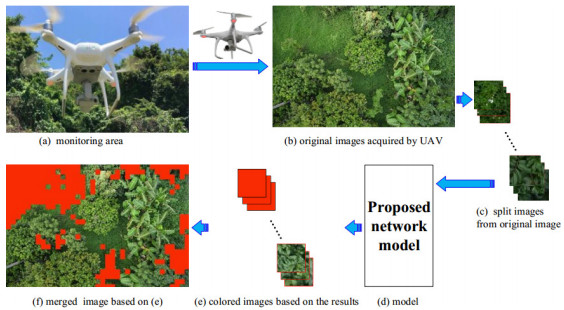
Citation: Douglas Wilson, Griffiths Keith, Buttar Harpal, Singh Ram, De Meester Fabien, Wilczynska Agnieszka, Takahashi Toru. Therapy through Social Medicine: Cultivating Connections and Inspiring Solutions for Healthy Living[J]. AIMS Medical Science, 2017, 4(2): 131-150. doi: 10.3934/medsci.2017.2.131
[1] |
Feng Chen, Xuqing Zhu, Jing Zheng, Tingting Xu, Kuan Wu, Chuhui Ru .
RHAMM regulates the growth and migration of lung adenocarcinoma A549 cell line by regulating Cdc2/CyclinB1 and MMP9 genes . Mathematical Biosciences and Engineering, 2020, 17(3): 2150-2163. doi: 10.3934/mbe.2020114 |
[2] | Yunfeng Liu, Guowei Sun, Lin Wang, Zhiming Guo . Establishing Wolbachia in the wild mosquito population: The effects of wind and critical patch size. Mathematical Biosciences and Engineering, 2019, 16(5): 4399-4414. doi: 10.3934/mbe.2019219 |
[3] | Yanmei Jiang, Mingsheng Liu, Jianhua Li, Jingyi Zhang . Reinforced MCTS for non-intrusive online load identification based on cognitive green computing in smart grid. Mathematical Biosciences and Engineering, 2022, 19(11): 11595-11627. doi: 10.3934/mbe.2022540 |
[4] | Diego Vicencio, Olga Vasilieva, Pedro Gajardo . Monotonicity properties arising in a simple model of Wolbachia invasion for wild mosquito populations. Mathematical Biosciences and Engineering, 2023, 20(1): 1148-1175. doi: 10.3934/mbe.2023053 |
[5] | Shikang Zheng, Kai Chen, Xinping Lin, Shiqian Liu, Jie Han, Guomin Wu . Quantitative analysis of facial proportions and facial attractiveness among Asians and Caucasians. Mathematical Biosciences and Engineering, 2022, 19(6): 6379-6395. doi: 10.3934/mbe.2022299 |
[6] | Martin Strugarek, Nicolas Vauchelet, Jorge P. Zubelli . Quantifying the survival uncertainty of Wolbachia-infected mosquitoes in a spatial model. Mathematical Biosciences and Engineering, 2018, 15(4): 961-991. doi: 10.3934/mbe.2018043 |
[7] | Yanfeng Shi, Shuo Qiu, Jiqiang Liu, Tinghuai Ma . Novel efficient lattice-based IBE schemes with CPK for fog computing. Mathematical Biosciences and Engineering, 2020, 17(6): 8105-8122. doi: 10.3934/mbe.2020411 |
[8] | Qian Shao, Rongrong Xuan, Yutao Wang, Jian Xu, Menglin Ouyang, Caoqian Yin, Wei Jin . Deep learning and radiomics analysis for prediction of placenta invasion based on T2WI. Mathematical Biosciences and Engineering, 2021, 18(5): 6198-6215. doi: 10.3934/mbe.2021310 |
[9] | Hui Li, Xintang Liu, Dongbao Jia, Yanyan Chen, Pengfei Hou, Haining Li . Research on chest radiography recognition model based on deep learning. Mathematical Biosciences and Engineering, 2022, 19(11): 11768-11781. doi: 10.3934/mbe.2022548 |
[10] | Yu Li, Meilong Zhu, Guangmin Sun, Jiayang Chen, Xiaorong Zhu, Jinkui Yang . Weakly supervised training for eye fundus lesion segmentation in patients with diabetic retinopathy. Mathematical Biosciences and Engineering, 2022, 19(5): 5293-5311. doi: 10.3934/mbe.2022248 |
As a perennial vine, Ipomoea cairica (L.) sweets are viewed as the noxious invasive weeds in China and are called as plant killer because of heavily covering other plants in wild lands [1]. Ipomoea cairica (L.) sweets like climbing, can rapiddly grow, propagate, and cover in its area. Consequently, Ipomoea cairica (L.) sweets can result in the serious harm to the biodiversity and stability of the ecosystem [2]. How to identify and monitor the Ipomoea cairica (L.) sweets in the wild is becoming very important since it can help the governments to take the necessary strategies and methods in the identified area to prevent and control the growth of the Ipomoea cairica (L.) sweets for biodiversity and stability of the ecosystem.
Currently, identifying and monitoring Ipomoea cairica (L.) sweets mainly depends on manual approaches of low efficiency and high cost [3]. With the quick development of image processing and artificial intelligence (AI), it is possible to help managements to make the precise efficient faster measures by efficiently identifying and monitoring Ipomoea cairica (L.) sweets [4]. Hyperspectral images have been used to identify the invasive species for monitoring and control their rapidly growth. Liu, et al. used the high-resolution imagery from Google Earth to monitor the Spartina alterniflora and showed that this approach can help governments to control Spartina alterniflora invasion [5], West et al. tested five models to identify Tamarik in satellite remote sensing data [6]. Bustamante et al. used hyperspectral sensors to detect Spartina densiflora Brongn [7]. Jomar et al. used imaging spectroscopy to detect Psidium cattleianum in Hawaiian Forests [8]. Khare et al. assess invasive plant species diversity in Pléiades 1A, RapidEye and Landsat-8 data [9]. However, it is difficult for these methods to detect the Ipomoea cairica (L.) sweets since the high-resolution hyperspectral images collected by aircraft and satellite are very high cost. For free low-resolution spectral data, some key information in these images is lost. As a result, the hyperspectral images are not suitable to monitor Ipomoea cairica (L.) sweets in the early stages.
