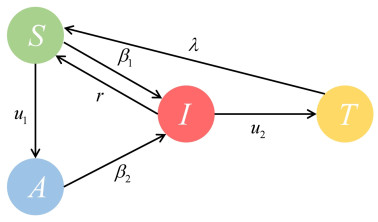
In this paper, an SAITS epidemic model based on a single layer static network is proposed and investigated. This model considers a combinational suppression control strategy to suppress the spread of epidemics, which includes transferring more individuals to compartments with low infection rate and with high recovery rate. The basic reproduction number of this model is calculated and the disease-free and endemic equilibrium points are discussed. An optimal control problem is formulated to minimize the number of infections with limited resources. The suppression control strategy is investigated and a general expression for the optimal solution is given based on the Pontryagin's principle of extreme value. The validity of the theoretical results is verified by numerical simulations and Monte Carlo simulations.
Citation: Wei Ding, Li Ding, Zhengmin Kong, Feng Liu. The SAITS epidemic spreading model and its combinational optimal suppression control[J]. Mathematical Biosciences and Engineering, 2023, 20(2): 3342-3354. doi: 10.3934/mbe.2023157
[1] | F. S. Vannucchi, S. Boccaletti . Chaotic spreading of epidemics in complex networks of excitable units. Mathematical Biosciences and Engineering, 2004, 1(1): 49-55. doi: 10.3934/mbe.2004.1.49 |
[2] | Tingting Xue, Long Zhang, Xiaolin Fan . Dynamic modeling and analysis of Hepatitis B epidemic with general incidence. Mathematical Biosciences and Engineering, 2023, 20(6): 10883-10908. doi: 10.3934/mbe.2023483 |
[3] | Cheng-Cheng Zhu, Jiang Zhu . Spread trend of COVID-19 epidemic outbreak in China: using exponential attractor method in a spatial heterogeneous SEIQR model. Mathematical Biosciences and Engineering, 2020, 17(4): 3062-3087. doi: 10.3934/mbe.2020174 |
[4] | Rongjian Lv, Hua Li, Qiubai Sun, Bowen Li . Model of strategy control for delayed panic spread in emergencies. Mathematical Biosciences and Engineering, 2024, 21(1): 75-95. doi: 10.3934/mbe.2024004 |
[5] | Pannathon Kreabkhontho, Watchara Teparos, Thitiya Theparod . Potential for eliminating COVID-19 in Thailand through third-dose vaccination: A modeling approach. Mathematical Biosciences and Engineering, 2024, 21(8): 6807-6828. doi: 10.3934/mbe.2024298 |
[6] | Mina Youssef, Caterina Scoglio . Mitigation of epidemics in contact networks through optimal contact adaptation. Mathematical Biosciences and Engineering, 2013, 10(4): 1227-1251. doi: 10.3934/mbe.2013.10.1227 |
[7] | Hamed Karami, Pejman Sanaei, Alexandra Smirnova . Balancing mitigation strategies for viral outbreaks. Mathematical Biosciences and Engineering, 2024, 21(12): 7650-7687. doi: 10.3934/mbe.2024337 |
[8] | Yong Zhou, Minrui Guo . Isolation in the control of epidemic. Mathematical Biosciences and Engineering, 2022, 19(11): 10846-10863. doi: 10.3934/mbe.2022507 |
[9] | Sara Y. Del Valle, J. M. Hyman, Nakul Chitnis . Mathematical models of contact patterns between age groups for predicting the spread of infectious diseases. Mathematical Biosciences and Engineering, 2013, 10(5&6): 1475-1497. doi: 10.3934/mbe.2013.10.1475 |
[10] | Junbo Jia, Wei Shi, Pan Yang, Xinchu Fu . Immunization strategies in directed networks. Mathematical Biosciences and Engineering, 2020, 17(4): 3925-3952. doi: 10.3934/mbe.2020218 |
In this paper, an SAITS epidemic model based on a single layer static network is proposed and investigated. This model considers a combinational suppression control strategy to suppress the spread of epidemics, which includes transferring more individuals to compartments with low infection rate and with high recovery rate. The basic reproduction number of this model is calculated and the disease-free and endemic equilibrium points are discussed. An optimal control problem is formulated to minimize the number of infections with limited resources. The suppression control strategy is investigated and a general expression for the optimal solution is given based on the Pontryagin's principle of extreme value. The validity of the theoretical results is verified by numerical simulations and Monte Carlo simulations.
