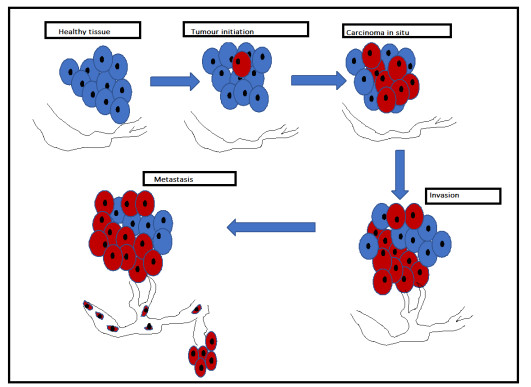
Cancer is a disease that arises from the uncontrolled growth of abnormal (tumor) cells in an organ and their subsequent spread into other parts of the body. If tumor cells spread to surrounding tissues or other organs, then the disease is life-threatening due to limited treatment options. This work applies an agent-based model to investigate the effect of intra-tumoral communication on tumor progression, plasticity, and invasion, with results suggesting that cell-cell and cell-extracellular matrix (ECM) interactions affect tumor cell behavior. Additionally, the model suggests that low initial healthy cell densities and ECM protein densities promote tumor progression, cell motility, and invasion. Furthermore, high ECM breakdown probabilities of tumor cells promote tumor invasion. Understanding the intra-tumoral communication under cellular stress can potentially lead to the design of successful treatment strategies for cancer.
Citation: Hasitha N. Weerasinghe, Pamela M. Burrage, Dan V. Nicolau Jr., Kevin Burrage. Agent-based modeling for the tumor microenvironment (TME)[J]. Mathematical Biosciences and Engineering, 2024, 21(11): 7621-7647. doi: 10.3934/mbe.2024335
[1] | Donggu Lee, Sunju Oh, Sean Lawler, Yangjin Kim . Bistable dynamics of TAN-NK cells in tumor growth and control of radiotherapy-induced neutropenia in lung cancer treatment. Mathematical Biosciences and Engineering, 2025, 22(4): 744-809. doi: 10.3934/mbe.2025028 |
[2] | Yi Shi, Xiaoqian Huang, Zhaolan Du, Jianjun Tan . Analysis of single-cell RNA-sequencing data identifies a hypoxic tumor subpopulation associated with poor prognosis in triple-negative breast cancer. Mathematical Biosciences and Engineering, 2022, 19(6): 5793-5812. doi: 10.3934/mbe.2022271 |
[3] | Chen Zheng, Zhaobang Tan . A novel identified pyroptosis-related prognostic signature of colorectal cancer. Mathematical Biosciences and Engineering, 2021, 18(6): 8783-8796. doi: 10.3934/mbe.2021433 |
[4] | Avner Friedman, Yangjin Kim . Tumor cells proliferation and migration under the influence of their microenvironment. Mathematical Biosciences and Engineering, 2011, 8(2): 371-383. doi: 10.3934/mbe.2011.8.371 |
[5] | Xin Lin, Xingyuan Li, Binqiang Ma, Lihua Hang . Identification of novel immunomodulators in lung squamous cell carcinoma based on transcriptomic data. Mathematical Biosciences and Engineering, 2022, 19(2): 1843-1860. doi: 10.3934/mbe.2022086 |
[6] | Yuan Yang, Lingshan Zhou, Xi Gou, Guozhi Wu, Ya Zheng, Min Liu, Zhaofeng Chen, Yuping Wang, Rui Ji, Qinghong Guo, Yongning Zhou . Comprehensive analysis to identify DNA damage response-related lncRNA pairs as a prognostic and therapeutic biomarker in gastric cancer. Mathematical Biosciences and Engineering, 2022, 19(1): 595-611. doi: 10.3934/mbe.2022026 |
[7] | Yu Jiang, Lijuan Lin, Huiming Lv, He Zhang, Lili Jiang, Fenfen Ma, Qiuyue Wang, Xue Ma, Shengjin Yu . Immune cell infiltration and immunotherapy in hepatocellular carcinoma. Mathematical Biosciences and Engineering, 2022, 19(7): 7178-7200. doi: 10.3934/mbe.2022339 |
[8] | Youshan Tao, J. Ignacio Tello . Nonlinear stability of a heterogeneous state in a PDE-ODE model for acid-mediated tumor invasion. Mathematical Biosciences and Engineering, 2016, 13(1): 193-207. doi: 10.3934/mbe.2016.13.193 |
[9] | Jing Liu, Xuefang Zhang, Ting Ye, Yongjian Dong, Wenfeng Zhang, Fenglin Wu, Huaben Bo, Hongwei Shao, Rongxin Zhang, Han Shen . Prognostic modeling of patients with metastatic melanoma based on tumor immune microenvironment characteristics. Mathematical Biosciences and Engineering, 2022, 19(2): 1448-1470. doi: 10.3934/mbe.2022067 |
[10] | Cristian Morales-Rodrigo . A therapy inactivating the tumor angiogenic factors. Mathematical Biosciences and Engineering, 2013, 10(1): 185-198. doi: 10.3934/mbe.2013.10.185 |
Cancer is a disease that arises from the uncontrolled growth of abnormal (tumor) cells in an organ and their subsequent spread into other parts of the body. If tumor cells spread to surrounding tissues or other organs, then the disease is life-threatening due to limited treatment options. This work applies an agent-based model to investigate the effect of intra-tumoral communication on tumor progression, plasticity, and invasion, with results suggesting that cell-cell and cell-extracellular matrix (ECM) interactions affect tumor cell behavior. Additionally, the model suggests that low initial healthy cell densities and ECM protein densities promote tumor progression, cell motility, and invasion. Furthermore, high ECM breakdown probabilities of tumor cells promote tumor invasion. Understanding the intra-tumoral communication under cellular stress can potentially lead to the design of successful treatment strategies for cancer.
Cancer is a disease that involves uncontrolled abnormal (tumor) cell growth. Due to this uncontrolled growth, the tumor cell density increases, which leads to a cell mass known as a tumor. The disease is usually incurable if the tumor cells spread to the surrounding tissues (invasion) or other organs (metastasis) (see Figure 1).
The tumor microenvironment (TME) is the environment that surrounds a tumor and consists of different types of cells such as cancer associated fibroblasts (CAF), cancer associated macrophages (CAM), T-cells, B-cells, pericytes, tumor cells, and the extracellular matrix (ECM) [1,2] (see Figure 2). Various components of the TME promote tumor progression, invasion, and metastasis [3,4,5,6,7,8]. Understanding how tumor cells adapt to their environment is one of the biggest challenges in developing successful cancer treatments. This research study seeks to understand the tumor cell behavior in the TME by investigating cell-cell and cell-ECM interactions through an agent-based model.
The physical barrier for all cells in the TME is the ECM. It contains angiogenic factors and chemokines, which give each tissue its tensile, comprehensive strength and elasticity [9]. Different proteins in the ECM make a surrounding membrane and an interstitial matrix [10,11,12,13]. Cell-ECM adhesion is most important for cell movement through the TME. ECM stiffness interrupts drug delivery and promotes metastasis [10,14]. When a tumor cell attaches to an ECM component, the tumor cells secrete collagenase proteases called matrix metalloproteinases (MMPs), which break down the ECM component [15,16,17,18]. The ECM stores pro-angiogenic growth factors that support the formation of new blood vessels close to the tumor cells [19,20].
Restricted nutrient or oxygen supply in the TME promotes resource competition between cells. Tumor cells follow different strategies such as scavenging ECM proteins via integrins, receptor-mediated albumin uptake, catabolism, macropinocytosis consumption of multiple components of the TME, and engulfment and degradation of entire live cells via entosis to increase their nutrient supply [21,22]. The metabolic reprogramming mechanism called the Warburg effect increases the energy of tumor cells more than healthy cells [23,24,25,26,27].
Cell competition can occur through the competition for nutrients or growth factors and the competition for space through mechanisms such as cell fitness sensing [28,29,30,31,32]. The fitness level of a cell determines the proliferation and apoptosis of a cell. Super competition is the process of eliminating healthy wild-type cells with tumor cells. Myelocytomatosis (MYC) protein and Yes-associated proteins (YAP) are two proteins that turn cells into so-called super competitors [33]. In MYC-mediated cell competition, the losers with a low level of MYC are killed by the winners with a high level of MYC [34]. Tumor cells with metabolic winner phenotypes compete with immune cells and escape from the TME [35]. Hence, cell competition promotes tumor progression by killing less fit cells in the TME.
An epithelial to mesenchymal transition (EMT) occurs due to the disruption of cell-cell adhesion and cellular polarity, remodeling of the cytoskeleton, and changes in the cell-matrix adhesion [3]. In the deleterious adaptation of cells, cell-cell adhesion can influence how cells either escape or remain restricted to certain domains [36,37,38,39].
Clinical experiments are time-consuming, expensive, and have issues capturing the system dynamics. However, some advantages of mathematical modeling are the ability to identify critical factors and the most sensitive parameters of a system, the ability to modify the model according to the objectives of the study, and the ability to predict the behavior of a system. Additionally, mathematical models can capture the dynamics of systems in time and space, thus enabling us to make sound analyses and predictions on which new treatment strategies can be developed.
Different mathematical models have been developed in the literature to analyze tumor growth, cancer metabolism, vasculature, tumor immunity, tumor microenvironment and heterogeneity, invasion, metastasis, and cancer treatments [40,41,42,43]. Basically, three modeling techniques have been used to understand cancer dynamics: discrete (agent-based), continuum, and hybrid. Agent-based models track and update individual cells according to a set of biological rules [44,45]. Through an agent-based model, one can link tumor heterogeneity to a cellular response. Furthermore, we can study how molecular signaling, cellular metabolism, mutation-induced phenotypic changes, angiogenesis, metastasis, immune response, and cancer treatments affect cancer progression [46,47]. Continuum models consider the tumor tissue as a continuous medium, and differential equations are used for modeling [48,49,50,51,52,53]. Diffusion models for tumors have been developed under different mechanisms such as negative feedback [54], discontinuous switches [55,56], non-uniformly distributed mitotic inhibitors [57,58], and through the effects of a necrotic core [59]. Compared to discrete models, it is easier to estimate parameters for continuum models based on laboratory experiments. On the other hand, some assumptions used in continuum modeling can be too simplistic. A hybrid model combines the benefits of discrete and continuous modeling and techniques and describes chemical reactions and tissue landscapes in a single model [60,61]. Hybrid or continuum-discrete models can bridge the gap between the cellular scale and the tumor scale. In the hybrid approach, tumor tissue is modeled, and contains discrete and continuum elements. Oxygen, nutrient, drug, growth factors, and certain tissue features are described as continuum fields, and cells are described as discrete elements.