The number of study has proved that the high-resolution digital images can be used to plants identification in various environments and shows their excellent results [10], especially the high-resolution images collected by low-altitude UAV because of retained good characteristics. Ge et al. used the aerial photographs to determine the Tamarix parviflora [11]. Jones et al. used the image analysis-based approach to detect Japanese Knotweed s.l. taxa in high-resolution, infra-red aerial images [12]. Dorigo et al. proposed an identifying method based on remote sensing to find F. japonica from low-cost images [13]. Sandino et al. used unmanned aerial vehicles (UAVs) and machine learning to detect the buffel grass and spinifex [14]. Hung et al. used feature learning to classify the invasive weed species based on the images from UAVs [15]. However, the detecting accuracy need be further improved, the efficient method with high accuracy need be developed for identifying invasive weed in the wild.
Now deep learning shows the excellent ability in image recognition fields and is widely applied to the object detection in agricultural. Pound et al. demonstrated deep approaches can offer excellent ability to identify root and shoot feature [16]. Alvaro et al. presented deep learning method to identify the tomato diseases [17]. Amanda et al. presented the transfer learning and deep CNN for pest damage and diseases detection, their results showed that their method can offer a fast, affordable method for digital disease detection [18]. Lu et al. used the deep CNN to identify the counts of maize tassels [19]. Though deep learning has widely applied to the agriculture fields, it still is difficult for identifying Ipomoea cairica (L.) sweets since it is very similar between Ipomoea cairica (L.) sweets and phorophyte.
To better address aforementioned challenges, we present a novel identifying and monitoring framework and method for Ipomoea cairica (L.) sweets based on unmanned aerial vehicle (UAV) and artificial intelligence (AI). In the proposed framework, we can low-costly collected the images with 8256 × 5504 pixels of the monitoring area by the UAV, and the collected images are split into more small sub-images with 224 × 224 pixels for identifying model. For identifying Ipomoea cairica (L.) sweets, we propose a novel deep CNN (ICSNet) which consists of 12 layers. Finally, the Ipomoea cairica (L.) sweets can be monitored by painting the area containing Ipomoea cairica (L.) sweets. Our experiment results show our method can automatically monitoring and eradicating Ipomoea cairica (L.) sweets in wilds.
In summary, the main contributions of this work are as follows:
1) A novel identifying and monitoring framework and method based on unmanned aerial vehicle (UAV) and artificial intelligence (AI) are proposed for Ipomoea cairica (L.) sweets
2) A deep CNN (ICSNet) is proposed to identifying Ipomoea cairica (L.) sweets, which can achieve the tradeoff between accuracy and time.
3) The framework and method are validated in our experiments and the experimental results show our method has the excellent performance.
The remainder is as follows. Section Ⅱ presents our methods. Section Ⅲ describes the experimental results and discussions. The conclusions are in Section Ⅳ.
For monitoring the Ipomoea cairica (L.) sweets, we propose a novel detecting framework for Ipomoea cairica (L.) sweets, which is shown in Figure 1. In our framework, monitoring the Ipomoea cairica (L.) sweets includes the following steps:
First step: The UAV is used to collect the images with 8256 × 5504 pixels of monitoring area, the whole images of monitoring area consists of sub-images with 8256 × 5504 pixels. The image sample is shown in Figure 1(b).
Second step: The collected images with 8256 × 5504 pixels are split into many more small images with 224 × 224 pixels. The samples are shown in Figure 1(c).
Third step: For each image with 224 × 224 pixels, we use the proposed deep CNN model to identify if the Ipomoea cairica (L.) sweets are contained in the images. This is corresponding to Figure 1(d).
Four step: Based on above identified results, we paint the images containing Ipomoea cairica (L.) sweets in red color. This is corresponding to Figure 1(e).
Five step: The painted images with 224 × 224 pixels is reassembled, as a result, the areas containing Ipomoea cairica (L.) sweets are covered by red color in the images with 8256 × 5504 pixels. This is corresponding to Figure 1(f). Finally, all the Ipomoea cairica (L.) sweets are identified in monitoring area.
For our framework and algorithm, in fact we can review it as a special case of object detection algorithm. For R-CNN and Fast R-CNN, they usually include three main steps: determining candidate region, feature extraction and classification. But slowly detecting speed is the lack of these algorithms. For our algorithm, we improve detection speed by in advance splitting the image into the little sub-image with fixed same size. Consequently, we can achieve the required monitoring accuracy by adjusting the size of sub-image, and detection time also can meet the actual requirements. Although, the object detection algorithms such as Yolo and SSD, which achieves the classification and positioning in one step, can improve the detection speed, their detection accuracy is lower than R-CNN and Fast R-CNN.
The multilayer neural network only consists of input, output and hidden layers. The actual needs decide the number of the hidden layers. Based on the original multilayer neural network, a more effective feature learning part is added in network. The part is the convolution layer and pooling layer which is set in front of fully connected layer. This is convolution neural network (CNN) which is shown in Figure 2. CNN deepen the number of layers in neural network and deep learning can be realized.
Here, we build a 12-layer deep CNN network model for identifying Ipomoea cairica (L.) sweets, which mainly consists of convolutional layer, pool layer, inception module, dropout layer, full connected layer. The input is the RGB image with the size of 224 × 224 × 3. The output consists of two neurons. The proposed network model is named ICSNet for short. The architecture of ICSNet is illustrated in Figure 3.
First layer: It is a convolution layer, which is with 64 3 × 3 filters and the stride is 2. The ReLU (rectified linear units) are used as the activation function for improving the convergence speed and simple gradient calculation. The ReLU can nonlinearly map output results of convolution layer. The output results of second layer is the data with the size of 112 × 112 × 64.
The pooling operator is connected after this layer for extracting the main features of a certain area, reducing the number of parameters, and preventing the model from over fitting. The maximum pooling with a 2 × 2 window and 2 strides is used in this layer. The pooling result is the data with the size of 56 × 56 × 64.
After pooling, the LRN (Local Response Normalization) is used to normalize the local response. The LRN enhances the larger response value and reduce the smaller response value. Thus, the LRN also can improve the generalization of the model. The output result of the LRN is the data with the size of 56 × 56 × 64.
