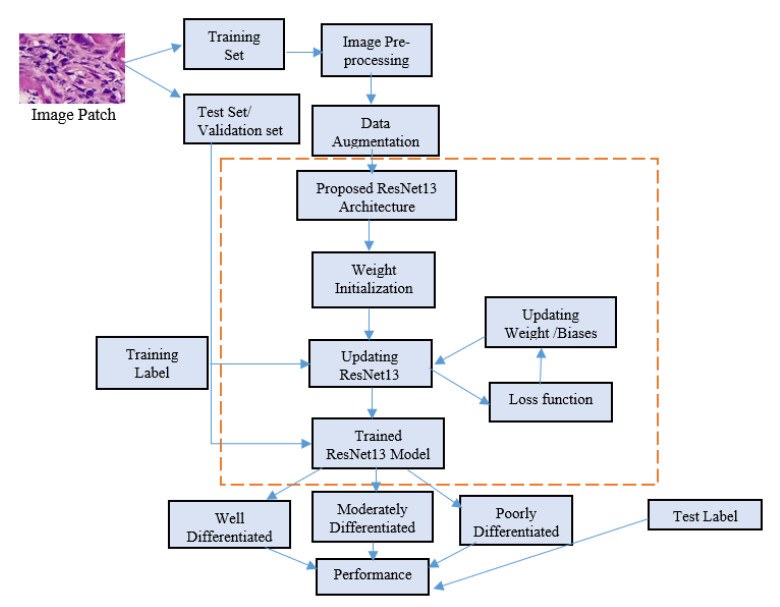
Oral cancer is a prevalent disease happening in the head and neck region. Due to the high occurrence rate and serious consequences of oral cancer, an accurate diagnosis of malignant oral tumors is a major priority. Thus, early diagnosis is very effective to give the patient a prompt response to treatment. The most efficient way for diagnosing oral cancer is from histopathological imaging, which provides a detailed view of inside cells. Accurate and automatic classification of oral histopathological images remains a difficult task due to the complex nature of cell images, staining methods, and imaging conditions. The use of deep learning in imaging techniques and computational diagnostics can assist doctors and physicians in automatically analysing Oral Squamous Cell Carcinoma biopsy images in a timely and efficient manner. Thus, it reduces the operational workload of the pathologist and enhance patient management. Training deeper neural networks takes considerable time and requires a lot of computing resources, due to the complexity of the network and the gradient diffusion problem. With this motivation and inspired by ResNet's significant successes to handle the gradient diffusion problem, in this study we suggest the novel improved ResNet-based model for the automated multistage classification of oral histopathology images. Three prospective candidate model blocks are presented, analyzed, and the best candidate model is chosen as the optimal one which can efficiently classify the oral lesions into well-differentiated, moderately-differentiated and poorly-differentiated in significantly reduced time, with 97.59% accuracy.
Citation: Santisudha Panigrahi, Ruchi Bhuyan, Kundan Kumar, Janmenjoy Nayak, Tripti Swarnkar. Multistage classification of oral histopathological images using improved residual network[J]. Mathematical Biosciences and Engineering, 2022, 19(2): 1909-1925. doi: 10.3934/mbe.2022090
[1] | Yufeng Li, Chengcheng Liu, Weiping Zhao, Yufeng Huang . Multi-spectral remote sensing images feature coverage classification based on improved convolutional neural network. Mathematical Biosciences and Engineering, 2020, 17(5): 4443-4456. doi: 10.3934/mbe.2020245 |
[2] | Shuai Cao, Biao Song . Visual attentional-driven deep learning method for flower recognition. Mathematical Biosciences and Engineering, 2021, 18(3): 1981-1991. doi: 10.3934/mbe.2021103 |
[3] | Boyang Wang, Wenyu Zhang . ACRnet: Adaptive Cross-transfer Residual neural network for chest X-ray images discrimination of the cardiothoracic diseases. Mathematical Biosciences and Engineering, 2022, 19(7): 6841-6859. doi: 10.3934/mbe.2022322 |
[4] | Long Wen, Yan Dong, Liang Gao . A new ensemble residual convolutional neural network for remaining useful life estimation. Mathematical Biosciences and Engineering, 2019, 16(2): 862-880. doi: 10.3934/mbe.2019040 |
[5] | Eric Ke Wang, Nie Zhe, Yueping Li, Zuodong Liang, Xun Zhang, Juntao Yu, Yunming Ye . A sparse deep learning model for privacy attack on remote sensing images. Mathematical Biosciences and Engineering, 2019, 16(3): 1300-1312. doi: 10.3934/mbe.2019063 |
[6] | S. Dinesh Krishnan, Danilo Pelusi, A. Daniel, V. Suresh, Balamurugan Balusamy . Improved graph neural network-based green anaconda optimization for segmenting and classifying the lung cancer. Mathematical Biosciences and Engineering, 2023, 20(9): 17138-17157. doi: 10.3934/mbe.2023764 |
[7] | Tongping Shen, Fangliang Huang, Xusong Zhang . CT medical image segmentation algorithm based on deep learning technology. Mathematical Biosciences and Engineering, 2023, 20(6): 10954-10976. doi: 10.3934/mbe.2023485 |
[8] | Jun Gao, Qian Jiang, Bo Zhou, Daozheng Chen . Convolutional neural networks for computer-aided detection or diagnosis in medical image analysis: An overview. Mathematical Biosciences and Engineering, 2019, 16(6): 6536-6561. doi: 10.3934/mbe.2019326 |
[9] | Hassan Ali Khan, Wu Jue, Muhammad Mushtaq, Muhammad Umer Mushtaq . Brain tumor classification in MRI image using convolutional neural network. Mathematical Biosciences and Engineering, 2020, 17(5): 6203-6216. doi: 10.3934/mbe.2020328 |
[10] | Jiyun Shen, Yiyi Xia, Yiming Lu, Weizhong Lu, Meiling Qian, Hongjie Wu, Qiming Fu, Jing Chen . Identification of membrane protein types via deep residual hypergraph neural network. Mathematical Biosciences and Engineering, 2023, 20(11): 20188-20212. doi: 10.3934/mbe.2023894 |
Oral cancer is a prevalent disease happening in the head and neck region. Due to the high occurrence rate and serious consequences of oral cancer, an accurate diagnosis of malignant oral tumors is a major priority. Thus, early diagnosis is very effective to give the patient a prompt response to treatment. The most efficient way for diagnosing oral cancer is from histopathological imaging, which provides a detailed view of inside cells. Accurate and automatic classification of oral histopathological images remains a difficult task due to the complex nature of cell images, staining methods, and imaging conditions. The use of deep learning in imaging techniques and computational diagnostics can assist doctors and physicians in automatically analysing Oral Squamous Cell Carcinoma biopsy images in a timely and efficient manner. Thus, it reduces the operational workload of the pathologist and enhance patient management. Training deeper neural networks takes considerable time and requires a lot of computing resources, due to the complexity of the network and the gradient diffusion problem. With this motivation and inspired by ResNet's significant successes to handle the gradient diffusion problem, in this study we suggest the novel improved ResNet-based model for the automated multistage classification of oral histopathology images. Three prospective candidate model blocks are presented, analyzed, and the best candidate model is chosen as the optimal one which can efficiently classify the oral lesions into well-differentiated, moderately-differentiated and poorly-differentiated in significantly reduced time, with 97.59% accuracy.
