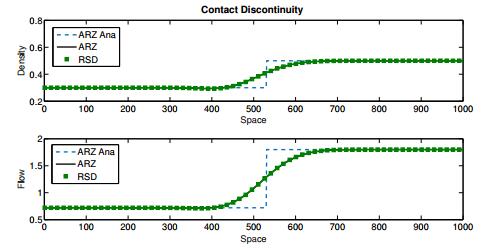
Citation: Dora Brites. Cell ageing: a flourishing field for neurodegenerative diseases[J]. AIMS Molecular Science, 2015, 2(3): 225-258. doi: 10.3934/molsci.2015.3.225
[1] | Akshaj Pole, Manjari Dimri, Goberdhan P. Dimri . Oxidative stress, cellular senescence and ageing. AIMS Molecular Science, 2016, 3(3): 300-324. doi: 10.3934/molsci.2016.3.300 |
[2] | Patrizia A d'Alessio, Marie C Béné, Chantal Menut . d-Limonene challenging anti-inflammatory strategies. AIMS Molecular Science, 2022, 9(2): 46-65. doi: 10.3934/molsci.2022003 |
[3] | Laetitia Weinhard, Paolo d'Errico, Tuan Leng Tay . Headmasters: Microglial regulation of learning and memory in health and disease. AIMS Molecular Science, 2018, 5(1): 63-89. doi: 10.3934/molsci.2018.1.63 |
[4] | Brandt D. Pence . Recent developments and future perspectives in aging and macrophage immunometabolism. AIMS Molecular Science, 2021, 8(3): 193-201. doi: 10.3934/molsci.2021015 |
[5] | Leena Latonen . Protein aggregation in neurodegenerative disease: the nucleolar connection. AIMS Molecular Science, 2015, 2(3): 324-331. doi: 10.3934/molsci.2015.3.324 |
[6] | Tsuyoshi Inoshita, Yuzuru Imai . Regulation of vesicular trafficking by Parkinson's disease-associated genes. AIMS Molecular Science, 2015, 2(4): 461-475. doi: 10.3934/molsci.2015.4.461 |
[7] | Ashok Chakraborty, Anil Diwan . Molecular mechanisms of neurodegenerative disease (NDD). AIMS Molecular Science, 2023, 10(3): 171-185. doi: 10.3934/molsci.2023012 |
[8] | Zhaoping Qin, Patrick Robichaud, Taihao Quan . Oxidative stress and CCN1 protein in human skin connective tissue aging. AIMS Molecular Science, 2016, 3(2): 269-279. doi: 10.3934/molsci.2016.2.269 |
[9] | Amena W. Smith, Swapan K. Ray, Arabinda Das, Kenkichi Nozaki, Baerbel Rohrer, Naren L. Banik . Calpain inhibition as a possible new therapeutic target in multiple sclerosis. AIMS Molecular Science, 2017, 4(4): 446-462. doi: 10.3934/molsci.2017.4.446 |
[10] | Uday Chintapula, Samir M Iqbal, Young-Tae Kim . A compendium of single cell analysis in aging and disease. AIMS Molecular Science, 2020, 7(1): 49-69. doi: 10.3934/molsci.2020004 |
Second order traffic models have been investigated since the early 70's (e.g. [27,33]) and refined with the introduction of the Aw-Rascle-Zhang (ARZ) model [3] and are still subject of current research. Traffic models in general range from individual based microscopic to averaged macroscopic considerations, see [9,15,19,32] and the references therein. The motivation for the consideration of second order traffic models is that on the one hand microscopic models may explode for a large number of cars and, on the other hand, first order models are supposed to be dependent on the traffic density only and hence lack of real-world effects. In particular, macroscopic traffic flow models with delay terms have gained special attention recently, see [30,26]. While second order traffic models without delay terms are well understood on a theoretical and analytical level [3,9,15], models of this type including time delays are less considered so far [26], and if considered, the delay is usually rewritten by a Taylor approximation [30,26]. In contrast, the explicit modeling of reaction times via delays is a well-known approach in microscopic traffic modeling, see e.g. [6], and therefore the intention is that the introduction of an explicit delay can make macroscopic models more reasonable. More precisely, we expect similar effects as in microscopic delayed models such as the developing of stop-and-go waves based on the reaction time.
Starting from car-following type models, our aim is therefore to develop and study second order macroscopic models with an explicit dependence on the delay term. For this purpose, we consider the explicit derivation of delayed second order models from delayed microscopic models in three different ways following [4,22,30]. We will end up with a system of equations for the traffic density
$ ∂tρ+∂x(ρv)=0,∂t(ρw)+∂x(ρvw)=0, $
|
(1) |
where
For the numerical simulation of the delayed macroscopic models, we use finite differences methods in a straightforward way and combine the method of lines with solvers for delayed differential equations. We analyze numerically the delayed models and compare the solutions to the classical the ARZ model. A comparison to real data and the corresponding fit of parameters (similar to [14]) is also presented.
This work is organized as follows: In section 2 we rigorously derive three second order macroscopic models based on the delayed microscopic model description according to [4,22,30]. The connection to the classical non-delayed ARZ model is also shown. In section 3, we introduce and discuss numerical methods for the solution of microscopic models with delay and second order delayed traffic models using ideas from [5]. In section 4, we present simulation results for comparisons of the different model level of descriptions and particularly stress on phenomena of the delayed macroscopic models.
In this section we derive macroscopic traffic models from delayed microscopic models of the car-following or follow-the-leader-type using three different approaches, cf. [4,22,30]. The resulting models are hyperbolic delay partial differential equations, where the time delay is explicitly kept and appears as an additional term in the equation for the velocity. As we will see, this new type of models gives rise to potential new dynamical behavior.
The microscopic model under consideration dates back to the 1950s and belongs to the class of car-following models, see e.g. [6]. In particular, we consider a system of ordinary differential equations for the location
$ ˙xi(t)=vi(t)˙vi(t)=C(vi+1(t−T)−vi(t−T))(xi+1(t−T)−xi(t−T))γ+1,i=1,…,N, $
|
(2) |
with
Apparently, the acceleration at time
$ ˙xi(t)=vi(t)˙vi(t)=vrefΔXγ(vi+1(t−T)−vi(t−T))(xi+1(t−T)−xi(t−T))γ+1,i=1,…,N. $
|
(3) |
The microscopic model equations (3) are the staring point for the macroscopic equations. We focus on three approaches, i.e. reverse spatial discretization [4], coarse graining [22], Taylor method [30], to derive the corresponding macroscopic delayed models. All approaches lead to second order traffic models that which mainly differ in the delay term.
The first approach we are interested in is based on a derivation proposed in [4], where the classical ARZ model is derived from (2). Here, we apply the same technique to the delayed equations (3) by identifying the local density
$ \rho_i(t): = \frac{\Delta X}{x_{i+1}(t)-x_i(t)}. $ |
We further define
$ P(\tau): = {vrefγτ−γifγ>0−vrefln(τ)ifγ=0. $
|
Then, the new variables
$ ˙τi(t)=˙xi+1(t)−˙xi(t)ΔX=vi+1(t)−vi(t)ΔX˙wi(t)=˙vi(t)+ddtP(τi(t))=vrefΔX(vi+1(t−T)−vi(t−T))τi(t−T)γ+1+P′(τi(t))˙τi(t)=vrefτi(t−T)γ+1(vi+1(t−T)−vi(t−T))ΔX−vrefτi(t)γ+1vi+1(t)−vi(t)ΔX $
|
(4) |
since
$ ∂tτ(x,t)=∂xv(x,t)∂tw(x,t)=vref(∂xv(x,t−T)ρ(x,t−T)γ+1−∂xv(x,t)ρ(x,t)γ+1), $
|
(5) |
where
$ ∂tτ(x,t)=∂xv(x,t),∂tw(x,t)=0. $
|
(6) |
In fact, a direct computation shows that (5) is equivalent to
$ ∂tτ(x,t)=∂xv(x,t)∂tv(x,t)=vref∂xv(x,t−T)ρ(x,t−T)γ+1 $
|
(7) |
applying the definitions of
Let us take a closer look at the right-hand side of the momentum equation (7). Compared to the classical model, we now get the temporal change in speed dependent on the density and the spatial derivative of speed from an earlier time. For the interpretation of
So far, we have considered the Lagrangian macroscopic equations (5) and (7), respectively. In a next step, we transform these equations into Eulerian coordinates by introducing the new variables
$ ∂xˆx=τ,∂xˆt=0,∂tˆx=v,∂tˆt=1, $
|
(8) |
or, exploiting
$ ∂tτ=∂ˆtτ+(∂ˆxτ)v,∂xv=(∂ˆxv)τ,∂tw=∂ˆtw+(∂ˆxw)v. $
|
(9) |
In the following, we write
$ ∂tρ(ˆx,t)+∂ˆx(ρ(ˆx,t)v(ˆx,t))=0∂t(ρ(ˆx,t)w(ˆx,t))+∂ˆx(ρ(ˆx,t)v(ˆx,t)w(ˆx,t))=vref(∂ˆxv(ˆx,t−T)ρ(ˆx,t−T)γ−∂ˆxv(ˆx,t)ρ(ˆx,t)γ) $
|
(10) |
which are obviously closely related to the undelayed ARZ model.
Another approach to derive a delayed macroscopic equation is the so-called coarse-graining (CG) proposed in [12,22]. Here, we focus on [22] and highlight the most important steps. Although the method is different from reverse spatial discretization (RSD) from subsection 2.2, we note that the resulting equations are quite similar.
We start by defining the density
$ ˆρ(x,t):=∑iδ(xi(t)−x),ˆq(x,t):=∑i˙xi(t)δ(xi(t)−x), $
|
(11) |
where
$ ρ(x,t):=∫Φ(x−x′,t−t′)ˆρ(x′,t′)dx′dt′,q(x,t):=∫Φ(x−x′,t−t′)ˆq(x′,t′)dx′dt′ $
|
(12) |
by applying the coarse graining envelope function
$ ∂∂tρ(x,t)+∂∂xq(x,t)=0∂∂tq(x,t)=ρ(x,t)⟨¨xi(t′)⟩(x,t)−∂∂x[ρ(x,t)⟨˙x2i(t′)⟩(x,t)] $
|
(13) |
with the bracketed average of a quantity
$ \langle f_i(x', t')\rangle_{(x, t)} = \frac{1}{\rho(x, t)}\int\Phi(x-x', t-t')\sum\limits_i f_i(x', t')\delta(x_i(t')-x')\text{d}x'\text{d}t'. $ |
By introducing
$ ρ(x,t)(∂∂tv(x,t)+v(x,t)∂∂xv(x,t))=ρ(x,t)⟨¨xi(t′)⟩(x,t)−∂∂x(ρ(x,t)Θ(x,t)). $
|
(14) |
The first equation for the density is obviously the same as in (10) and the derivation so far is independent on microscopic dynamics. However, the second equation for the momentum involves a term
Let us first consider the general case of a car-following model of the form
$ \ddot{x}_i(t) = B(\Delta x_i, \Delta \dot{x}_i, \dot{x}_i). $ |
Then coarse graining leads to the approximated momentum equation
$ \frac{\partial}{\partial t}v(x, t)+v(x, t)\frac{\partial}{\partial x}v(x, t)\approx B(\langle\Delta x_i\rangle, \langle\Delta \dot{x}_i\rangle, v), $ |
where we further approximate
$ ⟨Δxi⟩≈ρ−1(x,t)+12ρ(x,t)∂∂xρ−1(x,t)+16ρ2(x,t)∂2∂x2ρ−1(x,t)⟨Δ˙xi⟩≈ρ−1(x,t)∂∂xv(x,t)+12ρ2(x,t)∂2∂x2v(x,t). $
|
Plugging in and expanding around
$ B(⟨Δxi⟩,⟨Δ˙xi⟩,v(x,t))≈B(ρ−1(x,t),0,v(x,t))+B1(12ρ(x,t)∂∂xρ−1(x,t))+B2(ρ−1(x,t)∂∂xv(x,t)), $
|
where
$ ∂tv(x,t)+v(x,t)∂xv(x,t)=B(ρ−1(x,t),0,v(x,t))+B12ρ(x,t)∂xρ−1(x,t)+B2ρ(x,t)∂xv(x,t). $
|
(15) |
We remark that it is also possible to include terms of higher order leading to higher order approximations as well as higher order derivatives. Since we are interested in delayed models driven by (3), we can identify
$ B(Δxi,Δ˙xi,˙xi)=CΔ˙xi(x,t−T)Δxi(x,t−T)γ+1B1=0,B2=Cρ(x,t−T)γ+1,B3=0. $
|
(16) |
The system of equations is then
$ ∂tτ(x,t)=∂xv(x,t)∂tv(x,t)+v(x,t)∂xv(x,t)=vrefρ(x,t−T)γ∂xv(x,t). $
|
(17) |
In conservative form, we may write the system of equations
$ ∂tρ(x,t)+∂x(ρ(x,t)v(x,t))=0∂t(ρ(x,t)w(x,t))+∂x′(ρ(x,t)v(x,t)w(x,t))=vref[∂xv(x,t)ρ(x,t−T)γ−∂xv(x,t)ρ(x,t)γ] $
|
(18) |
which is (up to the term
For completeness, we also state the Lagrangian representation of (18). If we use the inverse transformation from subsection 2.2 and write the equations dependent on
$ ∂tτ(x,t)=∂xv(x,t)∂tv(x,t)=vrefρ(x,t−T)γ+1∂xv(x,t). $
|
(19) |
The last approach we present is based on a Taylor expansion which was originally introduced in [30]. However, in contrast to the original approach, we derive a second order delayed model instead of a first order model only. Furthermore, we keep the explicit delay while the new model is derived from the microscopic level and do not apply a diffusive approximation.
