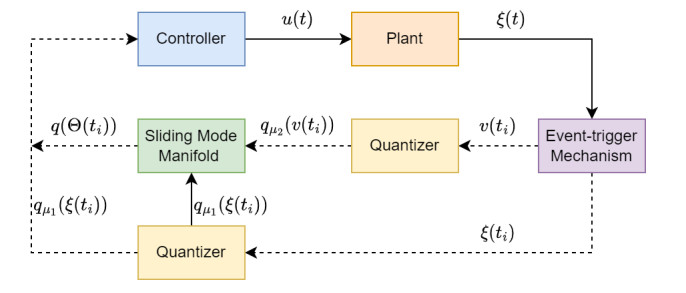
Network operation and maintenance (O & M) activities of data centers focus mainly on checking the operating states of devices. O & M engineers determine how services are running and the bearing capacity of a data center by checking the operating states of devices. However, this method cannot reflect the real transmission status of business data; therefore, engineers cannot fully comprehensively perceive the overall running conditions of businesses. In this paper, ERSPAN (Encapsulated Remote Switch Port Analyzer) technology is applied to deliver stream matching rules in the forwarding path of TCP packets and mirror the TCP packets into the network O & M AI collector, which is used to conduct an in-depth analysis on the TCP packets, collect traffic statistics, recapture the forwarding path, carry out delayed computing, and identify applications. This enables O & M engineers to comprehensively perceive the service bearing status in a data center, and form a tightly coupled correlation model between networks and services through end-to-end visualized modeling, providing comprehensive technical support for data center optimization and early warning of network risks.
Citation: Jiyuan Ren, Yunhou Zhang, Zhe Wang, Yang Song. Artificial intelligence-based network traffic analysis and automatic optimization technology[J]. Mathematical Biosciences and Engineering, 2022, 19(2): 1775-1785. doi: 10.3934/mbe.2022083
[1] | Jiao Wu, Shi Qiu, Ming Liu, Huayi Li, Yuan Liu . Finite-time velocity-free relative position coordinated control of spacecraft formation with dynamic event triggered transmission. Mathematical Biosciences and Engineering, 2022, 19(7): 6883-6906. doi: 10.3934/mbe.2022324 |
[2] | Wenjing Wang, Jingjing Dong, Dong Xu, Zhilian Yan, Jianping Zhou . Synchronization control of time-delay neural networks via event-triggered non-fragile cost-guaranteed control. Mathematical Biosciences and Engineering, 2023, 20(1): 52-75. doi: 10.3934/mbe.2023004 |
[3] | Jian Meng, Bin Zhang, Tengda Wei, Xinyi He, Xiaodi Li . Robust finite-time stability of nonlinear systems involving hybrid impulses with application to sliding-mode control. Mathematical Biosciences and Engineering, 2023, 20(2): 4198-4218. doi: 10.3934/mbe.2023196 |
[4] | Rui Ma, Jinjin Han, Li Ding . Finite-time trajectory tracking control of quadrotor UAV via adaptive RBF neural network with lumped uncertainties. Mathematical Biosciences and Engineering, 2023, 20(2): 1841-1855. doi: 10.3934/mbe.2023084 |
[5] | Yun Liu, Yuhong Huo . Predefined-time sliding mode control of chaotic systems based on disturbance observer. Mathematical Biosciences and Engineering, 2024, 21(4): 5032-5046. doi: 10.3934/mbe.2024222 |
[6] | Mingxia Gu, Zhiyong Yu, Haijun Jiang, Da Huang . Distributed consensus of discrete time-varying linear multi-agent systems with event-triggered intermittent control. Mathematical Biosciences and Engineering, 2024, 21(1): 415-443. doi: 10.3934/mbe.2024019 |
[7] | Guodong Zhao, Haitao Li, Ting Hou . Survey of semi-tensor product method in robustness analysis on finite systems. Mathematical Biosciences and Engineering, 2023, 20(6): 11464-11481. doi: 10.3934/mbe.2023508 |
[8] | Li Ma, Chang Cheng, Jianfeng Guo, Binhua Shi, Shihong Ding, Keqi Mei . Direct yaw-moment control of electric vehicles based on adaptive sliding mode. Mathematical Biosciences and Engineering, 2023, 20(7): 13334-13355. doi: 10.3934/mbe.2023594 |
[9] | Dong Xu, Xinling Li, Weipeng Tai, Jianping Zhou . Event-triggered stabilization for networked control systems under random occurring deception attacks. Mathematical Biosciences and Engineering, 2023, 20(1): 859-878. doi: 10.3934/mbe.2023039 |
[10] | Xiaoxiao Dong, Huan Qiao, Quanmin Zhu, Yufeng Yao . Event-triggered tracking control for switched nonlinear systems. Mathematical Biosciences and Engineering, 2023, 20(8): 14046-14060. doi: 10.3934/mbe.2023627 |
Network operation and maintenance (O & M) activities of data centers focus mainly on checking the operating states of devices. O & M engineers determine how services are running and the bearing capacity of a data center by checking the operating states of devices. However, this method cannot reflect the real transmission status of business data; therefore, engineers cannot fully comprehensively perceive the overall running conditions of businesses. In this paper, ERSPAN (Encapsulated Remote Switch Port Analyzer) technology is applied to deliver stream matching rules in the forwarding path of TCP packets and mirror the TCP packets into the network O & M AI collector, which is used to conduct an in-depth analysis on the TCP packets, collect traffic statistics, recapture the forwarding path, carry out delayed computing, and identify applications. This enables O & M engineers to comprehensively perceive the service bearing status in a data center, and form a tightly coupled correlation model between networks and services through end-to-end visualized modeling, providing comprehensive technical support for data center optimization and early warning of network risks.
