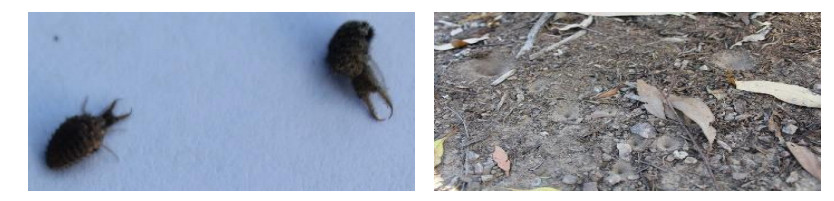
Multilevel thresholding has important research value in image segmentation and can effectively solve region analysis problems of complex images. In this paper, Otsu and Kapur's entropy are adopted among thresholding segmentation methods. They are used as the objective functions. When the number of threshold increases, the time complexity increases exponentially. In order to overcome this drawback, a modified ant lion optimizer algorithm based on opposition-based learning (MALO) is proposed to determine the optimum threshold values by the maximization of the objective functions. By introducing the opposition-based learning strategy, the search accuracy and convergence performance are increased. In addition to IEEE CEC 2017 benchmark functions validation, 11 state-of-the-art algorithms are selected for comparison. A series of experiments are conducted to evaluate the segmentation performance of the algorithm. The evaluation metrics include: fitness value, peak signal-to-noise ratio, structural similarity index, feature similarity index, and computational time. The experimental data are analyzed and discussed in details. The experimental results significantly demonstrate that the proposed method is superior over others, which can be considered as a powerful and efficient thresholding technique.
Citation: Shikai Wang, Kangjian Sun, Wanying Zhang, Heming Jia. Multilevel thresholding using a modified ant lion optimizer with opposition-based learning for color image segmentation[J]. Mathematical Biosciences and Engineering, 2021, 18(4): 3092-3143. doi: 10.3934/mbe.2021155
[1] | Xiaoxu Peng, Heming Jia, Chunbo Lang . Modified dragonfly algorithm based multilevel thresholding method for color images segmentation. Mathematical Biosciences and Engineering, 2019, 16(6): 6467-6511. doi: 10.3934/mbe.2019324 |
[2] | Wenqi Ji, Xiaoguang He . Kapur's entropy for multilevel thresholding image segmentation based on moth-flame optimization. Mathematical Biosciences and Engineering, 2021, 18(6): 7110-7142. doi: 10.3934/mbe.2021353 |
[3] | Shikai Wang, Heming Jia, Xiaoxu Peng . Modified salp swarm algorithm based multilevel thresholding for color image segmentation. Mathematical Biosciences and Engineering, 2020, 17(1): 700-724. doi: 10.3934/mbe.2020036 |
[4] | Nilkanth Mukund Deshpande, Shilpa Gite, Biswajeet Pradhan, Ketan Kotecha, Abdullah Alamri . Improved Otsu and Kapur approach for white blood cells segmentation based on LebTLBO optimization for the detection of Leukemia. Mathematical Biosciences and Engineering, 2022, 19(2): 1970-2001. doi: 10.3934/mbe.2022093 |
[5] | Shenghan Li, Linlin Ye . Multi-level thresholding image segmentation for rubber tree secant using improved Otsu's method and snake optimizer. Mathematical Biosciences and Engineering, 2023, 20(6): 9645-9669. doi: 10.3934/mbe.2023423 |
[6] | Hong Qi, Guanglei Zhang, Heming Jia, Zhikai Xing . A hybrid equilibrium optimizer algorithm for multi-level image segmentation. Mathematical Biosciences and Engineering, 2021, 18(4): 4648-4678. doi: 10.3934/mbe.2021236 |
[7] | Yating Fang, Baojiang Zhong . Cell segmentation in fluorescence microscopy images based on multi-scale histogram thresholding. Mathematical Biosciences and Engineering, 2023, 20(9): 16259-16278. doi: 10.3934/mbe.2023726 |
[8] | Yu Li, Meilong Zhu, Guangmin Sun, Jiayang Chen, Xiaorong Zhu, Jinkui Yang . Weakly supervised training for eye fundus lesion segmentation in patients with diabetic retinopathy. Mathematical Biosciences and Engineering, 2022, 19(5): 5293-5311. doi: 10.3934/mbe.2022248 |
[9] | Jiande Zhang, Chenrong Huang, Ying Huo, Zhan Shi, Tinghuai Ma . Multi-population cooperative evolution-based image segmentation algorithm for complex helical surface image. Mathematical Biosciences and Engineering, 2020, 17(6): 7544-7561. doi: 10.3934/mbe.2020385 |
[10] | Ran Zhou, Yanghan Ou, Xiaoyue Fang, M. Reza Azarpazhooh, Haitao Gan, Zhiwei Ye, J. David Spence, Xiangyang Xu, Aaron Fenster . Ultrasound carotid plaque segmentation via image reconstruction-based self-supervised learning with limited training labels. Mathematical Biosciences and Engineering, 2023, 20(2): 1617-1636. doi: 10.3934/mbe.2023074 |
Multilevel thresholding has important research value in image segmentation and can effectively solve region analysis problems of complex images. In this paper, Otsu and Kapur's entropy are adopted among thresholding segmentation methods. They are used as the objective functions. When the number of threshold increases, the time complexity increases exponentially. In order to overcome this drawback, a modified ant lion optimizer algorithm based on opposition-based learning (MALO) is proposed to determine the optimum threshold values by the maximization of the objective functions. By introducing the opposition-based learning strategy, the search accuracy and convergence performance are increased. In addition to IEEE CEC 2017 benchmark functions validation, 11 state-of-the-art algorithms are selected for comparison. A series of experiments are conducted to evaluate the segmentation performance of the algorithm. The evaluation metrics include: fitness value, peak signal-to-noise ratio, structural similarity index, feature similarity index, and computational time. The experimental data are analyzed and discussed in details. The experimental results significantly demonstrate that the proposed method is superior over others, which can be considered as a powerful and efficient thresholding technique.
With the emergence of computer technology, image processing has been widely used in many fields. Image segmentation is one of the classical topics in image processing [1]. It divides the original image into multiple sub-regions according to intensity, color, texture, and other attributes of the image [2]. Image segmentation is often the preprocessing phase of higher-class processing such as: image analysis, object recognition, and computer vision. Consequently, the performance of higher-class processing system depends on the accuracy of the segmentation technique adopted [3]. Researchers have proposed a range of segmentations, including edge detection, histogram based thresholding, region, feature clustering, and neural networks [4,5,6]. Histogram based thresholding is a simple and the most commonly used image segmentation method [7,8]. Thresholding methods can be divided into two categories: bi-level thresholding and multi-level thresholding. Bi-level thresholding means that the target and background can be clearly distinguished by a single threshold value. Multi-level thresholding denotes that the given image can be segmented into various classes by multiple threshold values [9,10,11].
Among available image thresholding techniques, Otsu and Kapur's entropy are two state-of-the-art thresholding methods [12,13,14]. Otsu and Kapur's entropy find the optimal threshold values according to some preset criteria. Otsu maximizes the variance of the histogram classes, and Kapur's entropy maximizes the entropy of the histogram. These thresholding techniques mentioned above can be easily extended to multilevel thresholding segmentation. However, the exhaustive search makes it inefficient to find the optimal threshold values, and the time complexity increases exponentially when the number of threshold increases. In order to overcome the above drawback, swarm intelligence (SI) algorithms are extensively used in multilevel thresholding problems [15,16]. Ibrahim et al. proposed the fish images segmentation model based on salp swarm algorithm, in which Otsu method is used to determine the threshold and extract fish from the original image [17]. Ouadfel et al. studied two metaheuristic algorithms: flower pollination algorithm and social spiders optimization. Then the performance of multilevel thresholding methods was evaluated comprehensively [18]. Infrared image is of great value in medical research. Díaz-Cortés applied dragonfly algorithm in medical image segmentation technology [19]. Satapathy et al. used Otsu method and a novel chaotic bat algorithm (CBA) to address bi-level and multi-level image thresholding problem. The chaotic map was considered to update the position of bat during the optimization search. The proposed CBA offered superior image quality measure values [20]. Salvi et al. presented an adaptive method for nuclei segmentation in H & E stained images, named multiscale adaptive nuclei analysis (MANA). High segmentation performances were obtained for different organ images [21]. Feng et al. invented a thresholding technique of 3D Otsu based on dimension decomposition rule. The test results on medical images showed that the proposed technique reduced time complexity without the loss of segmentation quality [22]. Zhao et al. proposed an improved ant colony optimizer (RCACO) using a random spare strategy and chaotic intensification strategy. The convergence speed and the convergence accuracy were main gains. Furthermore, it was also an excellent multilevel image segmentation algorithm [23]. In [24], the horizontal crossover search (HCS) and the vertical crossover search (VCS) were introduced into the ant colony optimizer for the first time. The proposed algorithm was named CCACO. The newly proposed algorithm was evaluated in the field of function optimization and image segmentation [24]. He et al. introduced the efficient krill herd (EKH) algorithm into image segmentation. The experimental results showed that the presented EKH algorithm was superior to the other algorithms [25]. Hilali-Jaghdam et al. proposed a method based on the classical and quantum genetic algorithms to solve the medical images segmentation. The Genetic Algorithm (GA) used a binary coding while the Quantum Genetic Algorithm (QGA) used the qubit encoding of individuals [26]. Wu et al. proposed an improved teaching-learning-based optimization algorithm (DI-TLBO) and successfully applied it in casting X-ray image segmentation for multi-level threshold [27].
Mirjalili proposed a novel nature-inspired algorithm called ant lion optimizer (ALO) in 2015 [28]. Obviously, ALO algorithm simulates the intelligent behavior of antlions in nature, including building traps, catching preys, and rebuilding traps. In 2018, Hadidian-Moghaddam et al. used ALO algorithm to solve the optimal sizing and siting of distributed generation. The results showed that ALO had better performance than particle swarm optimization (PSO) and genetic algorithm (GA) in this field [29]. Raju et al. applied ALO algorithm for optimization of the controller gains. The results showed that the controller performed better in stabilization time(s), peak overshoot, and oscillations [30]. In 2016, by using ALO algorithm, Saxena et al. solved the problem of antenna current and antenna position optimization [31]. In the paper of Umaheswari et al., ALO was first adopted to solve the integrated maintenance scheduling problem [32]. Dinakara et al. proposed to determine the optimal distributed generation size by ALO. This approach achieved the purpose of reducing system power loss and improving voltage profile [33]. In [34], ALO was performed multilevel thresholding based on the contextual information of the image. Contextual information enhanced the quality of the segmented image as it considered not only the pixel value but also its vicinity. Jin et al. proposed a method to image segmentation based on ALO and fuzzy c-means (FCM). The proposed method alleviated the problem that the segmentation results of the images were unsatisfactory due to the presence of noise [35]. Yue et al. proposed an improved ALO called BIALO to enhance industrial images. Three strategies improved exploration and exploitation capabilities of the native algorithm [36]. In fact, there are still several disadvantages of the native ALO, such as the slow convergence rate, falling easily into local optimum and so on. In view of this phenomenon, it is necessary to improve the performance of the native ALO. In the paper of Wu et al., chaos was introduced into the initialization stage of ALO algorithm [37]. The improvement of ALO algorithm by Subhashini was to change the elite weight [38]. Majhi et al. proposed a hybrid clustering method based on k-means and ALO algorithm for cluster analysis in 2018 [39]. Some authors also include opposition-based learning (OBL) in their methods. This scheme can look for the opposite direction of each candidate solution. If the opposite point is beneficial then it is used as the candidate solution before proceeding to the next iteration. OBL helps in further exploration and better probability to converge to the optimum. Sarkhel et al. embedded OBL into harmony search algorithm, which improved the convergence rate of the algorithm [40]. Ewees et al. proposed a grasshopper optimization algorithm based on OBL strategy. Experimental results proved that the modified algorithm was competitive on engineering optimization problems [41]. In 2016, Ahandani et al. combined OBL with shuffled bidirectional differential evolution algorithm, which sped up the search process the algorithm [42].
Inspired by these successful applications of OBL strategy, OBL strategy is introduced into ALO algorithm to avoid falling into the local optimum. The search accuracy and convergence performance are improved. Then Otsu and Kapur's entropy as objective functions are maximized by MALO to find the optimum threshold values. Finally, the provided images are segmented into some classes. In this paper, IEEE CEC2017 benchmark functions [43] are used to evaluate the effectiveness of MALO. 7 traditional algorithms and 4 improved algorithms are selected for comparisons. The quality of the segmented images is evaluated in terms of fitness value, peak signal-to-noise ratio (PSNR) [44,45], structural similarity index (SSIM) [46,47,48], feature similarity (FSIM) [49,50], and computational time. The experimental results confirm that the proposed method can be used effectively for multilevel thresholding.
The remainder of this paper is organized as follows: Section 2 outlines Otsu and Kapur's entropy methods for multilevel thresholding. Section 3 gives an overview of ALO followed by its mathematical model. ALO algorithm based on opposition-based learning for multilevel thresholding color image segmentation is presented in Section 4. Simulation experiments and results analysis are described in Section 5. Finally, Section 6 concludes the work and suggests some directions for future studies.
Thresholding segmentation processes the histogram of digital images. An algorithm is used as the segmentation criterion. The threshold that satisfies the criterion function is called the optimal threshold. Based on the optimal threshold, the image is divided into target region and background region. The image thresholding method can be summarized into two categories: bi-level thresholding segmentation and multilevel thresholding segmentation. Bi-level thresholding segmentation cannot completely extract the target for a particular image. Multilevel thresholding divides the whole image into multiple regions. Multilevel thresholding segmentation can highlight the features among image regions.
The color image is represented by three different 8-bit gray values of R, G, and B channels. ni denotes the number of pixels whose gray level is i. N denotes the total number of pixels. pi denotes the distribution probability of the ith gray value. They are defined as follows:
N=L−1∑i=0ni | (1) |
pi=niN | (2) |
L−1∑i=0pi=1 | (3) |
Suppose there are K thresholds of t1,t2,⋅⋅⋅,tK. They divide the gray level of a given image into K+1 classes: C0=[0,1,⋅⋅⋅,t1−1],C1=[t1,t1+1,⋅⋅⋅,t2−1],CK=[tK,tK+1,⋅⋅⋅,L−1].
The selection of threshold is related to the quality of the segmentation results. In this paper, Otsu and Kapur's entropy methods are adopted.
Otsu is an automatic threshold selection method for image segmentation, which was proposed in 1979. According to the grayscale characteristics of images, Otsu calculates the variance of class and determines the threshold. When the maximum variance of class is obtained, the threshold returned by the objective function is called the optimal threshold.
Equations (4)–(6) represent the probabilities of the class occurrence, the class mean levels, and the total mean level of the original image, respectively.
ωj=∑i∈Cjpij=0,1,⋅⋅⋅,K. | (4) |
μj=∑i∈Cjipiωj | (5) |
μT=L−1∑i=0ipi | (6) |
Then the total variance among the classes is:
σ2(t1,t2,⋅⋅⋅,tK)=k∑j=0ωj(μj−μT) | (7) |
When σ2(t1,t2,⋅⋅⋅,tK) takes the maximum value, (t1∗,t2∗,⋅⋅⋅,tk∗) is the optimal thresholding group of Otsu method.
In thresholding segmentation methods, Kapur's entropy method introduces the "entropy" of information theory into the segmentation. According to the additivity of entropy, the total entropy of the segmented image is:
ψ(t1,t2,⋅⋅⋅tK)=−t1−1∑i=0piP0lnpiP0−t2−1∑i=t1piP1lnpiP1⋅⋅⋅−L−1∑i=tKpiPKlnpiPK | (8) |
Pj=∑i∈Cjpij=0,1,⋅⋅⋅,K. | (9) |
where pi is the distribution probability of the ith gray value, Pk is the probability of each class.
Kapur's entropy method finds the optimal threshold values based on maximizing the total entropy. The optimal threshold is determined by the following equation:
{t1∗,t2∗,⋅⋅⋅,tK∗}=argmax0<t1<t2⋅⋅⋅<tK<L−1(ψ(t1,t2,⋅⋅⋅,tK)) | (10) |
Ant lion optimizer is a novel swarm intelligent optimization algorithm. By simulating the process of antlion preying on ants in nature, the practical optimization problem is solved. The antlion uses its jaw to dig a cone-shaped pit in sand. After digging the trap, the antlion hides underneath the bottom of the cone. Random walks of ants may fall into it. Once an ant falls into trap, the antlion throws sand to the edge of the pit and preys on it. After the antlion eats prey, it rebuilds its trap for the next hunt. Figure 1 shows several antlions and cone-shaped pits with different sizes. The details of the ALO algorithm are discussed as follows.
Since ants move randomly in nature, the following equation is established to simulate the random movement of ants.
X(t)=[0,cumsum(2r(t1)−1),⋅⋅⋅,cumsum(2r(tn)−1)] | (11) |
where the cumsum function evaluates the cumulative value of an array, r(t) function is defined as follows:
r(t)={1ifrand>0.50ifrand<0.5 | (12) |
where t shows the number of iterations in this study, rand is a random number that belongs to [0, 1].
In order to make random walks of ants in the search space, the following equation is needed to normalize Eq (11).
Xti=(Xti−ai)×(dti−cti)(bi−ai)+cti | (13) |
where ai denotes the minimum of random walk array X(t), bi denotes the maximum of random walk array X(t), cti denotes the lower boundary of ith space at tth iteration, and dti denotes the upper boundary of ith space at tth iteration.
The ants move randomly around the antlion, and the boundary of the area is affected by the position of the antlion. The calculation equations of cti and dti are as follows:
cti=Antliontj+ct | (14) |
dti=Antliontj+dt | (15) |
where cti denotes the lower boundary at tth iteration, and dti is the upper boundary at tth iteration. Antliontj shows the position of the jth antlion at tth iteration by the roulette wheel selection.
Once the ant enters the trap, in order to prevent it from escaping, the antlion immediately digs out the sand outside the hole to make the ant slide into the bottom of the hole. This process can be seen as the decreasing radius of the ant random walk. The equations are as follows:
ct=ctI | (16) |
dt=dtI | (17) |
where I is the ratio of boundary contraction, and its equation is defined as follows:
I=10ωtT | (18) |
where t is the current iteration, T is the maximum of iterations. ω is a fixed value, which can adjust the speed of ant moving to antlion.
According to the elite strategy, the antlion with the highest fitness value is considered as an elite, and the elite can affect the random walks of all ants. The antlion and the elite affect ant walking path. The ant's position update equation is as follows:
Antti=RtA+RtE2 | (19) |
where RtA is an ant random walks around an antlion selected by roulette wheel, RtE is this ant random walks around the elite.
If the fitness of the updated ant is better than that selected antlion, which means that the ant is caught and eaten by the antlion. Then the position of the antlion is updated to this position of ant. The two equations are used as follows:
Antliontj=Anttiiff(Antti)>f(Antliontj) | (20) |
where Antti shows the ith ant with the best fitness value at tth iteration, and f is the fitness function value.
ALO algorithm has drawbacks of falling easily into local optimum and lower search accuracy. In this section, a modified ALO algorithm is proposed. MALO uses opposition-based learning strategy to generate opposite solutions, which is helpful to search more effective regions. And then, MALO maximizes Otsu and Kapur's entropy methods to determine the optimal threshold.
Tizhoosh et al. proposed the concept of opposition-based learning in 2005. The main idea of this strategy is to generate opposite solutions. By comparing opposite solutions with current solutions, excellent individuals can enter the next generation. Mathematically, the strategy is defined as follows:
Suppose x=(x1,x2,…,xD) is a point in the D-dimensional search space (can be regarded as a feasible solution), xj=[aj,bj],j=1,2,…,D, and its corresponding opposite point ˜x=(˜x1,˜x2,…,˜xD) can be defined as:
˜x=aj+bj−xj | (21) |
The fitness values of the current solution and the opposite solution are calculated. By comparison, the solution with the optimal fitness value is preserved.
ALO algorithm has the advantages of simple principle, fewer parameter settings and so on. It also has the problems of slower convergence speed, falling easily into the local optimum, and lower search accuracy. In order to improve the exploration and exploitation of the ALO algorithm, OBL strategy is introduced in the native ALO. The probability of convergence to the global optimum is increased. For each iteration, the position of ant Antti is obtained by Eq (19). However, there may be a possibility that Antti is opposite or near the optimal position in the search space. Therefore, after each iteration, OBL is applied to generate the opposite solution ~Antti. The equation is as follows:
~Antti=ub+lb−Antti | (22) |
where ub denotes the upper bound and lb denotes the lower bound.
The fitness values of the new position and the original position are compared. After the evaluation, the position with high fitness values are retained. After this process, the modification algorithm has more search attempts in each iteration [51,52].
The time complexity of MALO is given based on four factors such as the number of search agents N, the number of dimension D, the maximum number of iterations T, the cost of function F. In the initialization process, the time complexity is O(N). The time complexity of sorting mechanism is O(N × logN). The time complexity of function evaluation is O(T × N × F). The time complexity is expressed as O(T × N × D) for the updating the positions. The time complexity of the updating phase by the OBL is O(T × N × D). The overall time complexity of MALO is O(N ×(1 + logN + T ×(F + 2D))).
The process of finding the optimal threshold is actually to find the optimal solution. However, it has high time complexity when dealing with multilevel thresholds. In order to achieve efficiency, it is entirely possible to use the modified ALO algorithm to do this. The basic steps are described as follows:
Firstly, test images in JPG format are read and histograms are obtained. The number of search agents and maximum number of iterations are initialized. MALO is implemented considering two different objective functions: Otsu and Kapur's entropy. Then, the fitness of initial population is calculated. The main loop begins with the second iteration. The updating position of ant is determined by a selected antlion by the roulette wheel and the elite. OBL strategy is applied to the obtained position of ant for generating the opposite solution. The fitness of original position and opposite position of ant are calculated and compared. The individual with high fitness value is preserved. If the fitness of ant is better than the antlion, then the position of antlion will be updated to the position of ant. This process is repeated until the maximum number of iterations is completed. The position of elite represents the optimal thresholds. The segmentation is carried out based on the obtained thresholds. Finally, the values of the evaluation measures and the segmented images are output. The pseudo code and the flowchart of MALO algorithm based multilevel thresholding are provided in Algorithm 1 and Figure 2, respectively.
In this section, in order to evaluate the quality of image segmentation based on MALO, a series of experiments are conducted. Firstly, Section 5.1 indicates the parameter settings of comparison algorithms, test images selected in the experiment, and operating environment. Section 5.2 introduces the metrics used to evaluate the image segmentation performance in the experiment. Section 5.3 uses IEEE CEC2017 benchmark functions to validate the effectiveness of MALO. Section 5.4 compares MALO with some traditional algorithms. The experimental data are analyzed, and the performance of image segmentation based on various algorithms is evaluated. Furthermore, Section 5.5 compares MALO with some improved algorithms. The future research is also conducted.
In this paper, 10 color images are selected from the Berkeley University database [53] for performance analysis. Figure 3 shows the original test images and the corresponding histograms. All images are in JPG format with a size of 481 × 321 pixels. Each algorithm runs each image 30 times separately. The number of threshold K includes: 4, 6, 8, 10, and 12.
In order to prove the superiority of the MALO algorithm, 7 traditional algorithms and 4 improved algorithms which have been proposed and widely applied to multilevel thresholding segmentation are selected for comparisons, including SSA [17], MVO [54], DA [19], FPA [18], PSO [55], SCA [34], MABC [56], IDSA [57], WOA-TH [58], and BDE [59]. These comparison algorithms have different search strategies and mathematical equations. The maximum of iterations for all algorithms is 500 and the population size is 25. We follow the same parameters in the original papers. The main parameters of various algorithms are shown in Table 1.
Algorithm | Parameters | Value |
SSA | Balance coefficient c1 | [0, 2] |
Random number c2, c3 | [0, 1] | |
Switch possibility | 0.5 | |
MVO | Wormhole Existence Probability | [0.2, 1] |
Travelling Distance Rate | [0, 1] | |
Random number r1, r2, r3 | [0, 1] | |
DA | Inertial weight ω | [0.5, 0.9] |
Seperation weight | [0, 0.2] | |
Alignment weight | [0, 0.2] | |
Cohesion weight | [0, 0.2] | |
Food factor | [0, 2] | |
Enemy factor | [0, 0.1] | |
FPA | Switch possibility | 0.4 |
Lévy constant β | 1.5 | |
PSO | Maximum inertia weight | 0.9 |
Minimum inertia weight | 0.4 | |
Learning factors c1, c2 | 2 | |
Maximum velocity | +120 | |
Minimum velocity | −120 | |
SCA | Movement direction r1 | [0, 2] |
Movement distance r2 | [0, 2π] | |
Random weight r3 | [0, 2] | |
Random number r4 | [0, 1] | |
Switch possibility | 0.5 | |
ALO | Switch possibility | 0.5 |
MABC | Random number r | [0, 1] |
IDSA | Random number r | [0, 1] |
WOA-TH | Parameter a | [0, 2] |
Constant b | 1 | |
Random number l | [−1, 1] | |
Constant a0 | 13 | |
Initial value G0 | 40 | |
BDE | Number of objectives | 1 |
Number of constraints | 0 | |
Number of decision variables | 4 | |
Scaling factor | 0.5 | |
Crossover probability | 0.2 |
All the experimental series were carried out on MATLAB R2016b, and the computer was configured as Intel(R) Core(TM) i5-4210U CPU @1.70 GHz, using Microsoft Windows 10 system.
Algorithm 1. Pseudo code of MALO algorithm based multilevel thresholding. | |
1 | Input the image and calculate components of the histogram. |
2 | Initialize parameters: SearchAgents_no (the number of search agents), T (maximum number of iterations). |
3 | Calculate the fitness of initial ants and antlions; |
4 | Find the best antlions and assume it as the elite; |
5 | While t < T |
6 | For each ant |
7 | Select an antlion using Roulette wheel; |
8 | Update c and d using Eqs (16) and (17); |
9 | Create a random walk and normalize it using Eqs (11) and (13); |
10 | Update the position of ant using Eq (19); |
11 | End for |
12 | For each the position of ant |
13 | Generate the opposite solution of the position of ant using Eq (22); |
14 | Calculate the fitness of original position and opposite position of ant; |
15 | Compare fitness values and keep ant fitness and position with high fitness value; |
16 | End for |
17 | Update antlion positions and finesses based of the ants Eq (20); |
18 | Update the position of elite if any antlions becomes fitter than it; |
19 | t = t + 1; |
20 | End while |
21 | Return elite fitness and position, which elite position represents the optimal thresholds. |
22 | Output the values of the evaluation measures and the segmented images. |
This paper evaluates the quality of image segmentation from the following five aspects:
1) Fitness value. Since Otsu and Kapur's entropy methods are maximization problem, the fitness values are expected to be as large as possible. The optimal fitness values show that the algorithm has high accuracy and convergence performance.
2) PSNR. It is an objective evaluation measure based on pixel error. A higher PSNR value indicates that the quality of the distorted image is better. However, it is based on the error between corresponding pixels and does not consider the visual characteristics of human eyes. Its calculation equation is as follows:
PSNR=10log10L2MSE(db) | (23) |
where L represents the scale range of the image. For an 8-bit image, L = 255. MSE is the mean square error between the original image and the processed image.
MSE=∑Mm=1∑Nn=1[R(m,n)−I(m,n)]2M×N | (24) |
where M × N is the size of the image, R(m,n) represents the gray value of coordinates at the reference image (m, n), and I(m,n) represents the gray value of coordinates at the distorted image (m, n).
3) SSIM. It is an objective evaluation measure based on structural similarity. It measures the image similarity from brightness, contrast, and structure. SSIM value range is [0, 1]. If the value is closer to 1, the image distortion is smaller. It is defined as follows:
SSIM(R,I)=(2μRμI+C1)(2σRI+C2)(μ2R+μ2I+C1)(σ2R+σ2I+C2) | (25) |
where UR and UI are the average gray values of the original image R and the segmented image I, respectively. σ2R and σ2I represent the variance of image R and image I, respectively. σRI is the covariance of image R and image I. C1=(0.01L)2, C2=(0.03L)2.
4) FSIM. Based on SSIM, researchers have proposed a new evaluation measure, namely feature similarity algorithm (FSIM). We use two complementary features of phase congruency (PC) and gradient magnitude (GM) to calculate FSIM.
FSIM=∑x∈ΩSL(x)×PCm(x)∑x∈ΩPCm(x) | (26) |
where Ω is the pixel field of the entire image, SL(x) represents the similarity value of each position x, and PCm(x) denotes the phase consistency measure.
SL(x)=[SPC(x)]α×[SG(x)]β | (27) |
PCm(x)=max(PC1(x),PC2(x)) | (28) |
where SPC(x) is the similarity measure of phase consistency, SG(x) represents the similarity measure of gradient magnitude, and α, β are both constants.
SPC(x)=2PC1(x)×PC2(x)+T1PC21(x)×PC22(x)+T1 | (29) |
SG(x)=2G1(x)×G2(x)+T2G21(x)×G22(x)+T2 | (30) |
where T1 and T2 are positive constants.
5) Computational time. The smaller the value, the faster the algorithm execution speed.
In this subsection, 29 IEEE CEC2017 benchmark functions are chosen to check the efficiency of algorithms. We skip "F2" of IEEE CEC2017 because of its unstable behavior. These functions are divided into four groups: unimodal (F1–F3), multimodal (F4–F10), hybrid (F11–F20), and composition (F21–F30). Furthermore, the relevant composition, dimension, range limitation and optimal position of 29 functions can be found in [43]. Meanwhile, all experiments are conducted 30 times.
In MALO, the OBL strategy can developed more in the later search period. Compared with the native ALO, it has excellent exploration and exploitation. For the OBL strategy, putting it into ALO as a strategy can greatly improve the convergence and high efficiency. The performance of algorithms is evaluated according to the mean value (Mean) and standard deviation (Std). The stability of each model is evaluated by Std value. Meanwhile, the best results has been highlighted in boldface in Table 2. From the table above, we can observe that MALO based method gives the satisfied results in general. For example, in the unimodal and multimodal functions, the proposed method gives better results in 10 out of 18 cases (9 functions and 2 indexes). In terms of hybrid functions, the MALO based method outperforms in 12 out of 20 cases (10 functions and 2 indexes) for others. In the composition functions, the proposed method gives better results in 10 out of 20 cases (10 functions and 2 indexes). All the other algorithms show a certain difference with MALO based method. The experimental results above are effectively proved the superior performance of proposed method. The ability to avoid local optimization has enhanced. Thus, it can be said that the proposed method in this paper is more effective than competitors in 29 benchmark functions, so this paper combines MALO with multilevel thresholding segmentation method to improve the image segmentation accuracy.
F | SSA | MVO | DA | FPA | PSO | SCA | ALO | MALO | |
F1 | Mean | 2.7525 × 103 | 2.0775 × 104 | 5.3030 × 107 | 9.4246 × 107 | 2.3768 × 103 | 1.1165 × 109 | 1.6205 × 103 | 2.2691 × 102 |
Std | 3.1853 × 103 | 1.0204 × 104 | 1.3048 × 108 | 1.6194 × 108 | 3.3853 × 103 | 2.6690 × 108 | 1.6927 × 103 | 7.5223 × 102 | |
F3 | Mean | 3.0002 × 102 | 3.0015 × 102 | 3.3792 × 103 | 5.6230 × 103 | 3.0009 × 102 | 3.0174 × 103 | 4.5012 × 102 | 4.4652 × 102 |
Std | 8.4151 × 10−2 | 7.5858 × 10−2 | 3.5353 × 103 | 3.7208 × 103 | 7.7137 × 10−2 | 1.6524 × 103 | 3.7837 × 102 | 3.1829 × 102 | |
F4 | Mean | 4.0989 × 102 | 4.0702 × 102 | 4.2856 × 102 | 4.6977 × 102 | 4.0507 × 102 | 4.6709 × 102 | 4.1395 × 102 | 4.0394 × 102 |
Std | 16.908 | 9.3281 | 29.903 | 61.297 | 1.2334 | 30.876 | 22.338 | 12.248 | |
F5 | Mean | 5.2912 × 102 | 5.2264 × 102 | 5.1732 × 102 | 5.6206 × 102 | 5.4292 × 102 | 5.5799 × 102 | 5.2180 × 102 | 5.1692 × 102 |
Std | 12.497 | 1.1788 × 10 | 8.4145 | 21.327 | 14.890 | 6.6091 | 13.640 | 2.8811 | |
F6 | Mean | 6.1533 × 102 | 6.0237 × 102 | 6.0125 × 102 | 6.3945 × 102 | 6.1021 × 102 | 6.2361 × 102 | 6.1562 × 102 | 6.7956 × 102 |
Std | 8.9433 | 3.1079 | 1.4272 | 16.075 | 8.8947 | 5.4162 | 8.8988 | 10.159 | |
F7 | Mean | 7.4500 × 102 | 7.3257 × 102 | 7.3766 × 102 | 7.9322 × 102 | 7.3056 × 102 | 7.8595 × 102 | 7.5048 × 102 | 7.2403 × 102 |
Std | 15.007 | 12.483 | 14.458 | 26.384 | 12.387 | 10.193 | 26.572 | 2.3596 | |
F8 | Mean | 8.2630 × 102 | 8.2696 × 102 | 8.1554 × 102 | 8.4529 × 102 | 8.2131 × 102 | 8.4537 × 102 | 8.2771× 102 | 8.1001 × 102 |
Std | 11.690 | 12.498 | 7.2712 | 16.236 | 7.9025 | 8.7128 | 14.163 | 9.4471 | |
F9 | Mean | 1.0897 × 103 | 9.0116 × 102 | 9.2457 × 102 | 1.7066 × 103 | 9.6607 × 102 | 1.0671 × 103 | 1.1272 × 103 | 1.0417 × 103 |
Std | 2.5134 × 102 | 2.8801 | 51.037 | 6.1484 × 102 | 1.1193 × 102 | 81.732 | 2.2796 × 102 | 1.8741 × 102 | |
F10 | Mean | 1.8446 × 103 | 1.7723 × 103 | 1.7989 × 103 | 2.1907 × 103 | 1.9995 × 103 | 2.5054 × 103 | 2.0639 × 103 | 1.2226 × 103 |
Std | 2.8235 × 102 | 2.8547 × 102 | 3.4047 × 102 | 3.9008 × 102 | 2.9524 × 102 | 2.0358 × 102 | 3.1110 × 102 | 1.7148 × 102 | |
F11 | Mean | 1.1840 × 103 | 1.1325 × 103 | 1.2913 × 103 | 1.2604 × 103 | 1.1388 × 103 | 1.2494 × 103 | 1.2125 × 103 | 9.3895 × 104 |
Std | 71.184 | 27.254 | 8.0087 × 102 | 1.1285 × 102 | 2.7432 × 105 | 76.249 | 74.386 | 24.745 | |
F12 | Mean | 3.1659 × 106 | 1.9237 × 106 | 8.5333 × 105 | 5.3131 × 106 | 2.8460 × 109 | 3.6304 × 107 | 2.9647 × 106 | 2.2571 × 104 |
Std | 3.9918 × 106 | 2.4011 × 106 | 1.0164 × 106 | 5.2872 × 106 | 1.4432 × 109 | 4.6673 × 107 | 3.6635 × 106 | 3.1055 × 104 | |
F13 | Mean | 1.7372 × 104 | 1.2349 × 104 | 1.6552 × 104 | 2.3449 × 104 | 3.2613 × 108 | 8.9513 × 104 | 1.3896 × 104 | 1.1517 × 104 |
Std | 1.2611 × 104 | 9.7419 × 103 | 9.0887 × 103 | 1.8794 × 104 | 2.7709 × 108 | 8.0007 × 104 | 1.2201 × 104 | 7.8833 × 103 | |
F14 | Mean | 3.3621 × 103 | 3.0338 × 103 | 4.8484 × 103 | 2.8067 × 103 | 2.8688 × 103 | 2.3311 × 103 | 2.6070 × 103 | 2.3278 × 103 |
Std | 2.7150 × 103 | 2.3148 × 103 | 1.8854 × 103 | 1.4364 × 103 | 2.1263 × 103 | 1.0895 × 103 | 1.6260 × 103 | 1.2880 × 103 | |
F15 | Mean | 6.2001 × 103 | 3.3924 × 103 | 6.4284 × 103 | 9.6586 × 103 | 3.9125 × 103 | 3.7996 × 103 | 1.3375 × 104 | 3.0564 × 103 |
Std | 4.2086 × 103 | 3.5797 × 103 | 5.0026 × 103 | 7.7162 × 103 | 3.7024 × 103 | 2.3791 × 103 | 1.0871 × 104 | 2.1255 × 103 | |
F16 | Mean | 1.8063 × 103 | 1.8309 × 103 | 1.7338 × 103 | 1.9840 × 103 | 1.8428 × 103 | 1.8126 × 103 | 1.8928 × 103 | 1.8066 × 103 |
Std | 1.4760 × 102 | 1.6725 × 102 | 1.2125 × 102 | 1.4716 × 102 | 1.2492 × 102 | 80.085 | 1.6074 × 102 | 1.0356 × 102 | |
F17 | Mean | 1.7831 × 103 | 1.7871 × 103 | 1.7693 × 103 | 1.8044 × 103 | 1.7796 × 103 | 1.7897 × 103 | 1.7795 × 103 | 1.7359 × 103 |
Std | 38.227 | 56.931 | 33.562 | 52.636 | 59.130 | 14.372 | 39.757 | 34.260 | |
F18 | Mean | 1.9046 × 104 | 1.7527 × 104 | 2.4433 × 104 | 1.5703 × 104 | 1.6595 × 104 | 4.2187 × 105 | 1.6102 × 104 | 1.6102 × 104 |
Std | 1.3350 × 104 | 1.1493 × 104 | 1.5706 × 104 | 1.2231 × 104 | 1.3320 × 104 | 4.6787 × 105 | 1.3045 × 104 | 1.2885 × 104 | |
F19 | Mean | 9.3321 × 103 | 4.8206 × 103 | 1.1236 × 104 | 9.2778 × 104 | 5.1293 × 103 | 1.0034 × 104 | 1.6927 × 104 | 1.9544 × 103 |
Std | 7.1553 × 103 | 4.0080 × 103 | 6.8870 × 103 | 2.0401 × 105 | 3.8240 × 103 | 8.8662 × 103 | 1.2359 × 104 | 3.1306 × 103 | |
F20 | Mean | 2.1580 × 103 | 2.1181 × 103 | 2.1072 × 103 | 2.1974 × 103 | 2.1351 × 103 | 2.1393 × 103 | 2.1520 × 103 | 2.1017 × 103 |
Std | 77.357 | 71.982 | 59.164 | 81.489 | 81.620 | 37.232 | 76.695 | 1.1244 × 102 | |
F21 | Mean | 2.2892 × 103 | 2.3067 × 103 | 2.3146 × 103 | 2.3260 × 103 | 2.3033 × 103 | 2.2951 × 103 | 2.3181 × 103 | 2.2919 × 103 |
Std | 58.652 | 43.950 | 23.354 | 56.359 | 69.965 | 67.337 | 23.092 | 23.025 | |
F22 | Mean | 2.3032 × 103 | 2.4325 × 103 | 2.3387 × 103 | 2.3737 × 103 | 2.3168 × 103 | 2.3923 × 103 | 2.3019 × 103 | 2.2108 × 103 |
Std | 1.5330 | 4.0570 × 102 | 1.5916 × 102 | 3.0758 × 102 | 86.302 | 36.970 | 14.139 | 4.3619 | |
F23 | Mean | 2.6246 × 103 | 2.6213 × 103 | 2.6263 × 103 | 2.6544 × 103 | 2.6993 × 103 | 2.6630 × 103 | 2.6330 × 103 | 2.6235 × 103 |
Std | 9.4651 | 8.4456 | 11.398 | 27.680 | 38.621 | 9.4154 | 13.016 | 8.6164 | |
F24 | Mean | 2.7448 × 103 | 2.7399E+03 | 2.7422E+03 | 2.7726E+03 | 2.7821E+03 | 2.7773E+03 | 2.7541E+03 | 2.7242E+03 |
Std | 48.315 | 45.915 | 47.575 | 55.733 | 1.1838 × 102 | 59.112 | 13.296 | 10.259 | |
F25 | Mean | 2.9316 × 103 | 2.9322 × 103 | 2.9355 × 103 | 2.9755 × 103 | 2.9227 × 103 | 2.9816 × 103 | 2.9352 × 103 | 2.9316 × 103 |
Std | 30.687 | 28.163 | 23.579 | 53.181 | 23.221 | 24.991 | 28.272 | 2.7704 × 102 | |
F26 | Mean | 2.9852 × 103 | 3.0412 × 103 | 3.1910 × 103 | 3.5113 × 103 | 3.2078 × 103 | 3.1238 × 103 | 3.0689 × 103 | 3.0597 × 103 |
Std | 2.7007 × 102 | 3.8042 × 102 | 3.9782 × 102 | 4.9123 × 102 | 5.0084 × 102 | 37.746 | 2.7801 × 102 | 2.5490 × 102 | |
F27 | Mean | 3.0942 × 103 | 3.0936 × 103 | 3.0975 × 103 | 3.1538 × 103 | 3.1493 × 103 | 3.1084 × 103 | 3.1028 × 103 | 3.0346 × 103 |
Std | 3.6648 | 3.4486 | 6.0913 | 55.897 | 67.675 | 4.4917 | 15.597 | 1.4249 | |
F28 | Mean | 3.3187 × 103 | 3.3510 × 103 | 3.3579 × 103 | 3.4196 × 103 | 3.2028 × 103 | 3.3398 × 103 | 3.3863 × 103 | 3.1170 × 103 |
Std | 1.6379 × 102 | 1.3529 × 102 | 95.702 | 1.6486 × 102 | 78.068 | 90.179 | 1.3401 × 102 | 1.0508 × 102 | |
F29 | Mean | 3.2573 × 103 | 3.2282 × 103 | 3.2212 × 103 | 3.4234 × 103 | 3.2563 × 103 | 3.2629 × 103 | 3.2707 × 103 | 3.1694 × 103 |
Std | 81.947 | 83.366 | 55.368 | 1.3273 × 102 | 82.282 | 33.745 | 94.979 | 22.526 | |
F30 | Mean | 5.4626 × 105 | 5.5507 × 105 | 1.0112 × 106 | 2.2409 × 106 | 6.8210 × 104 | 1.7827 × 106 | 7.0103 × 105 | 1.5116 × 104 |
Std | 5.8213 × 105 | 6.5747 × 105 | 1.3632 × 106 | 2.9983 × 106 | 6.4354 × 104 | 1.3111 × 106 | 2.1161 × 106 | 7.2776 × 104 |
The fitness value can be used as an index to evaluate the performance of segmentation method. All the experiments are conducted 30 times on 10 color images. Tables 3 and 4 respectively show average fitness values using Otsu and Kapur's entropy compared with other algorithms. In every line, the maximum is in bold. From these tables, it can be seen that MALO algorithm finds the maximum fitness values more times than other algorithms. However, the same objective function value obtained by several algorithms also occasionally occurs when the threshold value is small (such as K = 4, 6, and 8). Overall, the OBL strategy can improve the calculation accuracy of ALO algorithm, which helps to find the global optimal solution and improve the overall performance.