Numerous agent-based models have been developed for cancer modeling, thereby incorporating a wide range of features such as proliferation, cytotoxic behaviors, mechanical pressure, cell death, cell movement, treatments, mutations, angiogenic factors, immune system response, and energy availability [18,46,62,63,64,65,66,67,68,69,70,71,72,73,74,75,76,77,78,79,80,81,82,83,84,85,86]. For instance, Lettort et al. introduced an open-source package called PhysiBoss [87,88], which integrated intracellular signaling via Boolean modeling (MaBoss) [89] with multicellular behavior through agent-based modelling (PhysiCell) [90]. This approach is particularly advantageous to study the responses of heterogeneous cell populations to treatments, mutation effects, and different modes of invasion or isomorphic morphogenesis events [91]. However, this model does not account for ECM interactions within the tumor microenvironment (TME).
Previously developed agent-based models often only consider a few features of the TME. In order to understand the role of the TME in a greater depth, we focus on the eight hallmarks in our model suggested by Hanahan and colleagues [92,93,94]. The key hallmarks are given as follows:
● Sustaining proliferative signaling: Tumor cells can continuously signal themselves to grow, thus bypassing the normal regulatory mechanisms.
● Evading growth suppressors: Tumor cells can evade the growth-suppressing signals that would normally inhibit uncontrolled cell division.
● Enabling replicative immortality: Tumor cells maintain their telomeres, thus allowing them to divide indefinitely, unlike healthy cells that have a limited number of divisions.
● Resisting cell death: Tumor cells develop mechanisms to avoid programmed cell death, enabling their survival and proliferation.
● Deregulating cellular energy: Tumor cells reprogram their energy production to fuel rapid growth and adapt to the tumor microenvironment.
● Avoiding immune destruction: Tumor cells develop mechanisms to evade immune detection and destruction.
● Inducing angiogenesis: Tumors can stimulate the growth of new blood vessels to supply nutrients and oxygen, which are critical for their continued growth.
● Invasion and metastasis: Tumor cells can invade surrounding tissues and spread to distant organs, thus forming secondary tumors.
Figure 3 shows features of the model related to each cancer hallmark. In this study, we develop an agent-based model to study interactions between the tumor cells and the TME according to a set of biological rules. We emphasise that our model does not account for the formation of blood vessels. We acknowledge that the existence of blood vessels can play an important role in the tumor spread to the boundary of an organ and that the location of the tumor in the body is important; however, since we do not account for the blood vessel location, this important issue is beyond the scope of our model. Our aim is to keep the model simple but insightful. Furthermore, we observe the time that the first tumor cell exits the TME under various environmental conditions. This first cell exit time measures how long a tumor takes to spread to the surrounding tissues and other organs, and this information can help in the development of successful treatment strategies, as cancers are life-threatening when they invade or metastasize.
In Section 2, we describe the agent-based model that we developed to study the tumor cell behavior at the cellular level. Simulation results of this agent-based model are described in Section 3. Section 4 provides a discussion, and conclusions are provided in Section 5.
This model represents a two-dimensional tissue section that consists of healthy cells, tumor cells, and ECM proteins. The domain is a 100×100 grid with two regions (inner and outer regions). The outer region of the domain represents the invasive margin of the tissue. We assume that the domain is initially a healthy tissue (see Figure 4).
This tissue section develops into a tumor due to a series of mutations of a healthy cell. We assume that healthy cells are only located in the inner region and their movements are negligible as adhesion molecules keep the healthy cells in their intended locations [37,95]. We randomly locate ECM proteins in the domain and assume that they cannot move. Furthermore, tumor cells cannot occupy a grid position of an ECM protein. The boundary of the domain has no gaps, is said to be fully fenced at time zero, and represents the surrounding membrane of the tissue. The maximum population in the environment is 10,000, as we have a total of 10,000 grid positions in the domain.
The input parameters and the calculated output variables for the agent-based model are listed in Table 1.
Input parameter/Output variable | Description |
Healthy Cell Density (N(t)) | Division of the healthy cell population at time t by total of 10,000 grid positions |
Tumor Cell Density (A(t)) | Division of the tumor cell population at time t by total of 10,000 grid positions |
Cell Stickiness (Si) | Stickiness value of cell i to stick with neighboring cells |
Change in Stickiness (μs) | When a cell mutates, the stickiness of that cell is a uniformly distributed value between Sm−μs and Sm+μs, where Sm is the stickiness of the mother cell |
Jump Radius (Ji) | Measures cell movement and represents the number of steps a cell can move at a time |
Change in Jump Radius (μj) | When a cell mutates, the Jump Radius of that cell is a uniformly distributed value between Jm−μj and Jm+μj, where Jm is the jump radius of the mother cell |
Maximum Divisions of a Healthy Cell (K) | Healthy cells can divide only K number of times |
Number of Divided Times of a Cell (Di(t)) | The total number of divisions of cell i until time t. For a healthy cell, Di(t)≤K |
Cell Age (ai(t)) | The age of cell i at time t |
Division age (L) | A cell can divide when the age of that cell is greater than the division age |
Competition Rate (Ci) | If i is a healthy cell, then Ci=n/K, where n is the number of remaining cell divisions. If i is a tumor cell, then choose a random number Ci∈[0.5,1], as they are super-competitive. |
Probability of Dividing (Pd) | If the age of a cell reaches the cell division age, then Pd determines whether that cell divides or not at that time |
Probability of Death (Pa) | The chance of a cell death |
Probability of Cell Movement (Pm(i,t)) | If the stickiness of a cell i is S and the number of cells and obstacles in the neighborhood is n, then Pm(i,t)=(1−S)n |
Obstacle Density (R) | Division of the total number of ECM proteins by the total of 10,000 grid positions |
ECM Break Down Probability (Pb) | The chance that a tumor cell breaks down a fence component |
Number of Exit Cells (E(t)) | Total number of tumor cells that escaped the domain from time 0 to t |
Fence Density (f(t)) | Division of the number of ECM components at time t by the number of ECM components at time 0 |
Average Stickiness ˉS(t) | The average of the stickiness of the tumor cells at time t |
Average Jump Radius ˉJ(t) | The average of the jump radius of the tumor cells at time t |
This agent-based model observes the effect of different factors of the TME on tumor progression, plasticity, and invasion. The output variables N(t) and A(t) (healthy and tumor cell densities, respectively, at time t) measure tumor progression under different circumstances. We consider two output variables, ˉS(t) and ˉJ(t), to represent the effect of the tumor microenvironmental factors on the tumor cell plasticity. To measure the effect of different factors of the TME for tumor invasion, we use the two output variables, E(t) and f(t) - see Table 1.
Figure 5 represents the flow diagram of the model and we describe the set of rules associated with the agent-based model. We select a tumor cell in the domain and check the number of divisions of the cell. When a tumor cell has completed the maximum number of cell divisions of a healthy cell (K), it has a chance of dying. If a cell dies, then the grid position that the cell occupied becomes empty. If the cell's age is greater than the division age, then that cell has a chance to proliferate. When a cell proliferates, the daughter cell is placed in the first empty grid position in a clockwise direction starting from the North (using a Moore neighborhood). If all neighboring grid positions are occupied, then the mother cell competes with the weakest cell in the neighborhood determined by the competition rate, and that position is used by the daughter cell (see Figure 6). At any age, a cell can move to a new position depending on the number of cells and/or obstacles in the neighborhood and the cell stickiness. We assume that a tumor cell can move North, East, South or West (movement is restrained to a Von Neumann neighborhood). A detailed description of the model parameters, output variables, and rules is given in the Appendix.
The results of this agent-based model provide a good understanding of the individual tumor cell behavior under different conditions of the TME. Through this study, we can attempt to identify the most influential tumor microenvironmental factors that affect the tumor progression, cell plasticity, and invasion.
To study tumor cell behavior in the TME, we perform numerical simulations of the agent-based model using the MATLAB programming language. The relevant program codes can be accessed through https://github.com/Hasi1988/abm_for_TME. We observe the changes of the cell densities, average cell stickiness, average jump radii and fence densities with time. Furthermore, we observe the total number of tumor cells that exit from the domain. In this study, we also observe the effect of three factors on the TME: the initial healthy cell densities (N(0)), ECM protein densities (R) and ECM breakdown probabilities (Pb). We explore tumor progression, tumor plasticity, and tumor invasion under changes in each of these three factors. For each scenario, we perform 500 simulations. To investigate the effect of a factor, we change just that factor and keep the other parameter values, as in Table 2. For example, to investigate the effect of N(0), we only consider N(0)=0.243,0.324, and 0.405, while keeping R=0.1 and Pb=0.5. Other scenarios consider values of 0.25 and 0.75 as the ECM breakdown probabilities, and values of 0.05 and 0.2 as the obstacle densities.
