal-to-noise ratio (SNR) noise datasets. Figure 12 shows the same reconstructed images as Figure 10, calculated with the 20-dB SNR noise datasets. Table 6 shows the metrics of eight circle phantom cases on the 20-dB noise datasets. Table 7 shows the metrics of eight lung phantom cases on the 20-dB noise datasets. The GN method has almost no influence because stability comes from the NOSER regularization. The metrics of MS-DenseNet were still better. The 20-dB SNR noise did not have a significant influence on the normal conductivity region or the lung edge. Still, the conductivity prediction was more likely to lead to false distribution in the abnormal regions.
Metric | Method | Case 1 | Case 2 | Case 3 | Case 4 | Case 5 | Case 6 | Case 7 | Case 8 |
RMSE | GN | 0.2246 | 0.2322 | 0.2073 | 0.2960 | 0.1281 | 0.2833 | 0.2856 | 0.2689 |
DenseNet | 0.0938 | 0.0140 | 0.0884 | 0.0785 | 0.0733 | 0.1716 | 0.2560 | 0.2269 | |
MS-DenseNet | 0.0511 | 0.0083 | 0.0582 | 0.0342 | 0.0549 | 0.1195 | 0.0873 | 0.1468 | |
SSIM | GN | 0.7797 | 0.8224 | 0.7721 | 0.7161 | 0.7932 | 0.7720 | 0.6923 | 0.7190 |
DenseNet | 0.9709 | 0.9995 | 0.9664 | 0.9847 | 0.9416 | 0.9168 | 0.8133 | 0.8366 | |
MS-DenseNet | 0.9914 | 0.9998 | 0.9859 | 0.9972 | 0.9673 | 0.9598 | 0.9777 | 0.9370 | |
MAE | GN | 0.1470 | 0.1606 | 0.1147 | 0.1804 | 0.0764 | 0.1797 | 0.1774 | 0.1637 |
DenseNet | 0.0387 | 0.0090 | 0.0315 | 0.0311 | 0.0207 | 0.0443 | 0.0699 | 0.1201 | |
MS-DenseNet | 0.0226 | 0.0050 | 0.0195 | 0.0146 | 0.0153 | 0.0320 | 0.0349 | 0.0519 | |
ICC | GN | 0.8080 | 0.8429 | 0.8146 | 0.7671 | 0.8147 | 0.7773 | 0.7335 | 0.7927 |
DenseNet | 0.9718 | 0.9995 | 0.9686 | 0.9856 | 0.9428 | 0.9184 | 0.8132 | 0.8457 | |
MS-DenseNet | 0.9929 | 0.9998 | 0.9863 | 0.9976 | 0.9686 | 0.9609 | 0.9780 | 0.9384 |
Metric | Method | Case 1 | Case 2 | Case 3 | Case 4 | Case 5 | Case 6 | Case 7 | Case 8 |
RMSE | GN | 0.0471 | 0.0838 | 0.0629 | 0.0427 | 0.0543 | 0.0557 | 0.0504 | 0.0540 |
DenseNet | 0.0198 | 0.0066 | 0.0190 | 0.0164 | 0.0172 | 0.0127 | 0.0165 | 0.0174 | |
MS-DenseNet | 0.0159 | 0.0057 | 0.0161 | 0.0128 | 0.0117 | 0.0089 | 0.0119 | 0.0144 | |
SSIM | GN | 0.6785 | 0.6888 | 0.6371 | 0.7696 | 0.7331 | 0.7386 | 0.7761 | 0.7234 |
DenseNet | 0.9485 | 0.9983 | 0.9714 | 0.9696 | 0.9758 | 0.9881 | 0.9802 | 0.9747 | |
MS-DenseNet | 0.9684 | 0.9990 | 0.9797 | 0.9809 | 0.9890 | 0.9942 | 0.9898 | 0.9830 | |
MAE | GN | 0.0386 | 0.0691 | 0.0509 | 0.0340 | 0.0443 | 0.0459 | 0.0417 | 0.0445 |
DenseNet | 0.0112 | 0.0045 | 0.0108 | 0.0103 | 0.0097 | 0.0062 | 0.0122 | 0.0097 | |
MS-DenseNet | 0.0101 | 0.0047 | 0.0094 | 0.0083 | 0.0072 | 0.0054 | 0.0082 | 0.0090 | |
ICC | GN | 0.6949 | 0.7099 | 0.6564 | 0.7782 | 0.7466 | 0.7552 | 0.7901 | 0.7376 |
DenseNet | 0.9500 | 0.9984 | 0.9715 | 0.9697 | 0.9761 | 0.9881 | 0.9819 | 0.9747 | |
MS-DenseNet | 0.9684 | 0.9991 | 0.9797 | 0.9815 | 0.9892 | 0.9942 | 0.9900 | 0.9830 |
An EIT system was used for practical verification experiments, as shown in Figure 13. Different concentrations of saline formed the background (1.0 S/m) or chest region (0.4 S/m), and their conductivity values were measured by using a conductivity meter. The normal region was replaced by nylon rods (0.0 S/m), and the agar model replaced the abnormal (2.0 S/m) and lung (0.2 S/m) regions. The device was composed of a measurement region, an analog acquisition board, a digital acquisition board and the upper PC. The circle image region was made of acrylic, where the inner diameter was 19 cm. (The lung region was the same size as the phantom.) The analog acquisition board had an AD8039 device as the voltage-controlled current source to release the excitation current. The voltage signal was connected to AD8251 and AD8253 devices to complete the amplification. An OPA1612 device was used as the voltage follower buffer. The digital acquisition board used a programmable digital signal processing development board to collect digital signals and control electrode conversion. The agar model was made by heating 6% agar powder, sodium chloride, 3% hydroxyethyl cellulose and 1% formalin solution. The conductivity of the agar model was measured by using a conductivity meter before solidification.
The network trained on the simulation training set was directly applied to the experimental models without training on the experimental data. A successful transition to the experimental data reflects the robustness of the proposed MS-DenseNet method.
Figure 14 presents the experimental reconstructed images for five cases. Table 8 shows the metrics for five cases under the conditions of experimental voltage measurement. The conventional DenseNet incorrectly judged the normal region in Cases 1 and 4. In addition, more artifacts were generated in the center of the image region in Cases 2 and 3. The results of the MS-DenseNet reconstruction were more stable than those of the other methods, especially in terms of the judgment of abnormal region.
Metric | Method | Case 1 | Case 2 | Case 3 | Case 4 | Case 5 |
RMSE | GN | 0.2026 | 0.2664 | 0.3332 | 0.3280 | 0.1918 |
DenseNet | 0.1746 | 0.2009 | 0.3255 | 0.3871 | 0.0044 | |
MS-DenseNet | 0.1530 | 0.0792 | 0.1187 | 0.1572 | 0.0036 | |
SSIM | GN | 0.8101 | 0.7708 | 0.6969 | 0.7128 | 0.4041 |
DenseNet | 0.8924 | 0.8857 | 0.7560 | 0.6626 | 0.9993 | |
MS-DenseNet | 0.9161 | 0.9834 | 0.9704 | 0.9487 | 0.9997 | |
MAE | GN | 0.1310 | 0.1748 | 0.2233 | 0.2258 | 0.1229 |
DenseNet | 0.0689 | 0.1068 | 0.1911 | 0.2185 | 0.0040 | |
MS-DenseNet | 0.0554 | 0.0476 | 0.0668 | 0.0840 | 0.0032 | |
ICC | GN | 0.8532 | 0.8157 | 0.7741 | 0.7817 | 0.5395 |
DenseNet | 0.8923 | 0.8993 | 0.7762 | 0.6807 | 0.9995 | |
MS-DenseNet | 0.9162 | 0.9871 | 0.9743 | 0.9529 | 0.9998 |
The results of image reconstruction, shown in Figures 9-12, demonstrate the advantages of the MS-DenseNet method in terms of image accuracy. This section will discuss how certain operations or structures in the proposed method contribute to the performance of EIT image reconstruction. Each ablation experiment in the discussion used the same training datasets for experiments.