We start by expanding the delayed variables position
$ x_i(t-T) = x_i(t)-T\dot{x}_i(t)+\mathcal O(T^2) $ |
and equivalently
$ v_i(t-T) = v_i(t)-T\dot{v}_i(t)+\mathcal O(T^2). $ |
By using the microscopic description (3) we can characterize the following delayed system:
$ xi(t−T)=xi(t)−Tvi(t)+O(T2)vi(t−T)=vi(t)−T(C(vi+1(t−T)−vi(t−T))(xi+1(t−T)−xi(t−T))γ+1)+O(T2),i=1,…,N. $
|
(20) |
The definition
$ \Delta x_i(t-T)\approx\Delta x_i(t)-T\Delta v_i(t) $ |
and
$ \Delta v_i(t-T)\approx \Delta v_i(t)-T \Big(C\frac{\Delta v_{i+1}(t-T)}{(\Delta x_{i+1}(t-T))^{\gamma+1}}-C\frac{\Delta v_i(t-T)}{(\Delta x_i(t-T))^{\gamma+1}}\Big). $ |
To derive macroscopic equations, we use again
$ \partial_t \tau(x, t) = \partial_x v(x, t), $ |
where
$ ∂tvi(t)=CΔvi(t−T)Δxi(t−T)γ+1≈C1(Δxi(t)−TΔvi(t))γ+1×(Δvi(t)−T[CΔvi+1(t−T)(Δxi+1(t−T))γ+1−CΔvi(t−T)(Δxi(t−T))γ+1])≈C11ρi(t)γ+1−(γ+1)1ρi(t)γTΔvi(t)×(Δvi(t)−CT(Δvi+1(t−T)(ρi+1(t−T))γ+1−Δvi(t−T)(ρi(t−T))γ+1)). $
|
For simplicity, we omit the terms
$ ∂tτ(x,t)=∂xv(x,t)∂tv(x,t)=vrefρ(x,t)γ+11−(γ+1)ρ(x,t)T∂xv(x,t)×∂x(v(x,t)−2vrefT(ρ(x,t−T)γ+1∂xv(x,t−T))) $
|
(21) |
in Lagrangian coordinates. However, the interpretation of the right-hand side is now different compared to (7) and (19).
By the use of Taylor expansion, correction terms enter the model. This can be interpreted as a correction towards the fact that drivers do not react immediately but after some delay. These correction terms are, as in the previous models, dependent on the product of density, the spatial derivative of speed and the (current and past) state of the traffic. Note that in the case we ignore the terms depending on
As already seen, the introduction of time delays lead to a right-hand side in the equation for the velocity that includes different states of traffic. This can be interpreted as an anticipation of the change in traffic based on the current and past state. Hence, we are able to model the drivers ability to 'extrapolate' the traffic situation to change his/her driving behavior.
Similar to the microscopic case, the introduction of explicit delays requires the extension of the initial data time span to ensure well-posedness. For all states
We now aim to discuss some key properties of the delayed macroscopic models. First, we show that for vanishing delays the models coincide with the classical ARZ model. In this context, we also refer to the weak solutions of the ARZ model and investigate the changes caused by the delayed case. Second, we comment on the positivity of solutions. Closely related to this discussion is the question of stability. We inverstigate the system of delayed differential equations (DDEs) resulting from discretizing in space and derive properties to ensure stable solutions.
Lemma 3.1. The macroscopic delayed models (7), (19) and (21) converge to the undelayed ARZ model for
Proof. The first equation of all proposed models is mass conservation and has no explicit dependence on the delay
Therefore, the velocity equation needs to be expressed in terms of
$ \partial_t w(x, t) = v_{\text{ref}}\Big(\partial_x v(x, t-T) \rho(x, t-T)^{\gamma+1}-\partial_x v(x, t)\rho(x, t)^{\gamma+1}\Big) $ |
and
$ \partial_t w(x, t) = v_{\text{ref}}\Big(\partial_x v(x, t) \rho(x, t-T)^{\gamma+1}-\partial_x v(x, t)\rho(x, t)^{\gamma+1}\Big), $ |
respectively. The velocity equation for the model derived by Taylor expansion (21) reads
$ ∂tw(x,t)=vref1ρ(x,t)γ+1−(γ+1)1ρ(x,t)γT∂xv(x,t)×(∂xv(x,t)−2vrefT∂x[ρ(x,t−T)γ+1∂xv(x,t−T)])−vref∂xv(x,t)ρ(x,t)γ+1. $
|
Then, in the limit
In this section, we discuss the solutions to Riemann problems for the ARZ model (1) and explain how the delayed models fit into this framework. The ARZ model is a system of conservation laws and we have three basic types of weak solutions to the Riemann problem, i.e. contact discontinuities, shocks and rarefaction waves, see [3,15] for an overview. We define
$ f=(fρfρw)=(ρvρwv). $
|
(22) |
For further investigations, we consider the ARZ model in the form
$ \partial_t u + \nabla f\partial_x u = 0, $ |
where
$ λ1=v−ρP(ρ),r1=(1w)λ2=v,r2=(1w+ρP′(ρ)) $
|
(23) |
with
Now, let us focus on the weak solutions to Riemann problems with left state
$ u(t,x)=u0(x−vlt). $
|
(24) |
For the delayed models, we do not necessarily get the same solution for
For the shock solution, we consider the Rankine Hugoniot condition
$ f(u_l)-f(u_r) = s(u_l-u_r). $ |
This gives for every state a curve which can be connected to the latter by a shock. The shock solution belongs to the genuine nonlinear eigenvalue. In the ARZ model, a fixed
$ u(t,x)=u0(x−st). $
|
(25) |
It remains unclear how the delayed models fit into this framework due to the missing definition of a flux function and hence the evaluation of the Rankine Hugoniot condition.
Similar to the shock solution, the rarefaction wave also belongs to the genuine nonlinear eigenvalue
$ u(t,x)={ulx<λ1(ul)tv(xt)λ1(ul)<x<λ1(ur)urx>λ1(ur)t, $
|
(26) |
where
To illustrate the behavior of approximate solutions to the delayed model, we refer to our numerical study in section 4, in particular figures 5, 6 and 7.
The early proposed second order models, i.e. the Payne-Whitham-model, suffered from the problem that in some Riemann problems the density and velocity could become negative. This was first pointed out by Daganzo [10] and the ARZ model has been developed to overcome this drawback. Unfortunately, the delayed models we have derived, converge certainly to the classical ARZ model, but might lose the positivity of
For the first approach (reverse spatial discretization) we have to analyze equation (7). We investigate the case that we have non-negative initial data and need to ensure that the solution remains non-negative. However, the solution would become negative in the case
$ v_{\text{ref}}\partial_x v(x, t-T) \rho(x, t-T)^{\gamma+1} < 0 $ |
with
For the coarse-graining approach (19) we have a different situation:
$ \partial_tv(x, t) = v_{\text{ref}}\rho(x, t-T)^{\gamma+1}\partial_xv(x, t). $ |
Since the term
The last approach, the Taylor model (21), would become negative if
$ \frac{v_{\text{ref}}}{\frac{1}{\rho(y, t)^{\gamma+1}}-(\gamma+1)\frac{1}{\rho(x, t)^{\gamma}}T\partial_x v(x, t)}(\partial_x v(x, t)-2v_{\text{ref}}T\partial_x[\rho(x, t-T)^{\gamma+1}\partial_x v(x, t-T)]) $ |
is smaller than
$ -\rho(x, t)^{\gamma+1}\partial_x[\rho(x, t-T)^{\gamma+1}\partial_x v(x, t-T)] < 0 $ |
and can conclude that the speed can become negative.
Remark 1. Negative velocities also appear in the microscopic model (2). This is the case when a driver is quite close to the vehicle in front and the driver in front suddenly reduces the velocity within the given delay time, see e.g. [21] where this effect is seen for a similar microscopic model. The non-physical solutions obtained in this way are avoided by the choice of our numerical experiments.
From theory we know the concept of linear stability for DDEs, see [25]. To apply this idea to the PDE-type delayed models we have derived, we need to discretize the equations in space. For the model based on reverse spatial discretization (7), we get for
$ ∂tτ(xi,t)=v(xi+1,t)−v(xi,t)Δx∂tv(xi,t)=vref1τ(xi,t−T)v(xi+1,t−T)−v(xi,t−T)Δx $
|
exploiting
$ ∂tτ(xi,t)=−v(xi,t)Δx∂tv(xi,t)=vref1τ∗−v(xi,t−T)Δx. $
|
Inserting the disturbed steady state, we get
$ ∂tη1(xi,t)=−η2(xi,t)Δx∂tη2(xi,t)=vref1τ∗−η2(xi,t−T)Δx. $
|
Since
$ η0,2λ2eλ2t=vref1τ∗−η0,2eλ2(t−T)Δx. $
|
This equation is equivalent to
$ λ2=vref1τ∗−e−λ2TΔx. $
|
This type of equation can be solved for
$ λ2=1TW(−Tvrefτ∗Δx), $
|
and hence the solution for
$ η1(t)=−η0,2Δxλ2eλ2t+C. $
|
This means, we have stability in a linear sense if
First, we have to introduce suitable discretization schemes for the microscopic and macroscopic delayed models. We make use of already well-established numerical methods for undelayed models and discuss how the delay term can be treated within this framework.
The microscopic model (2) is a system of DDEs. The numerical discretization of the delay term can be done with method of steps, cf. [5]. The concept behind this method is to separate the original initial history problem into several initial value problems stated by ordinary differential equations (ODE) and then apply standard schemes for ODEs.
To do so, we need to split our foreseen time interval into sub-intervals of smaller size such that the delay is still included. If we look at the first interval, we have all delayed values given by the initial history since the interval is smaller than the delay. Therefore, we can plug in the history and get a classical ODE for this first interval that needs to be solved numerically. For the second interval, we can plug in the history and the solution of the first interval for the delayed parts and get again an ODE. Iteratively, we use this strategy to solve the problem on the whole time interval. A good interpolation of the solution is necessary since we might need values in later iterations which do not correspond to the fixed time grid points.
We use the Matlab solver dde23 to simulate (2). This solver uses an explicit Runge-Kutta (2, 3) pair combined with the method of steps.
0https://de.mathworks.com/help/matlab/ref/dde23.html
For the comparison with the microscopic model, we use a method-of-lines like approach for the discretization of the delayed macroscopic model. We discretize the model in space to get a system of DDEs and apply a forward finite differences discretization to (7), (19) and (21) in Lagrangian coordinates as follows:
Discretization of (7):
$ ∂tτ(xi,t)=v(xi+1,t)−v(xi,t)Δx∂tv(xi,t)=vrefv(xi+1,t−T)−v(xi,t−T)Δxρ(xi,t−T)γ+1. $
|
Discretization of (19):
$ ∂tτ(xi,t)=v(xi+1,t)−v(xi,t)Δx∂tv(xi,t)=vrefv(xi+1,t)−v(xi,t)Δxρ(xi,t−T)γ+1. $
|
Discretization of (21):
$ ∂tτ(xi,t)=v(xi+1,t)−v(xi,t)Δx∂tv(xi,t)=vrefρ(xi,t)γ+11−(γ+1)ρ(x,t)Tv(xi+1,t)−v(xi,t)Δx×(v(xi+1,t)−2vrefTρ(xi+1,t−T)v(xi+2,t−T)−v(xi+1,t−T)ΔxΔx−v(xi,t)+2vrefTρ(xi,t−T)v(xi+1,t−T)−v(xi,t−T)ΔxΔx). $
|
The resulting system can be solved with the method of steps again using the Matlab solver dde23. All discretization procedures need an initial history function. For simplicity, we choose a constant history function with the value of the states at
We remark that the different numerical schemes are used because they showed to be better suited for the respective equation. Moreover, due to the potential instabilities, we can not decrease
The first numerical experiment we focus on is the numerical comparison between the microscopic model (2) and the different derivations of delayed macroscopic models called reverse spatial discretization (RSD) - eq. (7), coarse-graining (CG) and Taylor expansion (TE). Therefore, we present the following scenario: We consider a street with constant initial density in the macroscopic case which means initially uniformly distributed cars in the microscopic situation, i.e.
We simulate a time interval of 100s and the delay is
We see that the models resolve the vacuum solution well and are close to each other.
If we now zoom in space and consider different values for
5 | 1 | 0.5 | 0.1 | ||
RSD | 0.074 | 0.0759 | 0.0706 | 0.0335 | |
RSD | 0.038 | 0.0337 | 0.027 | 0.0288 | |
CG | 0.0727 | 0.0746 | 0.0692 | 0.0308 | |
CG | 0.036 | 0.0305 | 0.0236 | 0.0288 | |
TE | 0.0651 | 0.0683 | 0.0657 | 0.0486 | |
TE | 0.0223 | 0.0181 | 0.0236 | 0.0288 |
In section 3.2 we have seen that weak solutions to the classical ARZ model are hard to interpret for the delayed models. However, from a numerical point of view, a first idea of weak solutions to the delayed model can be given. Here, we focus on the numerical solutions to the RSD model and make comparisons to the three different types of Riemann solutions, see (24) - (26), on the same grid sizes. The results are given in figures 5, 6 and 7.