Second layer: It is a convolution layer, which is with 128 3 × 3 filters and the stride is 1. The ReLU is selected as the activation function. The LRN is connected after this layer, the output results is the data with the size of 56×56×128. Then above data is processed by pooling operator with a 3 × 3 window and 2 strides. The output result of this layer is the data with the size of 28 × 28 × 128.
Third to eighth layer: These layers consist of three inception modules which consist of seven convolution modules and a pooling module. They are divided into the following four branches.
The first branch try convolution with 1 × 1 window on the output result of previous layer. The 1 × 1 convolution can efficiently enhance the expression ability, and at the same time, it can increase and reduce the dimension of the output channel. All four branches of the inception module use 1 × 1 convolution to perform low-cost (much less computation than 3 × 3 cross-channel feature transformation). Then 3 × 3 convolution is connected.
In the second branch, 1 × 1 convolution is used first, then 5 × 5 convolution is connected. The third branch is to use 3 × 3 maximum pooling with 2 strides and then connect 3 × 3 convolution. The fourth branch uses 1 × 1 convolution. Finally, the four branches are merged through an agammaegation operation at the end, and the output is the data with the size of 28 × 28 × 128.
After first inception modules, second and third inception modules are set in turn. The output is the data with the size of 28 × 28 × 480. Then, the pooling and LRN are performed, the data with the size of 14 × 14 × 480 is output.
Ninth layer: It is a convolution layer with 480 3 × 3 filters and their stride is 1. The ReLU is selected as the activation function. The result of this layer is the data with the size of 14 × 14 × 480. After convolution, the data is performed by LRN. Then, the maximum pooling with a 3 × 3 window and 2 strides is used. The output result is the data with the size of 6 × 6 × 480.
Tenth layer: This layer is dropout layer which is used to improve the generalization capability. The size of output result is 17,280.
Eleventh layer: This layer is the full connected layer with 100 neurons.
Twelfth layer: This layer is the Softmax activation function layer with 2 neurons.
In our ICSNet, we adopt the inception module from GoogLeNet, but only three inception modules are built in ICSNet. And we also add more convolution layers than AlextNet, but the number of convolution layers is less than VGGNet and ResNet. Consequently, the tradeoff between accuracy and time consuming can be achieved by our ICSNet. This is basic idea of our work.
We use the DJI Phantom 4 Advanced UAV to collect the color images with 8256 × 5504 pixels of Ipomoea cairica (L.) sweets in the monitoring area. The flight altitude is approximately 15 m above the ground. In our experiments, we set the distance according to the following three rules: 1) the clear images can be acquired; 2) the identifying accuracy can meet the actual requirements; 3) the UAV can be easily controlled. So we set the value of distance 15m above the ground according to our experience. The camera angle is adjusted in horizontal 45° for good front view since Ipomoea cairica (L.) sweets are a climbing weed. Finally, 100 raw images with 8256 × 5504 pixels are acquired. Figure 4 shows four samples of the scaled-down raw images.
We produce the dataset according to the following steps:
(1) The raw images are automatically divided into small images with 224 × 224 pixels by computer program. Figure 5 shows the samples of the images. Finally, 32000 samples with 224 × 224 pixels are selected, in which 24,000 samples contain Ipomoea cairica (L.) sweets and 8000 samples do not contain Ipomoea cairica (L.) sweets.
(2) The new samples are generated by horizontally and vertically rotating sample. Accordingly, 32000 samples are expanded to 96,000 samples.
(3) The new samples are generated by subtracting the mean of R, G and B from R, G, B value of sample, and Gray conversion. Finally, 288,000 samples are obtained to form the dataset in our experiments in which 216,000 samples contain Ipomoea cairica (L.) sweets and 72,000 samples do not contain Ipomoea cairica (L.) sweets.
In our experiments, the Accuracy, Precision, Recall, F1, AUC, min-batch size, computing cost and the number of layers are used to validate the performance of methods.
For test samples, TP denotes the number of correctly identified samples including Ipomoea cairica (L.) sweets, TN denotes the number of correctly identified samples without Ipomoea cairica (L.) sweets, FP denotes the number of incorrectly identified samples including Ipomoea cairica (L.) sweets, FN denotes the number of incorrectly identified samples without Ipomoea cairica (L.) sweets. The Accuracy, Precision, Recall, F1 are respectively written as follows:
Accuracy =TP+TNTP+TN+FP+FN×100% | (1) |
Precision =TPTP+TN×100% | (2) |
Recall =TP+TNTP+FN×100% | (3) |
F1=2× Precision × Recall Precision + Recall ×100% | (4) |
AUC is Area under the Curve of ROC (Receiver Operating Characteristics). The min-batch size is the number of samples in one batch. The time is the identifying time of samples. The number of layers is the number of convolutional and full connected layers, which can denote the complexity of the model. For obtaining valid results, the k-fold cross-validation (CV) is used to evaluate the algorithms and k is 10 in our experiments.
To efficiently validate the proposed method, we make comparisons with LeNet, AlexNet, GoogLeNet, VGG-19 and ResNet, all of which are deep CNNs [20].
(1) LeNet is firstly proposed by LeCun et al. in the 1990s, which includes three convolution layers, two sampling layers, two fully connected layers. At that time, it is difficult to run LeNet due to the limit of computation and memory capacity [21].
(2) AlexNet is proposed by Alex et al. and won the ILSVRC 2012 [22]. AlexNet achieves higher identifying accuracy than all the traditional machine learning algorithms. It is significant breakthrough for machine learning for classification.
(3) GoogLeNet is proposed by Christian of Google and is the winner of ILSVRC 2014 [23], in which inception layers, including different receptive areas with different kernel sizes capturing sparse correlation patterns, are integrated into CNN.
(4) VGG is proposed by Simonyan et al. in 2014, in which the convolution filter is a 3 × 3 filter and the stride is 2. VGG-11, VGG-16 and VGG-19 respectively include 11, 16 and 19 layers. The Softmax layer is the final layer for classification [24].
(5) ResNet is proposed by Kaiming et al. in 2015 to address to the vanishing gradient problem and shows the excellent ability of classification [25]. The popular ResNet consists of 49 convolution layers.
