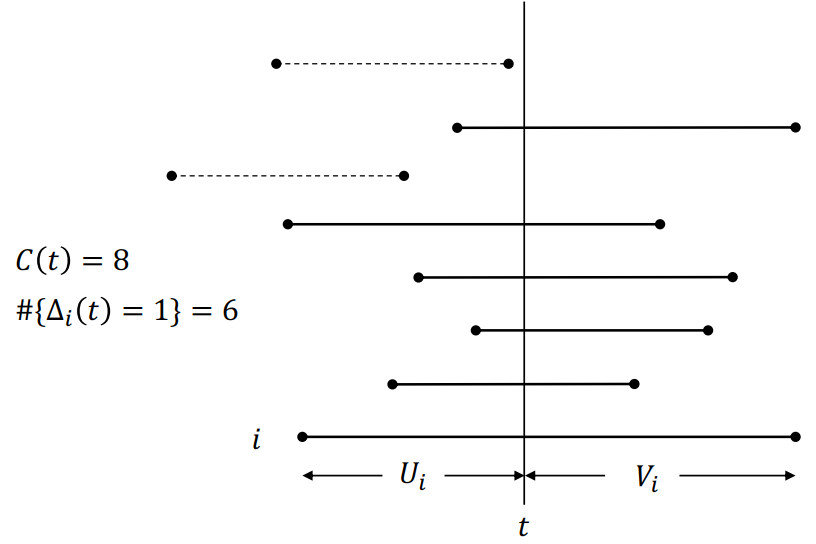
Traditional compartmental models of epidemic transmission often predict an initial phase of exponential growth, assuming uniform susceptibility and interaction within the population. However, empirical outbreak data frequently show early stages of sub-exponential growth in case incidences, challenging these assumptions and indicating that traditional models may not fully encompass the complexity of epidemic dynamics. This discrepancy has been addressed through models that incorporate early behavioral changes or spatial constraints within contact networks. In this paper, we propose the concept of "frailty", which represents the variability in individual susceptibility and transmission, as a more accurate approach to understanding epidemic growth. This concept shifts our understanding from a purely exponential model to a more nuanced, generalized model, depending on the level of heterogeneity captured by the frailty parameter. By incorporating this type of heterogeneity, often overlooked in traditional models, we present a novel mathematical framework. This framework enhances our understanding of how individual differences affect key epidemic metrics, including reproduction numbers, epidemic size, likelihood of stochastic extinction, impact of public health interventions, and accuracy of disease forecasts. By accounting for individual heterogeneity, our approach suggests that a more complex and detailed understanding of disease spread is necessary to accurately predict and manage outbreaks.
Citation: Ping Yan, Gerardo Chowell. Modeling sub-exponential epidemic growth dynamics through unobserved individual heterogeneity: a frailty model approach[J]. Mathematical Biosciences and Engineering, 2024, 21(10): 7278-7296. doi: 10.3934/mbe.2024321
[1] | Raimund Bürger, Gerardo Chowell, Leidy Yissedt Lara-Díıaz . Comparative analysis of phenomenological growth models applied to epidemic outbreaks. Mathematical Biosciences and Engineering, 2019, 16(5): 4250-4273. doi: 10.3934/mbe.2019212 |
[2] | Juan Pablo Aparicio, Carlos Castillo-Chávez . Mathematical modelling of tuberculosis epidemics. Mathematical Biosciences and Engineering, 2009, 6(2): 209-237. doi: 10.3934/mbe.2009.6.209 |
[3] | Huiping Zang, Shengqiang Liu, Yi Lin . Evaluations of heterogeneous epidemic models with exponential and non-exponential distributions for latent period: the Case of COVID-19. Mathematical Biosciences and Engineering, 2023, 20(7): 12579-12598. doi: 10.3934/mbe.2023560 |
[4] | Takeshi Miyama, Sung-mok Jung, Katsuma Hayashi, Asami Anzai, Ryo Kinoshita, Tetsuro Kobayashi, Natalie M. Linton, Ayako Suzuki, Yichi Yang, Baoyin Yuan, Taishi Kayano, Andrei R. Akhmetzhanov, Hiroshi Nishiura . Phenomenological and mechanistic models for predicting early transmission data of COVID-19. Mathematical Biosciences and Engineering, 2022, 19(2): 2043-2055. doi: 10.3934/mbe.2022096 |
[5] | Jinlong Lv, Songbai Guo, Jing-An Cui, Jianjun Paul Tian . Asymptomatic transmission shifts epidemic dynamics. Mathematical Biosciences and Engineering, 2021, 18(1): 92-111. doi: 10.3934/mbe.2021005 |
[6] | Yicang Zhou, Zhien Ma . Global stability of a class of discrete age-structured SIS models with immigration. Mathematical Biosciences and Engineering, 2009, 6(2): 409-425. doi: 10.3934/mbe.2009.6.409 |
[7] | Yukihiko Nakata, Ryosuke Omori . The change of susceptibility following infection can induce failure to predict outbreak potential by $\mathcal{R}_{0}$. Mathematical Biosciences and Engineering, 2019, 16(2): 813-830. doi: 10.3934/mbe.2019038 |
[8] | Cheng-Cheng Zhu, Jiang Zhu, Xiao-Lan Liu . Influence of spatial heterogeneous environment on long-term dynamics of a reaction-diffusion SVIR epidemic model with relaps. Mathematical Biosciences and Engineering, 2019, 16(5): 5897-5922. doi: 10.3934/mbe.2019295 |
[9] | Qinghua Liu, Siyu Yuan, Xinsheng Wang . A SEIARQ model combine with Logistic to predict COVID-19 within small-world networks. Mathematical Biosciences and Engineering, 2023, 20(2): 4006-4017. doi: 10.3934/mbe.2023187 |
[10] | Salihu S. Musa, Shi Zhao, Winnie Mkandawire, Andrés Colubri, Daihai He . An epidemiological modeling investigation of the long-term changing dynamics of the plague epidemics in Hong Kong. Mathematical Biosciences and Engineering, 2024, 21(10): 7435-7453. doi: 10.3934/mbe.2024327 |
Traditional compartmental models of epidemic transmission often predict an initial phase of exponential growth, assuming uniform susceptibility and interaction within the population. However, empirical outbreak data frequently show early stages of sub-exponential growth in case incidences, challenging these assumptions and indicating that traditional models may not fully encompass the complexity of epidemic dynamics. This discrepancy has been addressed through models that incorporate early behavioral changes or spatial constraints within contact networks. In this paper, we propose the concept of "frailty", which represents the variability in individual susceptibility and transmission, as a more accurate approach to understanding epidemic growth. This concept shifts our understanding from a purely exponential model to a more nuanced, generalized model, depending on the level of heterogeneity captured by the frailty parameter. By incorporating this type of heterogeneity, often overlooked in traditional models, we present a novel mathematical framework. This framework enhances our understanding of how individual differences affect key epidemic metrics, including reproduction numbers, epidemic size, likelihood of stochastic extinction, impact of public health interventions, and accuracy of disease forecasts. By accounting for individual heterogeneity, our approach suggests that a more complex and detailed understanding of disease spread is necessary to accurately predict and manage outbreaks.
