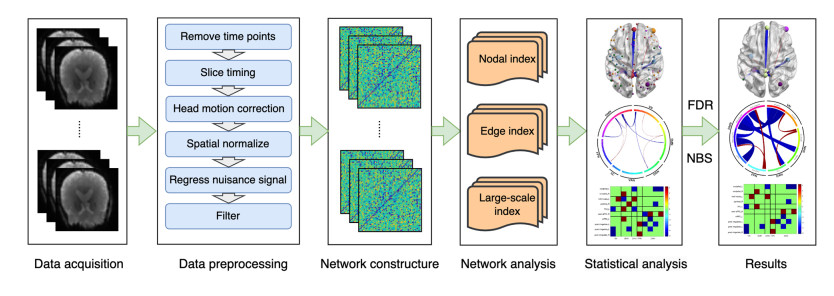
Our aim was to explore the aberrant intrinsic functional topology in methamphetamine-dependent individuals after six months of abstinence using resting-state functional magnetic imaging (rs-fMRI). Eleven methamphetamines (MA) abstainers who have abstained for six months and eleven healthy controls (HC) were recruited for rs-fMRI examination. The graph theory and functional connectivity (FC) analysis were employed to investigate the aberrant intrinsic functional brain topology between the two groups at multiple levels. Compared with the HC group, the characteristic shortest path length (Lp) showed a significant decrease at the global level, while the global efficiency (Eglob) and local efficiency (Eloc) showed an increase considerably. After FDR correction, we found significant group differences in nodal degree and nodal efficiency at the regional level in the ventral attentional network (VAN), dorsal attentional network (DAN), somatosensory network (SMN), visual network (VN) and default mode network (DMN). In addition, the NBS method presented the aberrations in edge-based FC, including frontoparietal network (FPN), subcortical network (SCN), VAN, DAN, SMN, VN and DMN. Moreover, the FC of large-scale functional brain networks revealed a decrease within the VN and SCN and between the networks. These findings suggest that some functions, e.g., visual processing skills, object recognition and memory, may not fully recover after six months of withdrawal. This leads to the possibility of relapse behavior when confronted with MA-related cues, which may contribute to explaining the relapse mechanism. We also provide an imaging basis for revealing the neural mechanism of MA-dependency after six months of abstinence.
Citation: Xiang Li, Jinyu Cong, Kunmeng Liu, Pingping Wang, Min Sun, Benzheng Wei. Aberrant intrinsic functional brain topology in methamphetamine-dependent individuals after six-months of abstinence[J]. Mathematical Biosciences and Engineering, 2023, 20(11): 19565-19583. doi: 10.3934/mbe.2023867
[1] | Guixiang Liang, Xiang Li, Hang Yuan, Min Sun, Sijun Qin, Benzheng Wei . Abnormal static and dynamic amplitude of low-frequency fluctuations in multiple brain regions of methamphetamine abstainers. Mathematical Biosciences and Engineering, 2023, 20(7): 13318-13333. doi: 10.3934/mbe.2023593 |
[2] | Banghua Yang, Xuelin Gu, Shouwei Gao, Ding Xu . Classification accuracy and functional difference prediction in different brain regions of drug abuser prefrontal lobe basing on machine-learning. Mathematical Biosciences and Engineering, 2021, 18(5): 5692-5706. doi: 10.3934/mbe.2021288 |
[3] | Chaofan Song, Tongqiang Liu, Huan Wang, Haifeng Shi, Zhuqing Jiao . Multi-modal feature selection with self-expression topological manifold for end-stage renal disease associated with mild cognitive impairment. Mathematical Biosciences and Engineering, 2023, 20(8): 14827-14845. doi: 10.3934/mbe.2023664 |
[4] | Xian Hua, Jing Li, Ting Wang, Junhong Wang, Shaojun Pi, Hangcheng Li, Xugang Xi . Evaluation of movement functional rehabilitation after stroke: A study via graph theory and corticomuscular coupling as potential biomarker. Mathematical Biosciences and Engineering, 2023, 20(6): 10530-10551. doi: 10.3934/mbe.2023465 |
[5] | Changwei Gong, Bing Xue, Changhong Jing, Chun-Hui He, Guo-Cheng Wu, Baiying Lei, Shuqiang Wang . Time-sequential graph adversarial learning for brain modularity community detection. Mathematical Biosciences and Engineering, 2022, 19(12): 13276-13293. doi: 10.3934/mbe.2022621 |
[6] | Qian Zheng, Xiaojuan Ba, Yiyang Xin, Jiaofen Nan, Xiao Cui, Lin Xu . Functional division of the dorsal striatum based on a graph neural network. Mathematical Biosciences and Engineering, 2024, 21(2): 2470-2487. doi: 10.3934/mbe.2024109 |
[7] | Liqiang Zhu, Ying-Cheng Lai, Frank C. Hoppensteadt, Jiping He . Characterization of Neural Interaction During Learning and Adaptation from Spike-Train Data. Mathematical Biosciences and Engineering, 2005, 2(1): 1-23. doi: 10.3934/mbe.2005.2.1 |
[8] | Hacı İsmail Aslan, Hoon Ko, Chang Choi . Classification of vertices on social networks by multiple approaches. Mathematical Biosciences and Engineering, 2022, 19(12): 12146-12159. doi: 10.3934/mbe.2022565 |
[9] | Edil D. Molina, Paul Bosch, José M. Sigarreta, Eva Tourís . On the variable inverse sum deg index. Mathematical Biosciences and Engineering, 2023, 20(5): 8800-8813. doi: 10.3934/mbe.2023387 |
[10] | Wei Kong, Feifan Xu, Shuaiqun Wang, Kai Wei, Gen Wen, Yaling Yu . Application of orthogonal sparse joint non-negative matrix factorization based on connectivity in Alzheimer's disease research. Mathematical Biosciences and Engineering, 2023, 20(6): 9923-9947. doi: 10.3934/mbe.2023435 |
Our aim was to explore the aberrant intrinsic functional topology in methamphetamine-dependent individuals after six months of abstinence using resting-state functional magnetic imaging (rs-fMRI). Eleven methamphetamines (MA) abstainers who have abstained for six months and eleven healthy controls (HC) were recruited for rs-fMRI examination. The graph theory and functional connectivity (FC) analysis were employed to investigate the aberrant intrinsic functional brain topology between the two groups at multiple levels. Compared with the HC group, the characteristic shortest path length (Lp) showed a significant decrease at the global level, while the global efficiency (Eglob) and local efficiency (Eloc) showed an increase considerably. After FDR correction, we found significant group differences in nodal degree and nodal efficiency at the regional level in the ventral attentional network (VAN), dorsal attentional network (DAN), somatosensory network (SMN), visual network (VN) and default mode network (DMN). In addition, the NBS method presented the aberrations in edge-based FC, including frontoparietal network (FPN), subcortical network (SCN), VAN, DAN, SMN, VN and DMN. Moreover, the FC of large-scale functional brain networks revealed a decrease within the VN and SCN and between the networks. These findings suggest that some functions, e.g., visual processing skills, object recognition and memory, may not fully recover after six months of withdrawal. This leads to the possibility of relapse behavior when confronted with MA-related cues, which may contribute to explaining the relapse mechanism. We also provide an imaging basis for revealing the neural mechanism of MA-dependency after six months of abstinence.
Methamphetamine (MA) is a new synthetic compound with strong central euphoric effects [1]. The prevalence of MA abuse is continuously increasing and ranking as the second most widely used illicit drug globally due to its ease of production, which has become a global public health problem [2]. As an amphetamine-type stimulant, MA abuse can cause various physical illnesses and psychiatric deficits [3]. Moreover, even after undergoing treatment for substance abuse, patients often relapse when they encounter high-risk environments that may trigger drug abuse, severely impacting families and society [4]. However, the exact relapse mechanisms of MA addiction have not been fully explored [5]. The main reason is that we have not clarified the altered functional brain imaging mechanism in MA withdrawal patients. Therefore, how to explore alterations in functional brain imaging and, thus, potential mechanisms of relapse in MA abstainers has become a hot topic.