Multi-scale convolution can be traced back to GoogLeNet [41], where the inception block demonstrated the advantage of operating with multi-scale convolutional kernels instead of single-sized kernels. The role of multi-scale convolution in neural networks is to capture information from data at different scales, and obtaining more input features is essential for EIT image reconstruction [41]. For the same feature map, the calculation expectations of the single-scale and multi-scale convolution operation methods were the same. Still, the operation of the multi-scale convolutional kernel was able to process the feature information of different scales simultaneously, and the simple zero-padding operation was able to control the output data of different convolutional kernels to the same size. Then, the output data were spliced together so that the next layer could extract the feature information of different scales simultaneously, as well as improve the utilization of the network's computing resources. The role of parallel dense blocks is similar to that of parallel multi-scale convolution, which is to extract multiple kinds of features and fuse them.
In order to verify whether the new dense blocks can improve the network's performance, ablation experiments should be performed. Without changing any other structures, three different types of dense blocks were respectively replaced with conventional dense blocks to compare their MSE.
Table 9 shows the impact of different dense block types on the noiseless circle phantom final output. The results prove that all three dense block types can improve the network's fitting performance and reduce the MSE in the final output. In contrast to Dense Block type1 and Dense Block type2, Dense Block type3 applied 5 × 5 convolution after 3 × 3 convolution, which is equivalent to obtaining a larger-sized convolutional kernel [42]. Dense Block type3 reflects a better effect in this experiment, which is due to the stronger spatial correlation of the arranged data in the voltage matrix, and it provides a feasible theoretical basis for the future design of a specific convolutional kernel determined by the Jacobian matrix.
Dense block | MSE |
Conventional Dense Block | 2.657 |
Dense block type1 | 1.737 |
Dense block type2 | 1.722 |
Dense block type3 | 1.633 |
In the conventional DenseNet, the front layer uses maximum pooling to extract feature information, while the average pooling layer is used for subsequent connected blocks to preserve important high-dimensional details [35]. This is suitable for image classification and other problems. But, the role of the pooling layer in image classification and image reconstruction is different. In the case of image classification, the input image contains information that is not useful for determining the final label, such as background information. For EIT image reconstruction, any input voltage measurement will affect the final result, and highly correlated measurements will have a significant impact on elements with small changes. This means that input characteristics should be saved to the next layer as much as possible. Max pooling was inferior to average pooling, and average pooling was inferior to 2 × 2 convolution in terms of ability to preserve features [43].
As shown in Table 10, different pooling settings were implemented in the conventional DenseNet to search for better metrics of circle datasets without any noise. Max pooling yielded the worst effect among the three methods. This is because max pooling ignores most of the information and only keeps the maximum value in a 2 × 2 matrix. When average pooling was applied, the MSE decreased. The average pooling was affected by all pixels in the 2 × 2 matrix, thus retaining all valid information in the next layer. The average pooling compressed all 2 × 2 matrices in one dimension with the same weight, resulting in indistinguishable data compression for the network. The 2 × 2 convolution operation effectively adjusted the importance of data in different dimensions while keeping all data valid. Therefore, a small amount of 2 × 2 convolution can be added to the pooling layer channel to realize a substantial improvement. However, considering that the introduction of 2 × 2 convolution increased the storage of the network and the effect of increasing the number of 2 × 2 convolutions after 75% of the channel is not apparent, the final pooling structure consisted of 75% 2 × 2 convolutions and 25% average pooling layers.
Net type | Max pooling | Average pooling | 2 × 2 convolution | MSE |
Type 1 | 100% | 0% | 0% | 3.028 |
Type 2 | 50% | 50% | 0% | 2.874 |
Type 3 | 25% | 75% | 0% | 2.706 |
Type 4 | 0% | 100% | 0% | 2.554 |
Type 5 | 0% | 50% | 50% | 1.866 |
Type 6 | 0% | 25% | 75% | 1.721 |
Type 7 | 0% | 0% | 100% | 1.634 |
The largest initial learning rate was selected under the premise of ensuring the stable decline of the network, because the network uses Adam as the solver, which prevents severe sway in the later epochs of the network training compared to the stochastic gradient descent method. However, an appropriate learning rate setting is more conducive to the learning of neural networks. Four different learning rate settings were implemented in the conventional DenseNet with the noiseless circle phantom to find a suitable learning rate setting. The Type (a) setting reduced the learning rate to 0.1 times the initial learning rate after 300 epochs. Figure 15(a) shows that, after the learning rate was reduced, the MSE exhibited a significant decrease in the beginning; but, the reduction of the MSE then began to slow down, and the final result was not as good as before the modification. Similarly, the Type (b) setting reduced the learning rate to 0.1, 0.01, 0.001, 0.0001 and 0.00001 times the initial learning rate after 100, 200, 300, 400 and 500 epochs, respectively. As shown in Figure 15(b), the same significant decrease in the learning rate occurred in the beginning, but the final MSE values were still not good. In Figure 15(c), an impulse learning setting was used; it maintained the initial learning rate in most epochs, it was but 0.1 times the initial learning rate for the last five epochs of every 100 epochs. The final MSE was almost the same as that associated with the classic fixed learning rate, but every pulse was able to trap the MSE into a local minimum. In Figure 15(d), the implemented learning rate is as follows:
s(i)=s×0.99i,i=1,2,3⋯600 | (13) |
where s is the initial learning rate, i is the number of epochs of the learning process and s(i) is the learning rate of i epochs. The results show that a slowly decreasing learning rate will reduce the performance of the DenseNet on EIT image data. A large number of locally optimal solutions were distributed around each epoch of training, and a small learning rate caused the training to fall into a local optimum. However, the network can be trained to a local optimum after nearing the global optimum. Finally, the learning rate setting was determined as a fixed initial learning rate in the first 590 epochs and 0.1 times the initial learning rate in the last 10 epochs.
To further demonstrate the performance of the proposed MS-DenseNet in terms of EIT image reconstruction, a comparative analysis was carried out with other methods that emerged in recent years. Table 11 shows a comparison of the evaluation metrics for EIT image reconstruction for different methods. The metrics in Table 11 are those based on noiseless datasets.
Methods\Metric | Year | RMSE | SSIM | ICC |
PLS [44] | 2018 | − | 0.95 | 0.93 |
CNN [26] | 2019 | − | − | 0.9472 |
ANHTV [45] | 2021 | 0.1818 | − | 0.91 |
BLS [46] | 2022 | 0.16 | − | 0.93 |
M-STGNN[47] | 2022 | 0.0378 | 0.9693 | − |
CWGAN-AM [48] | 2022 | − | 0.9836 | − |
MS-DenseNet | 2022 | 0.0464 | 0.9846 | 0.9851 |
Although the evaluation indexes of different datasets cannot be directly compared, the datasets from the selected literature are similar to those of this study, which has certain reference value. These characteristics will not change when other similar datasets are used to train and test the network. The comparison results also verified the good performance of the proposed network in terms of fitting and generalization ability.
MS-DenseNet was able to determine the exact locations and sizes of regions with different conductivities more accurately than traditional iterative methods. MS-DenseNet also has significant advantages over the conventional DenseNet, specifically in terms of the MAE and RMSE. But, in the case of the ICC and SSIM, the benefit was not as huge as imagined. The reason is that both the conventional DenseNet and MS-DenseNet were close to the maximum, which may be related to the complexity of the phantom design. In addition, when the size of the abnormal area was small and close to the middle area, the effect of the reconstructed image was worse than expected. In the future, the advantages of other traditional algorithms can be added to the neural network to further improve the robustness of the algorithm and use the dataset closer to the actual conductivity distribution of human tissue to enhance the network function.
With sufficient prior information, the method can image the conductivity distribution of human lungs and blood vessels in limbs. In the future, efforts on studying the problem of reduced noise immunity will be made, and the specific dataset will be more similar to the actual conductivity distribution of the human tissue to enhance the network function.