The scaling of growth during the initial phase of an infectious disease outbreak is influenced by a complex interplay of factors, many of which are not directly observable. These factors include the nature of the contact network over which the disease spreads [1,2,3,4,5], variability in individuals' risk of infection, and changes in behavior or the implementation of control measures [6]. To place our study in context within the evolution of epidemiological modeling, it is important to understand how these factors contribute to the non-linear dynamics of disease spread. Under the assumption of homogeneous mixing, a scenario where each individual has an equal chance of interacting with others, the incidence curve is expected to grow exponentially during the initial epidemic phase, provided there are no changes in susceptibility, behavior, or interventions [7]. This exponential growth presumes a constant rate intrinsic growth rate, a condition rarely met in real-world scenarios. In contrast, empirical data frequently reveal a sub-exponential growth in the early transmission phase, suggesting a more complex and heterogeneous interaction pattern among individuals. This pattern can emerge from spatial limitations within the contact network partly associated with the transmission mode of the disease, early behavioral modifications, or the introduction of public health interventions [6]. Moreover, the sub-exponential dynamic is intricately linked to the concept of "generation interval contraction", a phenomenon observed when the average time between successive cases in a chain of transmission shortens as the epidemic progresses [8]. This occurs when a susceptible individual is exposed to multiple infectious contacts, creating a competitive scenario for transmission. Such dynamics underscore the critical role of underlying network structures and behavioral responses in shaping the trajectory of an epidemic, leading to the investigation of complex network effects in epidemiological modeling [9,10,11,12,13,14].
The crucial task of accurately characterizing epidemic growth is underscored by the varied growth patterns observed in empirical data from both historical and recent outbreaks, as documented in the prior studies [15]. These diverse patterns challenge the conventional exponential growth assumption, underscoring the need for developing alternative modeling approaches that reflects the heterogeneity of epidemic processes. The two-parameter generalized-growth model (GGM) offers a nuanced method for estimating the epidemic growth scaling, employing the scaling of growth parameter 0<p<1 [15]. The exploration of this parameter space enables a deeper understanding of the underlying mechanisms driving different epidemic trajectories. Recent research further validates the utility of incorporating these nuanced growth dynamics into epidemic models. Studies have shown that models accounting for the possibility of early sub-exponential growth, rather than just assuming a simple exponential increase, significantly enhance the models' fit to empirical data and improve the accuracy of short-term epidemic forecasts [16,17,18,19,20]. This advancement in modeling aligns more closely with observed epidemic patterns and provides a more reliable foundation for predicting the course of outbreaks, thereby informing more effective public health interventions.
While the generalized-growth model captures the nuances of early sub-exponential growth patterns identified in empirical outbreak data, this model also suggests simple underlying mechanisms for these patterns. However, a comprehensive mathematical framework that integrates the classic mechanistic theories of infectious diseases with the observed variability in early growth dynamics remains elusive. To bridge this gap, our work introduces the concept of frailty from survival analysis into epidemic modeling, aiming to reflect the observed heterogeneity in individual disease susceptibility and transmission. By incorporating individual-level unobserved heterogeneity into established mechanistic transmission models, we aim to provide a more accurate and nuanced understanding of epidemic spread, better aligning theoretical predictions with empirical observations
Understanding the dynamics of epidemic growth is crucial for developing effective public health interventions. Traditional compartmental models often assume homogeneous mixing and uniform susceptibility within a population, leading to predictions of exponential growth during the initial phase of an epidemic. However, empirical observations frequently show sub-exponential growth, indicating that these assumptions may not capture the full complexity of real-world epidemic dynamics. One approach to addressing this discrepancy is the use of phenomenological models, which describe the observed growth patterns without necessarily detailing the underlying transmission mechanisms. To bridge the gap between phenomenological and mechanistic approaches, we introduce a frailty model, which incorporates individual-level heterogeneity into the epidemic growth dynamics. Frailty, a concept from survival analysis, represents unobserved variability in individual susceptibility and transmission potential. This variability can significantly impact the overall epidemic growth rate.
Population growth is described by the instantaneous increase in the total count C(t) at any given time t. This is mathematically represented as:
C′(t)=r(t)C(t). | (2.1) |
Within the realm of infectious diseases, C(t) signifies the cumulative count of infections at time t. In the context of our model, r(t) represents the instantaneous growth rate of the epidemic at time t. Unlike the constant growth rate r in the exponential model, r(t) can vary over time to reflect changes in the population's susceptibility and interaction patterns. This variation is influenced by the distribution of frailty among individuals, leading to a more nuanced understanding of epidemic growth.
The expression (2.1) has been connected with empirical models to describe population-based phenomena without "mechanically" modelling the transmission dynamics. For instance,
1) The exponential growth equation C′(t)=rC(t) is commonly used to approximate the early stages of an outbreak, assuming a very large population and during the first few generations of disease transmission.
2) The General Growth Model (GGM) as described in [6,15,21,22] is given by the growth equation
C′(t)=rC(t)p, 0≤p≤1 | (2.2) |
where the rate r(t)=r/C(t)1−p is a function that decreases over time t. Assuming the outbreak began with just one infected individual, meaning C(0)=1, there is a solution given by C(t)=(1+rθt)1/θ,where θ=1−p, leading to r(t)=r/(1+rθt). The GGM returns to the exponential growth model when θ→0.
3) The logistic growth model is described by the equation:
C′(t)=r(1−C(t)K)C(t), with r>0. | (2.3) |
Here, K=limt→∞C(t) represents the maximum possible value, known as the carrying capacity. It is evident that the growth rate, r(t)=r(1−C(t)K) also diminishes over time in accordance with the logistic rule. This can be explicitly expressed as:
r(t)=r(K−1)e−rt1+(K−1)e−rt. |
The exponential growth model is the special case when K approaches infinity.