Image | K | SSA | MVO | DA | FPA | PSO | SCA | ALO | MALO |
Cactus | 4 | 2126.2412 | 2126.2412 | 2126.2412 | 2084.2192 | 2126.2085 | 2091.2043 | 2126.1077 | 2126.2412 |
6 | 2188.8026 | 2188.7881 | 2188.7749 | 2152.9126 | 2180.5976 | 2151.3710 | 2188.6529 | 2188.8076 | |
8 | 2213.1588 | 2213.1207 | 2210.6763 | 2185.1975 | 2206.7101 | 2177.4155 | 2212.8736 | 2213.2108 | |
10 | 2224.0745 | 2224.9921 | 2224.4972 | 2202.9660 | 2224.8409 | 2205.3385 | 2223.8535 | 2225.0378 | |
12 | 2228.0349 | 2231.4043 | 2227.1859 | 2208.4757 | 2230.3736 | 2209.0205 | 2230.9917 | 2231.8810 | |
Kangaroo | 4 | 1114.7964 | 1114.7964 | 1114.7964 | 1088.7665 | 1114.7783 | 1088.6203 | 1114.7451 | 1114.7964 |
6 | 1164.7339 | 1164.7235 | 1162.2148 | 1131.7231 | 1157.9995 | 1142.5799 | 1164.5055 | 1164.7463 | |
8 | 1186.9998 | 1187.2317 | 1186.4981 | 1164.0753 | 1181.4079 | 1157.1404 | 1187.0901 | 1187.3921 | |
10 | 1195.5800 | 1198.8061 | 1198.1305 | 1175.6658 | 1195.7200 | 1172.3154 | 1197.6616 | 1199.0721 | |
12 | 1201.3621 | 1204.3553 | 1204.0964 | 1189.4752 | 1203.7897 | 1186.9269 | 1204.8168 | 1205.6190 | |
Temple | 4 | 1510.8080 | 1511.3099 | 1511.3110 | 1481.4555 | 1511.2952 | 1484.9151 | 1511.1720 | 1511.3110 |
6 | 1562.1584 | 1551.2497 | 1562.1657 | 1539.3204 | 1547.2460 | 1528.3151 | 1562.0323 | 1562.1677 | |
8 | 1575.4345 | 1581.9379 | 1580.1512 | 1558.9731 | 1577.2711 | 1552.2760 | 1578.5870 | 1582.0010 | |
10 | 1585.0462 | 1589.3769 | 1588.9537 | 1566.9471 | 1583.4601 | 1562.6224 | 1589.3855 | 1590.0709 | |
12 | 1593.4902 | 1592.3625 | 1594.7102 | 1577.6113 | 1589.2313 | 1577.5018 | 1595.2350 | 1596.7043 | |
Flower | 4 | 2319.2628 | 2319.2628 | 2319.2628 | 2300.8905 | 2319.2628 | 2305.0308 | 2319.2088 | 2319.2628 |
6 | 2373.7148 | 2373.9784 | 2373.9666 | 2349.7530 | 2366.7255 | 2348.8170 | 2373.7751 | 2374.0062 | |
8 | 2398.3260 | 2397.7703 | 2398.6865 | 2373.7415 | 2393.6744 | 2378.1142 | 2398.0974 | 2398.7056 | |
10 | 2408.6487 | 2409.1083 | 2410.1377 | 2392.7685 | 2409.5320 | 2393.4470 | 2409.9581 | 2410.1748 | |
12 | 2415.5456 | 2416.1915 | 2413.2197 | 2401.7458 | 2413.6013 | 2402.8505 | 2416.6995 | 2417.4773 | |
Mountain | 4 | 1922.6304 | 1922.6304 | 1922.6304 | 1880.8725 | 1922.6304 | 1899.0075 | 1922.5604 | 1922.6304 |
6 | 1966.3537 | 1969.5924 | 1969.5227 | 1950.3434 | 1964.7413 | 1937.2954 | 1969.3259 | 1969.6134 | |
8 | 1987.6882 | 1987.6512 | 1987.6006 | 1971.0006 | 1982.4353 | 1963.1189 | 1987.3940 | 1989.0684 | |
10 | 1998.2296 | 1998.3989 | 1999.2217 | 1976.2590 | 1995.3262 | 1980.4772 | 1998.6023 | 1999.5736 | |
12 | 2002.3212 | 2003.5176 | 2003.4479 | 1991.7908 | 2002.3326 | 1987.1898 | 2003.1927 | 2004.4332 | |
Tree | 4 | 4836.5287 | 4836.5254 | 4836.5287 | 4811.7023 | 4836.5184 | 4799.5909 | 4836.4226 | 4836.5287 |
6 | 4910.3426 | 4910.3170 | 4910.3328 | 4881.3483 | 4899.1851 | 4889.6357 | 4909.9888 | 4910.3389 | |
8 | 4938.5519 | 4938.7280 | 4939.0548 | 4895.7628 | 4932.5126 | 4897.8287 | 4939.0439 | 4939.2738 | |
10 | 4954.4191 | 4954.3250 | 4954.3494 | 4931.0184 | 4950.5741 | 4925.6878 | 4953.6297 | 4954.7408 | |
12 | 4961.5040 | 4962.0370 | 4961.7003 | 4947.5680 | 4958.4948 | 4933.2448 | 4961.9255 | 4962.8681 | |
Horse | 4 | 4169.9500 | 4169.9500 | 4169.9475 | 4146.0648 | 4169.9500 | 4125.8875 | 4169.9152 | 4169.9500 |
6 | 4223.2790 | 4226.2869 | 4226.2925 | 4206.4679 | 4226.2960 | 4184.3739 | 4226.1384 | 4226.2981 | |
8 | 4251.0736 | 4247.9571 | 4250.7202 | 4224.2255 | 4242.3477 | 4224.8735 | 4251.0627 | 4251.0806 | |
10 | 4264.1067 | 4264.0484 | 4263.6881 | 4245.5168 | 4263.0609 | 4242.4543 | 4263.8776 | 4264.5464 | |
12 | 4271.0552 | 4270.2470 | 4270.4589 | 4253.0366 | 4270.9270 | 4255.1561 | 4269.9287 | 4272.7303 | |
Bridge | 4 | 4386.0155 | 4386.0155 | 4386.0155 | 4348.0212 | 4386.0155 | 4364.6402 | 4385.8679 | 4386.0155 |
6 | 4448.8450 | 4456.6582 | 4456.6941 | 4430.7839 | 4456.6543 | 4416.6522 | 4448.6228 | 4456.6941 | |
8 | 4483.9318 | 4476.1230 | 4481.9799 | 4459.7426 | 4480.7550 | 4436.7087 | 4480.4869 | 4484.0957 | |
10 | 4495.8304 | 4494.1137 | 4497.7496 | 4475.7876 | 4494.2483 | 4472.8251 | 4497.6619 | 4498.1770 | |
12 | 4505.0626 | 4504.6934 | 4502.9179 | 4488.9690 | 4504.8794 | 4485.6536 | 4504.6902 | 4506.5159 | |
Pilot | 4 | 3854.8970 | 3854.8970 | 3854.8970 | 3838.0151 | 3854.8930 | 3807.6575 | 3854.8127 | 3854.8970 |
6 | 3902.7853 | 3896.5334 | 3902.7747 | 3884.5667 | 3902.7796 | 3869.1994 | 3902.6187 | 3902.7731 | |
8 | 3917.0447 | 3917.7421 | 3920.6198 | 3892.5037 | 3918.0121 | 3895.6690 | 3920.4228 | 3923.5580 | |
10 | 3931.0115 | 3928.4714 | 3933.5071 | 3915.8877 | 3931.1832 | 3904.4969 | 3932.5873 | 3934.2915 | |
12 | 3937.8965 | 3936.9459 | 3936.6517 | 3916.5100 | 3935.4087 | 3916.0317 | 3939.2200 | 3940.3098 | |
Dog | 4 | 2249.9855 | 2249.9839 | 2249.9855 | 2231.2675 | 2249.9855 | 2233.1946 | 2249.9484 | 2249.9855 |
6 | 2299.7269 | 2299.6899 | 2298.6466 | 2270.5848 | 2287.9683 | 2277.7186 | 2299.5470 | 2299.7446 | |
8 | 2320.5275 | 2317.0263 | 2320.4939 | 2297.8192 | 2303.3155 | 2296.9113 | 2320.2281 | 2320.5319 | |
10 | 2326.4485 | 2329.6067 | 2330.9585 | 2311.9971 | 2328.1692 | 2306.4227 | 2330.4593 | 2330.9965 | |
12 | 2334.7496 | 2336.2099 | 2334.3909 | 2323.5986 | 2332.0819 | 2320.2905 | 2335.7878 | 2337.4348 |
Image | K | SSA | MVO | DA | FPA | PSO | SCA | ALO | MALO |
Cactus | 4 | 18.5843 | 18.5843 | 18.5843 | 18.3930 | 18.5829 | 18.4259 | 18.5832 | 18.5843 |
6 | 23.8329 | 23.8233 | 23.8386 | 23.2412 | 23.8389 | 23.2857 | 23.8364 | 23.8420 | |
8 | 28.4940 | 28.3247 | 28.5120 | 27.0680 | 28.4911 | 27.3938 | 28.4814 | 28.5198 | |
10 | 32.6224 | 32.0489 | 32.7875 | 30.4517 | 32.8282 | 30.7148 | 32.7883 | 32.8463 | |
12 | 36.7255 | 35.3654 | 36.6688 | 34.7456 | 36.7983 | 34.2975 | 36.6721 | 36.8450 | |
Kangaroo | 4 | 18.9363 | 18.9353 | 18.9361 | 18.6549 | 18.9352 | 18.7771 | 18.9350 | 18.9363 |
6 | 24.4683 | 24.4414 | 24.4720 | 23.6425 | 24.4648 | 24.0457 | 24.4563 | 24.4753 | |
8 | 29.3790 | 29.2133 | 29.3699 | 28.7933 | 29.4014 | 28.2712 | 29.4026 | 29.4202 | |
10 | 33.8064 | 33.4514 | 33.8602 | 32.6267 | 33.1534 | 32.0603 | 33.8653 | 33.9054 | |
12 | 37.8707 | 37.1153 | 37.9876 | 36.2125 | 38.0001 | 35.4540 | 37.9065 | 38.0410 | |
Temple | 4 | 17.8159 | 17.8201 | 17.8061 | 17.4725 | 17.8046 | 17.5013 | 17.8195 | 17.8204 |
6 | 22.9388 | 22.7544 | 22.9388 | 22.2007 | 22.8940 | 22.0680 | 22.9246 | 22.9388 | |
8 | 27.5032 | 27.2493 | 27.4436 | 25.9640 | 27.4909 | 25.5465 | 27.5208 | 27.5351 | |
10 | 31.6211 | 30.6718 | 31.6425 | 29.5279 | 31.6160 | 28.9053 | 31.6706 | 31.7574 | |
12 | 34.2789 | 33.0925 | 35.3472 | 31.7622 | 34.7704 | 32.2631 | 35.3818 | 35.6116 | |
Flower | 4 | 18.7000 | 18.6993 | 18.7000 | 18.4447 | 18.6996 | 18.4765 | 18.6980 | 18.7005 |
6 | 24.0481 | 24.0186 | 24.0485 | 23.6039 | 24.0429 | 23.3247 | 24.0441 | 24.0511 | |
8 | 28.7647 | 28.6438 | 28.7955 | 27.6104 | 28.7721 | 27.7280 | 28.7727 | 28.8057 | |
10 | 33.0270 | 32.9200 | 33.1672 | 31.2963 | 33.1582 | 31.3512 | 33.1673 | 33.1976 | |
12 | 36.8699 | 35.9637 | 37.1214 | 34.8362 | 37.0372 | 34.9773 | 36.9291 | 37.1549 | |
Mountain | 4 | 17.7231 | 17.7035 | 17.7213 | 17.5421 | 17.7372 | 17.4362 | 17.7390 | 17.7213 |
6 | 23.1283 | 23.0802 | 23.0902 | 22.5402 | 23.1001 | 22.4117 | 23.1173 | 23.1119 | |
8 | 27.9637 | 27.6405 | 27.9315 | 26.6905 | 27.9354 | 26.4147 | 27.9302 | 27.9679 | |
10 | 32.1404 | 31.9221 | 32.3451 | 30.9813 | 32.2561 | 30.0080 | 32.3415 | 32.4426 | |
12 | 36.3399 | 34.9350 | 36.3823 | 34.0005 | 35.6920 | 33.6967 | 36.1384 | 36.4707 | |
Tree | 4 | 18.9965 | 18.9962 | 18.9965 | 18.8465 | 18.9961 | 18.8254 | 18.9953 | 18.9965 |
6 | 24.3933 | 24.4095 | 24.4100 | 23.8731 | 24.3889 | 23.4245 | 24.4083 | 24.4149 | |
8 | 29.2846 | 28.9004 | 29.2918 | 27.8265 | 29.2753 | 27.6207 | 29.2581 | 29.2936 | |
10 | 33.5397 | 33.0809 | 33.6613 | 31.4879 | 33.6439 | 31.7828 | 33.5491 | 33.6785 | |
12 | 37.3776 | 36.7041 | 37.4570 | 35.2858 | 37.5764 | 34.8717 | 37.5111 | 37.6312 | |
Horse | 4 | 18.6614 | 18.6259 | 18.6230 | 18.3914 | 18.6252 | 18.5251 | 18.6614 | 18.6619 |
6 | 24.0609 | 23.8743 | 24.0802 | 23.2431 | 24.0604 | 22.8699 | 24.0675 | 24.0806 | |
8 | 28.8403 | 28.5613 | 28.8907 | 27.9542 | 28.8416 | 27.6730 | 28.8914 | 28.8992 | |
10 | 33.2142 | 32.3696 | 33.2125 | 31.1950 | 33.2741 | 30.7256 | 33.2480 | 33.3135 | |
12 | 36.8467 | 35.6548 | 37.0718 | 34.9857 | 37.0695 | 33.8890 | 37.1782 | 37.3216 | |
Bridge | 4 | 18.1906 | 18.1436 | 18.1902 | 18.0033 | 18.1903 | 18.0580 | 18.1903 | 18.1907 |
6 | 23.5631 | 23.5510 | 23.5679 | 22.8880 | 23.5610 | 22.8246 | 23.5649 | 23.5682 | |
8 | 28.2604 | 27.9986 | 28.2930 | 27.0830 | 28.2859 | 26.7516 | 28.2786 | 28.3023 | |
10 | 32.5876 | 32.3366 | 32.5480 | 30.9047 | 32.5740 | 30.5593 | 32.5281 | 32.6189 | |
12 | 36.0703 | 35.4806 | 36.4991 | 33.5753 | 36.4588 | 33.0662 | 36.4400 | 36.5351 | |
Pilot | 4 | 17.9368 | 17.9352 | 17.9362 | 17.6027 | 17.9362 | 17.7058 | 17.9355 | 17.9168 |
6 | 23.0182 | 22.9648 | 23.0443 | 21.9322 | 23.0365 | 21.8927 | 23.0025 | 23.0447 | |
8 | 27.6539 | 27.4187 | 27.6678 | 26.2966 | 27.6452 | 25.7373 | 27.6307 | 27.6723 | |
10 | 31.6001 | 30.3305 | 31.7457 | 30.5445 | 31.7701 | 29.3977 | 31.7568 | 31.8639 | |
12 | 35.3121 | 34.3457 | 35.4310 | 32.7740 | 35.6276 | 31.7852 | 35.5945 | 35.7388 | |
Dog | 4 | 18.5258 | 18.5246 | 18.4850 | 18.2690 | 18.5254 | 18.2825 | 18.5244 | 18.5261 |
6 | 23.6922 | 23.6586 | 23.7386 | 22.7559 | 23.7121 | 22.7675 | 23.7319 | 23.7420 | |
8 | 28.3767 | 28.0194 | 28.3553 | 27.0852 | 28.3862 | 26.7027 | 28.3751 | 28.4079 | |
10 | 32.4951 | 31.5916 | 32.6709 | 31.2171 | 32.6231 | 29.8432 | 32.6604 | 32.6944 | |
12 | 36.4563 | 36.2405 | 36.3900 | 34.1968 | 35.8826 | 33.0948 | 36.5074 | 36.6079 |
In order to make the experimental data more intuitive, the following experiments are conducted. The relevant convergence curve and boxplot are drawn. As shown in Figure A1 and A2, the evolution curve reflects the convergence speed and the accuracy of the algorithm, thus reflecting the overall performance of the algorithm. It can be seen from the convergence curve that the MALO algorithm can obtain the approximate optimal solution, which further improves the overall performance. For Table A2(d), (h), MALO algorithm and DA algorithm eventually approach the same function value. But for the rest of graphs, it is clear that the MALO algorithm is superior to other algorithms in the optimization process. MALO algorithm can reach the maximum fitness value at nearly the 100th iteration, which is earlier than other algorithms. This phenomenon indicates that the convergence rate of algorithm is improved. On the whole, it also proves that by introducing the OBL strategy, the stability of the algorithm is enhanced. As shown in Figures 4 and 5, the boxplot can effectively reflect the stability of the algorithm. Taking the two images of cactus and kangaroo as examples, it can be seen that the boxplot of MALO algorithm has the most flat shape, the highest position, and no bad points. This shows that the MALO algorithm has the highest stability compared with other algorithms by analysis of the 30 fitness values obtained.
Tables A2 and A3 show PSNR values of Otsu and Kapur's entropy respectively. When the PSNR value is larger, the distortion of the segmented image is smaller. Through the data, it can be seen vertically that the PSNR value of high threshold is larger than that of low threshold. We see horizontally that the PSNR value of MALO algorithm is larger than other algorithms in most cases, especially at high threshold level. Tables A4 and A5 show SSIM values of Otsu and Kapur's entropy, respectively. Tables A6 and A7 show FSIM values of Otsu and Kapur's entropy, respectively. When the values of SSIM value and FSIM value are closer to 1, the images before and after segmentation are more similar and the segmentation effect is better. Comparing SSIM value and FSIM value, MALO algorithm is larger and closer to 1 than other algorithms. The maximum value is marked in bold. Most of the evaluation metrics obtained by MALO algorithm are optimal. The modified algorithm makes the image segmentation effect better.
Tables 5 and 6 show the average computational time (in second) of each algorithm using Otsu and Kapur's entropy, respectively. MALO enhanced the native ALO in many aspects, such as fitness value, PSNR, SSIM, and FSIM. However, it can be found from Tables 5 and 6 that MALO has the relatively high time consumption compared to the other algorithms. In fact, the high consumption is mainly caused by the high computational cost of the native ALO. The proposed improvement results in increasing the computational time of MALO as well. In short, in order to improve the overall performance of algorithm, it cannot guarantee to obtain optimal parameters in all cases.
Image | K | SSA | MVO | DA | FPA | PSO | SCA | ALO | MALO |
Cactus | 4 | 0.4100 | 0.8700 | 1.8700 | 0.6900 | 0.7700 | 0.7100 | 1.4050 | 1.7200 |
6 | 0.2500 | 0.7000 | 1.4900 | 0.6800 | 0.6700 | 0.6500 | 1.8700 | 2.1455 | |
8 | 0.2810 | 0.7800 | 1.5200 | 0.6800 | 0.7200 | 0.6800 | 2.3850 | 2.6000 | |
10 | 0.3200 | 0.7800 | 1.5600 | 0.7300 | 0.7600 | 0.7100 | 2.9545 | 3.2550 | |
12 | 0.3400 | 1.0600 | 1.6890 | 0.8630 | 0.9000 | 0.8400 | 4.8515 | 4.3270 | |
Kangaroo | 4 | 0.5050 | 0.8100 | 1.8420 | 0.6700 | 0.7300 | 0.9580 | 1.4200 | 1.5505 |
6 | 0.2690 | 0.7300 | 1.5200 | 0.6800 | 0.7600 | 0.6500 | 1.9600 | 2.1995 | |
8 | 0.3100 | 0.8300 | 1.6410 | 0.7010 | 0.7500 | 0.7400 | 2.4345 | 2.7155 | |
10 | 0.3400 | 0.8500 | 1.6000 | 0.7800 | 0.8200 | 0.7400 | 3.0750 | 3.3250 | |
12 | 0.3700 | 0.8400 | 1.6000 | 0.7800 | 0.8300 | 0.7900 | 3.7580 | 3.8305 | |
Temple | 4 | 0.3700 | 0.8540 | 1.9250 | 0.6530 | 1.3210 | 0.6510 | 1.4300 | 1.5605 |
6 | 0.2700 | 0.7600 | 1.5690 | 0.6500 | 0.7390 | 0.6510 | 1.9200 | 2.1755 | |
8 | 0.3000 | 0.8100 | 1.5800 | 0.7400 | 0.7600 | 0.6890 | 3.0805 | 3.5605 | |
10 | 0.4400 | 1.3800 | 2.3500 | 1.0690 | 0.7600 | 0.8100 | 3.7715 | 4.9285 | |
12 | 0.3600 | 0.8600 | 1.7200 | 0.7710 | 0.8000 | 0.7300 | 3.5765 | 4.2245 | |
Flower | 4 | 0.4250 | 1.1510 | 2.8120 | 0.8400 | 0.8710 | 0.8550 | 2.1855 | 2.2690 |
6 | 0.2700 | 0.7900 | 1.6000 | 0.7200 | 0.7700 | 0.7400 | 2.0005 | 3.2575 | |
8 | 0.3860 | 0.9860 | 2.5490 | 1.5090 | 0.8630 | 0.7330 | 4.1070 | 4.4885 | |
10 | 0.8540 | 1.6520 | 2.4080 | 0.9010 | 1.3190 | 1.5640 | 4.8340 | 4.6935 | |
12 | 0.5600 | 1.1700 | 2.1900 | 1.1300 | 0.8700 | 1.0000 | 3.7055 | 4.0505 | |
Mountain | 4 | 0.4700 | 1.1750 | 2.8030 | 0.7110 | 0.9170 | 0.8500 | 2.2840 | 2.2155 |
6 | 0.5170 | 0.9080 | 2.4520 | 0.7370 | 0.8370 | 0.7510 | 2.5625 | 2.1245 | |
8 | 0.2920 | 0.8200 | 1.7150 | 0.6800 | 0.9710 | 0.8810 | 3.3625 | 2.8755 | |
10 | 0.4300 | 0.8200 | 3.4100 | 0.7100 | 0.8010 | 0.8800 | 5.0305 | 4.2160 | |
12 | 0.4000 | 0.8820 | 1.5710 | 0.7900 | 1.1500 | 0.8550 | 4.4010 | 3.9205 | |
Tree | 4 | 0.4500 | 0.9200 | 1.9700 | 0.9800 | 1.5640 | 0.7900 | 1.6595 | 2.3955 |
6 | 0.4300 | 1.0950 | 2.6590 | 1.7460 | 1.0490 | 1.6700 | 3.1700 | 3.5230 | |
8 | 0.3410 | 1.4480 | 2.8000 | 0.9750 | 0.7530 | 0.6930 | 2.6675 | 3.7390 | |
10 | 0.3740 | 1.2760 | 2.3920 | 0.8310 | 0.8920 | 0.8130 | 3.6270 | 3.5155 | |
12 | 0.4500 | 0.9390 | 0.9390 | 0.9390 | 0.8990 | 0.8510 | 3.9570 | 5.2985 | |
Horse | 4 | 0.4040 | 0.8970 | 1.8600 | 0.6840 | 0.7480 | 0.9190 | 1.7475 | 1.8105 |
6 | 0.3140 | 0.8380 | 1.6460 | 0.8150 | 0.7450 | 0.6850 | 2.0365 | 2.2195 | |
8 | 0.3010 | 1.0490 | 1.8420 | 0.7300 | 0.8770 | 0.7740 | 3.2820 | 2.9730 | |
10 | 0.3300 | 0.8560 | 1.6410 | 0.7780 | 0.8540 | 0.7920 | 3.7315 | 3.8360 | |
12 | 0.3920 | 0.9120 | 1.7110 | 1.6600 | 1.6600 | 1.0670 | 4.2670 | 4.8615 | |
Bridge | 4 | 0.4200 | 1.9550 | 2.5860 | 1.7110 | 0.9140 | 0.7110 | 1.6845 | 1.8555 |
6 | 0.2660 | 0.8010 | 1.5530 | 0.7070 | 1.3360 | 1.0980 | 2.8115 | 3.0780 | |
8 | 0.3210 | 0.9610 | 2.7830 | 1.0120 | 0.9970 | 0.7930 | 4.5400 | 4.8560 | |
10 | 0.4110 | 1.5550 | 2.6160 | 1.0420 | 1.6320 | 0.9770 | 5.2685 | 5.8515 | |
12 | 0.5330 | 1.2210 | 2.9890 | 1.1920 | 1.9340 | 1.1170 | 4.6090 | 4.1150 | |
Pilot | 4 | 0.4400 | 0.9200 | 2.3630 | 0.7820 | 1.0780 | 0.8350 | 1.8890 | 1.8405 |
6 | 0.2810 | 1.6590 | 2.2270 | 0.7510 | 1.3550 | 0.8610 | 3.4105 | 2.8835 | |
8 | 0.4400 | 1.5610 | 2.2130 | 0.8350 | 1.0880 | 1.2600 | 3.6285 | 3.9075 | |
10 | 0.3700 | 1.0850 | 2.7580 | 1.2330 | 1.2590 | 1.3200 | 4.9585 | 5.6945 | |
12 | 0.3740 | 0.9200 | 1.7840 | 1.2990 | 1.1100 | 1.5060 | 5.2780 | 4.0805 | |
Dog | 4 | 0.4500 | 1.0160 | 2.4440 | 0.8390 | 0.8300 | 1.3690 | 1.7170 | 2.5915 |
6 | 0.2750 | 1.3830 | 2.4210 | 1.2500 | 0.8100 | 1.3300 | 3.1915 | 3.4480 | |
8 | 0.4700 | 0.9450 | 1.5800 | 0.7400 | 0.7400 | 0.7510 | 2.4325 | 2.8155 | |
10 | 0.3490 | 0.8300 | 1.5390 | 0.7190 | 0.8010 | 0.7220 | 3.0855 | 3.5100 | |
12 | 0.3300 | 0.8720 | 1.5700 | 0.7600 | 0.8700 | 0.7800 | 3.7950 | 4.3600 |
Image | K | SSA | MVO | DA | FPA | PSO | SCA | ALO | MALO |
Cactus | 4 | 0.6400 | 1.2900 | 1.9500 | 0.8000 | 1.6930 | 0.7800 | 1.5150 | 1.8455 |
6 | 0.4700 | 0.8500 | 1.5890 | 0.8210 | 0.8910 | 0.7900 | 2.0750 | 2.3905 | |
8 | 0.4900 | 0.9500 | 1.6810 | 0.8110 | 0.8900 | 0.8080 | 2.4650 | 4.2290 | |
10 | 0.6230 | 1.2750 | 2.0900 | 1.0400 | 0.9900 | 0.8490 | 3.1220 | 3.6495 | |
12 | 0.5380 | 1.0030 | 1.7180 | 0.9570 | 1.0110 | 0.8900 | 3.6600 | 4.1195 | |
Kangaroo | 4 | 0.5800 | 0.9600 | 1.8620 | 0.8300 | 0.8900 | 1.4690 | 2.2225 | 2.3925 |
6 | 0.9260 | 1.2200 | 2.6770 | 1.4180 | 0.9600 | 1.0310 | 3.2235 | 2.9170 | |
8 | 0.4800 | 0.8900 | 1.6200 | 0.8200 | 0.9000 | 1.1690 | 2.5200 | 3.1900 | |
10 | 0.5210 | 0.9200 | 1.6400 | 0.8900 | 0.8700 | 0.8400 | 3.0550 | 3.6250 | |
12 | 0.5200 | 0.9900 | 1.7500 | 0.8800 | 0.9400 | 0.8900 | 3.6400 | 4.1070 | |
Temple | 4 | 0.6100 | 1.6350 | 2.5860 | 1.2700 | 1.2480 | 0.9400 | 2.4355 | 2.8155 |
6 | 0.4700 | 1.0400 | 2.3770 | 1.0500 | 1.2900 | 0.7900 | 2.0400 | 2.4100 | |
8 | 0.4700 | 0.9100 | 1.6800 | 0.8000 | 0.8900 | 0.8000 | 2.4900 | 2.9895 | |
10 | 0.5200 | 0.9300 | 1.6500 | 0.8600 | 1.0000 | 0.8400 | 3.1050 | 3.6095 | |
12 | 0.5700 | 1.0000 | 1.8200 | 0.9300 | 0.9100 | 0.8700 | 5.4985 | 4.5950 | |
Flower | 4 | 0.6000 | 1.4130 | 2.6960 | 1.0000 | 1.2850 | 1.3000 | 2.3625 | 2.8995 |
6 | 1.0690 | 0.9900 | 2.8420 | 0.9500 | 0.9390 | 0.9860 | 2.6195 | 2.7010 | |
8 | 0.4800 | 0.9900 | 1.7200 | 0.9400 | 0.9200 | 0.8700 | 2.6020 | 3.0850 | |
10 | 0.5300 | 0.9700 | 1.8010 | 0.9100 | 0.9990 | 0.9600 | 3.2255 | 3.6850 | |
12 | 0.6100 | 1.0700 | 1.8100 | 0.9400 | 1.0000 | 1.3790 | 5.1945 | 4.5065 | |
Mountain | 4 | 0.5600 | 1.5490 | 1.9920 | 0.8570 | 0.8810 | 0.8310 | 1.5315 | 1.9575 |
6 | 0.4410 | 0.9230 | 1.7220 | 0.9020 | 0.8820 | 0.8800 | 2.0330 | 2.4990 | |
8 | 0.5100 | 0.9710 | 1.7300 | 0.9000 | 0.9520 | 0.8420 | 2.5850 | 3.1700 | |
10 | 0.5480 | 1.0300 | 1.7900 | 0.9040 | 0.9510 | 0.9000 | 5.1080 | 4.0555 | |
12 | 1.0660 | 1.0420 | 1.9110 | 0.9610 | 1.0690 | 0.9580 | 3.9095 | 4.3645 | |
Tree | 4 | 0.6200 | 1.3020 | 2.8450 | 1.0270 | 1.2090 | 1.3510 | 2.2510 | 2.7790 |
6 | 0.5000 | 1.2040 | 2.6420 | 1.3380 | 1.1410 | 0.8990 | 2.1775 | 3.1785 | |
8 | 0.5110 | 0.8790 | 1.6500 | 0.8300 | 0.8800 | 0.8600 | 2.4650 | 2.9350 | |
10 | 0.5000 | 0.9300 | 1.6800 | 0.9190 | 0.8500 | 0.9000 | 3.0445 | 3.5950 | |
12 | 0.6700 | 1.0090 | 1.8600 | 0.9100 | 0.9400 | 0.9400 | 3.7930 | 4.1300 | |
Horse | 4 | 0.6100 | 1.5700 | 3.2080 | 1.5370 | 1.6130 | 0.8400 | 2.5665 | 2.8115 |
6 | 1.0800 | 1.2290 | 2.5900 | 0.8000 | 0.8100 | 0.9800 | 2.0500 | 2.4145 | |
8 | 0.4600 | 0.8900 | 1.6000 | 0.8300 | 0.8600 | 0.8900 | 2.6550 | 3.2905 | |
10 | 0.5000 | 0.9900 | 1.6400 | 1.1500 | 0.8900 | 0.8500 | 3.0550 | 3.6400 | |
12 | 0.5400 | 1.9330 | 2.0100 | 0.9800 | 1.0100 | 1.0000 | 3.7850 | 4.4105 | |
Bridge | 4 | 0.6000 | 0.9500 | 1.7600 | 0.7600 | 0.8100 | 0.7300 | 1.4750 | 1.7950 |
6 | 0.4100 | 0.8400 | 1.5700 | 0.8200 | 0.8400 | 0.8000 | 1.9300 | 2.3950 | |
8 | 0.4400 | 0.8600 | 1.5900 | 0.8700 | 0.8600 | 0.7900 | 2.4900 | 2.9775 | |
10 | 0.4700 | 0.9100 | 1.6400 | 0.7900 | 0.9100 | 1.2500 | 3.7975 | 3.9840 | |
12 | 0.5230 | 1.0150 | 2.1800 | 0.8710 | 0.9340 | 0.9500 | 3.7080 | 4.3340 | |
Pilot | 4 | 0.5400 | 0.9400 | 1.7300 | 0.7500 | 1.0100 | 0.7700 | 1.4750 | 1.7600 |
6 | 0.3900 | 0.8100 | 1.5500 | 0.8300 | 0.8700 | 0.8800 | 2.0940 | 2.5195 | |
8 | 0.4600 | 0.8400 | 1.8120 | 0.7760 | 0.9500 | 0.8600 | 2.6975 | 3.1400 | |
10 | 0.5650 | 1.1600 | 2.2950 | 0.9460 | 0.9790 | 0.9690 | 3.5840 | 4.1245 | |
12 | 0.5800 | 1.3060 | 2.3550 | 1.2900 | 1.0490 | 1.3350 | 5.6445 | 8.5025 | |
Dog | 4 | 0.6600 | 1.2350 | 3.0700 | 1.0100 | 1.0550 | 1.6800 | 2.5700 | 3.0600 |
6 | 0.5050 | 1.0870 | 2.1600 | 0.9200 | 0.9200 | 0.8500 | 2.2025 | 2.8425 | |
8 | 0.5050 | 1.2550 | 2.9380 | 1.0800 | 1.8400 | 1.4900 | 4.5565 | 5.3650 | |
10 | 0.5920 | 1.4200 | 2.2400 | 0.9200 | 1.2000 | 0.9250 | 3.4000 | 3.9405 | |
12 | 0.5790 | 1.0750 | 1.8410 | 0.9500 | 0.9990 | 0.9140 | 3.8400 | 4.5330 |
Table A8 denotes the PSNR, SSIM and FSIM values obtained by MALO using the Otsu and Kapur's entropy. By comparison, it can be found that the average value of the evaluation metrics obtained by using the Otsu method is higher. For selected 10 images, using the Otsu method is just recommended. Wolpert and Macerday put forward the No Free Lunch (NFL) theorem [60]. Of course, Otsu and Kapur's entropy methods have different theoretical foundations, so the types of images they process also have different focuses.
Tables A9 and A10 give the optimal thresholds of MALO under Otsu and Kapur's entropy at 4, 6, 8, 10, and 12 levels. Taking the "Tree" image an examples, the segmented results based on different algorithms using Otsu at 4, 6, 8, 10, and 12 levels are presented in Figure 6. Taking the "Horse" image an examples, the segmented results based on different algorithms using Kapur's entropy at 4, 6, 8, 10, and 12 levels are presented in Figure 7. Different target regions correspond to different threshold ranges. Considering the segmented images presented in Figures 6 and 7, it can be found that the images with high levels contain more information and details than that with low levels. Furthermore, the MALO based method has achieved the desired goal, because the main target areas have been efficiently identified. To sum up, the proposed method is competent for most cases and can still be considered as a competitive technique for the multilevel thresholding color image segmentation.
In this subsection, the proposed method is further compared with some improved algorithms, including MABC, IDSA, WOA_TH, and BDE. PSNR and FSIM are utilized for analysis.
For visual analysis, the PSNR values obtained are represented as a line graph in Figures 8 and 9. From the figures we can observe that the MALO based method gives the higher values in general, which indicates that the segmented image is similar to the original image. For example, in the circumstance of "Flower" image through Otsu technique (for K = 12), the PSNR values are 29.5819, 29.4498, 29.3192, 29.6828, and 30.7280 for MABC, IDSA, WOA_TH, BDE, and PSO, respectively. It also can be seen that all algorithms give the similar results when the number of thresholds is small (such as K = 4). Whereas, when the number of thresholds increase, the difference between algorithms becomes greater and the MALO based method outperforms the others. On comparing the FSIM values, which are given in Figures 10 and 11, it can be observed that the values increase as the number of the thresholds increase. In the circumstance of "Temple", "Flower", "Mountain", "Tree", "Bridge", "Pilot", and "Dog" images through Otsu technique (for K = 12), the proposed algorithm is not the best. However, the proposed method gives the highest values on most cases through Kapur's entropy (for K = 12). These results indicate the precise search ability of MALO based method, which is suitable for color image segmentation.
In this paper, an ant lion optimizer algorithm based on opposition-based learning for multilevel thresholding color image segmentation is proposed. Among many thresholding segmentation methods, Otsu and Kapur's entropy are adopted. The proposed algorithm is used to find the optimal threshold for 10 color test images. IEEE CEC2017 benchmark functions are performed to verify the performance of the proposed algorithm. Furthermore, 7 traditional algorithms and 4 improved algorithms are selected for comparisons. The fitness value, PSNR, SSIM, FSIM, and computational time are used to evaluate the quality of segmentation. By the convergence curve and boxplot at K = 12, it can be seen that MALO algorithm can find larger objective function value more times at nearly the 100th iteration. In terms of PSNR, SSIM, and FSIM, the value obtained by the MALO algorithm is larger than other algorithms in most cases. It concludes that the segmentation performance based on MALO algorithm is superior. To sum up, a variety of experiments fully proves that MALO algorithm has higher search accuracy and convergence speed. However, high time consumption might be considered a principle limitation of this method.
In the future, the relevant research directions are given as follows:
(1) Explore to introduce other strategies and hybrid other algorithms in improving the performance of the ALO algorithm.
(2) Extend the algorithm to multi-objective problem for obtaining superior segmentation effect.
This work was supported by the Harbin Normal University doctoral research initiation fund project (XKB202014), Sanming University introduces high-level talents to start scientific research funding support project (20YG14), Guiding science and technology projects in Sanming City (2020-G-61), Educational research projects of young and middle-aged teachers in Fujian Province (JAT200618), Scientific research and development fund of Sanming University (B202009).
All authors declare no conflicts of interest in this paper.