Oral cancer is a chronic disease comes under the head and neck region, which includes the oral cavity, nasopharynx, and pharynx [1]. Due to the negligence of patients with precancerous signs, it leads to carcinoma stage in a short duration and turns into a life-threatening disease. Thus, the early detection of the oral tumor is essential for providing a better treatment plan to the patients by increasing the survival rate [2]. With the advent of computer-aided diagnostic (CAD) systems and histopathology of tissues, there is a massive accumulation of progressive digital histopathological images. Hence, there is a necessity for the automated analysis of these images. Many computer-aided methodologies have been developed for oral cancer diagnosis using machine learning and deep learning methods [3,4]. Deep learning is widely accepted by various researchers for analyzing the data for classification or prediction.
For the oral cancer analysis, biopsy sections are taken and put on a glass slide. For accurate diagnosis hematoxylin and eosin (H & E) stain for the slides is mostly used that are subsequently examined beneath a microscope. A pathologist uses a microscope to analyze the distortions (i.e., the numerous elements on the slide, such as cell organization, cell size, form and shape, and so on) to identify cancer. The patient is then diagnosed based on the pathologist's report by an oncologist. For that reason, the study should be as precise as possible. However, the whole manual observation process for feature analysis of each slide is arduous, time-consuming, and requires a significant amount of domain knowledge. Furthermore, the report may be skewed by the observer [5]. Due to these issues, an automated version of the procedure described above is the need which can reduce the bias and time by improving the feature evaluation process. Additionally, as digital histopathological images contain specific characteristics, special processing techniques are very much required for their analysis. Therefore, an automated diagnostic system is required for the screening of oral cancer, which can overcome the issues of the manual observation process. It will also be beneficial in large-scale observatories and cancer screening camps, where the vast majority of cases are benign. Therefore, the pathologist can concentrate on the cases that the system has identified as cancerous [6]. For various cancer diagnosis and grading applications, a computer-aided histopathology research has been performed [7,8]. Thereafter, many machine learning and deep learning models have been developed which can effectively predict the outcome of cancer type by extracting and identifying patterns and associations from datasets [9,10,11].
Oral malignancy can come in a variety of forms, such as "squamous cell carcinoma", "verrucous carcinoma", "minor carcinoma of the salivary gland", "lymph epithelial carcinoma", etc. From literature [12], it is seen that Squamous cell carcinoma (SCC) accounts for about 90% of oral disorders. In a healthy state, the throat and mouth are typically lined with stratified squamous cells, and only a single layer of this squamous cell's basement membrane is connected with it. By adhering to one layer, the inherent stability of the epithelial layer is protected. This inherent stability is lost when cancer develops. SCC is categorized into three types: "WDSCC (well-differentiated SCC)", "MDSCC (moderately-differentiated SCC)", and "PDSCC (poorly-differentiated SCC)" [13]. Well-differentiated cells are the low grade or grade Ⅰ tumors. These cells are well-organized and have the appearance of healthy tissue. The tumor cells of high grade or grade Ⅲ are poorly differentiated. Moderately differentiated cancer cells are those that don't appear to be well-differentiated or poorly-differentiated.
The progress of deep learning techniques has dramatically impacted the performances in various tasks of the medical domain [14,15]. To understand better representations, a deeper network has to be trained. The training and optimization of a deeper network are more complicated than a shallow network [16]. Although Convolutional neural networks have many successful breakthroughs for the image classification tasks [17,18,19], they are difficult to train for two reasons. First, due to the exponential decrease of the gradient the front layers train very slowly. Second, Convolutional Neural Network (CNN) models have more parameters leading to the complexity of the network, thus requires longer time to train [20]. Recent advances in deep learning methodologies can be abridged as learning methodologies, initializations, and activation functions for training more complex architectures. Though activation functions and batch normalization have made tremendous progress in decreasing the influence of exploding/vanishing gradients, optimizing a neural network with profound architecture remains a challenge [21]. To handle this challenge, we have proposed residual network architectures trained from scratch to classify the oral cancer histopathological images into three categories.
The following are the key contributions of this article:
1. We proposed three ResNet architectures for the multistage classification of the OSCC into well-differentiated, moderately-differentiated, and poorly-differentiated. These architectures work well by providing better accuracy and preserving the information across layers.
2. The study is focused in training the different proposed variants of ResNet from scratch and comparing their efficiency for the defined problem statement.
3. The proposed ResNet architectures provide faster convergence with less computational complexity to provide superior performance with a small dataset.
4. To prevent overfitting, data augmentation techniques, activation functions, and parameter optimizations are employed.
This article is systematically arranged as follows. In section 2, the relevant, related works are discussed. The material and methods for the experimentation as well as model structures and parameters are represented in Section 3. The comparison among different variants of ResNet and the experimental results are discussed in Section 4. Finally, the conclusion and future work is presented in Section 5.