4) The Richards model is represented by the equation:
C′(t)=r(1−[C(t)K]θ)C(t), θ>0. | (2.4) |
Beginning with an initial condition of C(0)=1, the model can also be solved analytically for C(t), leading to the expression:
r(t)=r(Kθ−1)e−rθt1+(Kθ−1)e−rθt. |
In this model, when K→∞, the growth rate decreases over time as a negative exponential function r(t)→re−rθt. It approaches constant value r as θ→0.
In these models, the growth rate r(t) phenomenologically incorporates a range of influences from both individual behaviors and environmental conditions that impact how the disease spreads. However, it is noteworthy that distinct "mechanical epidemic models" can yield identical phenomenon in terms of the cumulative growth. For instance, the logistic growth rate above may be derived from various deterministic transmission models. This includes, among others, the SI (Susceptible-Infected) and SIS (Susceptible-Infected-Susceptible) models [23].
We begin our discussion by looking into the exponential growth dynamics, which is defined by a steady growth rate, denoted as r(t)=r. This approximation of exponential growth is typical for the initial phase of an epidemic in various deterministic and stochastic models, under the assumption that the population size is infinitely large, making the depletion in susceptible individuals within the population negligible. These models further assume that mixing and transmission among the population are uniform. The growth rate, r, is then a function of additional parameters that are specific to particular mechanistic models. We will consider the following examples.
In the Simple Epidemic model, as outlined by Bailey in [24], also known as the SI model, the count of infectious persons at any specific time t is expressed by the equation I′(t)=β(1−I(t)/N)I(t). In this context, I(t) is equivalent to C(t), and N denotes the entire population size. The parameter β is the transmission rate per contact between susceptible and infectious individuals. As N tends toward infinity, C(t) converges to i0e−βt. Thus, r=β.
In the SIS and SIR (Susceptible-Infected-Recovered) models, which are framed using ordinary differential equations, the rate of change in the number of infected individuals at any given moment (time t) is described by the formula I′(t)=βS(t)I(t)/N−γI(t). In this context, N represents the total population size, and β has the same interpretation as it does in the SI model, indicating the transmission rate. S(t) and I(t) stand for the counts of susceptible and infected individuals at time t, respectively. The γ parameter represents the rate at which infected individuals recover, moving either back to being susceptible or gaining immunity. If the reduction in susceptible individuals is minor, the fraction S(t)/N can be approximated as 1. This simplification leads to the equation I′(t)=(β−γ)I(t). If β>γ, it suggests that the number of infected individuals, I(t), could increase exponentially without limit. Many standard texts denote r=β−γ, although this assumes conditions of time-homogenity, often described as being "under equilibrium conditions". In this paper, we delve deeper into these equilibrium conditions and examine whether it is accurate to model the initial growth of a disease outbreak, represented by C(t) with the equation C′(t)=(β−γ)C(t), from the outbreak's onset.
By introducing a new compartment, E(t), which tracks the number of people who are infected but not yet capable of spreading the infection as of time t, the SIS and SIR models are expanded into the SEIS and SEIR models, respectively. These models use ordinary differential equations to calculate how the numbers of latent and infectious individuals evolve over time. Specifically, the equations E′(t)=βS(t)I(t)N−αE(t) and I′(t)=αE(t)−γI(t) describe the rates of change for latent and infectious individuals at any moment. Here, the new parameter α represents the rate at which latent individuals progress to being infectious. Under the same set of equilibrium conditions as before, there exists an implicit function between r and the parameters (β,α,γ) through the equation (r+α)(r+γ)=βα. This relationship is also a recognized finding in standard mathematical epidemiology textbooks such as [25] and others.
These examples assume homogeneity so that the infection spread rate, denoted as β, remains the same for all individuals. Additionally, the natural increase rate, r, is less than or equal to β. The equation (r+α)(r+γ)=βα can be re-written as r=βαr+αrr+γ<β. The exception is the SI model, where there is no latent period and once individuals are infected, they stay infectious indefinitely, which means α=γ=0, leading to r=β.
From a stochastic viewpoint, considering C(t) as a counting process, the immediate increase of C(t) given that C(t)=n is explained as follows:
Pr{C(t+δ)=n+1|C(t)=n}=h(t)δ+o(δ)Pr{C(t+δ)=n|C(t)=n}=1−h(t)δ+o(δ)Pr{C(t+δ)>n+1|C(t)=n}=o(δ). | (2.5) |
In this context, we posit that every one of the n infected persons independently possesses a positive rate of ri(t)>0, i=1,...,n, which contributes to the emergence of a new infection. At the level of the entire population, the rate of immediate growth is represented by h(t), which is h(t)=∑ni=1ri(t). Assuming ri(t)=r(t) for all i=1,...,n, we arrive at h(t)=nr(t).
We further investigate the scenario of uninterrupted growth when the rate of change is constant r(t)=r. This situation is linked to an exponentially distributed random variable Y associated with a typical infected individual, characterized by the survival function ¯G(t)=Pr(Y>t)=e−rt. The random variable Y is well-defined and can be quantified based on the timing for each person who gets infected, thanks to its exponential distribution and the property of being memoryless.
The stochastic counterpart of the exponential growth C′(t)=rC(t) is the conditional probability
Pr{C(t+h)=n+1|C(t)=n}=nrh+o(h). |
The marginal distribution of C(t) at time t is negative binomial, with mean value satisfying the exponential growth E[C(t)]=i0ert, where i0=C(0) is the initial condition. Consequently, we arrive at the formulas:
r=ddtlogC(t)=−ddtlog¯G(t), | (2.6) |
where the first formula can be interpreted as r representing the growth rate of the total number of infected individuals; and the second formula views r as the hazard rate of the exponential distribution, considering it from the viewpoint of an average individual who is infected.
Yan and Chowell [23] highlight that, if the growth is exponential with constant rate r, it can be expressed by adjusting the transmission rate β based on the probability of individuals within the population being infectious at any particular moment, by considering a specific sample taken at time t. A binary indicator, Δi(t), is defined to indicate whether an individual i, who was infected prior to time t, is infectious at time t. This is represented by the probability Pr(Δi(t)=1), where a value of 1 signifies the individual is infectious, and a value of 0 indicating otherwise. Those individuals for whom Δi(t)=1 are grouped into what is called a "prevalence cohort". Under suitable equilibrium conditions, to be elaborated on in the following subsection, the relationship
r=βPr(Δi(t)=1) | (2.7) |
is established, indicating that the probability Pr(Δi(t)=1) stays constant, regardless of the time of sampling, t.