Provenance | Symbols | Paraphrase |
Color images | L | The gray value |
ni | The number of pixels with gray value of i | |
N | The total number of pixels | |
p | The distribution probability of gray value | |
K | The total number of threshold | |
t | The threshold | |
C | The class | |
R, G, B | The three channels of color images | |
Otsu | ω | The probabilities of class occurrence |
μ | The levels of class | |
σ2 | The total variance | |
Kapur's entropy | ψ | The total entropy |
ALO | X | The array of random walk |
ub | The upper bound of parameter | |
lb | The lower bound of parameter | |
t | The current iteration | |
T | The maximum of iterations | |
I | The ratio of boundary contraction | |
Time complexity | O | The complexity notation |
PSNR | MSE | The mean square error |
SSIM | R | The original image |
I | The segmented image | |
μR,μI | The average gray values of image | |
σ2R,σ2I | The variance of image | |
σRI | The covariance of image | |
FSIM | PC | Phase congruency |
GM | Gradient magnitude | |
Ω | The entire domain of image | |
SL(x) | The similarity value of each position x | |
PCm(x) | The phase consistency measure | |
SPC(x) | The similarity measure of phase consistency | |
SG(x) | The similarity measure of gradient magnitude |
IMAGE | K | SSA | MVO | DA | FPA | PSO | SCA | ALO | MALO |
Cactus | 4 | 19.1730 | 19.1730 | 19.1730 | 18.7263 | 19.1730 | 18.8974 | 19.1344 | 19.1901 |
6 | 21.4834 | 21.4806 | 21.4819 | 20.6216 | 21.0808 | 20.2685 | 21.4733 | 21.4849 | |
8 | 22.9129 | 23.0452 | 22.5947 | 21.8482 | 22.5738 | 23.0573 | 22.9754 | 23.7403 | |
10 | 23.8232 | 24.1443 | 23.9252 | 23.1394 | 23.9100 | 23.8865 | 24.0865 | 24.1499 | |
12 | 24.6895 | 25.1475 | 24.8778 | 24.9636 | 24.5394 | 25.1646 | 25.0007 | 25.2868 | |
Kangaroo | 4 | 22.0643 | 22.0643 | 22.0643 | 20.7955 | 22.0643 | 20.4905 | 22.0429 | 22.0797 |
6 | 24.8251 | 24.8350 | 24.5043 | 23.2999 | 24.4744 | 23.1015 | 24.8199 | 24.8836 | |
8 | 26.8199 | 27.0457 | 26.8403 | 24.3867 | 26.3434 | 24.2914 | 26.9047 | 27.0860 | |
10 | 28.1619 | 28.8131 | 28.5707 | 25.1187 | 28.1677 | 25.2235 | 28.4114 | 28.8186 | |
12 | 29.1767 | 30.0207 | 29.8385 | 27.1879 | 29.8151 | 26.9052 | 30.1672 | 30.3411 | |
Temple | 4 | 17.4713 | 17.4707 | 17.4713 | 19.7885 | 17.4736 | 17.1516 | 17.4005 | 17.6125 |
6 | 20.7143 | 19.8002 | 20.7137 | 20.7876 | 19.7566 | 18.2250 | 20.7661 | 21.4589 | |
8 | 22.0492 | 23.5836 | 22.5695 | 20.7664 | 22.5250 | 23.8550 | 23.4862 | 23.9977 | |
10 | 23.2619 | 25.2421 | 24.2747 | 23.4468 | 23.3367 | 22.7675 | 24.8846 | 25.7059 | |
12 | 26.8631 | 26.8151 | 25.5113 | 24.5639 | 24.7817 | 26.2330 | 26.4459 | 27.5232 | |
Flower | 4 | 22.6832 | 22.6832 | 22.6832 | 21.9099 | 22.6832 | 22.2661 | 22.6832 | 22.6916 |
6 | 25.1802 | 25.5484 | 25.5446 | 23.9499 | 25.2590 | 24.5962 | 25.5240 | 25.5506 | |
8 | 27.5586 | 27.3846 | 27.6921 | 25.3172 | 27.3576 | 26.1643 | 27.5337 | 27.6960 | |
10 | 28.6654 | 28.8544 | 29.0249 | 26.6791 | 28.8757 | 26.7323 | 29.1768 | 29.2169 | |
12 | 29.9882 | 30.1775 | 29.8010 | 28.1115 | 29.6455 | 27.8780 | 30.6120 | 30.7280 | |
Mountain | 4 | 19.7307 | 19.7307 | 19.7307 | 19.1368 | 19.7307 | 19.2754 | 19.7277 | 19.7307 |
6 | 21.8240 | 22.4882 | 22.3866 | 20.9344 | 22.1794 | 21.8654 | 22.3347 | 22.5039 | |
8 | 24.8724 | 24.1574 | 24.2907 | 22.8823 | 24.0400 | 23.4094 | 24.7870 | 24.9053 | |
10 | 25.9744 | 26.1368 | 26.1295 | 24.6068 | 25.8506 | 23.8109 | 26.0493 | 26.3373 | |
12 | 27.3911 | 26.9209 | 26.9014 | 25.3515 | 26.9991 | 25.7124 | 26.8751 | 27.4991 | |
Tree | 4 | 19.9284 | 19.9265 | 19.9284 | 19.3506 | 19.9206 | 19.0453 | 19.9284 | 19.9364 |
6 | 22.9219 | 22.9184 | 22.9126 | 21.8322 | 22.4339 | 22.4819 | 22.9276 | 22.9388 | |
8 | 25.1296 | 25.0004 | 25.0881 | 22.6562 | 24.4342 | 22.7555 | 25.1489 | 25.1704 | |
10 | 26.7206 | 26.6709 | 26.6389 | 24.1790 | 26.1891 | 24.3475 | 26.5608 | 26.7213 | |
12 | 27.5331 | 28.0382 | 27.7147 | 26.1871 | 27.2252 | 24.9152 | 27.8812 | 28.0724 | |
Horse | 4 | 19.1095 | 19.1095 | 19.1543 | 18.4918 | 19.1095 | 17.8797 | 19.1095 | 19.1569 |
6 | 22.6175 | 22.6343 | 22.6083 | 22.4411 | 22.6441 | 20.0880 | 22.5318 | 22.8301 | |
8 | 24.8257 | 24.6203 | 24.7383 | 23.2636 | 23.7326 | 22.2812 | 24.5913 | 24.8543 | |
10 | 26.7272 | 26.7701 | 26.3299 | 23.8301 | 26.2501 | 23.7805 | 26.4699 | 26.8845 | |
12 | 28.0067 | 27.6999 | 28.1864 | 25.0419 | 28.0623 | 24.4077 | 27.5665 | 28.3129 | |
Bridge | 4 | 18.7684 | 18.7684 | 18.7684 | 18.1883 | 18.7684 | 18.3216 | 18.7562 | 18.7684 |
6 | 21.0341 | 21.5192 | 21.5142 | 20.6458 | 21.5142 | 19.4138 | 21.0529 | 21.5346 | |
8 | 23.5582 | 22.9642 | 23.4355 | 21.6761 | 23.3575 | 20.6855 | 23.3521 | 23.7010 | |
10 | 25.0709 | 24.8332 | 25.2117 | 22.7421 | 24.8889 | 23.3572 | 25.3971 | 25.4265 | |
12 | 26.3616 | 26.8858 | 26.3203 | 25.5030 | 26.4849 | 25.2994 | 26.1879 | 27.0756 | |
Pilot | 4 | 16.9918 | 16.9918 | 16.9918 | 16.9571 | 16.9915 | 16.9918 | 17.0028 | 17.6971 |
6 | 19.4806 | 19.6603 | 19.3935 | 18.8999 | 19.4660 | 19.4742 | 19.4398 | 21.5230 | |
8 | 22.1137 | 21.7970 | 21.8114 | 19.8872 | 20.9936 | 20.1921 | 21.9259 | 22.4562 | |
10 | 23.3937 | 23.4926 | 22.5532 | 23.4677 | 22.5761 | 22.8645 | 23.6086 | 24.1912 | |
12 | 24.6032 | 24.8457 | 25.4417 | 23.3403 | 23.4735 | 24.3050 | 25.6425 | 25.8213 | |
Dog | 4 | 19.9346 | 19.9569 | 19.9346 | 20.2544 | 19.9346 | 18.6387 | 19.9734 | 19.9346 |
6 | 22.5690 | 22.6725 | 22.3348 | 21.6363 | 21.7981 | 20.9528 | 22.5891 | 22.6726 | |
8 | 24.3105 | 24.3265 | 24.3176 | 23.0185 | 22.9836 | 22.5716 | 24.3533 | 24.3548 | |
10 | 24.9193 | 26.0109 | 25.7454 | 24.4410 | 25.5648 | 23.1901 | 25.8904 | 26.4961 | |
12 | 26.7167 | 28.0094 | 26.3712 | 24.9053 | 26.4391 | 25.6498 | 27.6947 | 28.1328 |
IMAGE | K | SSA | MVO | DA | FPA | PSO | SCA | ALO | MALO |
Cactus | 4 | 17.1463 | 17.1849 | 17.1849 | 16.6326 | 17.1605 | 16.5963 | 17.1918 | 17.2250 |
6 | 18.7054 | 19.5152 | 18.8374 | 17.7723 | 18.9734 | 18.9692 | 18.9353 | 20.9242 | |
8 | 20.2196 | 21.8061 | 21.8129 | 20.2720 | 20.7186 | 20.1477 | 20.8937 | 23.1098 | |
10 | 21.6755 | 23.5236 | 23.5035 | 22.9740 | 23.3426 | 23.5817 | 23.6783 | 25.6436 | |
12 | 24.5328 | 25.7488 | 24.2717 | 25.8229 | 25.6387 | 22.2231 | 25.5971 | 26.7329 | |
Kangaroo | 4 | 18.7559 | 18.7559 | 18.7529 | 16.9273 | 18.7030 | 17.9415 | 18.7714 | 18.8067 |
6 | 21.2093 | 21.9397 | 21.7227 | 20.3904 | 21.8062 | 20.1191 | 22.1098 | 22.3708 | |
8 | 23.8181 | 24.5562 | 23.9062 | 20.5802 | 24.5744 | 22.1074 | 24.4808 | 24.6322 | |
10 | 25.1196 | 26.0125 | 25.7934 | 25.0967 | 25.7309 | 22.8701 | 25.9331 | 26.1974 | |
12 | 26.6277 | 27.4952 | 27.4380 | 24.5722 | 27.3277 | 24.0530 | 27.2209 | 28.0349 | |
Temple | 4 | 16.7418 | 18.6069 | 17.9079 | 17.7575 | 17.8108 | 16.4613 | 18.5579 | 18.6105 |
6 | 20.3140 | 20.4895 | 20.3140 | 20.7483 | 20.5577 | 21.6953 | 20.6648 | 23.1506 | |
8 | 24.9708 | 24.0553 | 23.2965 | 22.2220 | 24.5715 | 23.2979 | 25.0443 | 25.1273 | |
10 | 25.8405 | 25.8930 | 26.0409 | 23.4840 | 26.5620 | 21.8980 | 26.5010 | 26.7757 | |
12 | 27.0259 | 26.4958 | 27.5263 | 24.8266 | 27.3391 | 24.0635 | 27.5766 | 28.4027 | |
Flower | 4 | 21.4262 | 21.4353 | 21.4262 | 21.0771 | 21.4030 | 21.7291 | 21.3824 | 21.4046 |
6 | 23.9954 | 24.0070 | 24.0260 | 22.9845 | 23.9935 | 23.7137 | 24.0223 | 24.2923 | |
8 | 25.0019 | 25.3825 | 25.3936 | 24.4758 | 25.6350 | 25.5636 | 25.5517 | 26.3947 | |
10 | 26.1749 | 27.0175 | 26.8094 | 25.4329 | 26.9897 | 26.1739 | 26.8538 | 27.6016 | |
12 | 26.8429 | 28.2241 | 27.8388 | 25.9058 | 27.9865 | 27.9984 | 27.8198 | 29.2750 | |
Mountain | 4 | 15.7308 | 16.1695 | 16.1695 | 15.1291 | 14.6360 | 17.2397 | 14.6863 | 17.7501 |
6 | 19.1293 | 19.7275 | 19.3198 | 20.0548 | 19.8595 | 18.9344 | 19.2375 | 20.2658 | |
8 | 22.2621 | 22.4482 | 22.2780 | 20.3443 | 22.2996 | 20.2389 | 22.1739 | 23.7160 | |
10 | 20.8677 | 25.1887 | 23.4748 | 22.1128 | 24.6158 | 22.2042 | 24.5972 | 26.2667 | |
12 | 25.4993 | 26.3821 | 26.2452 | 24.7312 | 26.5979 | 22.1953 | 26.4684 | 26.6949 | |
Tree | 4 | 19.3878 | 19.4098 | 19.3878 | 19.4510 | 19.3660 | 19.0814 | 19.3485 | 19.3878 |
6 | 21.7283 | 21.9982 | 21.9410 | 20.7787 | 21.7496 | 20.5382 | 21.9919 | 22.0355 | |
8 | 23.5510 | 23.6198 | 23.4812 | 22.0054 | 23.5766 | 22.2770 | 23.5404 | 24.2661 | |
10 | 24.8234 | 25.5643 | 25.4307 | 23.3183 | 25.4128 | 22.1914 | 25.4025 | 25.7360 | |
12 | 26.1558 | 26.7973 | 26.3433 | 23.9995 | 26.5906 | 24.8922 | 26.5045 | 26.8461 | |
Horse | 4 | 18.9208 | 19.7557 | 19.0344 | 18.9208 | 19.6738 | 19.4317 | 19.5801 | 19.7788 |
6 | 21.2581 | 21.6962 | 21.6519 | 19.7265 | 21.3967 | 19.8505 | 21.6610 | 21.6974 | |
8 | 23.8699 | 23.6078 | 24.2314 | 22.0431 | 23.2680 | 21.4611 | 23.4581 | 24.2427 | |
10 | 25.5023 | 24.5116 | 25.5495 | 23.2916 | 25.8501 | 22.6324 | 26.0431 | 26.1268 | |
12 | 26.4346 | 25.3050 | 27.0205 | 24.1636 | 27.1689 | 24.0270 | 26.9024 | 27.4180 | |
Bridge | 4 | 17.7375 | 17.7101 | 17.7433 | 17.6562 | 17.7064 | 17.2277 | 17.7680 | 18.3564 |
6 | 21.0395 | 21.0300 | 21.0468 | 20.0621 | 21.0612 | 19.8616 | 21.0352 | 21.1004 | |
8 | 22.8402 | 23.4100 | 23.4820 | 21.0220 | 23.3430 | 21.3364 | 23.4706 | 23.6124 | |
10 | 24.6074 | 24.9201 | 24.1982 | 23.1209 | 24.7082 | 23.6599 | 24.6209 | 25.3739 | |
12 | 25.4895 | 26.0580 | 26.3086 | 23.1938 | 26.7399 | 24.1204 | 26.4986 | 27.4320 | |
Pilot | 4 | 15.9241 | 15.8597 | 15.9653 | 15.5965 | 15.8932 | 15.9792 | 15.9532 | 16.0238 |
6 | 19.9635 | 19.1615 | 20.4713 | 19.5345 | 20.6783 | 20.2829 | 19.8317 | 20.7123 | |
8 | 22.5976 | 22.0836 | 22.9972 | 22.6697 | 23.0674 | 22.7085 | 22.8982 | 23.1598 | |
10 | 24.5888 | 20.7618 | 24.7292 | 23.9268 | 24.7256 | 24.0374 | 24.5571 | 24.8228 | |
12 | 25.6096 | 24.6046 | 26.7532 | 24.9263 | 25.9880 | 25.6817 | 26.4241 | 27.1095 | |
Dog | 4 | 18.3708 | 18.4828 | 17.6137 | 16.0679 | 18.2476 | 18.2657 | 18.4844 | 18.2605 |
6 | 20.0477 | 20.7865 | 20.6360 | 19.9191 | 20.6773 | 18.4516 | 20.7274 | 21.9672 | |
8 | 22.3956 | 22.5129 | 22.5608 | 22.6757 | 22.5483 | 22.5758 | 22.3802 | 24.5205 | |
10 | 23.4554 | 25.3079 | 24.3555 | 24.4406 | 25.4561 | 21.6361 | 24.8465 | 26.3186 | |
12 | 25.8139 | 26.8848 | 26.0117 | 23.2895 | 26.5957 | 23.0829 | 26.4860 | 27.2748 |
IMAGE | K | SSA | MVO | DA | FPA | PSO | SCA | ALO | MALO |
Cactus | 4 | 0.6001 | 0.6001 | 0.6001 | 0.5854 | 0.6015 | 0.6001 | 0.5984 | 0.6063 |
6 | 0.6955 | 0.6961 | 0.6950 | 0.6716 | 0.6801 | 0.6568 | 0.6956 | 0.6963 | |
8 | 0.7438 | 0.7515 | 0.7268 | 0.7278 | 0.7361 | 0.7507 | 0.7487 | 0.7904 | |
10 | 0.7684 | 0.7838 | 0.7732 | 0.7495 | 0.7745 | 0.7814 | 0.7807 | 0.8391 | |
12 | 0.8012 | 0.8123 | 0.8157 | 0.8272 | 0.7927 | 0.8022 | 0.8050 | 0.8456 | |
Kangaroo | 4 | 0.7735 | 0.7735 | 0.7735 | 0.7156 | 0.7738 | 0.7046 | 0.7725 | 0.7735 |
6 | 0.8609 | 0.8615 | 0.8515 | 0.8179 | 0.8525 | 0.8033 | 0.8608 | 0.8627 | |
8 | 0.9018 | 0.9066 | 0.9029 | 0.8415 | 0.8958 | 0.8431 | 0.9041 | 0.9084 | |
10 | 0.9219 | 0.9325 | 0.9288 | 0.8581 | 0.9244 | 0.8658 | 0.9262 | 0.9331 | |
12 | 0.9352 | 0.9466 | 0.9426 | 0.9032 | 0.9449 | 0.9032 | 0.9474 | 0.9495 | |
Temple | 4 | 0.5829 | 0.5829 | 0.5829 | 0.5906 | 0.5825 | 0.5492 | 0.5793 | 0.6798 |
6 | 0.7234 | 0.6884 | 0.7242 | 0.7222 | 0.6836 | 0.6239 | 0.7249 | 0.7263 | |
8 | 0.7702 | 0.8149 | 0.7873 | 0.7265 | 0.7843 | 0.8102 | 0.8121 | 0.8152 | |
10 | 0.8069 | 0.8561 | 0.8329 | 0.7886 | 0.8084 | 0.7752 | 0.8480 | 0.8643 | |
12 | 0.8823 | 0.8883 | 0.8606 | 0.8254 | 0.8453 | 0.8546 | 0.8753 | 0.8917 | |
Flower | 4 | 0.4655 | 0.4655 | 0.4655 | 0.4194 | 0.4655 | 0.4655 | 0.4656 | 0.4916 |
6 | 0.5704 | 0.6806 | 0.6789 | 0.4849 | 0.6696 | 0.6800 | 0.6765 | 0.7406 | |
8 | 0.7883 | 0.7946 | 0.7974 | 0.6136 | 0.7867 | 0.7725 | 0.7911 | 0.8000 | |
10 | 0.8139 | 0.8286 | 0.8219 | 0.7771 | 0.8206 | 0.7406 | 0.8268 | 0.8294 | |
12 | 0.8364 | 0.8550 | 0.8310 | 0.8422 | 0.8372 | 0.8203 | 0.8644 | 0.8682 | |
Mountain | 4 | 0.6987 | 0.6987 | 0.6987 | 0.6715 | 0.6987 | 0.6987 | 0.6976 | 0.7081 |
6 | 0.7471 | 0.7758 | 0.7691 | 0.7237 | 0.7698 | 0.7761 | 0.7705 | 0.7932 | |
8 | 0.8293 | 0.8166 | 0.8127 | 0.7688 | 0.8101 | 0.8206 | 0.8311 | 0.8322 | |
10 | 0.8442 | 0.8536 | 0.8476 | 0.8254 | 0.8524 | 0.8261 | 0.8520 | 0.8603 | |
12 | 0.8682 | 0.8650 | 0.8626 | 0.8273 | 0.8731 | 0.8511 | 0.8617 | 0.8780 | |
Tree | 4 | 0.6406 | 0.6410 | 0.6406 | 0.6162 | 0.6419 | 0.6406 | 0.6412 | 0.6915 |
6 | 0.7535 | 0.7538 | 0.7535 | 0.6682 | 0.7420 | 0.7454 | 0.7538 | 0.7546 | |
8 | 0.7919 | 0.7890 | 0.7901 | 0.7176 | 0.7465 | 0.7515 | 0.7954 | 0.7960 | |
10 | 0.8229 | 0.8272 | 0.8263 | 0.7932 | 0.8190 | 0.8009 | 0.8258 | 0.8281 | |
12 | 0.8372 | 0.8505 | 0.8416 | 0.8307 | 0.8387 | 0.8142 | 0.8555 | 0.8579 | |
Horse | 4 | 0.7206 | 0.7206 | 0.7203 | 0.6791 | 0.7206 | 0.7155 | 0.7201 | 0.7206 |
6 | 0.7543 | 0.7624 | 0.7613 | 0.7617 | 0.7611 | 0.7700 | 0.7591 | 0.7744 | |
8 | 0.8102 | 0.8062 | 0.8103 | 0.7689 | 0.7945 | 0.7622 | 0.8114 | 0.8117 | |
10 | 0.8501 | 0.8510 | 0.8505 | 0.8026 | 0.8454 | 0.8162 | 0.8523 | 0.8534 | |
12 | 0.8709 | 0.8769 | 0.8739 | 0.8233 | 0.8757 | 0.8161 | 0.8686 | 0.8793 | |
Bridge | 4 | 0.7286 | 0.7286 | 0.7286 | 0.7286 | 0.7286 | 0.7293 | 0.7280 | 0.7398 |
6 | 0.8032 | 0.8180 | 0.8180 | 0.8022 | 0.8190 | 0.7728 | 0.8030 | 0.8193 | |
8 | 0.8626 | 0.8512 | 0.8566 | 0.8374 | 0.8604 | 0.8323 | 0.8620 | 0.8691 | |
10 | 0.8830 | 0.8909 | 0.8903 | 0.8447 | 0.8912 | 0.8886 | 0.8956 | 0.8993 | |
12 | 0.9021 | 0.9140 | 0.9031 | 0.8986 | 0.9041 | 0.9063 | 0.9087 | 0.9226 | |
Pilot | 4 | 0.7296 | 0.7296 | 0.7296 | 0.7222 | 0.7296 | 0.7296 | 0.7299 | 0.7399 |
6 | 0.7854 | 0.7857 | 0.7839 | 0.7540 | 0.7852 | 0.7849 | 0.7855 | 0.8224 | |
8 | 0.8420 | 0.8385 | 0.8383 | 0.7887 | 0.8218 | 0.7875 | 0.8390 | 0.8424 | |
10 | 0.8720 | 0.8651 | 0.8607 | 0.8555 | 0.8570 | 0.8401 | 0.8737 | 0.8788 | |
12 | 0.8905 | 0.8996 | 0.8951 | 0.8581 | 0.8726 | 0.8668 | 0.8947 | 0.9017 | |
Dog | 4 | 0.7003 | 0.7031 | 0.7003 | 0.7003 | 0.7003 | 0.6563 | 0.6999 | 0.7428 |
6 | 0.7700 | 0.7755 | 0.7624 | 0.7684 | 0.7514 | 0.7104 | 0.7694 | 0.7756 | |
8 | 0.8188 | 0.8190 | 0.8190 | 0.7735 | 0.7848 | 0.7632 | 0.8213 | 0.8240 | |
10 | 0.8314 | 0.8572 | 0.8510 | 0.8116 | 0.8470 | 0.7804 | 0.8544 | 0.8655 | |
12 | 0.8691 | 0.8928 | 0.8643 | 0.8246 | 0.8654 | 0.8566 | 0.8886 | 0.8948 |
IMAGE | K | SSA | MVO | DA | FPA | PSO | SCA | ALO | MALO |
Cactus | 4 | 0.4811 | 0.4831 | 0.4831 | 0.4466 | 0.4818 | 0.4497 | 0.4835 | 0.4858 |
6 | 0.5552 | 0.5975 | 0.5613 | 0.5064 | 0.5697 | 0.7353 | 0.5657 | 0.5690 | |
8 | 0.6254 | 0.7141 | 0.7143 | 0.6989 | 0.6473 | 0.6168 | 0.6574 | 0.7681 | |
10 | 0.7156 | 0.7719 | 0.7926 | 0.7684 | 0.7661 | 0.8080 | 0.7761 | 0.8677 | |
12 | 0.8238 | 0.8537 | 0.7953 | 0.8778 | 0.8524 | 0.7282 | 0.8510 | 0.8873 | |
Kangaroo | 4 | 0.6080 | 0.6080 | 0.6078 | 0.5032 | 0.6058 | 0.5645 | 0.6083 | 0.6116 |
6 | 0.7320 | 0.7635 | 0.7531 | 0.6689 | 0.7565 | 0.6881 | 0.7690 | 0.7782 | |
8 | 0.8263 | 0.8498 | 0.8304 | 0.7028 | 0.8493 | 0.7534 | 0.8476 | 0.8521 | |
10 | 0.8632 | 0.8867 | 0.8798 | 0.8570 | 0.8798 | 0.7602 | 0.8843 | 0.8913 | |
12 | 0.8976 | 0.9150 | 0.9128 | 0.8380 | 0.9111 | 0.8155 | 0.9096 | 0.9254 | |
Temple | 4 | 0.5367 | 0.6145 | 0.5713 | 0.5753 | 0.5657 | 0.5101 | 0.6117 | 0.6149 |
6 | 0.6933 | 0.6996 | 0.6933 | 0.7056 | 0.6976 | 0.7261 | 0.7014 | 0.7784 | |
8 | 0.8252 | 0.8034 | 0.7796 | 0.7400 | 0.8173 | 0.7918 | 0.8318 | 0.8465 | |
10 | 0.8469 | 0.8719 | 0.8522 | 0.7703 | 0.8665 | 0.7311 | 0.8648 | 0.8787 | |
12 | 0.8733 | 0.8941 | 0.8845 | 0.8153 | 0.8796 | 0.8086 | 0.8915 | 0.9021 | |
Flower | 4 | 0.3948 | 0.3985 | 0.3948 | 0.3969 | 0.3976 | 0.3976 | 0.3950 | 0.3994 |
6 | 0.4916 | 0.5129 | 0.4916 | 0.4830 | 0.4925 | 0.4923 | 0.4917 | 0.5247 | |
8 | 0.5171 | 0.5986 | 0.5385 | 0.5047 | 0.5538 | 0.5388 | 0.5387 | 0.6431 | |
10 | 0.5589 | 0.6425 | 0.5845 | 0.5280 | 0.5968 | 0.5964 | 0.5885 | 0.6606 | |
12 | 0.5737 | 0.6445 | 0.6168 | 0.5823 | 0.6299 | 0.7444 | 0.6410 | 0.7643 | |
Mountain | 4 | 0.4651 | 0.5563 | 0.5563 | 0.4348 | 0.4186 | 0.5804 | 0.4192 | 0.6240 |
6 | 0.6590 | 0.6916 | 0.6673 | 0.7108 | 0.6961 | 0.6653 | 0.6674 | 0.7131 | |
8 | 0.7628 | 0.7797 | 0.7716 | 0.6980 | 0.7612 | 0.7505 | 0.7695 | 0.8259 | |
10 | 0.7035 | 0.8433 | 0.7862 | 0.7690 | 0.8408 | 0.8062 | 0.8135 | 0.8792 | |
12 | 0.8537 | 0.8681 | 0.8645 | 0.8367 | 0.8718 | 0.7863 | 0.8668 | 0.9085 | |
Tree | 4 | 0.5888 | 0.5906 | 0.5888 | 0.6081 | 0.5874 | 0.5888 | 0.5866 | 0.6264 |
6 | 0.6716 | 0.6898 | 0.6728 | 0.6909 | 0.6708 | 0.6817 | 0.6770 | 0.7219 | |
8 | 0.7080 | 0.7157 | 0.7126 | 0.6873 | 0.7171 | 0.7503 | 0.7129 | 0.7737 | |
10 | 0.7271 | 0.7594 | 0.7631 | 0.7740 | 0.7656 | 0.6862 | 0.7653 | 0.8214 | |
12 | 0.7646 | 0.7871 | 0.7772 | 0.7040 | 0.7822 | 0.7977 | 0.8101 | 0.8792 | |
Horse | 4 | 0.6486 | 0.6486 | 0.6637 | 0.6911 | 0.6976 | 0.6675 | 0.6835 | 0.7070 |
6 | 0.7287 | 0.7486 | 0.7478 | 0.6954 | 0.7414 | 0.7463 | 0.7487 | 0.7802 | |
8 | 0.7789 | 0.7930 | 0.7922 | 0.7668 | 0.8010 | 0.7618 | 0.8035 | 0.8335 | |
10 | 0.8221 | 0.8388 | 0.8247 | 0.7824 | 0.8276 | 0.7849 | 0.8376 | 0.8697 | |
12 | 0.8410 | 0.8699 | 0.8567 | 0.7938 | 0.8691 | 0.8089 | 0.8598 | 0.8979 | |
Bridge | 4 | 0.6914 | 0.6927 | 0.6902 | 0.6790 | 0.6918 | 0.6897 | 0.6946 | 0.7176 |
6 | 0.7909 | 0.7974 | 0.7957 | 0.7433 | 0.7926 | 0.7813 | 0.7979 | 0.8053 | |
8 | 0.8336 | 0.8532 | 0.8522 | 0.7775 | 0.8505 | 0.8427 | 0.8564 | 0.8820 | |
10 | 0.8688 | 0.8812 | 0.8598 | 0.8144 | 0.8717 | 0.8636 | 0.8878 | 0.9160 | |
12 | 0.8836 | 0.9044 | 0.9271 | 0.8634 | 0.9124 | 0.8837 | 0.9116 | 0.9393 | |
Pilot | 4 | 0.7676 | 0.7675 | 0.7665 | 0.7440 | 0.7644 | 0.7686 | 0.7688 | 0.7512 |
6 | 0.8059 | 0.8241 | 0.8201 | 0.7920 | 0.8220 | 0.8259 | 0.7979 | 0.8317 | |
8 | 0.8584 | 0.8617 | 0.8621 | 0.8436 | 0.8620 | 0.8585 | 0.8619 | 0.8672 | |
10 | 0.8859 | 0.8857 | 0.8839 | 0.8781 | 0.8852 | 0.8787 | 0.8908 | 0.8937 | |
12 | 0.9031 | 0.9045 | 0.9100 | 0.8703 | 0.9036 | 0.8707 | 0.9009 | 0.9124 | |
Dog | 4 | 0.6534 | 0.6534 | 0.6218 | 0.5749 | 0.6510 | 0.6471 | 0.6582 | 0.6599 |
6 | 0.7021 | 0.7305 | 0.7252 | 0.7381 | 0.7333 | 0.6472 | 0.7290 | 0.7797 | |
8 | 0.7768 | 0.7801 | 0.7867 | 0.8020 | 0.7815 | 0.7875 | 0.7823 | 0.8486 | |
10 | 0.8039 | 0.8538 | 0.8278 | 0.8369 | 0.8574 | 0.7654 | 0.8338 | 0.9002 | |
12 | 0.8576 | 0.8860 | 0.8706 | 0.8378 | 0.8754 | 0.8326 | 0.8720 | 0.8889 |
IMAGE | K | SSA | MVO | DA | FPA | PSO | SCA | ALO | MALO |
Cactus | 4 | 0.8104 | 0.8104 | 0.8104 | 0.7856 | 0.8104 | 0.7991 | 0.8103 | 0.8104 |
6 | 0.8679 | 0.8673 | 0.8676 | 0.8305 | 0.8585 | 0.8280 | 0.8676 | 0.8681 | |
8 | 0.8967 | 0.8978 | 0.8936 | 0.8625 | 0.8912 | 0.8869 | 0.8974 | 0.8980 | |
10 | 0.9133 | 0.9153 | 0.9143 | 0.8868 | 0.9137 | 0.9029 | 0.9148 | 0.9153 | |
12 | 0.9250 | 0.9261 | 0.9264 | 0.9001 | 0.9218 | 0.9111 | 0.9272 | 0.9287 | |
Kangaroo | 4 | 0.8293 | 0.8293 | 0.8293 | 0.8005 | 0.8297 | 0.7913 | 0.8284 | 0.8293 |
6 | 0.8998 | 0.8999 | 0.8962 | 0.8585 | 0.8904 | 0.8642 | 0.9000 | 0.9001 | |
8 | 0.9325 | 0.9328 | 0.9325 | 0.8860 | 0.9246 | 0.8884 | 0.9330 | 0.9335 | |
10 | 0.9474 | 0.9521 | 0.9534 | 0.8914 | 0.9454 | 0.9055 | 0.9504 | 0.9541 | |
12 | 0.9607 | 0.9649 | 0.9638 | 0.9358 | 0.9594 | 0.9352 | 0.9630 | 0.9653 | |
Temple | 4 | 0.7391 | 0.7391 | 0.7391 | 0.7435 | 0.7388 | 0.7130 | 0.7374 | 0.7840 |
6 | 0.8178 | 0.7984 | 0.8181 | 0.8137 | 0.7968 | 0.7606 | 0.8189 | 0.8203 | |
8 | 0.8420 | 0.8693 | 0.8497 | 0.8270 | 0.8491 | 0.8671 | 0.8709 | 0.8722 | |
10 | 0.8628 | 0.8929 | 0.8805 | 0.8614 | 0.8615 | 0.8481 | 0.8888 | 0.8994 | |
12 | 0.9185 | 0.9135 | 0.8967 | 0.8820 | 0.8854 | 0.8994 | 0.9085 | 0.9301 | |
Flower | 4 | 0.7838 | 0.7838 | 0.7838 | 0.7604 | 0.7838 | 0.7726 | 0.7838 | 0.7841 |
6 | 0.8402 | 0.8413 | 0.8406 | 0.8177 | 0.8356 | 0.8142 | 0.8404 | 0.8418 | |
8 | 0.8829 | 0.8810 | 0.8864 | 0.8461 | 0.8790 | 0.8524 | 0.8828 | 0.8874 | |
10 | 0.9041 | 0.9100 | 0.9110 | 0.8639 | 0.9092 | 0.8791 | 0.9114 | 0.9120 | |
12 | 0.9246 | 0.9269 | 0.9191 | 0.8891 | 0.9195 | 0.8912 | 0.9333 | 0.9347 | |
Mountain | 4 | 0.8006 | 0.8006 | 0.8006 | 0.7658 | 0.8006 | 0.7918 | 0.7999 | 0.8006 |
6 | 0.8426 | 0.8561 | 0.8550 | 0.8225 | 0.8494 | 0.8335 | 0.8552 | 0.8563 | |
8 | 0.8893 | 0.8892 | 0.8866 | 0.8570 | 0.8824 | 0.8672 | 0.8901 | 0.8903 | |
10 | 0.9074 | 0.9094 | 0.9083 | 0.8789 | 0.9042 | 0.8793 | 0.9078 | 0.9142 | |
12 | 0.9217 | 0.9217 | 0.9214 | 0.8919 | 0.9211 | 0.8941 | 0.9182 | 0.9253 | |
Tree | 4 | 0.8055 | 0.8056 | 0.8055 | 0.7873 | 0.8060 | 0.8055 | 0.8058 | 0.8166 |
6 | 0.8562 | 0.8566 | 0.8562 | 0.8218 | 0.8479 | 0.8448 | 0.8562 | 0.8568 | |
8 | 0.8826 | 0.8825 | 0.8825 | 0.8408 | 0.8680 | 0.8423 | 0.8833 | 0.8840 | |
10 | 0.9038 | 0.9038 | 0.9041 | 0.8713 | 0.8981 | 0.8729 | 0.9026 | 0.9055 | |
12 | 0.9149 | 0.9203 | 0.9201 | 0.8984 | 0.9098 | 0.8789 | 0.9186 | 0.9208 | |
Horse | 4 | 0.8217 | 0.8217 | 0.8216 | 0.7960 | 0.8217 | 0.8099 | 0.8213 | 0.8217 |
6 | 0.8506 | 0.8504 | 0.8503 | 0.8472 | 0.8505 | 0.8388 | 0.8490 | 0.8506 | |
8 | 0.8849 | 0.8801 | 0.8848 | 0.8555 | 0.8687 | 0.8423 | 0.8827 | 0.8852 | |
10 | 0.9120 | 0.9082 | 0.9053 | 0.8773 | 0.9053 | 0.8708 | 0.9063 | 0.9122 | |
12 | 0.9269 | 0.9240 | 0.9279 | 0.8886 | 0.9254 | 0.8801 | 0.9202 | 0.9303 | |
Bridge | 4 | 0.8399 | 0.8399 | 0.8399 | 0.8276 | 0.8399 | 0.8276 | 0.8397 | 0.8399 |
6 | 0.8894 | 0.8971 | 0.8968 | 0.8706 | 0.8968 | 0.8613 | 0.8894 | 0.8974 | |
8 | 0.9235 | 0.9150 | 0.9223 | 0.8951 | 0.9203 | 0.8849 | 0.9202 | 0.9241 | |
10 | 0.9368 | 0.9350 | 0.9389 | 0.9098 | 0.9346 | 0.9192 | 0.9397 | 0.9398 | |
12 | 0.9478 | 0.9493 | 0.9470 | 0.9332 | 0.9469 | 0.9341 | 0.9453 | 0.9499 | |
Pilot | 4 | 0.7854 | 0.7854 | 0.7854 | 0.7765 | 0.7854 | 0.7736 | 0.7854 | 0.7855 |
6 | 0.8294 | 0.8260 | 0.8290 | 0.8070 | 0.8292 | 0.8290 | 0.8295 | 0.8345 | |
8 | 0.8623 | 0.8623 | 0.8619 | 0.8278 | 0.8531 | 0.8277 | 0.8617 | 0.8625 | |
10 | 0.8890 | 0.8819 | 0.8829 | 0.8710 | 0.8788 | 0.8547 | 0.8898 | 0.8918 | |
12 | 0.9033 | 0.9122 | 0.9079 | 0.8714 | 0.8905 | 0.8787 | 0.9101 | 0.9148 | |
Dog | 4 | 0.7911 | 0.7918 | 0.7911 | 0.7911 | 0.7911 | 0.7584 | 0.7911 | 0.7943 |
6 | 0.8497 | 0.8519 | 0.8467 | 0.8274 | 0.8347 | 0.8092 | 0.8494 | 0.8521 | |
8 | 0.8822 | 0.8793 | 0.8823 | 0.8436 | 0.8571 | 0.8452 | 0.8827 | 0.8834 | |
10 | 0.8932 | 0.9052 | 0.9049 | 0.8720 | 0.9002 | 0.8584 | 0.9050 | 0.9109 | |
12 | 0.9147 | 0.9277 | 0.9127 | 0.8882 | 0.9109 | 0.8965 | 0.9235 | 0.9288 |
IMAGE | K | SSA | MVO | DA | FPA | PSO | SCA | ALO | MALO |
Cactus | 4 | 0.7694 | 0.7700 | 0.7700 | 0.7508 | 0.7701 | 0.7453 | 0.7708 | 0.7711 |
6 | 0.8213 | 0.8263 | 0.8232 | 0.7858 | 0.8269 | 0.8315 | 0.8248 | 0.8363 | |
8 | 0.8598 | 0.8767 | 0.8766 | 0.8257 | 0.8672 | 0.8361 | 0.8674 | 0.8924 | |
10 | 0.8791 | 0.9048 | 0.8995 | 0.8791 | 0.9021 | 0.8787 | 0.9049 | 0.9227 | |
12 | 0.9164 | 0.9290 | 0.9154 | 0.9140 | 0.9278 | 0.8735 | 0.9260 | 0.9375 | |
Kangaroo | 4 | 0.7539 | 0.7539 | 0.7536 | 0.6738 | 0.7522 | 0.7172 | 0.7549 | 0.7574 |
6 | 0.8466 | 0.8609 | 0.8550 | 0.7787 | 0.8574 | 0.8118 | 0.8674 | 0.8713 | |
8 | 0.9112 | 0.9218 | 0.9113 | 0.8341 | 0.9214 | 0.8522 | 0.9196 | 0.9231 | |
10 | 0.9366 | 0.9456 | 0.9430 | 0.9110 | 0.9427 | 0.8605 | 0.9468 | 0.9482 | |
12 | 0.9543 | 0.9617 | 0.9609 | 0.9105 | 0.9617 | 0.8950 | 0.9596 | 0.9632 | |
Temple | 4 | 0.7190 | 0.7590 | 0.7310 | 0.7199 | 0.7282 | 0.6860 | 0.7570 | 0.7591 |
6 | 0.8107 | 0.8139 | 0.8107 | 0.7906 | 0.8129 | 0.7971 | 0.8111 | 0.8537 | |
8 | 0.8954 | 0.8796 | 0.8681 | 0.8172 | 0.8891 | 0.8519 | 0.8990 | 0.9067 | |
10 | 0.9103 | 0.9290 | 0.9146 | 0.8471 | 0.9256 | 0.7991 | 0.9226 | 0.9299 | |
12 | 0.9293 | 0.9389 | 0.9386 | 0.8743 | 0.9315 | 0.8680 | 0.9425 | 0.9501 | |
Flower | 4 | 0.7495 | 0.7505 | 0.7495 | 0.7293 | 0.7507 | 0.7504 | 0.7505 | 0.7508 |
6 | 0.8086 | 0.8097 | 0.8101 | 0.7886 | 0.8096 | 0.8024 | 0.8095 | 0.8202 | |
8 | 0.8317 | 0.8419 | 0.8411 | 0.8211 | 0.8494 | 0.8519 | 0.8464 | 0.8705 | |
10 | 0.8605 | 0.8796 | 0.8741 | 0.8586 | 0.8784 | 0.8538 | 0.8769 | 0.8935 | |
12 | 0.8736 | 0.9073 | 0.8994 | 0.8478 | 0.9015 | 0.8888 | 0.8969 | 0.9255 | |
Mountain | 4 | 0.7490 | 0.7600 | 0.7600 | 0.7470 | 0.7390 | 0.7640 | 0.7385 | 0.7857 |
6 | 0.8040 | 0.8101 | 0.8111 | 0.7933 | 0.8088 | 0.7753 | 0.8046 | 0.8142 | |
8 | 0.8467 | 0.8612 | 0.8539 | 0.8003 | 0.8485 | 0.8084 | 0.8498 | 0.8765 | |
10 | 0.8444 | 0.8899 | 0.8723 | 0.8368 | 0.8834 | 0.8370 | 0.8810 | 0.9131 | |
12 | 0.8988 | 0.9099 | 0.9081 | 0.8771 | 0.9113 | 0.8453 | 0.9067 | 0.9313 | |
Tree | 4 | 0.7803 | 0.7816 | 0.7803 | 0.7881 | 0.7804 | 0.7947 | 0.7800 | 0.7803 |
6 | 0.8303 | 0.8366 | 0.8311 | 0.8372 | 0.8304 | 0.8367 | 0.8340 | 0.8398 | |
8 | 0.8575 | 0.8602 | 0.8583 | 0.8362 | 0.8606 | 0.8490 | 0.8586 | 0.8795 | |
10 | 0.8741 | 0.8850 | 0.8855 | 0.8588 | 0.8862 | 0.8377 | 0.8889 | 0.8985 | |
12 | 0.8962 | 0.8987 | 0.8983 | 0.8545 | 0.9015 | 0.8627 | 0.9035 | 0.9141 | |
Horse | 4 | 0.7725 | 0.7725 | 0.7844 | 0.7942 | 0.8057 | 0.7902 | 0.7952 | 0.8125 |
6 | 0.8344 | 0.8467 | 0.8465 | 0.8092 | 0.8422 | 0.8260 | 0.8479 | 0.8604 | |
8 | 0.8722 | 0.8784 | 0.8781 | 0.8486 | 0.8800 | 0.8444 | 0.8832 | 0.8946 | |
10 | 0.8977 | 0.9053 | 0.9011 | 0.8600 | 0.9005 | 0.8459 | 0.9058 | 0.9087 | |
12 | 0.9126 | 0.9234 | 0.9201 | 0.8674 | 0.9186 | 0.8707 | 0.9207 | 0.9240 | |
Bridge | 4 | 0.8220 | 0.8221 | 0.8219 | 0.8042 | 0.8221 | 0.8083 | 0.8234 | 0.8378 |
6 | 0.8894 | 0.8909 | 0.8908 | 0.8475 | 0.8897 | 0.8495 | 0.8917 | 0.8928 | |
8 | 0.9137 | 0.9215 | 0.9209 | 0.8719 | 0.9208 | 0.8902 | 0.9215 | 0.9224 | |
10 | 0.9317 | 0.9350 | 0.9277 | 0.8925 | 0.9313 | 0.9038 | 0.9353 | 0.9467 | |
12 | 0.9394 | 0.9567 | 0.9453 | 0.9113 | 0.9503 | 0.9190 | 0.9476 | 0.9580 | |
Pilot | 4 | 0.7901 | 0.7894 | 0.7893 | 0.7740 | 0.7887 | 0.7850 | 0.7874 | 0.7912 |