The Coronavirus Disease 2019 (COVID-19) has spread all over the world and endangered people's normal lives. For analyzing the dynamical process of epidemic spreading, it is of great significance to establish the mathematical model of disease propagation on complex networks. In the real world, the population can be considered homogeneous due to its extremely huge size [1]. Therefore, the population can be partitioned into different compartments according to different states of individuals. Since there is no difference in propagation dynamics between individuals within the same compartment, the process of epidemic spreading can be described as the transfer of individuals between compartments. Many scholars have proposed different compartment models to study the mechanism of epidemic spreading in the population, such as SIS [2], SIR [3,4], SEIR [5], SVEIR [6], SEIRD [7], etc.
Since the spread of the disease will bring economic losses, crowd panic and other social instability, scientists have analyzed the impact of different suppression methods on the spreading of epidemics. For example, isolating and treating individuals who are already infected [8], vaccinating individuals who are not infected [9,10], controlling the movement of people [11] and requiring masks [12]. These suppression control strategies can be divided into two categories. One is to reduce the infection rate and the other is to increase the recovery rate.
The most popular way of reducing infection rates is vaccination. When individuals are vaccinated, their infection rate can be dramatically reduced, thereby inhibiting the spread of the disease. For instance, Shi et al. [13] proposed a class SIS model that integrates the effects of scenarios such as incomplete vaccination and incomplete immunization on disease transmission. The effect of isolation is similar to the vaccination. Ahuod S. Alsheri et al. [14] studied the effect of isolation rates on controlling the spread of COVID-19, using data given by the Ministry of Health of Saudi Arabia, and the result confirmed that early detection and isolation lead to a better control effect. The increase of recovery rates depends on government investment in the healthcare system and drug development, as well as the improvement of the individual's immunity and other related strategies.
It is worth noting that these strategies are always accompanied by some costs. Therefore, with limited resources, there is a strong need to develop optimal control strategies to suppress the widespread of epidemics at minimal cost. Many scholars have conducted relevant studies in this area. For example, Xu et al. [15] proposed a novel SIVRS mathematical model with viral variation and studied optimal control strategies for susceptible, infected and mutated individuals. Ana P. Lemos-Paião et al. [16] proposed a mathematical model of SIQR for treating cholera with quarantine, studied the model's disease-free and endemic equilibrium points and provided the optimal quarantine strategy to minimize the number of infectious individuals and costs. Laurent Miclo et al. [17] studied an setting with constrained ICU resources and high suppression costs. Results showed that the optimal control is discontinuous. Epidemics should be left unregulated initially, with a rapid social lockdown when approaching the ICU constraint, followed by gradual decontrol, and finally complete lifting of the regulation. Wu et al. [18] found that prevention and control measures such as isolation and vaccination can affect the accuracy of the model, and the accuracy is 6.7% higher when these factors are considered. Anuj Kumar et al. [19] proposed a nonlinear compartment model considering information-induced vaccination and treatment as two control strategies. The results showed that the execution of both strategies simultaneously was effective throughout the epidemic outbreak, while the execution of information-induced vaccination was effective only during the initial phase. G. Dimarco et al. [20] considered the heterogeneity described by the social contact of individuals and analysed the effects of introducing an optimal control strategy into the system, to limit selectively the mean number of contacts and reduce consequently the number of infected cases. The work most closely related to ours is [21], in which scholars have presented an optimal control problem on a SIR epidemic model including both vaccination and treatment control strategies on actual data, it has been demonstrated that using both control strategies simultaneously is more effective than using only one of the control strategies.