In a second experiment, we compare the delayed macroscopic models to the classical ARZ model for a delay of
Due to lemma 3.1, all delayed macroscopic models converge to the classical ARZ model, cf. Figure 8. However, the situation changes for a larger delay, e.g.
The third experiment compares our TE approach (21) to the first order convection-diffusion flow model (TCHS) derived in [30]. Since the TE approach is inspired by the derivation introduced in [30], we aim to point out how the two approaches differ regarding the explicit tracking of the delay, cf. discussion in subsection 2.4.
The model in [30] is given by
$ \partial_t\rho+\partial_x(\rho V(\rho)) = -T\partial_x((\rho V(\rho))^2\partial_x \rho) $ |
with
$ \partial_t\rho-\rho V(\rho)\partial_x\rho+\rho\partial_x(\rho V(\rho)) = -T\rho\partial_x((\rho V(\rho))^2\rho\partial_x \rho) $ |
This model is solved by a finite difference based method of lines discretization, where the ODE solver is a forward Euler method.
For our comparison, we consider a street with a smooth initial density profile such that we have a relatively high density in the beginning, i.e.
For the next numerical studies and applications, we introduce an alternative numerical discretization of the macroscopic models based on the Eulerian coordinates representation. This is useful since the scenarios we investigate are on a fixed road segment and so Eulerian coordinates are better suited to tackle the boundary conditions. To set up a suitable numerical scheme, we use known methods for hyperbolic partial differential (PDE) equations coupled to a splitting technique, cf. [23]. This means, considering for example the reverse spatial discretization (RSD), we split the model (10) into the classical ARZ model solved in a first step and an additional delayed part solved in a second step with a forward differences approach. We solve the hyperbolic PDE part with the Lax-Friedrichs method using the index
$ ρ(xj,t+Δt)=12(ρ(xj+1,t)+ρ(xj−1,t))−Δt2Δx(fρ(ρ(xj+1,t),ρw(xj+1,t))−fρ(ρ(xj−1,t),ρw(xj−1,t)))ρw∗(xj,t)12(ρw(xj+1,t)+ρw(xj−1,t))−Δt2Δx(fρw(ρ(xj+1,t),ρw(xj+1,t))−fρw(ρ(xj−1,t),ρw(xj−1,t)))ρw(xj,t+Δt)=vref×(ρw(xj+1,t−T)ρ(xj+1,t−T)−P(1ρ(xj+1,t−T))−ρw(xj−1,t−T)ρ(xj−1,t−T)+P(1ρ(xj−1,t−T))2Δxρ(xj,t−T)−ρw∗(xj+1,t)ρ(xj+1,t)−P(1ρ(xj+1,t))−ρw∗(xj−1,t)ρ(xj−1,t)+P(1ρ(xj−1,t))2Δxρ(xj,t)), $
|
where
Since the Lax-Friedrichs method is consistent, stable, monotone and
In the following, we introduce two examples to emphasize the impact of delayed macroscopic models in the case of real-world applications.
The first application we have in mind is a frequent traffic light switching. We intend to compare the model (10) to the classical ARZ model (1). The experimental setting is introduced in Figure 11, where at the end of the street, i.e. at
Initially, the density and the velocity are
We see that the presence of the delay term leads to phenomena which can not be observed in the classical ARZ model as for example the broader density plateaus to resolve the stop-and-go behavior. The difference of the two models can be also recognized for the speed
As a second application, we analyze the performance of the models regarding real-measured data. Therefore, we fit the classical (1) and delayed (10) model to real-life data and compare the numerical results.
We start to investigate the fundamental diagram, i.e. the relation between traffic density and traffic flow, for real data taken from the Minnesota Department of Transportation. The data shows a reverse lambda shape, see Figure 15. At low densities, there is a clear linear relation between flow and density. At medium densities however, we get a wide spread of flows, making a functional relation hard or even impossible to identify. We remark that in the classical ARZ model (1) the artificial variable
0http://data.dot.state.mn.us/datatools/
In a next step, we follow the approach in [14] to fit the classical and RSD model to this data. We use data collected by the Regional Transportation Management Center, a division of the Minnesota Department of Transportation (RTMC data), in the same way as described in [14] and approximate the relation between density
$ Q(ρ)=α[√1+(λp)2+(√1+(λ(1−p))2−√1+(λp)2)ρρmax−√1+λ2(ρρmax−p)2] $
|
and the resulting solution is plotted in Figure 15.
From
$ ∂t1ρ(x,t)=∂xv(x,t)∂tv(x,t)=ρ(x,t−T)2P′(ρ(x,t−T))∂xv(x,t−T). $
|
(27) |
Now, we test the data-fitted model (27) with RTMC data to check the performance. We follow again [14] and take a segment of street with no on- or off-ramps and three measurement stations. The first and the third station deliver the boundary values for our simulation, while the station in the middle is the reference point, i.e. we will compare the simulation results with the measured data of the second station. So we simulate the segment of road, use the real data as boundary values and get a simulated traffic profile. This profile we compare at the point of the second station with the real data. For a time interval of 600 seconds, i.e. 16:00-16:10 on a workday, the results are plotted in Figure 16 for the fitted delayed and undelayed ARZ model. The images represent the whole time sequence at the reference station in the middle of the segment.
It seems that the undelayed ARZ model tends to avoid deviations while the delayed model has stronger peaks. Beyond that, both models seem to fit the flow better than the density, but this might be due to the specific example. For further discussions on the data-fitted classical ARZ model, which might be useful for this kind of experiment, we refer to [14] and [13].
Instead, let us look at the error between the real data and the simulated the models. We therefore make use of the error defined in [14] which is
$ E(x, t) = \frac{|\rho_{\text{model}}(x, t)-\rho_{\text{data}}(x, t)|}{\Delta\rho}+\frac{|v_{\text{model}}(x, t)-v_{\text{data}}(x, t)|}{\Delta v} $ |
for every position
$ E = \frac{1}{T}\int_0^TE(x, t)\text{d}t. $ |
The normalization factors
$ \epsilon = 4\frac{\text{E}_{\text{undel}}-\text{E}_{\text{del}}}{(\text{E}_{\text{undel}}+\text{E}_{\text{del}})^2} $ |
where
Summarizing, we have derived a new type of macroscopic second order traffic models including a delay from a microscopic follow-the-leader model in three different ways. The proposed approaches are adapted from well-established models known for the derivation of undelayed macroscopic traffic flow models, i.e. reverse spatial discretization, coarse graining and Taylor expansion. We have also suggested different methods to numerically tackle the resulting equations. The numerical results show that the delayed macroscopic equations lead to reasonable results, in particular in comparison with the classical ARZ model.
Future work includes further theoretical investigations for the delayed PDEs that have been already established for related problems, see for example [1,2] and [31]. Furthermore, deeper investigations on suitable numerical methods for this kind of delayed equations are necessary. From an application point of view, an extension to traffic networks would be interesting.
[1] | Emde A, Hornstein E (2014) miRNAs at the interface of cellular stress and disease. Embo J 33: 1428-1437. |
[2] | Harries LW (2014) MicroRNAs as Mediators of the Ageing Process. Genes (Basel) 5: 656-670. |
[3] |
Chen Z, Trotman LC, Shaffer D, et al. (2005) Crucial role of p53-dependent cellular senescence in suppression of Pten-deficient tumorigenesis. Nature 436: 725-730. doi: 10.1038/nature03918
![]() |
[4] |
Galluzzi L, Bravo-San Pedro JM, Kroemer G (2014) Organelle-specific initiation of cell death. Nat Cell Biol 16: 728-736. doi: 10.1038/ncb3005
![]() |
[5] | Hung CW, Chen YC, Hsieh WL, et al. (2010) Ageing and neurodegenerative diseases. Ageing Res Rev 9 Suppl 1: S36-46. |
[6] |
Rodier F, Campisi J (2011) Four faces of cellular senescence. J Cell Biol 192: 547-556. doi: 10.1083/jcb.201009094
![]() |
[7] | Campisi J, Andersen JK, Kapahi P, et al. (2011) Cellular senescence: a link between cancer and age-related degenerative disease? Semin Cancer Biol 21: 354-359. |
[8] |
Coppé JP, Desprez PY, Krtolica A, et al. (2010) The senescence-associated secretory phenotype: the dark side of tumor suppression. Annu Rev Pathol 5: 99-118. doi: 10.1146/annurev-pathol-121808-102144
![]() |
[9] |
Lindsay J, Laurin D, Verreault R, et al. (2002) Risk factors for Alzheimer's disease: a prospective analysis from the Canadian Study of Health and Aging. Am J Epidemiol 156: 445-453. doi: 10.1093/aje/kwf074
![]() |
[10] |
Katz MJ, Lipton RB, Hall CB, et al. (2012) Age-specific and sex-specific prevalence and incidence of mild cognitive impairment, dementia, and Alzheimer dementia in blacks and whites: a report from the Einstein Aging Study. Alzheimer Dis Assoc Disord 26: 335-343. doi: 10.1097/WAD.0b013e31823dbcfc
![]() |
[11] |
Hindle JV (2010) Ageing, neurodegeneration and Parkinson's disease. Age Ageing 39: 156-161. doi: 10.1093/ageing/afp223
![]() |
[12] |
Van Den Eeden SK, Tanner CM, Bernstein AL, et al. (2003) Incidence of Parkinson's disease: variation by age, gender, and race/ethnicity. Am J Epidemiol 157: 1015-1022. doi: 10.1093/aje/kwg068
![]() |
[13] | Mehta P, Antao V, Kaye W, et al. (2014) Prevalence of amyotrophic lateral sclerosis - United States, 2010-2011. MMWR Surveill Summ 63 Suppl 7: 1-14. |
[14] | Kurtzke JF (1982) Epidemiology of amyotrophic lateral sclerosis. Adv Neurol 36: 281-302. |
[15] |
Choi JY, Morris JC, Hsu CY (1998) Aging and cerebrovascular disease. Neurol Clin 16: 687-711. doi: 10.1016/S0733-8619(05)70089-X
![]() |
[16] | Popa-Wagner A, Buga AM, Turner RC, et al. (2012) Cerebrovascular disorders: role of aging. J Aging Res 2012: 128146. |
[17] | Adalbert R, Coleman MP (2012) Axon pathology in age-related neurodegenerative disorders. Neuropathol Appl Neurobiol. |
[18] | Zlokovic BV (2011) Neurovascular pathways to neurodegeneration in Alzheimer's disease and other disorders. Nat Rev Neurosci 12: 723-738. |
[19] |
Chen RL, Kassem NA, Redzic ZB, et al. (2009) Age-related changes in choroid plexus and blood-cerebrospinal fluid barrier function in the sheep. Exp Gerontol 44: 289-296. doi: 10.1016/j.exger.2008.12.004
![]() |
[20] |
Mao P, Reddy PH (2011) Aging and amyloid beta-induced oxidative DNA damage and mitochondrial dysfunction in Alzheimer's disease: implications for early intervention and therapeutics. Biochim Biophys Acta 1812: 1359-1370. doi: 10.1016/j.bbadis.2011.08.005
![]() |
[21] | Castello MA, Soriano S (2014) On the origin of Alzheimer's disease. Trials and tribulations of the amyloid hypothesis. Ageing Res Rev 13: 10-12. |
[22] |
Herbig U, Ferreira M, Condel L, et al. (2006) Cellular senescence in aging primates. Science 311: 1257. doi: 10.1126/science.1122446
![]() |
[23] |
Jeyapalan JC, Sedivy JM (2008) Cellular senescence and organismal aging. Mech Ageing Dev 129: 467-474. doi: 10.1016/j.mad.2008.04.001
![]() |
[24] |
Bhat R, Crowe EP, Bitto A, et al. (2012) Astrocyte senescence as a component of Alzheimer's disease. PLoS One 7: e45069. doi: 10.1371/journal.pone.0045069
![]() |
[25] |