6 | 0.8303 | 0.8409 | 0.8326 | 0.8103 | 0.8337 | 0.8292 | 0.8284 | 0.8343 | |
8 | 0.8726 | 0.8726 | 0.8749 | 0.8554 | 0.8755 | 0.8649 | 0.8751 | 0.8758 | |
10 | 0.8991 | 0.8872 | 0.8983 | 0.8829 | 0.8984 | 0.8782 | 0.9023 | 0.9063 | |
12 | 0.9158 | 0.9158 | 0.9225 | 0.8776 | 0.9177 | 0.8890 | 0.9175 | 0.9237 | |
Dog | 4 | 0.7595 | 0.7594 | 0.7375 | 0.7074 | 0.7580 | 0.7413 | 0.7621 | 0.7625 |
6 | 0.8043 | 0.8208 | 0.8182 | 0.8037 | 0.8205 | 0.7507 | 0.8212 | 0.8490 | |
8 | 0.8582 | 0.8617 | 0.8609 | 0.8404 | 0.8615 | 0.8355 | 0.8609 | 0.8911 | |
10 | 0.8772 | 0.9033 | 0.8918 | 0.8788 | 0.9032 | 0.8278 | 0.8976 | 0.9269 | |
12 | 0.9092 | 0.9254 | 0.9151 | 0.8727 | 0.9178 | 0.8642 | 0.9187 | 0.9275 |
IMAGE | K | PSNR | SSIM | FSIM | |||
Otsu | Kapur | Otsu | Kapur | Otsu | Kapur | ||
Cactus | 4 | 19.1901 | 17.2250 | 0.6063 | 0.4858 | 0.8104 | 0.7711 |
6 | 21.4849 | 20.9242 | 0.6963 | 0.5690 | 0.8681 | 0.8363 | |
8 | 23.7403 | 23.1098 | 0.7904 | 0.7681 | 0.8980 | 0.8924 | |
10 | 24.1499 | 25.6436 | 0.8391 | 0.8677 | 0.9153 | 0.9227 | |
12 | 25.2868 | 26.7329 | 0.8456 | 0.8873 | 0.9287 | 0.9375 | |
Kangaroo | 4 | 22.0797 | 18.8067 | 0.7735 | 0.6116 | 0.8293 | 0.7574 |
6 | 24.8836 | 22.3708 | 0.8627 | 0.7782 | 0.9001 | 0.8713 | |
8 | 27.0860 | 24.6322 | 0.9084 | 0.8521 | 0.9335 | 0.9231 | |
10 | 28.8186 | 26.1974 | 0.9331 | 0.8913 | 0.9541 | 0.9482 | |
12 | 30.3411 | 28.0349 | 0.9495 | 0.9254 | 0.9653 | 0.9632 | |
Temple | 4 | 17.6125 | 18.6105 | 0.6798 | 0.6149 | 0.7840 | 0.7591 |
6 | 21.4589 | 23.1506 | 0.7263 | 0.7784 | 0.8203 | 0.8537 | |
8 | 23.9977 | 25.1273 | 0.8152 | 0.8465 | 0.8722 | 0.9067 | |
10 | 25.7059 | 26.7757 | 0.8643 | 0.8787 | 0.8994 | 0.9299 | |
12 | 27.5232 | 28.4027 | 0.8917 | 0.9021 | 0.9301 | 0.9501 | |
Flower | 4 | 22.6916 | 21.4046 | 0.4916 | 0.3994 | 0.7841 | 0.7508 |
6 | 25.5506 | 24.2923 | 0.7406 | 0.5247 | 0.8418 | 0.8202 | |
8 | 27.6960 | 26.3947 | 0.8000 | 0.6431 | 0.8874 | 0.8705 | |
10 | 29.2169 | 27.6016 | 0.8294 | 0.6606 | 0.9120 | 0.8935 | |
12 | 30.7280 | 29.2750 | 0.8682 | 0.7643 | 0.9347 | 0.9255 | |
Mountain | 4 | 19.7307 | 17.7501 | 0.7081 | 0.6240 | 0.8006 | 0.7857 |
6 | 22.5039 | 20.2658 | 0.7932 | 0.7131 | 0.8563 | 0.8142 | |
8 | 24.9053 | 23.7160 | 0.8322 | 0.8259 | 0.8903 | 0.8765 | |
10 | 26.3373 | 26.2667 | 0.8603 | 0.8792 | 0.9142 | 0.9131 | |
12 | 27.4991 | 26.6949 | 0.8780 | 0.9085 | 0.9253 | 0.9313 | |
Tree | 4 | 19.9364 | 19.3878 | 0.6915 | 0.6264 | 0.8166 | 0.7803 |
6 | 22.9388 | 22.0355 | 0.7546 | 0.7219 | 0.8568 | 0.8398 | |
8 | 25.1704 | 24.2661 | 0.7960 | 0.7737 | 0.8840 | 0.8795 | |
10 | 26.7213 | 25.7360 | 0.8281 | 0.8214 | 0.9055 | 0.8985 | |
12 | 28.0724 | 26.8461 | 0.8579 | 0.8792 | 0.9208 | 0.9141 | |
Horse | 4 | 19.1569 | 19.7788 | 0.7206 | 0.7070 | 0.8217 | 0.8125 |
6 | 22.8301 | 21.6974 | 0.7744 | 0.7802 | 0.8506 | 0.8604 | |
8 | 24.8543 | 24.2427 | 0.8117 | 0.8335 | 0.8852 | 0.8946 | |
10 | 26.8845 | 26.1268 | 0.8534 | 0.8697 | 0.9122 | 0.9087 | |
12 | 28.3129 | 27.4180 | 0.8793 | 0.8979 | 0.9303 | 0.9240 | |
Bridge | 4 | 18.7684 | 18.3564 | 0.7398 | 0.7176 | 0.8399 | 0.8378 |
6 | 21.5346 | 21.1004 | 0.8193 | 0.8053 | 0.8974 | 0.8928 | |
8 | 23.7010 | 23.6124 | 0.8691 | 0.8820 | 0.9241 | 0.9224 | |
10 | 25.4265 | 25.3739 | 0.8993 | 0.9160 | 0.9398 | 0.9467 | |
12 | 27.0756 | 27.4320 | 0.9226 | 0.9393 | 0.9499 | 0.9580 | |
Pilot | 4 | 17.6971 | 16.0238 | 0.7399 | 0.7512 | 0.7855 | 0.7912 |
6 | 21.5230 | 20.7123 | 0.8224 | 0.8317 | 0.8345 | 0.8343 | |
8 | 22.4562 | 23.1598 | 0.8424 | 0.8672 | 0.8625 | 0.8758 | |
10 | 24.1912 | 24.8228 | 0.8788 | 0.8937 | 0.8918 | 0.9063 | |
12 | 25.8213 | 27.1095 | 0.9017 | 0.9124 | 0.9148 | 0.9237 | |
Dog | 4 | 19.9346 | 18.2605 | 0.7428 | 0.6599 | 0.7943 | 0.7625 |
6 | 22.6726 | 21.9672 | 0.7756 | 0.7797 | 0.8521 | 0.8490 | |
8 | 24.3548 | 24.5205 | 0.8240 | 0.8486 | 0.8834 | 0.8911 | |
10 | 26.4961 | 26.3186 | 0.8655 | 0.9002 | 0.9109 | 0.9269 | |
12 | 28.1328 | 27.2748 | 0.8948 | 0.8889 | 0.9288 | 0.9275 |
IMAGE | K | R | G | B |
Cactus | 4 | 48, 79,124,194 | 58, 90,126,187 | 54, 85,123,180 |
6 | 40, 63, 85,115,155,209 | 50, 74, 97,121,159,212 | 46, 67, 88,115,146,192 | |
8 | 36, 54, 71, 89,113,144,181,225 | 45, 64, 82, 99,118,142,177,221 | 41, 58, 74, 91,112,137,165,204 | |
10 | 34, 49, 63, 77, 91,109,133,160,190,227 | 43, 58, 73, 87,101,115,133,159,192,228 | 38, 51, 64, 77, 92,111,131,153,178,214 | |
12 | 33, 46, 58, 70, 82, 95,113,134,156,182,209,235 | 40, 53, 66, 79, 91,102,114,129,148,173,200,232 | 37, 48, 60, 71, 82, 96,112,130,147,166,189,220 | |
Kangaroo | 4 | 54, 87,116,155 | 49, 87,112,143 | 43, 72,103,145 |
6 | 41, 72, 92,113,140,173 | 38, 73, 95,113,134,163 | 32, 58, 77, 99,128,163 | |
8 | 34, 62, 80, 95,111,131,156,186 | 32, 62, 82, 97,111,125,145,175 | 28, 51, 67, 82,100,122,148,180 | |
10 | 31, 56, 72, 85, 97,110,126,145,166,195 | 28, 55, 74, 88,100,111,123,138,156,185 | 23, 44, 59, 71, 84, 99,117,138,161,190 | |
12 | 24, 47, 63, 76, 87, 97,108,122,139,157,177,206 | 24, 47, 65, 79, 91,101,110,120,131,145,162,187 | 19, 37, 51, 63, 73, 84, 96,111,128,147,167,196 | |
Temple | 4 | 80,116,152,207 | 81,111,141,178 | 62, 87,115,145 |
6 | 65, 89,113,137,165,213 | 71, 93,115,138,165,208 | 53, 70, 88,107,127,151 | |
8 | 57, 75, 94,112,130,149,172,216 | 64, 81, 98,115,132,150,172,210 | 47, 61, 74, 89,104,120,138,157 | |
10 | 55, 71, 87,103,118,134,151,172,200,234 | 60, 74, 88,102,115,129,143,160,178,212 | 45, 58, 69, 81, 95,109,124,141,159,239 | |
12 | 51, 65, 78, 92,105,117,130,142,156,174,201,233 | 57, 70, 83, 96,108,120,133,147,162,179,201,229 | 42, 53, 61, 69, 79, 90,101,111,122,135,148,162 | |
Flower | 4 | 51, 98,143,201 | 31, 69,111,168 | 19, 44, 75,119 |
6 | 34, 66, 98,131,163,209 | 22, 47, 77,109,150,202 | 7, 18, 37, 58, 84,126 | |
8 | 30, 55, 79,103,129,155,182,220 | 10, 25, 45, 68, 92,120,159,207 | 6, 14, 26, 42, 59, 78,102,140 | |
10 | 25, 44, 84,104,126,149,169,192,226 | 10, 23, 39, 57, 76, 95,117,144,178,218 | 6, 14, 26, 40, 56, 73, 94,126,176,252 | |
12 | 21, 36, 53, 70, 87,104,122,141,159,177,201,232 | 9, 20, 33, 48, 63, 79, 95,112,133,158,187,221 | 5, 10, 16, 25, 36, 48, 60, 73, 90,109,139,194 | |
Mountain | 4 | 50, 76,111,190 | 58, 91,124,190 | 58, 94,131,192 |
6 | 40, 58, 76,100,135,201 | 48, 69, 94,118,144,204 | 53, 76, 97,125,149,196 | |
8 | 36, 52, 67, 82,103,130,167,221 | 45, 63, 79, 99,118,139,174,224 | 39, 59, 78, 96,121,142,157,199 | |
10 | 34, 48, 60, 71, 84,102,123,148,187,230 | 2, 44, 62, 78, 96,112,124,144,182,229 | 38, 57, 75, 88,105,125,143,156,183,224 | |
12 | 34, 47, 58, 67, 76, 88,103,119,138,161,195,230 | 9, 43, 58, 69, 82, 98,111,122,137,157,189,233 | 37, 53, 68, 79, 92,110,127,141,151,160,183,224 | |
Tree | 4 | 30, 67,103,155 | 51,106,161,207 | 54,120,185,225 |
6 | 13, 35, 65, 96,132,175 | 39, 72,112,152,183,216 | 38, 74,125,173,203,229 | |
8 | 12, 31, 52, 72, 94,116,148,185 | 36, 63, 98,133,161,184,207,231 | 30, 52, 81,119,157,186,208,231 | |
10 | 10, 23, 39, 56, 74, 94,114,139,169,197 | 30, 50, 73,100,127,150,169,187,208,231 | 28, 46, 68, 97,130,160,184,202,215,234 | |
12 | 9, 22, 37, 51, 66, 82, 97,115,136,157,181,203 | 28, 45, 63, 84,107,130,150,166,179,192,210,233 | 24, 37, 51, 69, 92,119,147,171,190,205,217,235 | |
Horse | 4 | 59,100,145,193 | 54, 94,142,190 | 33, 61, 86,147 |
6 | 49, 83,111,143,179,208 | 46, 78,104,139,177,203 | 27, 51, 72, 93,145,193 | |
8 | 42, 70, 93,114,139,169,194,215 | 38, 65, 89,110,137,169,193,212 | 23, 44, 63, 80,101,148,189,200 | |
10 | 34, 56, 77, 95,111,130,155,180,200,218 | 30, 51, 71, 88,103,119,143,172,194,212 | 18, 33, 47, 60, 72, 84, 98,123,162,194 | |
12 | 29, 48, 67, 83, 98,112,127,147,168,187,204,220 | 29, 46, 63, 79, 93,106,120,142,166,185,200,215 | 17, 31, 44, 56, 67, 78, 89,103,128,164,190,201 | |
Bridge | 4 | 73,113,158,216 | 69,109,151,210 | 35, 75,116,159 |
6 | 61, 89,116,145,180,225 | 61, 92,120,148,181,224 | 24, 51, 80,110,139,169 | |
8 | 55, 77, 99,120,142,168,198,233 | 52, 74, 96,118,140,165,197,233 | 17, 36, 58, 81,105,127,148,172 | |
10 | 50, 68, 86,104,121,139,161,184,211,239 | 48, 67, 86,104,122,140,160,183,211,239 | 13, 29, 46, 63, 81,100,119,136,153,174 | |
12 | 48, 64, 79, 95,110,125,140,158,178,199,224,246 | 44, 60, 76, 91,106,122,137,154,172,192,218,243 | 11, 25, 40, 55, 71, 88,105,121,135,149,166,182 | |
Pilot | 4 | 102,149,194,224 | 104,157,205,235 | 83,123,167,220 |
6 | 90,120,155,191,217,234 | 88,117,150,187,217,239 | 78,111,149,181,212,243 | |
8 | 80,102,127,157,187,210,225,238 | 81,103,127,154,185,209,225,241 | 66, 87,109,136,165,188,214,243 | |
10 | 77, 96,116,135,157,180,200,216,228,239 | 76, 95,114,134,158,185,206,220,233,246 | 61, 78, 96,115,137,162,181,195,217,244 | |
12 | 72, 89,105,121,138,158,180,197,212,223,232,242 | 72, 86,100,117,136,159,184,203,216,226,237,248 | 61, 77, 94,112,132,154,172,185,196,214,234,248 | |
Dog | 4 | 50, 84,115,157 | 73,107,145,188 | 52, 83,123,161 |
6 | 38, 66, 89,112,133,168 | 61, 84,108,136,161,193 | 45, 67, 93,125,156,178 | |
8 | 33, 58, 78, 97,115,130,150,184 | 57, 75, 93,112,135,156,171,199 | 42, 60, 79,102,131,157,175,193 | |
10 | 30, 52, 70, 86,102,118,132,151,183,243 | 53, 69, 84,100,117,136,154,167,181,207 | 36, 49, 64, 80, 99,122,145,163,177,196 | |
12 | 24, 42, 57, 71, 84, 97,109,121,132,147,170,197 | 48, 61, 73, 87,101,115,131,147,159,169,183,209 | 33, 44, 57, 70, 83,100,121,141,157,168,179,196 |
IMAGE | K | R | G | B |
Cactus | 4 | 63,103,146,189 | 73,115,151,196 | 68,111,163,206 |
6 | 58, 92,121,153,185,216 | 62, 92,123,151,184,215 | 60, 95,129,162,190,222 | |
8 | 50, 77,102,128,154,180,204,229 | 56, 81,106,129,152,177,202,226 | 19, 53, 80,106,134,163,190,221 | |
10 | 43, 63, 83,103,122,143,164,185,207,230 | 49, 69, 89,109,129,148,169,191,212,233 | 19, 48, 71, 93,115,139,163,186,208,230 | |
12 | 17, 42, 64, 83,101,120,139,159,178,197,215,234 | 46, 63, 80, 97,115,132,148,165,183,201,219,237 | 19, 42, 58, 76, 94,112,130,148,166,187,208,231 | |
Kangaroo | 4 | 52,113,158,203 | 51, 90,138,188 | 40, 97,150,203 |
6 | 43, 75,113,148,184,215 | 47, 83,123,153,186,220 | 36, 68,102,142,185,220 | |
8 | 32, 58, 84,113,141,169,198,227 | 35, 61, 86,113,139,168,194,220 | 31, 57, 84,108,136,165,193,220 | |
10 | 24, 45, 66, 90,115,138,162,186,208,231 | 21, 42, 63, 86,109,133,153,175,195,220 | 25, 47, 69, 91,114,138,161,185,207,231 | |
12 | 20, 40, 58, 78, 98,117,136,157,178,198,215,234 | 17, 38, 56, 75, 94,114,132,152,171,190,210,229 | 18, 36, 55, 74, 94,115,135,154,174,194,214,233 | |
Temple | 4 | 74,114,153,192 | 82,120,158,195 | 46, 79,113,145 |
6 | 64, 96,128,160,194,225 | 72,103,134,163,196,226 | 25, 49, 80,113,144,178 | |
8 | 40, 65, 91,116,142,166,194,225 | 62, 89,116,143,169,196,216,236 | 23, 44, 67, 89,111,133,155,178 | |
10 | 39, 60, 82,103,126,149,170,194,214,235 | 46, 68, 89,109,130,151,173,196,216,235 | 18, 31, 47, 64, 81, 99,118,137,156,183 | |
12 | 35, 51, 69, 88,106,125,142,159,176,194,213,238 | 41, 55, 73, 90,107,123,141,159,178,196,216,235 | 18, 31, 46, 63, 80, 97,111,127,144,158,178,187 | |
Flower | 4 | 53, 99,143,187 | 49,106,153,202 | 27, 75,118,175 |
6 | 42, 75,108,143,177,206 | 32, 69,106,140,175,211 | 20, 51, 82,113,145,175 | |
8 | 37, 65, 94,121,149,177,202,227 | 25, 54, 83,112,140,169,196,223 | 20, 47, 75,102,127,150,175,197 | |
10 | 28, 50, 73, 95,116,137,159,183,205,228 | 22, 45, 68, 92,115,138,161,184,207,230 | 17, 36, 55, 76, 97,116,135,155,175,197 | |
12 | 26, 46, 66, 84,102,121,140,158,176,195,214,233 | 20, 39, 58, 78, 98,116,136,155,175,196,216,236 | 14, 27, 42, 56, 71, 87,103,118,135,155,175,198 | |
Mountain | 4 | 53, 89,124,156 | 87,138,177,218 | 88,132,173,215 |
6 | 53, 91,129,168,199,232 | 73,103,138,172,207,241 | 19, 63, 95,132,173,215 | |
8 | 52, 81,107,134,162,188,216,245 | 51, 77,102,136,160,185,213,241 | 19, 46, 65, 87,109,133,173,210 | |
10 | 22, 51, 74, 97,123,147,168,192,218,245 | 51, 76,101,119,138,160,181,203,223,244 | 19, 45, 65, 87,109,131,151,173,199,227 | |
12 | 21, 40, 60, 82,103,124,145,167,185,205,224,247 | 48, 64, 82,101,120,137,154,172,188,206,224,244 | 19, 45, 65, 89,111,132,151,172,185,200,218,236 | |
Tree | 4 | 51, 92,134,182 | 63,112,157,206 | 56, 99,148,192 |
6 | 29, 63, 97,132,168,206 | 45, 77,110,141,170,207 | 44, 78,113,151,190,225 | |
8 | 25, 55, 83,110,134,162,190,218 | 39, 66, 94,123,152,180,206,230 | 38, 65, 91,118,145,170,196,225 | |
10 | 22, 43, 65, 88,111,133,155,178,198,219 | 33, 54, 75, 96,117,139,160,183,206,229 | 33, 55, 77, 99,119,140,160,182,203,225 | |
12 | 21, 42, 63, 84,108,131,149,167,183,200,218,235 | 29, 47, 65, 83,100,117,134,152,168,187,206,230 | 28, 46, 63, 81, 99,119,138,156,174,190,206,225 | |
Horse | 4 | 52, 91,136,187 | 68,124,176,235 | 53,117,174,214 |
6 | 43, 77,111,142,177,213 | 49, 86,126,161,193,235 | 35, 67,103,134,173,214 | |
8 | 35, 62, 88,115,141,169,194,222 | 38, 67, 96,127,156,184,211,235 | 30, 59, 89,114,142,173,192,214 | |
10 | 31, 55, 77, 99,123,144,167,191,213,235 | 32, 55, 78,102,127,150,172,193,213,235 | 22, 44, 64, 86,105,125,148,173,192,214 | |
12 | 27, 46, 66, 85,104,123,142,160,178,198,219,239 | 28, 46, 64, 82,100,120,136,156,176,195,215,235 | 19, 35, 51, 70, 87,103,119,136,155,173,190,214 | |
Bridge | 4 | 70,113,156,199 | 77,129,180,227 | 49, 96,142,194 |
6 | 63, 98,133,167,201,236 | 60, 93,126,159,193,230 | 32, 62, 92,122,155,194 | |
8 | 54, 80,106,132,158,184,209,236 | 52, 78,105,132,158,185,210,236 | 26, 49, 73, 97,121,146,171,195 | |
10 | 48, 70, 92,113,134,156,177,198,218,239 | 47, 68, 90,111,133,155,177,198,219,238 | 24, 45, 68, 90,112,133,155,176,194,209 | |
12 | 45, 62, 79, 97,114,132,149,167,184,201,220,239 | 44, 62, 80, 99,116,134,152,169,186,204,222,240 | 22, 40, 58, 74, 89,105,121,139,157,176,194,209 | |
Pilot | 4 | 94,134,169,204 | 100,146,191,220 | 74,106,139,173 |
6 | 81,110,139,168,197,221 | 81,108,135,163,192,220 | 41, 85,125,168,203,235 | |
8 | 72, 96,119,143,168,194,216,238 | 74, 98,121,145,168,191,210,231 | 34, 67, 92,118,144,171,203,235 | |
10 | 69, 87,106,125,144,164,183,202,220,238 | 70, 89,108,127,146,164,182,200,218,236 | 28, 42, 68, 91,115,139,163,182,205,235 | |
12 | 55, 70, 86,103,120,136,151,168,185,203,220,238 | 67, 82, 98,113,129,145,160,175,191,206,221,238 | 28, 42, 67, 88,110,130,149,168,185,205,227,245 | |
Dog | 4 | 43, 92,146,187 | 74,112,149,192 | 62,102,149,200 |
6 | 38, 73,110,145,174,206 | 62, 90,119,150,184,215 | 56, 91,124,157,195,217 | |
8 | 29, 55, 84,113,144,167,190,214 | 61, 87,113,138,159,184,206,233 | 46, 72, 97,124,152,175,196,217 | |
10 | 21, 40, 60, 80,100,120,144,167,190,214 | 55, 76, 99,120,140,159,182,198,215,233 | 18, 45, 68, 91,114,137,157,176,196,217 | |
12 | 20, 39, 57, 75, 92,110,127,144,160,179,198,217 | 49, 66, 82, 99,117,133,149,165,182,197,215,233 | 18, 39, 56, 72, 89,105,122,141,159,178,196,217 |
[1] |
N. M. Zaitoun, M. J. Aqel, Survey on Image Segmentation Techniques, Procedia Comput. Sci., 65 (2015), 797-806. doi: 10.1016/j.procs.2015.09.027
![]() |
[2] |
M. Sridevi, C. Mala, A Survey on Monochrome Image Segmentation Methods, Procedia Technol., 6 (2012), 548-555. doi: 10.1016/j.protcy.2012.10.066
![]() |
[3] |
A. K. M. Khairuzzaman, S. Chaudhury, Multilevel thresholding using grey wolf optimizer for image segmentation, Expert Syst. Appl., 86 (2017), 64-76. doi: 10.1016/j.eswa.2017.04.029
![]() |
[4] |
J. Tang, Y. Wang, C. Huang, H. Liu, N. Al-Nabhan, Image edge detection based on singular value feature vector and gradient operator, Math. Biosci. Eng., 17 (2020), 3721-3735. doi: 10.3934/mbe.2020209
![]() |
[5] | X. Song, Y. Wang, Q. Feng, Q. Wang, Improved graph cut model with features of superpixels and neighborhood patches for myocardium segmentation from ultrasound image, Infinite Study, 2019. |
[6] |
X. Lu, Z. You, M. Sun, J. Wu, Z. Zhang, Breast cancer mitotic cell detection using cascade convolutional neural network with U-Net, Math. Biosci. Eng., 18 (2021), 673-695. doi: 10.3934/mbe.2021036
![]() |
[7] |
H. Jia, K. Sun, W. Song, X. Peng, C. Lang, Y. Li, Multi-Strategy Emperor Penguin Optimizer for RGB Histogram-Based Color Satellite Image Segmentation Using Masi Entropy, IEEE Access, 7 (2019), 134448-134474. doi: 10.1109/ACCESS.2019.2942064
![]() |
[8] | S. Wang, H. Jia, X. Peng, Modified salp swarm algorithm based multilevel thresholding for color image segmentation, Math. Biosci. Eng., 17 (2019), 700-724. |
[9] |
A. Dirami, K. Hammouche, M. Diaf, P. Siarry, Fast multilevel thresholding for image segmentation through a multiphase level set method, Signal Process., 93 (2013), 139-153. doi: 10.1016/j.sigpro.2012.07.010
![]() |
[10] |
E. Hamuda, M. Glavin, E. Jones, A survey of image processing techniques for plant extraction and segmentation in the field, Comput. Electron. Agric., 125 (2016), 184-199. doi: 10.1016/j.compag.2016.04.024
![]() |
[11] |
S. Kotte, R. K. Pullakura, S. K. Injeti, Optimal multilevel thresholding selection for brain MRI image segmentation based on adaptive wind driven optimization, Measurement, 130 (2018), 340-361. doi: 10.1016/j.measurement.2018.08.007
![]() |
[12] |
N. Otsu, A threshold selection method from gray-level histograms, IEEE Trans. Syst. Man Cybern., 9 (1979), 62-66. doi: 10.1109/TSMC.1979.4310076
![]() |
[13] |
J. N. Kapur, P. Sahoo, A. K. C. Wong, A new method for gray-level picture thresholding using the entropy of the histogram, Comput. Vis. Graph Image Process., 29 (1985), 273-285. doi: 10.1016/0734-189X(85)90125-2
![]() |
[14] |
A. K.Bhandari, V. K. Singh, A. Kumar, G. K. Singh, Cuckoo search algorithm and wind driven optimization based study of satellite image segmentation for multilevel thresholding using Kapur's entropy, Expert Syst. Appl., 41 (2014), 3538-3560. doi: 10.1016/j.eswa.2013.10.059
![]() |
[15] |
M. A. E. Aziz, A. A. Ewees, A. E. Hassanien, Whale Optimization Algorithm and Moth-Flame Optimization for multilevel thresholding image segmentation, Expert Syst. Appl., 83 (2017), 242-256. doi: 10.1016/j.eswa.2017.04.023
![]() |
[16] | K. P. Baby Resma, M. S. Nair, Multilevel thresholding for image segmentation using Krill Herd Optimization algorithm, J. King Saud Univ. Comput. Inf. Sci., (2018), forthcoming. |
[17] | A. Ibrahim, A. Ahmed, S. Hussein, A. E. Hassanien, Fish image segmentation using Salp Swarm Algorithm, in The International Conference on Advanced Machine Learning Technologies and Applications (AMLTA2018), Springer, (2018), 42-51. |
[18] |
S. Ouadfel, A. Taleb-Ahmed, Social spiders optimization and flower pollination algorithm for multilevel image thresholding: A performance study, Expert Syst. Appl., 55 (2016), 566-584. doi: 10.1016/j.eswa.2016.02.024
![]() |
[19] |
M. Díaz-Cortés, N. Ortega-Sánchez, S. Hinojosa, D. Oliva, E. Cuevas, R. Rojas, et al., A multi-level thresholding method for breast thermograms analysis using Dragonfly algorithm, Infrared Phys. Technol., 93 (2018), 346-361. doi: 10.1016/j.infrared.2018.08.007
![]() |
[20] |
S. C. Satapathy, N. Sri Madhava Raja, V. Rajinikanth, A. S. Ashour, N. Dey, Multi-level image thresholding using Otsu and chaotic bat algorithm, Neural Comput. Appl., 29 (2018), 1285-1307. doi: 10.1007/s00521-016-2645-5
![]() |
[21] |
M. Salvi, F. Molinari, Multi-tissue and multi-scale approach for nuclei segmentation in H & E stained images, BioMed. Eng. OnLine., 17 (2018), 89. doi: 10.1186/s12938-018-0518-0
![]() |
[22] |
Y. Feng, H. Zhao, X. Li, X. Zhang, H. Li, A multi-scale 3D Otsu thresholding algorithm for medical image segmentation, Digital Signal Process., 60 (2017), 186-199. doi: 10.1016/j.dsp.2016.08.003
![]() |
[23] |
D. Zhao, L. Liu, F. Yu, A. A. Heidari, M. Wang, G. Liang, et al., Chaotic random spare ant colony optimization for multi-threshold image segmentation of 2D Kapur entropy, Knowl. Based Syst., 216 (2021), 106510. doi: 10.1016/j.knosys.2020.106510
![]() |
[24] |
D. Zhao, L. Liu, F. Yu, A. A. Heidari, M. Wang, D. Oliva, et al., Ant colony optimization with horizontal and vertical crossover search: Fundamental visions for multi-threshold image segmentation, Expert Syst. Appl., 167 (2021), 114122. doi: 10.1016/j.eswa.2020.114122
![]() |
[25] |
L. He, S. Huang, An efficient krill herd algorithm for color image multilevel thresholding segmentation problem, Appl. Soft Comput., 89 (2020), 106063. doi: 10.1016/j.asoc.2020.106063
![]() |
[26] |
I. Hilali-Jaghdam, A. B. Ishak, S. Abdel-Khalek, A. Jamal, Quantum and classical genetic algorithms for multilevel segmentation of medical images: A comparative study, Comput. Commun., 162 (2020), 83-93. doi: 10.1016/j.comcom.2020.08.010
![]() |
[27] |
B. Wu, J. Zhou, X. Ji, Y. Yin, X. Shen, An ameliorated teaching-learning-based optimization algorithm based study of image segmentation for multilevel thresholding using Kapur's entropy and Otsu's between class variance, Inf. Sci., 533 (2020), 72-107. doi: 10.1016/j.ins.2020.05.033
![]() |
[28] |
S. Mirjalili, The Ant Lion Optimizer, Adv. Eng. Software, 83 (2015), 80-98. doi: 10.1016/j.advengsoft.2015.01.010
![]() |
[29] |
M. J. Hadidian-Moghaddam, S. Arabi-Nowdeh, M. Bigdeli, D. Azizian, A multi-objective optimal sizing and siting of distributed generation using ant lion optimization technique, Ain Shams Eng. J., 9 (2018), 2101-2109. doi: 10.1016/j.asej.2017.03.001
![]() |
[30] |
M. Raju, L. C. Saikia, N. Sinha, Automatic generation control of a multi-area system using ant lion optimizer algorithm based PID plus second order derivative controller, Int. J. Electr. Power Energy Syst., 80 (2016), 52-63. doi: 10.1016/j.ijepes.2016.01.037
![]() |
[31] |
P. Saxena, A. Kothari, Ant Lion Optimization algorithm to control side lobe level and null depths in linear antenna arrays, Int. J. Electron. Commun., 70 (2016), 1339-1349. doi: 10.1016/j.aeue.2016.07.008
![]() |
[32] |
E. Umamaheswari, S. Ganesan, M. Abirami, S. Subramanian, Cost Effective Integrated Maintenance Scheduling in Power Systems using Ant Lion Optimizer, Energy Procedia, 117 (2017), 501-508. doi: 10.1016/j.egypro.2017.05.176
![]() |
[33] |
P. D. P. Reddy, V. C. V. Reddy, T. G. Manohar, Ant Lion optimization algorithm for optimal sizing of renewable energy resources for loss reduction in distribution systems, J. Electr. Syst. Inf. Technol., 5 (2018), 663-680. doi: 10.1016/j.jesit.2017.06.001
![]() |
[34] |
D. Oliva, S. Hinojosa, M. A. Elaziz, N. Ortega-Sánchez, Context based image segmentation using antlion optimization and sine cosine algorithm, Multimedia Tools Appl., 77 (2018), 25761-25797. doi: 10.1007/s11042-018-5815-x
![]() |
[35] | C. Jin, Z. Ye, L. Yan, Y. Cao, A. Zhang, L. Ma, et al., Image Segmentation Using Fuzzy C-means Optimized by Ant Lion Optimization, in 2019 10th IEEE International Conference on Intelligent Data Acquisition and Advanced Computing Systems: Technology and Applications (IDAACS), IEEE, (2019), 388-393. |
[36] |
X. Yue, H. Zhang, A Novel Industrial Image Contrast Enhancement Technique Based on an Improved Ant Lion Optimizer, Arab J. Sci. Eng., 46 (2021), 3235-3246. doi: 10.1007/s13369-020-05148-4
![]() |
[37] |
Z. Wu, D. Yu, X. Kang, Parameter identification of photovoltaic cell model based on improved ant lion optimizer, Energy Convers. Manage., 151 (2017), 107-115. doi: 10.1016/j.enconman.2017.08.088
![]() |
[38] |
K. R. Subhashini, J. K. Satapathy, Development of an Enhanced Ant Lion Optimization Algorithm and its Application in Antenna Array Synthesis, Appl. Soft Comput., 59 (2017), 153-173. doi: 10.1016/j.asoc.2017.05.007
![]() |
[39] |
S. K. Majhi, S. Biswal, Optimal cluster analysis using hybrid K-Means and Ant Lion Optimizer, Karbala Int. J. Mod. Sci., 4 (2018), 347-360. doi: 10.1016/j.kijoms.2018.09.001
![]() |
[40] |
R. Sarkhel, N. Das, A. K. Saha, M. Nasipuri, An improved Harmony Search Algorithm embedded with a novel piecewise opposition based learning algorithm, Eng. Appl. Artif. Intell., 67 (2018), 317-330. doi: 10.1016/j.engappai.2017.09.020
![]() |
[41] |
A. A. Ewees, M. A. Elaziz, E. H. Houssein, Improved grasshopper optimization algorithm using opposition-based learning, Expert Syst. Appl., 112 (2018), 156-172. doi: 10.1016/j.eswa.2018.06.023
![]() |
[42] |
M. A. Ahandani, Opposition-based learning in the shuffled bidirectional differential evolution algorithm, Swarm Evol. Comput., 26 (2016), 64-85. doi: 10.1016/j.swevo.2015.08.002
![]() |
[43] | N. H. Awad, M. Z. Ali, P. N. Suganthan, Ensemble sinusoidal differential covariance matrix adaptation with Euclidean neighborhood for solving CEC2017 benchmark problems, in 2017 IEEE Congress on Evolutionary Computation (CEC), IEEE, (2017), 372-379. |
[44] |
R. Roy, S. Laha, Optimization of Stego image retaining secret information using genetic algorithm with 8-connected PSNR, Procedia Comput. Sci., 60 (2015), 468-477. doi: 10.1016/j.procs.2015.08.168
![]() |
[45] |
A. Tanchenko, Visual-PSNR measure of image quality, J. Visual Commun. Image Represent., 25 (2014), 874-878. doi: 10.1016/j.jvcir.2014.01.008
![]() |
[46] |
Z. Wang, A. C. Bovik, H. R. Sheikh, E. P. Simoncelli, Image quality assessment: from error visibility to structural similarity, IEEE Trans. Image Process., 13 (2004), 600-612. doi: 10.1109/TIP.2003.819861
![]() |
[47] |
V. Bruni, D. Vitulano, An entropy based approach for SSIM speed up, Signal Process., 135 (2017), 198-209. doi: 10.1016/j.sigpro.2017.01.007
![]() |
[48] |
C. Li, A. C. Bovik, Content-partitioned structural similarity index for image quality assessment, Signal Process. Image Commun., 25 (2010), 517-526. doi: 10.1016/j.image.2010.03.004
![]() |
[49] |
L. Zhang, L. Zhang, X. Mou, D. Zhang, FSIM: A feature similarity index for image quality assessment, IEEE Trans. Image Process., 20 (2011), 2378-2386. doi: 10.1109/TIP.2011.2109730
![]() |
[50] |