Among the previous works, few works considered the control strategy of reducing the infection rate and increasing the recovery rate simultaneously to suppress the spread of epidemics, while considering the control cost. However, in reality, the government and other relevant departments mostly take a two-pronged approach when dealing with infectious diseases, that is, informing the public to reduce travel, wear masks as well as get vaccination and medical treatment in time. Therefore, it is necessary to design an epidemic model that incorporates both types of control strategies to more accurately study the effects of these suppression strategies on epidemic spreading.
Based on the above analysis, the main contents and contributions of this paper are as follows. Firstly, we propose a Susceptible-Alert-Infected-Treated-Susceptible (SAITS) epidemic model that incorporates a combinational control consisting of two types of strategies. One strategy is to get more individuals into the compartment with low infection rate, and the other is to get more individuals into the compartment with high recovery rate. The basic reproduction number of the model is calculated and the disease-free and endemic equilibrium points of the model are discussed. Secondly, the optimal control problem is investigated to minimize the cost of both infection and combinational suppression control, and a general expression of the optimal solution that reflects the trade-off between the infection cost and the combinational suppression control cost is obtained. Finally, to verify the theoretical results, Monte Carlo and numerical simulations are performed.
The rest of this paper is organized as follows. In Section 2, the SAITS epidemic model is developed. In Section 3, the basic reproduction number of the model and the disease-free and endemic equilibrium points are discussed. Meanwhile, an optimal control problem and its theoretical analysis is given. Monte Carlo and numerical simulations are performed to illustrate the results in Section 4. Conclusions are drawn in Section 5.
Consider an undirected unweighted graph representing the relationship between individuals in a population, which consists of N nodes, with the connectivity between nodes represented by an adjacency matrix Aij=[aij]∈RN×N, where aij=1 means node i and node j are connected, conversely, aij=0 means node i and node j are disconnected.
We propose an SAITS epidemic model in which individuals are divided into four compartments, namely, Susceptible (S), Alert (A), Infected (I) and Treated (T). Both the susceptible compartment S and the alert compartment A are collections of uninfected individuals. The infection rate of alert individuals is lower than susceptible individuals. Both the infected compartment I and the treated compartment T are collections of infected ones. The recovery rate of treated individuals is higher than infected ones.
A susceptible individual S or an alert individual A will become infected at the infection rate β1 or β2(0<β1<β2<1), respectively, if they come into contact with an infected individual I. An infected individual I or a treated individual T will return to the susceptible state at the recovery rate r or λ(0<λ<r<1), respectively. We believe that a susceptible individual will only enter the alert state if there is at least one infected individual in its neighborhood and itself is not infected next, an alert individual will not spread this awareness to other susceptible individuals, and the treated individual is not infectious.
We investigate a combinational suppression control strategy aimed at reducing infection rate and increasing recovery rate by transferring more individuals from compartment S to compartment A, while transferring more individuals from compartment I to compartment T. Under this strategy, a susceptible individual S will enter the alert state A with a probability of u1(0<u1<1) and an infected individual will enter the treated state T with a probability of u2(0<u2<1). The value of u1 and u2 reflects the strength of control strategy. The larger the value, the stronger the control effect. The flow chart of epidemic spreading is shown in Figure 1.