Parameter | Value |
Initial healthy cell density (N(0)) | 0.324 |
ECM breakdown probability (Pb) | 0.5 |
Obstacle/Protein density (R) | 0.1 |
Healthy cell stickiness (Si) | 0.9 |
Change in stickiness (μs) | 0.1 |
Healthy cell jump radius (Ji) | 1 |
Change in jump radius (μj) | 1 |
Maximum number of cell divisions of healthy cells (K) | 10 |
Division age (L) | 20 |
Probability of Dividing (Pd) | 0.6 |
Probability of Death (Pa) | 0.001 |
We assume that the healthy cells are only located in the inner region that consists of 81×81 grid positions. We consider that the degradation of the ECM by a tumor cell can be equally likely to either occur or not to occur by assuming the ECM breakdown probability as 0.5. Since the protein density differs from tissue to tissue [96], our model assumes that the ECM protein density is 0.1. Due to adhesion molecules, the healthy cells have a high stickiness and a low motility [37]. Hence, we assume that the cell stickiness and jump radius of healthy cells are 0.9 and 1, respectively. In 1965, Hayflick suggested that healthy cells can only divide a limited number of times by observing the division times of human diploid fibroblast cells [97]. However, damage signals such as DNA-damaging agents and oxidative stress can affect the early cessation of the division of cells [98]. Since the TME is a hypoxic environment, we assume that a healthy cell can divide a maximum of 10 times. Our model does not account for the formation of blood vessels, so whether angiogenesis has occurred is not relevant for our model. We wish to keep our model as simple as possible. We consider a dimensionless time from 0 to 24 by dividing this into 1800 sub-intervals. Hence, the time step length is 0.0133(24/1800). We assume that the age of each cell increases by 1 at each time step and the division age of a cell is 20. Since tumor cells can proliferate uncontrollably, we assume a high probability rate (0.6) for cell division. Furthermore, we assume a significantly small probability of death (0.001) due to the resistance to death of tumor cells [93]. Since the complexity of the TME and the condition of the environment can differ from patient to patient, the parameter values can vary according to the condition of the TME.
Figure 7 represents the progression of a tumor in healthy tissue. With uncontrolled growth, the tumor cell density increases by creating a crowded environment. Due to the lack of nutrients and space, tumor cells compete with the surrounding cells. Since the energy of healthy cells decreases with the number of divisions [99,100], most healthy cells are not strong enough to compete with super-competitive tumor cells. Hence, many healthy cells in the environment die and provide space for tumor cells. Moreover, tumor cells regulate the escape mechanisms to move from this crowded, stressful environment. By secreting MMPs, aggressive tumor cells degrade the ECM components in the boundary. At the next step, malignant tumor cells escape through the boundary and invade the surrounding tissues.
Subsections 2.1–2.3 describe the scenario outcomes when varying the initial healthy cell density, the ECM protein density, and the ECM breakdown probability for the tumor progression, plasticity, and invasion, respectively.
When a tumor develops, the healthy cell density of the environment decreases and the tumor cell density increases. We observe the healthy and tumor cell densities for each scenario to investigate the tumor progression.
We can observe from the scenarios exploring the tumor progression (see Figures 8, 9 and Table 3) through the following aspects:
Parameter values | Equilibrium of healthy cell density | Equilibrium of tumor cell density |
N(0)=0.243,R=0.1,Pb=0.5 | 0.0435 | 0.8499 |
N(0)=0.324,R=0.1,Pb=0.5 | 0.0537 | 0.8444 |
N(0)=0.405,R=0.1,Pb=0.5 | 0.0648 | 0.8318 |
N(0)=0.324,R=0.1,Pb=0.25 | 0.0547 | 0.8415 |
N(0)=0.324,R=0.1,Pb=0.75 | 0.0547 | 0.8414 |
N(0)=0.324,R=0.05,Pb=0.5 | 0.0442 | 0.8995 |
N(0)=0.324,R=0.2,Pb=0.5 | 0.0772 | 0.7227 |
● A larger number of initial healthy cells slightly slows the increase in tumor cell density and results in a slightly lower tumor cell density at equilibrium.
● The density of the healthy cells at equilibrium is reduced (due to tumor growth), but is closely related to the initial number of healthy cells.
● The density of obstacles (or proteins) in the ECM impacts the growth of the tumor. At equilibrium, the tumor cell density is significantly less for an obstacle density of 0.2 compared to a density of 0.05.
● Accordingly, more healthy cells survive at equilibrium if the ECM has a higher density of obstacles.
● It takes slightly longer for the tumor cell density to reach equilibrium for a larger initial healthy cell density or for a larger density of obstacles.
● Tumor cells compete for space and nutrients, and kill weak healthy cells. An environment with a low density of obstacles and a lower healthy cell density is advantageous for tumor cells. Conversely, the tumor progression is impacted in a crowded environment.
● Simulation results showed that the ECM breakdown probability does not significantly affect the tumor progression. The graphs were omitted as they largely overlapped, thus indicating minimal variation and a negligible impact on the tumor progression. This suggests that the ECM breakdown probability does not play a critical role in the progression of the tumor.
● Low variations at early times and after equilibrium show the homogeneous state of the environment. The high variation in the cell densities during the middle period suggests that the system is undergoing significant changes.
We investigate the ability of tumor cells to change their phenotypes according to their environment. In particular, we observe the change in the average cell stickiness and the average jump radius of the tumor cells. In these simulations, the initial stickiness value for a healthy cell is 0.9. The stickiness of a tumor cell differs depending on the change in stickiness and the stickiness of the mother cell - see Table 1. We neglect the movements of the healthy cells, as they have a high stickiness and a low motility. The jump radius of a tumor cell changes according to the jump radius of the mother cell and the change in jump radius - again see Table 1. Figure 10 and Table 4 show the average stickiness of tumor cells. From Tables 3 and 4 and Figures 9 and 10, we can make the following comments:
Parameter Values | Equilibrium of average cell stickiness | Equilibrium of average jump radius |
N(0)=0.243,R=0.1,Pb=0.5 | 0.6635 | 8.153 |
N(0)=0.324,R=0.1,Pb=0.5 | 0.6543 | 8.189 |
N(0)=0.405,R=0.1,Pb=0.5 | 0.6407 | 8.196 |
N(0)=0.324,R=0.1,Pb=0.25 | 0.654 | 8.162 |
N(0)=0.324,R=0.1,Pb=0.75 | 0.6541 | 8.158 |
N(0)=0.324,R=0.05,Pb=0.5 | 0.6946 | 8.266 |
N(0)=0.324,R=0.2,Pb=0.5 | 0.6099 | 7.833 |
● The average cell stickiness of the tumor cells reduces over time and is lower for increasing initial healthy cell densities. This stickiness value reduces to a lower value for higher values of obstacle densities.
● If the environment is crowded, then the tumor cells will lose their stickiness; hence, they will not be as likely to collectively move in a group, and this slows down the tumor progression.
● The average jump radius of the tumor cells increases more rapidly for lower initial healthy cell densities, although it approaches a similar equilibrium value regardless of N(0).
● The average jump radius of the tumor cells increases more rapidly and approaches a higher equilibrium value when the obstacle density is lower.
● Tumor cells regulate the cell plasticity mechanisms according to the various conditions of the TME. In a crowded environment, the tumor cells can reduce their stickiness and increase their jump radius, which affects their ability to move. Hence, the tumor cells promote cell plasticity.
● The initial healthy cell density and the ECM protein density influence the tumor cell plasticity regulations. The results suggest that if there are many environmental barriers, then the tumor cells try to migrate as individual cells by reducing their stickiness at a high rate. Otherwise, they may escape the environment as a collection of cells. Hence, the invasive pattern of the cells may differ according to the tumor microenvironmental conditions.
● The tumor cells have high motility rates under low initial healthy cell densities and ECM protein densities. Furthermore, they may try to move as a collection of cells under those conditions. Hence, the high initial healthy cell densities and ECM protein densities in the TME slow down the motility rates of the tumor cells.
● The simulation results showed that the ECM breakdown probability did not significantly affect the tumor cell plasticity. The graphs were omitted as they are similar to results already presented. This indicates a minimal variation and a negligible impact on the tumor cell plasticity. This suggests that the ECM breakdown probability does not play a critical role in the tumor cell plasticity.
We investigate the effects of the tumor microenvironmental factors that lead to an increased likelihood of tumor invasion. We measure this by determining the number of tumor cells that exit the tumor microenvironment, as well as the degradation of the fence density that provides greater chances of tumor invasion. See Figure 11 and average first cell exit times given in Table 5. We note the following:
Parameter values | Average first cell exit time | Standard deviation |
N(0)=0.243,R=0.1,Pb=0.5 | 5.0262 | 1.2432 |
N(0)=0.324,R=0.1,Pb=0.5 | 5.1932 | 1.291 |
N(0)=0.405,R=0.1,Pb=0.5 | 5.3869 | 1.4773 |
N(0)=0.324,R=0.1,Pb=0.25 | 5.289 | 1.3868 |
N(0)=0.324,R=0.1,Pb=0.75 | 5.0695 | 1.3949 |
N(0)=0.324,R=0.05,Pb=0.5 | 4.7101 | 1.3291 |
N(0)=0.324,R=0.2,Pb=0.5 | 5.9631 | 1.4075 |
● More tumor cells exit the TME when there is a reduced initial healthy cell density, as these conditions lead to faster tumor progression.
● More tumor cells exit the TME when the probability of a breakdown in the fence density of the ECM (Pb) increases.
● The density of the ECM proteins (R) impacts the tumor cell exit. More tumor cells exit the TME for an obstacle rate of 0.05 compared to a rate of 0.2.
● Accordingly, a lesser number of tumor cells exit for increased initial healthy cell densities, increased ECM proteins, and low ECM breakdown probabilities.
● A reduction of the fence density is slow for high initial healthy cell densities, low ECM breakdown probabilities, and high ECM protein densities.
● As the stickiness of a cell reduces to a lower value for the higher values of the initial healthy cell densities and obstacle densities (see Figure 10), many cells may individually exit the TME through existing gaps without degrading new fence components under these conditions.
● The first cell exit time is influenced by the density of the ECM obstacles/proteins; a lower density leads to an earlier first exit time.
● The breakdown probability does not have much influence on the first exit time, neither does the initial healthy cell density.
If a healthy tissue has many cells or ECM proteins, then the cell invasion speed is slow. Furthermore, the tumor cell invasion can slow if the ECM breakdown probability of the tumor cells is low. Moreover, the tumor cells start to exit the environment early for low initial healthy cell densities, high ECM breakdown probabilities, and low obstacle densities. We observe that the tumor progression is high under these tumor microenvironmental conditions. Hence, the tumor cells try to exit early, as the environment becomes quickly crowded under these conditions.