The expressions r=βr(r+γ)−1 and r=αβr(r+α)−1(r+γ)−1 in the aforementioned SIS, SIR, SEIS and SEIR models are the special cases of (2.7) where the durations of both the latent and infectious phases are assumed to follow exponential distributions with rates α and γ, respectively. This underlying assumption is crucial for models that utilize ordinary differential equations.
We begin by defining the random variable TI, which symbolizes the duration of infection among infected people. This duration follows a probability distribution with a density function denoted as fI(x), where x measures time starting from when a person becomes infectious. If we ignore any latent period, assuming individuals are infectious right from the infection's start and Y is independent of TI, the probability Pr(Y>TI) can be calculated using the integral ∫∞0e−rtfI(t)dt=L[fI](r), where L[fI](r) is the Laplace transform applied to the probability density function fI(x). This leads us to understand that, if all infected individuals have the same constant hazard rate r to produce new infections, then the chance of an individual causing a new infection at time t while still infectious is given by
Pr(Δi(t)=1)=Pr(Y≤TI)=1−L[fI](r). |
The probability Pr(Δi(t)=1) is established through a stochastic comparison between the random variable Y and the infectious period TI of infected individuals. Specifically, in instances where the infectious period follows an exponential distribution with an average γ−1, the expression r=β(1−L[fI](r))=βr/(r+γ) simplyfies to r=β−γ.
Now we introduce a random variable TE, representing the period of latency in individuals who are infected but not yet capable of transmitting the infection. We consider TE and TI to be sequential and independent of each other. Assuming Y is also independent from both TE and TI, we start with L[fE](r)=Pr(Y>TE) and Pr(TE<Y≤TE+TI)=Pr(Y>TE)Pr(TE<Y≤TE+TI|Y>TE). Given that the distribution of Y is exponential and memoryless, Pr(TE<Y≤TE+TI|Y>TE)=Pr(Y≤TI)=1−L[fI](r). Thus, Pr(Δi(t)=1)=Pr(TE<Y≤TE+TI) and
r=βL[fE](r)(1−L[fI](r)). | (2.8) |
Specifically, in instances where the both TE and TI ard exponentially distributed with averages α−1 and γ−1, respectively, the formula is expressed as r=β(1−L[fI](r))=βr/(r+γ).
We have assumed that all the C(t)=n infected individuals are homogeneous in the sense that they carry the same rate to produce a new infection independently from the other n−1 individuals. This rate r is further factorized in (2.7) as the product of transmission rate β and the sampling probability Pr(Δi(t)=1).
The constant growth rate implies that Pr(Δi(t)=1) is independent of time t. This is time-homogeneity. It requires that the dynamic system is at a state of equilibrium.
In the case without the latent period, (2.8) becomes r=β(1−L[fI](r)) and can be re-written as β(1−L[f]I(r))/r=1. Integration by parts, one can show that (1−L[fI](r))/r=∫∞0e−rt¯FI(t)dt, where ¯FI(x)=Pr(TI>x) is the survival function of the infectious period. Thus r=β(1−L[fI](r)) can be also written as βμI∫∞0e−rt¯FI(x)/μidt=1. Denote fw(x)=¯FI(x)/μi and R0=βμI, we have the equation
R0∫∞0e−rtfw(t)dt=1, where R0>1. | (2.9) |
The condition R0>1 is implied, because ∫∞0fw(t)dt=1 and L[fW](r)=∫∞0e−rtfW(t)dt<1.
The Eq (2.9) is recognized as the Euler-Lokta equation in mathematical biology involving two intrinsic parameters, R0 and r. R0 is widely known as the basic reproduction number. This equation links these parameters by stabilizing the dynamic system in a state of equilibrium. Furthermore, the function fw(x) adheres to the characteristics of a probability density function and is widely acknowledged as the equilibrium distribution according to renewal theory in the context of stochastic processes.
Figure 1 shows how the equilibrium distribution works, using an example where there are C(t)=8 individuals at time t, with 6 of them being infectious. As a result, the probability Pr(Δi(t)=1)=0.75. Each individual is represented by a beginning and an end point. For a given individual i where Δi(t)=1, two time-related random variables, Ui and Vi, are associated with them at time t. In renewal process, Ui refers to the backward recurrence time, which measures the time backward from t to when the individual became infectious. On the other hand, Vi represents the forward recurrence time, measuring the time from t until the individual is no longer infectious. For equilibrium to be achieved, it is necessary that Ui and Vi have the same distribution as the distribution of Wi, with each Wi being independent and identically distributed according to the equilibrium distribution, which has a probability density function denoted by fw. In addition, the distribution of the sum T(B)i=Ui+Vi is stochastically longer than that of the infectious period TI. This occurs because the distribution of infectious periods for individuals in the prevalence cohort at time t is biased towards longer durations. Individuals with longer infectious periods are more likely to be included in the cohort. The probability density of T(B)i is represented by xfI(x)/μI, which is referred to as the length-biased distribution.
Thus, under equilibrium, the following pair of relationships are equivalent:
r=β(1−L[fI](r))=βPr(Δi(t)=1)L[fW](r)=∫∞0e−rtfW(t)dt=Pr(Y>W)=1/R0 |
where the second line treats W as the forward recurrent time Vi and each of the infected individual at time t has a probability of 1/R0 failing to produce a secondary infection during the infectious period. In the special case of exponentially distributed infectious periods with μI=γ−1, the equilibrium distribution is identical to the original exponential distribution. Therefore L[fW](r)=L[fI](r)=γ/(r+γ), leading to r=β−γ=γ(R0−1).
Without further elaboration, adding a latent period TE not only gives (2.8) but also extends (2.9) as
R0L[fE](r)L[fW](r)=1, where R0>1. |
For further details, we refer to [23].
Challenges to the assumption of equilibrium conditions Even though numerous theoretical findings are based on the assumption of equilibrium conditions, it is improbable that these conditions hold true for data from the initial stages of a major epidemic outbreak. Consider the onset of an outbreak, where nearly every person infected is capable of spreading the disease, resembling the dynamics of an SI model. Here, the likelihood of an individual being sampled, Pr(Δi(t)=1), is close to 1, and the rate of infection, r, is approximately equal to β. However, as time goes on and some of the infected individuals recover by time t, the probability Pr(Δi(t)=1) drops below 1. Yet, it remains unrealistic to expect that the distributions of Ui and Vi align as they would under equilibrium conditions. When the system is not in equilibrium, the sampling probability not only varies with time t but also the likelihood of each infected individual being included in the prevalence cohort changes based on how recently they were infected relative to time t. In essence, time-nonhomogenity induces one aspect of individual heterogeneity.