With the development and use of network and wireless communications technologies, networked control systems (NCS) have gradually attracted attention for applications such as power networks, remote surgery, telephone networks, industrial and manufacturing systems, and new energy vehicles. It has some advantages such as convenience and efficiency in comparison to the conventional systems. In wireless network systems, signals can be exchanged through digital communication networks, which also presents new challenges for control engineers such as data quantization, event-driven sampling, etc. [1,2,3,4].
In some practical applications, such as unmanned submarines, UAVs, etc., limited resources are required for NCS due to the limited power supply of the device itself. The traditional control method is to use time-triggered control, where the controller transmits control signals to the system in a periodic manner, and the system transmits the state signals to the controller in a periodic manner. This leads to waste of resources and reduces the running time of the device. Therefore, the ET mechanism has been introduced, which helps reduce the burden on the network, saves resources and improves the efficiency of device resource utilization compared with the traditional periodic transmission. The fundamental principle behind the ET control approach is that the related sensors and controllers are only updated when an ET error exceeds a predetermined threshold or condition, also referred to as an ET rule [5,6,7,8,9,10,11,12,13].
In NCS, a shared network is frequently required for data exchange on nodes distributed throughout space, however the network's constrained transmission capacity could result in network congestion phenomenon. Consequently, quantization is required, and a quantizer is a device that maps a transmitted signal to a subset set and takes segmented constants. By quantifying the triggered state of the system instead of the system state itself, the computational load on the system transmission is significantly reduced [14,15].
The presence of unmatched uncertainty may affect the stability of linear system when the control signal transmissions are made over a network channel. SMC is an effective control method in robust nonlinear controllers [16,17,18]. It has a wide range of applications in theory and practical life owing to its advantages of being insensitive to internal uncertainties and extrinsic disturbances, meeting the matched condition, and being easy to operate. The classical SMC design usually consists of two stages: the reaching stage and the sliding stage. In the reaching stage, the system state trajectory moves toward the SM manifold, while in the sliding stage, the system state trajectory slides along the SM manifold to the origin. When dealing with unmatched uncertainties, the SMC can be used with classical control design methods such as LMI method, adaptive method, etc., or the ISMC can be applied. The ISMC approach is to design a high-frequency switching gain to force the system state to reach the ISM manifold, and in the presence of unmatched uncertainty, the integral action of the ISM manifold continues to drive the system state to the equilibrium point [19].
There has been a lot of literature on ET control combined with SMC. In [20,21], the ET linear SMC is discussed and the practical SM band is introduced; Using a dynamic time-varying trigger threshold, ET ISMC of linear systems with perturbations is considered in [22]; And in [23], the ET ISMC for T-S fuzzy systems in the presence of uncertainty is considered, and novel triggering conditions are proposed. In [24], a class of networked linear systems is considered and a control input-based ET SMC control is proposed, where the ET rule is dependent on the changes of the input values. A unified class of ET SMC framework is proposed in [25], which can achieve finite-time reachability at the SM manifold and can enable finite-time stabilization of the system state. In [26], the delayed memristive neural network synchronization problem is investigated, and continuous-time sampling ET SMC is proposed, and based on this, periodic-time sampling ET SMC is proposed.
The quantization control can also be combined with the SMC. In [27], a class of linear uncertain systems with quantized feedback stability problem is studied by SMC. The influence of matched and unmatched uncertainties can be practically eliminated by the quantization parameter adjustment strategies. In [28], the problem of distributed quantization of SMC in complex networks is investigated, and the ISM surface is quantized using an adaptive filter. In [29], the problem of synchronization of discrete-time recurrent neural networks with time-varying delay is investigated, and the stability analysis is carried out using uniform quantizer and logarithmic quantizer, respectively.
In the general, the ET SMC and the quantized SMC are two different types of robust control methods. However, in recent years, the combination of the ET control, the quantized control, and the SMC has gradually gained attention. In [30], an ET approach was used to study the robust sedimentation problem of quantized feedback SMC for a class of uncertain linear systems, quantizing the ET states rather than the states themselves, using linear SM manifold. In [21], a linear SM manifold is also used, but the system states are first quantized and then an ET mechanism is added to the quantized states. In [31], the quantization-based ET SM tracking control problem for mechanical systems is investigated, and the ET rule uses the disparity between the quantized incremental triggering state and the quantized incremental state. Using a dynamic uniform quantizer, the ET SMC problem for a type of continuous-time system with actuator faults and output feedback signal quantization is investigated in [32]. In [33], the problem of robust sedimentation of perturbed linear time-invariant systems based on ET SMC is studied. A logarithmic quantizer is used to quantify the state and the triggering state.