J. John, M. S. Nair, P. R. A. Kumar, M. Wilscy, A novel approach for detection and delineation of cell nuclei using feature similarity index measure, Biocybern. Biomed. Eng., 36 (2016), 76-88. doi: 10.1016/j.bbe.2015.11.002
![]() |
[51] |
S. K. Dinkar, K. Deep, Opposition based Laplacian Ant Lion Optimizer, J. Comput. Sci., 23 (2017), 71-90. doi: 10.1016/j.jocs.2017.10.007
![]() |
[52] |
M. Wang, X. Zhao, A. A. Heidari, H. Chen, Evaluation of constraint in photovoltaic models by exploiting an enhanced ant lion optimizer, Sol. Energy, 211 (2020), 503-521. doi: 10.1016/j.solener.2020.09.080
![]() |
[53] | The Berkeley Segmentation Dataset and Benchmark. Available from: https://www2.eecs.berkeley.edu/Research/Projects/CS/vision/bsds/. |
[54] |
H. Jia, X. Peng, W. Song, C. Lang, Z. Xing, K. Sun, Multiverse Optimization Algorithm Based on Lévy Flight Improvement for Multithreshold Color Image Segmentation, IEEE Access, 7 (2019), 32805-32844. doi: 10.1109/ACCESS.2019.2903345
![]() |
[55] |
A. K. M. Khairuzzaman, S. Chaudhury, Masi entropy based multilevel thresholding for image segmentation, Multimed. Tools Appl., 78 (2019), 33573-33591. doi: 10.1007/s11042-019-08117-8
![]() |
[56] |
A. K. Bhandari, A. Kumar, G. K. Singh, Modified artificial bee colony based computationally efficient multilevel thresholding for satellite image segmentation using Kapur's, Otsu and Tsallis functions, Expert Syst. Appl., 42 (2015), 1573-1601. doi: 10.1016/j.eswa.2014.09.049
![]() |
[57] |
S. Kotte, P. R. Kumar, S. K. Injeti, An efficient approach for optimal multilevel thresholding selection for gray scale images based on improved differential search algorithm, Ain Shams Eng. J., 9 (2018), 1043-1067. doi: 10.1016/j.asej.2016.06.007
![]() |
[58] |
V. K. Bohat, K. V. Arya, A new heuristic for multilevel thresholding of images, Expert Syst. Appl., 117 (2019), 176-203. doi: 10.1016/j.eswa.2018.08.045
![]() |
[59] |
A. K. Bhandari, A novel beta differential evolution algorithm-based fast multilevel thresholding for color image segmentation, Neural Comput. Appl., 32 (2020), 4583-4613. doi: 10.1007/s00521-018-3771-z
![]() |
[60] |
D. H. Wolpert, W. G. Macready, No free lunch theorems for optimization, IEEE Trans. Evol. Comput., 1 (1997), 67-82. doi: 10.1109/4235.585893
![]() |
1. | Jinhua You, Heming Jia, Di Wu, Honghua Rao, Changsheng Wen, Qingxin Liu, Laith Abualigah, Modified Artificial Gorilla Troop Optimization Algorithm for Solving Constrained Engineering Optimization Problems, 2023, 11, 2227-7390, 1256, 10.3390/math11051256 | |
2. | Rong Zheng, Abdelazim G. Hussien, He-Ming Jia, Laith Abualigah, Shuang Wang, Di Wu, An Improved Wild Horse Optimizer for Solving Optimization Problems, 2022, 10, 2227-7390, 1311, 10.3390/math10081311 | |
3. | Honghua Rao, Heming Jia, Di Wu, Changsheng Wen, Shanglong Li, Qingxin Liu, Laith Abualigah, A Modified Group Teaching Optimization Algorithm for Solving Constrained Engineering Optimization Problems, 2022, 10, 2227-7390, 3765, 10.3390/math10203765 | |
4. | Shikai Wang, Honghua Rao, Changsheng Wen, Heming Jia, Di Wu, Qingxin Liu, Laith Abualigah, Improved Remora Optimization Algorithm with Mutualistic Strategy for Solving Constrained Engineering Optimization Problems, 2022, 10, 2227-9717, 2606, 10.3390/pr10122606 | |
5. | Di Wu, Honghua Rao, Changsheng Wen, Heming Jia, Qingxin Liu, Laith Abualigah, Modified Sand Cat Swarm Optimization Algorithm for Solving Constrained Engineering Optimization Problems, 2022, 10, 2227-7390, 4350, 10.3390/math10224350 | |
6. | Di Wu, Shuang Wang, Qingxin Liu, Laith Abualigah, Heming Jia, Navid Razmjooy, An Improved Teaching-Learning-Based Optimization Algorithm with Reinforcement Learning Strategy for Solving Optimization Problems, 2022, 2022, 1687-5273, 1, 10.1155/2022/1535957 | |
7. | Laith Abualigah, Khaled H. Almotairi, Mohamed Abd Elaziz, Multilevel thresholding image segmentation using meta-heuristic optimization algorithms: comparative analysis, open challenges and new trends, 2022, 0924-669X, 10.1007/s10489-022-04064-4 | |
8. | Rong Zheng, Heming Jia, Laith Abualigah, Qingxin Liu, Shuang Wang, An improved arithmetic optimization algorithm with forced switching mechanism for global optimization problems, 2022, 19, 1551-0018, 473, 10.3934/mbe.2022023 | |
9. | Jie Xing, Hanli Zhao, Huiling Chen, Ruoxi Deng, Lei Xiao, Boosting Whale Optimizer with Quasi-Oppositional Learning and Gaussian Barebone for Feature Selection and COVID-19 Image Segmentation, 2023, 20, 1672-6529, 797, 10.1007/s42235-022-00297-8 | |
10. | Shuang Wang, Abdelazim G. Hussien, Heming Jia, Laith Abualigah, Rong Zheng, Enhanced Remora Optimization Algorithm for Solving Constrained Engineering Optimization Problems, 2022, 10, 2227-7390, 1696, 10.3390/math10101696 | |
11. | Arti Tiwari, Kamanasish Bhattacharjee, Millie Pant, Jana Nowakova, Vaclav Snasel, 2022, Chapter 27, 978-3-030-84909-2, 253, 10.1007/978-3-030-84910-8_27 | |
12. | Rong Zheng, Heming Jia, Laith Abualigah, Shuang Wang, Di Wu, An improved remora optimization algorithm with autonomous foraging mechanism for global optimization problems, 2022, 19, 1551-0018, 3994, 10.3934/mbe.2022184 | |
13. | Jie Xing, Xinsen Zhou, Hanli Zhao, Huiling Chen, Ali Asghar Heidari, Elite levy spreading differential evolution via ABC shrink-wrap for multi-threshold segmentation of breast cancer images, 2023, 82, 17468094, 104592, 10.1016/j.bspc.2023.104592 | |
14. | Essam H. Houssein, Doaa A. Abdelkareem, Marwa M. Emam, Mohamed Abdel Hameed, Mina Younan, An efficient image segmentation method for skin cancer imaging using improved golden jackal optimization algorithm, 2022, 149, 00104825, 106075, 10.1016/j.compbiomed.2022.106075 | |
15. | Hongmin Chen, Zhuo Wang, Heming Jia, Xindong Zhou, Laith Abualigah, Hybrid Slime Mold and Arithmetic Optimization Algorithm with Random Center Learning and Restart Mutation, 2023, 8, 2313-7673, 396, 10.3390/biomimetics8050396 | |
16. | D. Rasi, M. AntoBennet, P. N. Renjith, M. R. Arun, D. Vanathi, YOLO Based Deep Learning Model for Segmenting the Color Images, 2023, 11, 2347-470X, 359, 10.37391/ijeer.110217 | |
17. | Yijie Zhang, Yuhang Cai, Adaptive dynamic self-learning grey wolf optimization algorithm for solving global optimization problems and engineering problems, 2024, 21, 1551-0018, 3910, 10.3934/mbe.2024174 | |
18. | Laith Abualigah, Ali Diabat, Cuong-Le Thanh, Samir Khatir, Opposition-based Laplacian distribution with Prairie Dog Optimization method for industrial engineering design problems, 2023, 414, 00457825, 116097, 10.1016/j.cma.2023.116097 | |
19. | Shuang Wang, Heming Jia, Abdelazim G Hussien, Laith Abualigah, Guanjun Lin, Hongwei Wei, Zhenheng Lin, Krishna Gopal Dhal, Boosting aquila optimizer by marine predators algorithm for combinatorial optimization, 2024, 11, 2288-5048, 37, 10.1093/jcde/qwae004 | |
20. | Achraf Ben Miled, Mohammed Ahmed Elhossiny, Marwa Anwar Ibrahim Elghazawy, Ashraf F. A. Mahmoud, Faroug A. Abdalla, Enhanced Chaos Game Optimization for Multilevel Image Thresholding through Fitness Distance Balance Mechanism, 2024, 14, 1792-8036, 14945, 10.48084/etasr.7713 | |
21. | Xianwen Wang, Yingyuan Yang, Minhang Nan, Guanjun Bao, Guoyuan Liang, Optimum Multilevel Thresholding for Medical Brain Images Based on Tsallis Entropy, Incorporating Bayesian Estimation and the Cauchy Distribution, 2025, 15, 2076-3417, 2355, 10.3390/app15052355 |
Algorithm | Parameters | Value |
SSA | Balance coefficient c1 | [0, 2] |
Random number c2, c3 | [0, 1] | |
Switch possibility | 0.5 | |
MVO | Wormhole Existence Probability | [0.2, 1] |
Travelling Distance Rate | [0, 1] | |
Random number r1, r2, r3 | [0, 1] | |
DA | Inertial weight ω | [0.5, 0.9] |
Seperation weight | [0, 0.2] | |
Alignment weight | [0, 0.2] | |
Cohesion weight | [0, 0.2] | |
Food factor | [0, 2] | |
Enemy factor | [0, 0.1] | |
FPA | Switch possibility | 0.4 |
Lévy constant β | 1.5 | |
PSO | Maximum inertia weight | 0.9 |
Minimum inertia weight | 0.4 | |
Learning factors c1, c2 | 2 | |
Maximum velocity | +120 | |
Minimum velocity | −120 | |
SCA | Movement direction r1 | [0, 2] |
Movement distance r2 | [0, 2π] | |
Random weight r3 | [0, 2] | |
Random number r4 | [0, 1] | |
Switch possibility | 0.5 | |
ALO | Switch possibility | 0.5 |
MABC | Random number r | [0, 1] |
IDSA | Random number r | [0, 1] |
WOA-TH | Parameter a | [0, 2] |
Constant b | 1 | |
Random number l | [−1, 1] | |
Constant a0 | 13 | |
Initial value G0 | 40 | |
BDE | Number of objectives | 1 |
Number of constraints | 0 | |
Number of decision variables | 4 | |
Scaling factor | 0.5 | |
Crossover probability | 0.2 |
Algorithm 1. Pseudo code of MALO algorithm based multilevel thresholding. | |
1 | Input the image and calculate components of the histogram. |
2 | Initialize parameters: SearchAgents_no (the number of search agents), T (maximum number of iterations). |
3 | Calculate the fitness of initial ants and antlions; |
4 | Find the best antlions and assume it as the elite; |
5 | While t < T |
6 | For each ant |
7 | Select an antlion using Roulette wheel; |
8 | Update c and d using Eqs (16) and (17); |
9 | Create a random walk and normalize it using Eqs (11) and (13); |
10 | Update the position of ant using Eq (19); |
11 | End for |
12 | For each the position of ant |
13 | Generate the opposite solution of the position of ant using Eq (22); |
14 | Calculate the fitness of original position and opposite position of ant; |
15 | Compare fitness values and keep ant fitness and position with high fitness value; |
16 | End for |
17 | Update antlion positions and finesses based of the ants Eq (20); |
18 | Update the position of elite if any antlions becomes fitter than it; |
19 | t = t + 1; |
20 | End while |
21 | Return elite fitness and position, which elite position represents the optimal thresholds. |
22 | Output the values of the evaluation measures and the segmented images. |
F | SSA | MVO | DA | FPA | PSO | SCA | ALO | MALO | |
F1 | Mean | 2.7525 × 103 | 2.0775 × 104 | 5.3030 × 107 | 9.4246 × 107 | 2.3768 × 103 | 1.1165 × 109 | 1.6205 × 103 | 2.2691 × 102 |
Std | 3.1853 × 103 | 1.0204 × 104 | 1.3048 × 108 | 1.6194 × 108 | 3.3853 × 103 | 2.6690 × 108 | 1.6927 × 103 | 7.5223 × 102 | |
F3 | Mean | 3.0002 × 102 | 3.0015 × 102 | 3.3792 × 103 | 5.6230 × 103 | 3.0009 × 102 | 3.0174 × 103 | 4.5012 × 102 | 4.4652 × 102 |
Std | 8.4151 × 10−2 | 7.5858 × 10−2 | 3.5353 × 103 | 3.7208 × 103 | 7.7137 × 10−2 | 1.6524 × 103 | 3.7837 × 102 | 3.1829 × 102 | |
F4 | Mean | 4.0989 × 102 | 4.0702 × 102 | 4.2856 × 102 | 4.6977 × 102 | 4.0507 × 102 | 4.6709 × 102 | 4.1395 × 102 | 4.0394 × 102 |
Std | 16.908 | 9.3281 | 29.903 | 61.297 | 1.2334 | 30.876 | 22.338 | 12.248 | |
F5 | Mean | 5.2912 × 102 | 5.2264 × 102 | 5.1732 × 102 | 5.6206 × 102 | 5.4292 × 102 | 5.5799 × 102 | 5.2180 × 102 | 5.1692 × 102 |
Std | 12.497 | 1.1788 × 10 | 8.4145 | 21.327 | 14.890 | 6.6091 | 13.640 | 2.8811 | |
F6 | Mean | 6.1533 × 102 | 6.0237 × 102 | 6.0125 × 102 | 6.3945 × 102 | 6.1021 × 102 | 6.2361 × 102 | 6.1562 × 102 | 6.7956 × 102 |
Std | 8.9433 | 3.1079 | 1.4272 | 16.075 | 8.8947 | 5.4162 | 8.8988 | 10.159 | |
F7 | Mean | 7.4500 × 102 | 7.3257 × 102 | 7.3766 × 102 | 7.9322 × 102 | 7.3056 × 102 | 7.8595 × 102 | 7.5048 × 102 | 7.2403 × 102 |
Std | 15.007 | 12.483 | 14.458 | 26.384 | 12.387 | 10.193 | 26.572 | 2.3596 | |
F8 | Mean | 8.2630 × 102 | 8.2696 × 102 | 8.1554 × 102 | 8.4529 × 102 | 8.2131 × 102 | 8.4537 × 102 | 8.2771× 102 | 8.1001 × 102 |
Std | 11.690 | 12.498 | 7.2712 | 16.236 | 7.9025 | 8.7128 | 14.163 | 9.4471 | |
F9 | Mean | 1.0897 × 103 | 9.0116 × 102 | 9.2457 × 102 | 1.7066 × 103 | 9.6607 × 102 | 1.0671 × 103 | 1.1272 × 103 | 1.0417 × 103 |
Std | 2.5134 × 102 | 2.8801 | 51.037 | 6.1484 × 102 | 1.1193 × 102 | 81.732 | 2.2796 × 102 | 1.8741 × 102 | |
F10 | Mean | 1.8446 × 103 | 1.7723 × 103 | 1.7989 × 103 | 2.1907 × 103 | 1.9995 × 103 | 2.5054 × 103 | 2.0639 × 103 | 1.2226 × 103 |
Std | 2.8235 × 102 | 2.8547 × 102 | 3.4047 × 102 | 3.9008 × 102 | 2.9524 × 102 | 2.0358 × 102 | 3.1110 × 102 | 1.7148 × 102 | |
F11 | Mean | 1.1840 × 103 | 1.1325 × 103 | 1.2913 × 103 | 1.2604 × 103 | 1.1388 × 103 | 1.2494 × 103 | 1.2125 × 103 | 9.3895 × 104 |
Std | 71.184 | 27.254 | 8.0087 × 102 | 1.1285 × 102 | 2.7432 × 105 | 76.249 | 74.386 | 24.745 | |
F12 | Mean | 3.1659 × 106 | 1.9237 × 106 | 8.5333 × 105 | 5.3131 × 106 | 2.8460 × 109 | 3.6304 × 107 | 2.9647 × 106 | 2.2571 × 104 |
Std | 3.9918 × 106 | 2.4011 × 106 | 1.0164 × 106 | 5.2872 × 106 | 1.4432 × 109 | 4.6673 × 107 | 3.6635 × 106 | 3.1055 × 104 | |
F13 | Mean | 1.7372 × 104 | 1.2349 × 104 | 1.6552 × 104 | 2.3449 × 104 | 3.2613 × 108 | 8.9513 × 104 | 1.3896 × 104 | 1.1517 × 104 |
Std | 1.2611 × 104 | 9.7419 × 103 | 9.0887 × 103 | 1.8794 × 104 | 2.7709 × 108 | 8.0007 × 104 | 1.2201 × 104 | 7.8833 × 103 | |
F14 | Mean | 3.3621 × 103 | 3.0338 × 103 | 4.8484 × 103 | 2.8067 × 103 | 2.8688 × 103 | 2.3311 × 103 | 2.6070 × 103 | 2.3278 × 103 |
Std | 2.7150 × 103 | 2.3148 × 103 | 1.8854 × 103 | 1.4364 × 103 | 2.1263 × 103 | 1.0895 × 103 | 1.6260 × 103 | 1.2880 × 103 | |
F15 | Mean | 6.2001 × 103 | 3.3924 × 103 | 6.4284 × 103 | 9.6586 × 103 | 3.9125 × 103 | 3.7996 × 103 | 1.3375 × 104 | 3.0564 × 103 |
Std | 4.2086 × 103 | 3.5797 × 103 | 5.0026 × 103 | 7.7162 × 103 | 3.7024 × 103 | 2.3791 × 103 | 1.0871 × 104 | 2.1255 × 103 | |
F16 | Mean | 1.8063 × 103 | 1.8309 × 103 | 1.7338 × 103 | 1.9840 × 103 | 1.8428 × 103 | 1.8126 × 103 | 1.8928 × 103 | 1.8066 × 103 |
Std | 1.4760 × 102 | 1.6725 × 102 | 1.2125 × 102 | 1.4716 × 102 | 1.2492 × 102 | 80.085 | 1.6074 × 102 | 1.0356 × 102 | |
F17 | Mean | 1.7831 × 103 | 1.7871 × 103 | 1.7693 × 103 | 1.8044 × 103 | 1.7796 × 103 | 1.7897 × 103 | 1.7795 × 103 | 1.7359 × 103 |
Std | 38.227 | 56.931 | 33.562 | 52.636 | 59.130 | 14.372 | 39.757 | 34.260 | |
F18 | Mean | 1.9046 × 104 | 1.7527 × 104 | 2.4433 × 104 | 1.5703 × 104 | 1.6595 × 104 | 4.2187 × 105 | 1.6102 × 104 | 1.6102 × 104 |
Std | 1.3350 × 104 | 1.1493 × 104 | 1.5706 × 104 | 1.2231 × 104 | 1.3320 × 104 | 4.6787 × 105 | 1.3045 × 104 | 1.2885 × 104 | |
F19 | Mean | 9.3321 × 103 | 4.8206 × 103 | 1.1236 × 104 | 9.2778 × 104 | 5.1293 × 103 | 1.0034 × 104 | 1.6927 × 104 | 1.9544 × 103 |
Std | 7.1553 × 103 | 4.0080 × 103 | 6.8870 × 103 | 2.0401 × 105 | 3.8240 × 103 | 8.8662 × 103 | 1.2359 × 104 | 3.1306 × 103 | |
F20 | Mean | 2.1580 × 103 | 2.1181 × 103 | 2.1072 × 103 | 2.1974 × 103 | 2.1351 × 103 | 2.1393 × 103 | 2.1520 × 103 | 2.1017 × 103 |
Std | 77.357 | 71.982 | 59.164 | 81.489 | 81.620 | 37.232 | 76.695 | 1.1244 × 102 | |
F21 | Mean | 2.2892 × 103 | 2.3067 × 103 | 2.3146 × 103 | 2.3260 × 103 | 2.3033 × 103 | 2.2951 × 103 | 2.3181 × 103 | 2.2919 × 103 |
Std | 58.652 | 43.950 | 23.354 | 56.359 | 69.965 | 67.337 | 23.092 | 23.025 | |
F22 | Mean | 2.3032 × 103 | 2.4325 × 103 | 2.3387 × 103 | 2.3737 × 103 | 2.3168 × 103 | 2.3923 × 103 | 2.3019 × 103 | 2.2108 × 103 |
Std | 1.5330 | 4.0570 × 102 | 1.5916 × 102 | 3.0758 × 102 | 86.302 | 36.970 | 14.139 | 4.3619 | |
F23 | Mean | 2.6246 × 103 | 2.6213 × 103 | 2.6263 × 103 | 2.6544 × 103 | 2.6993 × 103 | 2.6630 × 103 | 2.6330 × 103 | 2.6235 × 103 |
Std | 9.4651 | 8.4456 | 11.398 | 27.680 | 38.621 | 9.4154 | 13.016 | 8.6164 | |
F24 | Mean | 2.7448 × 103 | 2.7399E+03 | 2.7422E+03 | 2.7726E+03 | 2.7821E+03 | 2.7773E+03 | 2.7541E+03 | 2.7242E+03 |
Std | 48.315 | 45.915 | 47.575 | 55.733 | 1.1838 × 102 | 59.112 | 13.296 | 10.259 | |
F25 | Mean | 2.9316 × 103 | 2.9322 × 103 | 2.9355 × 103 | 2.9755 × 103 | 2.9227 × 103 | 2.9816 × 103 | 2.9352 × 103 | 2.9316 × 103 |
Std | 30.687 | 28.163 | 23.579 | 53.181 | 23.221 | 24.991 | 28.272 | 2.7704 × 102 | |
F26 | Mean | 2.9852 × 103 | 3.0412 × 103 | 3.1910 × 103 | 3.5113 × 103 | 3.2078 × 103 | 3.1238 × 103 | 3.0689 × 103 | 3.0597 × 103 |
Std | 2.7007 × 102 | 3.8042 × 102 | 3.9782 × 102 | 4.9123 × 102 | 5.0084 × 102 | 37.746 | 2.7801 × 102 | 2.5490 × 102 | |
F27 | Mean | 3.0942 × 103 | 3.0936 × 103 | 3.0975 × 103 | 3.1538 × 103 | 3.1493 × 103 | 3.1084 × 103 | 3.1028 × 103 | 3.0346 × 103 |
Std | 3.6648 | 3.4486 | 6.0913 | 55.897 | 67.675 | 4.4917 | 15.597 | 1.4249 | |
F28 | Mean | 3.3187 × 103 | 3.3510 × 103 | 3.3579 × 103 | 3.4196 × 103 | 3.2028 × 103 | 3.3398 × 103 | 3.3863 × 103 | 3.1170 × 103 |
Std | 1.6379 × 102 | 1.3529 × 102 | 95.702 | 1.6486 × 102 | 78.068 | 90.179 | 1.3401 × 102 | 1.0508 × 102 | |
F29 | Mean | 3.2573 × 103 | 3.2282 × 103 | 3.2212 × 103 | 3.4234 × 103 | 3.2563 × 103 | 3.2629 × 103 | 3.2707 × 103 | 3.1694 × 103 |
Std | 81.947 | 83.366 | 55.368 | 1.3273 × 102 | 82.282 | 33.745 | 94.979 | 22.526 | |
F30 | Mean | 5.4626 × 105 | 5.5507 × 105 | 1.0112 × 106 | 2.2409 × 106 | 6.8210 × 104 | 1.7827 × 106 | 7.0103 × 105 | 1.5116 × 104 |
Std | 5.8213 × 105 | 6.5747 × 105 | 1.3632 × 106 | 2.9983 × 106 | 6.4354 × 104 | 1.3111 × 106 | 2.1161 × 106 | 7.2776 × 104 |
Image | K | SSA | MVO | DA | FPA | PSO | SCA | ALO | MALO |
Cactus | 4 | 2126.2412 | 2126.2412 | 2126.2412 | 2084.2192 | 2126.2085 | 2091.2043 | 2126.1077 | 2126.2412 |
6 | 2188.8026 | 2188.7881 | 2188.7749 | 2152.9126 | 2180.5976 | 2151.3710 | 2188.6529 | 2188.8076 | |
8 | 2213.1588 | 2213.1207 | 2210.6763 | 2185.1975 | 2206.7101 | 2177.4155 | 2212.8736 | 2213.2108 | |
10 | 2224.0745 | 2224.9921 | 2224.4972 | 2202.9660 | 2224.8409 | 2205.3385 | 2223.8535 | 2225.0378 | |
12 | 2228.0349 | 2231.4043 | 2227.1859 | 2208.4757 | 2230.3736 | 2209.0205 | 2230.9917 | 2231.8810 | |
Kangaroo | 4 | 1114.7964 | 1114.7964 | 1114.7964 | 1088.7665 | 1114.7783 | 1088.6203 | 1114.7451 | 1114.7964 |
6 | 1164.7339 | 1164.7235 | 1162.2148 | 1131.7231 | 1157.9995 | 1142.5799 | 1164.5055 | 1164.7463 | |
8 | 1186.9998 | 1187.2317 | 1186.4981 | 1164.0753 | 1181.4079 | 1157.1404 | 1187.0901 | 1187.3921 | |
10 | 1195.5800 | 1198.8061 | 1198.1305 | 1175.6658 | 1195.7200 | 1172.3154 | 1197.6616 | 1199.0721 | |
12 | 1201.3621 | 1204.3553 | 1204.0964 | 1189.4752 | 1203.7897 | 1186.9269 | 1204.8168 | 1205.6190 | |
Temple | 4 | 1510.8080 | 1511.3099 | 1511.3110 | 1481.4555 | 1511.2952 | 1484.9151 | 1511.1720 | 1511.3110 |
6 | 1562.1584 | 1551.2497 | 1562.1657 | 1539.3204 | 1547.2460 | 1528.3151 | 1562.0323 | 1562.1677 | |
8 | 1575.4345 | 1581.9379 | 1580.1512 | 1558.9731 | 1577.2711 | 1552.2760 | 1578.5870 | 1582.0010 | |
10 | 1585.0462 | 1589.3769 | 1588.9537 | 1566.9471 | 1583.4601 | 1562.6224 | 1589.3855 | 1590.0709 | |
12 | 1593.4902 | 1592.3625 | 1594.7102 | 1577.6113 | 1589.2313 | 1577.5018 | 1595.2350 | 1596.7043 | |
Flower | 4 | 2319.2628 | 2319.2628 | 2319.2628 | 2300.8905 | 2319.2628 | 2305.0308 | 2319.2088 | 2319.2628 |
6 | 2373.7148 | 2373.9784 | 2373.9666 | 2349.7530 | 2366.7255 | 2348.8170 | 2373.7751 | 2374.0062 | |
8 | 2398.3260 | 2397.7703 | 2398.6865 | 2373.7415 | 2393.6744 | 2378.1142 | 2398.0974 | 2398.7056 | |
10 | 2408.6487 | 2409.1083 | 2410.1377 | 2392.7685 | 2409.5320 | 2393.4470 | 2409.9581 | 2410.1748 | |
12 | 2415.5456 | 2416.1915 | 2413.2197 | 2401.7458 | 2413.6013 | 2402.8505 | 2416.6995 | 2417.4773 | |
Mountain | 4 | 1922.6304 | 1922.6304 | 1922.6304 | 1880.8725 | 1922.6304 | 1899.0075 | 1922.5604 | 1922.6304 |
6 | 1966.3537 | 1969.5924 | 1969.5227 | 1950.3434 | 1964.7413 | 1937.2954 | 1969.3259 | 1969.6134 | |
8 | 1987.6882 | 1987.6512 | 1987.6006 | 1971.0006 | 1982.4353 | 1963.1189 | 1987.3940 | 1989.0684 | |
10 | 1998.2296 | 1998.3989 | 1999.2217 | 1976.2590 | 1995.3262 | 1980.4772 | 1998.6023 | 1999.5736 | |
12 | 2002.3212 | 2003.5176 | 2003.4479 | 1991.7908 | 2002.3326 | 1987.1898 | 2003.1927 | 2004.4332 | |
Tree | 4 | 4836.5287 | 4836.5254 | 4836.5287 | 4811.7023 | 4836.5184 | 4799.5909 | 4836.4226 | 4836.5287 |
6 | 4910.3426 | 4910.3170 | 4910.3328 | 4881.3483 | 4899.1851 | 4889.6357 | 4909.9888 | 4910.3389 | |
8 | 4938.5519 | 4938.7280 | 4939.0548 | 4895.7628 | 4932.5126 | 4897.8287 | 4939.0439 | 4939.2738 | |
10 | 4954.4191 | 4954.3250 | 4954.3494 | 4931.0184 | 4950.5741 | 4925.6878 | 4953.6297 | 4954.7408 | |
12 | 4961.5040 | 4962.0370 | 4961.7003 | 4947.5680 | 4958.4948 | 4933.2448 | 4961.9255 | 4962.8681 | |
Horse | 4 | 4169.9500 | 4169.9500 | 4169.9475 | 4146.0648 | 4169.9500 | 4125.8875 | 4169.9152 | 4169.9500 |
6 | 4223.2790 | 4226.2869 | 4226.2925 | 4206.4679 | 4226.2960 | 4184.3739 | 4226.1384 | 4226.2981 | |
8 | 4251.0736 | 4247.9571 | 4250.7202 | 4224.2255 | 4242.3477 | 4224.8735 | 4251.0627 | 4251.0806 | |
10 | 4264.1067 | 4264.0484 | 4263.6881 | 4245.5168 | 4263.0609 | 4242.4543 | 4263.8776 | 4264.5464 | |
12 | 4271.0552 | 4270.2470 | 4270.4589 | 4253.0366 | 4270.9270 | 4255.1561 | 4269.9287 | 4272.7303 | |
Bridge | 4 | 4386.0155 | 4386.0155 | 4386.0155 | 4348.0212 | 4386.0155 | 4364.6402 | 4385.8679 | 4386.0155 |
6 | 4448.8450 | 4456.6582 | 4456.6941 | 4430.7839 | 4456.6543 | 4416.6522 | 4448.6228 | 4456.6941 | |
8 | 4483.9318 | 4476.1230 | 4481.9799 | 4459.7426 | 4480.7550 | 4436.7087 | 4480.4869 | 4484.0957 | |
10 | 4495.8304 | 4494.1137 | 4497.7496 | 4475.7876 | 4494.2483 | 4472.8251 | 4497.6619 | 4498.1770 | |
12 | 4505.0626 | 4504.6934 | 4502.9179 | 4488.9690 | 4504.8794 | 4485.6536 | 4504.6902 | 4506.5159 | |
Pilot | 4 | 3854.8970 | 3854.8970 | 3854.8970 | 3838.0151 | 3854.8930 | 3807.6575 | 3854.8127 | 3854.8970 |
6 | 3902.7853 | 3896.5334 | 3902.7747 | 3884.5667 | 3902.7796 | 3869.1994 | 3902.6187 | 3902.7731 | |
8 | 3917.0447 | 3917.7421 | 3920.6198 | 3892.5037 | 3918.0121 | 3895.6690 | 3920.4228 | 3923.5580 | |
10 | 3931.0115 | 3928.4714 | 3933.5071 | 3915.8877 | 3931.1832 | 3904.4969 | 3932.5873 | 3934.2915 | |
12 | 3937.8965 | 3936.9459 | 3936.6517 | 3916.5100 | 3935.4087 | 3916.0317 | 3939.2200 | 3940.3098 | |
Dog | 4 | 2249.9855 | 2249.9839 | 2249.9855 | 2231.2675 | 2249.9855 | 2233.1946 | 2249.9484 | 2249.9855 |
6 | 2299.7269 | 2299.6899 | 2298.6466 | 2270.5848 | 2287.9683 | 2277.7186 | 2299.5470 | 2299.7446 | |
8 | 2320.5275 | 2317.0263 | 2320.4939 | 2297.8192 | 2303.3155 | 2296.9113 | 2320.2281 | 2320.5319 | |
10 | 2326.4485 | 2329.6067 | 2330.9585 | 2311.9971 | 2328.1692 | 2306.4227 | 2330.4593 | 2330.9965 | |
12 | 2334.7496 | 2336.2099 | 2334.3909 | 2323.5986 | 2332.0819 | 2320.2905 | 2335.7878 | 2337.4348 |
Image | K | SSA | MVO | DA | FPA | PSO | SCA | ALO | MALO |
Cactus | 4 | 18.5843 | 18.5843 | 18.5843 | 18.3930 | 18.5829 | 18.4259 | 18.5832 | 18.5843 |
6 | 23.8329 | 23.8233 | 23.8386 | 23.2412 | 23.8389 | 23.2857 | 23.8364 | 23.8420 | |
8 | 28.4940 | 28.3247 | 28.5120 | 27.0680 | 28.4911 | 27.3938 | 28.4814 | 28.5198 | |
10 | 32.6224 | 32.0489 | 32.7875 | 30.4517 | 32.8282 | 30.7148 | 32.7883 | 32.8463 | |
12 | 36.7255 | 35.3654 | 36.6688 | 34.7456 | 36.7983 | 34.2975 | 36.6721 | 36.8450 | |
Kangaroo | 4 | 18.9363 | 18.9353 | 18.9361 | 18.6549 | 18.9352 | 18.7771 | 18.9350 | 18.9363 |
6 | 24.4683 | 24.4414 | 24.4720 | 23.6425 | 24.4648 | 24.0457 | 24.4563 | 24.4753 | |
8 | 29.3790 | 29.2133 | 29.3699 | 28.7933 | 29.4014 | 28.2712 | 29.4026 | 29.4202 | |
10 | 33.8064 | 33.4514 | 33.8602 | 32.6267 | 33.1534 | 32.0603 | 33.8653 | 33.9054 | |
12 | 37.8707 | 37.1153 | 37.9876 | 36.2125 | 38.0001 | 35.4540 | 37.9065 | 38.0410 | |
Temple | 4 | 17.8159 | 17.8201 | 17.8061 | 17.4725 | 17.8046 | 17.5013 | 17.8195 | 17.8204 |
6 | 22.9388 | 22.7544 | 22.9388 | 22.2007 | 22.8940 | 22.0680 | 22.9246 | 22.9388 | |
8 | 27.5032 | 27.2493 | 27.4436 | 25.9640 | 27.4909 | 25.5465 | 27.5208 | 27.5351 | |
10 | 31.6211 | 30.6718 | 31.6425 | 29.5279 | 31.6160 | 28.9053 | 31.6706 | 31.7574 | |
12 | 34.2789 | 33.0925 | 35.3472 | 31.7622 | 34.7704 | 32.2631 | 35.3818 | 35.6116 | |
Flower | 4 | 18.7000 | 18.6993 | 18.7000 | 18.4447 | 18.6996 | 18.4765 | 18.6980 | 18.7005 |
6 | 24.0481 | 24.0186 | 24.0485 | 23.6039 | 24.0429 | 23.3247 | 24.0441 | 24.0511 | |
8 | 28.7647 | 28.6438 | 28.7955 | 27.6104 | 28.7721 | 27.7280 | 28.7727 | 28.8057 | |
10 | 33.0270 | 32.9200 | 33.1672 | 31.2963 | 33.1582 | 31.3512 | 33.1673 | 33.1976 | |
12 | 36.8699 | 35.9637 | 37.1214 | 34.8362 | 37.0372 | 34.9773 | 36.9291 | 37.1549 | |
Mountain | 4 | 17.7231 | 17.7035 | 17.7213 | 17.5421 | 17.7372 | 17.4362 | 17.7390 | 17.7213 |
6 | 23.1283 | 23.0802 | 23.0902 | 22.5402 | 23.1001 | 22.4117 | 23.1173 | 23.1119 | |
8 | 27.9637 | 27.6405 | 27.9315 | 26.6905 | 27.9354 | 26.4147 | 27.9302 | 27.9679 | |
10 | 32.1404 | 31.9221 | 32.3451 | 30.9813 | 32.2561 | 30.0080 | 32.3415 | 32.4426 | |
12 | 36.3399 | 34.9350 | 36.3823 | 34.0005 | 35.6920 | 33.6967 | 36.1384 | 36.4707 | |
Tree | 4 | 18.9965 | 18.9962 | 18.9965 | 18.8465 | 18.9961 | 18.8254 | 18.9953 | 18.9965 |
6 | 24.3933 | 24.4095 | 24.4100 | 23.8731 | 24.3889 | 23.4245 | 24.4083 | 24.4149 | |
8 | 29.2846 | 28.9004 | 29.2918 | 27.8265 | 29.2753 | 27.6207 | 29.2581 | 29.2936 | |
10 | 33.5397 | 33.0809 | 33.6613 | 31.4879 | 33.6439 | 31.7828 | 33.5491 | 33.6785 | |
12 | 37.3776 | 36.7041 | 37.4570 | 35.2858 | 37.5764 | 34.8717 | 37.5111 | 37.6312 | |
Horse | 4 | 18.6614 | 18.6259 | 18.6230 | 18.3914 | 18.6252 | 18.5251 | 18.6614 | 18.6619 |
6 | 24.0609 | 23.8743 | 24.0802 | 23.2431 | 24.0604 | 22.8699 | 24.0675 | 24.0806 | |
8 | 28.8403 | 28.5613 | 28.8907 | 27.9542 | 28.8416 | 27.6730 | 28.8914 | 28.8992 | |
10 | 33.2142 | 32.3696 | 33.2125 | 31.1950 | 33.2741 | 30.7256 | 33.2480 | 33.3135 | |
12 | 36.8467 | 35.6548 | 37.0718 | 34.9857 | 37.0695 | 33.8890 | 37.1782 | 37.3216 | |
Bridge | 4 | 18.1906 | 18.1436 | 18.1902 | 18.0033 | 18.1903 | 18.0580 | 18.1903 | 18.1907 |
6 | 23.5631 | 23.5510 | 23.5679 | 22.8880 | 23.5610 | 22.8246 | 23.5649 | 23.5682 | |
8 | 28.2604 | 27.9986 | 28.2930 | 27.0830 | 28.2859 | 26.7516 | 28.2786 | 28.3023 | |
10 | 32.5876 | 32.3366 | 32.5480 | 30.9047 | 32.5740 | 30.5593 | 32.5281 | 32.6189 | |
12 | 36.0703 | 35.4806 | 36.4991 | 33.5753 | 36.4588 | 33.0662 | 36.4400 | 36.5351 | |
Pilot | 4 | 17.9368 | 17.9352 | 17.9362 | 17.6027 | 17.9362 | 17.7058 | 17.9355 | 17.9168 |