Researchers have performed oral cancer classification in the literature by using machine learning and deep learning techniques. Kim et al. [20] and Mohd et al. [9] have done the retroactive study for the early diagnosis of oral cancer and predicted the survival rate. Most of the research emphasizes on the application of machine learning methods to detect oral submucous fibrosis (OSF) [11,22,23]. The chronic nature OSF can lead to oral cancer on progression. Studies were done by T. Y. Rahman et al. [24] and T. Y. Rahman et al. [25] is based on the binary categorization of OSCC. These studies have reported that using Support Vector Machine (SVM) and Linear Discriminant Classifier (LDA, ) 100% classification accuracy was achieved using texture, color and shape features. The conventional approach followed by the pathologists is subjective in nature. Apart from that, the significant challenges for the manual assessment are the variability of the microscope, the quality of the stains/slides, domain knowledge of the pathologist, time allotted for each observation etc. These factors may result in a diagnostic mistake or delay in the follow-up process [26]. In contrast, automated systems applying deep learning techniques eradicate the necessity for domain proficiency and explicit feature engineering. Deep learning algorithms can now achieve superior classification accuracy for histopathological images without requiring manual feature representation of the input data due to the availability of high-performance GPUs [27,28]. Santisudha et al. have suggested deep learning-based models for the binary categorization of OSCC [29,30] that outperformed the other baseline models in this domain. For taking appropriate clinical decisions, a biopsy image is regarded as the gold standard in pathology [31]. Many studies are found in the literature associated with deep learning utilizing biopsy images for classification tasks [11,18]. Thus, deep learning approaches are predicted to outperform conventional machine learning methods without the need for selective feature engineering.
Navarun et al. [32] have employed transfer learning with four pre-trained models and compared them with a proposed CNN model to classify four types of OSCC. They have replaced the fully connected layers for random weight initialization to relearn from oral cancer histopathological images. The literature reveals the importance of using machine learning and deep learning methods for the classification of oral cancer.
Lamia H. et al. [33] have developed an automatic segmentation of brain tumor from MRI images by using Deep Residual Network. They have achieved superior performance with 3% faster computation time compared to other DCNN models. Hao Zhu et al. [34] have proposed a ResNet model for the remote sensing classification and achieved competitive performance. There are many articles highlighting the importance of ResNet in various domains such as malaria detection [35], mask detection [36], machine health monitoring [37] etc.
The issues associated with training deeper networks is the degradation problem in which, the accuracy of the deeper neural network will increase initially until reaching saturation. After that, the accuracy will diminish as the depth is increased [38]. To overcome this challenge as well as the increase of huge parameters of the models, we have proposed simple and improved residual networks with smaller dataset. To our knowledge, no other study has used histological images of oral cancer to demonstrate the efficiency of residual networks.
The proposed study considered the multistage classification or grading of the oral histopathological images using ResNet architecture's different variations and depth. The outcomes of all variations are compared, and the best performing approach for classification is determined.
For the present study, we have used oral biopsy images to evaluate and analyze the influence of using various residual blocks. The variants of residual blocks are compared and validated. First, the oral cancer histopathological image dataset is generated.
An expert pathologist performed ground-truth labeling by identifying the region of interest (ROI), from which the study dataset was created by extracting the image patches. Then, dataset split-up 70%, 10% and 20% training, validation and testing respectively is done based on the train-test strategy [16]. Thereafter we applied data pre-processing and data augmentation. Finally, classification is done with different candidate residual block generation. The diagrammatic depiction of the study approach used is shown in Figure 1.
The training dataset is used to fit the model i.e. the weights and biases. A validation dataset is used to tune the model hyperparameters i.e. the architecture of the model. Test dataset is used to evaluate the performance of the trained model.
Histological hematoxylin and eosin-stained sections of cancerous oral lesion are obtained from the Institute of Dental Sciences (IDS), SUM Hospital, Bhubaneswar, India. The data was gathered with consideration for the patient's concerns as well as ethics committee approval (Ref No./DMR/IMS.SH/SOA/1800040). The slides were viewed under Lawrence and Mayo research microscope and images captured with 5 MP CMOS camera, 100x resolution and stored digitally on the computer as high quality JPEG images. 400 image patches were collected from each category of oral cancer stages such as: "well-differentiated", "moderately-differentiated" and "poorly-differentiated". The image patches of size 256 × 256 were extracted. Sample image for each class is shown in Figure 2.
The median filter of size 3 × 3 has applied for the image patches to reduce the noise such as some bright and black pixels associated with capturing the images through the microscope [39,40].
Data augmentation is a simple yet effective strategy for preventing overfitting in models and improving the final results [41]. We need more image augmentations to improve the model's generalization capability. Different transformations are selected, including flipping, cropping, scaling, shifting, rotating (30°, 45°, 60°, 90°, 105°). Due to the small dataset available, we have applied heavy data augmentation to obtain 8400 training image patches. The aforementioned nine modifications are applied to 840 (70%) training image patches, including the original set, resulting in 8400 training image patches.
A deep convolution neural network has made several notable advancements in image classification [42]. However, to analyze the convergence of deeper networks, there lies the degradation challenge in developing deep learning models.
The accuracy of the deeper neural network will increase initially until reaching saturation then diminish as the depth is increased [38]. It is recognized that the influence is not caused by overfitting as the error grows in both the training as well as test sets. In 2015, this model was the winner of the ImageNet competition. ResNet is allowed to train profound neural networks with 150+ layers successfully. The ResNet50 was proposed by He et al. [43] with 50 residual network layers. The researchers compared various deep learning models and evaluated the deeper ResNet in a significant period of time, concluding that ResNet can enhance accuracy by increasing depth and outperforms other models for classification tasks. The key visual features of OSCC such as the structural variances of epithelial layers and the formation of keratin pearls extraction are the challenging task for the oral cancer classification. Here, the ResNet need to train from a very small oral histopathological image dataset available for this study. The primary motive of this study is to extract the key features of OSCC with limited dataset and to resolve the degradation issue of the neural network.
There are numerous different types of residual components, which can be further described based on the problem needs. The residual components of ResNet employed in this study are shown in Figure 3, which effectively overcomes the degradation problem. Figure 4. represents the common layered architecture of the ResNet for all the three variants of Figure 3 (a), (b) and(c). The residual component in Figure 3(a) is composed of three convolution layers in the main path and one convolution layer in skip path. The residual blocks have convolution sizes 1 × 1, 3 × 3, and 1 × 1. The Figure 3(b) residual block uses two convolutions of size 3 × 3 in the direct path and one convolution of 1x1 in the skip path. The Figure 3(c) residual block is similar to Figure 3(a) but without batch normalization. In all three models, the depth of 13 convolutions is considered with convolution kernel size is 3 × 3.
We have tried out with higher number of convolution layers and observed that 13 is the minimum number of convolution layers required to give optimal performance. Thus, the models can be referred as ResNet13-A, ResNet13-B and ResNet13-C in the following sections. For ResNet13-A and ResNet13-C, the corresponding residual blocks are repeated 3 times whereas for ResNet13-B the residual block is repeated four times to make the depth of the same in all cases. Thus, the three models can be comparable. The direct path and skip path in the residual module have the same output dimensions and can be combined directly.