The β parameter, often expressed as β=λp, where λ represents the rate of contact within the social network and p is the probability of transmission during an interaction between an infected and a susceptible person, implies several assumptions about uniformity across the interactions between agents, hosts, and the environment.
Regarding the infectious agent, like a virus in viral infections, it is assumed that there are no mutations that alters its infectiousness during the study, ensuring that infections acquired at various times confer the same level of infectiousness at the point of infection.
Concerning the hosts, it is assumed that:
1) All susceptible individuals have the same level of susceptibility;
2) An infected individuals maintain a consistent level of infectiousness throughout their infectious period;
3) All infected individuals have equal infectiousness.
In terms of the environment, it is assumed that there's uniform mixing, meaning each person is equally likely to interact with every other person in the population. In large populations, this social network can be simplified as a Bernoulli random graph like that described in Erdös and Rényi [26]. Viewing from a stochastic processes angle, the social contact network expands in a manner where the typical individual's number of contacts adheres to a stationary Poisson process with rate λ.
With these homogeneity assumptions, at any given moment during an epidemic, all infected individuals are equally infectious, regardless of when or how long ago they were infected. Each interaction between susceptible and infected individuals carries the same transmission probability p. Together, these assumptions of homogeneity in the agent-host-environment dynamic lead to the formulation β=λp. This equation defines the rate of new infections in the population as βS(t)I(t)N in deterministic models for disease transmission.
The growth rate can be expressed as r=λpδ, where δ=Pr(Δi(t)=1). Data gathered at the group level cannot distinguish λ,p and δ as distinct factors. Each factor reflects specific assumptions about homogeneity. We use a frailty model to describe variability among individuals in a phenomenological manner.
In the field of survival analysis, the proportional hazard model, denoted as ρ(t|z)=zρ0(t), accounts for variability in case individuals exhibit varying hazard rates. Here, ρ0(t)>0 represents a fundamental hazard rate, and z>0 is the frailty variable. This can also be depicted via survival functions, G(t|z)=e−zH0(t), H0(t)=∫t0ρ0(u)du.. The widely utilized Cox proportional hazard model formulates z as a log-linear regression based on observed variables.
The frailty model is a type of random effect model designed to account for unobserved heterogeneity among individuals. It posits that the variable z is random, with an expected mean of E(z)=1 and a probability density function represented by ξ(z). In scenarios where there is no variation among individuals, ξ(z) becomes a singular point where z is exactly 1, leading to no variability. Under these conditions, the survival function is defined as ¯G0(t)=e−H0(t). Consequently, when the population includes a diverse mix of individuals, the resulting survival function is derived from a combined distribution:
¯G(mixed)(t)=∫∞0¯G(t|z)ξ(z)dz=∫∞0e−zH0(t)ξ(z)dz=L[ξ](H0(t)) | (2.10) |
where L[ξ](s)=∫∞0e−zsξ(z)dz is the Laplace transform with respect to ξ(z) and L[ξ](H0(t)) is L[ξ](s) evaluated at s=H0(t). The hazard function becomes
ρ(mixed)(t)=−ddtlog¯G(mixed)(t)=−ddtlogL[ξ](H0(t)). | (2.11) |
We use the frailty framework with a fixed baseline hazard rate, denoted as ρ0(t)=r, and cumulative hazard rate H0(t)=rt. In the frailty model, the hazard rate given frailty z is ρ(t|z)=zr, leading to a mixed hazard rate expression ρ(mixed)(t)=−ddtlogL[ξ](rt). The following general observations are made:
1) For any non-degenerate probability density function ξ(z), the Laplace transform of ξ at rt, satisfies L[ξ](rt)=∫∞0e−zrtξ(z)dz≥e−rt. Referring to (2.6) when z is constantly 1, r=ddtlogC(t)=−ddtlog¯G(t), leading to the relationship C(t)=¯G(t)−1. Introducing the random effect (frailty) ξ(z) and assuming C(0)=1, we have ddtlogC(t)=−ddtlogL[ξ](rt), resulting in the inequality
C(t)=[L[ξ](rt)]−1≤ert for all t>0, | (2.12) |
indicating that the growth of C(t) is limited by the exponential function ert from above.
2) It has been demonstrated [27] that L[ξ](rt) is log-convex. As a survival function, its hazard rate ρ(t)=−ddtlogL[ξ](rt) decreases monotonically from an initial value of r at time 0. This reflects a decrease in the effective reproduction number, Rt, during the initial phase of epidemic growth, as noted by [21].
3) When comparing the variability of z using convex order (as detailed in the Appendix), it is found that higher variability in z results in a higher value of the Laplace transform L[ξ](s) for all s>0. This, in turn, means that the more variable z is, the higher the value of L[ξ](rt) and thus, the smaller the value of C(t) for any t>0.
Let ξ(z) be the p.d.f. of the Gamma distribution with E(z)=1 and variance var[z]=v>0. The Laplace transform is L[ξ](s)=(1+sν)−1/v. Letting C(0)=1, the expression C(t)=[L[ξ](rt)]−1 becomes
C(t)=(1+vrt)1/v, for all t>0. | (2.13) |
Meanwhile the instantaneous growth function is
r(t)=−ddtlogL[ξ](rt)=r1+vrt. |
Figure 2 illustrate r(t) and C(t) at different values of ν>0. The parameter r is a time-scale parameter and the x-axes in these plots are scaled to τ=rt. We focus on the range 0<v<1. During this range, (1+rvt)1/v is the same as the GGM (2.2) by letting v=1−p and C′(t)=[r/{1+r(1−p)t}]C(t) can be re-written as C′(t)=r(1+r(1−p)t)p1−p=rC(t)p, corresponding to the phenomenological model (2.2). When ν=1, the linear growth C(t)=1+rt corresponds to ¯G(mixed)(t)=[L[ξ](rt)]=(1+rt)−1 recognized as the survival function of a (continuous time) power-law distribution, analogous to the discrete power-law distribution in social network analysis. C(t) is a convex increasing function bounded by the exponential function from above and the linear function from below. This is the sub-exponential growth feature [6].