Dimri GP, Lee X, Basile G, et al. (1995) A biomarker that identifies senescent human cells in culture and in aging skin in vivo. Proc Natl Acad Sci U S A 92: 9363-9367. doi: 10.1073/pnas.92.20.9363
![]() |
[26] |
Sadallah S, Eken C, Schifferli JA (2011) Ectosomes as modulators of inflammation and immunity. Clin Exp Immunol 163: 26-32. doi: 10.1111/j.1365-2249.2010.04271.x
![]() |
[27] |
Weilner S, Schraml E, Redl H, et al. (2013) Secretion of microvesicular miRNAs in cellular and organismal aging. Exp Gerontol 48: 626-633. doi: 10.1016/j.exger.2012.11.017
![]() |
[28] |
Hayflick L (1965) The Limited in Vitro Lifetime of Human Diploid Cell Strains. Exp Cell Res 37: 614-636. doi: 10.1016/0014-4827(65)90211-9
![]() |
[29] |
Lee M, Lee JS (2014) Exploiting tumor cell senescence in anticancer therapy. BMB Rep 47: 51-59. doi: 10.5483/BMBRep.2014.47.2.005
![]() |
[30] |
Campisi J, di Fagagna FD (2007) Cellular senescence: when bad things happen to good cells. Nat Rev Mol Cell Biol 8: 729-740. doi: 10.1038/nrm2233
![]() |
[31] | Kurz DJ, Decary S, Hong Y, et al. (2000) Senescence-associated (beta)-galactosidase reflects an increase in lysosomal mass during replicative ageing of human endothelial cells. J Cell Sci 113 ( Pt 20): 3613-3622. |
[32] |
Lee BY, Han JA, Im JS, et al. (2006) Senescence-associated beta-galactosidase is lysosomal beta-galactosidase. Aging Cell 5: 187-195. doi: 10.1111/j.1474-9726.2006.00199.x
![]() |
[33] |
Campo-Trapero J, Cano-Sanchez J, Palacios-Sanchez B, et al. (2008) Cellular senescence in oral cancer and precancer and treatment implications: a review. Acta Oncol 47: 1464-1474. doi: 10.1080/02841860802183612
![]() |
[34] |
Collado M, Gil J, Efeyan A, et al. (2005) Tumour biology: senescence in premalignant tumours. Nature 436: 642. doi: 10.1038/436642a
![]() |
[35] |
Byun HO, Han NK, Lee HJ, et al. (2009) Cathepsin D and eukaryotic translation elongation factor 1 as promising markers of cellular senescence. Cancer Res 69: 4638-4647. doi: 10.1158/0008-5472.CAN-08-4042
![]() |
[36] |
Lopez-Otin C, Blasco MA, Partridge L, et al. (2013) The hallmarks of aging. Cell 153: 1194-1217. doi: 10.1016/j.cell.2013.05.039
![]() |
[37] |
Morrison JH, Hof PR (1997) Life and death of neurons in the aging brain. Science 278: 412-419. doi: 10.1126/science.278.5337.412
![]() |
[38] |
Ren JL, Pan JS, Lu YP, et al. (2009) Inflammatory signaling and cellular senescence. Cell Signal 21: 378-383. doi: 10.1016/j.cellsig.2008.10.011
![]() |
[39] |
Freund A, Orjalo AV, Desprez PY, et al. (2010) Inflammatory networks during cellular senescence: causes and consequences. Trends Mol Med 16: 238-246. doi: 10.1016/j.molmed.2010.03.003
![]() |
[40] |
Molofsky AV, Slutsky SG, Joseph NM, et al. (2006) Increasing p16INK4a expression decreases forebrain progenitors and neurogenesis during ageing. Nature 443: 448-452. doi: 10.1038/nature05091
![]() |
[41] |
Krouwer VJ, Hekking LH, Langelaar-Makkinje M, et al. (2012) Endothelial cell senescence is associated with disrupted cell-cell junctions and increased monolayer permeability. Vasc Cell 4: 12. doi: 10.1186/2045-824X-4-12
![]() |
[42] | Abbott NJ, Friedman A (2012) Overview and introduction: the blood-brain barrier in health and disease. Epilepsia 53 Suppl 6: 1-6. |
[43] |
Marques F, Sousa JC, Sousa N, et al. (2013) Blood-brain-barriers in aging and in Alzheimer's disease. Mol Neurodegener 8: 38. doi: 10.1186/1750-1326-8-38
![]() |
[44] |
Redzic ZB (2013) Studies on the human choroid plexus in vitro. Fluids Barriers CNS 10: 10. doi: 10.1186/2045-8118-10-10
![]() |
[45] | Klohs J, Rudin M, Shimshek DR, et al. (2014) Imaging of cerebrovascular pathology in animal models of Alzheimer's disease. Front Aging Neurosci 6: 32. |
[46] |
Jellinger KA (2002) Alzheimer disease and cerebrovascular pathology: an update. J Neural Transm 109: 813-836. doi: 10.1007/s007020200068
![]() |
[47] |
Carotenuto A, Rea R, Colucci L, et al. (2012) Late and early onset dementia: what is the role of vascular factors? A retrospective study. J Neurol Sci 322: 170-175. doi: 10.1016/j.jns.2012.07.066
![]() |
[48] |
Togo T, Akiyama H, Iseki E, et al. (2002) Occurrence of T cells in the brain of Alzheimer's disease and other neurological diseases. J Neuroimmunol 124: 83-92. doi: 10.1016/S0165-5728(01)00496-9
![]() |
[49] |
Lebson L, Nash K, Kamath S, et al. (2010) Trafficking CD11b-positive blood cells deliver therapeutic genes to the brain of amyloid-depositing transgenic mice. J Neurosci 30: 9651-9658. doi: 10.1523/JNEUROSCI.0329-10.2010
![]() |
[50] |
Stalder AK, Ermini F, Bondolfi L, et al. (2005) Invasion of hematopoietic cells into the brain of amyloid precursor protein transgenic mice. J Neurosci 25: 11125-11132. doi: 10.1523/JNEUROSCI.2545-05.2005
![]() |
[51] | Enciu AM, Gherghiceanu M, Popescu BO (2013) Triggers and effectors of oxidative stress at blood-brain barrier level: relevance for brain ageing and neurodegeneration. Oxid Med Cell Longev 2013: 297512. |
[52] |
Zhang R, Kadar T, Sirimanne E, et al. (2012) Age-related memory decline is associated with vascular and microglial degeneration in aged rats. Behav Brain Res 235: 210-217. doi: 10.1016/j.bbr.2012.08.002
![]() |
[53] |
Hawkes CA, Hartig W, Kacza J, et al. (2011) Perivascular drainage of solutes is impaired in the ageing mouse brain and in the presence of cerebral amyloid angiopathy. Acta Neuropathol 121: 431-443. doi: 10.1007/s00401-011-0801-7
![]() |
[54] |
Jantaratnotai N, Schwab C, Ryu JK, et al. (2010) Converging perturbed microvasculature and microglial clusters characterize Alzheimer disease brain. Curr Alzheimer Res 7: 625-636. doi: 10.2174/156720510793499039
![]() |
[55] |
Kovari E, Herrmann FR, Hof PR, et al. (2013) The relationship between cerebral amyloid angiopathy and cortical microinfarcts in brain ageing and Alzheimer's disease. Neuropathol Appl Neurobiol 39: 498-509. doi: 10.1111/nan.12003
![]() |
[56] |
Hartz AM, Bauer B, Soldner EL, et al. (2012) Amyloid-beta contributes to blood-brain barrier leakage in transgenic human amyloid precursor protein mice and in humans with cerebral amyloid angiopathy. Stroke 43: 514-523. doi: 10.1161/STROKEAHA.111.627562
![]() |
[57] |
Cardoso FL, Brites D, Brito MA (2010) Looking at the blood-brain barrier: molecular anatomy and possible investigation approaches. Brain Res Rev 64: 328-363. doi: 10.1016/j.brainresrev.2010.05.003
![]() |
[58] |
Sá-Pereira I, Brites D, Brito MA (2012) Neurovascular unit: a focus on pericytes. Mol Neurobiol 45: 327-347. doi: 10.1007/s12035-012-8244-2
![]() |
[59] |
Tsai PC, Liao YC, Wang YS, et al. (2013) Serum microRNA-21 and microRNA-221 as Potential Biomarkers for Cerebrovascular Disease. J Vasc Res 50: 346-354. doi: 10.1159/000351767
![]() |
[60] | Staszel T, Zapala B, Polus A, et al. (2011) Role of microRNAs in endothelial cell pathophysiology. Pol Arch Med Wewn 121: 361-366. |
[61] |
van Balkom BW, de Jong OG, Smits M, et al. (2013) Endothelial cells require miR-214 to secrete exosomes that suppress senescence and induce angiogenesis in human and mouse endothelial cells. Blood 121: 3997-4006, S3991-3915. doi: 10.1182/blood-2013-02-478925
![]() |
[62] |
Ungvari Z, Tucsek Z, Sosnowska D, et al. (2013) Aging-induced dysregulation of dicer1-dependent microRNA expression impairs angiogenic capacity of rat cerebromicrovascular endothelial cells. J Gerontol A Biol Sci Med Sci 68: 877-891. doi: 10.1093/gerona/gls242
![]() |
[63] |
Burke SN, Barnes CA (2006) Neural plasticity in the ageing brain. Nat Rev Neurosci 7: 30-40. doi: 10.1038/nrn1809
![]() |
[64] | Morrison JH, Baxter MG (2012) The ageing cortical synapse: hallmarks and implications for cognitive decline. Nat Rev Neurosci 13: 240-250. |
[65] |
Burke SN, Barnes CA (2010) Senescent synapses and hippocampal circuit dynamics. Trends Neurosci 33: 153-161. doi: 10.1016/j.tins.2009.12.003
![]() |
[66] |
Pakkenberg B, Gundersen HJ (1997) Neocortical neuron number in humans: effect of sex and age. J Comp Neurol 384: 312-320. doi: 10.1002/(SICI)1096-9861(19970728)384:2<312::AID-CNE10>3.0.CO;2-K
![]() |
[67] |
Arnold B, Cassady SJ, VanLaar VS, et al. (2011) Integrating multiple aspects of mitochondrial dynamics in neurons: age-related differences and dynamic changes in a chronic rotenone model. Neurobiol Dis 41: 189-200. doi: 10.1016/j.nbd.2010.09.006
![]() |
[68] |
Reitz C, Mayeux R (2014) Alzheimer disease: epidemiology, diagnostic criteria, risk factors and biomarkers. Biochem Pharmacol 88: 640-651. doi: 10.1016/j.bcp.2013.12.024
![]() |
[69] |
Grillo FW, Song S, Teles-Grilo Ruivo LM, et al. (2013) Increased axonal bouton dynamics in the aging mouse cortex. Proc Natl Acad Sci U S A 110: E1514-1523. doi: 10.1073/pnas.1218731110
![]() |
[70] |
Fabricius K, Jacobsen JS, Pakkenberg B (2013) Effect of age on neocortical brain cells in 90+ year old human females--a cell counting study. Neurobiol Aging 34: 91-99. doi: 10.1016/j.neurobiolaging.2012.06.009
![]() |
[71] |
Marner L, Nyengaard JR, Tang Y, et al. (2003) Marked loss of myelinated nerve fibers in the human brain with age. J Comp Neurol 462: 144-152. doi: 10.1002/cne.10714
![]() |
[72] |
Pertusa M, Garcia-Matas S, Rodriguez-Farre E, et al. (2007) Astrocytes aged in vitro show a decreased neuroprotective capacity. J Neurochem 101: 794-805. doi: 10.1111/j.1471-4159.2006.04369.x
![]() |
[73] |
Zlokovic BV (2008) The blood-brain barrier in health and chronic neurodegenerative disorders. Neuron 57: 178-201. doi: 10.1016/j.neuron.2008.01.003
![]() |
[74] |
Popescu BO, Toescu EC, Popescu LM, et al. (2009) Blood-brain barrier alterations in ageing and dementia. J Neurol Sci 283: 99-106. doi: 10.1016/j.jns.2009.02.321
![]() |
[75] | Bigagli E, Luceri C, Scartabelli T, et al. (2015) Long-term Neuroglial Cocultures as a Brain Aging Model: Hallmarks of Senescence, MicroRNA Expression Profiles, and Comparison With In Vivo Models. J Gerontol A Biol Sci Med Sci. |
[76] |
Loeffler DA, DeMaggio AJ, Juneau PL, et al. (1994) Effects of enhanced striatal dopamine turnover in vivo on glutathione oxidation. Clin Neuropharmacol 17: 370-379. doi: 10.1097/00002826-199408000-00009
![]() |
[77] |
Luo XG, Ding JQ, Chen SD (2010) Microglia in the aging brain: relevance to neurodegeneration. Mol Neurodegener 5: 12. doi: 10.1186/1750-1326-5-12
![]() |
[78] |
Damani MR, Zhao L, Fontainhas AM, et al. (2011) Age-related alterations in the dynamic behavior of microglia. Aging Cell 10: 263-276. doi: 10.1111/j.1474-9726.2010.00660.x
![]() |
[79] |
Sierra A, Gottfried-Blackmore AC, McEwen BS, et al. (2007) Microglia derived from aging mice exhibit an altered inflammatory profile. Glia 55: 412-424. doi: 10.1002/glia.20468
![]() |
[80] |
Streit WJ, Sammons NW, Kuhns AJ, et al. (2004) Dystrophic microglia in the aging human brain. Glia 45: 208-212. doi: 10.1002/glia.10319
![]() |
[81] |
Wasserman JK, Yang H, Schlichter LC (2008) Glial responses, neuron death and lesion resolution after intracerebral hemorrhage in young vs. aged rats. Eur J Neurosci 28: 1316-1328. doi: 10.1111/j.1460-9568.2008.06442.x
![]() |
[82] |
Streit WJ, Graeber MB (1993) Heterogeneity of microglial and perivascular cell populations: insights gained from the facial nucleus paradigm. Glia 7: 68-74. doi: 10.1002/glia.440070112