In this paper, we explored how various components of the tumor microenvironment promote tumor progression, invasion, and metastasis via a mathematical agent-based model. In this agent-based model, we simulated cell-cell and cell-ECM interactions according to a set of biological rules, underpinned by the eight hallmarks of cancer suggested by Hanahan and colleagues [92,93,94]. This allowed for a link between the tumor heterogeneity and the cellular response. Then, the output from the model suggested lines of enquiry into the system dynamics, thus augmenting clinical experiments that are often time-consuming and expensive. To get such insights into these dynamics (in time and space) is invaluable to predict possible new treatment strategies. We re-emphasize that we did not calibrating our model against experimental results. Rather, we adopted a standard approach of using detailed simulations to explore potential hypotheses.
Cancer is life-threatening if the tumor cells spread into the surrounding tissues and other organs. The results suggest that the ECM protein density, the initial healthy cell density, and the ECM degradation rate affect tumor cell invasion. Hence, by focusing on these three factors, we can explore the spread of tumor cell invasion. For example, the tumor cell invasion spread is slow if there are many ECM proteins and healthy cells in the domain. By using an agent-based model similar to the one described here, biologists, clinicians, and modelers can work together to test different hypotheses that can offer insights into the design of new therapies. In particular, this synergy between different groups allows the researchers to eliminate scenarios that are not appropriate in designing new cancer therapies.
Our agent-based model represents a two-dimensional tissue section that consists of healthy cells, tumor cells, and ECM proteins; the domain is a 100 × 100 grid that initially represents healthy tissue. The simulation started with one healthy cell mutating, which allowed a tumor to grow. By setting various parameters for the model (e.g., the density of the ECM proteins in the domain; the stickiness of the cells that affects the tumor cell movement; and the density of the fence around the boundary of the domain that affects the likelihood of a tumor cell exiting and spreading beyond the domain), the simulation tracked the density of healthy cells and tumor cells over time and thus provides a link between the parameters and tumor progression, invasion, and metastasis.
We observed that tumor progression was affected by the initial density of healthy cells in the domain and the density of the ECM proteins. We observed tumor cell plasticity in terms of a reduction in the tumor cell stickiness (that may be due to crowdedness of the environment) that influenced how the tumor cells escaped the domain and spread; additionally, this is associated with the probability of the ECM protein breakdown along the boundary of the domain.
Our model is a starting point to explore tumor growth, and a limitation is that it is only spatially two-dimensional and is based on a regular mesh. Consequently, it only handles a limited complexity, and there are still issues with how to associate the results from the simulations with clinical outcomes. Furthermore, there is clearly a need to carry out a sensitivity analysis; however, due to the number of parameters, this has not proved feasible in this present work. Additionally, it would be interesting to compare our lattice-based approach with off-lattice models, though this is beyond the scope of this present work.
This paper explored the effects of the initial healthy cell density, the ECM protein density, and the ECM breakdown probability for tumor progression, cell plasticity, and invasion using an agent-based model. Although the model was based on a two-dimensional spatial domain, we are able to demonstrate links between the initial healthy cell densities, the ECM protein densities, and the cell stickiness with tumor progression, cell motility, and invasion. We can easily change the model parameters to gain insights into the tumor cell behavior. Additionally, it is possible to modify the model to study different treatment strategies, such as the effect of T-cells on cancer dynamics, which will be further investigated at a later date. Incorporating T-cell effects can contribute to additional knowledge and offer new insights into novel treatment protocols; therefore, the study in this paper can be considered as an initial step in this direction.
The authors declare they have not used Artificial Intelligence (AI) tools in the creation of this article.
This research work was supported by the Australian Research Council Future Fellowship FT180100698.
The authors declare there is no conflict of interest.
A description of input parameters and calculated output variables for the agent-based model
(1) Healthy Cell Density (N(t)) - We calculate N(t) by dividing the current healthy cell population by total of 10,000 grid positions.
(2) Tumor Cell Density (A(t)) - We calculate A(t) by dividing the tumor cell population at time t by the total number of grid positions 10,000.
(3) Cell Stickiness (Si) - Cells can secrete substances that allow cells to stick with neighboring cells. The stickiness of cell i is represented by Si∈[0,1]. In cell proliferation, healthy daughter cells take the same stickiness as their mother cell. Otherwise, the stickiness of the daughter cell differs from the mother cell, depending on the change in stickiness. The movement of a cell depends on the stickiness of that cell. We assume that healthy cells have a high stickiness value [37].
(4) Change in Stickiness (μs) - When a cell mutates, the stickiness of that cell changes according to the stickiness of its mother cell. Assume that the change in stickiness is μs and the stickiness of the mother cell is S, then the stickiness of the daughter cell can take a uniformly distributed value between S−μs and S+μs.
(5) Jump Radius (Ji) - The jump radius of cell i is represented by Ji. It measures cell movement and represents the number of steps a cell can move at a time. We assume that the movement direction of a cell should be North, South, East or West. The jump radius of a tumor cell changes according to the jump radius of its mother cell. We assume that the jump radius of the daughter cell can increase by one, decrease by one or take the same jump radius as the mother cell, with a minimum value of 1.
(6) Change in Jump Radius (μj) - When a cell mutates, the jump radius of that cell changes according to the jump radius of its mother cell. Assume that the change in jump radius is μj and the jump radius of the mother cell is J, then the jump radius of the daughter cell should take a positive integer value chosen uniformly in the range [J−μj,J+μj]. In this model, we assume that μj=1.
(7) Maximum Divisions of a Healthy Cell (K) - Healthy cells can divide only a finite number of times. Due to genetic changes, tumor cells have the potential to reproduce unlimited times [92]. However, they have a slight chance of dying after completing the maximum number of cell divisions.
(8) Number of Divisions of a Cell (Di(t)) - The total number of divisions of cell i until time t is represented by Di(t). For a healthy cell, Di(t)≤K. Since tumor cells can proliferate uncontrollably, divisions of a tumor cell can take any positive integer value. However, tumor cells have a slight chance of dying when they complete K cell divisions.
(9) Cell Age (ai(t)) - The age of cell i at time t is represented by ai(t). At each time step, the age of each cell increases by one. When a cell reaches its reproduction age, it has a chance to proliferate. When a cell proliferates, the age of the mother cell and the daughter cell initialise to zero, and the number of divisions of that cell (Di(t)) increases by one.
(10) Division Age (L) - A cell can divide when the age of that cell is greater than the division age. The duration of the cell cycle differs from organism to organism and cell to cell. In this model, we assume that the division age of a cell is 20.
(11) Competition Rate (Ci) - The competition rate of cell i is represented by Ci. Each cell has a competitive rate that depends on the metabolism of that cell (the metabolism level of a healthy cell decreases with each cell division) [99,100]. If the maximum number of cell divisions of a cell is K and the number of remaining cell divisions of that cell is n, the competitive rate of that cell is n/K. The range of values for the competition rate of healthy cells is [0,1]. Since tumor cells have super-competitive phenotypes with their genetic changes, we assume that tumor cells have highly competitive rates. Hence, we choose a random competitive rate between [0.5,1]. If a tumor cell does not have enough space to proliferate, that cell competes with immediate neighboring cells. If a cell in the neighborhood has a lower competition rate than the middle cell, that cell dies, and the daughter cell occupies that grid position.
(12) Probability of Dividing (Pd) - If the age of a cell reaches the cell division age, the probability of dividing determines whether that cell divides or not at that time. Since healthy cells can control their cell growth, they only proliferate when they get signals to execute. Tumor cells have a high probability of cell division because they sustain proliferative signalling and evade growth suppressors.
(13) Probability of Death (Pa) - When a healthy cell completes its maximum number of cell divisions, that cell has a higher chance to die. Since tumor cells resist their death, the probability of death of tumor cells is small. When a cell dies, the grid position of that cell becomes empty.
(14) Obstacle Density (R) - The ECM provides a physical barrier for cell movements through proteins. In this study, we include obstacles in the domain to represent ECM proteins. If the next chosen grid position of a cell is occupied by an obstacle, the cell stays at its current position until it gets another chance to move. We assume that tumor cells cannot destroy obstacles. Hence obstacle density does not change with time.
(15) Probability of Cell Movement (Pm(i,t)) - The probability of cell movement depends on the number of cells or obstacles in the Moore neighborhood (eight surrounding grid positions) and the stickiness of the cell. The probability of movement of cell i at time t is represented by Pm(i,t). If the stickiness of a cell is S and the number of cells and obstacles in the neighborhood is n, the probability of movement of that cell is (1−S)n.
(16) Fence Density (f(t)) - The boundary of the domain has a fence that represents ECM components of the TME. Generally, cells cannot move through the surrounding membrane [16]. Hence we assume that initially, the domain is fully fenced. Once tumor cells reach the boundary, they attach to the fence components and secrete proteases to break down the fence components. This process makes gaps in the boundary, and cells can move through these gaps.
(17) ECM Break Down Probability (Pb) - When a tumor cell attaches to the ECM, some tumor cells secrete a high level of MMPs to break down the ECM component. Therefore some tumor cells that reach the boundary escape from the domain by making gaps in the boundary with a certain probability Pb.
(18) Number of Exit Cells (E(t)) - After reaching the boundary, tumor cells exit the domain through gaps in the boundary. The number of Exit Cells (E(t)) represents the total number of tumor cells that escaped the domain from time 0 to t.
(19) Average Stickiness (ˉS(t)) - The output variable ˉS(t) calculates the average stickiness of tumor cells at time t.
(20) Average Jump Radius (ˉJ(t)) - The output variable ˉJ(t) calculates the average of jump radii of tumor cells at time t.
A description about the flow chart that represents in Figure 5
If the cell survives or k≤K, we should check the age of that cell (ai). If ai≥L, the division age, generate a uniformly distributed random number r2∈[0,1]. That cell can divide if r2≤Pd. In the cell division process, the mother cell stays at the same position, and the daughter cell takes an unoccupied neighboring grid. The daughter cell placement can take a North, North-East, East, South-East, South, South-West, West or North-West grid, the first empty grid position in the clockwise direction starting from the North. If all neighboring grid positions are occupied, the mother cell competes with surrounding cells, and the weakest cell in the neighborhood dies, and that position is taken by the daughter cell. Figure 6 represents possible grid positions of the daughter cell after proliferation and how the position of the daughter cell can be chosen according to the cells or obstacles in the neighborhood.