We also draw a connection to previous studies [28,29], which demonstrate that an initial Gamma distribution of agent susceptibility and infectivity in the SIR model leads to power-law sub-exponential growth. These results, although derived through a different theoretical pathways, aligns with our findings on frailty-induced heterogeneity using a Gamma distribution. This similarity suggests a deeper link between different approaches to modeling heterogeneity in epidemics. it is also worth noting the recent work by
If ξ(z) be the p.d.f. of an inverse-Gaussian distribution with E(z)=1 and variance var[z]=v, the Laplace transform is L[ξ](s)=e(1−√1+2vs)/v. Letting C(0)=1, the expression (2.12) becomes
C(t)=[L[ξ](rt)]−1=e(√1+2vrt−1)/v, v>0 | (2.14) |
and C(t)→ert as v→0. Meanwhile,
r(t)=C′(t)C(t)=r√1+2vrt. |
However, the difference between C(t) given in (2.14) and C(t) given in (2.13) is small:
e(√1+2vrt−1)/v−(1+vrt)1/v=16v2r3t3+O(t4). |
It can be shown that, if E(z)=1 and variance var[z]=v>0, e(√1+2vrt−1)/v≥(1+vs)1/v for all s>0.
It is a special case of a more general result [30]:
e(1−√1+2vs)/v≤(1+vGs)−1/vG, for all v≤vG and s>0 | (2.15) |
where v on the left hand is the variance of an inverse-Gaussian distribution and vG on the right hand is the variance of a gamma distribution satisfying v≤vG. In both distributions, it is assumed the mean value E[z]=1. Relating (2.15) to C(t)=[L[ξ](rt)]−1, it implies that if one compares two growth curves CIG(t) and CGAM(t), generated by a frailty model with random effect distribution ξ(z) chosen from an inverse-Gaussian distribution with variance v and from a gamma distribution with variance vG, respectively, if v≤vG, CIG(t)≥CGAM(t).
If we replace the left hand side in the inequality (2.15) by the Laplace transform of a log-normal distribution with variance v, denoted by LLogN[ξ](s), the following statement is still true.
LLogN[ξ](s)≤(1+vGs)−1/vG, for all v≤vG and s>0. | (2.16) |
To show this, we briefly introduce Lκ-class distributions.
Definition 1. The distribution for X>0 with p.d.f. f(x) and finite mean μ is said to belong to the Lκ-class of distributions, if
L[f](s)=∫∞0e−sxfX(x)dx≤(1+μsκ)−κ. |
The p.d.f. of a log-normal distribution of a random variable X can be written as fX(x;γ,ς)=ς√2πxe−(ςlog(γx))22 [31] with mean E[X]=1γexp(12ς2) and variance var[X]=1γ2e1ς2(e1ς2−1). The gamma distribution with mean value μG and shape parameter κ has the variance expression vG=μ2G/κ. We compare a log-normal distribution with a gamma distribution with equal mean values and let μG=1γexp(12ς2). The condition var[X]≤μ2G/κ becomes e1ς2≤1+1κG. Immediately following Proposition 3.4 in [30], if ς−2≤log(1+1κ), the log-normal distribution belongs to the Lκ-class.
Applying the above to ξ(z) with mean μ=1, then vG=1/κ, the condition ς−2≤log(1+1κ) is v≤vG and the inequality (2.16) holds even though LLogN[ξ](s) does not have explicit expression. Since C(t)=[L[ξ](rt)]−1, it implies that if one compares two growth curves CIogN(t) and CGAM(t), generated by a frailty model with random effect distribution ξ(z) chosen from a log-normal distribution with variance v and from a gamma distribution with variance vG, respectively, if v≤vG, CIogN(t)≥CGAM(t).
With respect to the benchmark vG=1, of the three distributions for ξ(z), when E(z)=1 and variance var[z]=v≤1, they all belong to the L1−class satisfying L[ξ](s)≤(1+s)−1. This gives C(t)=[L[ξ](rt)]−1≥1+rt. We can add the Weibull distribution for ξ(z) into this class because [30] has also shown that the Weibull distribution with E(z)=1 and variance var[z]=v≤1 belongs to the L1−class. Therefore, for four distributions for ξ(z): the gamma, the inverse-Gaussian, the log-normal and the Weibull distribution, when E(z)=1 and variance var[z]=v≤1, C(t)=[L[ξ](rt)]−1 satisfies 1+rt≤C(t)<ert.
It is worth noting that a power-law sub-exponential diffusion growth has been demonstrated on the recent COVID-19 epidemic (for example in [32,33,34,35,36]).
Most popular compartmental epidemic models treat populations as homogeneous, with only a few key differences considered, such as age, gender, and location [7]. However, capturing all the factors that influence the transmission dynamics of infectious diseases in these models is practically impossible. This is partly because some relevant factors might be unknown, a challenge frequently encountered in epidemiology. Our analysis employs the frailty model from survival analysis, offering a robust framework for addressing this issue. This model is suitable for incorporating unobserved heterogeneity into the study of epidemic growth dynamics, without the need to define the specific mechanisms. By doing so, it allows us to explore the dynamic impacts of these unaccounted-for variables on disease transmission, providing insights that are not obtained from conventional models.
The frailty model has found increasing use across various applications, notably in epidemiology. In our study, we leverage the concept of frailty to provide a straightforward explanation for the observed diversity in the early growth dynamics of epidemics across different infectious diseases [15]. This approach allows us to delve into the phenomenon of sub-exponential epidemic growth by acknowledging the presence of unseen internal and external factors. These factors contribute to individual-level heterogeneity, a complexity that traditional mechanistic frameworks struggle to fully encompass.
The essence of the frailty framework lies in its ability to capture information about elusive individual heterogeneity through the structure of conditional hazards and the distribution of frailty (or heterogeneity) among individuals. However, a significant challenge we face is that of identifiability. Specifically, the hazard function (2.11) when the population arises from a random mixture of heterogeneous individuals involves a baseline hazard h0(t) and a random effect distribution ξ(z). Thus, different combinations of h0(t) and ξ(z) may produce the same marginal hazard rate h(mixed)(t). To overcome this issue, we propose limiting the baseline hazard rate to a constant value, h0(t)=r and also confining ξ(z) to a specific family of distributions, such as the gamma distribution.
By incorporating individual unobserved heterogeneity into standard mechanistic compartmental epidemic models through the frailty framework from survival analysis offers a significant opportunity to understand the consequences of heterogeneity on infectious disease dynamics and control. Moreover, the use of different statistical distributions to represent frailty highlights the flexibility and robustness of our approach. The connection to previous work [28,29] further underscores the relevance and potential of incorporating heterogeneity in epidemic modeling. For instance, Novozhilov (2008) examined the spread of epidemics in a closed heterogeneous population by introducing a model that incorporates individual variability in susceptibility and infectivity through an initial Gamma distribution. He demonstrated that this variability leads to power-law sub-exponential growth, highlighting how heterogeneity can significantly alter epidemic trajectories.