Figure 5 depicts two stacked layers building block that is determined as:
H(x)=R(x)+x; | (1) |
Where the input and output vectors of the residual blocks are x and H(x) respectively. The residual mapping to be learned is denoted by R(x).
Rectified linear unit (ReLU) is used as the activation function for our deep learning models [44]. The softmax function is used in the prediction layer to convert the output layer's results to a probability distribution for the multi-class classification task [45].
The proposed models were employed on a system having the subsequent specifications: Quadro P5200 with a six-core i7 processor, 32 GB of GDDR5 RAM, and NVIDIA-2560 CUDA processing cores, 16 GB GPU (32 GB GDDR5 graphics memory and 2560 CUDA cores). In addition, Keras (high-level neural network library run on TensorFlow or Theano) based on the python interface was used to implement the experimental framework of Reidual Network (ResNet) models for oral lesion classification.
During this study, various experiments have been carried out to evaluate ResNet's performance in detecting different stages of oral cancer.
Certain deep learning terms are already present in the suggested architectures, however hyperparameter tuning is done to obtain the optimum configuration for our task. ResNet is used, with an initial learning rate of 0.01 and 50 epochs. Furthermore, the network's mini-batch size is set to 32. Stochastic Gradient Descent with Momentum (SGDM) [46] and Adam [47] have been chosen as our optimizer techniques.
It is observed from the literature that, convergence is inhibited and degradation happens as the number of layers in a CNN grows larger [48]. The residual networks are computationally more efficient and can achieve a higher degree of accuracy from significantly enhanced depth. ResNet has received much interest in research due to its acceptance and effectiveness in image classification [35,36] and. many ResNet variations have been proposed. The performance of the different variants proposed in this study are compared in Figure 6.
Model comparison and results –All the variants of proposed Residual Network models are validated, compared and the results have been discussed in terms of
ⅰ. Learning curves
ⅱ. Evaluation metrics
ⅲ. Comparison with other cutting-edge models.
i. Learning curves
Learning curves are a visual representation of the incremental epoch-based evaluation of a classifier's learning performance. The learning curves are the accuracy and loss curves for training as well as validation set.
Figure 6 shows the accuracy and loss curves of the training progression for the proposed models over 50 epochs. It indicates that after each epoch, the training loss linearly decreases, and the training accuracy increases. Model ResNet13-C is not generalizing well compared to the other two models due to the absence of batch normalization as observed from Figure 6(c). From the learning curves of ResNet13-C, Figure 6(c), it's seen that the training accuracy is much higher than the validation accuracy. Thus it overfits the training dataset leading to not generalize well for the unseen data. Batch normalization is a method used for addressing the difficulties of training deep neural networks which stabilizes the learning process and significantly reduces the number of training epochs essential for training the networks [21].
The comparison of accuracy and loss values of the ResNet variants are shown in Figure 7. The accuracy and error rate differs amongst the different variants, and the model ResNet13-A perform better by achieving an accuracy of 97.59%. Model ResNet13-B becomes stable after 40 epoch by giving accuracy of 96.67% which is also comparable with model ResNet13-A with little lower performance. Including ReLU after the addition, there is a small performance difference. Conversely, the difference in validation accuracy for both Batch Normalization (BN) and non-BN networks is ≈ 15%, making it evident that the BN network's generalization capability is substantially higher than that of its non-BN counterparts. This evaluation shows that BN can help a network generalize more effectively. On average, ResNet13-A has a lower error rate than ResNet13-B and ResNet13-C.
The time taken for the execution of the model ResNet13-A and ResNet13-B are 21473 and 23848.5 seconds respectively. Though both the models have same convolutional depth, the model ResNet13-A is taking less time compared to the model ResNet13-B. Hence, as the ResNet13-A is providing better accuracy with less time compared to other models, it is adopted as the final proposed model structure.
ii. Evaluation metrics
To assess the effectiveness of our proposed model, from various classification performance measures, the benchmark metrics such as sensitivity, specificity, accuracy, precision and f-measure are used in this study. The following terminologies are used to represent sensitivity (true positive rate), specificity (true negative rate), and accuracy.
Sensitivity=TPR=∑liTPiTPi+FNilSpecificity=TNR=∑liTNiTNi+FPilAccuracy=Acc=∑liTPi+TNiTPi+TNi+FPi+FNilPrecision=∑liTPiTPi+FPilF−measure=2×Precision×Recallprecision+Recall |
Where TPi, FPi, TNi and FNi represents true-positive, false-positive, true-negative and false-negative classes respectively. l signifies the total classes.
In the medical domain, both sensitivity and specificity contribute significantly in assessing the strength of the model. The metric accuracy correlates with sensitivity and specificity while evaluating the model's performance. From Table 1, it can be observed that the values of sensitivity and specificity are quite balanced, which is required for an outstanding model. Thus, ResNet13-A should be considered as the best performing model for multiclass classification of oral lesions.
Sensitivity | Specificity | Accuracy | Precision | F-measure | |
Well-differentiated | 0.93 | 0.92 | 0.93 | 0.96 | 0.94 |
Moderately-differentiated | 0.94 | 0.93 | 0.93 | 0.98 | 0.95 |
Poorly-differentiated | 0.95 | 0.97 | 0.96 | 0.97 | 0.96 |
iii. Comparison with other cutting-edge models
A novel multistage cancer detection approach based on ResNet is proposed in this work which can handle the degradation issue. We compare the effectiveness of our model with machine and deep learning baseline architectures. The ResNet13-A model consists of convolutional layer, batch normalization, rectified linear activation unit and an identity mapping, which is more robust compared to the training procedure of CNN. Furthermore, the ability to extract higher-level data characteristics that are more complex, distinguishes ResNet13-A architecture from others. This comparison of different methodologies provided some light on the advantages of adopting ResNet over other techniques. In identifying distinct phases of cancer cells, the development system ResNet13-A model achieved the best average accuracy of 97.59 %, which is analogous to various cutting-edge methods.