Magnetic resonance imaging (MRI) is essential for clinical and brain science research [6,7]. Numerous studies have shown that long-term abuse causes irreversible damage to brain function and induces psychiatric symptoms or cognitive deficits [8,9,10]. After a period of withdrawal, the structure and function of the brain can be improved or restored to a certain extent [11,12]. For example, Zhang et al. [13] focus on the brain structure change in long-term abstinence of MA by voxel-based morphometry. They found differences in grey matter volumes in the cerebellum and right thalamus compared with normal subjects. In addition, they suggested that prolonged abstinence benefits cognitive function recovery. By the seed-based functional connectivity (FC) analysis, Li et al. [14] found disrupted FC between the cerebellum and several cerebral functional networks in addicts after six months of abstinence. Based on diffusion-tensor imaging, Fan et al. [15] revealed abnormal microstructures of several brain regions in MA individuals following prolonged withdrawal by voxel-based analysis.
Among various methods to explore brain changes, graph theory is an efficient tool for whole-brain analysis to detect functional differences [16,17]. This method not only measures the connectivity between brain regions, but also allows the detection and quantification of changes in the characteristic properties of each brain region to explore microscopic changes in the central system [18]. Hence, it is widely used in research to explore MA addiction or relapse mechanisms [19,20]. In a study of diffusion-tensor imaging, Zhou et al. [21] found that the brains of male addicts have small-world networks, with significantly efficient transmission and integration of information between brain regions. Mansoory et al. [22] adopted resting-state fMRI and graph theory to investigate the FC and local efficiency in the left and right hemispheres of the brain. They demonstrated the alterations in the default mode network (DMN), executive control network (ECN) and salience network (SN) in both hemispheres of the brain. Generally, these studies have shown structural or functional abnormalities in the brain of addicts in withdrawal at the topological level. This allows for a better understanding of abnormalities in information interaction between brain regions and coordination and cooperation between brain networks [23]. However, most relevant studies focus on an extended range of withdrawal, i.e., they did not focus on investigating a particular point in time. Studying brain abnormalities at specific withdrawal time points, such as the six-month voluntary MA withdrawal period in China, may better explain the mechanisms of relapse [14].
To the best of our knowledge, few functional brain imaging studies have explored functional alterations after six months of abstinence at the topological level. Consider that the brain changes during short-term withdrawal, i.e., less than six months, may reflect a compensatory response to MA rather than an actual recovery pathway. In the current study, we employed graph theory and FC analysis to explore aberrant intrinsic functional topology in MA-dependent individuals after six months of abstinence. We hypothesize that different brain areas remain dysfunctional, possibly leading to dysregulation of executive control, emotional processing and sensorimotor functions in MA-dependent individuals after six months of abstinence, which may provide imaging evidence for abnormal brain topology and a better understanding of the underlying relapse mechanisms.
To explore the aberrant intrinsic functional topology in MA-dependent individuals after six months of abstinence, we first acquired fMRI data from the subjects and preprocessed it. Then, we use the atlas to construct and analyze the individual brain networks according to three dimensions: Nodes, edges and large-scale brain networks. Finally, a two-sample t-test was performed on the different metrics of the two groups, corrected for multiple comparison methods, such as false discovery rate (FDR) or network-based statistic (NBS), to find the significant differences in brain regions. The flowchart of the study is shown in Figure 1.
We recruited 11 male MA abstainers and 11 age- and sex-matched HCs for the present study. The MA abstainers were recruited from the Shandong Detoxification Monitoring and Treatment Institute, while the HCs were recruited from the community. MA abstainers were diagnosed according to the Diagnostic and Statistical Manual on Mental Disorders (DSM-V) criteria and after that had received a six-month voluntary abstinence period. During withdrawal, addicts were given medication and physical exercises and showed no significant withdrawal symptoms by the end of the period.
The inclusion criteria included males, right-handed, ranging in age from 20 to 30 years old, without a history of psychiatric or neurological disorders, not taking medication two weeks before data collection that could affect the experiment results and informed consent. The exclusion criteria included subjects with severe aphasia, cognitive loss or inability to communicate, a history of alcohol or drug abuse, previous or existing severe liver or kidney damage or cardiovascular disease or organic mental disorders, contraindications to MRI scannings, such as the presence of metal objects, pacemakers, metal dentures and claustrophobia. In addition, the abstainers abuse only one MA drug and escape the physiological detoxification period with a negative MA urine test after six months of abstinence.
This study was approved by the Ethics Committee of the Affiliated Hospital of Shandong University of Traditional Chinese Medicine (Ethics No. 2018-078). All volunteers were informed of the experimental procedure and wrote the informed consent before scanning.
Brain imaging was performed on a Philips Ingenia 3.0T magnetic resonance scanner. The fMRI data were acquired using the T2 echo planar imaging sequence with the following parameters: TR = 2000 ms, TE = 30 ms, rotation angle = 90°, matrix = 64 × 64, FOV = 220 mm × 220 mm, slice thickness = 3 mm, thickness interval = 1 mm. After resting-state scanning, structural images of each subject were also collected for subsequent spatial alignment using the fast acquisition gradient echo sequence with the following parameters: Rotation angle = 9°, TR = 2300 ms, TE = 3.01 ms, matrix = 256 × 256, slice thickness = 1 mm. Foam padding and earplugs were used to reduce head motion and scanner noise. The scans were reviewed by two experienced neuroradiologists and showed no serious neuroanatomical abnormalities or image distortions associated with head movements in any of the participants.
The fMRI data were automatically preprocessed using the Data Processing and Analysis for Brain Imaging (DPABI) toolbox based on MATLAB with the standard process [24]. First, the first 10 scan time points data were removed to ensure scan signal stability. The remaining images were corrected by the slice-timing and head motion. They were then segmented and normalized to the standard Montreal Neurological Institute (MNI) space using the DARTEL tool. After alignment, the image is smoothed using a full-width half-height kernel to increase the signal-to-noise ratio. Then, the nuisance signals were regressed, including white matter signals and cerebrospinal fluid signals, linear trends and 24 Friston head movement parameters. The functional images were bandpass filtered (0.01–0.1 Hz).
The exclusion criteria were subjects with maximum head movement amplitude larger than 3 mm or rotation larger than 3° in the X, Y and Z axes. Furthermore, subjects with mean frame-wise displacement (mean FD) larger than 0.2 mm were also excluded from further analyses (P>0.05) [25].
We construct functional brain networks from node and edge perspectives via the Data Processing and Analysis for Brain Imaging Network analysis (DPABINet) toolbox, which has evolved from DPABI [26]. The functional brain networks of the node represent the different brain regions, while the edge represents the FC among the various brain regions. The Dosenbach atlas [27], which defines 160 ROIs across the whole brain, was used to segment the brain region. Since all scans did not fully cover the cerebellum, we excluded them from the analysis. After removing 18 brain regions from the cerebellum, 142 ROIs were retained. For each ROI, the mean BOLD signal intensity of the ROI was extracted, and the Pearson correlation coefficient of the time series between any ROIs was calculated to construct a whole-brain FC matrix, which has a dimension of 142 × 142 and contains a total of 10,011 edges. Then, we converted the FC matrix to z-scores using Fisher's r-to-z, making the matrix more normal.
Global and nodal topological matrices were analyzed using the graph theory analysis module of the DPABINet toolbox. Specifically, global network matrices include clustering coefficient (Cp), characteristic shortest path length (Lp), small-world network (α), global efficiency (Eglob) and local efficiency (Eloc). The nodal network matrices mostly include the nodal degree and nodal efficiency. In the constructed functional brain networks, the strength of FC between different brain regions is defined as the edge. Then, the ratio of edges present in the network to the maximum number of possible edges is defined as the sparsity of the network [18]. For each metric, we analyzed the global and nodal topological matrices and calculated the area under the curve (AUC) at a sparsity of 0.1–0.34 with an interval of 0.01 to avoid the results being dependent on a particular sparsity value. The calculation formulas of network matrices are presented as follows [28]:
Cp=2CN(N−1) | (1) |
Lp=1N(N−1)∑i,j,i≠jD(i,j) | (2) |
α=Cp/CprandLp/Lprand | (3) |
Eglob=1N(N−1)∑i,j,i≠j1D(i,j) | (4) |
where N represents the number of nodes in the network and C denotes the number of statistically significant connected edges in the network after multiple comparisons. D(i,j) represents the number of minimum steps required to connect the nodes i and j. Cprand and Lprand represent the clustering coefficient and characteristic shortest path length of the randomized network, respectively.
Demographic information was analyzed using SPSS statistical software (Version 26, IBM). Specifically, we adopted the two-sample t-test to compare the differences in clinical data between the two groups. In addition, we also conducted the two-sample t-tests to examine the differences in AUC of each network metric in global and nodal levels between the two groups. The results were corrected using the FDR method for multiple comparisons (P<0.05).