The following dynamic equations can be obtained from the proposed model
dSdt=rI−u1(t)S−β1SI+λT,dAdt=u1(t)S−β2AI,dIdt=β1SI+β2AI−rI−u2I,dTdt=u2(t)I−λT. | (2.1) |
Denote pSi(t), pAi(t), pIi(t), pTi(t), pSi(t),pAi(t),pIi(t),pTi(t)∈[0,1] as the probability of an individual i being susceptible, alert, infected and treated, respectively. It is clear that if the number of individuals is large enough, the average of the probabilities of all individuals in each state will be equal to the density of individuals in the corresponding state. The discrete-time mathematical model based on the microscopic Markov chain approach is thus proposed as
pSi(t+1)=(1−u1(t)−qSIi(t))pSi(t)+rpIi(t)+λpTi(t),pAi(t+1)=(1−qAIi(t))pAi(t)+u1(t)pSi(t),pIi(t+1)=(1−u2(t)−r)pIi(t)+qSIi(t)pSi(t)+qAIi(t)pAi(t),pTi(t+1)=(1−λ)pTi(t)+u2(t)pIi(t), | (2.2) |
where qSIi(t)=1−∏j∈Ni(1−β1pIjaij(t)) and qAIi(t)=1−∏j∈Ni(1−β2pIjaij(t)) represent the probability that a susceptible individual S or an alert individual A is infected by an adjacent infected individual, respectively.
When the states of the individual's neighbors are independent of each other and the infection rate is sufficiently small, under these two assumptions [22], we can deduce that
qSIi(t)≈N∑j=1β1pIjaij(t),qAIi(t)≈N∑j=1β2pIjaij(t). | (2.3) |
The mean-field model can then be written as
˙pSi(t)=(−u1(t)−N∑j=1β1pIjaij(t))pSi(t)+rpIi(t)+λpTi(t),˙pAi(t)=−N∑j=1β2pIjaij(t)pAi(t)+u1(t)pSi(t),˙pIi(t)=(−u2(t)−r)pIi(t)+N∑j=1β1pIjaij(t)pSi(t)+N∑j=1β2pIjaij(t)pAi(t),˙pTi(t)=−λpTi(t)+u2(t)pIi(t). | (2.4) |
According to the method proposed in [23], the basic reproduction number of Eq (2.1) is calculated
R0=ρ(FV−1)=β1+β2u2+r. | (3.1) |
It can be seen that the propagation threshold R0 is positively correlated with β1 and β2, and is negatively correlated with u2+r. Several sets of numerical simulation experiments are conducted to verify this conclusion, with the step of 0.2 and running 100 steps. The experimental results are shown in Figure 2. To make the results more accurate, the control variable method is utilized. When one variable is chosen for comparison, the other variables take the same values as the standard group. The parameters for the standard group are set as β1=0.30, β2=0.05, r=0.10, λ=0.50, u1=u2=0.2.
As can be seen in Figure 2(a), the proportion of infected individuals increases as β1 gets larger. Figure 2(b) shows that the proportion of infected individuals increases slightly as β2 gets larger. Figure 2(c) shows that as r increases, the proportion of infected individuals decreases at the rate of 4%. Figure 2(d) indicates that as λ increases, there is nearly no change in the rising process while the stable value becomes progressively larger. Figure 2(e) shows that the rise time remains constant with u1 increasing. Figure 2(f) indicates a large decrease in the stable value of the proportion of infected individuals with u2 increasing. Based on the analysis above, the effect of β2 on the proportion of infected individuals is the weakest, while the effect of u2 is the strongest. The effect of r is nearly linear, and the effect of β1 and λ is between the above two. From this, we can conclude that increasing the recovery rate in the population, for example, by researching specific drugs and increasing access to health care, is more important and more effective than reducing the infection rate.
In this section, a global optimization problem is constructed to achieve the goal of global optimization by finding the optimal weighting coefficients in a combinational suppression control strategy. In the process of controlling infectious disease, on the one hand, there is a population infection cost, denoted as J1, which is related to pIi, and on the other hand, there is a combinational optimal suppression control cost, denoted as J2, which is determined by the control strategy proposed in Section 2. Thus, the objective function is the sum of these two kinds of costs for all individuals over the time interval [0,T]. We consider the cumulative cost over the entire time interval, that is, the total cost, denoted by J. The following is the procedure of solving the optimal solution in this time interval.