While incorporating individual heterogeneity into epidemic models enhances their accuracy and predictive power, it also increases their complexity. This complexity can pose challenges in terms of model availability and computational feasibility. Therefore, a careful balance must be struck between the level of detail included and the practical utility of the model. Our approach aims to provide a nuanced understanding of epidemic dynamics through the concept of frailty, while maintaining a level of simplicity that ensures the model's applicability and ease of use.
In this paper we have distinguished the growth rate r as a scale parameter of time in the growth curve function from the instantaneous growth function r(t) given in (2.1). Importantly, our work here and a recent study [37] suggests that mathematical analyses that rely on the integration of mechanistic epidemic models and the frailty model are often feasible. For instance, in [37] authors incorporated the frailty framework into mechanistic models to explore the impact of individual variability in the level of compliance on a control intervention. Immediate research in this direction include studies that extend specific mechanistic models with the frailty framework to better understand the impact of individual unobserved heterogeneity on estimates of the reproduction number, epidemic size, stochastic extinction as well as the accuracy of epidemic forecasts.
The proposed frailty model provides a comprehensive mathematical framework to incorporate unobserved heterogeneity into epidemic growth models. By doing so, it bridges the gap between phenomenological observations and mechanistic theories, offering a more nuanced understanding of epidemic spread. This approach aligns with related work in the field, such as Novozhilov (2008) [28], and suggests promising directions for future research in modeling epidemic heterogeneity.
In summary, we demonstrate how the variability in early epidemic growth, ranging from sub-exponential to exponential, can be mathematically derived by incorporating individual unobserved heterogeneity into simple mechanistic models. This is achieved using the frailty model from survival analysis. By adopting the standard assumption that heterogeneity is gamma-distributed, we have derived a generalized growth equation based on the variance of the frailty distribution. These findings are in line with previous work [6], emphasizing the importance of including detailed individual-level characteristics, including dynamic contact networks, in transmission models to aim to capture sub-exponential epidemic growth dynamics.
The authors declare they have not used Artificial Intelligence (AI) tools in the creation of this article.
The authors declare there is no conflict of interest.
If T1 and T2 have equal mean value μ1=μ2 (should they exist), a general description of variability is based on "marjorization". Let f1 and f2 are the corresponding p.d.f. for T1 and T2, the verbal description for T2 being more dispersed (spread out) than T1 is reflected in Figure A1 about the change of signs between. f1 and f2 and their corresponding survival functions ¯F1 and ¯F2.
The verbal and graphic description gives the following definition:
Definition 2. T1≤cv T2 if and only if μ1=μ2 plus the following two statements:
1) f2(x)−f1(x) has two sign changes and the sign sequence is: +,−,+ (see Figure A1).
2) ¯F1(x)−¯F2(x) has one sign change and the sign sequence is: +,− (see Figure A1).
The above definition is verbal and has been proven to be equivalent to the following definition in mathematical terms:
Definition 3. T1≤cv T2 if E[Ψ(T1)]≤E[Ψ(T2)] for all convex functions Ψ(x) for which these expectations exist.
Therefore, the order T1≤cv T2 is called the convex order.
1) The convex order implies the ordering according to variance var(T)=E[(T−μ)2] because x2 is a convex function.
2) It also implies the ordering according to the Laplace transform E[e−sT] for all s>0, because e−sx is a convex function.
[1] |
L. Meyers, Contact network epidemiology: Bond percolation applied to infectious disease prediction and control, Bull. Am. Math. Soc., 44 (2007), 63–86. https://doi.org/10.1090/S0273-0979-06-01148-7 doi: 10.1090/S0273-0979-06-01148-7
![]() |
[2] |
J. O. Lloyd-Smith, S. J. Schreiber, P. E. Kopp, W. M. Getz, Superspreading and the effect of individual variation on disease emergence, Nature, 438 (2005), 355–359. https://doi.org/10.1038/nature04153 doi: 10.1038/nature04153
![]() |
[3] | L Danon, A. P. Ford, T. House, C. P. Jewell, M. J. Keeling, G. O. Roberts, et al., Networks and the epidemiology of infectious disease, Interdiscip. Perspect. Infect. Dis., 2011 (2011), 284909. |
[4] | M. J. Keeling, K. T. Eames, Networks and epidemic models, J. R. Soc. Int., 2 (2005), 295–307. |
[5] | S. Towers, Z. Feng, Social contact patterns and control strategies for influenza in the elderly, Math. Biosci., 204 (2012), 241–249. |
[6] |
G. Chowell, L. Sattenspiel, S. Bansal, C. Viboud, Mathematical models to characterize early epidemic growth: A Review, Phys. Life Rev., 18 (2016), 66–97. https://doi.org/10.1016/j.plrev.2016.07.005 doi: 10.1016/j.plrev.2016.07.005
![]() |
[7] | O. Diekmann, J. Heesterbeek, Mathematical Epidemiology of Infectious Diseases: Model Building, Analysis and Interpretation, Wiley, 2000. |
[8] |
E. Kenah, M. Lipsitch, J. M. Robins, Generation interval contraction and epidemic data analysis, Math. Biosci., 213 (2008), 71–79. https://doi.org/10.1016/j.mbs.2008.02.007 doi: 10.1016/j.mbs.2008.02.007
![]() |
[9] |
R. Pastor-Satorras, C. Castellano, P. Van Mieghem, A. Vespignani, Epidemic processes in complex networks, Rev. Mod. Phys., 87 (2015), 925–979. https://doi.org/10.1103/RevModPhys.87.925 doi: 10.1103/RevModPhys.87.925
![]() |
[10] |
M. J. Keeling, The effects of local spatial structure on epidemiological invasions, Proc. R. Soc. London Ser. B Biol. Sci., 266 (1999), 859–867. https://doi.org/10.1098/rspb.1999.0716 doi: 10.1098/rspb.1999.0716