The efficiency of the suggested model is compared to the earlier research approaches relating to the accuracy metric. While the majority of the literature uses machine learning techniques, the suggested model, CNN, and CapsuleNet are deep learning architectures. In a traditional machine learning technique that involves an expert-dependent handcrafted feature extraction technique, a small dataset (between 10 and 500) was used. The coarse features are extracted independently using the deep learning approach, and the efficiency improves as the data size grows. The SVM model by Krishnan et al. [40] and backpropagation-based ANN model by Belvin et al. [41] have outperformed our outcome by 2.07% and 0.33% respectively, since the features considered for the study are well-defined handcrafted features and the dataset evaluated is substantially less than the suggested method. Even though different datasets were used in the existing literature, the aim of the study is the same, and each of them examined oral cancer histopathological images. From this perspective, we should deduce from Table 2 that our method is highly comparable with current work done in the field of OSCC to the best of our knowledge, demonstrating the practicality of the solution proposed. This demonstrates that the proposed method for detecting multistage malignant tissue would be useful for computer-assisted diagnosis of OSCC. The ResNet design incorporates the skip connections to speed up computation and reduce training error compared to existing architectures.
Methodology | Image Size | Features | Classification methods | Accuracy (%) |
Krishnan et al. [10] | Normal-90 OSFWD-42 OSFD-26 |
71 features of wavelet family(LBP, Gabor, BMC) | SVM | 88.38 |
Krishnan et al. [3] | Normal-90 OSFWD-42 OSFD-26 |
HOS, LBP, LTE |
Fuzzy | 95.7 |
Krishnan et al. [40] | Normal-341 OSF-429 | Morphological and textural features | SVM | 99.66 |
Belvin et al. [49] | 16 malignant images with 192 patches | Texture features and Run Length features | Back propagation-based ANN | 97.92 |
Anuradha. K. et al. [50] | Energy, entropy, Contrast, Correlation, Homogeneity |
SVM | 92.5 | |
DevKumar et al. [4] | High grade-15 Low grade-25 Healthy-2 |
Identification of various layers –epithelial, subepithelial, keratin region and keratin pearls | Random Forest | 96.88 |
Santisudha et al. [29] | Malignant-214 Benign-172 |
CNN | 96.77 | |
Santisudha et al. [30] | Malignant-414 Benign-372 |
Capsule network | 97.35 | |
Navarun et al. [32] | Benign-1656 WDSCC-2634 MDSCC-2110 PDSCC - 1921 |
CNN | 97.5 | |
Nanditha B R et al. [51] | Benign-63 Malignant-269 |
Ensemble model (ResNet50 and VGG16) | 96.2 | |
Proposed method | WDSCC-400 MDSCC-400 PDSCC-400 |
Residual Network | 97.59 |
In this work, we have proposed a novel model for the classification of oral cancer into multiple stages based on histopathological image data. Three prospective candidate model blocks are trained from scratch, and the best candidate model is chosen as the optimal ResNet model (ResNet13-A) which is an automated computer-aided method to obtain high-performance results with less computational complexity and small dataset. Furthermore, performance metrics specifically accuracy, sensitivity, specificity, precision, F-measure and loss rates had been studied. The suggested model achieved 97.59% accuracy for multistage classification, which is comparable with several state-of-the-art approaches. Therefore, the proposed ResNet model is an efficient model for detecting multistage oral cancer, and that can be utilized as a diagnostic tool to help physicians in daily clinical screening.
We have not employed the dropout regularization in our present implementation, which has demonstrated to improve performance in deep networks. We will train the networks using dropout regularization in the future and assess its outcome. Furthermore, we intend to test our network on a suitably large dataset, which will broaden the scope of the findings given in this paper.
The dataset used for this study were obtained from the Institute of Dental Sciences (IDS), SUM Hospital, Bhubaneswar, India. Authors would like to thank and acknowledge the doctors and the pathologist from IDS for data collection.
The authors declare that there is no conflicts of interest associated with this publication, and there has been no significant financial support for this work that could have influenced its outcome.
[1] | National Institutes of Health, NIH Fact Sheets-Oral Cancer, (2018), 1–2. Available from: http://www.nidcr.nih.gov. |
[2] |
B. Timuçin, H. H. Yilmaz, Ü. Aydin, M. A. Aydin, M. C. Aksoy, Derya Yildirim, Early diagnosis of oral cancer, J. Int. Med. Res., 38 (2010), 737–749. doi: 10.1177/147323001003800302. doi: 10.1177/147323001003800302
![]() |
[3] |
M. M. R. Krishnan, V. Venkatraghavan, U. R. Acharya, M. Pal, R. R. Paul, L. C. Min, et al., Automated oral cancer identification using histopathological images: a hybrid feature extraction paradigm, Micron, 43 (2012), 352–364. doi: 10.1016/j.micron.2011.09.016. doi: 10.1016/j.micron.2011.09.016
![]() |
[4] |
D. D. Kumar, S. Bose, A. K. Maiti, B. Mitra, G. Mukherjee, P. K. Dutta, Automatic identification of clinically relevant regions from oral tissue histological images for oral squamous cell carcinoma diagnosis, Tissue Cell, 53 (2018), 111–119. doi: 10.1016/j.tice.2018.06.004. doi: 10.1016/j.tice.2018.06.004
![]() |
[5] |