All the algorithms in our experiments are coded in Matlab R2019a. The values of initial parameters are randomly generated for networks. The parameters are set as follows: the number of epochs, learning rate drop factor, learning rate drop period, batch size are 200, 0.0004, 0.6, 30 and 128, respectively. The operating system of PC is Window 7 and the GPU is a NVIDIA with 8 GB memory.
The results of six algorithms are listed in Table 1 and Figure 6. Compared with ICSNet, ICSNet_n do not include inception modules. As we can see, the accuracy of ICSNet is 93.00%, the highest among six algorithms. The identifying time of ICSNet is 7.439 s, ranking the third shortest among six algorithms. The number of layers of ICSNet is 12, ranking the third lest among six algorithms. The accuracy of ResNet is equal to our algorithm, but their identifying time is more than that of our algorithm. The time of LeNet and AlexNet is less than that of our algorithm, but their accuracy is obviously lower than our algorithm. The Precision of our algorithm is 93.20%, only is lower than that of ResNet. The Recall of our algorithm is 93.30%, equal to that of ResNet ranking the first highest among six algorithms. The F1 of our algorithm is 93.00%, equal to that of ResNet, ranking the highest among six algorithms. The AUC of our algorithm is 94%, the second highest among six algorithms. Consequently, there is the best tradeoff between accuracy and time. Compared with LeNet, AlexNet, the number of layers of ICSNet is more, accordingly, the accuracy of ICSNet becomes higher but the time cost is only increased a little. The reason is that the size of filters is 5 × 5 in LeNet, the size of filters is 5 × 5 or 11 × 11 in AlexNet, the size of filters is 3 × 3 or 1 × 1 in ICSNet. For our algorithm, the size of filters is smaller than LeNet and AlexNet.
Algorithms | Accuracy (%) | Precision (%) | Recall (%) | F1 (%) | AUC | Min-batch size | Time(s) | The number of Layers |
ICSNet | 93.00 | 93.20 | 93.30 | 93.00 | 94.00 | 128 | 7.439 | 12 |
ICSNet_n | 80.50 | 81.60 | 80.50 | 80.50 | 81.44 | 128 | 6.451 | 7 |
LeNet | 80.50 | 81.42 | 80.64 | 80.50 | 81.00 | 128 | 6.511 | 7 |
AlexNet | 85.00 | 84.35 | 84.41 | 84.38 | 85.00 | 128 | 6.953 | 8 |
VGG-19 | 91.00 | 92.30 | 92.00 | 92.15 | 91.50 | 28 | 16.342 | 19 |
GoogLeNet | 92.50 | 92.64 | 92.50 | 93.00 | 93.00 | 80 | 13.192 | 22 |
ResNet-18 | 91.10 | 92.42 | 92.15 | 92.24 | 92.00 | 20 | 13.012 | 18 |
ResNet-50 | 92.15 | 92.50 | 92.15 | 92.50 | 92.50 | 20 | 20.160 | 50 |
ResNet152 | 93.00 | 93.45 | 93.30 | 93.00 | 94.20 | 20 | 31.879 | 152 |
We also see that, the more the number of the layers of networks, the larger the min-batch size is. But the VGG-19 is just the opposite due to its too much layers. The inception module induces the number of parameters and computing cost of the GoogLeNet and ICSNet, and remains more high identifying ability.
Figure 7 shows the relation between Min-batch size and Accuracy, Epoches. As we see in Figure 7(a), when the Min-batch size is 128, the accuracy is the highest. This indicates the Min-batch size is relation to the accuracy of algorithm. In Figure 7(b), the larger is the Min-batch size, the larger is the number of epochs. When the Min-batch size is more than 256, the number of epochs is sharp large. The larger is the number of the epochs, the more is the consumed time when the algorithm converges. We also can see, when the Min-batch size is 128, the accuracy of algorithm is high, and the number of epochs is relatedly small.
The Table 2 lists the accuracy of each fold in test sets in the 10-folds CV and the confusion matrix of each fold. In Table 2, "A" denotes the image containing Ipomoea cairica (L.) sweets, "B" denotes the image without Ipomoea cairica (L.) sweets.
Fold | Class | Number | A | B | Accuracy (%) |
1 | A | 21600 | 20079 | 1521 | 93.00 |
B | 7200 | 495 | 6705 | ||
2 | A | 21600 | 20036 | 1564 | 93.00 |
B | 7200 | 452 | 6748 | ||
3 | A | 21600 | 20415 | 1185 | 94.48 |
B | 7200 | 405 | 6885 | ||
4 | A | 21600 | 20440 | 1160 | 94.41 |
B | 7200 | 539 | 6661 | ||
5 | A | 21600 | 19814 | 1786 | 90.12 |
B | 7200 | 748 | 6452 | ||
6 | A | 21600 | 20596 | 1004 | 95.42 |
B | 7200 | 315 | 6885 | ||
7 | A | 21600 | 19491 | 2109 | 89.60 |
B | 7200 | 886 | 6314 | ||
8 | A | 21600 | 19795 | 1805 | 91.22 |
B | 7200 | 729 | 6471 | ||
9 | A | 21600 | 21122 | 478 | 97.57 |
B | 7200 | 221 | 6979 | ||
10 | A | 21600 | 19966 | 1634 | 91.18 |
B | 7200 | 906 | 6294 | ||
Average | 93.00 |
Compared with LeNet, AlexNet, the number of layers of ICSNet is more, accordingly, the accuracy of ICSNet becomes higher but the time cost is only increased a little. Compared with GoogLeNet and ResNet, the time cost is obviously reduced. Since the layers of network is closely related to the time cost, our algorithm is designed considering their tradeoff.
The ICSNet aims to accurately identify Ipomoea cairica (L.) sweets and non-Ipomoea cairica (L.) sweets in the wild. Some incorrectly identified samples are shown in Figure 8. We can see that, in Figure 8(b–e) , (g), (k) and (l), the parts of Ipomoea cairica (L.) sweets are clear, but their proportions are very small. It is reason that they are incorrectly identified by our algorithms. For Figure 8 (f), (d) and (i), the bad quality of images affects the performance of our algorithm. The samples in Figure 8 (a) is too dark, so the misidentification is excusable. To solve the problem, in future work, we need consider two aspects: (1) Set the parameters of camera of UAV for good quality of acquired images. (2) Determine the right distance between UAV and Ipomoea cairica (L.) sweets for good quality of acquired images.