![]() |
[83] |
Streit WJ, Braak H, Xue QS, et al. (2009) Dystrophic (senescent) rather than activated microglial cells are associated with tau pathology and likely precede neurodegeneration in Alzheimer's disease. Acta Neuropathol 118: 475-485. doi: 10.1007/s00401-009-0556-6
![]() |
[84] |
Maezawa I, Zimin PI, Wulff H, et al. (2011) Amyloid-beta protein oligomer at low nanomolar concentrations activates microglia and induces microglial neurotoxicity. J Biol Chem 286: 3693-3706. doi: 10.1074/jbc.M110.135244
![]() |
[85] |
Williamson LL, Sholar PW, Mistry RS, et al. (2011) Microglia and memory: modulation by early-life infection. J Neurosci 31: 15511-15521. doi: 10.1523/JNEUROSCI.3688-11.2011
![]() |
[86] |
Varnum MM, Ikezu T (2012) The classification of microglial activation phenotypes on neurodegeneration and regeneration in Alzheimer's disease brain. Arch Immunol Ther Exp (Warsz) 60: 251-266. doi: 10.1007/s00005-012-0181-2
![]() |
[87] | Solito E, Sastre M (2012) Microglia function in Alzheimer's disease. Front Pharmacol 3: 14. |
[88] | Wojtera M, Sobow T, Kloszewska I, et al. (2012) Expression of immunohistochemical markers on microglia in Creutzfeldt-Jakob disease and Alzheimer's disease: morphometric study and review of the literature. Folia Neuropathol 50: 74-84. |
[89] |
Graeber MB, Streit WJ (2010) Microglia: biology and pathology. Acta Neuropathol 119: 89-105. doi: 10.1007/s00401-009-0622-0
![]() |
[90] | Njie EG, Boelen E, Stassen FR, et al. (2012) Ex vivo cultures of microglia from young and aged rodent brain reveal age-related changes in microglial function. Neurobiol Aging 33: 195 e191-112. |
[91] |
Weggen S, Eriksen JL, Das P, et al. (2001) A subset of NSAIDs lower amyloidogenic Abeta42 independently of cyclooxygenase activity. Nature 414: 212-216. doi: 10.1038/35102591
![]() |
[92] |
Martin BK, Szekely C, Brandt J, et al. (2008) Cognitive function over time in the Alzheimer's Disease Anti-inflammatory Prevention Trial (ADAPT): results of a randomized, controlled trial of naproxen and celecoxib. Arch Neurol 65: 896-905. doi: 10.1001/archneur.2008.65.7.nct70006
![]() |
[93] | Floden AM, Combs CK (2011) Microglia demonstrate age-dependent interaction with amyloid-beta fibrils. J Alzheimers Dis 25: 279-293. |
[94] | Caldeira C, Oliveira AF, Cunha C, et al. (2014) Microglia change from a reactive to an age-like phenotype with the time in culture. Front Cell Neurosci 8: 152. |
[95] |
Akbar AN, Henson SM (2011) Are senescence and exhaustion intertwined or unrelated processes that compromise immunity? Nat Rev Immunol 11: 289-295. doi: 10.1038/nri2959
![]() |
[96] | Augert A, D. B (2013) Immunosenescence and Senescence Immunosurveillance: One of the Possible Links Explaining the Cancer Incidence in Ageing Population. In: (Ed.) DWZ, editor. Senescence and Senescence-Related Disorders: InTech. pp. 87-111. |
[97] |
Currais A (2015) Ageing and inflammation - A central role for mitochondria in brain health and disease. Ageing Res Rev 21: 30-42. doi: 10.1016/j.arr.2015.02.001
![]() |
[98] |
Baylis D, Bartlett DB, Patel HP, et al. (2013) Understanding how we age: insights into inflammaging. Longev Healthspan 2: 8. doi: 10.1186/2046-2395-2-8
![]() |
[99] |
Olivieri F, Rippo MR, Monsurro V, et al. (2013) MicroRNAs linking inflamm-aging, cellular senescence and cancer. Ageing Res Rev 12: 1056-1068. doi: 10.1016/j.arr.2013.05.001
![]() |
[100] |
Sheridan GK, Murphy KJ (2013) Neuron-glia crosstalk in health and disease: fractalkine and CX3CR1 take centre stage. Open Biol 3: 130181. doi: 10.1098/rsob.130181
![]() |
[101] | Cuanalo-Contreras K, Mukherjee A, Soto C (2013) Role of protein misfolding and proteostasis deficiency in protein misfolding diseases and aging. Int J Cell Biol 2013: 638083. |
[102] |
Levine B, Kroemer G (2008) Autophagy in the pathogenesis of disease. Cell 132: 27-42. doi: 10.1016/j.cell.2007.12.018
![]() |
[103] |
Yamashima T (2013) Reconsider Alzheimer's disease by the‘calpain-cathepsin hypothesis’--a perspective review. Prog Neurobiol 105: 1-23. doi: 10.1016/j.pneurobio.2013.02.004
![]() |
[104] |
Guo JL, Lee VM (2014) Cell-to-cell transmission of pathogenic proteins in neurodegenerative diseases. Nat Med 20: 130-138. doi: 10.1038/nm.3457
![]() |
[105] | Baixauli F, Lopez-Otin C, Mittelbrunn M (2014) Exosomes and autophagy: coordinated mechanisms for the maintenance of cellular fitness. Front Immunol 5: 403. |
[106] |
Lipinski MM, Zheng B, Lu T, et al. (2010) Genome-wide analysis reveals mechanisms modulating autophagy in normal brain aging and in Alzheimer's disease. Proc Natl Acad Sci U S A 107: 14164-14169. doi: 10.1073/pnas.1009485107
![]() |
[107] |
Lehmann BD, Paine MS, Brooks AM, et al. (2008) Senescence-associated exosome release from human prostate cancer cells. Cancer Res 68: 7864-7871. doi: 10.1158/0008-5472.CAN-07-6538
![]() |
[108] |
Trifunovic A, Larsson NG (2008) Mitochondrial dysfunction as a cause of ageing. J Intern Med 263: 167-178. doi: 10.1111/j.1365-2796.2007.01905.x
![]() |
[109] | Cui H, Kong Y, Zhang H (2012) Oxidative stress, mitochondrial dysfunction, and aging. J Signal Transduct 2012: 646354. |
[110] | Chistiakov DA, Sobenin IA, Revin VV, et al. (2014) Mitochondrial aging and age-related dysfunction of mitochondria. Biomed Res Int 2014: 238463. |
[111] |
Twig G, Shirihai OS (2011) The interplay between mitochondrial dynamics and mitophagy. Antioxid Redox Signal 14: 1939-1951. doi: 10.1089/ars.2010.3779
![]() |
[112] | Palikaras K, Tavernarakis N (2012) Mitophagy in neurodegeneration and aging. Front Genet 3: 297. |
[113] |
Liu L, Sakakibara K, Chen Q, et al. (2014) Receptor-mediated mitophagy in yeast and mammalian systems. Cell Res 24: 787-795. doi: 10.1038/cr.2014.75
![]() |
[114] |
Zhang J (2013) Autophagy and Mitophagy in Cellular Damage Control. Redox Biol 1: 19-23. doi: 10.1016/j.redox.2012.11.008
![]() |
[115] |
Chao JF, Leung Y, Wang MF, et al. (2012) Nutraceuticals and their preventive or potential therapeutic value in Parkinson's disease. Nutr Rev 70: 373-386. doi: 10.1111/j.1753-4887.2012.00484.x
![]() |
[116] |
Thakurta IG, Chattopadhyay M, Ghosh A, et al. (2012) Dietary supplementation with N-acetyl cysteine, alpha-tocopherol and alpha-lipoic acid reduces the extent of oxidative stress and proinflammatory state in aged rat brain. Biogerontology 13: 479-488. doi: 10.1007/s10522-012-9392-5
![]() |
[117] | Navarro A, Bandez MJ, Lopez-Cepero JM, et al. (2011) High doses of vitamin E improve mitochondrial dysfunction in rat hippocampus and frontal cortex upon aging. Am J Physiol-Reg I 300: R827-R834. |
[118] |
Ames BN (2010) Optimal micronutrients delay mitochondrial decay and age-associated diseases. Mech Ageing Dev 131: 473-479. doi: 10.1016/j.mad.2010.04.005
![]() |
[119] |
Bossy-Wetzel E, Barsoum MJ, Godzik A, et al. (2003) Mitochondrial fission in apoptosis, neurodegeneration and aging. Curr Opin Cell Biol 15: 706-716. doi: 10.1016/j.ceb.2003.10.015
![]() |
[120] |
Tatsuta T, Langer T (2008) Quality control of mitochondria: protection against neurodegeneration and ageing. EMBO J 27: 306-314. doi: 10.1038/sj.emboj.7601972
![]() |
[121] |
Rubinsztein DC, Marino G, Kroemer G (2011) Autophagy and aging. Cell 146: 682-695. doi: 10.1016/j.cell.2011.07.030
![]() |
[122] |
Salminen A, Ojala J, Kaarniranta K (2011) Apoptosis and aging: increased resistance to apoptosis enhances the aging process. Cell Mol Life Sci 68: 1021-1031. doi: 10.1007/s00018-010-0597-y
![]() |
[123] |
Uittenbogaard M, Chiaramello A (2014) Mitochondrial biogenesis: a therapeutic target for neurodevelopmental disorders and neurodegenerative diseases. Curr Pharm Des 20: 5574-5593. doi: 10.2174/1381612820666140305224906
![]() |
[124] |
Onyango IG, Lu J, Rodova M, et al. (2010) Regulation of neuron mitochondrial biogenesis and relevance to brain health. Biochim Biophys Acta 1802: 228-234. doi: 10.1016/j.bbadis.2009.07.014
![]() |
[125] |
Xu R, Hu Q, Ma Q, et al. (2014) The protease Omi regulates mitochondrial biogenesis through the GSK3 beta/PGC-1 alpha pathway. Cell Death Dis 5: e1373. doi: 10.1038/cddis.2014.328
![]() |
[126] | Llorens-Martin M, Jurado J, Hernandez F, et al. (2014) GSK-3beta, a pivotal kinase in Alzheimer disease. Front Mol Neurosci 7: 46. |
[127] | Maixner DW, Weng HR (2013) The Role of Glycogen Synthase Kinase 3 Beta in Neuroinflammation and Pain. J Pharm Pharmacol (Los Angel) 1: 001. |
[128] |
Zhou X, Zhou J, Li X, et al. (2011) GSK-3beta inhibitors suppressed neuroinflammation in rat cortex by activating autophagy in ischemic brain injury. Biochem Biophys Res Commun 411: 271-275. doi: 10.1016/j.bbrc.2011.06.117
![]() |
[129] |
Nakanishi H (2003) Neuronal and microglial cathepsins in aging and age-related diseases. Ageing Res Rev 2: 367-381. doi: 10.1016/S1568-1637(03)00027-8
![]() |
[130] | Nixon RA (2000) A ""protease activation cascade"" in the pathogenesis of Alzheimer's disease. Ann N Y Acad Sci 924: 117-131. |
[131] |
Lee EB, Skovronsky DM, Abtahian F, et al. (2003) Secretion and intracellular generation of truncated Abeta in beta-site amyloid-beta precursor protein-cleaving enzyme expressing human neurons. J Biol Chem 278: 4458-4466. doi: 10.1074/jbc.M210105200
![]() |
[132] | Schechter I, Ziv E (2011) Cathepsins S, B and L with aminopeptidases display beta-secretase activity associated with the pathogenesis of Alzheimer's disease. Biol Chem 392: 555-569. |
[133] |
Zhang YW, Thompson R, Zhang H, et al. (2011) APP processing in Alzheimer's disease. Mol Brain 4: 3. doi: 10.1186/1756-6606-4-3
![]() |
[134] | Munger JS, Haass C, Lemere CA, et al. (1995) Lysosomal processing of amyloid precursor protein to A beta peptides: a distinct role for cathepsin S. Biochem J 311 (Pt 1): 299-305. |
[135] |
Turk V, Stoka V, Vasiljeva O, et al. (2012) Cysteine cathepsins: from structure, function and regulation to new frontiers. Biochim Biophys Acta 1824: 68-88. doi: 10.1016/j.bbapap.2011.10.002
![]() |
[136] |
Gan L, Ye S, Chu A, et al. (2004) Identification of cathepsin B as a mediator of neuronal death induced by Abeta-activated microglial cells using a functional genomics approach. J Biol Chem 279: 5565-5572. doi: 10.1074/jbc.M306183200
![]() |
[137] |
Petanceska S, Canoll P, Devi LA (1996) Expression of rat cathepsin S in phagocytic cells. J Biol Chem 271: 4403-4409. doi: 10.1074/jbc.271.8.4403
![]() |
[138] |
Guicciardi ME, Deussing J, Miyoshi H, et al. (2000) Cathepsin B contributes to TNF-alpha-mediated hepatocyte apoptosis by promoting mitochondrial release of cytochrome c. J Clin Invest 106: 1127-1137. doi: 10.1172/JCI9914
![]() |
[139] | Stoka V, Turk B, Schendel SL, et al. (2001) Lysosomal protease pathways to apoptosis. Cleavage of bid, not pro-caspases, is the most likely route. J Biol Chem 276: 3149-3157. |
[140] |
Boya P, Andreau K, Poncet D, et al. (2003) Lysosomal membrane permeabilization induces cell death in a mitochondrion-dependent fashion. J Exp Med 197: 1323-1334. doi: 10.1084/jem.20021952
![]() |
[141] |