Based on the above discussion, the cost of the optimization problem is expressed as
J=∫T0(J1+J2)dt, | (3.2) |
where
J1=N∑i=1dipIi(t), |
J2=c12u1(t)2+c22u2(t)2. |
We use the Hamiltonian method to solve this optimal control problem. Obviously, the Lagrangian function of the optimal problem is defined according to the objective Eq (3.2) as follows
L=c12u1(t)2+c22u2(t)2+N∑i=1dpIi(t), | (3.3) |
where c1 and c2 are the control strategy weighting factors and d is the infection cost factor.
The Pontryagin's maximum principle is used to solve the expression for optimal control. The Hamiltonian function is constructed by combining the state equation with the objective function by introducing the costate variables λ(t)=(λ1(t),λ2(t),λ3(t),λ4(t)), transforming the optimal control problem into a problem of solving the minimum of the Hamiltonian function.
Define the Hamiltonian function as follows
H=c12u1(t)2+c22u2(t)2+N∑i=1dipIi(t)+N∑i=1(λ1i˙pSi(t)+λ2i˙pAi(t)+λ3i˙pIi(t)+λ4i˙pTi(t)), | (3.4) |
where the costate variables satisfy the condition that ˙λ1i=∂H∂pSi, ˙λ2i=∂H∂pAi, ˙λ3i=∂H∂pIi, ˙λ4i=∂H∂pTi. Substituting Eq (2.4) into Eq (3.5), we can derive the expression for the accompanying variables
˙λ=Aλ+B, | (3.5) |
A=[u1(t)+N∑j=1β1pIj(t)aij−u1−N∑j=1β2pIj(t)aij00N∑j=1β2pIj(t)aij−N∑j=1β2pIj(t)aij0−r+N∑j=1β1aijpSj(t)N∑j=1β2aijpAj(t)r+u2−N∑j=1β2aijpAj(t)−N∑j=1β1aijpSj(t)−u2−λ00λ], | (3.6) |
B=[00−di0]. | (3.7) |
The following conditions need to be satisfied when calculating the optimal solution
∂H∂u1(t)=c1u1(t)+N∑i=1(λ2i−λ1i)pSi(t),∂H∂u2(t)=c2u2(t)+N∑i=1(λ4i−λ3i)pIi(t). | (3.8) |
Let ∂H∂ui=0, i=1,2, we can obtain the optimal weighted control at time t satisfying
∗u1=N∑i=1(λ2i−λ1i)pSi(t)c1,∗u2=N∑i=1(λ4i−λ3i)pIi(t)c2. | (3.9) |
Considering the property of the control space, we have the optimal control in the compact notation
∗u1=min{max{0,N∑i=1(λ2i−λ1i)pSi(t)c1},1},∗u2=min{max{0,N∑i=1(λ4i−λ3i)pIi(t)c2},1}. | (3.10) |
In this section, to verify the results of the disease-free equilibrium, endemic equilibrium and optimal control problems, an undirected BA scale-free network consisting of 1000 nodes and 9908 edges is constructed, which obeys a power-law distribution pk=σk−υ. Specifically, k is defined as the degree of an individual, and the power-law exponent υ=3.516. The nodes in this network have a minimum degree kmin=10 and a maximum degree kmax=172. To be more realistic, u1 is considered to have an initial value, since there is a certain proportion of individuals who can spontaneously switch from the S to the A, which is partly excluded from the cost calculation. In addition, u2 has an initial value that fully accounts for the cost.
With no additional control strategy, the initial proportion of individuals in S and I are set at 80% and 20% of the total population, and the initial value of control strategy are u1=u2=0.2. Two scenarios are discussed below, by averaging 20 independent Monte Carlo simulations with the step of 0.2. For better comparison, different time scales are chosen for the Monte Carlo simulation and numerical simulation. In the first case, the former runs for 1500 steps and the latter for 1000 steps; in the second case, the former runs for 50 steps and the latter for 250 steps. The experimental results are shown in Figure 3.