Based on the results of our algorithm, we can draw the area of Ipomoea cairica (L.) sweets in the raw images or map to help management to efficiently monitor the Ipomoea cairica (L.) sweets and take measures to protect the environment. The raw images are shown in Figure 9 (a) and (c), and the corresponding images, in which the area of Ipomoea cairica (L.) sweets is painted red, are shown in Figure 9 (b) and (d). In Figure 9 (a) and (c), the images in big yellow box are zoomed, which are corresponding the images in small yellow box. As we can see in Figure 9, the area of Ipomoea cairica (L.) sweets can be intuitively found.
Ipomoea cairica (L.) sweets is an invasive weed which has caused serious harm to the biodiversity and stability of the ecosystem. It is very important to accurately and rapidly identifying Ipomoea cairica (L.) sweets in the wild for management taking the necessary strategies to control the Ipomoea cairica (L.) sweets to rapidly grow in the wild. In this study, we propose an identification framework and method for Ipomoea cairica (L.) sweets based on UAV and AI.
The UAV can help us to low-costly acquire lots of high quality of images of monitoring areas. The deep CNN is designed to efficiently identify Ipomoea cairica (L.) sweets in the acquired images. To validate the efficiency of proposed method, we generated 288000 samples in our experiments, and made comparison with LeNet, AlexNet, GoogleNet, VGG and ResNet. The experimental results showed that our method was excellent, the identifying accuracy is 93.00% and the identifying time is 7.439 s. Our work can help to promote the performance of online monitoring systems for Ipomoea cairica (L.) sweets.
In our experiments, we set the distance according to the following three rules: 1) the clear images can be acquired; 2) the identifying accuracy can meet the actual requirements; 3) the UAV can be easily controlled. In addition, we also consider the sensor resolution. So we set the value of distance 15m above the ground according to our experience. When the value of distance is higher than 15 m, the collected images became more and more vague. This will affect the accuracy of model. When the value of distance is lower than 15 m, although we can collect clear images, this will consume the more time to collect the data and process data.
For our detection frame and model, the size of images can be set as the possible predefined size. The size of image affects not only the accuracy of model but also the monitoring accuracy. It is important to design an excellent model and algorithm in the special application. So, in future, we will further study the question.
Our model also can be regarded as the special GoogLeNet. The reason simplifying GoogLeNet is to achieve a tradeoff between accuracy and time. In addition, we also adopt the idea of VGGNet, that is 3 × 3 filters is used in our model to further reduce the time complexity. For our algorithm, the idea of ResNet is not adopted. The reason is that we find the performance cannot meet our requirements.
In the future, we will acquire more Ipomoea cairica (L.) sweets images to train ICSNet model for further improving the identifying accuracy of model. In addition, we also will build the dynamic monitoring system of Ipomoea cairica (L.) sweets.
The work is funded by the Scientific Research Team Project of Shenzhen Institute of Information Technology (SZIIT2019KJ022), Special Innovation Project of Guangdong Education Department (2019GKTSCX093) and National Natural Science Foundation of China (71971089).
The authors declare that there is no conflict of interests regarding the publication of article.
[1] | Hristova K, Pella D, Singh R, et al. (2014) Sofia declaration for prevention of cardiovascular diseases and type 2 diabetes mellitus: a scientific statement of the international college of cardiology and international college of nutrition. World Heart J 6: 824-829. |
[2] | Shehab A, Elkilany G, Singh R, et al. (2015) Coronary risk factors in Southwest Asia. World Heart J 7: 21-23. |
[3] | James K (1958) The Holy Bible. Deuteronomy, editor. London: Collins; 1611. |
[4] | Eliot TA (1942) Choice of Kipling's Verse (1943). Recessional (1898). London: Faber & Faber Ltd. |
[5] | WHO (2010) Water for health; WHO Guidelines for drinking-water quality. |
[6] | WHO (2004) Global strategy on diet, physical activity and health. Geneva: World Health Organization. |
[7] | Todorovic V, Micklewright A (2011) A pocket guide to clinical nutrition. 4th Edition. |
[8] | Finch S, Doyle W, Lowe C, et al. (2000) Report of the diet and nutrition survey. London: TSO: National diet and nutrition survey: people aged 65 years and over 1998. |
[9] | FAO (2015) State of food insecurity in the world (FAO). Rome: FAO. |
[10] | Chakraborty P (2016) Water demand management and its linkage to economically weaker social group: an analysis of basic issues and perspective. In: Basu S, Kumar, Zandi P, Chalaras SK, editors. Global environmental crises, challenges and sustainable solutions from multiple perspectives. Iran: Haghshenass publications. |
[11] | NHP-India (2015) National Vector Bourne Disease Control Programme (India). http://www.nhp.gov.in/national-vector-borne-disease-control-programme_pg. |