Further, two-sample t-tests with the NBS method were used to assess the detailed differences in edge-based FC strength between the two groups. The primary threshold for each edge is set at P<0.001 in the t-tests. The functional brain network is defined by the interconnectivity of suprathreshold edges in the topological space. The NBS method performs the statistical analysis on each edge to obtain the statistic t-value, detects the network composed of suprathreshold edges based on the t-value and then calculates the number of edges the network contains. A permutation test with 5000 iterations was employed to generate the distributions of suprathreshold edge numbers in the network for non-parametric testing.
To better describe the significant node and edge obtained in the multiple comparisons, we also classified and reported the results according to their affiliation with the network defined by Yeo et al. [29]. Since the limbic network contains few ROIs, we used the subcortical network instead. The seven networks were the visual network (VN), the somatosensory network (SMN), the dorsal attentional network (DAN), the ventral attentional network (VAN), the subcortical network (SCN), the frontoparietal network (FPN) and default mode network (DMN).
Finally, we have investigated the FC conditions between large-scale intra- and inter-network. The results were calculated by averaging the z-score of FC across all involved edges and yielded seven intra-network and twenty-two inter-network values. Then, the two-sample t-tests were adopted to investigate the effect of long-term MA administration on FC changes by comparing the FC of large-scale networks in MA abstainers with those in the healthy group. The FDR was used to correct the results. We counted the number of edges that fell into the seven intra-network and inter-network classes.
The demographic and clinical information of the two groups is shown in Table 1. A total of twenty-two subjects were included in this study. The mean duration and amount of drug use in the MA group are 48.95 ± 13.32 months and 0.36 ± 0.31 g, respectively. In addition, the two groups had no significant difference in age and education level (P>0.05).
Characteristics | MA Mean ± SD | HC Mean ± SD | P-value |
Age (years) | 29.43 ± 4.25 | 30.81 ± 3.22 | 0.375 |
Education level (years) | 12.74 ± 2.41 | 13.35 ± 2.18 | 0.576 |
Duration of drug use (months) | 48.95 ± 13.32 | - | - |
Amount of drug use (g/d) | 0.36 ± 0.31 | - | - |
Note: MA: methamphetamine, HC: healthy controls, SD: standard deviation, -: no data. |
In the sparsity range of 0.1 to 0.34, we found significantly lower Lp and higher Eglob in the MA group, with statistically differences between the two groups (P<0.05). Nevertheless, Eloc was only statistically different between the two groups within a sparsity range of 0.1 to 0.25 (P<0.05). In addition, although the subjects in the two groups met the small-world networks, no statistical differences existed between groups for Cp and α (P>0.05). The results are shown in Figures 2 and 3.
At the region level, there were significant group differences between nodal degree and nodal efficiency after FDR correction (P<0.05). Compared with the HC group, the MA group showed significantly increased nodal degree in the FPN (e.g., left ventral anterior prefrontal cortex (vent aPFC_L) and left inferior parietal lobule (IPL_L)), VAN (e.g., right ventral prefrontal cortex (vPFC_R), right ventral prefrontal cortex (vFC_R) and left mid insula (Mid insula_L)), DAN (e.g., right dorsal frontal cortex (dFC_R)), SMN (e.g., left mid insula (Mid insula_L)), VN (e.g., right occipital lobe (Occipital_R)) and DMN (e.g., right precuneus (Precuneus_R)). The results are shown in Table 2 and Figure 4(a). Similarly, in terms of nodal efficiency, we found that all seven large-scale brain networks in the MA group contained some brain regions that were significantly higher compared to the HC group. Differences were found mostly in VAN (e.g., right ventral prefrontal cortex (vPFC_R)), right ventral frontal cortex (vFC_R), left mid insula (Mid insula_L)), as well as in FPN (right ventral inferior prefrontal cortex (vIPFC_R)), DAN (e.g., right dorsal frontal cortex (dFC_R) and left occipital lobe (Occipital_L)), SCN (e.g., bilateral basal ganglia (basal ganglia_L and basal ganglia_R), right thalamus (thalamus_R)), SMN (e.g., bilateral mid insula (Mid insula_L and Mid insula_R)), VN (left occipital lobe (Precuneus_L)) and DMN (left precuneus (Occipital_L)). The statistical and visualization results are shown in Table 3 and Figure 4(b).
ROIs | Coordinates (x, y, z) | Networks | MA Mean ± SD | HA Mean ± SD | P-value |
vent aPFC_L | (-43, 47, 2) | FPN | 5.260 ± 1.377 | 3.263 ± 0.787 | 0.002 |
IPL_L | (-48, -47, 49) | FPN | 6.397 ± 2.028 | 3.551 ± 1.054 | 0.002 |
vPFC_R | (34, 32, 7) | VAN | 2.428 ± 0.951 | 0.594 ± 0.705 | 0.001 |
vFC_R | (43, 1, 12) | VAN | 4.501 ± 1.305 | 0.976 ± 0.824 | 0.001 |
Mid insula_L1 | (-42, -3, 11) | VAN | 6.255 ± 3.215 | 1.164 ± 0.477 | 0.001 |
dFC_R | (44, 8, 34) | DAN | 5.611 ± 1.565 | 2.329 ± 1.640 | 0.001 |
Mid insula_L2 | (-36, -12, 15) | SMN | 5.643 ± 3.246 | 1.657 ± 1.718 | 0.005 |
Occipital_R | (36, -60, -8) | VN | 3.034 ± 1.585 | 0.878 ± 0.450 | 0.001 |
Precuneus_R | (11, -68, 42) | DMN | 4.739 ± 2.882 | 2.109 ± 0.266 | 0.015 |
Note: MA: methamphetamine, HC: healthy controls, SD: standard deviation, L: left, R: right. |
ROIs | Coordinates (x, y, z) | Networks | MA Mean ± SD | HA Mean ± SD | P-value |
vIPFC_R | (39, 42, 16) | FPN | 0.127 ± 0.010 | 0.097 ± 0.018 | 0.002 |
vPFC_R | (34, 32, 7) | VAN | 0.112 ± 0.008 | 0.045 ± 0.034 | 0.001 |
vFC_R | (43, 1, 12) | VAN | 0.130 ± 0.018 | 0.052 ± 0.036 | 0.001 |
Mid insula_L1 | (-42, -3, 11) | VAN | 0.134 ± 0.027 | 0.085 ± 0.019 | 0.002 |
dFC_R | (44, 8, 34) | DAN | 0.137 ± 0.020 | 0.097 ± 0.017 | 0.001 |
Occipital_L1 | (-29, -75, 28) | DAN | 0.141 ± 0.026 | 0.100 ± 0.026 | 0.006 |
basal ganglia_L | (-20, 6, 7) | SCN | 0.105 ± 0.027 | 0.049 ± 0.029 | 0.001 |
basal ganglia_R | (1, -26, 31) | SCN | 0.118 ± 0.012 | 0.085 ± 0.017 | 0.002 |
thalamus_R | (11, -12, 6) | SCN | 0.124 ± 0.014 | 0.087 ± 0.016 | 0.001 |
Mid insula_L2 | (33, -12, 16) | SMN | 0.124 ± 0.025 | 0.072 ± 0.033 | 0.002 |
Mid insula_L3 | (-36, -12, 15) | SMN | 0.137 ± 0.026 | 0.072 ± 0.034 | 0.001 |
Precuneus_L | (-3, -38, 45) | VN | 0.123 ± 0.023 | 0.096 ± 0.012 | 0.006 |
Occipital_L2 | (-34, -60, -5) | DMN | 0.122 ± 0.023 | 0.038 ± 0.043 | 0.001 |
Note: MA: methamphetamine, HC: healthy controls, SD: standard deviation, L: left, R: right. |
The NBS-corrected results showed ten nodes and ten edges with significant FC differences between the MA and HC groups (P<0.05). Among these results, four edges have significantly higher FC, while six are significantly lower than the HC group (Figure 5). The most significant increase edge is the FC between the left post cingulate (post cingulate_L) and right vent anterior prefrontal cortex (vent aPFC_R) brain regions (Figure 6(a), (b)). The most significant decrease edge is the FC between the left post cingulate and left medial prefrontal cortex (mPFC_L) (Figure 6(c), (d)). The edges with differences were mostly distributed in the VN, SMN, DAN, FPN and DMN (Figure 7).
Compared to the HC group, we found that the MA group significantly decreased within-network FC of the VN and SCN. In addition, the FC value between the VN and SCN of seven networks has reduced significantly (P<0.05). No significant differences were found for other large-scale brain networks. The T-value diagram and visualization results are shown in Table 4 and Figure 8.