For the first case, the parameters of the epidemic model are β1=0.50, β2=0, r=0.10, λ=0.50. When β2=0, all individuals will eventually enter state A after a time. This deduction is shown in Figure 3(a), (b), which show the change of the population proportion in each compartment under Monte Carlo simulation and numerical simulation, respectively. It can be seen that the proportion of infected individuals dropped to zero, and the model reached a disease-free equilibrium.
For the second case, the parameters of the epidemic model are β1=0.30, β2=0.10, r=0.10, λ=0.30. Figure 3(c), (d) show the change of the population density in each state under Monte Carlo simulation and numerical simulation, respectively. As shown in these figures, an endemic equilibrium state is reached. The proportion of I and A in Figure 3(c) is slightly smaller than that in Figure 3(d), the proportion of S and T is slightly larger.
Figure 3 shows that the curves under Monte Carlo simulation rises and falls faster than that under numerical simulation with a large spike. When β2=0, the system will reach a disease-free equilibrium, and when β2≠0, the system will enter an endemic equilibrium.
To evaluate the performance of the combinational optimal suppression control mechanism proposed in Section 3.2, we introduce the following heuristic control strategy, where the range of c1,c2 is [0, 1],
u1(t)=u1(0)+c1pSi,u2(t)=u2(0)+c2pIi. | (4.1) |
In this paper, we choose c1=c2=0.5.
In Figure 4, we give comparative results of the cumulative cost, proportion of infected persons and control strategies for the same network under the respective actions of optimal control strategy, heuristic control strategy and no control strategy. Optimal control refers to the combinational optimal suppression control described in Section 3.2. All Monte Carlo simulation results are obtained by averaging 20 independent experiments, where the propagation parameters are β1=0.50, β2=0.05, r=0.002, λ=0.50, c1=c2=10, d=0.02 and u1=0.2, u2=0.6 are taken as the initial values of the control strategy. Experiments run 40 steps at the step of 0.05 and at this time, a steady state has been reached.
As can be seen in Figure 4(a), the total cost under the optimal control strategy is the lowest, and that under no control strategy is the highest. Due to the fact that the heuristic control seeks only the best control effect regardless of cost, as is shown in Figure 4(b), (c), (f), the heuristic control strategy has the fewest infected individuals and the highest control cost. For the optimal control, the control strategy u2 is only taken at the beginning of the outbreak of the disease in Figure 4(e), while the control strategy u1 is slightly decreased in Figure 4(d). This is because it is more effective to use both strategies than either one alone, and improving the recovery rate is more effective than reducing the infection rate. To minimize the cost, the optimal control reduces the cost of the latter while increasing the cost of the former. Figure 4(c) shows that the optimal control strategy is stopped after the spread is suppressed, so its control cost is a little higher than no control strategy, but the curve is parallel to it as they all have initial control cost. It can be seen that both optimal control and heuristic control can suppress the spread of the disease. The heuristic is more costly, so its control effect is better. The optimal control strategy is more effective than other strategies at minimizing the total cost.
This paper presents the SAITS epidemic model with a combinational optimal suppression control strategy, which considers two measures to control the spread of the disease, moving more individuals to the compartment with low infection rate or the compartment with high recovery rate. The basic reproduction number of this model is calculated and the effect of each parameter on the outbreak threshold is discussed. It is found that increasing the recovery rate is more effective in suppressing the spread of the disease. We present an optimal problem with a trade-off in resource allocation considering the cost of the combinational optimal suppression control and global infection, and propose the structure of a general solution. A series of Monte Carlo simulations are performed to demonstrate the validity of the theoretical results. The costs of three control strategies, optimal, heuristic and no control strategies, are compared, and the experimental results show that optimal control is more effective in minimizing costs compared with other control strategies.
Our work provides new explanations for the dynamics of epidemic transmission, and conclusions drawn from the optimal control problem may provide effective ways for governments or other organizations to suppress viral or other transmission processes.
This work was supported by the National Key Research and Development Program of China under Grant 2019YFE0118700 and the National Natural Science Foundation of China under Grant 61873194.