This paper proposed an EIT image reconstruction method based on DenseNet with multi-scale convolution. Regarding the proposed network, it has three different multi-scale convolutions that form different types of dense blocks to extract feature information in parallel, which improved the generalization ability of the network. The hybrid pooling module, including a 2 × 2 convolutional layer and average pooling layer, was used to improve the information flow of the voltage data and reduce the loss of information during the pooling process. For the network training, a global learning rate setting has been proposed, which was demonstrated to optimize the fitting effect of the network. The simulation dataset and measured data were tested and analyzed with RMSE, SSIM, MAE and ICC as evaluation indicators. The results showed that the method proposed in this paper is competitive with other methods and can obtain reconstructed images with clear boundaries, fewer artifacts, higher resolution, high robustness and anti-noise ability.
This research was funded in part by the National Natural Science Foundation of China under Grant Nos. U22A20221, 61836011 and 71790614. The authors are also grateful for funding from the Fundamental Research Funds for the Central Universities 2020GFZD008, the 111 Project (B16009), Natural Science Foundation of Liaoning Province (2021-MS093, 2022-MS-119, 2021-BS-054) and the Basic Scientific Research Project of the Education Department of Liaoning Province in 2021(LJKZ0014).
The authors declare that there is no conflict of interest.
[1] |
Bajaj M, Vijh AM (1995) Trading Behavior and the Unbiasedness of the Market Reaction to Dividend Announcement. J Finance 50: 255-279. doi: 10.1111/j.1540-6261.1995.tb05173.x
![]() |
[2] |
Baker M, Stein J (2004) Market Liquidity as a Sentiment Indicator. J Financ Mark 7: 271-299. doi: 10.1016/j.finmar.2003.11.005
![]() |
[3] | Bamber LS, Barron OE, Stober TL (1997) Trading Volume and Different Aspects of Disagreement Coincident with Earnings Announcements. The Account Rev 72: 575-597. |
[4] |
Bamber LS, Barron OE, Stober TL (1999) Differential Interpretations and Trading Volume. J Financ Quant Anal 34: 369-386. doi: 10.2307/2676264
![]() |
[5] |
Bamber LS, Barron OE, Stevens DE (2011) Trading Volume around Earnings Announcements and Other Financial Reports: Theory, Research Design, Empirical Evidence, and Directions for Future Research. Contemp Account Res 28: 431-471. doi: 10.1111/j.1911-3846.2010.01061.x
![]() |
[6] |
Barber BM, Odean T (2008) All That Glitters: The Effect of Attention and News on the Buying Behavior of Individual and Institutional Investors. Rev Financ Stud 21: 785–818. doi: 10.1093/rfs/hhm079
![]() |
[7] |
Barron OE, Harris DG, Stanford M (2005) Evidence That Investors Trade on Private Event-Period Information around Earnings Announcements. Account Rev 80: 403-421. doi: 10.2308/accr.2005.80.2.403
![]() |
[8] |
Basci E, Ozyildinm S, Aydogan K (1996) A Note on Price–Volume Dynamics in an Emerging Stock Market. J Banking Finance 20: 389–400. doi: 10.1016/0378-4266(95)00003-8
![]() |
[9] |
Beaver WH (1968) The Information Content of Annual Earnings Announcements. Empirical Research in Accounting: Selected Studies, Supplement to J Account Res 6: 67–92. doi: 10.2307/2490070
![]() |
[10] |
Beggs A, Graddy K (2009) Anchoring Effects: Evidence from Art Auctions. Am Econ Rev 99: 1027-1039. doi: 10.1257/aer.99.3.1027
![]() |
[11] |
Biswas A, Burton S (1993) Consumer Perceptions of Tensile Price Claims in Advertisements: An Assessment of Claim Types across Different Discount Levels. J Acad Mark Sci 21: 217–229. doi: 10.1177/0092070393213005
![]() |
[12] |
Blume ME, Mackinlay AC, Terker B (1989) Order Imbalances and Stock Movements on October 19 and 20, 1987. J Finance 44: 827–848. doi: 10.1111/j.1540-6261.1989.tb02626.x
![]() |
[13] |
Bowman NA, Bastedo MN (2011) Anchoring Effects in World University Rankings: Exploring Biases in Reputation Scores. Higher Educ 61: 431-444. doi: 10.1007/s10734-010-9339-1
![]() |
[14] |
Caginalpa G, Desantisa M (2011) Stock Price Dynamics: Nonlinear Trend, Volume, Volatility, Resistance and Money Supply. Quant Finance 11: 849-861. doi: 10.1080/14697680903220356
![]() |
[15] |
Campbell JY, Grossman SJ, Wang J (1993) Trading Volume and Serial Correlation in Stock Returns. Q J Econ 108: 905–939. doi: 10.2307/2118454
![]() |
[16] |
Campbell SD, Sharpe SA (2009) Anchoring Bias in Consensus Forecasts and its Effect on Market Prices. J Financ Quant Anal 44: 369-390. doi: 10.1017/S0022109009090127
![]() |
[17] |
Cen L, Hilary G, Wei KCJ (2013) The Role of Anchoring Bias in the Equity Market: Evidence from Analysts' Earnings Forecasts and Stock Returns. J Financ Quant Anal 48: 47-76. doi: 10.1017/S0022109012000609
![]() |
[18] |
Cervone D, Peake PK (1986) Anchoring, Efficacy, and Action: The Influence of Judgmental Heuristics on Self-Efficacy Judgment and Behavior. J Pers Soc Psychol 50: 492–501. doi: 10.1037/0022-3514.50.3.492
![]() |
[19] |
Chapman GB, Johnson EJ (1994) The Limits of Anchoring. J Behav Decis Making, 7: 223–242. doi: 10.1002/bdm.3960070402
![]() |
[20] |
Chen G, Firth M, Rui OM (2001) The Dynamic Relation between Stock Returns, Trading Volume and Volatility. Financ Rev 36: 153-174. doi: 10.1111/j.1540-6288.2001.tb00024.x
![]() |
[21] | Chordia T, Huh S-W, Subrahmanyam A (2007) The Cross-Section of Expected Trading Activity. Rev Financ Stud 30: 709-740. |