[12] | Anon (2016) Food and Agriculture Organization. http://www.fao.org/worldfoodsituation/en/. |
[13] | Kubień S (1839) Resko: Stories of the old. The genius of agriculture. |
[14] | Whitson A, Walster H (1918) Soils and soil fertility St Paul, MN USA: Webb. |
[15] | Jejeebho, Santhya K (2011) Sexual and reproductive health of young people in India: A review of policies, laws and programmes. India Habitat Centre, New Delhi: Population Council. |
[16] | De Meester F (2008) Wild-type land based foods in health promotion and disease prevention: the LDL-CC:HDL-CC model. In: De Meester F, Watson RR, eds. Wild type foods in health promotion and disease prevention. NJ: Humana Press 3-30. |
[17] | De Meester F (2014) Obesity is a "communicable" mind disease. Approaches Aging Control 18: 7-10. |
[18] | Madhya Pradesh becomes first state to have 'Happiness' Department. Press Trust India 2016. |
[19] | Khaishgi A (2016) Happiness is a serious job: UAE's Minister of Happiness embraces new role. The National, UAE. |
[20] | UN summit agenda [press release]. 7 September 2000. |
[21] | Mohamed KS, Sathianandan T, Zacharia P, et al. (2010) Depleted and collapsed marine fish stocks along south-west coast of India-a simple criterion to assess the status. Meenakumari B, Boopendranath M, Leela Edwin, Sankar T, Gopal N, Ninan G, editors: Society of Fisheries Technologists, Cochin, Philipose Varughese, S. |
[22] |
Fox-Rushby J, Hanson K (2001) Calculating and presenting disability adjusted life years (DALYs) in cost-effectiveness analysis. Health Policy Plan 16: 326-331. doi: 10.1093/heapol/16.3.326
![]() |
[23] | Larson b (2013) Calculating disability-adjusted-life-years lost (DALYs) in discrete-time. Cost Eff Resour Alloc 11. |
[24] | Anon (2017) NGO verification. http://www.ngosindia.com/add-your-ngo/ngo-verification/. |
[25] |
Ruiz M, Sharma A (2016) Application of GIS in public health in India: A literature-based review, analysis, and recommendations. Indian J Public Health 60: 51-58. doi: 10.4103/0019-557X.177308
![]() |
[26] | Griffiths K, Wilson D, Singh R, et al. (2014) Effect of dietary phytoestrogens on human growth regulation: imprinting in health & disease. Indian J Med Res 140: S82-S90. |
[27] |
Reddy K (2015) India's aspirations for universal health coverage. N Engl J Med 373: 1-5. doi: 10.1056/NEJMp1414214
![]() |
[28] | ESCAP (2015) India and the MDGs:Towards a sustainable future for all. United Nations. |
[29] | Dubbudu R (2015) Has India achieved the Millennium Development Goals (MDG)? FACTLY. |
[30] |
Kumar S, Kumar N, Vivekadhish S (2016) Millennium Development Goals (MDGs) to Sustainable Development Goals (SDGs): Addressing unfinished agenda and strengthening sustainable development and partnership. Indian J Community Med 41: 1-4. doi: 10.4103/0970-0218.170955
![]() |
[31] | Anon (2015) New York: The Economist; 2015. The economics of optimism. The debate heats up about what goals the world should set itself for 2030. (Date last accessed 18/02/2017). |
[32] |
Liu L, Oza S, Hogan D, et al. (2015) Global, regional, and national causes of child mortality in 2000–13, with projections to inform post-2015 priorities: an updated systematic analysis. Lancet 385: 430-440. doi: 10.1016/S0140-6736(14)61698-6
![]() |
[33] | WHO (2015) Trends in Maternal Mortality: 1990 to 2013 Estimates by WHO, UNICEF, UNFPA, The World Bank and the United Nations Population Division. |
[34] | Anon (2015) Progress on sanitation and drinking water 2015 update and MDG assessment-ISBN 978 92 4 1501945. In: UNICEF, editor. USA: UNICEF and WHO. |
[35] | Anon (2015) Ministry of Statistics and Programme Implementation, Government of India; India Country Report. In: Social Statistics Division, editor. New Dehli, India: Government of India. |
[36] | Dubey K (2014) Last speech of Indira Gandhi…." every drop of my blood will invigorate India and strengthen it ". Times of Congress. |
[37] | Green-Foundation (2016) Green Foundation: Janadhanya established 2006 http://greenfoundation.in/content/who-we-are. |
[38] | Anon (2013) Indian scientists warn PM Manmohan Singh over GM crop dangers. |
[39] | Anon (2009) National Programme for the prevention and control of diabetes, cardiovascular diseases and stroke: A guide for health workers. Srivastava R, Nandan D, editors: NPDCS. |
[40] | National Health Mission (2013) http://nrhm.gov.in/nhm/nrhm.html: Government of India. |
[41] | Anon (2006) Public Health Foundation of India, Delhi. http://www.phfi.org/. |
[42] | Anon. Integrated Disease Surveillance Programme. http://www.idsp.nic.in/index4.php?lang=1&level=0&linkid=313&lid=15922004 |
[43] | Basu T. International College of Nutrition.: http://www.icnhealthfoods.com |
[44] | Buttar H, Li T, Ravi N (2005) Prevention of cardiovascular diseases: Role of exercise, dietary interventions, obesity and smoking cessation. Exp Clin Cardiol 10: 229-249. |
[45] |
West E (1964) Adam Smith's two views on the division of labour. Economica 31: 23-32. doi: 10.2307/2550924
![]() |
[46] | Chauhan A, Singh R, Ozimek L, et al. (2016) Saturated fatty acid and sugar; how much is too much for health? A scientific statement of the International College of Nutrition. World Heart J 8: 71-78. |
[47] |
Balarajan Y, Selvaraj S, Subramanian S (2011) Health care and equity in India. Lancet 377: 505-515. doi: 10.1016/S0140-6736(10)61894-6
![]() |