The authors declare there is no conflict of interest.
[1] |
D. Chakrabarti, Y. Wang, C. Wang, J. Leskovec, C. Faloutsos, Epidemic thresholds in real networks, ACM Trans. Inf. Syst. Secur., 10 (2008), 1–26. https://doi.org/10.1145/1284680.1284681 doi: 10.1145/1284680.1284681
![]() |
[2] |
F. Di Lauro, J. C. Croix, M. Dashti, L. Berthouze, I. Z. Kiss, Network inference from population-level observation of epidemics, Sci. Rep., 10 (2020), 18779. https://doi.org/10.1038/s41598-020-75558-9 doi: 10.1038/s41598-020-75558-9
![]() |
[3] |
Y. Zhao, C. Huepe, P. Romanczuk, Contagion dynamics in self-organized systems of self-propelled agents, Sci. Rep., 12 (2022), 2588. https://doi.org/10.1038/s41598-022-06083-0 doi: 10.1038/s41598-022-06083-0
![]() |
[4] |
G. Albi, L. Pareschi, M. Zanella, Control with uncertain data of socially structured compartmental epidemic models, J. Math. Bio., 82 (2021), 63. https://doi.org/10.1007/s00285-021-01617-y doi: 10.1007/s00285-021-01617-y
![]() |
[5] |
J. M. Mendes, P. S. Coelho, Addressing hospitalisations with non-error-free data by generalised SEIR modelling of COVID-19 pandemic, Sci. Rep., 11 (2021), 19617. https://doi.org/10.1038/s41598-021-98975-w doi: 10.1038/s41598-021-98975-w
![]() |
[6] |
M. Shoaib, N. Anwar, I. Ahmad, S. Naz, A. K. Kiani, M. A. Z. Raja, Intelligent networks knacks for numerical treatment of nonlinear multi-delays SVEIR epidemic systems with vaccination, Int. J. Mod. Phys. B, 36 (2022). https://doi.org/10.1142/S0217979222501004 doi: 10.1142/S0217979222501004
![]() |
[7] |
G. Albi, L. Pareschi, M. Zanella, Modelling lockdown measures in epidemic outbreaks using selective socio-economic containment with uncertainty, Math. Biosci. Eng., 18 (2021), 7161–7190. https://doi.org/10.3934/mbe.2021355 doi: 10.3934/mbe.2021355
![]() |
[8] |
P. Di Giamberardino, D. Iacoviello, Evaluation of the effect of different policies in the containment of epidemic spreads for the COVID-19 case, Biomed. Signal Process. Control, 65 (2021), 102325. https://doi.org/10.1016/j.bspc.2020.102325 doi: 10.1016/j.bspc.2020.102325
![]() |
[9] |
K. M. A. Kabir, K. Kuga, J. Tanimoto, Effect of information spreading to suppress the disease contagion on the epidemic vaccination game, Chaos, Solitons Fractals, 119 (2019), 180–187. https://doi.org/10.1016/j.chaos.2018.12.023 doi: 10.1016/j.chaos.2018.12.023
![]() |
[10] |
X. Wang, D. Jia, S. Gao, C. Xia, X. Li, Z. Wang, Vaccination behavior by coupling the epidemic spreading with the human decision under the game theory, Appl. Math. Comput., 380 (2020), 125232. https://doi.org/10.1016/j.amc.2020.125232 doi: 10.1016/j.amc.2020.125232
![]() |
[11] |
M. Chinazzi, J. T. Davis, M. Ajelli, C. Gioannini, M. Litvinova, S. Merler, et al., The effect of travel restrictions on the spread of the 2019 novel coronavirus (COVID-19) outbreak, Science, 368 (2020), 395–400. https://doi.org/10.1126/science.aba9757 doi: 10.1126/science.aba9757
![]() |
[12] |