Any alive cell can move to a new grid position depending on the number of cells and obstacles in the neighborhood, and cell stickiness. We generate a uniformly distributed random number r3∈[0,1] and compare it with Pm(i) of that cell that depends on the number of cells in the neighborhood and their stickiness. If r3≤Pm(i), the cell moves to a new grid position. A tumor cell can move North, East, South or West. If r3>Pm(i), the cell stays at the same grid position. Moreover, cell movement distance depends on the jump radius of that cell. The randomly selected new cell position should be an empty grid position.
[1] |
R. Baghban, L. Roshangar, R. Jahanban-Esfahlan, K. Seidi, A. Ebrahimi-Kalan, M. Jaymand, et al., Tumor microenvironment complexity and therapeutic implications at a glance, Cell Commun. Signaling, 18 (2020), 1–19. https://doi.org/10.1186/s12964-020-0530-4 doi: 10.1186/s12964-020-0530-4
![]() |
[2] |
F. R. Balkwill, M. Capasso, T. Hagemann, The tumor microenvironment at a glance, J. Cell Sci., 125 (2012), 5591–5596. https://doi.org/10.1242/jcs.116392 doi: 10.1242/jcs.116392
![]() |
[3] |
B. Coban, C. Bergonzini, A. J. Zweemer, E. H. Danen, Metastasis: crosstalk between tissue mechanics and tumour cell plasticity, Br. J. Cancer, 124 (2021), 49–57. https://doi.org/10.1038/s41416-020-01150-7 doi: 10.1038/s41416-020-01150-7
![]() |
[4] |
M. L. Taddei, E. Giannoni, G. Comito, P. Chiarugi, Microenvironment and tumor cell plasticity: an easy way out, Cancer Lett., 341 (2013), 80–96. https://doi.org/10.1016/j.canlet.2013.01.042 doi: 10.1016/j.canlet.2013.01.042
![]() |
[5] |
A. L. Ribeiro, O. K. Okamoto, Combined effects of pericytes in the tumor microenvironment, Stem Cells Int., 1 (2015), 868475. https://doi.org/10.1155/2015/868475 doi: 10.1155/2015/868475
![]() |
[6] | B. M. Lopes-Bastos, W. G. Jiang, J. Cai, Tumour-endothelial cell communications: important and indispensable mediators of tumour angiogenesis, Anticancer Res., 36 (2016), 1119–1126. |
[7] |
F. Xing, J. Saidou, K. Watabe, Cancer associated fibroblasts (CAFs) in tumor microenvironment, Front. Biosci., 15 (2010), 166. https://doi.org/10.2741/3613 doi: 10.2741/3613
![]() |
[8] |
M. R. Galdiero, E. Bonavita, I. Barajon, C. Garlanda, A. Mantovani, S. Jaillon, Tumor associated macrophages and neutrophils in cancer, Immunobiology, 218 (2013), 1402–1410. https://doi.org/10.1016/j.imbio.2013.06.003 doi: 10.1016/j.imbio.2013.06.003
![]() |
[9] |
A. M. Høye, J. T. Erler, Structural ECM components in the premetastatic and metastatic niche, Am. J. Physiol. Cell Physiol., 310 (2016), C955–C967. https://doi.org/10.1152/ajpcell.00326.2015 doi: 10.1152/ajpcell.00326.2015
![]() |
[10] |
P. Lu, V. M. Weaver, Z. Werb, The extracellular matrix: a dynamic niche in cancer progression, J. Cell Biol., 196 (2012), 395–406. https://doi.org/10.1083/jcb.201102147 doi: 10.1083/jcb.201102147
![]() |
[11] |
A. D. Theocharis, S. S. Skandalis, C. Gialeli, N. K. Karamanos, Extracellular matrix structure, Adv. Drug Delivery Rev., 97 (2016), 4–27. https://doi.org/10.1016/j.addr.2015.11.001 doi: 10.1016/j.addr.2015.11.001
![]() |
[12] |
B. Yue, Biology of the extracellular matrix: an overview, J. Glaucoma, 23 (2014), S20–S23. https://doi.org/10.1097/IJG.0000000000000108 doi: 10.1097/IJG.0000000000000108
![]() |
[13] |
J. Huang, L. Zhang, D. Wan, L. Zhou, S. Zheng, S. Lin, et al., Extracellular matrix and its therapeutic potential for cancer treatment, Signal Transduction Targeted Ther., 6 (2021), 1–24. https://doi.org/10.1038/s41392-021-00544-0 doi: 10.1038/s41392-021-00544-0
![]() |
[14] | K. Yuan, R. K. Singh, G. Rezonzew, G. P. Siegal, In vitro matrices for studying tumor cell invasion, in Cell Motility in Cancer Invasion and Metastasis, Springer, (2006), 25–54. https://doi.org/10.1007/b103440 |
[15] |
N. M. Hooper, Y. Itoh, H. Nagase, Matrix metalloproteinases in cancer, Essays Biochem., 38 (2002), 21–36. https://doi.org/10.1042/bse0380021 doi: 10.1042/bse0380021
![]() |
[16] |
L. A. Liotta, U. P. Thorgeirsson, S. Garbisa, Role of collagenases in tumor cell invasion, Cancer Metastasis Rev., 1 (1982), 277–288. https://doi.org/10.1007/BF00124213 doi: 10.1007/BF00124213
![]() |
[17] | T. R. Cox, The matrix in cancer, Nat. Rev. Cancer, 21 (2021), 217–238. https://doi.org/10.1038/s41568-020-00329-7 |
[18] |
S. Turner, J. A. Sherratt, Intercellular adhesion and cancer invasion: a discrete simulation using the extended potts model, J. Theor. Biol., 216 (2002), 85–100. https://doi.org/10.1006/jtbi.2001.2522 doi: 10.1006/jtbi.2001.2522
![]() |
[19] |
M. DePalma, D. Biziato, T. V. Petrova, Microenvironmental regulation of tumour angiogenesis, Nat. Rev. Cancer, 17 (2017), 457–474. https://doi.org/10.1038/nrc.2017.51 doi: 10.1038/nrc.2017.51
![]() |
[20] |
R. J. Gillies, J. S. Brown, A. R. Anderson, R. A. Gatenby, Eco-evolutionary causes and consequences of temporal changes in intratumoural blood flow, Nat. Rev. Cancer, 18 (2018), 576–585. https://doi.org/10.1038/s41568-018-0030-7 doi: 10.1038/s41568-018-0030-7
![]() |
[21] |
B. T. Finicle, V. Jayashankar, A. L. Edinger, Nutrient scavenging in cancer, Nat. Rev. Cancer, 18 (2018), 619–633. https://doi.org/10.1038/s41568-018-0048-x doi: 10.1038/s41568-018-0048-x
![]() |
[22] | C. García-Jiménez, C. R. Goding, Starvation and pseudo-starvation as drivers of cancer metastasis through translation reprogramming, Cell Metab., 29 (2019), 254–267. |
[23] |
R. J. DeBerardinis, N. S. Chandel, Fundamentals of cancer metabolism, Sci. Adv., 2 (2016), e1600200. https://doi.org/10.1126/sciadv.1600200 doi: 10.1126/sciadv.1600200
![]() |
[24] |
M. G. Vander Heiden, Targeting cancer metabolism: a therapeutic window opens, Nat. Rev. Drug Discovery, 10 (2011), 671–684. https://doi.org/10.1038/nrd3504 doi: 10.1038/nrd3504
![]() |
[25] |
B. Kalyanaraman, Teaching the basics of cancer metabolism: Developing antitumor strategies by exploiting the differences between normal and cancer cell metabolism, Redox Biol., 12 (2017), 833–842. https://doi.org/10.1016/j.redox.2017.04.018 doi: 10.1016/j.redox.2017.04.018
![]() |
[26] |
R. A. Cairns, I. S. Harris, T. W. Mak, Regulation of cancer cell metabolism, Nat. Rev. Cancer, 11 (2011), 85–95. https://doi.org/10.1038/nrc2981 doi: 10.1038/nrc2981
![]() |
[27] |
C. A. Lyssiotis, A. C. Kimmelman, Metabolic interactions in the tumor microenvironment, Trends Cell Biol., 27 (2017), 863–875. https://doi.org/10.1016/j.tcb.2017.06.003 doi: 10.1016/j.tcb.2017.06.003
![]() |
[28] |
S. Bowling, K. Lawlor, T. A. Rodríguez, Cell competition: the winners and losers of fitness selection, Development, 146 (2019), dev167486. https://doi.org/10.1242/dev.167486 doi: 10.1242/dev.167486
![]() |
[29] |
A. Gutiérrez-Martínez, W. Q. G. Sew, M. Molano-Fernández, M. Carretero-Junquera, H. Herranz, Mechanisms of oncogenic cell competition-paths of victory, Semin. Cancer Biol., 63 (2020), 27–35. https://doi.org/10.1016/j.semcancer.2019.05.015 doi: 10.1016/j.semcancer.2019.05.015
![]() |
[30] |
R. Levayer, Solid stress, competition for space and cancer: The opposing roles of mechanical cell competition in tumour initiation and growth, Nat. Rev. Cancer, 63 (2020), 69–80. https://doi.org/10.1016/j.semcancer.2019.05.004 doi: 10.1016/j.semcancer.2019.05.004
![]() |
[31] |
E. Moreno, Is cell competition relevant to cancer?, Nat. Rev. Cancer, 8 (2008), 141–147. https://doi.org/10.1038/nrc2252 doi: 10.1038/nrc2252
![]() |
[32] | M. Vishwakarma, E. Piddini, Outcompeting cancer, Nat. Rev. Cancer, 20 (2020), 187–198. https://doi.org/10.1038/s41568-019-0231-8 |
[33] |
T. M. Parker, V. Henriques, A. Beltran, H. Nakshatri, R. Gogna, Cell competition and tumor heterogeneity, Nat. Rev. Cancer, 63 (2020), 1–10. https://doi.org/10.1016/j.semcancer.2019.09.003 doi: 10.1016/j.semcancer.2019.09.003
![]() |
[34] |