Networks | VN | SMN | DAN | VAN | SCN | FPN | DMN |
VN | -2.7325* | - | - | - | - | - | - |
SMN | 0 | 0 | - | - | - | - | - |
DAN | 0 | 0 | 0 | - | - | - | - |
VAN | 0 | 0 | 0 | 0 | - | - | - |
SCN | -2.6490* | - | - | - | -3.1505* | - | - |
FPN | 0 | 0 | 0 | 0 | 0 | 0 | - |
DMN | 0 | 0 | 0 | 0 | 0 | 0 | 0 |
Note: * represents a statistically significant difference (P<0.05). |
In the present study, we compared the aberrant intrinsic functional brain topology at different levels between MA individuals after six months of abstinence and HC groups by graph theory and FC analysis methods. We found that the Lp showed a significantly decreased in global levels, while the Eglob and Eloc showed an increase considerably compared with the HC group. At the regional level, we also found significant group differences in nodal degree and nodal efficiency in several brain networks after FDR correction. In addition, NBS methods extensively presented the anomalies in edge-based FC, involving almost all brain functional networks. Moreover, the FC of large-scale functional brain networks revealed a decrease within the VN and SCN and between the networks.
The human brain typically has a highly modular small-world network architecture, with different functional areas separated from each other and forming highly connected hub regions [30]. The properties of small-world networks can be reflected by well-defined metrics [31], such as Cp, Lp, Eglob and Eloc. Specifically, Cp represents the degree of aggregation between brain network nodes, and Lp can reflect the average value of information transfer pathways between different brain regions. Eglob and Eloc measure the brain's ability to transmit global and local information, respectively [32]. Small-world networks generally have high Cp and short Lp, ensuring efficient data transmission and processing while maintaining a balance between local specialization and global functional connectivity of the brain [33]. Liu et al. [34] found that MA disorder patients had economic small-world properties. We found that the brains of MA individuals after six months of abstinence also have small-world properties, suggesting a certain balance of integration and differentiation of brain functions. Moreover, compared to healthy individuals, MA withdrawal individuals showed lower Lp and higher Eloc and Eglob, indicating enhanced brain defenses and a tendency to convert the random networks. This is similar to the findings in patients with chronic MA addiction on EEG data [32,35]. These findings infer that long-term MA abuse disrupts the brain balance of small-world networks, and it still fails to recover to normal levels even after six months of abstinence.
In addition, we found a significant increase in nodal degree and efficiency in brain regions such as the frontal cortex, mid-insula, inferior parietal lobule, occipital lobe and precuneus, which are mostly distributed in the seven brain functional networks. These brain regions are closely associated with human cognition, emotional control, visual processing and dopamine secretion [36]. Previous studies have shown that long-term MA abuse can damage neurons, causing compensatory neuronal adaptations and eventually manifesting lasting cognitive or neurological deficits [37]. Li et al. [19] showed similar results in a population study of MA use disorders. Nodal degree and nodal efficiency reflect the efficiency of information interaction between different brain regions [32]. We hypothesize that long-term MA abuse leads to addiction in the brain, causing frequent communication between brain areas responsible for cognition, emotional control, visual processing and dopamine release. Addiction is the response of the brain to the environment, and withdrawing is challenging. Therefore, the findings may explain the high rate of MA relapse to some extent.
The edge-based FC analysis found several abnormal edges compared with the HC group, mostly distributed in the DMN, FPN, DAN, SMN and VN. It has been reported that MA addicts have clinical manifestations such as psychotic symptoms, anxiety, sleep disorder or craving after withdrawal, among which depression is the most apparent psychiatric symptom in the early stage of MA withdrawal [38]. The DMN is abnormal in many addictions and mental diseases, such as schizophrenia, depression, anxiety and heroin addiction [39]. Postcingulate is an essential component of the DMN. The emergence of multiple psychiatric disorders is associated with functional abnormalities in the posterior cingulate gyrus [40]. The mPFC_L is implicated in self-processing decisions, such as personal information, autobiographical memories, future goals, or family decisions. The present study found significantly weakened FC between the mPFC_L and the post-cingulate, which may be related to cognitive deficits or psychiatric disorders in MA abstainers.
The FPN involves a wide range of exogenous cognitive processing and is critical for executive functions (e.g., working memory) and cognitive flexibility [41]. It can be divided into a left and a right part. The left FPN can be considered the language network, which also belongs to the cognitive category [42]. The vent aPFC_R is an essential component of the right FPN related to cognitive control [43]. In addition, the right FPN also has the main functions of activity inhibition, somatosensory perception, and pain processing, which is the core of the top-down cognitive control system [44]. Numerous studies have shown that the cognitive control system of the substance-addicted population is dysfunctional [9,10]. Previous research has shown that post-cingulate activity increases when heroin addicts are exposed to drug cues [45]. We found increased FC activity between the post-cingulate and vent aPFC_R, which may be related to the eagerness to seek drugs after methamphetamine withdrawals and ignore the harms of addictive behaviors. Unfortunately, after withdrawal, it still failed to improve the arousal of MA-independent individuals.
DAN allows one to focus attention and ignore external noise or changes in the environment [46]. As one of the core brain regions, the temporoparietal junction (TPJ) plays an essential role in attention and social cognition. TPJ is intensely active in visual detection, especially when the target is unexpected and needs to be redirected. For example, TPJ activity increases significantly during redirection but decreases during executive attention. The mid-insula is one of the components of SMN and is deeply involved in the brain reward-addicted system [10]. We found a significant increase in FC between TPJ and mid-insula and a substantial decrease between TPJ and occipital. It is hypothesized that it may be related to long-term abstinence from MA. After withdrawal, MA addicts redirect their attention and reshape the mapping of the reward system to the preoccupation system, thus gradually decreasing their concentration on drugs. In addition, Li et al. [19] studied the characteristics of the white matter structure network. They found that the connection strength between the reward and visual system in MA addicts was significantly enhanced. The present study also found that the FC between the occipital and mid-insula increased significantly, suggesting that it is related to long-term MA consumption. The long-term drug-seeking behaviors of MA addicts lead to long-term excitability on visual related functional regions. The long-term prominent visual stimulation leads to adaptive changes in the reward and visual systems [47]. The information transmitted between the visual and reward systems increases, leading to MA addicts being sensitive to drug-related clues. Although MA addicts reshape the mapping of the reward system to the preoccupation system to reduce preoccupation with the drug, the damage to the visual and the reward system from long-term MA abuse has not been restored, which may be one of the reasons why MA addicts relapse.
We also found significantly reduced FC between post-cingulate and parietal and between post-cingulate and occipital. The parietal is responsible for integrating sensory information from the outside and sensory feedback from the inside and merging this information into a coherent expression describing the connection between the body and the environment. Zhao et al. [48] studied the proactive inhibition ability of smokers. The findings showed that FC within the DMN and between the SMN-DMN was significantly decreased as the active inhibitory load increased. Moreover, it highlights that cigarette cues interfere with the effective dynamic interactions of active inhibition-related network modules. We hypothesize that with more profound withdrawal, the active inhibition function of MA addicts continues to strengthen, and the active inhibition of the informational links between the DMN-SMN and the DMN-VN is conducive to reducing the interference of drug cues.
The large-scale FC analysis also found that the FC between VN-VN, SCN-SCN and VN-SCN was significantly decreased. SCN covers the dopamine neurotransmitter projection and transmission areas, such as the ventral dorsal tegmental area (VTA) of the midbrain, nucleus accumbens and substantia nigra [49]. The SCN is often associated with reward-seeking, encoding and impulsive behaviors related to the dopamine projection pathway [50]. Previous studies have pointed out that MA smoking primarily acts on the dopamine system, damaging neurons with long-term use, causing compensatory neuronal adaptations and eventually manifesting lasting cognitive or neurological deficits [51]. It is speculated that long-term MA intake will destroy the dopamine release pathway, thus leading to a significant decline in the internal FC of SCN. In addition, some studies have pointed out that the cingulate executive network can regulate SCN and affect individual performance in cognitive tasks, especially reward-related cognitive inhibition [52]. Moreover, Matteo et al. [53] found that early VN attention deficits can affect the SCN in Parkinson disease. Sun et al. [54] found that the lingual gyrus can regulate the human hormone level. The study found that the lingual gyrus region in the VN was activated. We hypothesized that long-term MA abuse would stimulate the above brain regions, altering hormone levels in the body and causing MA addicts to experience abnormal behavior, such as visual decline. However, after six months of withdrawal, MA addicts do not return to normal levels.