Conus S, Perozzo R, Reinheckel T, et al. (2008) Caspase-8 is activated by cathepsin D initiating neutrophil apoptosis during the resolution of inflammation. J Exp Med 205: 685-698. doi: 10.1084/jem.20072152
![]() |
[142] |
Blomgran R, Zheng L, Stendahl O (2007) Cathepsin-cleaved Bid promotes apoptosis in human neutrophils via oxidative stress-induced lysosomal membrane permeabilization. J Leukoc Biol 81: 1213-1223. doi: 10.1189/jlb.0506359
![]() |
[143] | Lemere CA, Munger JS, Shi GP, et al. (1995) The lysosomal cysteine protease, cathepsin S, is increased in Alzheimer's disease and Down syndrome brain. An immunocytochemical study. Am J Pathol 146: 848-860. |
[144] |
Wendt W, Lubbert H, Stichel CC (2008) Upregulation of cathepsin S in the aging and pathological nervous system of mice. Brain Res 1232: 7-20. doi: 10.1016/j.brainres.2008.07.067
![]() |
[145] |
Barclay J, Clark AK, Ganju P, et al. (2007) Role of the cysteine protease cathepsin S in neuropathic hyperalgesia. Pain 130: 225-234. doi: 10.1016/j.pain.2006.11.017
![]() |
[146] |
Clark AK, Grist J, Al-Kashi A, et al. (2012) Spinal cathepsin S and fractalkine contribute to chronic pain in the collagen-induced arthritis model. Arthritis Rheum 64: 2038-2047. doi: 10.1002/art.34351
![]() |
[147] | Xu J, Wang H, Ding K, et al. (2013) Inhibition of cathepsin S produces neuroprotective effects after traumatic brain injury in mice. Mediators Inflamm 2013: 187873. |
[148] |
Clark AK, Yip PK, Malcangio M (2009) The liberation of fractalkine in the dorsal horn requires microglial cathepsin S. J Neurosci 29: 6945-6954. doi: 10.1523/JNEUROSCI.0828-09.2009
![]() |
[149] |
Clark AK, Malcangio M (2012) Microglial signalling mechanisms: Cathepsin S and Fractalkine. Exp Neurol 234: 283-292. doi: 10.1016/j.expneurol.2011.09.012
![]() |
[150] |
Clark AK, Yip PK, Grist J, et al. (2007) Inhibition of spinal microglial cathepsin S for the reversal of neuropathic pain. Proc Natl Acad Sci U S A 104: 10655-10660. doi: 10.1073/pnas.0610811104
![]() |
[151] |
Cribbs DH, Berchtold NC, Perreau V, et al. (2012) Extensive innate immune gene activation accompanies brain aging, increasing vulnerability to cognitive decline and neurodegeneration: a microarray study. J Neuroinflammation 9: 179. doi: 10.1186/1742-2094-9-179
![]() |
[152] |
Cho SH, Sun B, Zhou Y, et al. (2011) CX3CR1 protein signaling modulates microglial activation and protects against plaque-independent cognitive deficits in a mouse model of Alzheimer disease. J Biol Chem 286: 32713-32722. doi: 10.1074/jbc.M111.254268
![]() |
[153] |
Rogers JT, Morganti JM, Bachstetter AD, et al. (2011) CX3CR1 deficiency leads to impairment of hippocampal cognitive function and synaptic plasticity. J Neurosci 31: 16241-16250. doi: 10.1523/JNEUROSCI.3667-11.2011
![]() |
[154] |
Bachstetter AD, Morganti JM, Jernberg J, et al. (2011) Fractalkine and CX 3 CR1 regulate hippocampal neurogenesis in adult and aged rats. Neurobiol Aging 32: 2030-2044. doi: 10.1016/j.neurobiolaging.2009.11.022
![]() |
[155] |
Wynne AM, Henry CJ, Huang Y, et al. (2010) Protracted downregulation of CX3CR1 on microglia of aged mice after lipopolysaccharide challenge. Brain Behav Immun 24: 1190-1201. doi: 10.1016/j.bbi.2010.05.011
![]() |
[156] |
Kim TS, Lim HK, Lee JY, et al. (2008) Changes in the levels of plasma soluble fractalkine in patients with mild cognitive impairment and Alzheimer's disease. Neurosci Lett 436: 196-200. doi: 10.1016/j.neulet.2008.03.019
![]() |
[157] |
Lee S, Varvel NH, Konerth ME, et al. (2010) CX3CR1 deficiency alters microglial activation and reduces beta-amyloid deposition in two Alzheimer's disease mouse models. Am J Pathol 177: 2549-2562. doi: 10.2353/ajpath.2010.100265
![]() |
[158] |
Noda M, Doi Y, Liang J, et al. (2011) Fractalkine attenuates excito-neurotoxicity via microglial clearance of damaged neurons and antioxidant enzyme heme oxygenase-1 expression. J Biol Chem 286: 2308-2319. doi: 10.1074/jbc.M110.169839
![]() |
[159] |
Bolmont T, Haiss F, Eicke D, et al. (2008) Dynamics of the microglial/amyloid interaction indicate a role in plaque maintenance. J Neurosci 28: 4283-4292. doi: 10.1523/JNEUROSCI.4814-07.2008
![]() |
[160] | Mrak RE (2012) Microglia in Alzheimer brain: a neuropathological perspective. Int J Alzheimers Dis 2012: 165021. |
[161] |
Condello C, Yuan P, Schain A, et al. (2015) Microglia constitute a barrier that prevents neurotoxic protofibrillar Abeta42 hotspots around plaques. Nat Commun 6: 6176. doi: 10.1038/ncomms7176
![]() |
[162] |
Liu Z, Condello C, Schain A, et al. (2010) CX3CR1 in microglia regulates brain amyloid deposition through selective protofibrillar amyloid-beta phagocytosis. J Neurosci 30: 17091-17101. doi: 10.1523/JNEUROSCI.4403-10.2010
![]() |
[163] |
Li C, Zhao R, Gao K, et al. (2011) Astrocytes: implications for neuroinflammatory pathogenesis of Alzheimer's disease. Curr Alzheimer Res 8: 67-80. doi: 10.2174/156720511794604543
![]() |
[164] |
Zhao J, O'Connor T, Vassar R (2011) The contribution of activated astrocytes to Abeta production: implications for Alzheimer's disease pathogenesis. J Neuroinflammation 8: 150. doi: 10.1186/1742-2094-8-150
![]() |
[165] | Persengiev SP, Kondova, II, Bontrop RE (2012) The Impact of MicroRNAs on Brain Aging and Neurodegeneration. Curr Gerontol Geriatr Res 2012: 359369. |
[166] | Basaiawmoit RV, Rattan SIS (2010) Cellular stress and protein misfolding during aging. In: Bross P, Gregersen N, editors. Protein Misfolding and Cellular Stress in Disease and Aging Concepts and Protocols. Springer, New York: Humana Press. pp. 107-117. |
[167] | Brignull HR, Morley JF, Morimoto RI (2007) The stress of misfolded proteins: C. elegans models for neurodegenerative disease and aging. In: Csermely P, Laszlo V, editors. Advances in Experimental Medicine and Biology - Molecular Aspects of the Stress Response: Chaperones, Membranes and Networks. New York, U.S.A.: Landes Bioscience/Eurekah.com. |
[168] |
Hipp MS, Park SH, Hartl FU (2014) Proteostasis impairment in protein-misfolding and -aggregation diseases. Trends Cell Biol 24: 506-514. doi: 10.1016/j.tcb.2014.05.003
![]() |
[169] |
Morimoto RI (2008) Proteotoxic stress and inducible chaperone networks in neurodegenerative disease and aging. Genes Dev 22: 1427-1438. doi: 10.1101/gad.1657108
![]() |
[170] |
Soti C, Csermely P (2003) Aging and molecular chaperones. Exp Gerontol 38: 1037-1040. doi: 10.1016/S0531-5565(03)00185-2
![]() |
[171] |
Clos J, Westwood JT, Becker PB, et al. (1990) Molecular cloning and expression of a hexameric Drosophila heat shock factor subject to negative regulation. Cell 63: 1085-1097. doi: 10.1016/0092-8674(90)90511-C
![]() |
[172] |
Cuervo AM, Wong E (2014) Chaperone-mediated autophagy: roles in disease and aging. Cell Res 24: 92-104. doi: 10.1038/cr.2013.153
![]() |
[173] |
Vasudevan S, Tong Y, Steitz JA (2007) Switching from repression to activation: microRNAs can up-regulate translation. Science 318: 1931-1934. doi: 10.1126/science.1149460
![]() |
[174] |
Roberts TC (2014) The MicroRNA Biology of the Mammalian Nucleus. Mol Ther Nucleic Acids 3: e188. doi: 10.1038/mtna.2014.40
![]() |
[175] |
Jung HJ, Suh Y (2012) MicroRNA in Aging: From Discovery to Biology. Curr Genomics 13: 548-557. doi: 10.2174/138920212803251436
![]() |
[176] |
Smith-Vikos T, Slack FJ (2012) MicroRNAs and their roles in aging. J Cell Sci 125: 7-17. doi: 10.1242/jcs.099200
![]() |
[177] |
Inukai S, de Lencastre A, Turner M, et al. (2012) Novel microRNAs differentially expressed during aging in the mouse brain. PLoS One 7: e40028. doi: 10.1371/journal.pone.0040028
![]() |
[178] |
Zhang H, Yang H, Zhang C, et al. (2015) Investigation of microRNA expression in human serum during the aging process. J Gerontol A Biol Sci Med Sci 70: 102-109. doi: 10.1093/gerona/glu145
![]() |
[179] | Lehrbach NJ, Castro C, Murfitt KJ, et al. (2012) Post-developmental microRNA expression is required for normal physiology, and regulates aging in parallel to insulin/IGF-1 signaling in C. elegans. RNA 18: 2220-2235. |
[180] |
Jiang M, Xiang Y, Wang D, et al. (2012) Dysregulated expression of miR-146a contributes to age-related dysfunction of macrophages. Aging Cell 11: 29-40. doi: 10.1111/j.1474-9726.2011.00757.x
![]() |
[181] | Olivieri F, Rippo MR, Procopio AD, et al. (2013) Circulating inflamma-miRs in aging and age-related diseases. Front Genet 4: 121. |
[182] |
Fichtlscherer S, De Rosa S, Fox H, et al. (2010) Circulating microRNAs in patients with coronary artery disease. Circ Res 107: 677-684. doi: 10.1161/CIRCRESAHA.109.215566
![]() |
[183] |
Noren Hooten N, Abdelmohsen K, Gorospe M, et al. (2010) microRNA expression patterns reveal differential expression of target genes with age. PLoS One 5: e10724. doi: 10.1371/journal.pone.0010724
![]() |
[184] |
Ponomarev ED, Veremeyko T, Weiner HL (2013) MicroRNAs are universal regulators of differentiation, activation, and polarization of microglia and macrophages in normal and diseased CNS. Glia 61: 91-103. doi: 10.1002/glia.22363
![]() |
[185] |
Saba R, Gushue S, Huzarewich RL, et al. (2012) MicroRNA 146a (miR-146a) is over-expressed during prion disease and modulates the innate immune response and the microglial activation state. PLoS One 7: e30832. doi: 10.1371/journal.pone.0030832
![]() |
[186] |
Lukiw WJ (2007) Micro-RNA speciation in fetal, adult and Alzheimer's disease hippocampus. Neuroreport 18: 297-300. doi: 10.1097/WNR.0b013e3280148e8b
![]() |
[187] |
Sethi P, Lukiw WJ (2009) Micro-RNA abundance and stability in human brain: specific alterations in Alzheimer's disease temporal lobe neocortex. Neurosci Lett 459: 100-104. doi: 10.1016/j.neulet.2009.04.052
![]() |
[188] |
Hebert SS, Horre K, Nicolai L, et al. (2008) Loss of microRNA cluster miR-29a/b-1 in sporadic Alzheimer's disease correlates with increased BACE1/beta-secretase expression. Proc Natl Acad Sci U S A 105: 6415-6420. doi: 10.1073/pnas.0710263105
![]() |
[189] |
Guedes JR, Custodia CM, Silva RJ, et al. (2014) Early miR-155 upregulation contributes to neuroinflammation in Alzheimer's disease triple transgenic mouse model. Hum Mol Genet 23: 6286-6301. doi: 10.1093/hmg/ddu348
![]() |
[190] |
Janelsins MC, Mastrangelo MA, Oddo S, et al. (2005) Early correlation of microglial activation with enhanced tumor necrosis factor-alpha and monocyte chemoattractant protein-1 expression specifically within the entorhinal cortex of triple transgenic Alzheimer's disease mice. J Neuroinflammation 2: 23. doi: 10.1186/1742-2094-2-23
![]() |
[191] |
Provost P (2010) MicroRNAs as a molecular basis for mental retardation, Alzheimer's and prion diseases. Brain Res 1338: 58-66. doi: 10.1016/j.brainres.2010.03.069
![]() |
[192] |
Bang C, Thum T (2012) Exosomes: new players in cell-cell communication. Int J Biochem Cell Biol 44: 2060-2064. doi: 10.1016/j.biocel.2012.08.007
![]() |
[193] | Thery C, Regnault A, Garin J, et al. (1999) Molecular characterization of dendritic cell-derived exosomes. Selective accumulation of the heat shock protein hsc73. J Cell Biol 147: 599-610. |
[194] |
Llorente A, Skotland T, Sylvanne T, et al. (2013) Molecular lipidomics of exosomes released by PC-3 prostate cancer cells. Biochim Biophys Acta 1831: 1302-1309. doi: 10.1016/j.bbalip.2013.04.011