The aforementioned literature considers, only under matched uncertainty, the combination of linear SMC, ET mechanism, and quantization mechanism, and this paper considers, in the existence of unmatched uncertainty, the combination of ET control, ISMC, and quantization control. The main challenges faced are as follows. 1) How to quantify the ET ISM manifold when the integral term is challenging to measure; 2) In the existence of unmatched uncertainty, how the system state guarantee a finite time to reach the practical SM band.
Based on [34], a matched and unmatched uncertain linear time-invariant system is considered, and the main objective is to design quantization-based ET ISMC to handle matched and unmatched uncertainties and perturbations. The main contributions of this paper are summarized as follows:
1) In this paper, the combination of quantization control, ET control, and ISMC is simultaneously considered. The quantized ET ISMC algorithm based on the convergence law subject to matched and mismatched uncertainties and perturbations is proposed to achieve finite time arrival of the linear system state at the SM surface and to realize asymptotic stability of the linear system state.
2) This paper proposes a quantization method for the ISM surface. The system state and the integral term are quantized separately for the ISM surface, and the virtual variable is introduced to replace the integral term, and two static uniform quantizers are used to quantize the system state and the virtual variable separately.
3) A positive lower bound on the inter-event time is derived to avoid Zeno behavior of the system.
The rest of the paper is organized as follows: Section 2 contains the preliminary and problem statements, including the construction of the ISM surface and the signal quantization approaches. In Section 3, the quantized ET ISMC is presented. And in Section 4, it is proved that the finite time to reach the ISM surface and that the system will not occur Zeno phenomenon during the operation of the system. Thus, simulation results of a numerical example and a mass-spring damping system are given in Section 5. In Section 6, this paper is concluded.
Notations: The sets of real numbers and integers are indicated by R and Z respectively. The set of positive real numbers and the set of positive integers are R>0 and Z>0. Rn denotes the n-dimensional Euclidean space in the real numbers. ∥⋅∥ denotes the matrix norm, and ∣⋅∣ denotes the absolute value.
Consider an uncertain linear NCS of the following form
˙ξ(t)=Aξ(t)+(b+Δb)u(t)+(b+Δb)fm(t,ξ)+pun(t,ξ), | (2.1) |
where ξ(t)∈Rn is the system state, u(t)∈R indicates the control input, and ξ(0)=ξ0 is assumed to be the initial value of the state ξ(t). Thus, the matrice A∈Rn×n is the system matrix and b∈Rn represents the input matrix. The fm(t,ξ) denotes the matched perturbation caused by external disturbance, and pun(t,ξ) denotes unmatched uncertainties. The modeling error Δb is unknown and has the same dimension as b. Unmatched uncertainties are caused by modeling errors, inaccurate measurements, etc. Further, we can obtain the rewritten form of system (2.1) as follows
˙ξ(t)=Aξ(t)+bu(t)+bfm(t,ξ)+fu(t,ξ), | (2.2) |
where fu(t,ξ)=Δb[u(t)+fm(t,ξ)]+pun(t,ξ). Suppose that the nominal system ˙ξ(t)=Aξ(t)+bu(t) is controllable.
In this paper, the following assumption is required in the rest of the paper.
Assumption 1. Suppose that the matching and mismatching uncertainty fm(t,ξ) and fu(t,ξ) have known upper bounds, i.e., supt≥0|fm(t,ξ)|≤Fm, and supt≥0‖fu(t,ξ)‖≤Fu, where Fm and Fu are known positive constants.
Remark. 1 The above assumption are necessary for the proof of finite-time reachability of the SM surface and minimum inter-event time.
Here related lemma and definition are introduced.
Lemma 1. [35]Consider linear non-autonomous system ˙ξ=f(t,ξ), f(0)=0. Assume that there exists a continuous positive definite function V(x):Rn→R satisfying the following equation:
˙V(ξ)≤−η1Vκ(ξ)−η2V(ξ) | (2.3) |
where η1>0, η2>0, and κ∈(0,1). Then the system is finite time stable. Furthermore, the convergence time satisfies
tf≤1η2(1−κ)ln[η2V1−κ(ξ(0))+η1η1]. | (2.4) |
In order to construct the ISM surface represented by Θ, the concepts of ideal and practical SM are presented as in [36].
Definition 1. (Ideal SM) There exists t1∈[t0,∞) such that the SM trajectory is Θ=0 in the case of t≥t1, then it is called the ideal SM.
Definition 2. (Practical SM) There exists t1∈[t0,∞) such that the SM trajectory stays in the vicinity of the SM surface with the bound given by ε in the case of t≥t1, then it is called the practical SM. Here, ε is referred to as practical SM band.
At the beginning, the ISM manifold is modeled as
Θ(t)=Gξ(t)−Gξ(0)−G∫t0(Aξ(τ)+bu0(τ))dτ, | (2.5) |
where G represents the projection matrix, and G is usually taken to be bT in a way that makes det(Gb)≠0. The ISM surface has no reaching stage, the system state is on the ISM surface at the beginning, which greatly reduces the chattering phenomenon.
In (2.2), the matched and unmatched uncertainties are assumed to be 0, and the control input of the nominal system is u0, the equation of the state trajectory of the ideal system is
˙ξ(t)=Aξ(t)+bu0(t). | (2.6) |
Next, the nominal control u0(t) is designed so that the nominal system (2.6) is stable and SM motion occurs. The control law is now designed as
u(t)=u0(t)+u1(t), | (2.7) |
where u1(t) is the discontinuous control employed to counteract the uncertainty impacting the system. The control input u0(t) is intended to have state feedback controller in the following forms:
u0(t)=−Kξ(t), | (2.8) |
where K∈R1×n is the state feedback gain vector. Further, we choose the value of K such that (A−bK) is Hurwitz.