Although our work revealed some abnormal brain networks, this study still has limitations that should be acknowledged. First, the sample size of this study was relatively small because of the strict exclusion criteria. This has an impact on the statistical power of the sample. Therefore, we will include more subjects to validate the experimental results in the future. Second, we focused only on alterations in topological properties of the brain between men with withdrawal and HC. It is necessary for future studies to include female abstainers. Third, the current study is cross-sectional and does not determine the causal effect of long-term interventional treatment on abnormal brain function. Further studies should explore the correlation between abnormal brain networks and clinical symptoms. In addition, the optical coherence tomography (OCT) imaging technique recently emerged as an alternative to fMRI in studying neural functional imaging of the brain and retina, which allows the functional response of neural circuits in the brain and retina [55,56,57,58]. Thus, we could adopt the OCT imaging technique to explore the aberrant intrinsic functional topology in methamphetamine-dependent individuals for future research.
In general, we investigate the aberrant intrinsic functional brain topology between MA individuals after six months of abstinence and HC groups via graph theory analysis. Results showed aberrant intrinsic functional brain topology at global and regional levels, and abnormal FC within or between several brain networks, including the FPN, VAN, DAN, SMN, VN, SCN and DMN. These findings suggest that MA-dependent individuals still have abnormal brain topology even when not exposed to drugs for long periods. After six months of withdrawal, some visual processing skills, object recognition and memory deficits may not fully recover. This leads to the possibility of relapse behavior in MA-dependent individuals when confronted with MA-related cues, which may explain the relapse mechanism to a certain extent. Furthermore, the study provides an imaging basis for revealing the neural mechanism of MA-dependent after six months of abstinence. We hope that the results will be helpful in future neurobiological mechanisms and the treatment of MA addiction.
The authors declare they have not used Artificial Intelligence (AI) tools in the creation of this article.
This work is supported by the National Natural Science Foundation of China (Grant No. 6187225), the Natural Science Foundation of Shandong Province (Grant Nos. ZR2020KF013, ZR2020ZD44, ZR2019ZD04, ZR2020QF043 and ZR2022QG051), Introduction and Cultivation Program for Young Creative Talents in Colleges and Universities of Shandong Province (Grant No. 2019-173), the science and technology of Traditional Chinese Medicine project of Shandong Province (Grant No. Q-2023045) and the Special fund of Qilu Health and Health Leading Talents Training Project.
The authors declare that there are no conflicts of interest.
[1] |
B. Han, W. M. Compton, C. M. Jones, E. B. Einstein, N. D. Volkow, Methamphetamine use, methamphetamine use disorder, and associated overdose deaths among US adults, JAMA psychiatry, 78 (2021), 1329–1342. https://doi.org/10.1001/jamapsychiatry.2021.2588 doi: 10.1001/jamapsychiatry.2021.2588
![]() |
[2] |
M. Shukla, B. Vincent, Methamphetamine abuse disturbs the dopaminergic system to impair hippocampal-based learning and memory: An overview of animal and human investigations, Neurosci. Biobehav. Rev., 131 (2021), 541–559. https://doi.org/10.1016/j.neubiorev.2021.09.016 doi: 10.1016/j.neubiorev.2021.09.016
![]() |
[3] |
V. Manja, A. Nrusimha, Y. Gao, A. Sheikh, M. McGovern, P. A. Heidenreich, et al., Methamphetamine-associated heart failure: A systematic review of observational studies, Heart, 109 (2023), 168–177. https://doi.org/10.1136/heartjnl-2022-321610 doi: 10.1136/heartjnl-2022-321610
![]() |
[4] |
C. W. Li, S. W. W. Ku, P. Y. Huang, L. Y. Chen, H. T. Wei, C. Strong, et al., Factors associated with methamphetamine dependency among men who have sex with men engaging in chemsex: Findings from the COMeT study in Taiwan, Int. J. Drug Policy, 93 (2021), 103119. https://doi.org/10.1016/j.drugpo.2021.103119 doi: 10.1016/j.drugpo.2021.103119
![]() |
[5] |
S. P. Xu, K. Zhang, T. Y. Luo, Development of the risk of relapse assessment scale for methamphetamine abusers in China, Drug Alcohol Depend., 227 (2021), 108992. https://doi.org/10.1016/j.drugalcdep.2021.108992 doi: 10.1016/j.drugalcdep.2021.108992
![]() |
[6] |
H. Yuan, X. Yu, X. Li, S. Qin, G. Liang, T. Bai, et al., Research on resting spontaneous brain activity and functional connectivity of acupuncture at uterine acupoints, Digital Chin. Med., 5 (2022), 59–67. https://doi.org/10.1016/j.dcmed.2022.03.006 doi: 10.1016/j.dcmed.2022.03.006
![]() |
[7] | X. Li, B. Wei, T. Li, N. Zhang, MwoA auxiliary diagnosis via RSN-based 3D deep multiple instance learning with spatial attention mechanism, in 2020 11th International Conference on Awareness Science and Technology (iCAST), IEEE, (2020), 1–6. https://doi.org/10.1109/iCAST51195.2020.9319486 |
[8] |
A. P. Daiwile, S. Jayanthi, J. L. Cadet, Sex differences in methamphetamine use disorder perused from pre-clinical and clinical studies: Potential therapeutic impacts, Neurosci. Biobehav. Rev., 137 (2022), 104674. https://doi.org/10.1016/j.neubiorev.2022.104674 doi: 10.1016/j.neubiorev.2022.104674
![]() |
[9] |
H. Mizoguchi, K. Yamada, Methamphetamine use causes cognitive impairment and altered decision-making, Neurochem. Int., 124 (2019), 106–113. https://doi.org/10.1016/j.neuint.2018.12.019 doi: 10.1016/j.neuint.2018.12.019
![]() |
[10] |
S. Sabrini, G. Y. Wang, J. C. Lin, J. K. Ian, L. E. Curley, Methamphetamine use and cognitive function: A systematic review of neuroimaging research, Drug Alcohol Depend., 194 (2019), 75–87. https://doi.org/10.1016/j.drugalcdep.2018.08.041 doi: 10.1016/j.drugalcdep.2018.08.041
![]() |
[11] |
S. J. Nieto, L. A. Ray, Applying the addictions neuroclinical assessment to derive neurofunctional domains in individuals who use methamphetamine, Behav. Brain Res., 427 (2022), 113876. https://doi.org/10.1016/j.bbr.2022.113876 doi: 10.1016/j.bbr.2022.113876
![]() |
[12] |
G. X. Liang, X. Li, H. Yuan, M. Sun, S. J. Qin, B. Z. Wei, Abnormal static and dynamic amplitude of low-frequency fluctuations in multiple brain regions of methamphetamine abstainers, Math. Biosci. Eng., 20 (2023), 13318–13333. https://doi.org/10.3934/mbe.2023593 doi: 10.3934/mbe.2023593