![]() |
[195] |
Valadi H, Ekstrom K, Bossios A, et al. (2007) Exosome-mediated transfer of mRNAs and microRNAs is a novel mechanism of genetic exchange between cells. Nat Cell Biol 9: 654-659. doi: 10.1038/ncb1596
![]() |
[196] |
Yoon YJ, Kim OY, Gho YS (2014) Extracellular vesicles as emerging intercellular communicasomes. BMB Rep 47: 531-539. doi: 10.5483/BMBRep.2014.47.10.164
![]() |
[197] |
Pan BT, Teng K, Wu C, et al. (1985) Electron microscopic evidence for externalization of the transferrin receptor in vesicular form in sheep reticulocytes. J Cell Biol 101: 942-948. doi: 10.1083/jcb.101.3.942
![]() |
[198] |
Lee Y, El Andaloussi S, Wood MJ (2012) Exosomes and microvesicles: extracellular vesicles for genetic information transfer and gene therapy. Hum Mol Genet 21: R125-134. doi: 10.1093/hmg/dds317
![]() |
[199] |
Kastelowitz N, Yin H (2014) Exosomes and microvesicles: identification and targeting by particle size and lipid chemical probes. Chembiochem 15: 923-928. doi: 10.1002/cbic.201400043
![]() |
[200] | Villarroya-Beltri C, Gutierrez-Vazquez C, Sanchez-Cabo F, et al. (2013) Sumoylated hnRNPA2B1 controls the sorting of miRNAs into exosomes through binding to specific motifs. Nat Commun 4: 2980. |
[201] |
Cocucci E, Meldolesi J (2015) Ectosomes and exosomes: shedding the confusion between extracellular vesicles. Trends Cell Biol 25: 364-372. doi: 10.1016/j.tcb.2015.01.004
![]() |
[202] | Choi DS, Kim DK, Kim YK, et al. (2014) Proteomics of extracellular vesicles: Exosomes and ectosomes. Mass Spectrom Rev 34: 474-490. |
[203] |
Cao J (2014) The functional role of long non-coding RNAs and epigenetics. Biol Proced Online 16: 11. doi: 10.1186/1480-9222-16-11
![]() |
[204] | Gezer U, Ozgur E, Cetinkaya M, et al. (2014) Long non-coding RNAs with low expression levels in cells are enriched in secreted exosomes. Cell Biol Int 38: 1076-1079. |
[205] |
Mittelbrunn M, Gutierrez-Vazquez C, Villarroya-Beltri C, et al. (2011) Unidirectional transfer of microRNA-loaded exosomes from T cells to antigen-presenting cells. Nat Commun 2: 282. doi: 10.1038/ncomms1285
![]() |
[206] |
Gupta A, Pulliam L (2014) Exosomes as mediators of neuroinflammation. J Neuroinflammation 11: 68. doi: 10.1186/1742-2094-11-68
![]() |
[207] |
Potolicchio I, Carven GJ, Xu X, et al. (2005) Proteomic analysis of microglia-derived exosomes: metabolic role of the aminopeptidase CD13 in neuropeptide catabolism. J Immunol 175: 2237-2243. doi: 10.4049/jimmunol.175.4.2237
![]() |
[208] | Turola E, Furlan R, Bianco F, et al. (2012) Microglial microvesicle secretion and intercellular signaling. Front Physiol 3: 149. |
[209] |
Danzer KM, Kranich LR, Ruf WP, et al. (2012) Exosomal cell-to-cell transmission of alpha synuclein oligomers. Mol Neurodegener 7: 42. doi: 10.1186/1750-1326-7-42
![]() |
[210] |
Banigan MG, Kao PF, Kozubek JA, et al. (2013) Differential expression of exosomal microRNAs in prefrontal cortices of schizophrenia and bipolar disorder patients. PLoS One 8: e48814. doi: 10.1371/journal.pone.0048814
![]() |
[211] |
Beveridge NJ, Cairns MJ (2012) MicroRNA dysregulation in schizophrenia. Neurobiol Dis 46: 263-271. doi: 10.1016/j.nbd.2011.12.029
![]() |
[212] | Mellios N, Sur M (2012) The Emerging Role of microRNAs in Schizophrenia and Autism Spectrum Disorders. Front Psychiatry 3: 39. |
[213] |
Mundalil Vasu M, Anitha A, Thanseem I, et al. (2014) Serum microRNA profiles in children with autism. Mol Autism 5: 40. doi: 10.1186/2040-2392-5-40
![]() |
[214] |
Dujardin S, Begard S, Caillierez R, et al. (2014) Ectosomes: a new mechanism for non-exosomal secretion of tau protein. PLoS One 9: e100760. doi: 10.1371/journal.pone.0100760
![]() |
[215] |
Rajendran L, Honsho M, Zahn TR, et al. (2006) Alzheimer's disease beta-amyloid peptides are released in association with exosomes. Proc Natl Acad Sci U S A 103: 11172-11177. doi: 10.1073/pnas.0603838103
![]() |
[216] |
Yuyama K, Sun H, Mitsutake S, et al. (2012) Sphingolipid-modulated exosome secretion promotes clearance of amyloid-beta by microglia. J Biol Chem 287: 10977-10989. doi: 10.1074/jbc.M111.324616
![]() |
[217] |
Yuyama K, Sun H, Usuki S, et al. (2015) A potential function for neuronal exosomes: sequestering intracerebral amyloid-beta peptide. FEBS Lett 589: 84-88. doi: 10.1016/j.febslet.2014.11.027
![]() |
[218] |
Joshi P, Turola E, Ruiz A, et al. (2014) Microglia convert aggregated amyloid-beta into neurotoxic forms through the shedding of microvesicles. Cell Death Differ 21: 582-593. doi: 10.1038/cdd.2013.180
![]() |
[219] | Joshi P, Benussi L, Furlan R, et al. (2015) Extracellular Vesicles in Alzheimer's Disease: Friends or Foes? Focus on Abeta-Vesicle Interaction. Int J Mol Sci 16: 4800-4813. |
[220] |
Bahrini I, Song JH, Diez D, et al. (2015) Neuronal exosomes facilitate synaptic pruning by up-regulating complement factors in microglia. Sci Rep 5: 7989. doi: 10.1038/srep07989
![]() |
[221] |
Fitzner D, Schnaars M, van Rossum D, et al. (2011) Selective transfer of exosomes from oligodendrocytes to microglia by macropinocytosis. J Cell Sci 124: 447-458. doi: 10.1242/jcs.074088
![]() |
[222] |
Basso M, Pozzi S, Tortarolo M, et al. (2013) Mutant copper-zinc superoxide dismutase (SOD1) induces protein secretion pathway alterations and exosome release in astrocytes: implications for disease spreading and motor neuron pathology in amyotrophic lateral sclerosis. J Biol Chem 288: 15699-15711. doi: 10.1074/jbc.M112.425066
![]() |
[223] |
Grad LI, Yerbury JJ, Turner BJ, et al. (2014) Intercellular propagated misfolding of wild-type Cu/Zn superoxide dismutase occurs via exosome-dependent and -independent mechanisms. Proc Natl Acad Sci U S A 111: 3620-3625. doi: 10.1073/pnas.1312245111
![]() |
[224] |
Forsberg K, Jonsson PA, Andersen PM, et al. (2010) Novel antibodies reveal inclusions containing non-native SOD1 in sporadic ALS patients. PLoS One 5: e11552. doi: 10.1371/journal.pone.0011552
![]() |
[225] |
Xu D, Tahara H (2013) The role of exosomes and microRNAs in senescence and aging. Adv Drug Deliv Rev 65: 368-375. doi: 10.1016/j.addr.2012.07.010
![]() |
[226] |
Mitsuhashi M, Taub DD, Kapogiannis D, et al. (2013) Aging enhances release of exosomal cytokine mRNAs by Abeta1-42-stimulated macrophages. FASEB J 27: 5141-5150. doi: 10.1096/fj.13-238980
![]() |
[227] | Kawikova I, Askenase PW (2014) Diagnostic and therapeutic potentials of exosomes in CNS diseases. Brain Res [in press]. |
[228] |
Properzi F, Logozzi M, Fais S (2013) Exosomes: the future of biomarkers in medicine. Biomark Med 7: 769-778. doi: 10.2217/bmm.13.63
![]() |
[229] | Fiandaca MS, Kapogiannis D, Mapstone M, et al. (2014) Identification of preclinical Alzheimer's disease by a profile of pathogenic proteins in neurally derived blood exosomes: A case-control study. Alzheimers Dement [in press]. |
[230] | Cheng L, Doecke JD, Sharples RA, et al. (2014) Prognostic serum miRNA biomarkers associated with Alzheimer's disease shows concordance with neuropsychological and neuroimaging assessment. Mol Psychiatry [in press]. |
[231] | Liu CG, Song J, Zhang YQ, et al. (2014) MicroRNA-193b is a regulator of amyloid precursor protein in the blood and cerebrospinal fluid derived exosomal microRNA-193b is a biomarker of Alzheimer's disease. Mol Med Rep 10: 2395-2400. |
[232] | Prendecki M, Dorszewska J (2014) The Role of MicroRNA in the Pathogenesis and Diagnosis of Neurodegenerative Diseases. Austin Alzheimers J Parkinsons Dis 1: 10. |
[233] | Lin J, Li J, Huang B, et al. (2015) Exosomes: novel biomarkers for clinical diagnosis. ScientificWorldJournal 2015: 657086. |
[234] | Cheng L, Sharples RA, Scicluna BJ, et al. (2014) Exosomes provide a protective and enriched source of miRNA for biomarker profiling compared to intracellular and cell-free blood. J Extracell Vesicles 3. |
[235] |
Kalani A, Tyagi A, Tyagi N (2014) Exosomes: mediators of neurodegeneration, neuroprotection and therapeutics. Mol Neurobiol 49: 590-600. doi: 10.1007/s12035-013-8544-1
![]() |
[236] |
Lai RC, Yeo RW, Tan KH, et al. (2013) Exosomes for drug delivery - a novel application for the mesenchymal stem cell. Biotechnol Adv 31: 543-551. doi: 10.1016/j.biotechadv.2012.08.008
![]() |
[237] |
Limke TL, Rao MS (2003) Neural stem cell therapy in the aging brain: pitfalls and possibilities. J Hematother Stem Cell Res 12: 615-623. doi: 10.1089/15258160360732641
![]() |
[238] | Wong WT (2013) Microglial aging in the healthy CNS: phenotypes, drivers, and rejuvenation. Front Cell Neurosci 7: 22. |
[239] |
Lapasset L, Milhavet O, Prieur A, et al. (2011) Rejuvenating senescent and centenarian human cells by reprogramming through the pluripotent state. Genes Dev 25: 2248-2253. doi: 10.1101/gad.173922.111
![]() |
[240] | Manukyan M, Singh PB (2014) Epigenome rejuvenation: HP1beta mobility as a measure of pluripotent and senescent chromatin ground states. Sci Rep 4: 4789. |
[241] |
Lunn JS, Sakowski SA, Hur J, et al. (2011) Stem cell technology for neurodegenerative diseases. Ann Neurol 70: 353-361. doi: 10.1002/ana.22487
![]() |
[242] |
Mattson MP, Chan SL, Duan W (2002) Modification of brain aging and neurodegenerative disorders by genes, diet, and behavior. Physiol Rev 82: 637-672. doi: 10.1152/physrev.00004.2002
![]() |
[243] |
Dantuma E, Merchant S, Sugaya K (2010) Stem cells for the treatment of neurodegenerative diseases. Stem Cell Res Ther 1: 37. doi: 10.1186/scrt37
![]() |
[244] | Thomsen GM, Gowing G, Svendsen S, et al. (2014) The past, present and future of stem cell clinical trials for ALS. Exp Neurol 262 Pt B: 127-137. |
[245] |
Sikora E (2013) Rejuvenation of senescent cells-the road to postponing human aging and age-related disease? Exp Gerontol 48: 661-666. doi: 10.1016/j.exger.2012.09.008
![]() |
[246] |
Miller RA, Harrison DE, Astle CM, et al. (2014) Rapamycin-mediated lifespan increase in mice is dose and sex dependent and metabolically distinct from dietary restriction. Aging Cell 13: 468-477. doi: 10.1111/acel.12194
![]() |
[247] |
Ehninger D, Neff F, Xie K (2014) Longevity, aging and rapamycin. Cell Mol Life Sci 71: 4325-4346. doi: 10.1007/s00018-014-1677-1
![]() |
[248] |
Saemann MD, Haidinger M, Hecking M, et al. (2009) The multifunctional role of mTOR in innate immunity: implications for transplant immunity. Am J Transplant 9: 2655-2661. doi: 10.1111/j.1600-6143.2009.02832.x
![]() |
[249] |
Soliman GA (2013) The role of mechanistic target of rapamycin (mTOR) complexes signaling in the immune responses. Nutrients 5: 2231-2257. doi: 10.3390/nu5062231
![]() |
[250] |
Olivieri F, Mazzanti I, Abbatecola AM, et al. (2012) Telomere/Telomerase system: a new target of statins pleiotropic effect? Curr Vasc Pharmacol 10: 216-224. doi: 10.2174/157016112799305076
![]() |
[251] |
Balu M, Sangeetha P, Haripriya D, et al. (2005) Rejuvenation of antioxidant system in central nervous system of aged rats by grape seed extract. Neurosci Lett 383: 295-300. doi: 10.1016/j.neulet.2005.04.042
![]() |
[252] |