[22] | Crouch RL (1970) A Nonlinear Test of the Random-Walk Hypothesis. Am Econ Rev 60: 199-202. |
[23] |
De Long B, Shleifer A, Summers L, Waldmann R (1990) Positive Feedback Investment Strategies and Destabilizing Rational Speculation. J Finance 45: 379-386. doi: 10.1111/j.1540-6261.1990.tb03695.x
![]() |
[24] | Ehrbeck T, Waldman R (1996) Why are Professional Forecasters Biased? Agency Versus Behavioral Explanations. Q J Econ 111: 21-40. |
[25] |
English B (2008) When Knowledge Matters-Differential Effects of Available Knowledge in Standard and Basic Anchoring Tasks. Eur J Soc Psychol 38: 896-904. doi: 10.1002/ejsp.479
![]() |
[26] |
English B, Mussweiler T (2001) Sentencing under Uncertainty: Anchoring Effects in the Courtroom. J Appl Soc Psychol 31: 1535–1551. doi: 10.1111/j.1559-1816.2001.tb02687.x
![]() |
[27] | Epps TW (1975) Security Price Changes and Transaction Volumes: Theory and Evidence. Am Econ Rev 65: 586–597. |
[28] |
Epps TW (1977) Security Price Changes and Transaction Volumes: Some Additional Evidence. J Financ Quant Anal 12: 141–146. doi: 10.2307/2330293
![]() |
[29] |
Epps TW, Epps ML (1976) The Stochastic Dependence of Security Price Changes and Transaction Volumes: Implications for the Mixture-of-Distributions Hypothesis. Econometrica 44: 305-321. doi: 10.2307/1912726
![]() |
[30] |
Fisher KL, Statman M (2000) Cognitive Biases in Market Forecasts. J Portf Manage 27: 72-81. doi: 10.3905/jpm.2000.319785
![]() |
[31] |
Galinsky AD, Mussweiler T (2001) First Offers as Anchors: The Role of Perspective-Taking and Negotiator Focus. J Pers Soc Psychol 81: 657–669. doi: 10.1037/0022-3514.81.4.657
![]() |
[32] |
Gallant R, Rossi P, Tauchen G (1992) Stock Prices and Volume. Rev Financ Stud 5: 199-242. doi: 10.1093/rfs/5.2.199
![]() |
[33] |
Garfinkel JA, Sokobin J (2006) Volume, Opinion Divergence, and Returns: A Study of Post-Earnings Announcement Drift. J Account Res 44: 85-112. doi: 10.1111/j.1475-679X.2006.00193.x
![]() |
[34] |
Gervais S, Kaniel R, Mingelgrin DH (2001) The High Volume Return Premium. J Finance 56: 877–919. doi: 10.1111/0022-1082.00349
![]() |
[35] |
Glaser M, Weber M (2009) Which Past Returns Affect Trading Volume? J Financ Mark 12: 1-31. doi: 10.1016/j.finmar.2008.03.001
![]() |
[36] | Griffin M, Nardari F, Stulz RM (2007) Do Investors Trade More When Stocks Have Performed Well? Evidence from 46 Countries. Rev Financ Stud 20: 905–951. |
[37] | Gruen DK, Gizycki MC (1993) Explaining Forward Discount Bias: Is It Anchoring? Princeton University Woodrow Wilson School Discussion Paper in Economics 164. |
[38] | Harris L (1983) The Joint Dislrihuiion of Speculative Prices and of Daily Trading Volume. Working Paper, University of Southern CA. |
[39] |
Harris M, Raviv A (1993) Differences of Opinion Make a Horse Race. Rev Financ Stud 6: 473-506. doi: 10.1093/rfs/5.3.473
![]() |
[40] |
Hirshleifer D, Subrahmanyam A, Titman S (1994) Security Analysis and Trading Patterns When Some Investors Receive Information before Others. J Finance 49: 1665-1698. doi: 10.1111/j.1540-6261.1994.tb04777.x
![]() |
[41] |
Hirshleifer D, Subrahmanyam A, Titman S (2006) Feedback and the Success of Irrational Investors. J Financ Econ 81: 311-338. doi: 10.1016/j.jfineco.2005.05.006
![]() |
[42] | Holthausen RW, Verrecchia RE (1990) The Effect of Informedness and Consensus on Price and Volume Behavior. Account Rev 65: 191-208. |
[43] |
Hong H, Stein JC (1999) A Unified Theory of Underreaction, Momentum Trading, and Overreaction in Asset Markets. J Finance 54: 2143-2184. doi: 10.1111/0022-1082.00184
![]() |
[44] |
Hong H, Stein JC (2007) Disagreement and the Stock Market. J Econ Perspect 21: 109-128. doi: 10.1257/jep.21.2.109
![]() |
[45] |
Hong H, Yu J (2009) Gone Fishin': Seasonality in Trading Activity and Asset Prices. J Financ Mark 12: 672-702. doi: 10.1016/j.finmar.2009.06.001
![]() |
[46] |
Huddart S, Lang M, Yetman MH (2009) Volume and Price Patterns around a Stock's 52-Week Highs and Lows: Theory and Evidence. Manage Sci 55: 16-31. doi: 10.1287/mnsc.1080.0920
![]() |
[47] | Israeli D (2015) Trading Volume Reactions to Earnings Announcements and Future Stock Returns. Working Paper, Interdisciplinary Center Herzliya. |
[48] |
Jacowitz KE, Kahneman D (1995) Measures of Anchoring in Estimation Tasks. Pers Soc Psychol Bull 21: 1161–1166. doi: 10.1177/01461672952111004
![]() |
[49] | Jain PC, Joh G-H (1986) The Dependence between Hourly Prices and Trading Volume. Working Paper, The Wharton School, University of Pennsylvania. |
[50] | Kahneman D, Slovic P, Tversky A (1982) Judgment under Uncertainty: Heuristics and Biases, New York: Cambridge University Press. |
[51] |
Kandel E, Pearson N (1995) Differential Interpretation of Public Signals and Trade in Speculative Markets. J Polit Econ 103: 831-872. doi: 10.1086/262005
![]() |
[52] |
Karpoff JM (1986) A Theory of Trading Volume. J Finance, 41: 1069-1087. doi: 10.1111/j.1540-6261.1986.tb02531.x
![]() |
[53] |
Karpoff JM (1987) The Relation between Price Changes and Trading Volume: A Survey. J Financ Quant Anal 22: 109-126. doi: 10.2307/2330874
![]() |
[54] | Khan SU, Rizwan F (2008) Trading Volume and Stock Returns: Evidence from Pakistan's Stock Market. Int Rev Bus Res Pap 4: 151-162. |
[55] |
Kim O, Verrecchia RE (1991) Market Reaction to Anticipated Announcements. J Financ Econ 30: 273-309. doi: 10.1016/0304-405X(91)90033-G
![]() |
[56] |