[48] | Coonin V (2014) From marble to flesh. The biography of Michelangelo's David. Florence: B'gruppo. |
[49] | Hunt J (1953) The ascent of Everest. London: Hodder & Stoughton. |
[50] |
Singh R, Dubnov G, Niaz M, et al. (2002) Effect of an Indo-Mediterranean diet on progression of coronary artery disease in high risk patients (Indo-Mediterranean Diet Heart Study): a randomised single-blind trial. The Lancet 360: 1455-1461. doi: 10.1016/S0140-6736(02)11472-3
![]() |
[51] |
Lyseen A, Nøhr C, Sørensen E, et al. (2014) A review and framework for categorizing current research and development in health related Geographical Information Systems (GIS) studies. Yearb Med Inform 9: 110-124. doi: 10.15265/IY-2014-0008
![]() |
[52] | Bernatzky A (1989) Ecological principles in town planning. Environment and Health; a holistic approach. Aldershot, England: Avebury, Gower Publishing Company. |
[53] |
FitzGerald E, Frasso R, Dean L, et al (2013) Community-generated recommendations regarding the urban nutrition and tobacco environments: a photoelicitation study in Philadelphia. Prev Chronic Dis 10: 120204. DOI: http://dx.doi.org/10.5888/pcd10.120204 . doi: 10.5888/pcd10.120204
![]() |
[54] |
Powell L, Baob Y (2009) Food prices, access to food outlets and child weight. Economics Hum Biology 7: 64-72. doi: 10.1016/j.ehb.2009.01.004
![]() |
[55] |
Chapman1 K, Nicholas P, Banovic D, et al. (2006) The extent and nature of food promotion directed to children in Australian supermarkets. Health Promot Int 21: 331-339. doi: 10.1093/heapro/dal028
![]() |
[56] |
Sallis J, Glanz K (2006) The role of built environments in physical activity, eating, and obesity in childhood. Future Children 16: 89-108. doi: 10.1353/foc.2006.0009
![]() |
[57] |
Laraia B, Siega-Riz A, Kaufman J, et al. (2004) Proximity of supermarkets is positively associated with diet quality index for pregnancy. Prevent Med 39: 869-875. doi: 10.1016/j.ypmed.2004.03.018
![]() |
[58] |
Ball K JM, Jackson M (2014) The feasibility and appeal of mobile 'apps' for supporting healthy food purchasing and consumption among socioeconomically disadvantaged women: a pilot study. Health Promot J Australia 25: 79-82. doi: 10.1071/HE13096
![]() |
[59] | Griffiths K, Aggarwal B, Singh R, et al. (2016) Food antioxidants and their anti-inflammatory properties: a potential role in cardiovascular diseases and cancer prevention. Diseases 4: 1-15. |
[60] | Griffiths K, Adlercreutz H, Boyle P, et al. (1996) Nutrition and Cancer. Saxon Beck, Oxford: Isis Medical Media Ltd. |
[61] | Griffiths K, Denis L, Turkes A (2002) Oestrogens, phyto-oestrogens and the pathogenesis of prostatic disease. London: Martin Dunitz Ltd p420. |
[62] | Trudeau V, Turque N, Le Mevel S, et al. (2005) Assessment of estrogenic endocrine-disrupting chemical actions in the brain using in vivo somatic gene transfer. Environmental Health Perspect 113: 329-334. |
[63] | He Z, Ferguson S, Cui L, et al. (2011) Development of the sexually dimorphic nucleus of the preoptic area and the influence of estrogen-like compounds. Neural Regen Res 8: 2763-2774. |
[64] | Itharat A, Onsaard E, Singh R, et al. (2016) Flavonoids consumption and the heart. World Heart J 8: 103-108. |
[65] |
Hollenberg N, Fisher N, McCullough M (2009) Flavanols, the Kuna, cocoa consumption, and nitric oxide. J Am Soc Hypertens 3: 105-112. doi: 10.1016/j.jash.2008.11.001
![]() |
[66] |
Aggarwal B, Ichikawa H, Garodia P, et al. (2006) From traditional Ayurvedic medicine to modern medicine: identification of therapeutic targets for suppression of inflammation and cancer. Expert Opinion Therapeutic Targets 10: 87-118. doi: 10.1517/14728222.10.1.87
![]() |
[67] |
Sacco R, Roth G, Reddy K, et al. (2016) The heart of 25 by 25: achieving the goal of reducing global and regional premature deaths from cardiovascular diseases and stroke: a modeling study from the American Heart Association and World Heart Federation. Circulation 133: e674-690. doi: 10.1161/CIR.0000000000000395
![]() |
[68] | Expert-Group, International College of Cardiology (2016). The challenges of prevention of cardiovascular diseases. A scientific statement of the International College of Cardiology. World Heart J 8 (4) In Press. |
[69] | Health: Dementia now leading cause of death [press release]. BBC, November 16th 2016. |
[70] | Gogtay N, Bhatt H, Dalvi S, et al. (2002) The use and safety of non-allopathic Indian medicines. Drug Saf 25 (14): 1005-1019. |
[71] | Ghosh A, Lobo A (2017) Bay of Bengal: depleted fish stocks and huge dead zone signal tipping point. The Guardian https://www.theguardian.com/environment/2017/jan/31/bay-bengal-depleted-fish-stocks-pollution-climate-change-migration. |
[72] | Verma S, Singh S (2008) Current and future status of herbal medicines. Vet World 1: 347-350. |
[73] | Beckett K (2017) Understanding access and benefit sharing. The Biologist 64: 26-27. |
[74] |
Cressey D (2017) Treaty to stop biopiracy threatens to delay flu vaccines. Nature 542: 148. doi: 10.1038/542148a
![]() |
[75] | UN (2011) Nagoya protocol on access to genetic resources and the fair and equitable sharing of benefits arising from their utilization to the convention on biological diversity. In: Programme. SotCoBDUNE, editor. 413 St. Jacques Street West, Suite 800 Montreal, Quebec, Canada H2Y 1N9: Secretariat of the Convention on Biological Diversity. Canada. |