Acosta S, Jernberg J, Sanberg CD, et al. (2010) NT-020, a natural therapeutic approach to optimize spatial memory performance and increase neural progenitor cell proliferation and decrease inflammation in the aged rat. Rejuvenation Res 13: 581-588. doi: 10.1089/rej.2009.1011
![]() |
[253] |
Prokopov AF (2010) A case of recovery from dementia following rejuvenative treatment. Rejuvenation Res 13: 217-219. doi: 10.1089/rej.2009.0947
![]() |
[254] |
Pitozzi V, Jacomelli M, Catelan D, et al. (2012) Long-term dietary extra-virgin olive oil rich in polyphenols reverses age-related dysfunctions in motor coordination and contextual memory in mice: role of oxidative stress. Rejuvenation Res 15: 601-612. doi: 10.1089/rej.2012.1346
![]() |
[255] | Gillette-Guyonnet S, Secher M, Vellas B (2013) Nutrition and neurodegeneration: epidemiological evidence and challenges for future research. Br J Clin Pharmacol 75: 738-755. |
[256] | Kidd PM (2005) Neurodegeneration from mitochondrial insufficiency: nutrients, stem cells, growth factors, and prospects for brain rebuilding using integrative management. Altern Med Rev 10: 268-293. |
[257] |
Maruszak A, Zekanowski C (2011) Mitochondrial dysfunction and Alzheimer's disease. Prog Neuropsychopharmacol Biol Psychiatry 35: 320-330. doi: 10.1016/j.pnpbp.2010.07.004
![]() |
[258] |
Safdar A, Bourgeois JM, Ogborn DI, et al. (2011) Endurance exercise rescues progeroid aging and induces systemic mitochondrial rejuvenation in mtDNA mutator mice. P Natl Acad Sci U S A 108: 4135-4140. doi: 10.1073/pnas.1019581108
![]() |
[259] |
Garcia-Matas S, Paul RK, Molina-Martinez P, et al. (2015) In vitro caloric restriction induces protective genes and functional rejuvenation in senescent SAMP8 astrocytes. Aging Cell 14: 334-344. doi: 10.1111/acel.12259
![]() |
[260] |
Suhr ST, Chang EA, Tjong J, et al. (2010) Mitochondrial rejuvenation after induced pluripotency. PLoS One 5: e14095. doi: 10.1371/journal.pone.0014095
![]() |
[261] | Masotti A, Celluzzi A, Petrini S, et al. (2014) Aged iPSCs display an uncommon mitochondrial appearance and fail to undergo in vitro neurogenesis. Aging (Albany NY) 6: 1094-1108. |
[262] |
Johansson JU, Woodling NS, Wang Q, et al. (2015) Prostaglandin signaling suppresses beneficial microglial function in Alzheimer's disease models. J Clin Invest 125: 350-364. doi: 10.1172/JCI77487
![]() |
[263] | Simard AR, Rivest S (2004) Bone marrow stem cells have the ability to populate the entire central nervous system into fully differentiated parenchymal microglia. Faseb J 18: 998-1000. |
[264] | Jung S, Schwartz M (2012) Non-identical twins - microglia and monocyte-derived macrophages in acute injury and autoimmune inflammation. Front Immunol 3: 89. |
[265] | London A, Cohen M, Schwartz M (2013) Microglia and monocyte-derived macrophages: functionally distinct populations that act in concert in CNS plasticity and repair. Front Cell Neurosci 7: 34. |
[266] | Danielyan L, Beer-Hammer S, Stolzing A, et al. (2014) Intranasal delivery of bone marrow-derived mesenchymal stem cells, macrophages, and microglia to the brain in mouse models of Alzheimer's and Parkinson's disease. Cell Transplant 23 Suppl 1: S123-139. |
[267] |
Le Blon D, Hoornaert C, Daans J, et al. (2014) Distinct spatial distribution of microglia and macrophages following mesenchymal stem cell implantation in mouse brain. Immunol Cell Biol 92: 650-658. doi: 10.1038/icb.2014.49
![]() |
[268] | Brites D, Vaz AR (2014) Microglia Centered Pathogenesis in ALS: Insights in Cell Interconnectivity. Front Cell Neurosci 8: 117. |
[269] |
Feldman EL, Boulis NM, Hur J, et al. (2014) Intraspinal neural stem cell transplantation in amyotrophic lateral sclerosis: phase 1 trial outcomes. Ann Neurol 75: 363-373. doi: 10.1002/ana.24113
![]() |
[270] | Mazzini L, Gelati M, Profico DC, et al. (2015) Human neural stem cell transplantation in ALS: initial results from a phase I trial. J Transl Med 13: 371. |
[271] |
Diaz-Amarilla P, Olivera-Bravo S, Trias E, et al. (2011) Phenotypically aberrant astrocytes that promote motoneuron damage in a model of inherited amyotrophic lateral sclerosis. Proc Natl Acad Sci U S A 108: 18126-18131. doi: 10.1073/pnas.1110689108
![]() |
[272] |
Meyer K, Ferraiuolo L, Miranda CJ, et al. (2014) Direct conversion of patient fibroblasts demonstrates non-cell autonomous toxicity of astrocytes to motor neurons in familial and sporadic ALS. Proc Natl Acad Sci U S A 111: 829-832. doi: 10.1073/pnas.1314085111
![]() |
[273] |
Kondo T, Funayama M, Tsukita K, et al. (2014) Focal transplantation of human iPSC-derived glial-rich neural progenitors improves lifespan of ALS mice. Stem Cell Reports 3: 242-249. doi: 10.1016/j.stemcr.2014.05.017
![]() |
[274] |
Sareen D, Gowing G, Sahabian A, et al. (2014) Human induced pluripotent stem cells are a novel source of neural progenitor cells (iNPCs) that migrate and integrate in the rodent spinal cord. J Comp Neurol 522: 2707-2728. doi: 10.1002/cne.23578
![]() |
[275] |
Drago D, Cossetti C, Iraci N, et al. (2013) The stem cell secretome and its role in brain repair. Biochimie 95: 2271-2285. doi: 10.1016/j.biochi.2013.06.020
![]() |
[276] | Haas C (2012) Strategies, development, and pitfalls of therapeutic options for Alzheimer's disease. J Alzheimers Dis 28: 241-281. |
[277] | Li XY, Bao XJ, Wang RZ (2015) Potential of neural stem cell-based therapies for Alzheimer's disease. J Neurosci Res [in press]. |
[278] |
Zhang W, Wang PJ, Sha HY, et al. (2014) Neural Stem Cell Transplants Improve Cognitive Function Without Altering Amyloid Pathology in an APP/PS1 Double Transgenic Model of Alzheimer's Disease. Mol Neurobiol 50: 423-437. doi: 10.1007/s12035-014-8640-x
![]() |
[279] |
Yan Y, Ma T, Gong K, et al. (2014) Adipose-derived mesenchymal stem cell transplantation promotes adult neurogenesis in the brains of Alzheimer's disease mice. Neural Regen Res 9: 798-805. doi: 10.4103/1673-5374.131596
![]() |
[280] | Services USDoHaH, National Plan to Address Alzheimer’s Disease: 2013 Update. 2013. Available from: http://aspe.hhs.gov/daltcp/napa/NatlPlan2013.pdf |
[281] |
Prince M, Bryce R, Albanese E, et al. (2013) The global prevalence of dementia: a systematic review and metaanalysis. Alzheimers Dement 9: 63-75 e62. doi: 10.1016/j.jalz.2012.11.007
![]() |
[282] |
Yang Z, Lin PJ, Levey A (2013) Monetary costs of dementia in the United States. N Engl J Med 369: 489. doi: 10.1056/NEJMc1305541
![]() |
[283] |
Hurd MD, Martorell P, Langa KM (2013) Monetary costs of dementia in the United States. N Engl J Med 369: 489-490. doi: 10.1056/NEJMc1305541
![]() |
[284] | Qiu C, Kivipelto M, von Strauss E (2009) Epidemiology of Alzheimer's disease: occurrence, determinants, and strategies toward intervention. Dialogues Clin Neurosci 11: 111-128. |
[285] |
Ruzankina Y, Brown EJ (2007) Relationships between stem cell exhaustion, tumour suppression and ageing. Brit J Cancer 97: 1189-1193. doi: 10.1038/sj.bjc.6604029
![]() |
[286] | Conover JC, Shook BA (2011) Aging of the subventricular zone neural stem cell niche. Aging Dis 2: 49-63. |
[287] | Artegiani B, Calegari F (2012) Age-related cognitive decline: can neural stem cells help us? Aging (Albany NY) 4: 176-186. |
[288] |
van Wijngaarden P, Franklin RJ (2013) Ageing stem and progenitor cells: implications for rejuvenation of the central nervous system. Development 140: 2562-2575. doi: 10.1242/dev.092262
![]() |
[289] | Howlett SE, Rockwood K (2014) Ageing: develop models of frailty. Nature 512: 253-253. |
[290] | Bowling A, Iliffe S (2006) Which model of successful ageing should be used? Baseline findings from a British longitudinal survey of ageing. Age Ageing 35: 607-614. |
[291] |
Bowling A, Dieppe P (2005) What is successful ageing and who should define it? Brit Med J 331: 1548-1551.292. doi: 10.1136/bmj.331.7531.1548
![]() |
[292] |
Vanhooren V, Libert C (2013) The mouse as a model organism in aging research: Usefulness, pitfalls and possibilities. Ageing Res Rev 12: 8-21. doi: 10.1016/j.arr.2012.03.010
![]() |
1. | Dora Brites, Adelaide Fernandes, Neuroinflammation and Depression: Microglia Activation, Extracellular Microvesicles and microRNA Dysregulation, 2015, 9, 1662-5102, 10.3389/fncel.2015.00476 | |
2. | Tao Wang, Yan Yuan, Hui Zou, Jinlong Yang, Shiwen Zhao, Yonggang Ma, Yi Wang, Jianchun Bian, Xuezhong Liu, Jianhong Gu, Zongping Liu, Jiaqiao Zhu, The ER stress regulator Bip mediates cadmium-induced autophagy and neuronal senescence, 2016, 6, 2045-2322, 10.1038/srep38091 | |
3. | Dora Brites, Regulatory function of microRNAs in microglia , 2020, 68, 0894-1491, 1631, 10.1002/glia.23846 | |
4. | Fabíola de Carvalho Chaves de Siqueira Mendes, Luisa Taynah Vasconcelos Barbosa Paixão, Daniel Guerreiro Diniz, Daniel Clive Anthony, Dora Brites, Cristovam Wanderley Picanço Diniz, Marcia Consentino Kronka Sosthenes, Sedentary Life and Reduced Mastication Impair Spatial Learning and Memory and Differentially Affect Dentate Gyrus Astrocyte Subtypes in the Aged Mice, 2021, 15, 1662-453X, 10.3389/fnins.2021.632216 | |
5. | Amanda de Oliveira Ferreira Leite, João Bento Torres Neto, Renata Rodrigues dos Reis, Luciane Lobato Sobral, Aline Cristine Passos de Souza, Nonata Trévia, Roseane Borner de Oliveira, Nara Alves de Almeida Lins, Daniel Guerreiro Diniz, José Antonio Picanço Diniz, Pedro Fernando da Costa Vasconcelos, Daniel Clive Anthony, Dora Brites, Cristovam Wanderley Picanço Diniz, Unwanted Exacerbation of the Immune Response in Neurodegenerative Disease: A Time to Review the Impact, 2021, 15, 1662-5102, 10.3389/fncel.2021.749595 | |
6. | Julie Joseph, Benjamin Rahmani, Yonesha Cole, Neha Puttagunta, Edward Lin, Zafar K. Khan, Pooja Jain, Can Soluble Immune Checkpoint Molecules on Exosomes Mediate Inflammation?, 2022, 17, 1557-1890, 381, 10.1007/s11481-021-10018-3 | |
7. | Dario Carvalho-Paulo, João Bento Torres Neto, Carlos Santos Filho, Thais Cristina Galdino de Oliveira, Aline Andrade de Sousa, Renata Rodrigues dos Reis, Zaire Alves dos Santos, Camila Mendes de Lima, Marcus Augusto de Oliveira, Nivin Mazen Said, Sinara Franco Freitas, Marcia Consentino Kronka Sosthenes, Giovanni Freitas Gomes, Ediely Pereira Henrique, Patrick Douglas Côrrea Pereira, Lucas Silva de Siqueira, Mauro André Damasceno de Melo, Cristovam Guerreiro Diniz, Nara Gyzely de Morais Magalhães, José Antonio Picanço Diniz, Pedro Fernando da Costa Vasconcelos, Daniel Guerreiro Diniz, Daniel Clive Anthony, David Francis Sherry, Dora Brites, Cristovam Wanderley Picanço Diniz, Microglial Morphology Across Distantly Related Species: Phylogenetic, Environmental and Age Influences on Microglia Reactivity and Surveillance States, 2021, 12, 1664-3224, 10.3389/fimmu.2021.683026 |
5 | 1 | 0.5 | 0.1 | ||
RSD | 0.074 | 0.0759 | 0.0706 | 0.0335 | |
RSD | 0.038 | 0.0337 | 0.027 | 0.0288 | |
CG | 0.0727 | 0.0746 | 0.0692 | 0.0308 | |
CG | 0.036 | 0.0305 | 0.0236 | 0.0288 | |
TE | 0.0651 | 0.0683 | 0.0657 | 0.0486 | |
TE | 0.0223 | 0.0181 | 0.0236 | 0.0288 |
5 | 1 | 0.5 | 0.1 | ||
RSD | 0.074 | 0.0759 | 0.0706 | 0.0335 | |
RSD | 0.038 | 0.0337 | 0.027 | 0.0288 | |
CG | 0.0727 | 0.0746 | 0.0692 | 0.0308 | |
CG | 0.036 | 0.0305 | 0.0236 | 0.0288 | |
TE | 0.0651 | 0.0683 | 0.0657 | 0.0486 | |
TE | 0.0223 | 0.0181 | 0.0236 | 0.0288 |