6 | 23.0182 | 22.9648 | 23.0443 | 21.9322 | 23.0365 | 21.8927 | 23.0025 | 23.0447 | |
8 | 27.6539 | 27.4187 | 27.6678 | 26.2966 | 27.6452 | 25.7373 | 27.6307 | 27.6723 | |
10 | 31.6001 | 30.3305 | 31.7457 | 30.5445 | 31.7701 | 29.3977 | 31.7568 | 31.8639 | |
12 | 35.3121 | 34.3457 | 35.4310 | 32.7740 | 35.6276 | 31.7852 | 35.5945 | 35.7388 | |
Dog | 4 | 18.5258 | 18.5246 | 18.4850 | 18.2690 | 18.5254 | 18.2825 | 18.5244 | 18.5261 |
6 | 23.6922 | 23.6586 | 23.7386 | 22.7559 | 23.7121 | 22.7675 | 23.7319 | 23.7420 | |
8 | 28.3767 | 28.0194 | 28.3553 | 27.0852 | 28.3862 | 26.7027 | 28.3751 | 28.4079 | |
10 | 32.4951 | 31.5916 | 32.6709 | 31.2171 | 32.6231 | 29.8432 | 32.6604 | 32.6944 | |
12 | 36.4563 | 36.2405 | 36.3900 | 34.1968 | 35.8826 | 33.0948 | 36.5074 | 36.6079 |
Image | K | SSA | MVO | DA | FPA | PSO | SCA | ALO | MALO |
Cactus | 4 | 0.4100 | 0.8700 | 1.8700 | 0.6900 | 0.7700 | 0.7100 | 1.4050 | 1.7200 |
6 | 0.2500 | 0.7000 | 1.4900 | 0.6800 | 0.6700 | 0.6500 | 1.8700 | 2.1455 | |
8 | 0.2810 | 0.7800 | 1.5200 | 0.6800 | 0.7200 | 0.6800 | 2.3850 | 2.6000 | |
10 | 0.3200 | 0.7800 | 1.5600 | 0.7300 | 0.7600 | 0.7100 | 2.9545 | 3.2550 | |
12 | 0.3400 | 1.0600 | 1.6890 | 0.8630 | 0.9000 | 0.8400 | 4.8515 | 4.3270 | |
Kangaroo | 4 | 0.5050 | 0.8100 | 1.8420 | 0.6700 | 0.7300 | 0.9580 | 1.4200 | 1.5505 |
6 | 0.2690 | 0.7300 | 1.5200 | 0.6800 | 0.7600 | 0.6500 | 1.9600 | 2.1995 | |
8 | 0.3100 | 0.8300 | 1.6410 | 0.7010 | 0.7500 | 0.7400 | 2.4345 | 2.7155 | |
10 | 0.3400 | 0.8500 | 1.6000 | 0.7800 | 0.8200 | 0.7400 | 3.0750 | 3.3250 | |
12 | 0.3700 | 0.8400 | 1.6000 | 0.7800 | 0.8300 | 0.7900 | 3.7580 | 3.8305 | |
Temple | 4 | 0.3700 | 0.8540 | 1.9250 | 0.6530 | 1.3210 | 0.6510 | 1.4300 | 1.5605 |
6 | 0.2700 | 0.7600 | 1.5690 | 0.6500 | 0.7390 | 0.6510 | 1.9200 | 2.1755 | |
8 | 0.3000 | 0.8100 | 1.5800 | 0.7400 | 0.7600 | 0.6890 | 3.0805 | 3.5605 | |
10 | 0.4400 | 1.3800 | 2.3500 | 1.0690 | 0.7600 | 0.8100 | 3.7715 | 4.9285 | |
12 | 0.3600 | 0.8600 | 1.7200 | 0.7710 | 0.8000 | 0.7300 | 3.5765 | 4.2245 | |
Flower | 4 | 0.4250 | 1.1510 | 2.8120 | 0.8400 | 0.8710 | 0.8550 | 2.1855 | 2.2690 |
6 | 0.2700 | 0.7900 | 1.6000 | 0.7200 | 0.7700 | 0.7400 | 2.0005 | 3.2575 | |
8 | 0.3860 | 0.9860 | 2.5490 | 1.5090 | 0.8630 | 0.7330 | 4.1070 | 4.4885 | |
10 | 0.8540 | 1.6520 | 2.4080 | 0.9010 | 1.3190 | 1.5640 | 4.8340 | 4.6935 | |
12 | 0.5600 | 1.1700 | 2.1900 | 1.1300 | 0.8700 | 1.0000 | 3.7055 | 4.0505 | |
Mountain | 4 | 0.4700 | 1.1750 | 2.8030 | 0.7110 | 0.9170 | 0.8500 | 2.2840 | 2.2155 |
6 | 0.5170 | 0.9080 | 2.4520 | 0.7370 | 0.8370 | 0.7510 | 2.5625 | 2.1245 | |
8 | 0.2920 | 0.8200 | 1.7150 | 0.6800 | 0.9710 | 0.8810 | 3.3625 | 2.8755 | |
10 | 0.4300 | 0.8200 | 3.4100 | 0.7100 | 0.8010 | 0.8800 | 5.0305 | 4.2160 | |
12 | 0.4000 | 0.8820 | 1.5710 | 0.7900 | 1.1500 | 0.8550 | 4.4010 | 3.9205 | |
Tree | 4 | 0.4500 | 0.9200 | 1.9700 | 0.9800 | 1.5640 | 0.7900 | 1.6595 | 2.3955 |
6 | 0.4300 | 1.0950 | 2.6590 | 1.7460 | 1.0490 | 1.6700 | 3.1700 | 3.5230 | |
8 | 0.3410 | 1.4480 | 2.8000 | 0.9750 | 0.7530 | 0.6930 | 2.6675 | 3.7390 | |
10 | 0.3740 | 1.2760 | 2.3920 | 0.8310 | 0.8920 | 0.8130 | 3.6270 | 3.5155 | |
12 | 0.4500 | 0.9390 | 0.9390 | 0.9390 | 0.8990 | 0.8510 | 3.9570 | 5.2985 | |
Horse | 4 | 0.4040 | 0.8970 | 1.8600 | 0.6840 | 0.7480 | 0.9190 | 1.7475 | 1.8105 |
6 | 0.3140 | 0.8380 | 1.6460 | 0.8150 | 0.7450 | 0.6850 | 2.0365 | 2.2195 | |
8 | 0.3010 | 1.0490 | 1.8420 | 0.7300 | 0.8770 | 0.7740 | 3.2820 | 2.9730 | |
10 | 0.3300 | 0.8560 | 1.6410 | 0.7780 | 0.8540 | 0.7920 | 3.7315 | 3.8360 | |
12 | 0.3920 | 0.9120 | 1.7110 | 1.6600 | 1.6600 | 1.0670 | 4.2670 | 4.8615 | |
Bridge | 4 | 0.4200 | 1.9550 | 2.5860 | 1.7110 | 0.9140 | 0.7110 | 1.6845 | 1.8555 |
6 | 0.2660 | 0.8010 | 1.5530 | 0.7070 | 1.3360 | 1.0980 | 2.8115 | 3.0780 | |
8 | 0.3210 | 0.9610 | 2.7830 | 1.0120 | 0.9970 | 0.7930 | 4.5400 | 4.8560 | |
10 | 0.4110 | 1.5550 | 2.6160 | 1.0420 | 1.6320 | 0.9770 | 5.2685 | 5.8515 | |
12 | 0.5330 | 1.2210 | 2.9890 | 1.1920 | 1.9340 | 1.1170 | 4.6090 | 4.1150 | |
Pilot | 4 | 0.4400 | 0.9200 | 2.3630 | 0.7820 | 1.0780 | 0.8350 | 1.8890 | 1.8405 |
6 | 0.2810 | 1.6590 | 2.2270 | 0.7510 | 1.3550 | 0.8610 | 3.4105 | 2.8835 | |
8 | 0.4400 | 1.5610 | 2.2130 | 0.8350 | 1.0880 | 1.2600 | 3.6285 | 3.9075 | |
10 | 0.3700 | 1.0850 | 2.7580 | 1.2330 | 1.2590 | 1.3200 | 4.9585 | 5.6945 | |
12 | 0.3740 | 0.9200 | 1.7840 | 1.2990 | 1.1100 | 1.5060 | 5.2780 | 4.0805 | |
Dog | 4 | 0.4500 | 1.0160 | 2.4440 | 0.8390 | 0.8300 | 1.3690 | 1.7170 | 2.5915 |
6 | 0.2750 | 1.3830 | 2.4210 | 1.2500 | 0.8100 | 1.3300 | 3.1915 | 3.4480 | |
8 | 0.4700 | 0.9450 | 1.5800 | 0.7400 | 0.7400 | 0.7510 | 2.4325 | 2.8155 | |
10 | 0.3490 | 0.8300 | 1.5390 | 0.7190 | 0.8010 | 0.7220 | 3.0855 | 3.5100 | |
12 | 0.3300 | 0.8720 | 1.5700 | 0.7600 | 0.8700 | 0.7800 | 3.7950 | 4.3600 |
Image | K | SSA | MVO | DA | FPA | PSO | SCA | ALO | MALO |
Cactus | 4 | 0.6400 | 1.2900 | 1.9500 | 0.8000 | 1.6930 | 0.7800 | 1.5150 | 1.8455 |
6 | 0.4700 | 0.8500 | 1.5890 | 0.8210 | 0.8910 | 0.7900 | 2.0750 | 2.3905 | |
8 | 0.4900 | 0.9500 | 1.6810 | 0.8110 | 0.8900 | 0.8080 | 2.4650 | 4.2290 | |
10 | 0.6230 | 1.2750 | 2.0900 | 1.0400 | 0.9900 | 0.8490 | 3.1220 | 3.6495 | |
12 | 0.5380 | 1.0030 | 1.7180 | 0.9570 | 1.0110 | 0.8900 | 3.6600 | 4.1195 | |
Kangaroo | 4 | 0.5800 | 0.9600 | 1.8620 | 0.8300 | 0.8900 | 1.4690 | 2.2225 | 2.3925 |
6 | 0.9260 | 1.2200 | 2.6770 | 1.4180 | 0.9600 | 1.0310 | 3.2235 | 2.9170 | |
8 | 0.4800 | 0.8900 | 1.6200 | 0.8200 | 0.9000 | 1.1690 | 2.5200 | 3.1900 | |
10 | 0.5210 | 0.9200 | 1.6400 | 0.8900 | 0.8700 | 0.8400 | 3.0550 | 3.6250 | |
12 | 0.5200 | 0.9900 | 1.7500 | 0.8800 | 0.9400 | 0.8900 | 3.6400 | 4.1070 | |
Temple | 4 | 0.6100 | 1.6350 | 2.5860 | 1.2700 | 1.2480 | 0.9400 | 2.4355 | 2.8155 |
6 | 0.4700 | 1.0400 | 2.3770 | 1.0500 | 1.2900 | 0.7900 | 2.0400 | 2.4100 | |
8 | 0.4700 | 0.9100 | 1.6800 | 0.8000 | 0.8900 | 0.8000 | 2.4900 | 2.9895 | |
10 | 0.5200 | 0.9300 | 1.6500 | 0.8600 | 1.0000 | 0.8400 | 3.1050 | 3.6095 | |
12 | 0.5700 | 1.0000 | 1.8200 | 0.9300 | 0.9100 | 0.8700 | 5.4985 | 4.5950 | |
Flower | 4 | 0.6000 | 1.4130 | 2.6960 | 1.0000 | 1.2850 | 1.3000 | 2.3625 | 2.8995 |
6 | 1.0690 | 0.9900 | 2.8420 | 0.9500 | 0.9390 | 0.9860 | 2.6195 | 2.7010 | |
8 | 0.4800 | 0.9900 | 1.7200 | 0.9400 | 0.9200 | 0.8700 | 2.6020 | 3.0850 | |
10 | 0.5300 | 0.9700 | 1.8010 | 0.9100 | 0.9990 | 0.9600 | 3.2255 | 3.6850 | |
12 | 0.6100 | 1.0700 | 1.8100 | 0.9400 | 1.0000 | 1.3790 | 5.1945 | 4.5065 | |
Mountain | 4 | 0.5600 | 1.5490 | 1.9920 | 0.8570 | 0.8810 | 0.8310 | 1.5315 | 1.9575 |
6 | 0.4410 | 0.9230 | 1.7220 | 0.9020 | 0.8820 | 0.8800 | 2.0330 | 2.4990 | |
8 | 0.5100 | 0.9710 | 1.7300 | 0.9000 | 0.9520 | 0.8420 | 2.5850 | 3.1700 | |
10 | 0.5480 | 1.0300 | 1.7900 | 0.9040 | 0.9510 | 0.9000 | 5.1080 | 4.0555 | |
12 | 1.0660 | 1.0420 | 1.9110 | 0.9610 | 1.0690 | 0.9580 | 3.9095 | 4.3645 | |
Tree | 4 | 0.6200 | 1.3020 | 2.8450 | 1.0270 | 1.2090 | 1.3510 | 2.2510 | 2.7790 |
6 | 0.5000 | 1.2040 | 2.6420 | 1.3380 | 1.1410 | 0.8990 | 2.1775 | 3.1785 | |
8 | 0.5110 | 0.8790 | 1.6500 | 0.8300 | 0.8800 | 0.8600 | 2.4650 | 2.9350 | |
10 | 0.5000 | 0.9300 | 1.6800 | 0.9190 | 0.8500 | 0.9000 | 3.0445 | 3.5950 | |
12 | 0.6700 | 1.0090 | 1.8600 | 0.9100 | 0.9400 | 0.9400 | 3.7930 | 4.1300 | |
Horse | 4 | 0.6100 | 1.5700 | 3.2080 | 1.5370 | 1.6130 | 0.8400 | 2.5665 | 2.8115 |
6 | 1.0800 | 1.2290 | 2.5900 | 0.8000 | 0.8100 | 0.9800 | 2.0500 | 2.4145 | |
8 | 0.4600 | 0.8900 | 1.6000 | 0.8300 | 0.8600 | 0.8900 | 2.6550 | 3.2905 | |
10 | 0.5000 | 0.9900 | 1.6400 | 1.1500 | 0.8900 | 0.8500 | 3.0550 | 3.6400 | |
12 | 0.5400 | 1.9330 | 2.0100 | 0.9800 | 1.0100 | 1.0000 | 3.7850 | 4.4105 | |
Bridge | 4 | 0.6000 | 0.9500 | 1.7600 | 0.7600 | 0.8100 | 0.7300 | 1.4750 | 1.7950 |
6 | 0.4100 | 0.8400 | 1.5700 | 0.8200 | 0.8400 | 0.8000 | 1.9300 | 2.3950 | |
8 | 0.4400 | 0.8600 | 1.5900 | 0.8700 | 0.8600 | 0.7900 | 2.4900 | 2.9775 | |
10 | 0.4700 | 0.9100 | 1.6400 | 0.7900 | 0.9100 | 1.2500 | 3.7975 | 3.9840 | |
12 | 0.5230 | 1.0150 | 2.1800 | 0.8710 | 0.9340 | 0.9500 | 3.7080 | 4.3340 | |
Pilot | 4 | 0.5400 | 0.9400 | 1.7300 | 0.7500 | 1.0100 | 0.7700 | 1.4750 | 1.7600 |
6 | 0.3900 | 0.8100 | 1.5500 | 0.8300 | 0.8700 | 0.8800 | 2.0940 | 2.5195 | |
8 | 0.4600 | 0.8400 | 1.8120 | 0.7760 | 0.9500 | 0.8600 | 2.6975 | 3.1400 | |
10 | 0.5650 | 1.1600 | 2.2950 | 0.9460 | 0.9790 | 0.9690 | 3.5840 | 4.1245 | |
12 | 0.5800 | 1.3060 | 2.3550 | 1.2900 | 1.0490 | 1.3350 | 5.6445 | 8.5025 | |
Dog | 4 | 0.6600 | 1.2350 | 3.0700 | 1.0100 | 1.0550 | 1.6800 | 2.5700 | 3.0600 |
6 | 0.5050 | 1.0870 | 2.1600 | 0.9200 | 0.9200 | 0.8500 | 2.2025 | 2.8425 | |
8 | 0.5050 | 1.2550 | 2.9380 | 1.0800 | 1.8400 | 1.4900 | 4.5565 | 5.3650 | |
10 | 0.5920 | 1.4200 | 2.2400 | 0.9200 | 1.2000 | 0.9250 | 3.4000 | 3.9405 | |
12 | 0.5790 | 1.0750 | 1.8410 | 0.9500 | 0.9990 | 0.9140 | 3.8400 | 4.5330 |
Provenance | Symbols | Paraphrase |
Color images | L | The gray value |
ni | The number of pixels with gray value of i | |
N | The total number of pixels | |
p | The distribution probability of gray value | |
K | The total number of threshold | |
t | The threshold | |
C | The class | |
R, G, B | The three channels of color images | |
Otsu | ω | The probabilities of class occurrence |
μ | The levels of class | |
σ2 | The total variance | |
Kapur's entropy | ψ | The total entropy |
ALO | X | The array of random walk |
ub | The upper bound of parameter | |
lb | The lower bound of parameter | |
t | The current iteration | |
T | The maximum of iterations | |
I | The ratio of boundary contraction | |
Time complexity | O | The complexity notation |
PSNR | MSE | The mean square error |
SSIM | R | The original image |
I | The segmented image | |
μR,μI | The average gray values of image | |
σ2R,σ2I | The variance of image | |
σRI | The covariance of image | |
FSIM | PC | Phase congruency |
GM | Gradient magnitude | |
Ω | The entire domain of image | |
SL(x) | The similarity value of each position x | |
PCm(x) | The phase consistency measure | |
SPC(x) | The similarity measure of phase consistency | |
SG(x) | The similarity measure of gradient magnitude |
IMAGE | K | SSA | MVO | DA | FPA | PSO | SCA | ALO | MALO |
Cactus | 4 | 19.1730 | 19.1730 | 19.1730 | 18.7263 | 19.1730 | 18.8974 | 19.1344 | 19.1901 |
6 | 21.4834 | 21.4806 | 21.4819 | 20.6216 | 21.0808 | 20.2685 | 21.4733 | 21.4849 | |
8 | 22.9129 | 23.0452 | 22.5947 | 21.8482 | 22.5738 | 23.0573 | 22.9754 | 23.7403 | |
10 | 23.8232 | 24.1443 | 23.9252 | 23.1394 | 23.9100 | 23.8865 | 24.0865 | 24.1499 | |
12 | 24.6895 | 25.1475 | 24.8778 | 24.9636 | 24.5394 | 25.1646 | 25.0007 | 25.2868 | |
Kangaroo | 4 | 22.0643 | 22.0643 | 22.0643 | 20.7955 | 22.0643 | 20.4905 | 22.0429 | 22.0797 |
6 | 24.8251 | 24.8350 | 24.5043 | 23.2999 | 24.4744 | 23.1015 | 24.8199 | 24.8836 | |
8 | 26.8199 | 27.0457 | 26.8403 | 24.3867 | 26.3434 | 24.2914 | 26.9047 | 27.0860 | |
10 | 28.1619 | 28.8131 | 28.5707 | 25.1187 | 28.1677 | 25.2235 | 28.4114 | 28.8186 | |
12 | 29.1767 | 30.0207 | 29.8385 | 27.1879 | 29.8151 | 26.9052 | 30.1672 | 30.3411 | |
Temple | 4 | 17.4713 | 17.4707 | 17.4713 | 19.7885 | 17.4736 | 17.1516 | 17.4005 | 17.6125 |
6 | 20.7143 | 19.8002 | 20.7137 | 20.7876 | 19.7566 | 18.2250 | 20.7661 | 21.4589 | |
8 | 22.0492 | 23.5836 | 22.5695 | 20.7664 | 22.5250 | 23.8550 | 23.4862 | 23.9977 | |
10 | 23.2619 | 25.2421 | 24.2747 | 23.4468 | 23.3367 | 22.7675 | 24.8846 | 25.7059 | |
12 | 26.8631 | 26.8151 | 25.5113 | 24.5639 | 24.7817 | 26.2330 | 26.4459 | 27.5232 | |
Flower | 4 | 22.6832 | 22.6832 | 22.6832 | 21.9099 | 22.6832 | 22.2661 | 22.6832 | 22.6916 |
6 | 25.1802 | 25.5484 | 25.5446 | 23.9499 | 25.2590 | 24.5962 | 25.5240 | 25.5506 | |
8 | 27.5586 | 27.3846 | 27.6921 | 25.3172 | 27.3576 | 26.1643 | 27.5337 | 27.6960 | |
10 | 28.6654 | 28.8544 | 29.0249 | 26.6791 | 28.8757 | 26.7323 | 29.1768 | 29.2169 | |
12 | 29.9882 | 30.1775 | 29.8010 | 28.1115 | 29.6455 | 27.8780 | 30.6120 | 30.7280 | |
Mountain | 4 | 19.7307 | 19.7307 | 19.7307 | 19.1368 | 19.7307 | 19.2754 | 19.7277 | 19.7307 |
6 | 21.8240 | 22.4882 | 22.3866 | 20.9344 | 22.1794 | 21.8654 | 22.3347 | 22.5039 | |
8 | 24.8724 | 24.1574 | 24.2907 | 22.8823 | 24.0400 | 23.4094 | 24.7870 | 24.9053 | |
10 | 25.9744 | 26.1368 | 26.1295 | 24.6068 | 25.8506 | 23.8109 | 26.0493 | 26.3373 | |
12 | 27.3911 | 26.9209 | 26.9014 | 25.3515 | 26.9991 | 25.7124 | 26.8751 | 27.4991 | |
Tree | 4 | 19.9284 | 19.9265 | 19.9284 | 19.3506 | 19.9206 | 19.0453 | 19.9284 | 19.9364 |
6 | 22.9219 | 22.9184 | 22.9126 | 21.8322 | 22.4339 | 22.4819 | 22.9276 | 22.9388 | |
8 | 25.1296 | 25.0004 | 25.0881 | 22.6562 | 24.4342 | 22.7555 | 25.1489 | 25.1704 | |
10 | 26.7206 | 26.6709 | 26.6389 | 24.1790 | 26.1891 | 24.3475 | 26.5608 | 26.7213 | |
12 | 27.5331 | 28.0382 | 27.7147 | 26.1871 | 27.2252 | 24.9152 | 27.8812 | 28.0724 | |
Horse | 4 | 19.1095 | 19.1095 | 19.1543 | 18.4918 | 19.1095 | 17.8797 | 19.1095 | 19.1569 |
6 | 22.6175 | 22.6343 | 22.6083 | 22.4411 | 22.6441 | 20.0880 | 22.5318 | 22.8301 | |
8 | 24.8257 | 24.6203 | 24.7383 | 23.2636 | 23.7326 | 22.2812 | 24.5913 | 24.8543 | |
10 | 26.7272 | 26.7701 | 26.3299 | 23.8301 | 26.2501 | 23.7805 | 26.4699 | 26.8845 | |
12 | 28.0067 | 27.6999 | 28.1864 | 25.0419 | 28.0623 | 24.4077 | 27.5665 | 28.3129 | |
Bridge | 4 | 18.7684 | 18.7684 | 18.7684 | 18.1883 | 18.7684 | 18.3216 | 18.7562 | 18.7684 |
6 | 21.0341 | 21.5192 | 21.5142 | 20.6458 | 21.5142 | 19.4138 | 21.0529 | 21.5346 | |
8 | 23.5582 | 22.9642 | 23.4355 | 21.6761 | 23.3575 | 20.6855 | 23.3521 | 23.7010 | |
10 | 25.0709 | 24.8332 | 25.2117 | 22.7421 | 24.8889 | 23.3572 | 25.3971 | 25.4265 | |
12 | 26.3616 | 26.8858 | 26.3203 | 25.5030 | 26.4849 | 25.2994 | 26.1879 | 27.0756 | |
Pilot | 4 | 16.9918 | 16.9918 | 16.9918 | 16.9571 | 16.9915 | 16.9918 | 17.0028 | 17.6971 |
6 | 19.4806 | 19.6603 | 19.3935 | 18.8999 | 19.4660 | 19.4742 | 19.4398 | 21.5230 | |
8 | 22.1137 | 21.7970 | 21.8114 | 19.8872 | 20.9936 | 20.1921 | 21.9259 | 22.4562 | |
10 | 23.3937 | 23.4926 | 22.5532 | 23.4677 | 22.5761 | 22.8645 | 23.6086 | 24.1912 | |
12 | 24.6032 | 24.8457 | 25.4417 | 23.3403 | 23.4735 | 24.3050 | 25.6425 | 25.8213 | |
Dog | 4 | 19.9346 | 19.9569 | 19.9346 | 20.2544 | 19.9346 | 18.6387 | 19.9734 | 19.9346 |
6 | 22.5690 | 22.6725 | 22.3348 | 21.6363 | 21.7981 | 20.9528 | 22.5891 | 22.6726 | |
8 | 24.3105 | 24.3265 | 24.3176 | 23.0185 | 22.9836 | 22.5716 | 24.3533 | 24.3548 | |
10 | 24.9193 | 26.0109 | 25.7454 | 24.4410 | 25.5648 | 23.1901 | 25.8904 | 26.4961 | |
12 | 26.7167 | 28.0094 | 26.3712 | 24.9053 | 26.4391 | 25.6498 | 27.6947 | 28.1328 |
IMAGE | K | SSA | MVO | DA | FPA | PSO | SCA | ALO | MALO |
Cactus | 4 | 17.1463 | 17.1849 | 17.1849 | 16.6326 | 17.1605 | 16.5963 | 17.1918 | 17.2250 |
6 | 18.7054 | 19.5152 | 18.8374 | 17.7723 | 18.9734 | 18.9692 | 18.9353 | 20.9242 | |
8 | 20.2196 | 21.8061 | 21.8129 | 20.2720 | 20.7186 | 20.1477 | 20.8937 | 23.1098 | |
10 | 21.6755 | 23.5236 | 23.5035 | 22.9740 | 23.3426 | 23.5817 | 23.6783 | 25.6436 | |
12 | 24.5328 | 25.7488 | 24.2717 | 25.8229 | 25.6387 | 22.2231 | 25.5971 | 26.7329 | |
Kangaroo | 4 | 18.7559 | 18.7559 | 18.7529 | 16.9273 | 18.7030 | 17.9415 | 18.7714 | 18.8067 |
6 | 21.2093 | 21.9397 | 21.7227 | 20.3904 | 21.8062 | 20.1191 | 22.1098 | 22.3708 | |
8 | 23.8181 | 24.5562 | 23.9062 | 20.5802 | 24.5744 | 22.1074 | 24.4808 | 24.6322 | |
10 | 25.1196 | 26.0125 | 25.7934 | 25.0967 | 25.7309 | 22.8701 | 25.9331 | 26.1974 | |
12 | 26.6277 | 27.4952 | 27.4380 | 24.5722 | 27.3277 | 24.0530 | 27.2209 | 28.0349 | |
Temple | 4 | 16.7418 | 18.6069 | 17.9079 | 17.7575 | 17.8108 | 16.4613 | 18.5579 | 18.6105 |
6 | 20.3140 | 20.4895 | 20.3140 | 20.7483 | 20.5577 | 21.6953 | 20.6648 | 23.1506 | |
8 | 24.9708 | 24.0553 | 23.2965 | 22.2220 | 24.5715 | 23.2979 | 25.0443 | 25.1273 | |
10 | 25.8405 | 25.8930 | 26.0409 | 23.4840 | 26.5620 | 21.8980 | 26.5010 | 26.7757 | |
12 | 27.0259 | 26.4958 | 27.5263 | 24.8266 | 27.3391 | 24.0635 | 27.5766 | 28.4027 | |
Flower | 4 | 21.4262 | 21.4353 | 21.4262 | 21.0771 | 21.4030 | 21.7291 | 21.3824 | 21.4046 |
6 | 23.9954 | 24.0070 | 24.0260 | 22.9845 | 23.9935 | 23.7137 | 24.0223 | 24.2923 | |
8 | 25.0019 | 25.3825 | 25.3936 | 24.4758 | 25.6350 | 25.5636 | 25.5517 | 26.3947 | |
10 | 26.1749 | 27.0175 | 26.8094 | 25.4329 | 26.9897 | 26.1739 | 26.8538 | 27.6016 | |
12 | 26.8429 | 28.2241 | 27.8388 | 25.9058 | 27.9865 | 27.9984 | 27.8198 | 29.2750 | |
Mountain | 4 | 15.7308 | 16.1695 | 16.1695 | 15.1291 | 14.6360 | 17.2397 | 14.6863 | 17.7501 |
6 | 19.1293 | 19.7275 | 19.3198 | 20.0548 | 19.8595 | 18.9344 | 19.2375 | 20.2658 | |
8 | 22.2621 | 22.4482 | 22.2780 | 20.3443 | 22.2996 | 20.2389 | 22.1739 | 23.7160 | |
10 | 20.8677 | 25.1887 | 23.4748 | 22.1128 | 24.6158 | 22.2042 | 24.5972 | 26.2667 | |
12 | 25.4993 | 26.3821 | 26.2452 | 24.7312 | 26.5979 | 22.1953 | 26.4684 | 26.6949 | |
Tree | 4 | 19.3878 | 19.4098 | 19.3878 | 19.4510 | 19.3660 | 19.0814 | 19.3485 | 19.3878 |
6 | 21.7283 | 21.9982 | 21.9410 | 20.7787 | 21.7496 | 20.5382 | 21.9919 | 22.0355 | |
8 | 23.5510 | 23.6198 | 23.4812 | 22.0054 | 23.5766 | 22.2770 | 23.5404 | 24.2661 | |
10 | 24.8234 | 25.5643 | 25.4307 | 23.3183 | 25.4128 | 22.1914 | 25.4025 | 25.7360 | |
12 | 26.1558 | 26.7973 | 26.3433 | 23.9995 | 26.5906 | 24.8922 | 26.5045 | 26.8461 | |
Horse | 4 | 18.9208 | 19.7557 | 19.0344 | 18.9208 | 19.6738 | 19.4317 | 19.5801 | 19.7788 |
6 | 21.2581 | 21.6962 | 21.6519 | 19.7265 | 21.3967 | 19.8505 | 21.6610 | 21.6974 | |
8 | 23.8699 | 23.6078 | 24.2314 | 22.0431 | 23.2680 | 21.4611 | 23.4581 | 24.2427 | |
10 | 25.5023 | 24.5116 | 25.5495 | 23.2916 | 25.8501 | 22.6324 | 26.0431 | 26.1268 | |
12 | 26.4346 | 25.3050 | 27.0205 | 24.1636 | 27.1689 | 24.0270 | 26.9024 | 27.4180 | |
Bridge | 4 | 17.7375 | 17.7101 | 17.7433 | 17.6562 | 17.7064 | 17.2277 | 17.7680 | 18.3564 |
6 | 21.0395 | 21.0300 | 21.0468 | 20.0621 | 21.0612 | 19.8616 | 21.0352 | 21.1004 | |
8 | 22.8402 | 23.4100 | 23.4820 | 21.0220 | 23.3430 | 21.3364 | 23.4706 | 23.6124 | |
10 | 24.6074 | 24.9201 | 24.1982 | 23.1209 | 24.7082 | 23.6599 | 24.6209 | 25.3739 | |
12 | 25.4895 | 26.0580 | 26.3086 | 23.1938 | 26.7399 | 24.1204 | 26.4986 | 27.4320 | |
Pilot | 4 | 15.9241 | 15.8597 | 15.9653 | 15.5965 | 15.8932 | 15.9792 | 15.9532 | 16.0238 |
6 | 19.9635 | 19.1615 | 20.4713 | 19.5345 | 20.6783 | 20.2829 | 19.8317 | 20.7123 | |
8 | 22.5976 | 22.0836 | 22.9972 | 22.6697 | 23.0674 | 22.7085 | 22.8982 | 23.1598 | |
10 | 24.5888 | 20.7618 | 24.7292 | 23.9268 | 24.7256 | 24.0374 | 24.5571 | 24.8228 | |
12 | 25.6096 | 24.6046 | 26.7532 | 24.9263 | 25.9880 | 25.6817 | 26.4241 | 27.1095 | |
Dog | 4 | 18.3708 | 18.4828 | 17.6137 | 16.0679 | 18.2476 | 18.2657 | 18.4844 | 18.2605 |
6 | 20.0477 | 20.7865 | 20.6360 | 19.9191 | 20.6773 | 18.4516 | 20.7274 | 21.9672 | |
8 | 22.3956 | 22.5129 | 22.5608 | 22.6757 | 22.5483 | 22.5758 | 22.3802 | 24.5205 | |
10 | 23.4554 | 25.3079 | 24.3555 | 24.4406 | 25.4561 | 21.6361 | 24.8465 | 26.3186 | |
12 | 25.8139 | 26.8848 | 26.0117 | 23.2895 | 26.5957 | 23.0829 | 26.4860 | 27.2748 |
IMAGE | K | SSA | MVO | DA | FPA | PSO | SCA | ALO | MALO |
Cactus | 4 | 0.6001 | 0.6001 | 0.6001 | 0.5854 | 0.6015 | 0.6001 | 0.5984 | 0.6063 |
6 | 0.6955 | 0.6961 | 0.6950 | 0.6716 | 0.6801 | 0.6568 | 0.6956 | 0.6963 | |
8 | 0.7438 | 0.7515 | 0.7268 | 0.7278 | 0.7361 | 0.7507 | 0.7487 | 0.7904 | |
10 | 0.7684 | 0.7838 | 0.7732 | 0.7495 | 0.7745 | 0.7814 | 0.7807 | 0.8391 | |
12 | 0.8012 | 0.8123 | 0.8157 | 0.8272 | 0.7927 | 0.8022 | 0.8050 | 0.8456 | |
Kangaroo | 4 | 0.7735 | 0.7735 | 0.7735 | 0.7156 | 0.7738 | 0.7046 | 0.7725 | 0.7735 |
6 | 0.8609 | 0.8615 | 0.8515 | 0.8179 | 0.8525 | 0.8033 | 0.8608 | 0.8627 | |
8 | 0.9018 | 0.9066 | 0.9029 | 0.8415 | 0.8958 | 0.8431 | 0.9041 | 0.9084 | |
10 | 0.9219 | 0.9325 | 0.9288 | 0.8581 | 0.9244 | 0.8658 | 0.9262 | 0.9331 | |
12 | 0.9352 | 0.9466 | 0.9426 | 0.9032 | 0.9449 | 0.9032 | 0.9474 | 0.9495 | |
Temple | 4 | 0.5829 | 0.5829 | 0.5829 | 0.5906 | 0.5825 | 0.5492 | 0.5793 | 0.6798 |
6 | 0.7234 | 0.6884 | 0.7242 | 0.7222 | 0.6836 | 0.6239 | 0.7249 | 0.7263 | |
8 | 0.7702 | 0.8149 | 0.7873 | 0.7265 | 0.7843 | 0.8102 | 0.8121 | 0.8152 | |
10 | 0.8069 | 0.8561 | 0.8329 | 0.7886 | 0.8084 | 0.7752 | 0.8480 | 0.8643 | |
12 | 0.8823 | 0.8883 | 0.8606 | 0.8254 | 0.8453 | 0.8546 | 0.8753 | 0.8917 | |
Flower | 4 | 0.4655 | 0.4655 | 0.4655 | 0.4194 | 0.4655 | 0.4655 | 0.4656 | 0.4916 |
6 | 0.5704 | 0.6806 | 0.6789 | 0.4849 | 0.6696 | 0.6800 | 0.6765 | 0.7406 | |
8 | 0.7883 | 0.7946 | 0.7974 | 0.6136 | 0.7867 | 0.7725 | 0.7911 | 0.8000 | |
10 | 0.8139 | 0.8286 | 0.8219 | 0.7771 | 0.8206 | 0.7406 | 0.8268 | 0.8294 | |
12 | 0.8364 | 0.8550 | 0.8310 | 0.8422 | 0.8372 | 0.8203 | 0.8644 | 0.8682 | |
Mountain | 4 | 0.6987 | 0.6987 | 0.6987 | 0.6715 | 0.6987 | 0.6987 | 0.6976 | 0.7081 |
6 | 0.7471 | 0.7758 | 0.7691 | 0.7237 | 0.7698 | 0.7761 | 0.7705 | 0.7932 | |
8 | 0.8293 | 0.8166 | 0.8127 | 0.7688 | 0.8101 | 0.8206 | 0.8311 | 0.8322 | |
10 | 0.8442 | 0.8536 | 0.8476 | 0.8254 | 0.8524 | 0.8261 | 0.8520 | 0.8603 | |
12 | 0.8682 | 0.8650 | 0.8626 | 0.8273 | 0.8731 | 0.8511 | 0.8617 | 0.8780 | |
Tree | 4 | 0.6406 | 0.6410 | 0.6406 | 0.6162 | 0.6419 | 0.6406 | 0.6412 | 0.6915 |
6 | 0.7535 | 0.7538 | 0.7535 | 0.6682 | 0.7420 | 0.7454 | 0.7538 | 0.7546 | |
8 | 0.7919 | 0.7890 | 0.7901 | 0.7176 | 0.7465 | 0.7515 | 0.7954 | 0.7960 | |
10 | 0.8229 | 0.8272 | 0.8263 | 0.7932 | 0.8190 | 0.8009 | 0.8258 | 0.8281 | |
12 | 0.8372 | 0.8505 | 0.8416 | 0.8307 | 0.8387 | 0.8142 | 0.8555 | 0.8579 | |
Horse | 4 | 0.7206 | 0.7206 | 0.7203 | 0.6791 | 0.7206 | 0.7155 | 0.7201 | 0.7206 |
6 | 0.7543 | 0.7624 | 0.7613 | 0.7617 | 0.7611 | 0.7700 | 0.7591 | 0.7744 | |
8 | 0.8102 | 0.8062 | 0.8103 | 0.7689 | 0.7945 | 0.7622 | 0.8114 | 0.8117 | |
10 | 0.8501 | 0.8510 | 0.8505 | 0.8026 | 0.8454 | 0.8162 | 0.8523 | 0.8534 | |
12 | 0.8709 | 0.8769 | 0.8739 | 0.8233 | 0.8757 | 0.8161 | 0.8686 | 0.8793 | |
Bridge | 4 | 0.7286 | 0.7286 | 0.7286 | 0.7286 | 0.7286 | 0.7293 | 0.7280 | 0.7398 |
6 | 0.8032 | 0.8180 | 0.8180 | 0.8022 | 0.8190 | 0.7728 | 0.8030 | 0.8193 | |
8 | 0.8626 | 0.8512 | 0.8566 | 0.8374 | 0.8604 | 0.8323 | 0.8620 | 0.8691 | |
10 | 0.8830 | 0.8909 | 0.8903 | 0.8447 | 0.8912 | 0.8886 | 0.8956 | 0.8993 | |
12 | 0.9021 | 0.9140 | 0.9031 | 0.8986 | 0.9041 | 0.9063 | 0.9087 | 0.9226 | |
Pilot | 4 | 0.7296 | 0.7296 | 0.7296 | 0.7222 | 0.7296 | 0.7296 | 0.7299 | 0.7399 |
6 | 0.7854 | 0.7857 | 0.7839 | 0.7540 | 0.7852 | 0.7849 | 0.7855 | 0.8224 | |
8 | 0.8420 | 0.8385 | 0.8383 | 0.7887 | 0.8218 | 0.7875 | 0.8390 | 0.8424 | |
10 | 0.8720 | 0.8651 | 0.8607 | 0.8555 | 0.8570 | 0.8401 | 0.8737 | 0.8788 | |
12 | 0.8905 | 0.8996 | 0.8951 | 0.8581 | 0.8726 | 0.8668 | 0.8947 | 0.9017 | |
Dog | 4 | 0.7003 | 0.7031 | 0.7003 | 0.7003 | 0.7003 | 0.6563 | 0.6999 | 0.7428 |
6 | 0.7700 | 0.7755 | 0.7624 | 0.7684 | 0.7514 | 0.7104 | 0.7694 | 0.7756 | |
8 | 0.8188 | 0.8190 | 0.8190 | 0.7735 | 0.7848 | 0.7632 | 0.8213 | 0.8240 | |
10 | 0.8314 | 0.8572 | 0.8510 | 0.8116 | 0.8470 | 0.7804 | 0.8544 | 0.8655 | |
12 | 0.8691 | 0.8928 | 0.8643 | 0.8246 | 0.8654 | 0.8566 | 0.8886 | 0.8948 |
IMAGE | K | SSA | MVO | DA | FPA | PSO | SCA | ALO | MALO |
Cactus | 4 | 0.4811 | 0.4831 | 0.4831 | 0.4466 | 0.4818 | 0.4497 | 0.4835 | 0.4858 |
6 | 0.5552 | 0.5975 | 0.5613 | 0.5064 | 0.5697 | 0.7353 | 0.5657 | 0.5690 | |
8 | 0.6254 | 0.7141 | 0.7143 | 0.6989 | 0.6473 | 0.6168 | 0.6574 | 0.7681 | |
10 | 0.7156 | 0.7719 | 0.7926 | 0.7684 | 0.7661 | 0.8080 | 0.7761 | 0.8677 | |
12 | 0.8238 | 0.8537 | 0.7953 | 0.8778 | 0.8524 | 0.7282 | 0.8510 | 0.8873 | |
Kangaroo | 4 | 0.6080 | 0.6080 | 0.6078 | 0.5032 | 0.6058 | 0.5645 | 0.6083 | 0.6116 |
6 | 0.7320 | 0.7635 | 0.7531 | 0.6689 | 0.7565 | 0.6881 | 0.7690 | 0.7782 | |
8 | 0.8263 | 0.8498 | 0.8304 | 0.7028 | 0.8493 | 0.7534 | 0.8476 | 0.8521 | |
10 | 0.8632 | 0.8867 | 0.8798 | 0.8570 | 0.8798 | 0.7602 | 0.8843 | 0.8913 | |
12 | 0.8976 | 0.9150 | 0.9128 | 0.8380 | 0.9111 | 0.8155 | 0.9096 | 0.9254 | |
Temple | 4 | 0.5367 | 0.6145 | 0.5713 | 0.5753 | 0.5657 | 0.5101 | 0.6117 | 0.6149 |
6 | 0.6933 | 0.6996 | 0.6933 | 0.7056 | 0.6976 | 0.7261 | 0.7014 | 0.7784 | |
8 | 0.8252 | 0.8034 | 0.7796 | 0.7400 | 0.8173 | 0.7918 | 0.8318 | 0.8465 | |
10 | 0.8469 | 0.8719 | 0.8522 | 0.7703 | 0.8665 | 0.7311 | 0.8648 | 0.8787 | |
12 | 0.8733 | 0.8941 | 0.8845 | 0.8153 | 0.8796 | 0.8086 | 0.8915 | 0.9021 | |
Flower | 4 | 0.3948 | 0.3985 | 0.3948 | 0.3969 | 0.3976 | 0.3976 | 0.3950 | 0.3994 |
6 | 0.4916 | 0.5129 | 0.4916 | 0.4830 | 0.4925 | 0.4923 | 0.4917 | 0.5247 | |
8 | 0.5171 | 0.5986 | 0.5385 | 0.5047 | 0.5538 | 0.5388 | 0.5387 | 0.6431 | |
10 | 0.5589 | 0.6425 | 0.5845 | 0.5280 | 0.5968 | 0.5964 | 0.5885 | 0.6606 | |
12 | 0.5737 | 0.6445 | 0.6168 | 0.5823 | 0.6299 | 0.7444 | 0.6410 | 0.7643 | |
Mountain | 4 | 0.4651 | 0.5563 | 0.5563 | 0.4348 | 0.4186 | 0.5804 | 0.4192 | 0.6240 |
6 | 0.6590 | 0.6916 | 0.6673 | 0.7108 | 0.6961 | 0.6653 | 0.6674 | 0.7131 | |
8 | 0.7628 | 0.7797 | 0.7716 | 0.6980 | 0.7612 | 0.7505 | 0.7695 | 0.8259 | |
10 | 0.7035 | 0.8433 | 0.7862 | 0.7690 | 0.8408 | 0.8062 | 0.8135 | 0.8792 | |
12 | 0.8537 | 0.8681 | 0.8645 | 0.8367 | 0.8718 | 0.7863 | 0.8668 | 0.9085 | |
Tree | 4 | 0.5888 | 0.5906 | 0.5888 | 0.6081 | 0.5874 | 0.5888 | 0.5866 | 0.6264 |
6 | 0.6716 | 0.6898 | 0.6728 | 0.6909 | 0.6708 | 0.6817 | 0.6770 | 0.7219 | |
8 | 0.7080 | 0.7157 | 0.7126 | 0.6873 | 0.7171 | 0.7503 | 0.7129 | 0.7737 | |
10 | 0.7271 | 0.7594 | 0.7631 | 0.7740 | 0.7656 | 0.6862 | 0.7653 | 0.8214 | |
12 | 0.7646 | 0.7871 | 0.7772 | 0.7040 | 0.7822 | 0.7977 | 0.8101 | 0.8792 | |
Horse | 4 | 0.6486 | 0.6486 | 0.6637 | 0.6911 | 0.6976 | 0.6675 | 0.6835 | 0.7070 |