Taking the derivative of Eq (2.5) along system (2.2) and using (2.8), the result thus derive as
˙Θ(t)=G˙ξ(t)−G(A−bK)ξ(t). | (2.9) |
Replacing (2.2) into (2.9), and the result derived is
˙Θ(t)=G(Aξ(t)+bu(t)+bfm(t,ξ)+fu(t,ξ))−G(A−bK)ξ(t)=Gbu(t)+Gbfm(t,ξ)+Gfu(t,ξ)+GbKξ(t). | (2.10) |
In [37], Gao has presented the constant plus proportional rate reaching law for continuous time systems. By considering the matching disturbances, the reaching law is reformulated as
˙Θ(t)=−Q1Θ(t)−Qsgn(Θ(t))+Gbfm(t,ξ)+Gfu(t,ξ), | (2.11) |
where Q1 and Q will be mentioned below, equating relations (2.9) and (2.11) to solve for u(t), then the ISMC result is obtained as
u(t)=−(Gb)−1(GbKξ(t)+Q1Θ(t)+Qsgn(Θ(t))). | (2.12) |
The resulting control law (2.12) guarantees a finite time for the system (2.2) to reach the SM surface, and it forces the SM motion.
In this paper, SMC is investigated by ET control and quantization control, and the corresponding system block diagram is presented in Figure 1.
As can be seen from Figure 1, ξ(ti) and v(ti) are generated by the ET mechanism and transmitted to different quantizers through the network channels, respectively, and the resulting qμ1(ξ(ti)) and qμ2(v(ti)) are used to generate the quantized SM surface.
A quantizer is a piecewise constant function q:Rr→D, where D is a finite subset of the vector space Rr. Assuming that z is the variable to be quantized, the quantizer can be seen as a device that converts a real-valued signal into a segmented constant-valued signal. Mathematically, it can be framed as a function round(⋅) that takes the nearest integer, i.e.,
qμ(z)=μ⋅round(zμ), | (2.13) |
where the quantizer parameter μ>0 is defined as the quantization sensitivity and qμ(⋅) is a uniform quantizer with its level μ. Denote the quantization error as
eμ=qμ(z)−z. | (2.14) |
Therefore, it can be obtained as
|eμ|=|qμ(z)−z|≤Δμ, | (2.15) |
where Δ=√p2, p is the dimensions of the vector z.
Defining Ti as the inter-event time, and Ti=ti+1−ti. Denoting the ET error as
e(t)=GbKξ(ti)+Q1Θ(ti)−GbKξ(t)−Q1Θ(t). | (2.16) |
Setting the ET threshold, and furthermore, considering the positive constants α∈(0,∞) and σ∈(0,1], it follows that
||e(t)||≤σα | (2.17) |
which is valid for all t>0. Let the next ET moment be ti+1. Therefore, the next ET moment ti+1 is
ti+1=inf[t>ti:||e(t)||≥σα]. | (2.18) |
In a NCS, the transmission signal uses an ET protocol in which the ET control law is only updated at the moment of sampling. In the controller, a zero-order keeper (ZOH) is employed in the ET controller to maintain the feedback information between any two triggering moments. Then, it is represented by
u(t)=u(ti), | (2.19) |
for t∈[ti,ti+1) and i∈Z≥0, where ti,ti+1∈T, T is the set of triggering moments, ξ(ti) is the value of the state ξ(t) at the triggering moment. The value of the SM surface Θ(t) at the triggering moment is
Θ(ti)=Gξ(ti)−Gx(0)−G∫ti0(A−bK)ξ(τ)dτ. | (2.20) |
Then, consider quantization of the triggering state ξ(ti)
qμ1(ξ(ti))=μ1⋅round(ξ(ti)μ1), | (2.21) |
where qμ1(ξ(ti)) is the quantized value of the triggering state ξ(ti). Then the corresponding quantization error is
|qμ1(ξ(ti))−ξ(ti)|≤Δ1μ1. | (2.22) |
where p1 is the dimensions of the vector ξ(ti), Δ1=√p12, μ1>0 is the quantization sensitivity.
To quantize the SM surface, the virtual variable v(t) is introduced as
v(t)=∫t0(A−bK)ξ(τ)dτ. | (2.23) |
Then the value of v(ti) at the triggering instant is
v(ti)=∫ti0(A−bK)ξ(τ)dτ. | (2.24) |
Consider quantization of the virtual variable v(ti) is
qμ2(v(ti))=μ2⋅round(v(ti)μ2),μ2>0, | (2.25) |
where qμ2(v(ti))is the quantized value of the triggering state v(ti).