S. Di Giacomo, M. Sollazzo, D. De Biase, M. Ragazzi, P. Bellosta, A. Pession, et al., Human cancer cells signal their competitive fitness through MYC activity, Sci. Rep., 7 (2017), 1–12. https://doi.org/10.1038/s41598-017-13002-1 doi: 10.1038/s41598-017-13002-1
![]() |
[35] |
E. Madan, M. L. Peixoto, P. Dimitrion, T. D. Eubank, M. Yekelchyk, S. Talukdar, et al., Cell competition boosts clonal evolution and hypoxic selection in cancer, Trends Cell Biol., 12 (2020), 967–978. https://doi.org/10.1016/j.tcb.2020.10.002 doi: 10.1016/j.tcb.2020.10.002
![]() |
[36] |
U. Cavallaro, G. Christofori, Cell adhesion in tumor invasion and metastasis: loss of the glue is not enough, Biochim. Biophys. Acta, Rev. Cancer, 1552 (2001), 39–45. https://doi.org/10.1016/S0304-419X(01)00038-5 doi: 10.1016/S0304-419X(01)00038-5
![]() |
[37] |
M. Janiszewska, M. C. Primi, T. Izard, Cell adhesion in cancer: Beyond the migration of single cells, J. Biol. Chem., 295 (2020), 2495–2505. https://doi.org/10.1074/jbc.REV119.007759 doi: 10.1074/jbc.REV119.007759
![]() |
[38] |
M. C. Moh, S. Shen, The roles of cell adhesion molecules in tumor suppression and cell migration: a new paradox, Cell Adhes. Migr., 3 (2009), 334–336. https://doi.org/10.4161/cam.3.4.9246 doi: 10.4161/cam.3.4.9246
![]() |
[39] |
H. Son, A. Moon, Epithelial-mesenchymal transition and cell invasion, Toxicol. Res., 26 (2010), 245–252. https://doi.org/10.5487/TR.2010.26.4.245 doi: 10.5487/TR.2010.26.4.245
![]() |
[40] |
P. M. Altrock, L. L. Liu, F. Michor, The mathematics of cancer: integrating quantitative models, Nat. Rev. Cancer, 15 (2015), 730–745. https://doi.org/10.1038/nrc4029 doi: 10.1038/nrc4029
![]() |
[41] |
G. Jordão, J. N. Tavares, Mathematical models in cancer therapy, Biosystems, 162 (2017), 12–23. https://doi.org/10.1016/j.biosystems.2017.08.007 doi: 10.1016/j.biosystems.2017.08.007
![]() |
[42] |
V. Quaranta, A. M. Weaver, P. T. Cummings, A. R. Anderson, Mathematical modeling of cancer: The future of prognosis and treatment, Clin. Chim. Acta, 357 (2005), 173–179. https://doi.org/10.1016/j.cccn.2005.03.023 doi: 10.1016/j.cccn.2005.03.023
![]() |
[43] |
H. N. Weerasinghe, P. M. Burrage, K. Burrage, D. V. Nicolau Jr, Mathematical models of cancer cell plasticity, J. Oncol., 2019 (2019), 2403483. https://doi.org/10.1155/2019/2403483 doi: 10.1155/2019/2403483
![]() |
[44] | P. Macklin, M. E. Edgerton, Discrete cell modeling, in Multiscale Modeling of Cancer: an Integrated Experimental and Mathematical Modeling Approach, Cambridge University Press, (2010), 88–122. https://doi.org/10.1017/CBO9780511781452 |
[45] |
J. Metzcar, Y. Wang, R. Heiland, P. Macklin, A review of cell-based computational modeling in cancer biology, JCO Clin. Cancer Inf., 2 (2019), 1–13. https://doi.org/10.1200/CCI.18.00069 doi: 10.1200/CCI.18.00069
![]() |
[46] |
Z. Wang, J. D. Butner, V. Cristini, T. S. Deisboeck, Integrated PK-PD and agent-based modeling in oncology, J. Pharmacokinet. Pharmacodyn., 42 (2015), 179189. https://doi.org/10.1007/s10928-015-9403-7 doi: 10.1007/s10928-015-9403-7
![]() |
[47] |
C. K. Macnamara, Biomechanical modelling of cancer: Agent-based force-based models of solid tumours within the context of the tumour microenvironment, Comput. Syst. Oncol., 1 (2021), e1018. https://doi.org/10.1002/cso2.1018 doi: 10.1002/cso2.1018
![]() |
[48] |
J. S. Lowengrub, H. B. Frieboes, F. Jin, Y. L. Chuang, X. Li, P. Macklin, et al., Nonlinear modelling of cancer: bridging the gap between cells and tumours, Nonlinearity, 23 (2009), R1–R91. https://doi.org/10.1088/0951-7715/23/1/R01 doi: 10.1088/0951-7715/23/1/R01
![]() |
[49] |
R. Sachs, L. Hlatky, P. Hahnfeldt, Simple ODE models of tumor growth and anti-angiogenic or radiation treatment, Math. Comput. Modell., 33 (2001), 1297–1305. https://doi.org/10.1016/S0895-7177(00)00316-2 doi: 10.1016/S0895-7177(00)00316-2
![]() |
[50] |
H. Enderling, A. R. Anderson, M. A. Chaplain, A. J. Munro, J. S. Vaidya, Mathematical modelling of radiotherapy strategies for early breast cancer, J. Theor. Biol., 241 (2006), 158–171. https://doi.org/10.1016/j.jtbi.2005.11.015 doi: 10.1016/j.jtbi.2005.11.015
![]() |
[51] | H. Enderling, M. A. Chaplain, A. R. Anderson, J. S. Vaidya, A mathematical model of breast cancer development, local treatment and recurrence, J. Theor. Biol., 246 (2007), 245–259. |
[52] |
V. Andasari, M. A. Chaplain, Intracellular modelling of cell-matrix adhesion during cancer cell invasion, Math. Modell. Nat. Phenom., 7 (2012), 29–48. https://doi.org/10.1051/mmnp/20127103 doi: 10.1051/mmnp/20127103
![]() |
[53] | J. C. Larsen, A mathematical model of adoptive T cell therapy, JP J. Appl. Math., 15 (2017), 1–33. |
[54] |
L. Glass, Instability and mitotic patterns in tissue growth, IFAC Proc. Vol., 6 (1973), 129–131. https://doi.org/10.1016/S1474-6670(17)67989-8 doi: 10.1016/S1474-6670(17)67989-8
![]() |
[55] |
R. Shymko, L. Glass, Cellular and geometric control of tissue growth and mitotic instability, J. Theor. Biol., 63 (1976), 355–374. https://doi.org/10.1016/0022-5193(76)90039-4 doi: 10.1016/0022-5193(76)90039-4
![]() |
[56] |
M. Chaplain, Avascular growth, angiogenesis and vascular growth in solid tumours: The mathematical modelling of the stages of tumour development, Math. Comput. Modell., 23 (1996), 47–87. https://doi.org/10.1016/0895-7177(96)00019-2 doi: 10.1016/0895-7177(96)00019-2
![]() |
[57] |
J. A. Adam, A simplified mathematical model of tumor growth, Math. Biosci., 81 (1986), 229–244. https://doi.org/10.1016/0025-5564(86)90119-7 doi: 10.1016/0025-5564(86)90119-7
![]() |
[58] |
J. A. Adam, A mathematical model of tumor growth by diffusion, Math. Biosci., 94 (1989), 155. https://doi.org/10.1016/0895-7177(88)90533-X doi: 10.1016/0895-7177(88)90533-X
![]() |
[59] |
J. A. Adam, S. Maggelakis, Mathematical models of tumor growth. iv. effects of a necrotic core, Math. Biosci., 97 (1989), 121–136. https://doi.org/10.1016/0025-5564(89)90045-X doi: 10.1016/0025-5564(89)90045-X
![]() |
[60] | T. S. Deisboeck, Z. Wang, P. Macklin, V. Cristini, Multiscale cancer modeling, Ann. Rev. Biomed. Eng., 13 (2011), 127–155. https://doi.org/10.1201/b10407 |
[61] | E. Gavagnin, C. A. Yates, Stochastic and deterministic modeling of cell migration, in Handbook of Statistics, 39 (2018), 37–91. https://doi.org/10.1016/bs.host.2018.06.002 |
[62] |
A. S. Qi, X. Zheng, C. Y. Du, B. S. An, A cellular automaton model of cancerous growth, J. Theor. Biol., 161 (1993), 1–12. https://doi.org/10.1006/jtbi.1993.1035 doi: 10.1006/jtbi.1993.1035
![]() |
[63] |
J. Smolle, R. Hofmann-Wellenhof, R. Kofler, L. Cerroni, J. Haas, H. Kerl, Computer simulations of histologic patterns in melanoma using a cellular automaton provide correlations with prognosis, J. Invest. Dermatol., 105 (1995), 797–801. https://doi.org/10.1111/1523-1747.ep12326559 doi: 10.1111/1523-1747.ep12326559
![]() |
[64] |
S. F. Junior, M. Martins, M. Vilela, A growth model for primary cancer, Physica A, 261 (1998), 569–580. https://doi.org/10.1016/S0378-4371(98)00318-5 doi: 10.1016/S0378-4371(98)00318-5
![]() |
[65] |
H. Hatzikirou, A. Deutsch, Cellular automata as microscopic models of cell migration in heterogeneous environments, Curr. Topics Dev. Biol., 81 (2008), 401–434. https://doi.org/10.1016/S0070-2153(07)81014-3 doi: 10.1016/S0070-2153(07)81014-3
![]() |
[66] |
B. Chopard, R. Ouared, A. Deutsch, H. Hatzikirou, D. Wolf-Gladrow, Lattice-gas cellular automaton models for biology: from fluids to cells, Acta Biotheor., 58 (2010), 329–340. https://doi.org/10.1007/s10441-010-9118-5 doi: 10.1007/s10441-010-9118-5
![]() |
[67] |
Y. Jiang, J. Pjesivac-Grbovic, C. Cantrell, J. P. Freyer, A multiscale model for avascular tumor growth, Biophys. J., 89 (2005), 3884–3894. https://doi.org/10.1529/biophysj.105.060640 doi: 10.1529/biophysj.105.060640
![]() |
[68] |