Kim O, Verrecchia RE (1994) Market Liquidity and Volume around Earnings Announcements. J Account Econ 17: 41–67. doi: 10.1016/0165-4101(94)90004-3
![]() |
[57] |
Kim O, Verrecchia RE (1997) Pre-Announcement and Event-Period Private Information. J Account Econ 24: 395-419. doi: 10.1016/S0165-4101(98)00013-5
![]() |
[58] |
Kliger D, Kudryavtsev A (2010) The Availability Heuristic and Investors' Reaction to Company-Specific Events. J Behav Finance 11: 50-65. doi: 10.1080/15427561003591116
![]() |
[59] | Kudryavtsev A, Cohen G (2010a) Illusion of Relevance: Anchoring in Economic and Financial Knowledge. Int J Econ Res 1: 86-101. |
[60] | Kudryavtsev A, Cohen G (2010b) Anchoring and Pre-Existing Knowledge in Economic and Financial Settings. Am J Soc Manage Sci 1: 164-180. |
[61] |
Lakonishok J, Vermaelen T (1986) Tax-Induced Trading around Ex-Dividend Days. J Financ Econ 16: 287-319. doi: 10.1016/0304-405X(86)90032-2
![]() |
[62] | Lee SB, Rui OM (2002) The Dynamic Relationship between Stock Return and Trading Volume: Domestic and Cross-Country Evidence. J Banking Finance 26: 51-78. |
[63] | Leung TC, Tsang KP (2013) Anchoring and Loss Aversion in the Housing Market: Implications on Price Dynamics, China Econ Rev 24: 42-54. |
[64] |
Llorente G, Michaely R, Saar G, Wang J (2002) Dynamic Volume-Return Relation of Individual Stocks. Rev Financ Stud 15: 1005-1047. doi: 10.1093/rfs/15.4.1005
![]() |
[65] |
Lo AW, Wang J (2006) Trading Volume: Implications of an Intertemporal Capiral Asset Pricing Model. J Finance 61: 2805–2840. doi: 10.1111/j.1540-6261.2006.01005.x
![]() |
[66] |
Mussweiler T (2001) The Durability of Anchoring Effects. Eur J Soc Psychol 31: 431–442. doi: 10.1002/ejsp.52
![]() |
[67] | Mussweiler T, Strack F (1999a) Comparing is Believing: A Selective Accessibility Model of Judgmental Anchoring, In: Stroebe W, Hewstone M, European review of social psychology, Chichester: Wiley, 10: 135-167. |
[68] | Mussweiler T, Strack F (1999b) Hypothesis-Consistent Testing and Semantic Priming in the Anchoring Paradigm: A Selective Accessibility Model. J Exp Soc Psychol 35: 136–164. |
[69] |
Mussweiler T, Strack F (2000) Numeric Judgment under Uncertainty: The Role of Knowledge in Anchoring. J Exp Soc Psychol 36: 495–518. doi: 10.1006/jesp.1999.1414
![]() |
[70] |
Ndjadingwe E, Radikoko I (2015) Investigating the Effects of Dividends Pay-out on Stock Prices and Traded Equity Volumes of BSE Listed Firms. Int J Innovation and Econ Dev 1: 24-37. doi: 10.18775/ijied.1849-7551-7020.2015.14.2002
![]() |
[71] | Osborne MFM (1959) Brownian Motion in the Stock Market. Oper Res 1: 145-173. |
[72] | Pathirawasam C (2011) The Relationship between Trading Volume and Stock Returns. J Competitiveness 3: 41-49. |
[73] |
Pisedtasalasai A, Gunasekarage A (2007) Causal and Dynamic Relationships among Stock Returns, Return Volatility and Trading Volume: Evidence from Emerging Markets in South-East Asia. Asia-Pac Financ Mark 14: 277-297. doi: 10.1007/s10690-008-9063-3
![]() |
[74] |
Plous S (1989) Thinking the Unthinkable: The Effects of Anchoring on Likelihood Estimates of Nuclear War. J Appl Soc Psychol 19: 67–91. doi: 10.1111/j.1559-1816.1989.tb01221.x
![]() |
[75] | Remorov R (2014) Stock Price and Trading Volume during Market Crashes. Int J Mark Stud 6: 21-30. |
[76] | Rutledge DJS (1984) Trading Volume and Price Variability: New Evidence on the Price Effects of Speculation, In: Peck AE, Selected Writings on Futures Markets: Research Directions in Commodity Markets, Chicago: Chicago Board of Trade, 237-251. |
[77] |
Saatccioglu K, Starks LT (1998) The Stock Price–Volume Relationship in Emerging Stock Markets: The Case of Latin America. Int J Forecast 14: 215–225. doi: 10.1016/S0169-2070(98)00028-4
![]() |
[78] |
Safvenblad P (2000) Trading Volume and Autocorrelation: Empirical Evidence from the Stockholm Stock Exchange. J Banking Finance 24: 1275–1287. doi: 10.1016/S0378-4266(99)00071-0
![]() |
[79] |
Schwert W (1989) Why Does Stock Market Volatility Change Over Time? J Finance 44: 1115-1155. doi: 10.1111/j.1540-6261.1989.tb02647.x
![]() |
[80] |
Simonson I, Drolet A (2004) Anchoring Effects on Consumers' Willingness-to-Pay and Willingness-to-Accept. J Consum Res 31: 681-690. doi: 10.1086/425103
![]() |
[81] |
Statman M, Thorley S, Vorkink K (2006) Investor Overconfidence and Trading Volume. Rev Financ Stud 19: 1531-1565. doi: 10.1093/rfs/hhj032
![]() |
[82] |
Strack F, Mussweiler T (1997) Explaining the Enigmatic Anchoring Effect: Mechanisms of Selective Accessibility. J Pers Soc Psychol 73: 437–446. doi: 10.1037/0022-3514.73.3.437
![]() |
[83] | Tran QT, Mai YD (2015) Stock Market Reaction to Dividend Announcements from a Special Institutional Environment of Vietnamese Stock Market. Int J Econ Finance 7: 50-58. |
[84] |
Tversky A, Kahneman D (1973) Availability: A Heuristic for Judging Frequency and Probability. Cognit Psychol 4: 207–232. doi: 10.1016/0010-0285(73)90012-1
![]() |
[85] |
Tversky A, Kahneman D (1974) Judgment under Uncertainty: Heuristics and Biases. Sci 185: 1124-1131. doi: 10.1126/science.185.4157.1124
![]() |
[86] | Varian HR (1989) Differences of Opinion in Financial Markets. In Financial Risk: Theory, Evidence and Implications: Proceedings of the 11th Annual Economic Policy Conference of the Federal Reserve Bank of St. Louis, 3–37. |
[87] |
Verrecchia RE (1981) On the Relationship between Volume Reaction and Consensus of Investors: Implications for Interpreting Tests of Information Content. J Account Res 19: 271-283. doi: 10.2307/2490975