![]() |
[11] |
M. Barthélemy, A. Barrat, R. Pastor-Satorras, A. Vespignani, Dynamical patterns of epidemic outbreaks in complex heterogeneous networks, J. Theor. Biol., 235 (2005), 275–288. https://doi.org/10.1016/j.jtbi.2005.01.011 doi: 10.1016/j.jtbi.2005.01.011
![]() |
[12] |
Y. Wang, Z. Wei, J. Cao, Epidemic dynamics of influenza-like diseases spreading in complex networks, Nonlinear Dyn., 101 (2020), 1801–1820. https://doi.org/10.1007/s11071-020-05867-1 doi: 10.1007/s11071-020-05867-1
![]() |
[13] | P. Ji, J. Ye, Y. Mu, W. Lin, Y. Tian, C. Hens, et al., Signal propagation in complex networks, Phys. Rep., 1017 (2023), 1–96. |
[14] |
O. Artime, M. Grassia, M. De Domenico, J. P. Gleeson, H. A. Makse, G. Mangioni, Robustness and resilience of complex networks, Nat. Rev. Phys., 6 (2024), 114–131. https://doi.org/10.1038/s42254-023-00676-y doi: 10.1038/s42254-023-00676-y
![]() |
[15] |
C. Viboud, L. Simonsen, G. Chowell, A generalized-growth model to characterize the early ascending phase of infectious disease outbreaks, Epidemics, 15 (2016), 27–37. https://doi.org/10.1016/j.epidem.2016.01.002 doi: 10.1016/j.epidem.2016.01.002
![]() |
[16] |
R. Bürger, G. Chowell, L. Y. Lara-Díaz, Measuring differences between phenomenological growth models applied to epidemiology, Math. Biosci., 334 (2021), 108558. https://doi.org/10.1016/j.mbs.2021.108558 doi: 10.1016/j.mbs.2021.108558
![]() |
[17] | A. Smirnova, L. deCamp, G. Chowell, Forecasting epidemics through nonparametric estimation of time-dependent transmission rates using the SEIR model, Bull. Math. Biol., 81 (2019), 4343–4365. |
[18] | D. Shanafelt, G. Jones, M. Lima, C. Perrings, G. Chowell, Forecasting the foot-and-mouth disease epidemic in the United Kingdom, EcoHealth, forthcoming. |
[19] |
B. Pell, Y. Kuang, C. Viboud, G. Chowell, Using phenomenological models for forecasting the 2015 Ebola challenge, Epidemics, 22 (2018), 62–70. https://doi.org/10.1016/j.epidem.2016.11.002 doi: 10.1016/j.epidem.2016.11.002
![]() |
[20] | G. Chowell, D. Hincapie-Palacio, J. Ospina, B. Pell, A. Tariq, S. Dahal, et al., Using phenomenological models to characterize transmissibility and forecast patterns and final burden of Zika epidemics, PLOS Curr., 8 (2016). |
[21] |
G. Chowell, C. Viboud, L. Simonsen, S. Moghadas, Characterizing the reproduction number of epidemics with early sub-exponential growth dynamics, J. R. Soc. Interface, 13 (2016), 20160659. https://doi.org/10.1098/rsif.2016.0659 doi: 10.1098/rsif.2016.0659
![]() |
[22] | G. Chowell, C. Viboud, Is it growing exponentially fast?–Impact of assuming exponential growth for characterizing and forecasting epidemics with initial near-exponential growth dynamics, Infect. Dis. Model., 1 (2016), 71–78. |
[23] | P. Yan, G. Chowell, Quantitative methods for investigating infectious disease outbreaks, Cham, Switzerland, Springer, 2019. |
[24] | N. T. J. Bailey, The Mathematical Theory of Infectious Diseases and Its Applications, Second Edition, The Griffin & Company Ltd, 1975. |
[25] | F. Brauer, C. Castillo-Chávez, Mathematical Models in Population Biology and Epidemiology, Springer, 2001. |
[26] | P. Erdös, A. Rényi, On the evolution of random graphs, Bull. Inst. Int. Stat., 38 (1961), 343–347. |
[27] | A. W. Marshall, I. Olkin, Life Distributions: Structure of Nonparametric, Semiparametric and Parametric Families, Springer, 2007. |
[28] |
A. S. Novozhilov, On the spread of epidemics in a closed heterogeneous population, Math. Biosci., 215 (2008), 177–185. https://doi.org/10.1182/asheducation-2008.1.177 doi: 10.1182/asheducation-2008.1.177
![]() |
[29] |
C. Rose, A. J. Medford, C. F. Goldsmith, T. Vegge, J. S. Weitz, A. A. Peterson, Heterogeneity in susceptibility dictates the order of epidemic models, J. Theor. Biol., 528 (2021), 110839. https://doi.org/10.1016/j.jtbi.2021.110839 doi: 10.1016/j.jtbi.2021.110839
![]() |
[30] |
B. Klar, A note on the L-class of life distributions, J. Appl. Prob., 39 (2002), 11–19. https://doi.org/10.1239/jap/1019737984 doi: 10.1239/jap/1019737984
![]() |
[31] | J. D. Kalbfleisch, R. L. Prentice, Statistical Analysis for Failure Time Data, Second Edition, Wiley & Sons, New York, 2002. |
[32] | N. L. Komarova, L. M. Schang, D. Wodarz, Patterns of the COVID-19 pandemic spread around the world: exponential versus power laws, J. R. Soc. Interface, 17 (2020), 20200518. |
[33] |
D. G. Xenikos, A. Asimakopoulos, Power-law growth of the COVID-19 fatality incidents in Europe, Infect. Dis. Modell., 6 (2021), 743–750. https://doi.org/10.1016/j.idm.2021.05.001 doi: 10.1016/j.idm.2021.05.001
![]() |
[34] | A. Tariq, E. A. Undurraga, C. C. Laborde, K. Vogt-Geisse, R. Luo, R. Rothenberg, et al., Transmission dynamics and control of COVID-19 in Chile, March-October, 2020. PLoS Neglected Trop. Dis., 15 (2021), e0009070. https://doi.org/10.1371/journal.pntd.0009070 |
[35] |
G. Chowell, S. Dahal, A. Tariq, K. Roosa, J. M. Hyman, R. Luo, An ensemble n-sub-epidemic modeling framework for short-term forecasting epidemic trajectories: Application to the COVID-19 pandemic in the USA, PLoS Comput. Biol., 18 (2022), e1010602. https://doi.org/10.1371/journal.ppat.1010602 doi: 10.1371/journal.ppat.1010602
![]() |
[36] | G. Chowell, R. Rothenberg, K. Roosa, A. Tariq, J. M. Hyman, R. Luo, Sub-epidemic model forecasts during the first wave of the COVID-19 pandemic in the USA and European hotspots, in Mathematics of Public Health: Proceedings of the Seminar on the Mathematical Modelling of COVID-19, (2022), 85–137. |
[37] |
P. Yan, A frailty model for intervention effectiveness against disease transmission when implemented with unobservable heterogeneity, Math. Biosci. Eng., 15 (2018), 275–298. https://doi.org/10.3934/mbe.2018012 doi: 10.3934/mbe.2018012
![]() |
1. | Gerardo Chowell, Pavel Skums, Investigating and forecasting infectious disease dynamics using epidemiological and molecular surveillance data, 2024, 51, 15710645, 294, 10.1016/j.plrev.2024.10.011 |