A. Shirinifard, J. S. Gens, B. L. Zaitlen, N. J. Popławski, M. Swat, J. A. Glazier, 3D multi-cell simulation of tumor growth and angiogenesis, PloS One, 4 (2009), e7190. https://doi.org/10.1371/journal.pone.0007190 doi: 10.1371/journal.pone.0007190
![]() |
[69] |
A. Szabó, R. M. Merks, Cellular potts modeling of tumor growth, tumor invasion, and tumor evolution, Front. Oncol., 3 (2013), 87. https://doi.org/10.3389/fonc.2013.00087 doi: 10.3389/fonc.2013.00087
![]() |
[70] |
C. E. Donaghey, CELLSIM: cell cycle simulation made easy, Int. Rev. Cytol., 66 (1980), 171–210. https://doi.org/10.1016/S0074-7696(08)61974-9 doi: 10.1016/S0074-7696(08)61974-9
![]() |
[71] |
W. Duchting, G. Dehl, Spatial structure of tumor growth: A simulation study, IEEE Trans. Syst. Man Cybern., 10 (1980), 292–296. https://doi.org/10.1109/TSMC.1980.4308502 doi: 10.1109/TSMC.1980.4308502
![]() |
[72] |
W. Duchting, T. Vogelsaenger, Aspects of modelling and simulating tumor growth and treatment, J. Cancer Res. Clin. Oncol., 105 (1983), 1–12. https://doi.org/10.1007/BF00391824 doi: 10.1007/BF00391824
![]() |
[73] |
W. Duchting, T. Vogelsaenger, Recent progress in modelling and simulation of three dimensional tumor growth and treatment, Biosystems, 18 (1985), 79–91. https://doi.org/10.1016/0303-2647(85)90061-9 doi: 10.1016/0303-2647(85)90061-9
![]() |
[74] |
W. Duchting, T. Vogelsaenger, Three-dimensional pattern generation applied to spheroidal tumor growth in a nutrient medium, Int. J. Biomed. Comput., 12 (1981), 377–392. https://doi.org/10.1016/0020-7101(81)90050-7 doi: 10.1016/0020-7101(81)90050-7
![]() |
[75] | W. Duchting, T. Ginsberg, W. Ulmer, Modelling of tumor growth and treatment, Z. Angew. Math. Mech., 76 (1996), 347–350. |
[76] |
J. E. Schmitz, A. R. Kansaland, S. Torquato, A cellular automaton model of brain tumor treatment and resistance, J. Theor. Med., 4 (2002), 223–239. https://doi.org/10.1080/1027366031000086674 doi: 10.1080/1027366031000086674
![]() |
[77] |
C. Gong, O. Milberg, B. Wang, P. Vicini, R. Narwal, L. Roskos, et al., A computational multiscale agent-based model for simulating spatio-temporal tumour immune response to PD1 and PDL1 inhibition, J. R. Soc. Interface, 14 (2017), 20170320. https://doi.org/10.1098/rsif.2017.0320 doi: 10.1098/rsif.2017.0320
![]() |
[78] |
H. Xie, Y. Jiao, Q. Fan, M. Hai, J. Yang, Z. Hu, et al., Modeling three-dimensional invasive solid tumor growth in heterogeneous microenvironment under chemotherapy, PloS One, 13 (2018), e0206292. https://doi.org/10.1371/journal.pone.0206292 doi: 10.1371/journal.pone.0206292
![]() |
[79] |
A. R. Anderson, M. A. Chaplain, Continuous and discrete mathematical models of tumor induced angiogenesis, Bull. Math. Biol., 60 (1998), 857–899. https://doi.org/10.1006/bulm.1998.0042 doi: 10.1006/bulm.1998.0042
![]() |
[80] |
A. R. Anderson, M. A. Chaplain, E. L. Newman, R. J. Steele, A. M. Thompson, Mathematical modelling of tumour invasion and metastasis, Comput. Math. Methods Med., 2 (2000), 129–154. https://doi.org/10.1080/10273660008833042 doi: 10.1080/10273660008833042
![]() |
[81] | D. Dréau, D. Stanimirov, T. Carmichael, M. Hadzikadic, An agent-based model of solid tumor progression, in International Conference on Bioinformatics and Computational Biology, Springer, (2009), 187–198. https://doi.org/10.1007/978-3-642-00727-9_19 |
[82] |
P. Gerlee, A. Anderson, Evolution of cell motility in an individual-based model of tumour growth, J. Theor. Biol., 259 (2009), 67–83. https://doi.org/10.1016/j.jtbi.2009.03.005 doi: 10.1016/j.jtbi.2009.03.005
![]() |
[83] |
C. A. Athale, T. S. Deisboeck, The effects of EGF-receptor density on multiscale tumor growth patterns, J. Theor. Biol., 238 (2006), 771–779. https://doi.org/10.1016/j.jtbi.2005.06.029 doi: 10.1016/j.jtbi.2005.06.029
![]() |
[84] |
D. Walker, N. T. Georgopoulos, J. Southgate, Anti-social cells: predicting the influence of e-cadherin loss on the growth of epithelial cell populations, J. Theor. Biol., 262 (2010), 425–440. https://doi.org/10.1016/j.jtbi.2009.10.002 doi: 10.1016/j.jtbi.2009.10.002
![]() |
[85] |
H. Byrne, D. Drasdo, Individual-based and continuum models of growing cell populations: a comparison, J. Math. Biol., 58 (2009), 657–687. https://doi.org/10.1007/s00285-008-0212-0 doi: 10.1007/s00285-008-0212-0
![]() |
[86] |
S. Bekisz, L. Geris, Cancer modeling: From mechanistic to data-driven approaches, and from fundamental insights to clinical applications, J. Comput. Sci., 46 (2020), 101198. https://doi.org/10.1016/j.jocs.2020.101198 doi: 10.1016/j.jocs.2020.101198
![]() |
[87] |
G. Letort, A. Montagud, G. Stoll, R. Heiland, E. Barillot, P. Macklin, et al., PhysiBoSS: a multi-scale agent-based modelling framework integrating physical dimension and cell signalling, Bioinformatics, 35 (2019), 1188–1196. https://doi.org/10.1093/bioinformatics/bty766 doi: 10.1093/bioinformatics/bty766
![]() |
[88] |
M. Ponce-de Leon, A. Montagud, V. Noel, A. Meert, G. Pradas, E. Barillot, et al., Physiboss 2.0: a sustainable integration of stochastic boolean and agent-based modelling frameworks, npj Syst. Biol. Appl., 9 (2023), 54. https://doi.org/10.1038/s41540-023-00314-4 doi: 10.1038/s41540-023-00314-4
![]() |
[89] |
G. Stoll, B. Caron, E. Viara, A. Dugourd, A. Zinovyev, A. Naldi, et al., Maboss 2.0: an environment for stochastic Boolean modeling, Bioinformatics, 33 (2017), 2226–2228. https://doi.org/10.1093/bioinformatics/btx123 doi: 10.1093/bioinformatics/btx123
![]() |
[90] |
A. Ghaffarizadeh, R. Heiland, S. H. Friedman, S. M. Mumenthaler, P. Macklin, PhysiCell: an open source physics-based cell simulator for 3-D multicellular systems, PLoS Comput. Biol., 14 (2018), e1005991. https://doi.org/10.1371/journal.pcbi.1005991 doi: 10.1371/journal.pcbi.1005991
![]() |
[91] |
R. J. Preen, L. Bull, A. Adamatzky, Towards an evolvable cancer treatment simulator, Biosystems, 182 (2019), 1–7. https://doi.org/10.1016/j.biosystems.2019.05.005 doi: 10.1016/j.biosystems.2019.05.005
![]() |
[92] | D. Hanahan, R. A. Weinberg, The hallmarks of cancer, Cell, 100 (2000), 57–70. https://doi.org/10.1093/med/9780199656103.003.0001 |
[93] |
D. Hanahan, R. A. Weinberg, Hallmarks of cancer: the next generation, Cell, 144 (2011), 646–674. https://doi.org/10.1016/j.cell.2011.02.013 doi: 10.1016/j.cell.2011.02.013
![]() |
[94] |
D. Hanahan, L. M. Coussens, Accessories to the crime: functions of cells recruited to the tumor microenvironment, Cancer Cell, 21 (2012), 309–322. https://doi.org/10.1016/j.ccr.2012.02.022 doi: 10.1016/j.ccr.2012.02.022
![]() |
[95] | E. Ruoslahti, How cancer spreads, Sci. Am., 275 (1996), 72–77. https://doi.org/10.1038/scientificamerican0996-72 |
[96] |
L. Jiang, M. Wang, S. Lin, R. Jian, X. Li, J. Chan, et al., A quantitative proteome map of the human body, Cell, 183 (2020), 269–283. https://doi.org/10.1016/j.cell.2020.08.036 doi: 10.1016/j.cell.2020.08.036
![]() |
[97] |
L. Hayflick, The limited in vitro lifetime of human diploid cell strains, Exp. Cell. Res., 37 (1965), 614–636. https://doi.org/10.1016/B978-1-4832-3075-7.50017-7 doi: 10.1016/B978-1-4832-3075-7.50017-7
![]() |
[98] | N. F. Mathon, A. C. Lloyd, Cell senescence and cancer, Nat. Rev. Cancer, 1 (2001), 203–213. https://doi.org/10.1038/35106045 |
[99] | R. DiLoreto, C. T. Murphy, The cell biology of aging, Mol. Biol. Cell, 26 (2015), 4524–4531. https://doi.org/10.1091/mbc.E14-06-1084 |
[100] | A. Catic, Cellular metabolism and aging, in Metabolic Aspects of Aging, vol. 155 of Progress in Molecular Biology and Translational Science, Academic Press, (2018), 85–107. https://doi.org/10.1016/bs.pmbts.2017.12.003 |
Input parameter/Output variable | Description |
Healthy Cell Density (N(t)) | Division of the healthy cell population at time t by total of 10,000 grid positions |