![]() |
[88] |
Westerfield R (1977) The Distribution of Common Stock Price Changes: An Application of Transactions Time and Subordinated Stochastic Models. J Financ Quant Anal 12: 743-765. doi: 10.2307/2330254
![]() |
[89] |
Wilson TD, Houston C, Etling KM, Brekke N (1996) A New Look at Anchoring Effects: Basic Anchoring and its Antecedents. J Exp Psychol: Gen 125: 387–402. doi: 10.1037/0096-3445.125.4.387
![]() |
[90] |
Wood RA, McInish TH, Ord KJ (1985) An Investigation of Transactions Data for NYSE Stocks. J Finance 40: 723-739. doi: 10.1111/j.1540-6261.1985.tb04996.x
![]() |
[91] |
Xu P, Rui OM, Kim S (2002) Risk Shift Following Dividend Change Announcement: The Role of Trading. Rev Quant Finance Account 19: 45-63. doi: 10.1023/A:1015778208868
![]() |
[92] |
Ying CC (1966) Stock Market Prices and Volumes of Sales. Econometrica 34: 676-686. doi: 10.2307/1909776
![]() |
[93] | Ziebart DA (1990) The Association between Consensus of Beliefs and Trading Activity Surrounding Earnings Announcements. Account Rev 65: 477-488. |
[94] | Zielonka P (2004) Technical Analysis as the Representation of Typical Cognitive Biases. Int Rev Financ Anal 13: 217-225. |
1. | Amlan Jyoti Kalita, Abhijit Boruah, Tapan Das, Nirmal Mazumder, Shyam K. Jaiswal, Guan-Yu Zhuo, Ankur Gogoi, Nayan M. Kakoty, Fu-Jen Kao, 2024, Chapter 1, 978-981-97-5344-4, 1, 10.1007/978-981-97-5345-1_1 | |
2. | Kyler Howard, Chris Rocheleau, Trevor Overton, Joel Barraza Nava, Mason Faldet, Kristina Moen, Summer Soller, Tyler Stephens, Esther van de Lagemaat, Natalie Wijesinghe, Kaylee Wong Dolloff, Nilton Barbosa da Rosa, Jennifer L. Mueller, A comparison of techniques to improve pulmonary EIT image resolution using a database of simulated EIT images, 2025, 460, 03770427, 116415, 10.1016/j.cam.2024.116415 | |
3. | Grzegorz Kłosowski, Monika Kulisz, Tomasz Rymarczyk, Łukasz Skowron, Paweł Olszewski, Konrad Niderla, Application of machine learning in electrical process tomography with variable frequency measurement sequences, 2025, 247, 02632241, 116770, 10.1016/j.measurement.2025.116770 |
Net block | Layer | Activation function | ||
Dense Block 1 | − | − | Dense Block type3 (k = 8) |
ReLU |
Dense Block 2 | Dense Block type1 (k = 8) |
Dense Block type2 (k = 8) |
Dense Block type3 (k = 32) |
ReLU |
Dense Block 3 | Dense Block type1 (k = 16) |
Dense Block type2 (k = 16) |
Dense Block type3 (k = 96) |
ReLU |
Final output | Fully Connect | / | / | Sigmoid |
Phantom | Method | RMSE | SSIM | MAE | ICC |
Circle Phantom | GN | 0.1905 | 0.7856 | 0.1193 | 0.8160 |
DenseNet | 0.0650 | 0.9702 | 0.0256 | 0.9711 | |
MS-DenseNet | 0.0464 | 0.9846 | 0.0194 | 0.9851 | |
Lung Phantom | GN | 0.0704 | 0.7017 | 0.0578 | 0.7115 |
DenseNet | 0.0123 | 0.9899 | 0.0074 | 0.9900 | |
MS-DenseNet | 0.0100 | 0.9937 | 0.0061 | 0.9931 |
Metric | Method | Case 1 | Case 2 | Case 3 | Case 4 | Case 5 | Case 6 | Case 7 | Case 8 |
RMSE | GN | 0.2246 | 0.2322 | 0.2073 | 0.2960 | 0.1281 | 0.2833 | 0.2856 | 0.2689 |
DenseNet | 0.0903 | 0.0111 | 0.0904 | 0.0718 | 0.0652 | 0.1700 | 0.2496 | 0.2274 | |
MS-DenseNet | 0.0538 | 0.0055 | 0.0473 | 0.0391 | 0.0322 | 0.1216 | 0.0745 | 0.1510 | |
SSIM | GN | 0.7797 | 0.8224 | 0.7721 | 0.7161 | 0.7932 | 0.7720 | 0.6923 | 0.7190 |
DenseNet | 0.9722 | 0.9997 | 0.9649 | 0.9871 | 0.9531 | 0.9185 | 0.8208 | 0.8351 | |
MS-DenseNet | 0.9906 | 0.9999 | 0.9907 | 0.9963 | 0.9891 | 0.9585 | 0.9840 | 0.9330 | |
MAE | GN | 0.1470 | 0.1606 | 0.1147 | 0.1804 | 0.0764 | 0.1797 | 0.1774 | 0.1637 |
DenseNet | 0.0343 | 0.0069 | 0.0351 | 0.0305 | 0.0209 | 0.0430 | 0.0688 | 0.1183 | |
MS-DenseNet | 0.0227 | 0.0032 | 0.0173 | 0.0153 | 0.0114 | 0.0319 | 0.0322 | 0.0543 | |
ICC | GN | 0.8080 | 0.8429 | 0.8146 | 0.7671 | 0.8147 | 0.7773 | 0.7335 | 0.7927 |
DenseNet | 0.9723 | 0.9997 | 0.9669 | 0.9882 | 0.9552 | 0.9201 | 0.8206 | 0.8451 | |
MS-DenseNet | 0.9926 | 0.9999 | 0.9910 | 0.9966 | 0.9892 | 0.9596 | 0.9841 | 0.9347 |
Metric | Method | Case 1 | Case 2 | Case 3 | Case 4 | Case 5 | Case 6 | Case 7 | Case 8 |
RMSE | GN | 0.0471 | 0.0838 | 0.0629 | 0.0427 | 0.0543 | 0.0557 | 0.0504 | 0.0540 |
DenseNet | 0.0194 | 0.0073 | 0.0203 | 0.0170 | 0.0178 | 0.0122 | 0.0143 | 0.0172 | |
MS-DenseNet | 0.0147 | 0.0042 | 0.0156 | 0.0121 | 0.0113 | 0.0082 | 0.0106 | 0.0138 | |
SSIM | GN | 0.6785 | 0.6888 | 0.6371 | 0.7696 | 0.7331 | 0.7386 | 0.7761 | 0.7234 |
DenseNet | 0.9511 | 0.9980 | 0.9684 | 0.9681 | 0.9747 | 0.9891 | 0.9846 | 0.9750 | |
MS-DenseNet | 0.9720 | 0.9994 | 0.9809 | 0.9829 | 0.9897 | 0.9952 | 0.9917 | 0.9844 | |
MAE | GN | 0.0386 | 0.0691 | 0.0509 | 0.0340 | 0.0443 | 0.0459 | 0.0417 | 0.0445 |
DenseNet | 0.0111 | 0.0045 | 0.0126 | 0.0112 | 0.0099 | 0.0050 | 0.0096 | 0.0094 | |
MS-DenseNet | 0.0089 | 0.0034 | 0.0090 | 0.0069 | 0.0066 | 0.0044 | 0.0071 | 0.0079 | |
ICC | GN | 0.6949 | 0.7099 | 0.6564 | 0.7782 | 0.7466 | 0.7552 | 0.7901 | 0.7376 |
DenseNet | 0.9521 | 0.9980 | 0.9684 | 0.9686 | 0.9747 | 0.9892 | 0.9850 | 0.9753 | |
MS-DenseNet | 0.9725 | 0.9995 | 0.9809 | 0.9831 | 0.9897 | 0.9952 | 0.9918 | 0.9844 |
Phantom | Method | RMSE | SSIM | MAE | ICC |
Circle Phantom | GN | 0.1905 | 0.7856 | 0.1193 | 0.8160 |
DenseNet | 0.0903 | 0.9481 | 0.0387 | 0.9497 | |
MS-DenseNet | 0.0770 | 0.9630 | 0.0324 | 0.9640 | |
Lung Phantom | GN | 0.0704 | 0.7017 | 0.0578 | 0.7115 |
DenseNet | 0.0189 | 0.9774 | 0.0137 | 0.9809 | |