6 | 0.7287 | 0.7486 | 0.7478 | 0.6954 | 0.7414 | 0.7463 | 0.7487 | 0.7802 | |
8 | 0.7789 | 0.7930 | 0.7922 | 0.7668 | 0.8010 | 0.7618 | 0.8035 | 0.8335 | |
10 | 0.8221 | 0.8388 | 0.8247 | 0.7824 | 0.8276 | 0.7849 | 0.8376 | 0.8697 | |
12 | 0.8410 | 0.8699 | 0.8567 | 0.7938 | 0.8691 | 0.8089 | 0.8598 | 0.8979 | |
Bridge | 4 | 0.6914 | 0.6927 | 0.6902 | 0.6790 | 0.6918 | 0.6897 | 0.6946 | 0.7176 |
6 | 0.7909 | 0.7974 | 0.7957 | 0.7433 | 0.7926 | 0.7813 | 0.7979 | 0.8053 | |
8 | 0.8336 | 0.8532 | 0.8522 | 0.7775 | 0.8505 | 0.8427 | 0.8564 | 0.8820 | |
10 | 0.8688 | 0.8812 | 0.8598 | 0.8144 | 0.8717 | 0.8636 | 0.8878 | 0.9160 | |
12 | 0.8836 | 0.9044 | 0.9271 | 0.8634 | 0.9124 | 0.8837 | 0.9116 | 0.9393 | |
Pilot | 4 | 0.7676 | 0.7675 | 0.7665 | 0.7440 | 0.7644 | 0.7686 | 0.7688 | 0.7512 |
6 | 0.8059 | 0.8241 | 0.8201 | 0.7920 | 0.8220 | 0.8259 | 0.7979 | 0.8317 | |
8 | 0.8584 | 0.8617 | 0.8621 | 0.8436 | 0.8620 | 0.8585 | 0.8619 | 0.8672 | |
10 | 0.8859 | 0.8857 | 0.8839 | 0.8781 | 0.8852 | 0.8787 | 0.8908 | 0.8937 | |
12 | 0.9031 | 0.9045 | 0.9100 | 0.8703 | 0.9036 | 0.8707 | 0.9009 | 0.9124 | |
Dog | 4 | 0.6534 | 0.6534 | 0.6218 | 0.5749 | 0.6510 | 0.6471 | 0.6582 | 0.6599 |
6 | 0.7021 | 0.7305 | 0.7252 | 0.7381 | 0.7333 | 0.6472 | 0.7290 | 0.7797 | |
8 | 0.7768 | 0.7801 | 0.7867 | 0.8020 | 0.7815 | 0.7875 | 0.7823 | 0.8486 | |
10 | 0.8039 | 0.8538 | 0.8278 | 0.8369 | 0.8574 | 0.7654 | 0.8338 | 0.9002 | |
12 | 0.8576 | 0.8860 | 0.8706 | 0.8378 | 0.8754 | 0.8326 | 0.8720 | 0.8889 |
IMAGE | K | SSA | MVO | DA | FPA | PSO | SCA | ALO | MALO |
Cactus | 4 | 0.8104 | 0.8104 | 0.8104 | 0.7856 | 0.8104 | 0.7991 | 0.8103 | 0.8104 |
6 | 0.8679 | 0.8673 | 0.8676 | 0.8305 | 0.8585 | 0.8280 | 0.8676 | 0.8681 | |
8 | 0.8967 | 0.8978 | 0.8936 | 0.8625 | 0.8912 | 0.8869 | 0.8974 | 0.8980 | |
10 | 0.9133 | 0.9153 | 0.9143 | 0.8868 | 0.9137 | 0.9029 | 0.9148 | 0.9153 | |
12 | 0.9250 | 0.9261 | 0.9264 | 0.9001 | 0.9218 | 0.9111 | 0.9272 | 0.9287 | |
Kangaroo | 4 | 0.8293 | 0.8293 | 0.8293 | 0.8005 | 0.8297 | 0.7913 | 0.8284 | 0.8293 |
6 | 0.8998 | 0.8999 | 0.8962 | 0.8585 | 0.8904 | 0.8642 | 0.9000 | 0.9001 | |
8 | 0.9325 | 0.9328 | 0.9325 | 0.8860 | 0.9246 | 0.8884 | 0.9330 | 0.9335 | |
10 | 0.9474 | 0.9521 | 0.9534 | 0.8914 | 0.9454 | 0.9055 | 0.9504 | 0.9541 | |
12 | 0.9607 | 0.9649 | 0.9638 | 0.9358 | 0.9594 | 0.9352 | 0.9630 | 0.9653 | |
Temple | 4 | 0.7391 | 0.7391 | 0.7391 | 0.7435 | 0.7388 | 0.7130 | 0.7374 | 0.7840 |
6 | 0.8178 | 0.7984 | 0.8181 | 0.8137 | 0.7968 | 0.7606 | 0.8189 | 0.8203 | |
8 | 0.8420 | 0.8693 | 0.8497 | 0.8270 | 0.8491 | 0.8671 | 0.8709 | 0.8722 | |
10 | 0.8628 | 0.8929 | 0.8805 | 0.8614 | 0.8615 | 0.8481 | 0.8888 | 0.8994 | |
12 | 0.9185 | 0.9135 | 0.8967 | 0.8820 | 0.8854 | 0.8994 | 0.9085 | 0.9301 | |
Flower | 4 | 0.7838 | 0.7838 | 0.7838 | 0.7604 | 0.7838 | 0.7726 | 0.7838 | 0.7841 |
6 | 0.8402 | 0.8413 | 0.8406 | 0.8177 | 0.8356 | 0.8142 | 0.8404 | 0.8418 | |
8 | 0.8829 | 0.8810 | 0.8864 | 0.8461 | 0.8790 | 0.8524 | 0.8828 | 0.8874 | |
10 | 0.9041 | 0.9100 | 0.9110 | 0.8639 | 0.9092 | 0.8791 | 0.9114 | 0.9120 | |
12 | 0.9246 | 0.9269 | 0.9191 | 0.8891 | 0.9195 | 0.8912 | 0.9333 | 0.9347 | |
Mountain | 4 | 0.8006 | 0.8006 | 0.8006 | 0.7658 | 0.8006 | 0.7918 | 0.7999 | 0.8006 |
6 | 0.8426 | 0.8561 | 0.8550 | 0.8225 | 0.8494 | 0.8335 | 0.8552 | 0.8563 | |
8 | 0.8893 | 0.8892 | 0.8866 | 0.8570 | 0.8824 | 0.8672 | 0.8901 | 0.8903 | |
10 | 0.9074 | 0.9094 | 0.9083 | 0.8789 | 0.9042 | 0.8793 | 0.9078 | 0.9142 | |
12 | 0.9217 | 0.9217 | 0.9214 | 0.8919 | 0.9211 | 0.8941 | 0.9182 | 0.9253 | |
Tree | 4 | 0.8055 | 0.8056 | 0.8055 | 0.7873 | 0.8060 | 0.8055 | 0.8058 | 0.8166 |
6 | 0.8562 | 0.8566 | 0.8562 | 0.8218 | 0.8479 | 0.8448 | 0.8562 | 0.8568 | |
8 | 0.8826 | 0.8825 | 0.8825 | 0.8408 | 0.8680 | 0.8423 | 0.8833 | 0.8840 | |
10 | 0.9038 | 0.9038 | 0.9041 | 0.8713 | 0.8981 | 0.8729 | 0.9026 | 0.9055 | |
12 | 0.9149 | 0.9203 | 0.9201 | 0.8984 | 0.9098 | 0.8789 | 0.9186 | 0.9208 | |
Horse | 4 | 0.8217 | 0.8217 | 0.8216 | 0.7960 | 0.8217 | 0.8099 | 0.8213 | 0.8217 |
6 | 0.8506 | 0.8504 | 0.8503 | 0.8472 | 0.8505 | 0.8388 | 0.8490 | 0.8506 | |
8 | 0.8849 | 0.8801 | 0.8848 | 0.8555 | 0.8687 | 0.8423 | 0.8827 | 0.8852 | |
10 | 0.9120 | 0.9082 | 0.9053 | 0.8773 | 0.9053 | 0.8708 | 0.9063 | 0.9122 | |
12 | 0.9269 | 0.9240 | 0.9279 | 0.8886 | 0.9254 | 0.8801 | 0.9202 | 0.9303 | |
Bridge | 4 | 0.8399 | 0.8399 | 0.8399 | 0.8276 | 0.8399 | 0.8276 | 0.8397 | 0.8399 |
6 | 0.8894 | 0.8971 | 0.8968 | 0.8706 | 0.8968 | 0.8613 | 0.8894 | 0.8974 | |
8 | 0.9235 | 0.9150 | 0.9223 | 0.8951 | 0.9203 | 0.8849 | 0.9202 | 0.9241 | |
10 | 0.9368 | 0.9350 | 0.9389 | 0.9098 | 0.9346 | 0.9192 | 0.9397 | 0.9398 | |
12 | 0.9478 | 0.9493 | 0.9470 | 0.9332 | 0.9469 | 0.9341 | 0.9453 | 0.9499 | |
Pilot | 4 | 0.7854 | 0.7854 | 0.7854 | 0.7765 | 0.7854 | 0.7736 | 0.7854 | 0.7855 |
6 | 0.8294 | 0.8260 | 0.8290 | 0.8070 | 0.8292 | 0.8290 | 0.8295 | 0.8345 | |
8 | 0.8623 | 0.8623 | 0.8619 | 0.8278 | 0.8531 | 0.8277 | 0.8617 | 0.8625 | |
10 | 0.8890 | 0.8819 | 0.8829 | 0.8710 | 0.8788 | 0.8547 | 0.8898 | 0.8918 | |
12 | 0.9033 | 0.9122 | 0.9079 | 0.8714 | 0.8905 | 0.8787 | 0.9101 | 0.9148 | |
Dog | 4 | 0.7911 | 0.7918 | 0.7911 | 0.7911 | 0.7911 | 0.7584 | 0.7911 | 0.7943 |
6 | 0.8497 | 0.8519 | 0.8467 | 0.8274 | 0.8347 | 0.8092 | 0.8494 | 0.8521 | |
8 | 0.8822 | 0.8793 | 0.8823 | 0.8436 | 0.8571 | 0.8452 | 0.8827 | 0.8834 | |
10 | 0.8932 | 0.9052 | 0.9049 | 0.8720 | 0.9002 | 0.8584 | 0.9050 | 0.9109 | |
12 | 0.9147 | 0.9277 | 0.9127 | 0.8882 | 0.9109 | 0.8965 | 0.9235 | 0.9288 |
IMAGE | K | SSA | MVO | DA | FPA | PSO | SCA | ALO | MALO |
Cactus | 4 | 0.7694 | 0.7700 | 0.7700 | 0.7508 | 0.7701 | 0.7453 | 0.7708 | 0.7711 |
6 | 0.8213 | 0.8263 | 0.8232 | 0.7858 | 0.8269 | 0.8315 | 0.8248 | 0.8363 | |
8 | 0.8598 | 0.8767 | 0.8766 | 0.8257 | 0.8672 | 0.8361 | 0.8674 | 0.8924 | |
10 | 0.8791 | 0.9048 | 0.8995 | 0.8791 | 0.9021 | 0.8787 | 0.9049 | 0.9227 | |
12 | 0.9164 | 0.9290 | 0.9154 | 0.9140 | 0.9278 | 0.8735 | 0.9260 | 0.9375 | |
Kangaroo | 4 | 0.7539 | 0.7539 | 0.7536 | 0.6738 | 0.7522 | 0.7172 | 0.7549 | 0.7574 |
6 | 0.8466 | 0.8609 | 0.8550 | 0.7787 | 0.8574 | 0.8118 | 0.8674 | 0.8713 | |
8 | 0.9112 | 0.9218 | 0.9113 | 0.8341 | 0.9214 | 0.8522 | 0.9196 | 0.9231 | |
10 | 0.9366 | 0.9456 | 0.9430 | 0.9110 | 0.9427 | 0.8605 | 0.9468 | 0.9482 | |
12 | 0.9543 | 0.9617 | 0.9609 | 0.9105 | 0.9617 | 0.8950 | 0.9596 | 0.9632 | |
Temple | 4 | 0.7190 | 0.7590 | 0.7310 | 0.7199 | 0.7282 | 0.6860 | 0.7570 | 0.7591 |
6 | 0.8107 | 0.8139 | 0.8107 | 0.7906 | 0.8129 | 0.7971 | 0.8111 | 0.8537 | |
8 | 0.8954 | 0.8796 | 0.8681 | 0.8172 | 0.8891 | 0.8519 | 0.8990 | 0.9067 | |
10 | 0.9103 | 0.9290 | 0.9146 | 0.8471 | 0.9256 | 0.7991 | 0.9226 | 0.9299 | |
12 | 0.9293 | 0.9389 | 0.9386 | 0.8743 | 0.9315 | 0.8680 | 0.9425 | 0.9501 | |
Flower | 4 | 0.7495 | 0.7505 | 0.7495 | 0.7293 | 0.7507 | 0.7504 | 0.7505 | 0.7508 |
6 | 0.8086 | 0.8097 | 0.8101 | 0.7886 | 0.8096 | 0.8024 | 0.8095 | 0.8202 | |
8 | 0.8317 | 0.8419 | 0.8411 | 0.8211 | 0.8494 | 0.8519 | 0.8464 | 0.8705 | |
10 | 0.8605 | 0.8796 | 0.8741 | 0.8586 | 0.8784 | 0.8538 | 0.8769 | 0.8935 | |
12 | 0.8736 | 0.9073 | 0.8994 | 0.8478 | 0.9015 | 0.8888 | 0.8969 | 0.9255 | |
Mountain | 4 | 0.7490 | 0.7600 | 0.7600 | 0.7470 | 0.7390 | 0.7640 | 0.7385 | 0.7857 |
6 | 0.8040 | 0.8101 | 0.8111 | 0.7933 | 0.8088 | 0.7753 | 0.8046 | 0.8142 | |
8 | 0.8467 | 0.8612 | 0.8539 | 0.8003 | 0.8485 | 0.8084 | 0.8498 | 0.8765 | |
10 | 0.8444 | 0.8899 | 0.8723 | 0.8368 | 0.8834 | 0.8370 | 0.8810 | 0.9131 | |
12 | 0.8988 | 0.9099 | 0.9081 | 0.8771 | 0.9113 | 0.8453 | 0.9067 | 0.9313 | |
Tree | 4 | 0.7803 | 0.7816 | 0.7803 | 0.7881 | 0.7804 | 0.7947 | 0.7800 | 0.7803 |
6 | 0.8303 | 0.8366 | 0.8311 | 0.8372 | 0.8304 | 0.8367 | 0.8340 | 0.8398 | |
8 | 0.8575 | 0.8602 | 0.8583 | 0.8362 | 0.8606 | 0.8490 | 0.8586 | 0.8795 | |
10 | 0.8741 | 0.8850 | 0.8855 | 0.8588 | 0.8862 | 0.8377 | 0.8889 | 0.8985 | |
12 | 0.8962 | 0.8987 | 0.8983 | 0.8545 | 0.9015 | 0.8627 | 0.9035 | 0.9141 | |
Horse | 4 | 0.7725 | 0.7725 | 0.7844 | 0.7942 | 0.8057 | 0.7902 | 0.7952 | 0.8125 |
6 | 0.8344 | 0.8467 | 0.8465 | 0.8092 | 0.8422 | 0.8260 | 0.8479 | 0.8604 | |
8 | 0.8722 | 0.8784 | 0.8781 | 0.8486 | 0.8800 | 0.8444 | 0.8832 | 0.8946 | |
10 | 0.8977 | 0.9053 | 0.9011 | 0.8600 | 0.9005 | 0.8459 | 0.9058 | 0.9087 | |
12 | 0.9126 | 0.9234 | 0.9201 | 0.8674 | 0.9186 | 0.8707 | 0.9207 | 0.9240 | |
Bridge | 4 | 0.8220 | 0.8221 | 0.8219 | 0.8042 | 0.8221 | 0.8083 | 0.8234 | 0.8378 |
6 | 0.8894 | 0.8909 | 0.8908 | 0.8475 | 0.8897 | 0.8495 | 0.8917 | 0.8928 | |
8 | 0.9137 | 0.9215 | 0.9209 | 0.8719 | 0.9208 | 0.8902 | 0.9215 | 0.9224 | |
10 | 0.9317 | 0.9350 | 0.9277 | 0.8925 | 0.9313 | 0.9038 | 0.9353 | 0.9467 | |
12 | 0.9394 | 0.9567 | 0.9453 | 0.9113 | 0.9503 | 0.9190 | 0.9476 | 0.9580 | |
Pilot | 4 | 0.7901 | 0.7894 | 0.7893 | 0.7740 | 0.7887 | 0.7850 | 0.7874 | 0.7912 |
6 | 0.8303 | 0.8409 | 0.8326 | 0.8103 | 0.8337 | 0.8292 | 0.8284 | 0.8343 | |
8 | 0.8726 | 0.8726 | 0.8749 | 0.8554 | 0.8755 | 0.8649 | 0.8751 | 0.8758 | |
10 | 0.8991 | 0.8872 | 0.8983 | 0.8829 | 0.8984 | 0.8782 | 0.9023 | 0.9063 | |
12 | 0.9158 | 0.9158 | 0.9225 | 0.8776 | 0.9177 | 0.8890 | 0.9175 | 0.9237 | |
Dog | 4 | 0.7595 | 0.7594 | 0.7375 | 0.7074 | 0.7580 | 0.7413 | 0.7621 | 0.7625 |
6 | 0.8043 | 0.8208 | 0.8182 | 0.8037 | 0.8205 | 0.7507 | 0.8212 | 0.8490 | |
8 | 0.8582 | 0.8617 | 0.8609 | 0.8404 | 0.8615 | 0.8355 | 0.8609 | 0.8911 | |
10 | 0.8772 | 0.9033 | 0.8918 | 0.8788 | 0.9032 | 0.8278 | 0.8976 | 0.9269 | |
12 | 0.9092 | 0.9254 | 0.9151 | 0.8727 | 0.9178 | 0.8642 | 0.9187 | 0.9275 |
IMAGE | K | PSNR | SSIM | FSIM | |||
Otsu | Kapur | Otsu | Kapur | Otsu | Kapur | ||
Cactus | 4 | 19.1901 | 17.2250 | 0.6063 | 0.4858 | 0.8104 | 0.7711 |
6 | 21.4849 | 20.9242 | 0.6963 | 0.5690 | 0.8681 | 0.8363 | |
8 | 23.7403 | 23.1098 | 0.7904 | 0.7681 | 0.8980 | 0.8924 | |
10 | 24.1499 | 25.6436 | 0.8391 | 0.8677 | 0.9153 | 0.9227 | |
12 | 25.2868 | 26.7329 | 0.8456 | 0.8873 | 0.9287 | 0.9375 | |
Kangaroo | 4 | 22.0797 | 18.8067 | 0.7735 | 0.6116 | 0.8293 | 0.7574 |
6 | 24.8836 | 22.3708 | 0.8627 | 0.7782 | 0.9001 | 0.8713 | |
8 | 27.0860 | 24.6322 | 0.9084 | 0.8521 | 0.9335 | 0.9231 | |
10 | 28.8186 | 26.1974 | 0.9331 | 0.8913 | 0.9541 | 0.9482 | |
12 | 30.3411 | 28.0349 | 0.9495 | 0.9254 | 0.9653 | 0.9632 | |
Temple | 4 | 17.6125 | 18.6105 | 0.6798 | 0.6149 | 0.7840 | 0.7591 |
6 | 21.4589 | 23.1506 | 0.7263 | 0.7784 | 0.8203 | 0.8537 | |
8 | 23.9977 | 25.1273 | 0.8152 | 0.8465 | 0.8722 | 0.9067 | |
10 | 25.7059 | 26.7757 | 0.8643 | 0.8787 | 0.8994 | 0.9299 | |
12 | 27.5232 | 28.4027 | 0.8917 | 0.9021 | 0.9301 | 0.9501 | |
Flower | 4 | 22.6916 | 21.4046 | 0.4916 | 0.3994 | 0.7841 | 0.7508 |
6 | 25.5506 | 24.2923 | 0.7406 | 0.5247 | 0.8418 | 0.8202 | |
8 | 27.6960 | 26.3947 | 0.8000 | 0.6431 | 0.8874 | 0.8705 | |
10 | 29.2169 | 27.6016 | 0.8294 | 0.6606 | 0.9120 | 0.8935 | |
12 | 30.7280 | 29.2750 | 0.8682 | 0.7643 | 0.9347 | 0.9255 | |
Mountain | 4 | 19.7307 | 17.7501 | 0.7081 | 0.6240 | 0.8006 | 0.7857 |
6 | 22.5039 | 20.2658 | 0.7932 | 0.7131 | 0.8563 | 0.8142 | |
8 | 24.9053 | 23.7160 | 0.8322 | 0.8259 | 0.8903 | 0.8765 | |
10 | 26.3373 | 26.2667 | 0.8603 | 0.8792 | 0.9142 | 0.9131 | |
12 | 27.4991 | 26.6949 | 0.8780 | 0.9085 | 0.9253 | 0.9313 | |
Tree | 4 | 19.9364 | 19.3878 | 0.6915 | 0.6264 | 0.8166 | 0.7803 |
6 | 22.9388 | 22.0355 | 0.7546 | 0.7219 | 0.8568 | 0.8398 | |
8 | 25.1704 | 24.2661 | 0.7960 | 0.7737 | 0.8840 | 0.8795 | |
10 | 26.7213 | 25.7360 | 0.8281 | 0.8214 | 0.9055 | 0.8985 | |
12 | 28.0724 | 26.8461 | 0.8579 | 0.8792 | 0.9208 | 0.9141 | |
Horse | 4 | 19.1569 | 19.7788 | 0.7206 | 0.7070 | 0.8217 | 0.8125 |
6 | 22.8301 | 21.6974 | 0.7744 | 0.7802 | 0.8506 | 0.8604 | |
8 | 24.8543 | 24.2427 | 0.8117 | 0.8335 | 0.8852 | 0.8946 | |
10 | 26.8845 | 26.1268 | 0.8534 | 0.8697 | 0.9122 | 0.9087 | |
12 | 28.3129 | 27.4180 | 0.8793 | 0.8979 | 0.9303 | 0.9240 | |
Bridge | 4 | 18.7684 | 18.3564 | 0.7398 | 0.7176 | 0.8399 | 0.8378 |
6 | 21.5346 | 21.1004 | 0.8193 | 0.8053 | 0.8974 | 0.8928 | |
8 | 23.7010 | 23.6124 | 0.8691 | 0.8820 | 0.9241 | 0.9224 | |
10 | 25.4265 | 25.3739 | 0.8993 | 0.9160 | 0.9398 | 0.9467 | |
12 | 27.0756 | 27.4320 | 0.9226 | 0.9393 | 0.9499 | 0.9580 | |
Pilot | 4 | 17.6971 | 16.0238 | 0.7399 | 0.7512 | 0.7855 | 0.7912 |
6 | 21.5230 | 20.7123 | 0.8224 | 0.8317 | 0.8345 | 0.8343 | |
8 | 22.4562 | 23.1598 | 0.8424 | 0.8672 | 0.8625 | 0.8758 | |
10 | 24.1912 | 24.8228 | 0.8788 | 0.8937 | 0.8918 | 0.9063 | |
12 | 25.8213 | 27.1095 | 0.9017 | 0.9124 | 0.9148 | 0.9237 | |
Dog | 4 | 19.9346 | 18.2605 | 0.7428 | 0.6599 | 0.7943 | 0.7625 |
6 | 22.6726 | 21.9672 | 0.7756 | 0.7797 | 0.8521 | 0.8490 | |
8 | 24.3548 | 24.5205 | 0.8240 | 0.8486 | 0.8834 | 0.8911 | |
10 | 26.4961 | 26.3186 | 0.8655 | 0.9002 | 0.9109 | 0.9269 | |
12 | 28.1328 | 27.2748 | 0.8948 | 0.8889 | 0.9288 | 0.9275 |
IMAGE | K | R | G | B |
Cactus | 4 | 48, 79,124,194 | 58, 90,126,187 | 54, 85,123,180 |
6 | 40, 63, 85,115,155,209 | 50, 74, 97,121,159,212 | 46, 67, 88,115,146,192 | |
8 | 36, 54, 71, 89,113,144,181,225 | 45, 64, 82, 99,118,142,177,221 | 41, 58, 74, 91,112,137,165,204 | |
10 | 34, 49, 63, 77, 91,109,133,160,190,227 | 43, 58, 73, 87,101,115,133,159,192,228 | 38, 51, 64, 77, 92,111,131,153,178,214 | |
12 | 33, 46, 58, 70, 82, 95,113,134,156,182,209,235 | 40, 53, 66, 79, 91,102,114,129,148,173,200,232 | 37, 48, 60, 71, 82, 96,112,130,147,166,189,220 | |
Kangaroo | 4 | 54, 87,116,155 | 49, 87,112,143 | 43, 72,103,145 |
6 | 41, 72, 92,113,140,173 | 38, 73, 95,113,134,163 | 32, 58, 77, 99,128,163 | |
8 | 34, 62, 80, 95,111,131,156,186 | 32, 62, 82, 97,111,125,145,175 | 28, 51, 67, 82,100,122,148,180 | |
10 | 31, 56, 72, 85, 97,110,126,145,166,195 | 28, 55, 74, 88,100,111,123,138,156,185 | 23, 44, 59, 71, 84, 99,117,138,161,190 | |
12 | 24, 47, 63, 76, 87, 97,108,122,139,157,177,206 | 24, 47, 65, 79, 91,101,110,120,131,145,162,187 | 19, 37, 51, 63, 73, 84, 96,111,128,147,167,196 | |
Temple | 4 | 80,116,152,207 | 81,111,141,178 | 62, 87,115,145 |
6 | 65, 89,113,137,165,213 | 71, 93,115,138,165,208 | 53, 70, 88,107,127,151 | |
8 | 57, 75, 94,112,130,149,172,216 | 64, 81, 98,115,132,150,172,210 | 47, 61, 74, 89,104,120,138,157 | |
10 | 55, 71, 87,103,118,134,151,172,200,234 | 60, 74, 88,102,115,129,143,160,178,212 | 45, 58, 69, 81, 95,109,124,141,159,239 | |
12 | 51, 65, 78, 92,105,117,130,142,156,174,201,233 | 57, 70, 83, 96,108,120,133,147,162,179,201,229 | 42, 53, 61, 69, 79, 90,101,111,122,135,148,162 | |
Flower | 4 | 51, 98,143,201 | 31, 69,111,168 | 19, 44, 75,119 |
6 | 34, 66, 98,131,163,209 | 22, 47, 77,109,150,202 | 7, 18, 37, 58, 84,126 | |
8 | 30, 55, 79,103,129,155,182,220 | 10, 25, 45, 68, 92,120,159,207 | 6, 14, 26, 42, 59, 78,102,140 | |
10 | 25, 44, 84,104,126,149,169,192,226 | 10, 23, 39, 57, 76, 95,117,144,178,218 | 6, 14, 26, 40, 56, 73, 94,126,176,252 | |
12 | 21, 36, 53, 70, 87,104,122,141,159,177,201,232 | 9, 20, 33, 48, 63, 79, 95,112,133,158,187,221 | 5, 10, 16, 25, 36, 48, 60, 73, 90,109,139,194 | |
Mountain | 4 | 50, 76,111,190 | 58, 91,124,190 | 58, 94,131,192 |
6 | 40, 58, 76,100,135,201 | 48, 69, 94,118,144,204 | 53, 76, 97,125,149,196 | |
8 | 36, 52, 67, 82,103,130,167,221 | 45, 63, 79, 99,118,139,174,224 | 39, 59, 78, 96,121,142,157,199 | |
10 | 34, 48, 60, 71, 84,102,123,148,187,230 | 2, 44, 62, 78, 96,112,124,144,182,229 | 38, 57, 75, 88,105,125,143,156,183,224 | |
12 | 34, 47, 58, 67, 76, 88,103,119,138,161,195,230 | 9, 43, 58, 69, 82, 98,111,122,137,157,189,233 | 37, 53, 68, 79, 92,110,127,141,151,160,183,224 | |
Tree | 4 | 30, 67,103,155 | 51,106,161,207 | 54,120,185,225 |
6 | 13, 35, 65, 96,132,175 | 39, 72,112,152,183,216 | 38, 74,125,173,203,229 | |
8 | 12, 31, 52, 72, 94,116,148,185 | 36, 63, 98,133,161,184,207,231 | 30, 52, 81,119,157,186,208,231 | |
10 | 10, 23, 39, 56, 74, 94,114,139,169,197 | 30, 50, 73,100,127,150,169,187,208,231 | 28, 46, 68, 97,130,160,184,202,215,234 | |
12 | 9, 22, 37, 51, 66, 82, 97,115,136,157,181,203 | 28, 45, 63, 84,107,130,150,166,179,192,210,233 | 24, 37, 51, 69, 92,119,147,171,190,205,217,235 | |
Horse | 4 | 59,100,145,193 | 54, 94,142,190 | 33, 61, 86,147 |
6 | 49, 83,111,143,179,208 | 46, 78,104,139,177,203 | 27, 51, 72, 93,145,193 | |
8 | 42, 70, 93,114,139,169,194,215 | 38, 65, 89,110,137,169,193,212 | 23, 44, 63, 80,101,148,189,200 | |
10 | 34, 56, 77, 95,111,130,155,180,200,218 | 30, 51, 71, 88,103,119,143,172,194,212 | 18, 33, 47, 60, 72, 84, 98,123,162,194 | |
12 | 29, 48, 67, 83, 98,112,127,147,168,187,204,220 | 29, 46, 63, 79, 93,106,120,142,166,185,200,215 | 17, 31, 44, 56, 67, 78, 89,103,128,164,190,201 | |
Bridge | 4 | 73,113,158,216 | 69,109,151,210 | 35, 75,116,159 |
6 | 61, 89,116,145,180,225 | 61, 92,120,148,181,224 | 24, 51, 80,110,139,169 | |
8 | 55, 77, 99,120,142,168,198,233 | 52, 74, 96,118,140,165,197,233 | 17, 36, 58, 81,105,127,148,172 | |
10 | 50, 68, 86,104,121,139,161,184,211,239 | 48, 67, 86,104,122,140,160,183,211,239 | 13, 29, 46, 63, 81,100,119,136,153,174 | |
12 | 48, 64, 79, 95,110,125,140,158,178,199,224,246 | 44, 60, 76, 91,106,122,137,154,172,192,218,243 | 11, 25, 40, 55, 71, 88,105,121,135,149,166,182 | |
Pilot | 4 | 102,149,194,224 | 104,157,205,235 | 83,123,167,220 |
6 | 90,120,155,191,217,234 | 88,117,150,187,217,239 | 78,111,149,181,212,243 | |
8 | 80,102,127,157,187,210,225,238 | 81,103,127,154,185,209,225,241 | 66, 87,109,136,165,188,214,243 | |
10 | 77, 96,116,135,157,180,200,216,228,239 | 76, 95,114,134,158,185,206,220,233,246 | 61, 78, 96,115,137,162,181,195,217,244 | |
12 | 72, 89,105,121,138,158,180,197,212,223,232,242 | 72, 86,100,117,136,159,184,203,216,226,237,248 | 61, 77, 94,112,132,154,172,185,196,214,234,248 | |
Dog | 4 | 50, 84,115,157 | 73,107,145,188 | 52, 83,123,161 |
6 | 38, 66, 89,112,133,168 | 61, 84,108,136,161,193 | 45, 67, 93,125,156,178 | |
8 | 33, 58, 78, 97,115,130,150,184 | 57, 75, 93,112,135,156,171,199 | 42, 60, 79,102,131,157,175,193 | |
10 | 30, 52, 70, 86,102,118,132,151,183,243 | 53, 69, 84,100,117,136,154,167,181,207 | 36, 49, 64, 80, 99,122,145,163,177,196 | |
12 | 24, 42, 57, 71, 84, 97,109,121,132,147,170,197 | 48, 61, 73, 87,101,115,131,147,159,169,183,209 | 33, 44, 57, 70, 83,100,121,141,157,168,179,196 |
IMAGE | K | R | G | B |
Cactus | 4 | 63,103,146,189 | 73,115,151,196 | 68,111,163,206 |
6 | 58, 92,121,153,185,216 | 62, 92,123,151,184,215 | 60, 95,129,162,190,222 | |
8 | 50, 77,102,128,154,180,204,229 | 56, 81,106,129,152,177,202,226 | 19, 53, 80,106,134,163,190,221 | |
10 | 43, 63, 83,103,122,143,164,185,207,230 | 49, 69, 89,109,129,148,169,191,212,233 | 19, 48, 71, 93,115,139,163,186,208,230 | |
12 | 17, 42, 64, 83,101,120,139,159,178,197,215,234 | 46, 63, 80, 97,115,132,148,165,183,201,219,237 | 19, 42, 58, 76, 94,112,130,148,166,187,208,231 | |
Kangaroo | 4 | 52,113,158,203 | 51, 90,138,188 | 40, 97,150,203 |
6 | 43, 75,113,148,184,215 | 47, 83,123,153,186,220 | 36, 68,102,142,185,220 | |
8 | 32, 58, 84,113,141,169,198,227 | 35, 61, 86,113,139,168,194,220 | 31, 57, 84,108,136,165,193,220 | |
10 | 24, 45, 66, 90,115,138,162,186,208,231 | 21, 42, 63, 86,109,133,153,175,195,220 | 25, 47, 69, 91,114,138,161,185,207,231 | |
12 | 20, 40, 58, 78, 98,117,136,157,178,198,215,234 | 17, 38, 56, 75, 94,114,132,152,171,190,210,229 | 18, 36, 55, 74, 94,115,135,154,174,194,214,233 | |
Temple | 4 | 74,114,153,192 | 82,120,158,195 | 46, 79,113,145 |
6 | 64, 96,128,160,194,225 | 72,103,134,163,196,226 | 25, 49, 80,113,144,178 | |
8 | 40, 65, 91,116,142,166,194,225 | 62, 89,116,143,169,196,216,236 | 23, 44, 67, 89,111,133,155,178 | |
10 | 39, 60, 82,103,126,149,170,194,214,235 | 46, 68, 89,109,130,151,173,196,216,235 | 18, 31, 47, 64, 81, 99,118,137,156,183 | |
12 | 35, 51, 69, 88,106,125,142,159,176,194,213,238 | 41, 55, 73, 90,107,123,141,159,178,196,216,235 | 18, 31, 46, 63, 80, 97,111,127,144,158,178,187 | |
Flower | 4 | 53, 99,143,187 | 49,106,153,202 | 27, 75,118,175 |
6 | 42, 75,108,143,177,206 | 32, 69,106,140,175,211 | 20, 51, 82,113,145,175 | |
8 | 37, 65, 94,121,149,177,202,227 | 25, 54, 83,112,140,169,196,223 | 20, 47, 75,102,127,150,175,197 | |
10 | 28, 50, 73, 95,116,137,159,183,205,228 | 22, 45, 68, 92,115,138,161,184,207,230 | 17, 36, 55, 76, 97,116,135,155,175,197 | |
12 | 26, 46, 66, 84,102,121,140,158,176,195,214,233 | 20, 39, 58, 78, 98,116,136,155,175,196,216,236 | 14, 27, 42, 56, 71, 87,103,118,135,155,175,198 | |
Mountain | 4 | 53, 89,124,156 | 87,138,177,218 | 88,132,173,215 |
6 | 53, 91,129,168,199,232 | 73,103,138,172,207,241 | 19, 63, 95,132,173,215 | |
8 | 52, 81,107,134,162,188,216,245 | 51, 77,102,136,160,185,213,241 | 19, 46, 65, 87,109,133,173,210 | |
10 | 22, 51, 74, 97,123,147,168,192,218,245 | 51, 76,101,119,138,160,181,203,223,244 | 19, 45, 65, 87,109,131,151,173,199,227 | |
12 | 21, 40, 60, 82,103,124,145,167,185,205,224,247 | 48, 64, 82,101,120,137,154,172,188,206,224,244 | 19, 45, 65, 89,111,132,151,172,185,200,218,236 | |
Tree | 4 | 51, 92,134,182 | 63,112,157,206 | 56, 99,148,192 |
6 | 29, 63, 97,132,168,206 | 45, 77,110,141,170,207 | 44, 78,113,151,190,225 | |
8 | 25, 55, 83,110,134,162,190,218 | 39, 66, 94,123,152,180,206,230 | 38, 65, 91,118,145,170,196,225 | |
10 | 22, 43, 65, 88,111,133,155,178,198,219 | 33, 54, 75, 96,117,139,160,183,206,229 | 33, 55, 77, 99,119,140,160,182,203,225 | |
12 | 21, 42, 63, 84,108,131,149,167,183,200,218,235 | 29, 47, 65, 83,100,117,134,152,168,187,206,230 | 28, 46, 63, 81, 99,119,138,156,174,190,206,225 | |
Horse | 4 | 52, 91,136,187 | 68,124,176,235 | 53,117,174,214 |
6 | 43, 77,111,142,177,213 | 49, 86,126,161,193,235 | 35, 67,103,134,173,214 | |
8 | 35, 62, 88,115,141,169,194,222 | 38, 67, 96,127,156,184,211,235 | 30, 59, 89,114,142,173,192,214 | |
10 | 31, 55, 77, 99,123,144,167,191,213,235 | 32, 55, 78,102,127,150,172,193,213,235 | 22, 44, 64, 86,105,125,148,173,192,214 | |
12 | 27, 46, 66, 85,104,123,142,160,178,198,219,239 | 28, 46, 64, 82,100,120,136,156,176,195,215,235 | 19, 35, 51, 70, 87,103,119,136,155,173,190,214 | |
Bridge | 4 | 70,113,156,199 | 77,129,180,227 | 49, 96,142,194 |
6 | 63, 98,133,167,201,236 | 60, 93,126,159,193,230 | 32, 62, 92,122,155,194 | |
8 | 54, 80,106,132,158,184,209,236 | 52, 78,105,132,158,185,210,236 | 26, 49, 73, 97,121,146,171,195 | |
10 | 48, 70, 92,113,134,156,177,198,218,239 | 47, 68, 90,111,133,155,177,198,219,238 | 24, 45, 68, 90,112,133,155,176,194,209 | |
12 | 45, 62, 79, 97,114,132,149,167,184,201,220,239 | 44, 62, 80, 99,116,134,152,169,186,204,222,240 | 22, 40, 58, 74, 89,105,121,139,157,176,194,209 | |
Pilot | 4 | 94,134,169,204 | 100,146,191,220 | 74,106,139,173 |
6 | 81,110,139,168,197,221 | 81,108,135,163,192,220 | 41, 85,125,168,203,235 | |
8 | 72, 96,119,143,168,194,216,238 | 74, 98,121,145,168,191,210,231 | 34, 67, 92,118,144,171,203,235 | |
10 | 69, 87,106,125,144,164,183,202,220,238 | 70, 89,108,127,146,164,182,200,218,236 | 28, 42, 68, 91,115,139,163,182,205,235 | |
12 | 55, 70, 86,103,120,136,151,168,185,203,220,238 | 67, 82, 98,113,129,145,160,175,191,206,221,238 | 28, 42, 67, 88,110,130,149,168,185,205,227,245 | |
Dog | 4 | 43, 92,146,187 | 74,112,149,192 | 62,102,149,200 |
6 | 38, 73,110,145,174,206 | 62, 90,119,150,184,215 | 56, 91,124,157,195,217 | |
8 | 29, 55, 84,113,144,167,190,214 | 61, 87,113,138,159,184,206,233 | 46, 72, 97,124,152,175,196,217 | |
10 | 21, 40, 60, 80,100,120,144,167,190,214 | 55, 76, 99,120,140,159,182,198,215,233 | 18, 45, 68, 91,114,137,157,176,196,217 | |
12 | 20, 39, 57, 75, 92,110,127,144,160,179,198,217 | 49, 66, 82, 99,117,133,149,165,182,197,215,233 | 18, 39, 56, 72, 89,105,122,141,159,178,196,217 |
Algorithm | Parameters | Value |
SSA | Balance coefficient c1 | [0, 2] |
Random number c2, c3 | [0, 1] | |
Switch possibility | 0.5 | |
MVO | Wormhole Existence Probability | [0.2, 1] |
Travelling Distance Rate | [0, 1] | |
Random number r1, r2, r3 | [0, 1] | |
DA | Inertial weight ω | [0.5, 0.9] |
Seperation weight | [0, 0.2] | |
Alignment weight | [0, 0.2] | |
Cohesion weight | [0, 0.2] | |
Food factor | [0, 2] | |
Enemy factor | [0, 0.1] | |
FPA | Switch possibility | 0.4 |
Lévy constant β | 1.5 | |
PSO | Maximum inertia weight | 0.9 |
Minimum inertia weight | 0.4 | |
Learning factors c1, c2 | 2 | |
Maximum velocity | +120 | |
Minimum velocity | −120 | |
SCA | Movement direction r1 | [0, 2] |
Movement distance r2 | [0, 2π] | |
Random weight r3 | [0, 2] | |
Random number r4 | [0, 1] | |
Switch possibility | 0.5 | |
ALO | Switch possibility | 0.5 |
MABC | Random number r | [0, 1] |
IDSA | Random number r | [0, 1] |
WOA-TH | Parameter a | [0, 2] |
Constant b | 1 | |
Random number l | [−1, 1] | |
Constant a0 | 13 | |
Initial value G0 | 40 | |
BDE | Number of objectives | 1 |
Number of constraints | 0 | |
Number of decision variables | 4 | |
Scaling factor | 0.5 | |
Crossover probability | 0.2 |
Algorithm 1. Pseudo code of MALO algorithm based multilevel thresholding. | |
1 | Input the image and calculate components of the histogram. |
2 | Initialize parameters: SearchAgents_no (the number of search agents), T (maximum number of iterations). |
3 | Calculate the fitness of initial ants and antlions; |
4 | Find the best antlions and assume it as the elite; |
5 | While t < T |
6 | For each ant |
7 | Select an antlion using Roulette wheel; |
8 | Update c and d using Eqs (16) and (17); |
9 | Create a random walk and normalize it using Eqs (11) and (13); |
10 | Update the position of ant using Eq (19); |
11 | End for |
12 | For each the position of ant |
13 | Generate the opposite solution of the position of ant using Eq (22); |
14 | Calculate the fitness of original position and opposite position of ant; |
15 | Compare fitness values and keep ant fitness and position with high fitness value; |