[76] | Mohanaan M, Hay K, Mor N (2016) Quality Of health care in India: challenges,priorities, and the road ahead. Health Affairs 35. |
[77] | Das S, Pal S, Ghosal M (2012) Dementia: Indian scenario. Neurol India:60 (6) 618-624. |
[78] | Brijnath B (2014) Love and the culture of dementiacare in India. New York: Berghahn p240. |
[79] |
Malik M, Jacob K (2015) Psychological morbidity among co-residents of older people in rural South India: prevalence and risk factors. Int J Social Psychiatry 61: 183-187. doi: 10.1177/0020764014539287
![]() |
[80] |
Prabhakar P, Chandra S, Supriya M, et al. (2015) Vitamin D status and vascular dementia due to cerebral small vessel disease in the elderly Asian Indian population. J Neurol Sci 359: 108-111. doi: 10.1016/j.jns.2015.10.050
![]() |
1. | Nur Adibah Mohidem, Nik Norasma Che’Ya, Abdul Shukor Juraimi, Wan Fazilah Fazlil Ilahi, Muhammad Huzaifah Mohd Roslim, Nursyazyla Sulaiman, Mohammadmehdi Saberioon, Nisfariza Mohd Noor, How Can Unmanned Aerial Vehicles Be Used for Detecting Weeds in Agricultural Fields?, 2021, 11, 2077-0472, 1004, 10.3390/agriculture11101004 | |
2. | M. Shyamala Devi, J. Arun Pandian, Mahesh Baburao Lonare, Yeluri Praveen, 2022, Efficient Net Transfer Learning based Early Prediction of Monkey Pox Lesion, 978-1-6654-9764-0, 1297, 10.1109/ICOSEC54921.2022.9952099 | |
3. | Pu Zhang, Research on intelligent elevation control method of shearer drum based on deep learning, 2023, 1751-8806, 10.1049/sfw2.12089 | |
4. | Tieying Huang, Rahim Khan, Facility Layout Optimization of Urban Public Sports Services under the Background of Deep Learning, 2022, 2022, 1687-5273, 1, 10.1155/2022/1748319 | |
5. | Fei Luo, Jackrit Suthakorn, Robot Fault Detection Based on Big Data, 2023, 2023, 1687-5257, 1, 10.1155/2023/8375382 | |
6. | Waqas Ahmed, Vamsi Krishna Kommineni, Birgitta König-Ries, Jitendra Gaikwad, Luiz Gadelha, Sheeba Samuel, Evaluating the method reproducibility of deep learning models in biodiversity research, 2025, 11, 2376-5992, e2618, 10.7717/peerj-cs.2618 | |
7. | Fei Xing, Ru An, Xulin Guo, Xiaoji Shen, Mapping invasive noxious weed species in the alpine grassland ecosystems using very high spatial resolution UAV hyperspectral imagery and a novel deep learning model, 2024, 61, 1548-1603, 10.1080/15481603.2024.2327146 |
Algorithms | Accuracy (%) | Precision (%) | Recall (%) | F1 (%) | AUC | Min-batch size | Time(s) | The number of Layers |
ICSNet | 93.00 | 93.20 | 93.30 | 93.00 | 94.00 | 128 | 7.439 | 12 |
ICSNet_n | 80.50 | 81.60 | 80.50 | 80.50 | 81.44 | 128 | 6.451 | 7 |
LeNet | 80.50 | 81.42 | 80.64 | 80.50 | 81.00 | 128 | 6.511 | 7 |
AlexNet | 85.00 | 84.35 | 84.41 | 84.38 | 85.00 | 128 | 6.953 | 8 |
VGG-19 | 91.00 | 92.30 | 92.00 | 92.15 | 91.50 | 28 | 16.342 | 19 |
GoogLeNet | 92.50 | 92.64 | 92.50 | 93.00 | 93.00 | 80 | 13.192 | 22 |
ResNet-18 | 91.10 | 92.42 | 92.15 | 92.24 | 92.00 | 20 | 13.012 | 18 |
ResNet-50 | 92.15 | 92.50 | 92.15 | 92.50 | 92.50 | 20 | 20.160 | 50 |
ResNet152 | 93.00 | 93.45 | 93.30 | 93.00 | 94.20 | 20 | 31.879 | 152 |
Fold | Class | Number | A | B | Accuracy (%) |
1 | A | 21600 | 20079 | 1521 | 93.00 |
B | 7200 | 495 | 6705 | ||
2 | A | 21600 | 20036 | 1564 | 93.00 |
B | 7200 | 452 | 6748 | ||
3 | A | 21600 | 20415 | 1185 | 94.48 |
B | 7200 | 405 | 6885 | ||
4 | A | 21600 | 20440 | 1160 | 94.41 |
B | 7200 | 539 | 6661 | ||
5 | A | 21600 | 19814 | 1786 | 90.12 |
B | 7200 | 748 | 6452 | ||
6 | A | 21600 | 20596 | 1004 | 95.42 |
B | 7200 | 315 | 6885 | ||
7 | A | 21600 | 19491 | 2109 | 89.60 |
B | 7200 | 886 | 6314 | ||
8 | A | 21600 | 19795 | 1805 | 91.22 |
B | 7200 | 729 | 6471 | ||
9 | A | 21600 | 21122 | 478 | 97.57 |
B | 7200 | 221 | 6979 | ||
10 | A | 21600 | 19966 | 1634 | 91.18 |
B | 7200 | 906 | 6294 | ||
Average | 93.00 |
Algorithms | Accuracy (%) | Precision (%) | Recall (%) | F1 (%) | AUC | Min-batch size | Time(s) | The number of Layers |
ICSNet | 93.00 | 93.20 | 93.30 | 93.00 | 94.00 | 128 | 7.439 | 12 |
ICSNet_n | 80.50 | 81.60 | 80.50 | 80.50 | 81.44 | 128 | 6.451 | 7 |
LeNet | 80.50 | 81.42 | 80.64 | 80.50 | 81.00 | 128 | 6.511 | 7 |
AlexNet | 85.00 | 84.35 | 84.41 | 84.38 | 85.00 | 128 | 6.953 | 8 |
VGG-19 | 91.00 | 92.30 | 92.00 | 92.15 | 91.50 | 28 | 16.342 | 19 |
GoogLeNet | 92.50 | 92.64 | 92.50 | 93.00 | 93.00 | 80 | 13.192 | 22 |
ResNet-18 | 91.10 | 92.42 | 92.15 | 92.24 | 92.00 | 20 | 13.012 | 18 |
ResNet-50 | 92.15 | 92.50 | 92.15 | 92.50 | 92.50 | 20 | 20.160 | 50 |
ResNet152 | 93.00 | 93.45 | 93.30 | 93.00 | 94.20 | 20 | 31.879 | 152 |
Fold | Class | Number | A | B | Accuracy (%) |
1 | A | 21600 | 20079 | 1521 | 93.00 |
B | 7200 | 495 | 6705 | ||
2 | A | 21600 | 20036 | 1564 | 93.00 |
B | 7200 | 452 | 6748 | ||
3 | A | 21600 | 20415 | 1185 | 94.48 |
B | 7200 | 405 | 6885 | ||
4 | A | 21600 | 20440 | 1160 | 94.41 |
B | 7200 | 539 | 6661 | ||
5 | A | 21600 | 19814 | 1786 | 90.12 |
B | 7200 | 748 | 6452 | ||
6 | A | 21600 | 20596 | 1004 | 95.42 |
B | 7200 | 315 | 6885 | ||
7 | A | 21600 | 19491 | 2109 | 89.60 |
B | 7200 | 886 | 6314 | ||
8 | A | 21600 | 19795 | 1805 | 91.22 |
B | 7200 | 729 | 6471 | ||
9 | A | 21600 | 21122 | 478 | 97.57 |
B | 7200 | 221 | 6979 | ||
10 | A | 21600 | 19966 | 1634 | 91.18 |
B | 7200 | 906 | 6294 | ||
Average | 93.00 |