M. N. Gurcan, L. E. Boucheron, A. Can, A. Madabhushi, N. M. Rajpoot, B. Yener, Histopathological image analysis: A review, IEEE Rev. Biomed. Eng., 2 (2009), 147–171. doi: 10.1109/RBME.2009.2034865. doi: 10.1109/RBME.2009.2034865
![]() |
[6] |
D. Komura, I. Shumpei, Machine learning methods for histopathological image analysis, Comput. Struct. Biotechnol. J., 16 (2018), 34–42. doi: 10.1016/j.csbj.2018.01.001. doi: 10.1016/j.csbj.2018.01.001
![]() |
[7] | D. Wang, A. Khosla, R. Gargeya, H. Irshad, A. H. Beck, Deep learning for identifying metastatic breast cancer, (2016), arXiv preprint arXiv: 1606.05718. |
[8] | D. Wang, D. J. Foran, J. Ren, H. Zhong, I. Y. Kim, X. Qi, Exploring automatic prostate histopathology image gleason grading via local structure modelling, in 2015 37th Annual International Conference of the IEEE Engineering in Medicine and Biology Society (EMBC), (2015), 2649–2652. doi: 10.1109/EMBC.2015.7318936. |
[9] | F. Mohd, N. Noor, Z. A. Bakar, Z. A. Rajion, Analysis of oral cancer prediction using features selection with machine learning, in 2015 The 7th International Conference on Information Technology (ICIT), (2015), 283–288. doi: 10.15849/icit.2015.0058. |
[10] | M. M. R. Krishnan, C. Chakraborty, A. K. Ray, Wavelet based texture classification of oral histopathological sections, Int. J. Microsc. Sci. Technol. Appl. Educ., 2 (2010), 897–906. |
[11] | R. Patra, C. Chakraborty, J. Chatterjee, Textural analysis of spinous layer for grading oral submucous fibrosis, Int J. Comput Appl., 47 (2012), 975–8887. |
[12] |
P. H. Montero, S. G. Patel, Cancer of the oral cavity, Surg. Oncol. Clin., 24 (2015), 491–508. doi: 10.1016/j.soc.2015.03.006. doi: 10.1016/j.soc.2015.03.006
![]() |
[13] |
M. Akhter, S. Hossain, Q. B. Rahman, M.R. Molla, A study on histological grading of oral squamous cell carcinoma and its co-relationship with regional metastasis, J. Oral Maxillofac. Pathol., 15 (2011), 168. doi: 10.4103/0973-029X.84485. doi: 10.4103/0973-029X.84485
![]() |
[14] |
M. Y. Chen, H. S. Chiang, E. Lughofer, E. Egrioglu, Deep learning: Emerging trends, applications and research challenges. Soft Comput., 24 (2020), 7835–7838. doi: 10.1007/s00500-020-04939-z. doi: 10.1007/s00500-020-04939-z
![]() |
[15] | A. Krizhevsky, I. Sutskever, G. E. Hinton, Imagenet classification with deep convolutional neural networks, Adv. Neural Inf. Process. Syst., 25 (2012), 1097–1105. |
[16] |
S. P. Mohanty, D. P. Hughes, M. Salathe, Using deep learning for image-based plant disease detection, Front. Plant Sci., 7 (2016), 1419. doi: 10.3389/fpls.2016.01419. doi: 10.3389/fpls.2016.01419
![]() |
[17] | S. H. Kassani, P. H. Kassani, M. J. Wesolowski, K. A. Schneider, R. Deters, Classification of histopathological biopsy images using ensemble of deep learning networks, (2019), arXiv preprint arXiv: 1909.11870. |
[18] | K. Simonyan, A. Zisserman, Very deep convolutional networks for large-scale image recognition, (2014), arXiv preprint arXiv: 1409.1556. |
[19] | C. Szegedy, W. Liu, Y. Jia, P. Sermanet, S. Reed, D. Anguelov, et al., Going deeper with convolutions, in Proceedings of the IEEE conference on computer vision and pattern recognition, (2015), 1–9. doi: 10.1109/CVPR.2015.7298594. |
[20] |
D. W. Kim, S. Lee, S. Kwon, W. Nam, I. H. Cha, H. J. Kim, Deep learning-based survival prediction of oral cancer patients, Sci. Rep., 9 (2019), 1–10. doi: 10.1038/s41598-019-43372-7. doi: 10.1038/s41598-019-43372-7
![]() |
[21] | D. Gaur, J. Folz, A. Dengel, Training Deep Neural Networks Without Batch Normalization, (2020), arXiv preprint arXiv: 2008.07970. |
[22] |
M. Krishnan, U. Acharya, C. Chakraborty, A. Ray, Automated diagnosis of oral cancer using higher order spectra features and local binary pattern: A comparative study, Technol. Cancer Res. Treat., 10 (2011), 443–455. doi: 10.7785/tcrt.2012.500221. doi: 10.7785/tcrt.2012.500221
![]() |
[23] | S. K. Prabhakar, H. Rajaguru, Performance analysis of linear layer neural networks for oral cancer classification, in 2017 6th ICT International Student Project Conference (ICT-ISPC), IEEE, (2017), 1–4. doi: 10.1109/ICT-ISPC.2017.8075357. |
[24] |
T. Rahman, L. Mahanta, C. Chakraborty, A. Das, J. Sarma, Textural pattern classification for oral squamous cell carcinoma, J. Microsc., 269 (2018), 85–93. doi: 10.1111/jmi.12611. doi: 10.1111/jmi.12611
![]() |
[25] | T. Y. Rahman, L. B. Mahanta, A. K. Das, J. D. Sarma, Automated oral squamous cell carcinoma identification using shape, texture and color features of whole image strips, Tissue Cell, 63 (2020), 101322. |
[26] |
P. G. Betta, A. Andrion, A. Donna, F. Mollo, M. Scelsi, G. Zai, C. Magnani, Malignant mesothelioma of the pleura. The reproducibility of the immunohistological diagnosis, Pathol. Res. Pract., 193 (1997), 759–765. doi: 10.1016/S03440338(97)80054-4. doi: 10.1016/S03440338(97)80054-4
![]() |
[27] |
L. Shi, W. Liu, H. Zhang, Y. Xie, D. Wang, A survey of GPU-based medical image computing techniques, Quant. Imaging Med. Surg., 2 (2012), 188. doi: 10.3978/j.issn.2223-4292.2012.08.02. doi: 10.3978/j.issn.2223-4292.2012.08.02
![]() |
[28] |
C. L. Srinidhi, O. Ciga, A. L. Martel, Deep neural network models for computational histopathology: A survey, Med. Image Anal., 67 (2021), 101813. doi: 10.1016/j.media.2020.101813. doi: 10.1016/j.media.2020.101813
![]() |
[29] | S. Panigrahi, T. Swarnkar, Automated Classification of Oral Cancer Histopathology images using Convolutional Neural Network, in 2019 IEEE International Conference on Bioinformatics and Biomedicine (BIBM), (2019), 1232–1234. doi: 10.1109/BIBM47256.2019.8982979. |