Therefore, the corresponding quantization error is
|qμ2(v(ti))−v(ti)|≤Δ2μ2, | (2.26) |
where p2 is the dimensions of the vector v(ti), Δ2=√p22, μ2>0 is the quantization sensitivity. The quantized triggering SM surface q(Θ(ti)) is
q(Θ(ti))=Gqμ1(ξ(ti))−Gξ(0)−Gqμ2(v(ti)). | (2.27) |
In the ET control law (2.19), the quantized ET control law (3.1) can be obtained by replacing the ET state ξ(ti) with the quantized ET state qμ1(ξ(ti)). Therefore, for the quantized ET implementation of the ISM, the control law (2.12) can be written as
u(t)=−(Gb)−1(GbKqμ1(ξ(ti))+Q1q(Θ(ti))+Qsgn(q(Θ(ti)))) | (3.1) |
for all t∈[ti,ti+1), and i∈Z≥0.
The ET rule is designed so that the quantized ET control law (3.1) is updated at every ti only when the ET error exceeds some pre-defined threshold value. qμ1(ξ(ti)) is the ET state ξ(ti) obtained after quantization.
Remark. 2 During the sliding motion Θ(t)=˙Θ(t)=0, based on the Eq (2.9), it can be derived that the system state ξ(t) is bounded and can be represented as ‖ξ(t)‖=‖exp((A−bK)t)ξ0(t)‖. Further, we can choose K such that A−bK becomes Hurwitz matrix, so that the norm of the system state ξ(t) is bounded, i.e., ‖ξ(t)‖≤δexp(−βt), where δ and β are positive constants. If the last event of state ξ(t) happened at the moment ti, then
‖ξ(t)‖≤‖ξ(ti)‖, for all t∈[ti,ti+1), | (3.2) |
i.e.
‖ξ(ti)‖≤δexp(−βti). | (3.3) |
From (2.20), (2.22), (2.26), (2.27), it is obtained that
|Θ(ti)−q(Θ(ti))|=|Gξ(ti)−Gξ(0)−Gv(ti)−Gqμ1(ξ(ti))+Gξ(0)+Gqμ2(v(ti))=|Gξ(ti)−Gqμ1(ξ(ti))+Gqμ2(v(ti))−Gv(ti)|≤‖G‖Δ1μ1+‖G‖Δ2μ2, | (3.4) |
we choose
M=‖G‖Δ1μ1+‖G‖Δ2μ2, | (3.5) |
then we have
|Θ(ti)−q(Θ(ti))|≤M. | (3.6) |
Remark. 3 While the system state trajectory reaches the SM surface, if the control law is not updated, the system state trajectory will cross the SM surface and move away from it. The ET error starts to increase, and when the ET error exceeds the upper threshold, the signal is updated to redirect the system trajectory towards the SM surface. This process keeps going until the system state trajectory reaches its origin. From (2.16), it is known that
|Q1Θ(t)−Q1Θ(ti)|=GbKξ(ti)−GbKξ(t)−e(t). | (3.7) |
The sliding trajectory's greatest deviation is
|Θ(t)−Θ(ti)|≤|σαQ1|+|GbKQ1|‖ξ(t)−ξ(ti)‖. | (3.8) |
The quantization error of SM function Θ(t) can be obtained by (3.4) and (3.8)
|Θ(t)−q(Θ(ti))|=|Θ(t)−Θ(ti)+Θ(ti)−q(Θ(ti))|≤|σαQ1|+|GbKQ1|‖ξ(t)−ξ(ti)‖+‖G‖Δ1μ1+‖G‖Δ2μ2. | (3.9) |
It can be derived that
P=|σαQ1|+|GbKQ1|δexp(−βti)+M. | (3.10) |
The range of sgn(Θ(t))≠sgn(q(Θ(ti))) is
Ω={Θ(t):|Θ(t)−q(Θ(ti))|<P}. | (3.11) |
Theorem 1. Considering the system (2.2) in addition to the ISM manifold (2.5). Under the quantized ET control law (3.1), the system state trajectory can reach the SM surface in finite time even in the presence of unmatched perturbations, if there exist Q satisfying
Q≥|Gb|Fm+‖G‖Fu+|Gb||K|Δ1μ1+Q1M+σα+η, | (4.1) |
where η is a positive scalar, and the practical SM is implemented in the vicinity of Θ(t)=0 within a band
Bξ={ξ(t)∈Rn:|Θ(t)|≤|σαQ1|+|GbKQ1|‖ξ(t)−ξ(ti)‖}. | (4.2) |
Proof. Outside of the region Ω, then it will satisfy sgn(Θ(t))=sgn(q(Θ(ti))). Choose the Lyapunov candidate as V=12Θ2. Differentiating V along the system (2.2) and using the derivative of the ISM surface in (2.9), then, we have