![]() |
[13] |
Z. X. Zhang, L. He, S. C. Huang, L. D. Fan, Y. N. Li, P. Li, et al., Alteration of brain structure with long-term abstinence of methamphetamine by voxel-based morphometry, Front. Psychiatry, 9 (2018), 722. https://doi.org/10.3389/fpsyt.2018.00722 doi: 10.3389/fpsyt.2018.00722
![]() |
[14] |
X. T. Li, H. Su, N. Zhong, T. Z. Chen, J. Du, K. Xiao, et al., Aberrant resting-state cerebellar-cerebral functional connectivity in methamphetamine-dependent individuals after six months abstinence, Front. Psychiatry, 11 (2020), 191. https://doi.org/10.3389/fpsyt.2020.00191 doi: 10.3389/fpsyt.2020.00191
![]() |
[15] |
L. Fan, Q. Zhang, S. Liang, H. Li, Z. He, J. Sun, et al., Imaging changes in brain microstructural in long-term abstinent from methamphetamine-dependence (in Chinese), J. Cent. South Univ. (Med. Sci.), 44 (2019), 491–500. https://doi.org/10.11817/j.issn.1672-7347.2019.05.004 doi: 10.11817/j.issn.1672-7347.2019.05.004
![]() |
[16] |
X. Y. Qi, Y. Y. Wang, Y. Z. Lu, Q. Zhao, Y. F. Chen, C. L. Zhou, et al., Enhanced brain network flexibility by physical exercise in female methamphetamine users, Cognit. Neurodyn., 2022 (2022), 1–17. https://doi.org/10.1007/s11571-022-09848-5 doi: 10.1007/s11571-022-09848-5
![]() |
[17] |
C. Y. Jia, Q. F. Long, T. Ernst, Y. Q. Shang, L. D. Chang, T. Adali, Independent component and graph theory analyses reveal normalized brain networks on resting-state functional MRI after working memory training in people with HIV, J. Magn. Reson. Imaging, 57 (2023), 1552–1564. https://doi.org/10.1002/jmri.28439 doi: 10.1002/jmri.28439
![]() |
[18] |
O. Sporns, Graph theory methods: applications in brain networks, Dialogues Clin. Neurosci., 20 (2018), 111–121. https://doi.org/10.31887/DCNS.2018.20.2/osporns doi: 10.31887/DCNS.2018.20.2/osporns
![]() |
[19] |
W. Li, L. Wang, Z. Lyu, J. J. Chen, Y. B. Li, Y. C. Sun, et al., Difference in topological organization of white matter structural connectome between methamphetamine and heroin use disorder, Behav. Brain Res., 422 (2022), 113752. https://doi.org/10.1016/j.bbr.2022.113752 doi: 10.1016/j.bbr.2022.113752
![]() |
[20] |
M. Siyah Mansoory, M. A. Oghabian, A. H. Jafari, A. Shahbabaie, Analysis of resting-state fMRI topological graph theory properties in methamphetamine drug users applying box-counting fractal dimension, Basic Clin. Neurosci., 8 (2017), 371–385. https://doi.org/10.18869/nirp.bcn.8.5.371 doi: 10.18869/nirp.bcn.8.5.371
![]() |
[21] |
Y. A. Zhou, Y. Hu, Q. J. Wang, Z. Yang, J. G. Li, Y. J. Ma, et al., Association between white matter microstructure and cognitive function in patients with methamphetamine use disorder, Hum. Brain Mapp., 44 (2023), 304–314. https://doi.org/10.1002/hbm.26020 doi: 10.1002/hbm.26020
![]() |
[22] |
M. S. Mansoory, A. Allahverdy, M. Behboudi, M. Khodamoradi, Local efficiency analysis of restingstate functional brain network in methamphetamine users, Behav. Brain Res., 434 (2022), 114022. https://doi.org/10.1016/j.bbr.2022.114022 doi: 10.1016/j.bbr.2022.114022
![]() |
[23] |
F. Miraglia, F. Vecchio, C. Pappalettera, L. Nucci, M. Cotelli, E. Judica, et al., Brain connectivity and graph theory analysis in Alzheimer's and Parkinson's Disease: The contribution of electrophysiological techniques, Brain Sci., 12 (2022), 402. https://doi.org/10.3390/brainsci12030402 doi: 10.3390/brainsci12030402
![]() |
[24] |
C. G. Yan, X. D. Wang, X. N. Zuo, Y. F. Zang, DPABI: Data processing & analysis for (resting-state) brain imaging, Neuroinformatics, 14 (2016), 339–351. https://doi.org/10.1007/s12021-016-9299-4 doi: 10.1007/s12021-016-9299-4
![]() |
[25] |
C. G. Yan, R. C. Craddock, Y. He, M. P. Milham, Addressing head motion dependencies for small-world topologies in functional connectomics, Front. Hum. Neurosci., 7 (2013), 910. https://doi.org/10.3389/fnhum.2013.00910 doi: 10.3389/fnhum.2013.00910
![]() |
[26] |
C. Yan, Y. Zang, DPARSF: A MATLAB toolbox for "pipeline" data analysis of resting-state fMRI, Front. Syst. Neurosci., 4 (2010), 13. https://doi.org/10.3389/fnsys.2010.00013 doi: 10.3389/fnsys.2010.00013
![]() |
[27] |
N. U. F. Dosenbach, B. Nardos, A. L. Cohen, D. A. Fair, J. D. Power, J. A. Church, et al., Prediction of individual brain maturity using fMRI, Science, 329 (2010), 1358–1361. https://doi.org/10.1126/science.1194144 doi: 10.1126/science.1194144
![]() |
[28] |
M. Koutrouli, E. Karatzas, D. Paez-Espino, G. A. Pavlopoulos, A Guide to conquer the biological network Era using graph theory, Front. Bioeng. Biotechnol., 8 (2020), 34. https://doi.org/10.3389/fbioe.2020.00034 doi: 10.3389/fbioe.2020.00034
![]() |
[29] |
B. T. T. Yeo, F. M. Krienen, J. Sepulcre, M. R. Sabuncu, D. Lashkari, M. Hollinshead, et al., The organization of the human cerebral cortex estimated by intrinsic functional connectivity, J. Neurophysiol., 106 (2011), 1125–1165. https://doi.org/10.1152/jn.00338.2011 doi: 10.1152/jn.00338.2011
![]() |
[30] |
G. Li, Y. D. Luo, Z. R. Zhang, Y. T. Xu, W. D. Jiao, Y. H. Jiang, et al., Effects of mental fatigue on < i > Small-World < /i > brain functional network organization, Neural Plast., 2019 (2019), 1716074. https://doi.org/10.1155/2019/1716074 doi: 10.1155/2019/1716074
![]() |
[31] |
L. J. Nestor, D. G. Ghahremani, E. D. London, Reduced neural functional connectivity during working memory performance in methamphetamine use disorder, Drug Alcohol Depend., 243 (2023), 109764. https://doi.org/10.1016/j.drugalcdep.2023.109764 doi: 10.1016/j.drugalcdep.2023.109764
![]() |
[32] |
M. Ahmadlou, K. Ahmadi, M. Rezazade, E. Azad-Marzabadi, Global organization of functional brain connectivity in methamphetamine abusers, Clin. Neurophysiol., 124 (2013), 1122–1131. https://doi.org/10.1016/j.clinph.2012.12.003 doi: 10.1016/j.clinph.2012.12.003
![]() |
[33] |
S. Arvin, A. N. Glud, K. Yonehara, Short- and long-range connections differentially modulate the dynamics and state of small-world networks, Front. Comput. Neurosci., 15 (2022), 783474. https://doi.org/10.3389/fncom.2021.783474 doi: 10.3389/fncom.2021.783474
![]() |
[34] |
Y. Liu, Q. Li, T. Y. Zhang, L. Wang, Y. R. Wang, J. J. Chen, et al., Differences in small-world networks between methamphetamine and heroin use disorder patients and their relationship with psychiatric symptoms, Brain Imaging Behav., 16 (2022), 1973–1982. https://doi.org/10.1007/s11682-022-00667-0 doi: 10.1007/s11682-022-00667-0
![]() |
[35] |