[30] | S. Panigrahi, J. Das, T. Swarnkar, Capsule network based analysis of histopathological images of oral squamous cell carcinoma, J. King Saud Univ.-Comput. Inf. Sci., (2020), In press. doi: 10.1016/j.jksuci.2020.11.003. |
[31] |
O. Driemel, M. Kunkel, M. Hullmann, F. V. Eggeling, U. Müller‐Richter, H. Kosmehl, et al., Diagnosis of oral squamous cell carcinoma and its precursor lesions, JDDG: J. Dtsch. Dermatol. Ges., 5 (2007), 1095–1100. doi: 10.1111/j.1610-0387.2007.06397.x. doi: 10.1111/j.1610-0387.2007.06397.x
![]() |
[32] |
D. Navarun, E. Hussain, L. B. Mahanta, Automated classification of cells into multiple classes in epithelial tissue of oral squamous cell carcinoma using transfer learning and convolutional neural network, Neural Netw., 128 (2020), 47–60. doi: 10.1016/j.neunet.2020.05.003. doi: 10.1016/j.neunet.2020.05.003
![]() |
[33] |
L. H. Shehab, O. M. Fahmy, S. M. Gasser, M.S. El-Mhallawy, et al., An efficient brain tumor image segmentation based on deep residual networks (ResNets), J. King Saud Univ. Eng. Sci., 33 (2021), 404–412. doi: 10.1016/j.jksues.2020.06.001. doi: 10.1016/j.jksues.2020.06.001
![]() |
[34] |
H. Zhu, M. Ma, W. Ma, L. Jiao, S. Hong, J. Shen, et al., A spatial-channel progressive fusion ResNet for remote sensing classification, Inf. Fusion, 70 (2021), 72–87. doi: 10.1016/j.inffus.2020.12.008. doi: 10.1016/j.inffus.2020.12.008
![]() |
[35] | P. A. Pattanaik, M. Mohit, Z. K. Mohammad, S. N. Panda. Malaria detection using deep residual networks with mobile microscopy, J. King Saud Univ.-Comput. Inf. Sci., (2020), In press. doi: 10.1016/j.jksuci.2020.07.003. |
[36] |
L. Mohamed, M. Gunasekaran, H. N. T. Mohamed, M. N. K. Eldeen, Fighting against COVID-19: A novel deep learning model based on YOLO-v2 with ResNet-50 for medical face mask detection, Sustain. Cities Soc., 65 (2021), 102600. doi: 10.1016/j.scs.2020.102600. doi: 10.1016/j.scs.2020.102600
![]() |
[37] |
J. Duan, T. Shi, H. Zhou, J. Xuan, S. Wang, A novel ResNet-based model structure and its applications in machine health monitoring, J. Vib. Control, 27 (2021), 1036–1050. doi: 10.1177/1077546320936506. doi: 10.1177/1077546320936506
![]() |
[38] | X. Zhang, X. Zhou, M. Lin, J. Sun, Shufflenet: An extremely efficient convolutional neural network for mobile devices, in Proceedings of the IEEE conference on computer vision and pattern recognition, (2018), 6848–6856. doi: 10.1109/CVPR.2018.00716. |
[39] | F. Y. Shih, Image processing and pattern recognition: fundamentals and techniques, John Wiley & Sons, (2010). |
[40] |
M. M. R. Krishnan, C. Chakraborty, R. R. Paul, A. K. Ray, Hybrid segmentation, characterization and classification of basal cell nuclei from histopathological images of normal oral mucosa and oral submucous fibrosis, Expert Syst. Appl., 39 (2012), 1062–1077. doi: 10.1016/j.eswa.2011.07.107. doi: 10.1016/j.eswa.2011.07.107
![]() |
[41] | L. Perez, J. Wang, The effectiveness of data augmentation in image classification using deep learning, (2017), arXiv preprint arXiv: 1712.04621. |
[42] | F. Chollet, Xception: Deep learning with depthwise separable convolutions, in Proceedings of the IEEE conference on computer vision and pattern recognition, (2017), 1251–1258. doi: 10.1109/CVPR.2017.195 |
[43] | K. He, X. Zhang, S. Ren, J. Sun, Deep residual learning for image recognition, in Proceedings of the IEEE conference on computer vision and pattern recognition, (2016). doi: 10.1109/CVPR.2016.90. |
[44] | V. Nair, G. E. Hinton, Rectified linear units improve restricted Boltzmann machines, in International Conference on Machine Learning (ICML), (2010), 807–814. |
[45] | W. Liu, Y. Wen, Z. Yu, M. Yang, Large-margin softmax loss for convolutional neural networks, in Proceedings of the 33rd International Conference on Machine Learning (2016), 507–516. |
[46] | I. Sutskever, J. Martens, G. Dahl, G. Hinton, On the importance of initialization and momentum in deep learning, in International Conference on Machine Learning, PMLR, 28 (2013), 1139–1147. |
[47] | D. P. Kingma, J. Ba, Adam: A method for stochastic optimization, (2014), arXiv preprint arXiv: 1412.6980. |
[48] | K. He, J. Sun, Convolutional neural networks at constrained time cost, in Proceedings of the IEEE conference on computer vision and pattern recognition, (2015), 5353–5360. doi: 10.1109/CVPR.2015.7299173. |
[49] |
T. Belvin, V. Kumar, S. Saini, Texture analysis based segmentation and classification of oral cancer lesions in color images using ANN, IEEE International Conference on Signal Processing, Computing and Control (ISPCC), (2013), 1–5. doi: 10.1109/ISPCC.2013.6663401. doi: 10.1109/ISPCC.2013.6663401
![]() |
[50] | K. Anuradha, K. Sankaranarayanan, Detection of Oral Tumors using Marker Controlled Segmentation, Int. J. Comp. Appl., 52 (2012), 15–18. |
[51] |
B. R. Nanditha, A. Geetha, H. S. Chandrashekar, M. S. Dinesh, S. Murali, An Ensemble Deep Neural Network Approach for Oral Cancer Screening, Int. J. Online Biomed. Eng., 17 (2021), 121–134. doi: 10.3991/ijoe.v17i02.19207. doi: 10.3991/ijoe.v17i02.19207
![]() |
1. | John Adeoye, Liuling Hui, Yu-Xiong Su, Data-centric artificial intelligence in oncology: a systematic review assessing data quality in machine learning models for head and neck cancer, 2023, 10, 2196-1115, 10.1186/s40537-023-00703-w | |