MS-DenseNet | 0.0140 | 0.9880 | 0.0097 | 0.9881 |
Metric | Method | Case 1 | Case 2 | Case 3 | Case 4 | Case 5 | Case 6 | Case 7 | Case 8 |
RMSE | GN | 0.2246 | 0.2322 | 0.2073 | 0.2960 | 0.1281 | 0.2833 | 0.2856 | 0.2689 |
DenseNet | 0.0938 | 0.0140 | 0.0884 | 0.0785 | 0.0733 | 0.1716 | 0.2560 | 0.2269 | |
MS-DenseNet | 0.0511 | 0.0083 | 0.0582 | 0.0342 | 0.0549 | 0.1195 | 0.0873 | 0.1468 | |
SSIM | GN | 0.7797 | 0.8224 | 0.7721 | 0.7161 | 0.7932 | 0.7720 | 0.6923 | 0.7190 |
DenseNet | 0.9709 | 0.9995 | 0.9664 | 0.9847 | 0.9416 | 0.9168 | 0.8133 | 0.8366 | |
MS-DenseNet | 0.9914 | 0.9998 | 0.9859 | 0.9972 | 0.9673 | 0.9598 | 0.9777 | 0.9370 | |
MAE | GN | 0.1470 | 0.1606 | 0.1147 | 0.1804 | 0.0764 | 0.1797 | 0.1774 | 0.1637 |
DenseNet | 0.0387 | 0.0090 | 0.0315 | 0.0311 | 0.0207 | 0.0443 | 0.0699 | 0.1201 | |
MS-DenseNet | 0.0226 | 0.0050 | 0.0195 | 0.0146 | 0.0153 | 0.0320 | 0.0349 | 0.0519 | |
ICC | GN | 0.8080 | 0.8429 | 0.8146 | 0.7671 | 0.8147 | 0.7773 | 0.7335 | 0.7927 |
DenseNet | 0.9718 | 0.9995 | 0.9686 | 0.9856 | 0.9428 | 0.9184 | 0.8132 | 0.8457 | |
MS-DenseNet | 0.9929 | 0.9998 | 0.9863 | 0.9976 | 0.9686 | 0.9609 | 0.9780 | 0.9384 |
Metric | Method | Case 1 | Case 2 | Case 3 | Case 4 | Case 5 | Case 6 | Case 7 | Case 8 |
RMSE | GN | 0.0471 | 0.0838 | 0.0629 | 0.0427 | 0.0543 | 0.0557 | 0.0504 | 0.0540 |
DenseNet | 0.0198 | 0.0066 | 0.0190 | 0.0164 | 0.0172 | 0.0127 | 0.0165 | 0.0174 | |
MS-DenseNet | 0.0159 | 0.0057 | 0.0161 | 0.0128 | 0.0117 | 0.0089 | 0.0119 | 0.0144 | |
SSIM | GN | 0.6785 | 0.6888 | 0.6371 | 0.7696 | 0.7331 | 0.7386 | 0.7761 | 0.7234 |
DenseNet | 0.9485 | 0.9983 | 0.9714 | 0.9696 | 0.9758 | 0.9881 | 0.9802 | 0.9747 | |
MS-DenseNet | 0.9684 | 0.9990 | 0.9797 | 0.9809 | 0.9890 | 0.9942 | 0.9898 | 0.9830 | |
MAE | GN | 0.0386 | 0.0691 | 0.0509 | 0.0340 | 0.0443 | 0.0459 | 0.0417 | 0.0445 |
DenseNet | 0.0112 | 0.0045 | 0.0108 | 0.0103 | 0.0097 | 0.0062 | 0.0122 | 0.0097 | |
MS-DenseNet | 0.0101 | 0.0047 | 0.0094 | 0.0083 | 0.0072 | 0.0054 | 0.0082 | 0.0090 | |
ICC | GN | 0.6949 | 0.7099 | 0.6564 | 0.7782 | 0.7466 | 0.7552 | 0.7901 | 0.7376 |
DenseNet | 0.9500 | 0.9984 | 0.9715 | 0.9697 | 0.9761 | 0.9881 | 0.9819 | 0.9747 | |
MS-DenseNet | 0.9684 | 0.9991 | 0.9797 | 0.9815 | 0.9892 | 0.9942 | 0.9900 | 0.9830 |
Metric | Method | Case 1 | Case 2 | Case 3 | Case 4 | Case 5 |
RMSE | GN | 0.2026 | 0.2664 | 0.3332 | 0.3280 | 0.1918 |
DenseNet | 0.1746 | 0.2009 | 0.3255 | 0.3871 | 0.0044 | |
MS-DenseNet | 0.1530 | 0.0792 | 0.1187 | 0.1572 | 0.0036 | |
SSIM | GN | 0.8101 | 0.7708 | 0.6969 | 0.7128 | 0.4041 |
DenseNet | 0.8924 | 0.8857 | 0.7560 | 0.6626 | 0.9993 | |
MS-DenseNet | 0.9161 | 0.9834 | 0.9704 | 0.9487 | 0.9997 | |
MAE | GN | 0.1310 | 0.1748 | 0.2233 | 0.2258 | 0.1229 |
DenseNet | 0.0689 | 0.1068 | 0.1911 | 0.2185 | 0.0040 | |
MS-DenseNet | 0.0554 | 0.0476 | 0.0668 | 0.0840 | 0.0032 | |
ICC | GN | 0.8532 | 0.8157 | 0.7741 | 0.7817 | 0.5395 |
DenseNet | 0.8923 | 0.8993 | 0.7762 | 0.6807 | 0.9995 | |
MS-DenseNet | 0.9162 | 0.9871 | 0.9743 | 0.9529 | 0.9998 |
Dense block | MSE |
Conventional Dense Block | 2.657 |
Dense block type1 | 1.737 |
Dense block type2 | 1.722 |
Dense block type3 | 1.633 |
Net type | Max pooling | Average pooling | 2 × 2 convolution | MSE |
Type 1 | 100% | 0% | 0% | 3.028 |
Type 2 | 50% | 50% | 0% | 2.874 |
Type 3 | 25% | 75% | 0% | 2.706 |
Type 4 | 0% | 100% | 0% | 2.554 |
Type 5 | 0% | 50% | 50% | 1.866 |
Type 6 | 0% | 25% | 75% | 1.721 |
Type 7 | 0% | 0% | 100% | 1.634 |
Methods\Metric | Year | RMSE | SSIM | ICC |
PLS [44] | 2018 | − | 0.95 | 0.93 |
CNN [26] | 2019 | − | − | 0.9472 |
ANHTV [45] | 2021 | 0.1818 | − | 0.91 |
BLS [46] | 2022 | 0.16 | − | 0.93 |
M-STGNN[47] | 2022 | 0.0378 | 0.9693 | − |
CWGAN-AM [48] | 2022 | − | 0.9836 | − |
MS-DenseNet | 2022 | 0.0464 | 0.9846 | 0.9851 |
Net block | Layer | Activation function | ||
Dense Block 1 | − | − | Dense Block type3 (k = 8) |
ReLU |
Dense Block 2 | Dense Block type1 (k = 8) |
Dense Block type2 (k = 8) |
Dense Block type3 (k = 32) |
ReLU |
Dense Block 3 | Dense Block type1 (k = 16) |
Dense Block type2 (k = 16) |
Dense Block type3 (k = 96) |
ReLU |
Final output | Fully Connect | / | / | Sigmoid |
Phantom | Method | RMSE | SSIM | MAE | ICC |
Circle Phantom | GN | 0.1905 | 0.7856 | 0.1193 | 0.8160 |
DenseNet | 0.0650 | 0.9702 | 0.0256 | 0.9711 | |
MS-DenseNet | 0.0464 | 0.9846 | 0.0194 | 0.9851 | |
Lung Phantom | GN | 0.0704 | 0.7017 | 0.0578 | 0.7115 |
DenseNet | 0.0123 | 0.9899 | 0.0074 | 0.9900 | |
MS-DenseNet | 0.0100 | 0.9937 | 0.0061 | 0.9931 |
Metric | Method | Case 1 | Case 2 | Case 3 | Case 4 | Case 5 | Case 6 | Case 7 | Case 8 |
RMSE | GN | 0.2246 | 0.2322 | 0.2073 | 0.2960 | 0.1281 | 0.2833 | 0.2856 | 0.2689 |
DenseNet | 0.0903 | 0.0111 | 0.0904 | 0.0718 | 0.0652 | 0.1700 | 0.2496 | 0.2274 | |
MS-DenseNet | 0.0538 | 0.0055 | 0.0473 | 0.0391 | 0.0322 | 0.1216 | 0.0745 | 0.1510 | |
SSIM | GN | 0.7797 | 0.8224 | 0.7721 | 0.7161 | 0.7932 | 0.7720 | 0.6923 | 0.7190 |
DenseNet | 0.9722 | 0.9997 | 0.9649 | 0.9871 | 0.9531 | 0.9185 | 0.8208 | 0.8351 | |
MS-DenseNet | 0.9906 | 0.9999 | 0.9907 | 0.9963 | 0.9891 | 0.9585 | 0.9840 | 0.9330 | |
MAE | GN | 0.1470 | 0.1606 | 0.1147 | 0.1804 | 0.0764 | 0.1797 | 0.1774 | 0.1637 |
DenseNet | 0.0343 | 0.0069 | 0.0351 | 0.0305 | 0.0209 | 0.0430 | 0.0688 | 0.1183 | |
MS-DenseNet | 0.0227 | 0.0032 | 0.0173 | 0.0153 | 0.0114 | 0.0319 | 0.0322 | 0.0543 | |
ICC | GN | 0.8080 | 0.8429 | 0.8146 | 0.7671 | 0.8147 | 0.7773 | 0.7335 | 0.7927 |