H. Khajehpour, B. Makkiabadi, H. Ekhtiari, S. Bakht, A. Noroozi, F. Mohagheghian, Disrupted resting-state brain functional network in methamphetamine abusers: A brain source space study by EEG, PLoS One, 14 (2019), e0226249. https://doi.org/10.1371/journal.pone.0226249 doi: 10.1371/journal.pone.0226249
![]() |
[36] |
F. X. Vollenweider, K. H. Preller, Psychedelic drugs: neurobiology and potential for treatment of psychiatric disorders, Nat. Rev. Neurosci., 21 (2020), 611–624. https://doi.org/10.1038/s41583-020-0367-2 doi: 10.1038/s41583-020-0367-2
![]() |
[37] |
B. Kim, J. Yun, B. Park, Methamphetamine-induced neuronal damage: Neurotoxicity and neuroinflammation, Biomol. Ther., 28 (2020), 381–388. https://doi.org/10.4062/biomolther.2020.044 doi: 10.4062/biomolther.2020.044
![]() |
[38] |
J. Zhang, H. Su, J. Y. Tao, Y. Xie, Y. M. Sun, L. R. Li, et al., Relationship of impulsivity and depression during early methamphetamine withdrawal in Han Chinese population, Addict. Behav., 43 (2015), 7–10. https://doi.org/10.1016/j.addbeh.2014.10.032 doi: 10.1016/j.addbeh.2014.10.032
![]() |
[39] |
A. Scalabrini, B. Vai, S. Poletti, S. Damiani, C. Mucci, C. Colombo, et al., All roads lead to the default-mode network-global source of DMN abnormalities in major depressive disorder, Neuropsychopharmacology, 45 (2020), 2058–2069. https://doi.org/10.1038/s41386-020-0785-x doi: 10.1038/s41386-020-0785-x
![]() |
[40] |
B. L. Foster, S. R. Koslov, L. Aponik-Gremillion, M. E. Monko, B. Y. Hayden, S. R. Heilbronner, A tripartite view of the posterior cingulate cortex, Nat. Rev. Neurosci., 24 (2023), 173–189. https://doi.org/10.1038/s41583-022-00661-x doi: 10.1038/s41583-022-00661-x
![]() |
[41] |
C. Caldinelli, R. Cusack, The fronto-parietal network is not a flexible hub during naturalistic cognition, Hum. Brain Mapp., 43 (2022), 750–759. https://doi.org/10.1002/hbm.25684 doi: 10.1002/hbm.25684
![]() |
[42] |
H. Zheng, Q. Zhou, J. J. Yang, Q. Lu, H. D. Qiu, C. He, et al., Altered functional connectivity of the default mode and frontal control networks in patients with insomnia, CNS Neurosci. Ther., 29 (2023), 2318–2326. https://doi.org/10.1111/cns.14183 doi: 10.1111/cns.14183
![]() |
[43] |
R. De Micco, N. Piramide, F. Di Nardo, M. Siciliano, M. Cirillo, A. Russo, et al., Resting-state network connectivity changes in drug-naive Parkinson's disease patients with probable REM sleep behavior disorder, J. Neural Transm., 130 (2023), 43–51. https://doi.org/10.1007/s00702-022-02565-7 doi: 10.1007/s00702-022-02565-7
![]() |
[44] |
S. Tikoo, F. Cardona, S. Tommasin, C. Giannì, G. Conte, N. Upadhyay, et al., Resting-state functional connectivity in drug-naive pediatric patients with Tourette syndrome and obsessive-compulsive disorder, J. Psychiatr. Res., 129 (2020), 129–140. https://doi.org/10.1016/j.jpsychires.2020.06.021 doi: 10.1016/j.jpsychires.2020.06.021
![]() |
[45] |
G. Dong, E. DeVito, J. Huang, X. Du, Diffusion tensor imaging reveals thalamus and posterior cingulate cortex abnormalities in internet gaming addicts. J. Psychiatr. Res., 46 (2012), 1212–1216. https://doi.org/10.1016/j.jpsychires.2012.05.015 doi: 10.1016/j.jpsychires.2012.05.015
![]() |
[46] |
Y. Katsumi, D. Putcha, R. Eckbo, B. Wong, M. Quimby, S. McGinnis, et al., Anterior dorsal attention network tau drives visual attention deficits in posterior cortical atrophy, Brain, 146 (2023), 295–306. https://doi.org/10.1093/brain/awac245 doi: 10.1093/brain/awac245
![]() |
[47] |
H. Y. Tan, T. Z. Chen, J. Du, R. J. Li, H. F. Jiang, C. L. Deng, et al., Drug-related virtual reality cue reactivity is associated with gamma activity in reward and executive control circuit in methamphetamine use disorders, Arch. Med. Res., 50 (2019), 509–517. https://doi.org/10.1016/j.arcmed.2019.09.003 doi: 10.1016/j.arcmed.2019.09.003
![]() |
[48] |
H. C. Zhao, M. J. Ge, O. Turel, A. Bechara, Q. H. He, Brain modular connectivity interactions can predict proactive inhibition in smokers when facing smoking cues, Addict. Biol., 28 (2023), e13284. https://doi.org/10.1111/adb.13284 doi: 10.1111/adb.13284
![]() |
[49] |
S. Yilmaz, Impaired biological rhythm in men with methamphetamine use disorder: The relationship with sleep quality and depression, J. Subst. Use, 28 (2023), 280–286. https://doi.org/10.1080/14659891.2022.2098847 doi: 10.1080/14659891.2022.2098847
![]() |
[50] |
L. Cerliani, M. Mennes, R. M. Thomas, A. Di Martino, M. Thioux, C. Keysers, Increased functional connectivity between subcortical and cortical resting-state networks in autism spectrum disorder, JAMA Psychiatry, 72 (2015), 767–777. https://doi.org/10.1001/jamapsychiatry.2015.0101 doi: 10.1001/jamapsychiatry.2015.0101
![]() |
[51] |
A. Moaveni, Y. F. Feyzi, S. T. Rahideh, R. Arezoomandan, The relationship between serum brain-derived neurotrophic level and neurocognitive functions in chronic methamphetamine users, Neurosci. Lett., 772 (2022), 136478. https://doi.org/10.1016/j.neulet.2022.136478 doi: 10.1016/j.neulet.2022.136478
![]() |
[52] |
R. Nusslock, G. H. Brody, C. C. Armstrong, A. L. Carroll, L. H. Sweet, T. Y. Yu, et al., Higher peripheral inflammatory signaling associated with lower resting-state functional brain connectivity in emotion regulation and central executive networks, Biol. Psychiatry, 86 (2019), 153–162. https://doi.org/10.1016/j.biopsych.2019.03.968 doi: 10.1016/j.biopsych.2019.03.968
![]() |
[53] |
M. Esposito, M. Tamietto, G. C. Geminiani, A. Celeghin, A subcortical network for implicit visuo-spatial attention: Implications for Parkinson's disease, Cortex, 141 (2021), 421–435. https://doi.org/10.1016/j.cortex.2021.05.003 doi: 10.1016/j.cortex.2021.05.003
![]() |
[54] |
Y. Z. Sun, Y. Ding, T. Y. Yu, C. Y. Chen, P. Li, X. G. Yang, et al., Effect of Tiaoshen acupuncture on anxiety after methamphetamine withdrawal (in Chinese), Chin. Acupunct. Moxibust., 42 (2022), 277–280. https://doi.org/10.13703/j.0255-2930.20210213-k0001 doi: 10.13703/j.0255-2930.20210213-k0001
![]() |
[55] |
R. K. Meleppat, C. R. Fortenbach, Y. F. Jian, E. S. Martinez, K. Wagner, B. S. Modjtahedi, et al., In vivo imaging of retinal and choroidal morphology and vascular plexuses of vertebrates using swept-source optical coherence tomography, Transl. Vision Sci. Technol., 11 (2022), 11. https://doi.org/10.1167/tvst.11.8.11 doi: 10.1167/tvst.11.8.11
![]() |
[56] |
R. K. Meleppat, K. E. Ronning, S. J. Karlen, M. E. Burns, E. N. Pugh, R. J. Zawadzki, In vivo multimodal retinal imaging of disease-related pigmentary changes in retinal pigment epithelium, Sci. Rep., 11 (2021), 16252. https://doi.org/10.1038/s41598-021-95320-z doi: 10.1038/s41598-021-95320-z