2. | John Adeoye, Abdulwarith Akinshipo, Mohamad Koohi-Moghadam, Peter Thomson, Yu-Xiong Su, Construction of machine learning-based models for cancer outcomes in low and lower-middle income countries: A scoping review, 2022, 12, 2234-943X, 10.3389/fonc.2022.976168 | |
3. | Dominika Petríková, Ivan Cimrák, Survey of Recent Deep Neural Networks with Strong Annotated Supervision in Histopathology, 2023, 11, 2079-3197, 81, 10.3390/computation11040081 | |
4. | Yutao Men, Zixian Zhao, Wei Chen, Hang Wu, Guang Zhang, Feng Luo, Ming Yu, Research on workflow recognition for liver rupture repair surgery, 2024, 21, 1551-0018, 1844, 10.3934/mbe.2024080 | |
5. | Vishnu Priya Veeraraghavan, Shikhar Daniel, Arun Kumar Dasari, Kaladhar Reddy Aileni, Chaitra patil, Santosh R. Patil, Harnessing artificial intelligence for predictive modelling in oral oncology: Opportunities, challenges, and clinical Perspectives, 2024, 11, 27729060, 100591, 10.1016/j.oor.2024.100591 | |
6. | Astrid Laurent-Bellue, Aymen Sadraoui, Laura Claude, Julien Calderaro, Katia Posseme, Eric Vibert, Daniel Cherqui, Olivier Rosmorduc, Maïté Lewin, Jean-Christophe Pesquet, Catherine Guettier, Deep Learning Classification and Quantification of Pejorative and Nonpejorative Architectures in Resected Hepatocellular Carcinoma from Digital Histopathologic Images, 2024, 194, 00029440, 1684, 10.1016/j.ajpath.2024.05.007 | |
7. | Prerna Kulkarni, Nidhi Sarwe, Abhishek Pingale, Yash Sarolkar, Rutuja Rajendra Patil, Gitanjali Shinde, Gagandeep Kaur, Exploring the efficacy of various CNN architectures in diagnosing oral cancer from squamous cell carcinoma, 2024, 13, 22150161, 103034, 10.1016/j.mex.2024.103034 | |
8. | Sanjeev B. Khanagar, Lubna Alkadi, Maryam A. Alghilan, Sara Kalagi, Mohammed Awawdeh, Lalitytha Kumar Bijai, Satish Vishwanathaiah, Ali Aldhebaib, Oinam Gokulchandra Singh, Application and Performance of Artificial Intelligence (AI) in Oral Cancer Diagnosis and Prediction Using Histopathological Images: A Systematic Review, 2023, 11, 2227-9059, 1612, 10.3390/biomedicines11061612 | |
9. | Swathi Prabhu, Keerthana Prasad, Thuong Hoang, Xuequan Lu, Sandhya I., Multi-organ squamous cell carcinoma classification using feature interpretation technique for explainability, 2024, 44, 02085216, 312, 10.1016/j.bbe.2024.03.001 | |
10. | Swathi Prabhu, Keerthana Prasad, Thuong Hoang, Xuequan Lu, MultiSCCHisto-Net-KD: A deep network for multi-organ explainable squamous cell carcinoma diagnosis with knowledge distillation, 2025, 185, 00104825, 109469, 10.1016/j.compbiomed.2024.109469 |
Sensitivity | Specificity | Accuracy | Precision | F-measure | |
Well-differentiated | 0.93 | 0.92 | 0.93 | 0.96 | 0.94 |
Moderately-differentiated | 0.94 | 0.93 | 0.93 | 0.98 | 0.95 |
Poorly-differentiated | 0.95 | 0.97 | 0.96 | 0.97 | 0.96 |
Methodology | Image Size | Features | Classification methods | Accuracy (%) |
Krishnan et al. [10] | Normal-90 OSFWD-42 OSFD-26 |
71 features of wavelet family(LBP, Gabor, BMC) | SVM | 88.38 |
Krishnan et al. [3] | Normal-90 OSFWD-42 OSFD-26 |
HOS, LBP, LTE |
Fuzzy | 95.7 |
Krishnan et al. [40] | Normal-341 OSF-429 | Morphological and textural features | SVM | 99.66 |
Belvin et al. [49] | 16 malignant images with 192 patches | Texture features and Run Length features | Back propagation-based ANN | 97.92 |
Anuradha. K. et al. [50] | Energy, entropy, Contrast, Correlation, Homogeneity |
SVM | 92.5 | |
DevKumar et al. [4] | High grade-15 Low grade-25 Healthy-2 |
Identification of various layers –epithelial, subepithelial, keratin region and keratin pearls | Random Forest | 96.88 |
Santisudha et al. [29] | Malignant-214 Benign-172 |
CNN | 96.77 | |
Santisudha et al. [30] | Malignant-414 Benign-372 |
Capsule network | 97.35 | |
Navarun et al. [32] | Benign-1656 WDSCC-2634 MDSCC-2110 PDSCC - 1921 |
CNN | 97.5 | |
Nanditha B R et al. [51] | Benign-63 Malignant-269 |
Ensemble model (ResNet50 and VGG16) | 96.2 | |
Proposed method | WDSCC-400 MDSCC-400 PDSCC-400 |
Residual Network | 97.59 |
Sensitivity | Specificity | Accuracy | Precision | F-measure | |
Well-differentiated | 0.93 | 0.92 | 0.93 | 0.96 | 0.94 |
Moderately-differentiated | 0.94 | 0.93 | 0.93 | 0.98 | 0.95 |
Poorly-differentiated | 0.95 | 0.97 | 0.96 | 0.97 | 0.96 |
Methodology | Image Size | Features | Classification methods | Accuracy (%) |
Krishnan et al. [10] | Normal-90 OSFWD-42 OSFD-26 |
71 features of wavelet family(LBP, Gabor, BMC) | SVM | 88.38 |
Krishnan et al. [3] | Normal-90 OSFWD-42 OSFD-26 |
HOS, LBP, LTE |
Fuzzy | 95.7 |
Krishnan et al. [40] | Normal-341 OSF-429 | Morphological and textural features | SVM | 99.66 |
Belvin et al. [49] | 16 malignant images with 192 patches | Texture features and Run Length features | Back propagation-based ANN | 97.92 |
Anuradha. K. et al. [50] | Energy, entropy, Contrast, Correlation, Homogeneity |
SVM | 92.5 | |
DevKumar et al. [4] | High grade-15 Low grade-25 Healthy-2 |
Identification of various layers –epithelial, subepithelial, keratin region and keratin pearls | Random Forest | 96.88 |
Santisudha et al. [29] | Malignant-214 Benign-172 |
CNN | 96.77 | |
Santisudha et al. [30] | Malignant-414 Benign-372 |
Capsule network | 97.35 | |
Navarun et al. [32] | Benign-1656 WDSCC-2634 MDSCC-2110 PDSCC - 1921 |
CNN | 97.5 | |
Nanditha B R et al. [51] | Benign-63 Malignant-269 |
Ensemble model (ResNet50 and VGG16) | 96.2 | |
Proposed method | WDSCC-400 MDSCC-400 PDSCC-400 |
Residual Network | 97.59 |