Tumor Cell Density (A(t)) | Division of the tumor cell population at time t by total of 10,000 grid positions |
Cell Stickiness (Si) | Stickiness value of cell i to stick with neighboring cells |
Change in Stickiness (μs) | When a cell mutates, the stickiness of that cell is a uniformly distributed value between Sm−μs and Sm+μs, where Sm is the stickiness of the mother cell |
Jump Radius (Ji) | Measures cell movement and represents the number of steps a cell can move at a time |
Change in Jump Radius (μj) | When a cell mutates, the Jump Radius of that cell is a uniformly distributed value between Jm−μj and Jm+μj, where Jm is the jump radius of the mother cell |
Maximum Divisions of a Healthy Cell (K) | Healthy cells can divide only K number of times |
Number of Divided Times of a Cell (Di(t)) | The total number of divisions of cell i until time t. For a healthy cell, Di(t)≤K |
Cell Age (ai(t)) | The age of cell i at time t |
Division age (L) | A cell can divide when the age of that cell is greater than the division age |
Competition Rate (Ci) | If i is a healthy cell, then Ci=n/K, where n is the number of remaining cell divisions. If i is a tumor cell, then choose a random number Ci∈[0.5,1], as they are super-competitive. |
Probability of Dividing (Pd) | If the age of a cell reaches the cell division age, then Pd determines whether that cell divides or not at that time |
Probability of Death (Pa) | The chance of a cell death |
Probability of Cell Movement (Pm(i,t)) | If the stickiness of a cell i is S and the number of cells and obstacles in the neighborhood is n, then Pm(i,t)=(1−S)n |
Obstacle Density (R) | Division of the total number of ECM proteins by the total of 10,000 grid positions |
ECM Break Down Probability (Pb) | The chance that a tumor cell breaks down a fence component |
Number of Exit Cells (E(t)) | Total number of tumor cells that escaped the domain from time 0 to t |
Fence Density (f(t)) | Division of the number of ECM components at time t by the number of ECM components at time 0 |
Average Stickiness ˉS(t) | The average of the stickiness of the tumor cells at time t |
Average Jump Radius ˉJ(t) | The average of the jump radius of the tumor cells at time t |
Parameter | Value |
Initial healthy cell density (N(0)) | 0.324 |
ECM breakdown probability (Pb) | 0.5 |
Obstacle/Protein density (R) | 0.1 |
Healthy cell stickiness (Si) | 0.9 |
Change in stickiness (μs) | 0.1 |
Healthy cell jump radius (Ji) | 1 |
Change in jump radius (μj) | 1 |
Maximum number of cell divisions of healthy cells (K) | 10 |
Division age (L) | 20 |
Probability of Dividing (Pd) | 0.6 |
Probability of Death (Pa) | 0.001 |
Parameter values | Equilibrium of healthy cell density | Equilibrium of tumor cell density |
N(0)=0.243,R=0.1,Pb=0.5 | 0.0435 | 0.8499 |
N(0)=0.324,R=0.1,Pb=0.5 | 0.0537 | 0.8444 |
N(0)=0.405,R=0.1,Pb=0.5 | 0.0648 | 0.8318 |
N(0)=0.324,R=0.1,Pb=0.25 | 0.0547 | 0.8415 |
N(0)=0.324,R=0.1,Pb=0.75 | 0.0547 | 0.8414 |
N(0)=0.324,R=0.05,Pb=0.5 | 0.0442 | 0.8995 |
N(0)=0.324,R=0.2,Pb=0.5 | 0.0772 | 0.7227 |
Parameter Values | Equilibrium of average cell stickiness | Equilibrium of average jump radius |
N(0)=0.243,R=0.1,Pb=0.5 | 0.6635 | 8.153 |
N(0)=0.324,R=0.1,Pb=0.5 | 0.6543 | 8.189 |
N(0)=0.405,R=0.1,Pb=0.5 | 0.6407 | 8.196 |
N(0)=0.324,R=0.1,Pb=0.25 | 0.654 | 8.162 |
N(0)=0.324,R=0.1,Pb=0.75 | 0.6541 | 8.158 |
N(0)=0.324,R=0.05,Pb=0.5 | 0.6946 | 8.266 |
N(0)=0.324,R=0.2,Pb=0.5 | 0.6099 | 7.833 |
Parameter values | Average first cell exit time | Standard deviation |
N(0)=0.243,R=0.1,Pb=0.5 | 5.0262 | 1.2432 |
N(0)=0.324,R=0.1,Pb=0.5 | 5.1932 | 1.291 |
N(0)=0.405,R=0.1,Pb=0.5 | 5.3869 | 1.4773 |
N(0)=0.324,R=0.1,Pb=0.25 | 5.289 | 1.3868 |
N(0)=0.324,R=0.1,Pb=0.75 | 5.0695 | 1.3949 |
N(0)=0.324,R=0.05,Pb=0.5 | 4.7101 | 1.3291 |
N(0)=0.324,R=0.2,Pb=0.5 | 5.9631 | 1.4075 |
Input parameter/Output variable | Description |
Healthy Cell Density (N(t)) | Division of the healthy cell population at time t by total of 10,000 grid positions |
Tumor Cell Density (A(t)) | Division of the tumor cell population at time t by total of 10,000 grid positions |
Cell Stickiness (Si) | Stickiness value of cell i to stick with neighboring cells |
Change in Stickiness (μs) | When a cell mutates, the stickiness of that cell is a uniformly distributed value between Sm−μs and Sm+μs, where Sm is the stickiness of the mother cell |
Jump Radius (Ji) | Measures cell movement and represents the number of steps a cell can move at a time |
Change in Jump Radius (μj) | When a cell mutates, the Jump Radius of that cell is a uniformly distributed value between Jm−μj and Jm+μj, where Jm is the jump radius of the mother cell |
Maximum Divisions of a Healthy Cell (K) | Healthy cells can divide only K number of times |
Number of Divided Times of a Cell (Di(t)) | The total number of divisions of cell i until time t. For a healthy cell, Di(t)≤K |
Cell Age (ai(t)) | The age of cell i at time t |
Division age (L) | A cell can divide when the age of that cell is greater than the division age |
Competition Rate (Ci) | If i is a healthy cell, then Ci=n/K, where n is the number of remaining cell divisions. If i is a tumor cell, then choose a random number Ci∈[0.5,1], as they are super-competitive. |
Probability of Dividing (Pd) | If the age of a cell reaches the cell division age, then Pd determines whether that cell divides or not at that time |
Probability of Death (Pa) | The chance of a cell death |
Probability of Cell Movement (Pm(i,t)) | If the stickiness of a cell i is S and the number of cells and obstacles in the neighborhood is n, then Pm(i,t)=(1−S)n |
Obstacle Density (R) | Division of the total number of ECM proteins by the total of 10,000 grid positions |
ECM Break Down Probability (Pb) | The chance that a tumor cell breaks down a fence component |
Number of Exit Cells (E(t)) | Total number of tumor cells that escaped the domain from time 0 to t |
Fence Density (f(t)) | Division of the number of ECM components at time t by the number of ECM components at time 0 |
Average Stickiness ˉS(t) | The average of the stickiness of the tumor cells at time t |
Average Jump Radius ˉJ(t) | The average of the jump radius of the tumor cells at time t |
Parameter | Value |
Initial healthy cell density (N(0)) | 0.324 |
ECM breakdown probability (Pb) | 0.5 |
Obstacle/Protein density (R) | 0.1 |
Healthy cell stickiness (Si) | 0.9 |
Change in stickiness (μs) | 0.1 |
Healthy cell jump radius (Ji) | 1 |
Change in jump radius (μj) | 1 |
Maximum number of cell divisions of healthy cells (K) | 10 |
Division age (L) | 20 |
Probability of Dividing (Pd) | 0.6 |
Probability of Death (Pa) | 0.001 |
Parameter values | Equilibrium of healthy cell density | Equilibrium of tumor cell density |
N(0)=0.243,R=0.1,Pb=0.5 | 0.0435 | 0.8499 |
N(0)=0.324,R=0.1,Pb=0.5 | 0.0537 | 0.8444 |
N(0)=0.405,R=0.1,Pb=0.5 | 0.0648 | 0.8318 |
N(0)=0.324,R=0.1,Pb=0.25 | 0.0547 | 0.8415 |
N(0)=0.324,R=0.1,Pb=0.75 | 0.0547 | 0.8414 |
N(0)=0.324,R=0.05,Pb=0.5 | 0.0442 | 0.8995 |
N(0)=0.324,R=0.2,Pb=0.5 | 0.0772 | 0.7227 |
Parameter Values | Equilibrium of average cell stickiness | Equilibrium of average jump radius |
N(0)=0.243,R=0.1,Pb=0.5 | 0.6635 | 8.153 |
N(0)=0.324,R=0.1,Pb=0.5 | 0.6543 | 8.189 |
N(0)=0.405,R=0.1,Pb=0.5 | 0.6407 | 8.196 |
N(0)=0.324,R=0.1,Pb=0.25 | 0.654 | 8.162 |
N(0)=0.324,R=0.1,Pb=0.75 | 0.6541 | 8.158 |
N(0)=0.324,R=0.05,Pb=0.5 | 0.6946 | 8.266 |
N(0)=0.324,R=0.2,Pb=0.5 | 0.6099 | 7.833 |
Parameter values | Average first cell exit time | Standard deviation |
N(0)=0.243,R=0.1,Pb=0.5 | 5.0262 | 1.2432 |
N(0)=0.324,R=0.1,Pb=0.5 | 5.1932 | 1.291 |
N(0)=0.405,R=0.1,Pb=0.5 | 5.3869 | 1.4773 |
N(0)=0.324,R=0.1,Pb=0.25 | 5.289 | 1.3868 |
N(0)=0.324,R=0.1,Pb=0.75 | 5.0695 | 1.3949 |
N(0)=0.324,R=0.05,Pb=0.5 | 4.7101 | 1.3291 |
N(0)=0.324,R=0.2,Pb=0.5 | 5.9631 | 1.4075 |