˙V(Θ(t))=Θ(t)˙Θ(t)=Θ(t)[G˙ξ(t)−G(A−bK)ξ(t)]=Θ(t)[G(Aξ(t)+bu+bfm(t,ξ)+fu(t,ξ))−G(A−bK)ξ(t)]=Θ(t)[Gbu(t)+Gbfm(t,ξ)+Gfu(t,ξ)+GbKξ(t)]. | (4.3) |
Substituting the designed control law in (3.1), it can be concluded that
˙V(Θ(t))=Θ(t)[−GbKqμ1(ξ(ti))−Q1q(Θ(ti))−Qsgn(q(Θ(ti)))+Gbfm(t,ξ)+Gfu(t,ξ)+GbKξ(t)]=−Θ(t)[GbKqμ1(ξ(ti))+GbKξ(ti)−GbKξ(ti)−GbKξ(t)−Q1Θ(t)+Q1Θ(ti)−Q1Θ(ti)+Q1Θ(t)+Q1q(Θ(ti))−Gbfm(t,ξ)−Gfu(t,ξ)+Qsgn(q(Θ(ti)))]. | (4.4) |
From (2.16), we can obtain
˙V(Θ(t))=−Θ(t)[GbKqμ1(ξ(ti))−GbKξ(ti)+e(t)+Q1Θ(t)+Q1q(Θ(ti))−Q1Θ(ti)−Gbfm(t,ξ)−Gfu(t,ξ)+Qsgn(q(Θ(ti)))]. | (4.5) |
Take (2.22), (2.17), and (3.6) into (4.5), one can further get that
˙V(Θ(t))≤−Θ(t)[GbKΔ1μ1+e(t)+Q1Θ(t)+Q1M+Qsgn(q(Θ(ti)))−Gbfm(t,ξ)−Gfu(t,ξ)]. | (4.6) |
Take the norm and sort it out,
˙V(Θ(t))≤|Θ(t)|‖e(t)‖+|Θ(t)||Gb|‖K‖Δ1μ1+|Θ(t)|Q1M+|Θ(t)||Gb|fm(t,ξ)+|Θ(t)|‖G‖fu(t,ξ)−Q1Θ2(t)−|Θ(t)|Q. | (4.7) |
It yields from Assumption 1 that
˙V(Θ(t))≤−|Θ(t)|(Q−|Gb|‖K‖Δ1μ1−Q1M−σα−|Gb|Fm−‖G‖Fu)−Q1|Θ(t)|2. | (4.8) |
Substituting (4.1) into (4.8), one gets
˙V(Θ(t))≤−|Θ(t)|η−Q1|Θ(t)|2. | (4.9) |
Let η1=√2η and η2=2Q1, then we obtain
˙V(Θ(t))≤η1V12(t)−η2V(t). | (4.10) |
Combining with Lemma 1, we have
tf≤2η2ln[η2V12(0)+η1η1]. | (4.11) |
When the system state trajectory has reached the SM manifold, the system state is asymptotically stable within the practical SM band. Thus, this proof of Theorem 1 is completed.
Remark. 4 From (3.8), set Θ(ti)=0, and then, the maximum SM band can be derived. The maximum band is given by (4.2).
Next, to guarantee that the system is Zeno-free, it must be demonstrated that the lower bound on the inter-event time must be greater than a positive constant. The following proof shows that there exists the lower bound on the inter-event time.
Theorem 2. Considering the system (2.2) in addition to the quantized ET ISMC in (3.1), if the inter-event execution time is generated from (2.18), the lower bound of the inter-event time indicates that
Ti>σαk1δ+k2+k3+k4, | (4.12) |
where
k1=(|Gb|‖K‖+Q1‖G‖)‖A‖+(Q1‖GA‖+Q1|Gb|‖K‖),
k2=(|Gb|‖K‖+Q1‖G‖)‖b‖×‖−(Gb)−1(GbKqμ1(ξ(ti))+Q1q(Θ(ti))+Qsgn(q(Θ(ti))))‖,
k3=(|Gb|‖K‖+Q1‖G‖)‖b‖Fm,
k4=(|Gb|‖K‖+Q1‖G‖)Fu.
Proof. Let Ti be the inter-event time, i.e. the time required for the event error to grow from zero to σα. Define the set Γ=(t:‖e(t)‖=0) where t∈[ti,ti+1). From (2.2) and (2.9), we have
‖ddte(t)‖=‖ddt(GbKξ(ti)+Q1Θ(ti)−GbKξ(t)−Q1Θ(t))‖≤‖GbK˙ξ(t)+Q1˙Θ(t)‖≤‖GbK˙ξ(t)‖+‖Q1(G˙ξ(t)−G(A−bK)ξ(t))‖≤(|Gb|‖K‖+Q1‖G‖)‖˙ξ(t)‖+(Q1‖GA‖+Q1|Gb|‖K‖)‖ξ(t)‖≤(|Gb|‖K‖+Q1‖G‖)‖Aξ(t)+bu(t)+bfm(t,ξ)+fu(t,ξ)‖+(Q1‖GA‖+Q1|Gb|‖K‖)‖ξ(t)‖. | (4.13) |
It yields from Assumption 1 that
‖ddte(t)‖≤[(|Gb|‖K‖+Q1‖G‖)‖A‖+(Q1‖GA‖+Q1|Gb|‖K‖)]‖ξ(t)‖+(|Gb|‖K‖+Q1‖G‖)‖b‖‖u(t)‖+(|Gb|‖K‖+Q1‖G‖)‖b‖Fm+(|Gb|‖K‖+Q1‖G‖)Fu. | (4.14) |
Using the designed control law in (3.1) and (3.3), thus we obtain
‖ddte(t)‖≤[(|Gb|‖K‖+Q1‖G‖)‖A‖+(Q1‖GA‖+Q1|Gb|‖K‖)]δexp(−βti)+(|Gb|‖K‖+Q1‖G‖)‖b‖×‖−(Gb)−1(GbKqμ1(ξ(ti))+Q1q(Θ(ti))+Qsgn(q(Θ(ti))))‖+(|Gb|‖K‖+Q1‖G‖)‖b‖Fm+(|Gb|‖K‖+Q1‖G‖)Fu. | (4.15) |
Noticing that e(ti)=0, one can get that
‖e(t)‖≤∫tti(k1δe−βt+k2+k3+k4)dt. | (4.16) |
Thus it yields that
‖e(t)‖≤(k1δ+k2+k3+k4)(t−ti). | (4.17) |
The next event will occur only when the norm of the error exceeds a predefined threshold. Therefore, Ti is expressed by (4.12), and that is the conclusion of the demonstration of the theorem.