DenseNet | 0.9723 | 0.9997 | 0.9669 | 0.9882 | 0.9552 | 0.9201 | 0.8206 | 0.8451 | |
MS-DenseNet | 0.9926 | 0.9999 | 0.9910 | 0.9966 | 0.9892 | 0.9596 | 0.9841 | 0.9347 |
Metric | Method | Case 1 | Case 2 | Case 3 | Case 4 | Case 5 | Case 6 | Case 7 | Case 8 |
RMSE | GN | 0.0471 | 0.0838 | 0.0629 | 0.0427 | 0.0543 | 0.0557 | 0.0504 | 0.0540 |
DenseNet | 0.0194 | 0.0073 | 0.0203 | 0.0170 | 0.0178 | 0.0122 | 0.0143 | 0.0172 | |
MS-DenseNet | 0.0147 | 0.0042 | 0.0156 | 0.0121 | 0.0113 | 0.0082 | 0.0106 | 0.0138 | |
SSIM | GN | 0.6785 | 0.6888 | 0.6371 | 0.7696 | 0.7331 | 0.7386 | 0.7761 | 0.7234 |
DenseNet | 0.9511 | 0.9980 | 0.9684 | 0.9681 | 0.9747 | 0.9891 | 0.9846 | 0.9750 | |
MS-DenseNet | 0.9720 | 0.9994 | 0.9809 | 0.9829 | 0.9897 | 0.9952 | 0.9917 | 0.9844 | |
MAE | GN | 0.0386 | 0.0691 | 0.0509 | 0.0340 | 0.0443 | 0.0459 | 0.0417 | 0.0445 |
DenseNet | 0.0111 | 0.0045 | 0.0126 | 0.0112 | 0.0099 | 0.0050 | 0.0096 | 0.0094 | |
MS-DenseNet | 0.0089 | 0.0034 | 0.0090 | 0.0069 | 0.0066 | 0.0044 | 0.0071 | 0.0079 | |
ICC | GN | 0.6949 | 0.7099 | 0.6564 | 0.7782 | 0.7466 | 0.7552 | 0.7901 | 0.7376 |
DenseNet | 0.9521 | 0.9980 | 0.9684 | 0.9686 | 0.9747 | 0.9892 | 0.9850 | 0.9753 | |
MS-DenseNet | 0.9725 | 0.9995 | 0.9809 | 0.9831 | 0.9897 | 0.9952 | 0.9918 | 0.9844 |
Phantom | Method | RMSE | SSIM | MAE | ICC |
Circle Phantom | GN | 0.1905 | 0.7856 | 0.1193 | 0.8160 |
DenseNet | 0.0903 | 0.9481 | 0.0387 | 0.9497 | |
MS-DenseNet | 0.0770 | 0.9630 | 0.0324 | 0.9640 | |
Lung Phantom | GN | 0.0704 | 0.7017 | 0.0578 | 0.7115 |
DenseNet | 0.0189 | 0.9774 | 0.0137 | 0.9809 | |
MS-DenseNet | 0.0140 | 0.9880 | 0.0097 | 0.9881 |
Metric | Method | Case 1 | Case 2 | Case 3 | Case 4 | Case 5 | Case 6 | Case 7 | Case 8 |
RMSE | GN | 0.2246 | 0.2322 | 0.2073 | 0.2960 | 0.1281 | 0.2833 | 0.2856 | 0.2689 |
DenseNet | 0.0938 | 0.0140 | 0.0884 | 0.0785 | 0.0733 | 0.1716 | 0.2560 | 0.2269 | |
MS-DenseNet | 0.0511 | 0.0083 | 0.0582 | 0.0342 | 0.0549 | 0.1195 | 0.0873 | 0.1468 | |
SSIM | GN | 0.7797 | 0.8224 | 0.7721 | 0.7161 | 0.7932 | 0.7720 | 0.6923 | 0.7190 |
DenseNet | 0.9709 | 0.9995 | 0.9664 | 0.9847 | 0.9416 | 0.9168 | 0.8133 | 0.8366 | |
MS-DenseNet | 0.9914 | 0.9998 | 0.9859 | 0.9972 | 0.9673 | 0.9598 | 0.9777 | 0.9370 | |
MAE | GN | 0.1470 | 0.1606 | 0.1147 | 0.1804 | 0.0764 | 0.1797 | 0.1774 | 0.1637 |
DenseNet | 0.0387 | 0.0090 | 0.0315 | 0.0311 | 0.0207 | 0.0443 | 0.0699 | 0.1201 | |
MS-DenseNet | 0.0226 | 0.0050 | 0.0195 | 0.0146 | 0.0153 | 0.0320 | 0.0349 | 0.0519 | |
ICC | GN | 0.8080 | 0.8429 | 0.8146 | 0.7671 | 0.8147 | 0.7773 | 0.7335 | 0.7927 |
DenseNet | 0.9718 | 0.9995 | 0.9686 | 0.9856 | 0.9428 | 0.9184 | 0.8132 | 0.8457 | |
MS-DenseNet | 0.9929 | 0.9998 | 0.9863 | 0.9976 | 0.9686 | 0.9609 | 0.9780 | 0.9384 |
Metric | Method | Case 1 | Case 2 | Case 3 | Case 4 | Case 5 | Case 6 | Case 7 | Case 8 |
RMSE | GN | 0.0471 | 0.0838 | 0.0629 | 0.0427 | 0.0543 | 0.0557 | 0.0504 | 0.0540 |
DenseNet | 0.0198 | 0.0066 | 0.0190 | 0.0164 | 0.0172 | 0.0127 | 0.0165 | 0.0174 | |
MS-DenseNet | 0.0159 | 0.0057 | 0.0161 | 0.0128 | 0.0117 | 0.0089 | 0.0119 | 0.0144 | |
SSIM | GN | 0.6785 | 0.6888 | 0.6371 | 0.7696 | 0.7331 | 0.7386 | 0.7761 | 0.7234 |
DenseNet | 0.9485 | 0.9983 | 0.9714 | 0.9696 | 0.9758 | 0.9881 | 0.9802 | 0.9747 | |
MS-DenseNet | 0.9684 | 0.9990 | 0.9797 | 0.9809 | 0.9890 | 0.9942 | 0.9898 | 0.9830 | |
MAE | GN | 0.0386 | 0.0691 | 0.0509 | 0.0340 | 0.0443 | 0.0459 | 0.0417 | 0.0445 |
DenseNet | 0.0112 | 0.0045 | 0.0108 | 0.0103 | 0.0097 | 0.0062 | 0.0122 | 0.0097 | |
MS-DenseNet | 0.0101 | 0.0047 | 0.0094 | 0.0083 | 0.0072 | 0.0054 | 0.0082 | 0.0090 | |
ICC | GN | 0.6949 | 0.7099 | 0.6564 | 0.7782 | 0.7466 | 0.7552 | 0.7901 | 0.7376 |
DenseNet | 0.9500 | 0.9984 | 0.9715 | 0.9697 | 0.9761 | 0.9881 | 0.9819 | 0.9747 | |
MS-DenseNet | 0.9684 | 0.9991 | 0.9797 | 0.9815 | 0.9892 | 0.9942 | 0.9900 | 0.9830 |
Metric | Method | Case 1 | Case 2 | Case 3 | Case 4 | Case 5 |
RMSE | GN | 0.2026 | 0.2664 | 0.3332 | 0.3280 | 0.1918 |
DenseNet | 0.1746 | 0.2009 | 0.3255 | 0.3871 | 0.0044 | |
MS-DenseNet | 0.1530 | 0.0792 | 0.1187 | 0.1572 | 0.0036 | |
SSIM | GN | 0.8101 | 0.7708 | 0.6969 | 0.7128 | 0.4041 |
DenseNet | 0.8924 | 0.8857 | 0.7560 | 0.6626 | 0.9993 | |
MS-DenseNet | 0.9161 | 0.9834 | 0.9704 | 0.9487 | 0.9997 | |
MAE | GN | 0.1310 | 0.1748 | 0.2233 | 0.2258 | 0.1229 |
DenseNet | 0.0689 | 0.1068 | 0.1911 | 0.2185 | 0.0040 | |
MS-DenseNet | 0.0554 | 0.0476 | 0.0668 | 0.0840 | 0.0032 | |
ICC | GN | 0.8532 | 0.8157 | 0.7741 | 0.7817 | 0.5395 |
DenseNet | 0.8923 | 0.8993 | 0.7762 | 0.6807 | 0.9995 | |
MS-DenseNet | 0.9162 | 0.9871 | 0.9743 | 0.9529 | 0.9998 |
Dense block | MSE |
Conventional Dense Block | 2.657 |
Dense block type1 | 1.737 |
Dense block type2 | 1.722 |
Dense block type3 | 1.633 |
Net type | Max pooling | Average pooling | 2 × 2 convolution | MSE |
Type 1 | 100% | 0% | 0% | 3.028 |
Type 2 | 50% | 50% | 0% | 2.874 |
Type 3 | 25% | 75% | 0% | 2.706 |
Type 4 | 0% | 100% | 0% | 2.554 |
Type 5 | 0% | 50% | 50% | 1.866 |
Type 6 | 0% | 25% | 75% | 1.721 |
Type 7 | 0% | 0% | 100% | 1.634 |
Methods\Metric | Year | RMSE | SSIM | ICC |
PLS [44] | 2018 | − | 0.95 | 0.93 |
CNN [26] | 2019 | − | − | 0.9472 |
ANHTV [45] | 2021 | 0.1818 | − | 0.91 |
BLS [46] | 2022 | 0.16 | − | 0.93 |
M-STGNN[47] | 2022 | 0.0378 | 0.9693 | − |
CWGAN-AM [48] | 2022 | − | 0.9836 | − |
MS-DenseNet | 2022 | 0.0464 | 0.9846 | 0.9851 |