![]() |
[57] |
R. K. Meleppat, P. F. Zhang, M. J. Ju, S. K. Manna, Y. Jian, E. N. Pugh, et al., Directional optical coherence tomography reveals melanin concentration-dependent scattering properties of retinal pigment epithelium, J. Biomed. Opt., 24 (2019), 066011. https://doi.org/10.1117/1.Jbo.24.6.066011 doi: 10.1117/1.Jbo.24.6.066011
![]() |
[58] |
K. M. Ratheesh, L. K. Seah, V. M. Murukeshan, Spectral phase-based automatic calibration scheme for swept source-based optical coherence tomography systems, Phys. Med. Biol., 61 (2016), 7652–7663. https://doi.org/10.1088/0031-9155/61/21/7652 doi: 10.1088/0031-9155/61/21/7652
![]() |
1. | Kareem Al-Khalil, Ryan P. Bell, Sheri L. Towe, Jessica R. Cohen, Syam Gadde, James Mu, Shana A. Hall, Christina S. Meade, Hub disruption in HIV disease and cocaine use: A connectomics analysis of brain function, 2024, 263, 03768716, 112416, 10.1016/j.drugalcdep.2024.112416 |
Characteristics | MA Mean ± SD | HC Mean ± SD | P-value |
Age (years) | 29.43 ± 4.25 | 30.81 ± 3.22 | 0.375 |
Education level (years) | 12.74 ± 2.41 | 13.35 ± 2.18 | 0.576 |
Duration of drug use (months) | 48.95 ± 13.32 | - | - |
Amount of drug use (g/d) | 0.36 ± 0.31 | - | - |
Note: MA: methamphetamine, HC: healthy controls, SD: standard deviation, -: no data. |
ROIs | Coordinates (x, y, z) | Networks | MA Mean ± SD | HA Mean ± SD | P-value |
vent aPFC_L | (-43, 47, 2) | FPN | 5.260 ± 1.377 | 3.263 ± 0.787 | 0.002 |
IPL_L | (-48, -47, 49) | FPN | 6.397 ± 2.028 | 3.551 ± 1.054 | 0.002 |
vPFC_R | (34, 32, 7) | VAN | 2.428 ± 0.951 | 0.594 ± 0.705 | 0.001 |
vFC_R | (43, 1, 12) | VAN | 4.501 ± 1.305 | 0.976 ± 0.824 | 0.001 |
Mid insula_L1 | (-42, -3, 11) | VAN | 6.255 ± 3.215 | 1.164 ± 0.477 | 0.001 |
dFC_R | (44, 8, 34) | DAN | 5.611 ± 1.565 | 2.329 ± 1.640 | 0.001 |
Mid insula_L2 | (-36, -12, 15) | SMN | 5.643 ± 3.246 | 1.657 ± 1.718 | 0.005 |
Occipital_R | (36, -60, -8) | VN | 3.034 ± 1.585 | 0.878 ± 0.450 | 0.001 |
Precuneus_R | (11, -68, 42) | DMN | 4.739 ± 2.882 | 2.109 ± 0.266 | 0.015 |
Note: MA: methamphetamine, HC: healthy controls, SD: standard deviation, L: left, R: right. |
ROIs | Coordinates (x, y, z) | Networks | MA Mean ± SD | HA Mean ± SD | P-value |
vIPFC_R | (39, 42, 16) | FPN | 0.127 ± 0.010 | 0.097 ± 0.018 | 0.002 |
vPFC_R | (34, 32, 7) | VAN | 0.112 ± 0.008 | 0.045 ± 0.034 | 0.001 |
vFC_R | (43, 1, 12) | VAN | 0.130 ± 0.018 | 0.052 ± 0.036 | 0.001 |
Mid insula_L1 | (-42, -3, 11) | VAN | 0.134 ± 0.027 | 0.085 ± 0.019 | 0.002 |
dFC_R | (44, 8, 34) | DAN | 0.137 ± 0.020 | 0.097 ± 0.017 | 0.001 |
Occipital_L1 | (-29, -75, 28) | DAN | 0.141 ± 0.026 | 0.100 ± 0.026 | 0.006 |
basal ganglia_L | (-20, 6, 7) | SCN | 0.105 ± 0.027 | 0.049 ± 0.029 | 0.001 |
basal ganglia_R | (1, -26, 31) | SCN | 0.118 ± 0.012 | 0.085 ± 0.017 | 0.002 |
thalamus_R | (11, -12, 6) | SCN | 0.124 ± 0.014 | 0.087 ± 0.016 | 0.001 |
Mid insula_L2 | (33, -12, 16) | SMN | 0.124 ± 0.025 | 0.072 ± 0.033 | 0.002 |
Mid insula_L3 | (-36, -12, 15) | SMN | 0.137 ± 0.026 | 0.072 ± 0.034 | 0.001 |
Precuneus_L | (-3, -38, 45) | VN | 0.123 ± 0.023 | 0.096 ± 0.012 | 0.006 |
Occipital_L2 | (-34, -60, -5) | DMN | 0.122 ± 0.023 | 0.038 ± 0.043 | 0.001 |
Note: MA: methamphetamine, HC: healthy controls, SD: standard deviation, L: left, R: right. |
Networks | VN | SMN | DAN | VAN | SCN | FPN | DMN |
VN | -2.7325* | - | - | - | - | - | - |
SMN | 0 | 0 | - | - | - | - | - |
DAN | 0 | 0 | 0 | - | - | - | - |
VAN | 0 | 0 | 0 | 0 | - | - | - |
SCN | -2.6490* | - | - | - | -3.1505* | - | - |
FPN | 0 | 0 | 0 | 0 | 0 | 0 | - |
DMN | 0 | 0 | 0 | 0 | 0 | 0 | 0 |
Note: * represents a statistically significant difference (P<0.05). |
Characteristics | MA Mean ± SD | HC Mean ± SD | P-value |
Age (years) | 29.43 ± 4.25 | 30.81 ± 3.22 | 0.375 |
Education level (years) | 12.74 ± 2.41 | 13.35 ± 2.18 | 0.576 |
Duration of drug use (months) | 48.95 ± 13.32 | - | - |
Amount of drug use (g/d) | 0.36 ± 0.31 | - | - |
Note: MA: methamphetamine, HC: healthy controls, SD: standard deviation, -: no data. |
ROIs | Coordinates (x, y, z) | Networks | MA Mean ± SD | HA Mean ± SD | P-value |
vent aPFC_L | (-43, 47, 2) | FPN | 5.260 ± 1.377 | 3.263 ± 0.787 | 0.002 |
IPL_L | (-48, -47, 49) | FPN | 6.397 ± 2.028 | 3.551 ± 1.054 | 0.002 |
vPFC_R | (34, 32, 7) | VAN | 2.428 ± 0.951 | 0.594 ± 0.705 | 0.001 |
vFC_R | (43, 1, 12) | VAN | 4.501 ± 1.305 | 0.976 ± 0.824 | 0.001 |
Mid insula_L1 | (-42, -3, 11) | VAN | 6.255 ± 3.215 | 1.164 ± 0.477 | 0.001 |
dFC_R | (44, 8, 34) | DAN | 5.611 ± 1.565 | 2.329 ± 1.640 | 0.001 |
Mid insula_L2 | (-36, -12, 15) | SMN | 5.643 ± 3.246 | 1.657 ± 1.718 | 0.005 |
Occipital_R | (36, -60, -8) | VN | 3.034 ± 1.585 | 0.878 ± 0.450 | 0.001 |
Precuneus_R | (11, -68, 42) | DMN | 4.739 ± 2.882 | 2.109 ± 0.266 | 0.015 |
Note: MA: methamphetamine, HC: healthy controls, SD: standard deviation, L: left, R: right. |
ROIs | Coordinates (x, y, z) | Networks | MA Mean ± SD | HA Mean ± SD | P-value |
vIPFC_R | (39, 42, 16) | FPN | 0.127 ± 0.010 | 0.097 ± 0.018 | 0.002 |
vPFC_R | (34, 32, 7) | VAN | 0.112 ± 0.008 | 0.045 ± 0.034 | 0.001 |
vFC_R | (43, 1, 12) | VAN | 0.130 ± 0.018 | 0.052 ± 0.036 | 0.001 |
Mid insula_L1 | (-42, -3, 11) | VAN | 0.134 ± 0.027 | 0.085 ± 0.019 | 0.002 |
dFC_R | (44, 8, 34) | DAN | 0.137 ± 0.020 | 0.097 ± 0.017 | 0.001 |
Occipital_L1 | (-29, -75, 28) | DAN | 0.141 ± 0.026 | 0.100 ± 0.026 | 0.006 |
basal ganglia_L | (-20, 6, 7) | SCN | 0.105 ± 0.027 | 0.049 ± 0.029 | 0.001 |
basal ganglia_R | (1, -26, 31) | SCN | 0.118 ± 0.012 | 0.085 ± 0.017 | 0.002 |
thalamus_R | (11, -12, 6) | SCN | 0.124 ± 0.014 | 0.087 ± 0.016 | 0.001 |
Mid insula_L2 | (33, -12, 16) | SMN | 0.124 ± 0.025 | 0.072 ± 0.033 | 0.002 |
Mid insula_L3 | (-36, -12, 15) | SMN | 0.137 ± 0.026 | 0.072 ± 0.034 | 0.001 |
Precuneus_L | (-3, -38, 45) | VN | 0.123 ± 0.023 | 0.096 ± 0.012 | 0.006 |
Occipital_L2 | (-34, -60, -5) | DMN | 0.122 ± 0.023 | 0.038 ± 0.043 | 0.001 |
Note: MA: methamphetamine, HC: healthy controls, SD: standard deviation, L: left, R: right. |
Networks | VN | SMN | DAN | VAN | SCN | FPN | DMN |
VN | -2.7325* | - | - | - | - | - | - |
SMN | 0 | 0 | - | - | - | - | - |
DAN | 0 | 0 | 0 | - | - | - | - |
VAN | 0 | 0 | 0 | 0 | - | - | - |
SCN | -2.6490* | - | - | - | -3.1505* | - | - |
FPN | 0 | 0 | 0 | 0 | 0 | 0 | - |
DMN | 0 | 0 | 0 | 0 | 0 | 0 | 0 |
Note: * represents a statistically significant difference (P<0.05). |