S. Kwon, A. D. Joshi, C. Lo, D. A. Drew, L. H. Nguyen, C. Guo, et al., Association of social distancing and face mask use with risk of COVID-19, Nat. Commun., 12 (2021), 3737. https://doi.org/10.1038/s41467-021-24115-7 doi: 10.1038/s41467-021-24115-7
![]() |
[13] |
H. Shi, Z. Duan, G. Chen, R. Li, Epidemic spreading on networks with vaccination, Chin. Phys. B, 18 (2009), 3309–3317. https://doi.org/10.1088/1674-1056/18/8/035 doi: 10.1088/1674-1056/18/8/035
![]() |
[14] |
A. S. Alsheri, A. A. Alraeza, M. R. Afia, Mathematical modeling of the effect of quarantine rate on controlling the infection of COVID19 in the population of Saudi Arabia, Alexandria Eng. J., 61 (2022), 6843–6850. https://doi.org/10.1016/j.aej.2021.12.033 doi: 10.1016/j.aej.2021.12.033
![]() |
[15] |
D. Xu, X. Xu, Y. Xie, C. Yang, Optimal control of an SIVRS epidemic spreading model with virus variation based on complex networks, Commun. Nonlinear Sci. Numer. Simul., 48 (2017), 200–210. https://doi.org/10.1016/j.cnsns.2016.12.025 doi: 10.1016/j.cnsns.2016.12.025
![]() |
[16] |
A. P. Lemos-Paião, C. J. Silva, D. F. M. Torres, An epidemic model for cholera with optimal control treatment, J. Comput. Appl. Math., 318 (2017), 168–180. https://doi.org/10.1016/j.cam.2016.11.002 doi: 10.1016/j.cam.2016.11.002
![]() |
[17] |
L. Miclo, D. Spiro, J. Weibull, Optimal epidemic suppression under an ICU constraint: An analytical solution, J. Math. Econ., 101 (2022), 102669. https://doi.org/10.1016/j.jmateco.2022.102669 doi: 10.1016/j.jmateco.2022.102669
![]() |
[18] |
Y. Wu, Y. Sun, M. Lin, SQEIR: An epidemic virus spread analysis and prediction model, Comput. Electr. Eng., 102 (2022), 108230. https://doi.org/10.1016/j.compeleceng.2022.108230 doi: 10.1016/j.compeleceng.2022.108230
![]() |
[19] |
A. Kumar, P. K. Srivastava, Y. Dong, Y. Takeuchi, Optimal control of infectious disease: Information-induced vaccination and limited treatment, Phys. A: Stat. Mech. Appl., 542 (2020), 123196. https://doi.org/10.1016/j.physa.2019.123196 doi: 10.1016/j.physa.2019.123196
![]() |
[20] |
G. Dimarco, G. Toscani, M. Zanella, Optimal control of epidemic spreading in the presence of social heterogeneity, Philos. Trans. R. Soc. A, 380 (2022), 2224. https://doi.org/10.1098/rsta.2021.0160 doi: 10.1098/rsta.2021.0160
![]() |
[21] |
G. Zaman, Y. Kang, G. Cho, I. H. Jung, Optimal strategy of vaccination & treatment in an SIR epidemic model, Math. Comput. Simul., 136 (2017), 63–77. https://doi.org/10.1016/j.matcom.2016.11.010 doi: 10.1016/j.matcom.2016.11.010
![]() |
[22] |
Y. Pan, Z. Yan, The impact of individual heterogeneity on the coupled awareness-epidemic dynamics in multiplex networks, Chaos, 28 (2018), 063123. https://doi.org/10.1063/1.5000280 doi: 10.1063/1.5000280
![]() |
[23] |
P. van den Driessche, J. Watmough, Reproduction numbers and sub-threshold endemic equilibria for compartmental models of disease transmission, Math. Biosci., 180 (2002), 29–48. https://doi.org/10.1016/S0025-5564(02)00108-6 doi: 10.1016/S0025-5564(02)00108-6
![]() |