This section shows the effectiveness of the control strategy in two instances. The first example is a numerical model, and the second example employs a mass-spring damper system.
Example 1
This numerical example is to demonstrate the proposed perturbed continuous-time linear system under the quantized ET ISMC, and the system parameters are described as follows
A=[101513−20−101701515],b=[0−35], |
fm(t,ξ)=0.3sin(2∗π∗t),fu(t,ξ)=[0.5sin(2πt)00.5sin(2πt)]. |
The initial state of the linear system is designed to be ξ0=[−1,1,1]T, the projection matrix G is set as G=bT=[0,−3,5]. It was calculated that K=[165.26,52.9310,82.3188], other design parameters are designed to be σ=0.4, α=50, and the reaching gain Q1=4. By calculation, the switching gain Q is given as Q=291. The quantization sensitivity μ1 and μ2 are both taken as 0.05.
Simulation results of the perturbed continuous-time linear system (2.2) with the designed quantized ET control law in (3.1) are presented in Figures 2–6. It can be noticed from Figures 2 and 4 that the system states can be well steered into the ISM surface in (2.5), then they finally slides to the origin. Figure 3 represents the proposed variation of the quantized ET ISMC. The evolution of ‖e(t)‖ under the quantized ET ISMC is presented in Figure 5. Further, it shows from Figure 6 that no Zeno execution of ET condition occurs during the whole system implementation.
For resource-constrained systems, the fewer number of control signals updates means the system has a longer lifespan and a more efficient utilization of resources. Based on this, this paper proposes a performance index for comparing the number of control signals updates. In Table 1, we present the comparison between the number of control updates obtained by the time-triggered control scheme, the ET control scheme and the quantized ET control scheme. Thus, as can be observed in Table 1, the number of control updates in the quantized ET control method is fewer than in the ET control method and much fewer than in the time-triggered control method, which has resulted in reduced control calculations and minimum use of resources without affecting the stability of the system.
Triggering mechanism | No. of control updates |
Time triggered control scheme in (2.12) (τ = 0.001) | 1001 |
ET control scheme in (2.19) | 314 |
Quantization ET control scheme in (3.1) | 208 |
Example 2
The second example considers a mass-spring damper system with the system configuration shown in Figure 7. u represents input control, the states ξ1 and ξ2 represent the position and speed of the car, respectively. M represents the mass of the cart, and k denotes the spring constant. The damping coefficient associated with the system is set as h. The perturbation term is chosen as f. The disturbance may be caused by the frictional forces acting on the mass-spring damper system. In practice, the spring constant k and the damping coefficient h shift their nominal values with time, and the actual velocity of the system is usually hard to acquire; therefore, system uncertainty D needs to be taken into account when performing system modeling. Let M=1kg, k=0.333N/m, h=1.1Ns/m, f=0.5sin(10t), D=0.1sin(10t).
The system equation of state is
˙ξ(t)=[01−0.333−1.1]ξ+[01](u+f)+[D0]. | (5.1) |
The initial state of the system is designed to be ξ0=[2.2,−1.7]T. Other parameters are designed as G=bT=[0,1], k=[25.1467,9.0], σ=0.4, α=5. Q1=4, Fm=0.5, Fu=0.1, Q=3.5. The quantization sensitivity μ1 and μ2 are both taken as 0.05.
Simulation results of the mass-spring damper system (5.1) with the designed quantized ET control law in (3.1) are presented in Figures 8–12. From Figure 8 and Figure 10, it can be drawn that the system state trajectory reaches the SM manifold in a finite time and is confined within the SM band. Figure 9 represents the proposed variation of the quantized ET ISMC. The evolution of ‖e(t)‖ under the quantized ET ISMC is presented in Figure 11. In addition, it is observed from Figure 12 that no Zeno phenomenon occurs in the system during the operation of the system. Taking the performance index of Table 1 for comparison, the number of control updates for all strategies, such as time-triggered control strategy, ET control strategy, and quantized ET control strategy, are calculated and listed in Table 2. As can be seen from Table 2, the ET control strategy with quantized state measurements has much lesser control updates than the ET control strategy and lesser than the time-triggered control strategy in terms of the minimum use of control computations and resources.
Triggering mechanism | No. of control updates |
Time triggered control scheme in (2.12)(τ =0.001) | 2001 |
ET control scheme in (2.19) | 42 |
Quantization ET control scheme in (3.1) | 25 |
The stability problem of networked linear systems with matched uncertainty and unmatched uncertainty under quantized ET ISMC and the Zeno phenomenon problem are studied in this paper. To begin with, the priori knowledge such as finite-time reachability and actual SM band is introduced. The ISM surface is constructed, and the convergence law is used to derive the control input. On the basis of this, the system introduces the ET mechanism, and under the ET mechanism, the system state and SM surface are quantized respectively, which leads to quantized ET ISMC. Then, it is demonstrated that the system trajectory can reach the SM surface in a finite time, and no Zeno phenomenon occurs during the system operation. Finally, a numerical example and an example of a mass-spring damper are given to illustrate the effectiveness of the proposed quantized ET ISMC. In future work, we will continue to study the combination of quantized control, ET control, and SMC, and explore their applications under other conditions, such as nonlinear systems, fault-tolerant control, fuzzy systems, etc.
The authors declare they have not used Artificial Intelligence (AI) tools in the creation of this article.
This work was supported by the Shandong Provincial Natural Science Foundation ZR2022MF324 and ZR2021MF003, and the Shandong University of Science and Technology Research Fund 2018 TDJH101.
We declare that we have no conflict of interest.
[1] | S. Smoot, Private cloud computing: Integration, virtualization and service-oriented infrastructure, Mechanical Industry Press, 2013. |
[2] | Q. Wang, TCP/IP protocol analysis, J. North. Jiaotong Univ., 1 (1995), 112–117. doi: CNKI:SUN:BFJT.0.1995-01-025. |
[3] | Z. Y. He, O. Li, B. W. Yang, Y. Liu, Formal description of TCP protocol based on timed Colored Petri Net, Comput. Eng., 37 (2011), 77–80. doi: CNKI:SUN:JSJC.0.2011-18-028. |
[4] | C. Yang, Y. Q. Gui, Network traffic analysis and control strategies, Comput. Knowl. Technol., 10 (2011), 26–28+62. doi: CNKI:SUN:BGDH.0.2011-10-012. |
[5] | H. L. Zhang, H. Wang, A sFlow-based network traffic analysis method, Comput. Eng. Sci., 8 (2007), 61–63+73. doi: CNKI:SUN:JSJK.0.2007-08-020. |
[6] | R. Lv, W. Dai, G. Cao, Research on multiple combinations of network traffic analysis methods, Small Microcomput. Syst., 31 (2010), 631–634. |
[7] | L. Chen, B. Dong, X. Wang, Design and implementation of a global network traffic analysis system based on SNMP protocol, J. Dalian Univ. Technol., 45 (2005), 69–72. |
[8] | M. Xia, Research and application of root cause analysis of abnormal behavior of ethernet networks, Liaoning Univ. Eng. Technol., 2019. |
[9] | G. Zhang, Design and Implementation of SNMP-based Performance Monitoring System, Ph.D thesis, Beijing Jiaotong University, 2008. |
[10] | X. Li, Design and Implementation of SNMP-based Network Device Performance Monitoring System, Ph.D thesis, Beijing University, 2009. |
[11] |
X. Zhang, J. You, A gated dilated causal convolution based encoder-decoder for network traffic forecasting, IEEE Access, 8 (2020), 6087–6097. doi: 10.1109/ACCESS.2019.2963449. doi: 10.1109/ACCESS.2019.2963449
![]() |
[12] | B. Pfülb, C. Hardegen, A. Gepperth, S. Rieger, A study of deep learning for network traffic data forecasting, in International Conference on Artificial Neural Networks, (2019), 497–512. doi: 10.1007/978-3-030-30490-4_40. |
[13] |
S. H. Mousavi, M. Khansari, R. Rahmani, A fully scalable big data framework for botnet detection based on network traffic analysis, Inf. Sci., 512 (2020), 629–640. doi: 10.1016/j.ins.2019.10.018. doi: 10.1016/j.ins.2019.10.018
![]() |
[14] | M. Kim, H. Kong, S. Hong, S. Chung, J. W. Hong, A flow-based method for abnormal network traffic detection, in 2004 IEEE/IFIP network operations and management symposium (IEEE Cat. No. 04CH37507), 1 (2004), 599–612. |
[15] |
E. Corchado, Á. Herrero, Neural visualization of network traffic data for intrusion detection, Appl. Soft Comput., 11 (2011), 2042–2056. doi: 10.1016/j.asoc.2010.07.002. doi: 10.1016/j.asoc.2010.07.002
![]() |
[16] |
T. J. M. Bench-Capon, P. E. Dunne, Argumentation in artificial intelligence, Artif. Intell., 171 (2007), 619–641. doi: 10.1016/j.artint.2007.05.001. doi: 10.1016/j.artint.2007.05.001
![]() |
Triggering mechanism | No. of control updates |
Time triggered control scheme in (2.12) (τ = 0.001) | 1001 |
ET control scheme in (2.19) | 314 |
Quantization ET control scheme in (3.1) | 208 |
Triggering mechanism | No. of control updates |
Time triggered control scheme in (2.12)(τ =0.001) | 2001 |
ET control scheme in (2.19) | 42 |
Quantization ET control scheme in (3.1) | 25 |
Triggering mechanism | No. of control updates |
Time triggered control scheme in (2.12) (τ = 0.001) | 1001 |
ET control scheme in (2.19) | 314 |
Quantization ET control scheme in (3.1) | 208 |
Triggering mechanism | No. of control updates |
Time triggered control scheme in (2.12)(τ =0.001) | 2001 |
ET control scheme in (2.19) | 42 |
Quantization ET control scheme in (3.1) | 25 |