16 | End for |
17 | Update antlion positions and finesses based of the ants Eq (20); |
18 | Update the position of elite if any antlions becomes fitter than it; |
19 | t = t + 1; |
20 | End while |
21 | Return elite fitness and position, which elite position represents the optimal thresholds. |
22 | Output the values of the evaluation measures and the segmented images. |
F | SSA | MVO | DA | FPA | PSO | SCA | ALO | MALO | |
F1 | Mean | 2.7525 × 103 | 2.0775 × 104 | 5.3030 × 107 | 9.4246 × 107 | 2.3768 × 103 | 1.1165 × 109 | 1.6205 × 103 | 2.2691 × 102 |
Std | 3.1853 × 103 | 1.0204 × 104 | 1.3048 × 108 | 1.6194 × 108 | 3.3853 × 103 | 2.6690 × 108 | 1.6927 × 103 | 7.5223 × 102 | |
F3 | Mean | 3.0002 × 102 | 3.0015 × 102 | 3.3792 × 103 | 5.6230 × 103 | 3.0009 × 102 | 3.0174 × 103 | 4.5012 × 102 | 4.4652 × 102 |
Std | 8.4151 × 10−2 | 7.5858 × 10−2 | 3.5353 × 103 | 3.7208 × 103 | 7.7137 × 10−2 | 1.6524 × 103 | 3.7837 × 102 | 3.1829 × 102 | |
F4 | Mean | 4.0989 × 102 | 4.0702 × 102 | 4.2856 × 102 | 4.6977 × 102 | 4.0507 × 102 | 4.6709 × 102 | 4.1395 × 102 | 4.0394 × 102 |
Std | 16.908 | 9.3281 | 29.903 | 61.297 | 1.2334 | 30.876 | 22.338 | 12.248 | |
F5 | Mean | 5.2912 × 102 | 5.2264 × 102 | 5.1732 × 102 | 5.6206 × 102 | 5.4292 × 102 | 5.5799 × 102 | 5.2180 × 102 | 5.1692 × 102 |
Std | 12.497 | 1.1788 × 10 | 8.4145 | 21.327 | 14.890 | 6.6091 | 13.640 | 2.8811 | |
F6 | Mean | 6.1533 × 102 | 6.0237 × 102 | 6.0125 × 102 | 6.3945 × 102 | 6.1021 × 102 | 6.2361 × 102 | 6.1562 × 102 | 6.7956 × 102 |
Std | 8.9433 | 3.1079 | 1.4272 | 16.075 | 8.8947 | 5.4162 | 8.8988 | 10.159 | |
F7 | Mean | 7.4500 × 102 | 7.3257 × 102 | 7.3766 × 102 | 7.9322 × 102 | 7.3056 × 102 | 7.8595 × 102 | 7.5048 × 102 | 7.2403 × 102 |
Std | 15.007 | 12.483 | 14.458 | 26.384 | 12.387 | 10.193 | 26.572 | 2.3596 | |
F8 | Mean | 8.2630 × 102 | 8.2696 × 102 | 8.1554 × 102 | 8.4529 × 102 | 8.2131 × 102 | 8.4537 × 102 | 8.2771× 102 | 8.1001 × 102 |
Std | 11.690 | 12.498 | 7.2712 | 16.236 | 7.9025 | 8.7128 | 14.163 | 9.4471 | |
F9 | Mean | 1.0897 × 103 | 9.0116 × 102 | 9.2457 × 102 | 1.7066 × 103 | 9.6607 × 102 | 1.0671 × 103 | 1.1272 × 103 | 1.0417 × 103 |
Std | 2.5134 × 102 | 2.8801 | 51.037 | 6.1484 × 102 | 1.1193 × 102 | 81.732 | 2.2796 × 102 | 1.8741 × 102 | |
F10 | Mean | 1.8446 × 103 | 1.7723 × 103 | 1.7989 × 103 | 2.1907 × 103 | 1.9995 × 103 | 2.5054 × 103 | 2.0639 × 103 | 1.2226 × 103 |
Std | 2.8235 × 102 | 2.8547 × 102 | 3.4047 × 102 | 3.9008 × 102 | 2.9524 × 102 | 2.0358 × 102 | 3.1110 × 102 | 1.7148 × 102 | |
F11 | Mean | 1.1840 × 103 | 1.1325 × 103 | 1.2913 × 103 | 1.2604 × 103 | 1.1388 × 103 | 1.2494 × 103 | 1.2125 × 103 | 9.3895 × 104 |
Std | 71.184 | 27.254 | 8.0087 × 102 | 1.1285 × 102 | 2.7432 × 105 | 76.249 | 74.386 | 24.745 | |
F12 | Mean | 3.1659 × 106 | 1.9237 × 106 | 8.5333 × 105 | 5.3131 × 106 | 2.8460 × 109 | 3.6304 × 107 | 2.9647 × 106 | 2.2571 × 104 |
Std | 3.9918 × 106 | 2.4011 × 106 | 1.0164 × 106 | 5.2872 × 106 | 1.4432 × 109 | 4.6673 × 107 | 3.6635 × 106 | 3.1055 × 104 | |
F13 | Mean | 1.7372 × 104 | 1.2349 × 104 | 1.6552 × 104 | 2.3449 × 104 | 3.2613 × 108 | 8.9513 × 104 | 1.3896 × 104 | 1.1517 × 104 |
Std | 1.2611 × 104 | 9.7419 × 103 | 9.0887 × 103 | 1.8794 × 104 | 2.7709 × 108 | 8.0007 × 104 | 1.2201 × 104 | 7.8833 × 103 | |
F14 | Mean | 3.3621 × 103 | 3.0338 × 103 | 4.8484 × 103 | 2.8067 × 103 | 2.8688 × 103 | 2.3311 × 103 | 2.6070 × 103 | 2.3278 × 103 |
Std | 2.7150 × 103 | 2.3148 × 103 | 1.8854 × 103 | 1.4364 × 103 | 2.1263 × 103 | 1.0895 × 103 | 1.6260 × 103 | 1.2880 × 103 | |
F15 | Mean | 6.2001 × 103 | 3.3924 × 103 | 6.4284 × 103 | 9.6586 × 103 | 3.9125 × 103 | 3.7996 × 103 | 1.3375 × 104 | 3.0564 × 103 |
Std | 4.2086 × 103 | 3.5797 × 103 | 5.0026 × 103 | 7.7162 × 103 | 3.7024 × 103 | 2.3791 × 103 | 1.0871 × 104 | 2.1255 × 103 | |
F16 | Mean | 1.8063 × 103 | 1.8309 × 103 | 1.7338 × 103 | 1.9840 × 103 | 1.8428 × 103 | 1.8126 × 103 | 1.8928 × 103 | 1.8066 × 103 |
Std | 1.4760 × 102 | 1.6725 × 102 | 1.2125 × 102 | 1.4716 × 102 | 1.2492 × 102 | 80.085 | 1.6074 × 102 | 1.0356 × 102 | |
F17 | Mean | 1.7831 × 103 | 1.7871 × 103 | 1.7693 × 103 | 1.8044 × 103 | 1.7796 × 103 | 1.7897 × 103 | 1.7795 × 103 | 1.7359 × 103 |
Std | 38.227 | 56.931 | 33.562 | 52.636 | 59.130 | 14.372 | 39.757 | 34.260 | |
F18 | Mean | 1.9046 × 104 | 1.7527 × 104 | 2.4433 × 104 | 1.5703 × 104 | 1.6595 × 104 | 4.2187 × 105 | 1.6102 × 104 | 1.6102 × 104 |
Std | 1.3350 × 104 | 1.1493 × 104 | 1.5706 × 104 | 1.2231 × 104 | 1.3320 × 104 | 4.6787 × 105 | 1.3045 × 104 | 1.2885 × 104 | |
F19 | Mean | 9.3321 × 103 | 4.8206 × 103 | 1.1236 × 104 | 9.2778 × 104 | 5.1293 × 103 | 1.0034 × 104 | 1.6927 × 104 | 1.9544 × 103 |
Std | 7.1553 × 103 | 4.0080 × 103 | 6.8870 × 103 | 2.0401 × 105 | 3.8240 × 103 | 8.8662 × 103 | 1.2359 × 104 | 3.1306 × 103 | |
F20 | Mean | 2.1580 × 103 | 2.1181 × 103 | 2.1072 × 103 | 2.1974 × 103 | 2.1351 × 103 | 2.1393 × 103 | 2.1520 × 103 | 2.1017 × 103 |
Std | 77.357 | 71.982 | 59.164 | 81.489 | 81.620 | 37.232 | 76.695 | 1.1244 × 102 | |
F21 | Mean | 2.2892 × 103 | 2.3067 × 103 | 2.3146 × 103 | 2.3260 × 103 | 2.3033 × 103 | 2.2951 × 103 | 2.3181 × 103 | 2.2919 × 103 |
Std | 58.652 | 43.950 | 23.354 | 56.359 | 69.965 | 67.337 | 23.092 | 23.025 | |
F22 | Mean | 2.3032 × 103 | 2.4325 × 103 | 2.3387 × 103 | 2.3737 × 103 | 2.3168 × 103 | 2.3923 × 103 | 2.3019 × 103 | 2.2108 × 103 |
Std | 1.5330 | 4.0570 × 102 | 1.5916 × 102 | 3.0758 × 102 | 86.302 | 36.970 | 14.139 | 4.3619 | |
F23 | Mean | 2.6246 × 103 | 2.6213 × 103 | 2.6263 × 103 | 2.6544 × 103 | 2.6993 × 103 | 2.6630 × 103 | 2.6330 × 103 | 2.6235 × 103 |
Std | 9.4651 | 8.4456 | 11.398 | 27.680 | 38.621 | 9.4154 | 13.016 | 8.6164 | |
F24 | Mean | 2.7448 × 103 | 2.7399E+03 | 2.7422E+03 | 2.7726E+03 | 2.7821E+03 | 2.7773E+03 | 2.7541E+03 | 2.7242E+03 |
Std | 48.315 | 45.915 | 47.575 | 55.733 | 1.1838 × 102 | 59.112 | 13.296 | 10.259 | |
F25 | Mean | 2.9316 × 103 | 2.9322 × 103 | 2.9355 × 103 | 2.9755 × 103 | 2.9227 × 103 | 2.9816 × 103 | 2.9352 × 103 | 2.9316 × 103 |
Std | 30.687 | 28.163 | 23.579 | 53.181 | 23.221 | 24.991 | 28.272 | 2.7704 × 102 | |
F26 | Mean | 2.9852 × 103 | 3.0412 × 103 | 3.1910 × 103 | 3.5113 × 103 | 3.2078 × 103 | 3.1238 × 103 | 3.0689 × 103 | 3.0597 × 103 |
Std | 2.7007 × 102 | 3.8042 × 102 | 3.9782 × 102 | 4.9123 × 102 | 5.0084 × 102 | 37.746 | 2.7801 × 102 | 2.5490 × 102 | |
F27 | Mean | 3.0942 × 103 | 3.0936 × 103 | 3.0975 × 103 | 3.1538 × 103 | 3.1493 × 103 | 3.1084 × 103 | 3.1028 × 103 | 3.0346 × 103 |
Std | 3.6648 | 3.4486 | 6.0913 | 55.897 | 67.675 | 4.4917 | 15.597 | 1.4249 | |
F28 | Mean | 3.3187 × 103 | 3.3510 × 103 | 3.3579 × 103 | 3.4196 × 103 | 3.2028 × 103 | 3.3398 × 103 | 3.3863 × 103 | 3.1170 × 103 |
Std | 1.6379 × 102 | 1.3529 × 102 | 95.702 | 1.6486 × 102 | 78.068 | 90.179 | 1.3401 × 102 | 1.0508 × 102 | |
F29 | Mean | 3.2573 × 103 | 3.2282 × 103 | 3.2212 × 103 | 3.4234 × 103 | 3.2563 × 103 | 3.2629 × 103 | 3.2707 × 103 | 3.1694 × 103 |
Std | 81.947 | 83.366 | 55.368 | 1.3273 × 102 | 82.282 | 33.745 | 94.979 | 22.526 | |
F30 | Mean | 5.4626 × 105 | 5.5507 × 105 | 1.0112 × 106 | 2.2409 × 106 | 6.8210 × 104 | 1.7827 × 106 | 7.0103 × 105 | 1.5116 × 104 |
Std | 5.8213 × 105 | 6.5747 × 105 | 1.3632 × 106 | 2.9983 × 106 | 6.4354 × 104 | 1.3111 × 106 | 2.1161 × 106 | 7.2776 × 104 |
Image | K | SSA | MVO | DA | FPA | PSO | SCA | ALO | MALO |
Cactus | 4 | 2126.2412 | 2126.2412 | 2126.2412 | 2084.2192 | 2126.2085 | 2091.2043 | 2126.1077 | 2126.2412 |
6 | 2188.8026 | 2188.7881 | 2188.7749 | 2152.9126 | 2180.5976 | 2151.3710 | 2188.6529 | 2188.8076 | |
8 | 2213.1588 | 2213.1207 | 2210.6763 | 2185.1975 | 2206.7101 | 2177.4155 | 2212.8736 | 2213.2108 | |
10 | 2224.0745 | 2224.9921 | 2224.4972 | 2202.9660 | 2224.8409 | 2205.3385 | 2223.8535 | 2225.0378 | |
12 | 2228.0349 | 2231.4043 | 2227.1859 | 2208.4757 | 2230.3736 | 2209.0205 | 2230.9917 | 2231.8810 | |
Kangaroo | 4 | 1114.7964 | 1114.7964 | 1114.7964 | 1088.7665 | 1114.7783 | 1088.6203 | 1114.7451 | 1114.7964 |
6 | 1164.7339 | 1164.7235 | 1162.2148 | 1131.7231 | 1157.9995 | 1142.5799 | 1164.5055 | 1164.7463 | |
8 | 1186.9998 | 1187.2317 | 1186.4981 | 1164.0753 | 1181.4079 | 1157.1404 | 1187.0901 | 1187.3921 | |
10 | 1195.5800 | 1198.8061 | 1198.1305 | 1175.6658 | 1195.7200 | 1172.3154 | 1197.6616 | 1199.0721 | |
12 | 1201.3621 | 1204.3553 | 1204.0964 | 1189.4752 | 1203.7897 | 1186.9269 | 1204.8168 | 1205.6190 | |
Temple | 4 | 1510.8080 | 1511.3099 | 1511.3110 | 1481.4555 | 1511.2952 | 1484.9151 | 1511.1720 | 1511.3110 |
6 | 1562.1584 | 1551.2497 | 1562.1657 | 1539.3204 | 1547.2460 | 1528.3151 | 1562.0323 | 1562.1677 | |
8 | 1575.4345 | 1581.9379 | 1580.1512 | 1558.9731 | 1577.2711 | 1552.2760 | 1578.5870 | 1582.0010 | |
10 | 1585.0462 | 1589.3769 | 1588.9537 | 1566.9471 | 1583.4601 | 1562.6224 | 1589.3855 | 1590.0709 | |
12 | 1593.4902 | 1592.3625 | 1594.7102 | 1577.6113 | 1589.2313 | 1577.5018 | 1595.2350 | 1596.7043 | |
Flower | 4 | 2319.2628 | 2319.2628 | 2319.2628 | 2300.8905 | 2319.2628 | 2305.0308 | 2319.2088 | 2319.2628 |
6 | 2373.7148 | 2373.9784 | 2373.9666 | 2349.7530 | 2366.7255 | 2348.8170 | 2373.7751 | 2374.0062 | |
8 | 2398.3260 | 2397.7703 | 2398.6865 | 2373.7415 | 2393.6744 | 2378.1142 | 2398.0974 | 2398.7056 | |
10 | 2408.6487 | 2409.1083 | 2410.1377 | 2392.7685 | 2409.5320 | 2393.4470 | 2409.9581 | 2410.1748 | |
12 | 2415.5456 | 2416.1915 | 2413.2197 | 2401.7458 | 2413.6013 | 2402.8505 | 2416.6995 | 2417.4773 | |
Mountain | 4 | 1922.6304 | 1922.6304 | 1922.6304 | 1880.8725 | 1922.6304 | 1899.0075 | 1922.5604 | 1922.6304 |
6 | 1966.3537 | 1969.5924 | 1969.5227 | 1950.3434 | 1964.7413 | 1937.2954 | 1969.3259 | 1969.6134 | |
8 | 1987.6882 | 1987.6512 | 1987.6006 | 1971.0006 | 1982.4353 | 1963.1189 | 1987.3940 | 1989.0684 | |
10 | 1998.2296 | 1998.3989 | 1999.2217 | 1976.2590 | 1995.3262 | 1980.4772 | 1998.6023 | 1999.5736 | |
12 | 2002.3212 | 2003.5176 | 2003.4479 | 1991.7908 | 2002.3326 | 1987.1898 | 2003.1927 | 2004.4332 | |
Tree | 4 | 4836.5287 | 4836.5254 | 4836.5287 | 4811.7023 | 4836.5184 | 4799.5909 | 4836.4226 | 4836.5287 |
6 | 4910.3426 | 4910.3170 | 4910.3328 | 4881.3483 | 4899.1851 | 4889.6357 | 4909.9888 | 4910.3389 | |
8 | 4938.5519 | 4938.7280 | 4939.0548 | 4895.7628 | 4932.5126 | 4897.8287 | 4939.0439 | 4939.2738 | |
10 | 4954.4191 | 4954.3250 | 4954.3494 | 4931.0184 | 4950.5741 | 4925.6878 | 4953.6297 | 4954.7408 | |
12 | 4961.5040 | 4962.0370 | 4961.7003 | 4947.5680 | 4958.4948 | 4933.2448 | 4961.9255 | 4962.8681 | |
Horse | 4 | 4169.9500 | 4169.9500 | 4169.9475 | 4146.0648 | 4169.9500 | 4125.8875 | 4169.9152 | 4169.9500 |
6 | 4223.2790 | 4226.2869 | 4226.2925 | 4206.4679 | 4226.2960 | 4184.3739 | 4226.1384 | 4226.2981 | |
8 | 4251.0736 | 4247.9571 | 4250.7202 | 4224.2255 | 4242.3477 | 4224.8735 | 4251.0627 | 4251.0806 | |
10 | 4264.1067 | 4264.0484 | 4263.6881 | 4245.5168 | 4263.0609 | 4242.4543 | 4263.8776 | 4264.5464 | |
12 | 4271.0552 | 4270.2470 | 4270.4589 | 4253.0366 | 4270.9270 | 4255.1561 | 4269.9287 | 4272.7303 | |
Bridge | 4 | 4386.0155 | 4386.0155 | 4386.0155 | 4348.0212 | 4386.0155 | 4364.6402 | 4385.8679 | 4386.0155 |
6 | 4448.8450 | 4456.6582 | 4456.6941 | 4430.7839 | 4456.6543 | 4416.6522 | 4448.6228 | 4456.6941 | |
8 | 4483.9318 | 4476.1230 | 4481.9799 | 4459.7426 | 4480.7550 | 4436.7087 | 4480.4869 | 4484.0957 | |
10 | 4495.8304 | 4494.1137 | 4497.7496 | 4475.7876 | 4494.2483 | 4472.8251 | 4497.6619 | 4498.1770 | |
12 | 4505.0626 | 4504.6934 | 4502.9179 | 4488.9690 | 4504.8794 | 4485.6536 | 4504.6902 | 4506.5159 | |
Pilot | 4 | 3854.8970 | 3854.8970 | 3854.8970 | 3838.0151 | 3854.8930 | 3807.6575 | 3854.8127 | 3854.8970 |
6 | 3902.7853 | 3896.5334 | 3902.7747 | 3884.5667 | 3902.7796 | 3869.1994 | 3902.6187 | 3902.7731 | |
8 | 3917.0447 | 3917.7421 | 3920.6198 | 3892.5037 | 3918.0121 | 3895.6690 | 3920.4228 | 3923.5580 | |
10 | 3931.0115 | 3928.4714 | 3933.5071 | 3915.8877 | 3931.1832 | 3904.4969 | 3932.5873 | 3934.2915 | |
12 | 3937.8965 | 3936.9459 | 3936.6517 | 3916.5100 | 3935.4087 | 3916.0317 | 3939.2200 | 3940.3098 | |
Dog | 4 | 2249.9855 | 2249.9839 | 2249.9855 | 2231.2675 | 2249.9855 | 2233.1946 | 2249.9484 | 2249.9855 |
6 | 2299.7269 | 2299.6899 | 2298.6466 | 2270.5848 | 2287.9683 | 2277.7186 | 2299.5470 | 2299.7446 | |
8 | 2320.5275 | 2317.0263 | 2320.4939 | 2297.8192 | 2303.3155 | 2296.9113 | 2320.2281 | 2320.5319 | |
10 | 2326.4485 | 2329.6067 | 2330.9585 | 2311.9971 | 2328.1692 | 2306.4227 | 2330.4593 | 2330.9965 | |
12 | 2334.7496 | 2336.2099 | 2334.3909 | 2323.5986 | 2332.0819 | 2320.2905 | 2335.7878 | 2337.4348 |
Image | K | SSA | MVO | DA | FPA | PSO | SCA | ALO | MALO |
Cactus | 4 | 18.5843 | 18.5843 | 18.5843 | 18.3930 | 18.5829 | 18.4259 | 18.5832 | 18.5843 |
6 | 23.8329 | 23.8233 | 23.8386 | 23.2412 | 23.8389 | 23.2857 | 23.8364 | 23.8420 | |
8 | 28.4940 | 28.3247 | 28.5120 | 27.0680 | 28.4911 | 27.3938 | 28.4814 | 28.5198 | |
10 | 32.6224 | 32.0489 | 32.7875 | 30.4517 | 32.8282 | 30.7148 | 32.7883 | 32.8463 | |
12 | 36.7255 | 35.3654 | 36.6688 | 34.7456 | 36.7983 | 34.2975 | 36.6721 | 36.8450 | |
Kangaroo | 4 | 18.9363 | 18.9353 | 18.9361 | 18.6549 | 18.9352 | 18.7771 | 18.9350 | 18.9363 |
6 | 24.4683 | 24.4414 | 24.4720 | 23.6425 | 24.4648 | 24.0457 | 24.4563 | 24.4753 | |
8 | 29.3790 | 29.2133 | 29.3699 | 28.7933 | 29.4014 | 28.2712 | 29.4026 | 29.4202 | |
10 | 33.8064 | 33.4514 | 33.8602 | 32.6267 | 33.1534 | 32.0603 | 33.8653 | 33.9054 | |
12 | 37.8707 | 37.1153 | 37.9876 | 36.2125 | 38.0001 | 35.4540 | 37.9065 | 38.0410 | |
Temple | 4 | 17.8159 | 17.8201 | 17.8061 | 17.4725 | 17.8046 | 17.5013 | 17.8195 | 17.8204 |
6 | 22.9388 | 22.7544 | 22.9388 | 22.2007 | 22.8940 | 22.0680 | 22.9246 | 22.9388 | |
8 | 27.5032 | 27.2493 | 27.4436 | 25.9640 | 27.4909 | 25.5465 | 27.5208 | 27.5351 | |
10 | 31.6211 | 30.6718 | 31.6425 | 29.5279 | 31.6160 | 28.9053 | 31.6706 | 31.7574 | |
12 | 34.2789 | 33.0925 | 35.3472 | 31.7622 | 34.7704 | 32.2631 | 35.3818 | 35.6116 | |
Flower | 4 | 18.7000 | 18.6993 | 18.7000 | 18.4447 | 18.6996 | 18.4765 | 18.6980 | 18.7005 |
6 | 24.0481 | 24.0186 | 24.0485 | 23.6039 | 24.0429 | 23.3247 | 24.0441 | 24.0511 | |
8 | 28.7647 | 28.6438 | 28.7955 | 27.6104 | 28.7721 | 27.7280 | 28.7727 | 28.8057 | |
10 | 33.0270 | 32.9200 | 33.1672 | 31.2963 | 33.1582 | 31.3512 | 33.1673 | 33.1976 | |
12 | 36.8699 | 35.9637 | 37.1214 | 34.8362 | 37.0372 | 34.9773 | 36.9291 | 37.1549 | |
Mountain | 4 | 17.7231 | 17.7035 | 17.7213 | 17.5421 | 17.7372 | 17.4362 | 17.7390 | 17.7213 |
6 | 23.1283 | 23.0802 | 23.0902 | 22.5402 | 23.1001 | 22.4117 | 23.1173 | 23.1119 | |
8 | 27.9637 | 27.6405 | 27.9315 | 26.6905 | 27.9354 | 26.4147 | 27.9302 | 27.9679 | |
10 | 32.1404 | 31.9221 | 32.3451 | 30.9813 | 32.2561 | 30.0080 | 32.3415 | 32.4426 | |
12 | 36.3399 | 34.9350 | 36.3823 | 34.0005 | 35.6920 | 33.6967 | 36.1384 | 36.4707 | |
Tree | 4 | 18.9965 | 18.9962 | 18.9965 | 18.8465 | 18.9961 | 18.8254 | 18.9953 | 18.9965 |
6 | 24.3933 | 24.4095 | 24.4100 | 23.8731 | 24.3889 | 23.4245 | 24.4083 | 24.4149 | |
8 | 29.2846 | 28.9004 | 29.2918 | 27.8265 | 29.2753 | 27.6207 | 29.2581 | 29.2936 | |
10 | 33.5397 | 33.0809 | 33.6613 | 31.4879 | 33.6439 | 31.7828 | 33.5491 | 33.6785 | |
12 | 37.3776 | 36.7041 | 37.4570 | 35.2858 | 37.5764 | 34.8717 | 37.5111 | 37.6312 | |
Horse | 4 | 18.6614 | 18.6259 | 18.6230 | 18.3914 | 18.6252 | 18.5251 | 18.6614 | 18.6619 |
6 | 24.0609 | 23.8743 | 24.0802 | 23.2431 | 24.0604 | 22.8699 | 24.0675 | 24.0806 | |
8 | 28.8403 | 28.5613 | 28.8907 | 27.9542 | 28.8416 | 27.6730 | 28.8914 | 28.8992 | |
10 | 33.2142 | 32.3696 | 33.2125 | 31.1950 | 33.2741 | 30.7256 | 33.2480 | 33.3135 | |
12 | 36.8467 | 35.6548 | 37.0718 | 34.9857 | 37.0695 | 33.8890 | 37.1782 | 37.3216 | |
Bridge | 4 | 18.1906 | 18.1436 | 18.1902 | 18.0033 | 18.1903 | 18.0580 | 18.1903 | 18.1907 |
6 | 23.5631 | 23.5510 | 23.5679 | 22.8880 | 23.5610 | 22.8246 | 23.5649 | 23.5682 | |
8 | 28.2604 | 27.9986 | 28.2930 | 27.0830 | 28.2859 | 26.7516 | 28.2786 | 28.3023 | |
10 | 32.5876 | 32.3366 | 32.5480 | 30.9047 | 32.5740 | 30.5593 | 32.5281 | 32.6189 | |
12 | 36.0703 | 35.4806 | 36.4991 | 33.5753 | 36.4588 | 33.0662 | 36.4400 | 36.5351 | |
Pilot | 4 | 17.9368 | 17.9352 | 17.9362 | 17.6027 | 17.9362 | 17.7058 | 17.9355 | 17.9168 |
6 | 23.0182 | 22.9648 | 23.0443 | 21.9322 | 23.0365 | 21.8927 | 23.0025 | 23.0447 | |
8 | 27.6539 | 27.4187 | 27.6678 | 26.2966 | 27.6452 | 25.7373 | 27.6307 | 27.6723 | |
10 | 31.6001 | 30.3305 | 31.7457 | 30.5445 | 31.7701 | 29.3977 | 31.7568 | 31.8639 | |
12 | 35.3121 | 34.3457 | 35.4310 | 32.7740 | 35.6276 | 31.7852 | 35.5945 | 35.7388 | |
Dog | 4 | 18.5258 | 18.5246 | 18.4850 | 18.2690 | 18.5254 | 18.2825 | 18.5244 | 18.5261 |
6 | 23.6922 | 23.6586 | 23.7386 | 22.7559 | 23.7121 | 22.7675 | 23.7319 | 23.7420 | |
8 | 28.3767 | 28.0194 | 28.3553 | 27.0852 | 28.3862 | 26.7027 | 28.3751 | 28.4079 | |
10 | 32.4951 | 31.5916 | 32.6709 | 31.2171 | 32.6231 | 29.8432 | 32.6604 | 32.6944 | |
12 | 36.4563 | 36.2405 | 36.3900 | 34.1968 | 35.8826 | 33.0948 | 36.5074 | 36.6079 |
Image | K | SSA | MVO | DA | FPA | PSO | SCA | ALO | MALO |
Cactus | 4 | 0.4100 | 0.8700 | 1.8700 | 0.6900 | 0.7700 | 0.7100 | 1.4050 | 1.7200 |
6 | 0.2500 | 0.7000 | 1.4900 | 0.6800 | 0.6700 | 0.6500 | 1.8700 | 2.1455 | |
8 | 0.2810 | 0.7800 | 1.5200 | 0.6800 | 0.7200 | 0.6800 | 2.3850 | 2.6000 | |
10 | 0.3200 | 0.7800 | 1.5600 | 0.7300 | 0.7600 | 0.7100 | 2.9545 | 3.2550 | |
12 | 0.3400 | 1.0600 | 1.6890 | 0.8630 | 0.9000 | 0.8400 | 4.8515 | 4.3270 | |
Kangaroo | 4 | 0.5050 | 0.8100 | 1.8420 | 0.6700 | 0.7300 | 0.9580 | 1.4200 | 1.5505 |
6 | 0.2690 | 0.7300 | 1.5200 | 0.6800 | 0.7600 | 0.6500 | 1.9600 | 2.1995 | |
8 | 0.3100 | 0.8300 | 1.6410 | 0.7010 | 0.7500 | 0.7400 | 2.4345 | 2.7155 | |
10 | 0.3400 | 0.8500 | 1.6000 | 0.7800 | 0.8200 | 0.7400 | 3.0750 | 3.3250 | |
12 | 0.3700 | 0.8400 | 1.6000 | 0.7800 | 0.8300 | 0.7900 | 3.7580 | 3.8305 | |
Temple | 4 | 0.3700 | 0.8540 | 1.9250 | 0.6530 | 1.3210 | 0.6510 | 1.4300 | 1.5605 |
6 | 0.2700 | 0.7600 | 1.5690 | 0.6500 | 0.7390 | 0.6510 | 1.9200 | 2.1755 | |
8 | 0.3000 | 0.8100 | 1.5800 | 0.7400 | 0.7600 | 0.6890 | 3.0805 | 3.5605 | |
10 | 0.4400 | 1.3800 | 2.3500 | 1.0690 | 0.7600 | 0.8100 | 3.7715 | 4.9285 | |
12 | 0.3600 | 0.8600 | 1.7200 | 0.7710 | 0.8000 | 0.7300 | 3.5765 | 4.2245 | |
Flower | 4 | 0.4250 | 1.1510 | 2.8120 | 0.8400 | 0.8710 | 0.8550 | 2.1855 | 2.2690 |
6 | 0.2700 | 0.7900 | 1.6000 | 0.7200 | 0.7700 | 0.7400 | 2.0005 | 3.2575 | |
8 | 0.3860 | 0.9860 | 2.5490 | 1.5090 | 0.8630 | 0.7330 | 4.1070 | 4.4885 | |
10 | 0.8540 | 1.6520 | 2.4080 | 0.9010 | 1.3190 | 1.5640 | 4.8340 | 4.6935 | |
12 | 0.5600 | 1.1700 | 2.1900 | 1.1300 | 0.8700 | 1.0000 | 3.7055 | 4.0505 | |
Mountain | 4 | 0.4700 | 1.1750 | 2.8030 | 0.7110 | 0.9170 | 0.8500 | 2.2840 | 2.2155 |
6 | 0.5170 | 0.9080 | 2.4520 | 0.7370 | 0.8370 | 0.7510 | 2.5625 | 2.1245 | |
8 | 0.2920 | 0.8200 | 1.7150 | 0.6800 | 0.9710 | 0.8810 | 3.3625 | 2.8755 | |
10 | 0.4300 | 0.8200 | 3.4100 | 0.7100 | 0.8010 | 0.8800 | 5.0305 | 4.2160 | |
12 | 0.4000 | 0.8820 | 1.5710 | 0.7900 | 1.1500 | 0.8550 | 4.4010 | 3.9205 | |
Tree | 4 | 0.4500 | 0.9200 | 1.9700 | 0.9800 | 1.5640 | 0.7900 | 1.6595 | 2.3955 |
6 | 0.4300 | 1.0950 | 2.6590 | 1.7460 | 1.0490 | 1.6700 | 3.1700 | 3.5230 | |
8 | 0.3410 | 1.4480 | 2.8000 | 0.9750 | 0.7530 | 0.6930 | 2.6675 | 3.7390 | |
10 | 0.3740 | 1.2760 | 2.3920 | 0.8310 | 0.8920 | 0.8130 | 3.6270 | 3.5155 | |
12 | 0.4500 | 0.9390 | 0.9390 | 0.9390 | 0.8990 | 0.8510 | 3.9570 | 5.2985 | |
Horse | 4 | 0.4040 | 0.8970 | 1.8600 | 0.6840 | 0.7480 | 0.9190 | 1.7475 | 1.8105 |
6 | 0.3140 | 0.8380 | 1.6460 | 0.8150 | 0.7450 | 0.6850 | 2.0365 | 2.2195 | |
8 | 0.3010 | 1.0490 | 1.8420 | 0.7300 | 0.8770 | 0.7740 | 3.2820 | 2.9730 | |
10 | 0.3300 | 0.8560 | 1.6410 | 0.7780 | 0.8540 | 0.7920 | 3.7315 | 3.8360 | |
12 | 0.3920 | 0.9120 | 1.7110 | 1.6600 | 1.6600 | 1.0670 | 4.2670 | 4.8615 | |
Bridge | 4 | 0.4200 | 1.9550 | 2.5860 | 1.7110 | 0.9140 | 0.7110 | 1.6845 | 1.8555 |
6 | 0.2660 | 0.8010 | 1.5530 | 0.7070 | 1.3360 | 1.0980 | 2.8115 | 3.0780 | |
8 | 0.3210 | 0.9610 | 2.7830 | 1.0120 | 0.9970 | 0.7930 | 4.5400 | 4.8560 | |
10 | 0.4110 | 1.5550 | 2.6160 | 1.0420 | 1.6320 | 0.9770 | 5.2685 | 5.8515 | |
12 | 0.5330 | 1.2210 | 2.9890 | 1.1920 | 1.9340 | 1.1170 | 4.6090 | 4.1150 | |
Pilot | 4 | 0.4400 | 0.9200 | 2.3630 | 0.7820 | 1.0780 | 0.8350 | 1.8890 | 1.8405 |
6 | 0.2810 | 1.6590 | 2.2270 | 0.7510 | 1.3550 | 0.8610 | 3.4105 | 2.8835 | |
8 | 0.4400 | 1.5610 | 2.2130 | 0.8350 | 1.0880 | 1.2600 | 3.6285 | 3.9075 | |
10 | 0.3700 | 1.0850 | 2.7580 | 1.2330 | 1.2590 | 1.3200 | 4.9585 | 5.6945 | |
12 | 0.3740 | 0.9200 | 1.7840 | 1.2990 | 1.1100 | 1.5060 | 5.2780 | 4.0805 | |
Dog | 4 | 0.4500 | 1.0160 | 2.4440 | 0.8390 | 0.8300 | 1.3690 | 1.7170 | 2.5915 |
6 | 0.2750 | 1.3830 | 2.4210 | 1.2500 | 0.8100 | 1.3300 | 3.1915 | 3.4480 | |
8 | 0.4700 | 0.9450 | 1.5800 | 0.7400 | 0.7400 | 0.7510 | 2.4325 | 2.8155 | |
10 | 0.3490 | 0.8300 | 1.5390 | 0.7190 | 0.8010 | 0.7220 | 3.0855 | 3.5100 | |
12 | 0.3300 | 0.8720 | 1.5700 | 0.7600 | 0.8700 | 0.7800 | 3.7950 | 4.3600 |
Image | K | SSA | MVO | DA | FPA | PSO | SCA | ALO | MALO |
Cactus | 4 | 0.6400 | 1.2900 | 1.9500 | 0.8000 | 1.6930 | 0.7800 | 1.5150 | 1.8455 |
6 | 0.4700 | 0.8500 | 1.5890 | 0.8210 | 0.8910 | 0.7900 | 2.0750 | 2.3905 | |
8 | 0.4900 | 0.9500 | 1.6810 | 0.8110 | 0.8900 | 0.8080 | 2.4650 | 4.2290 | |
10 | 0.6230 | 1.2750 | 2.0900 | 1.0400 | 0.9900 | 0.8490 | 3.1220 | 3.6495 | |
12 | 0.5380 | 1.0030 | 1.7180 | 0.9570 | 1.0110 | 0.8900 | 3.6600 | 4.1195 | |
Kangaroo | 4 | 0.5800 | 0.9600 | 1.8620 | 0.8300 | 0.8900 | 1.4690 | 2.2225 | 2.3925 |
6 | 0.9260 | 1.2200 | 2.6770 | 1.4180 | 0.9600 | 1.0310 | 3.2235 | 2.9170 | |
8 | 0.4800 | 0.8900 | 1.6200 | 0.8200 | 0.9000 | 1.1690 | 2.5200 | 3.1900 | |
10 | 0.5210 | 0.9200 | 1.6400 | 0.8900 | 0.8700 | 0.8400 | 3.0550 | 3.6250 | |
12 | 0.5200 | 0.9900 | 1.7500 | 0.8800 | 0.9400 | 0.8900 | 3.6400 | 4.1070 | |
Temple | 4 | 0.6100 | 1.6350 | 2.5860 | 1.2700 | 1.2480 | 0.9400 | 2.4355 | 2.8155 |
6 | 0.4700 | 1.0400 | 2.3770 | 1.0500 | 1.2900 | 0.7900 | 2.0400 | 2.4100 | |
8 | 0.4700 | 0.9100 | 1.6800 | 0.8000 | 0.8900 | 0.8000 | 2.4900 | 2.9895 | |
10 | 0.5200 | 0.9300 | 1.6500 | 0.8600 | 1.0000 | 0.8400 | 3.1050 | 3.6095 | |
12 | 0.5700 | 1.0000 | 1.8200 | 0.9300 | 0.9100 | 0.8700 | 5.4985 | 4.5950 | |
Flower | 4 | 0.6000 | 1.4130 | 2.6960 | 1.0000 | 1.2850 | 1.3000 | 2.3625 | 2.8995 |
6 | 1.0690 | 0.9900 | 2.8420 | 0.9500 | 0.9390 | 0.9860 | 2.6195 | 2.7010 | |
8 | 0.4800 | 0.9900 | 1.7200 | 0.9400 | 0.9200 | 0.8700 | 2.6020 | 3.0850 | |
10 | 0.5300 | 0.9700 | 1.8010 | 0.9100 | 0.9990 | 0.9600 | 3.2255 | 3.6850 | |
12 | 0.6100 | 1.0700 | 1.8100 | 0.9400 | 1.0000 | 1.3790 | 5.1945 | 4.5065 | |
Mountain | 4 | 0.5600 | 1.5490 | 1.9920 | 0.8570 | 0.8810 | 0.8310 | 1.5315 | 1.9575 |
6 | 0.4410 | 0.9230 | 1.7220 | 0.9020 | 0.8820 | 0.8800 | 2.0330 | 2.4990 | |
8 | 0.5100 | 0.9710 | 1.7300 | 0.9000 | 0.9520 | 0.8420 | 2.5850 | 3.1700 | |
10 | 0.5480 | 1.0300 | 1.7900 | 0.9040 | 0.9510 | 0.9000 | 5.1080 | 4.0555 | |
12 | 1.0660 | 1.0420 | 1.9110 | 0.9610 | 1.0690 | 0.9580 | 3.9095 | 4.3645 | |
Tree | 4 | 0.6200 | 1.3020 | 2.8450 | 1.0270 | 1.2090 | 1.3510 | 2.2510 | 2.7790 |
6 | 0.5000 | 1.2040 | 2.6420 | 1.3380 | 1.1410 | 0.8990 | 2.1775 | 3.1785 | |
8 | 0.5110 | 0.8790 | 1.6500 | 0.8300 | 0.8800 | 0.8600 | 2.4650 | 2.9350 | |
10 | 0.5000 | 0.9300 | 1.6800 | 0.9190 | 0.8500 | 0.9000 | 3.0445 | 3.5950 | |
12 | 0.6700 | 1.0090 | 1.8600 | 0.9100 | 0.9400 | 0.9400 | 3.7930 | 4.1300 | |
Horse | 4 | 0.6100 | 1.5700 | 3.2080 | 1.5370 | 1.6130 | 0.8400 | 2.5665 | 2.8115 |
6 | 1.0800 | 1.2290 | 2.5900 | 0.8000 | 0.8100 | 0.9800 | 2.0500 | 2.4145 | |
8 | 0.4600 | 0.8900 | 1.6000 | 0.8300 | 0.8600 | 0.8900 | 2.6550 | 3.2905 | |
10 | 0.5000 | 0.9900 | 1.6400 | 1.1500 | 0.8900 | 0.8500 | 3.0550 | 3.6400 | |
12 | 0.5400 | 1.9330 | 2.0100 | 0.9800 | 1.0100 | 1.0000 | 3.7850 | 4.4105 | |
Bridge | 4 | 0.6000 | 0.9500 | 1.7600 | 0.7600 | 0.8100 | 0.7300 | 1.4750 | 1.7950 |
6 | 0.4100 | 0.8400 | 1.5700 | 0.8200 | 0.8400 | 0.8000 | 1.9300 | 2.3950 | |
8 | 0.4400 | 0.8600 | 1.5900 | 0.8700 | 0.8600 | 0.7900 | 2.4900 | 2.9775 | |
10 | 0.4700 | 0.9100 | 1.6400 | 0.7900 | 0.9100 | 1.2500 | 3.7975 | 3.9840 | |
12 | 0.5230 | 1.0150 | 2.1800 | 0.8710 | 0.9340 | 0.9500 | 3.7080 | 4.3340 | |
Pilot | 4 | 0.5400 | 0.9400 | 1.7300 | 0.7500 | 1.0100 | 0.7700 | 1.4750 | 1.7600 |
6 | 0.3900 | 0.8100 | 1.5500 | 0.8300 | 0.8700 | 0.8800 | 2.0940 | 2.5195 | |
8 | 0.4600 | 0.8400 | 1.8120 | 0.7760 | 0.9500 | 0.8600 | 2.6975 | 3.1400 | |
10 | 0.5650 | 1.1600 | 2.2950 | 0.9460 | 0.9790 | 0.9690 | 3.5840 | 4.1245 | |
12 | 0.5800 | 1.3060 | 2.3550 | 1.2900 | 1.0490 | 1.3350 | 5.6445 | 8.5025 | |
Dog | 4 | 0.6600 | 1.2350 | 3.0700 | 1.0100 | 1.0550 | 1.6800 | 2.5700 | 3.0600 |
6 | 0.5050 | 1.0870 | 2.1600 | 0.9200 | 0.9200 | 0.8500 | 2.2025 | 2.8425 | |
8 | 0.5050 | 1.2550 | 2.9380 | 1.0800 | 1.8400 | 1.4900 | 4.5565 | 5.3650 | |
10 | 0.5920 | 1.4200 | 2.2400 | 0.9200 | 1.2000 | 0.9250 | 3.4000 | 3.9405 | |
12 | 0.5790 | 1.0750 | 1.8410 | 0.9500 | 0.9990 | 0.9140 | 3.8400 | 4.5330 |
Provenance | Symbols | Paraphrase |
Color images | L | The gray value |
ni | The number of pixels with gray value of i | |
N | The total number of pixels | |
p | The distribution probability of gray value | |
K | The total number of threshold | |
t | The threshold | |
C | The class | |
R, G, B | The three channels of color images | |
Otsu | ω | The probabilities of class occurrence |
μ | The levels of class | |
σ2 | The total variance | |
Kapur's entropy | ψ | The total entropy |
ALO | X | The array of random walk |
ub | The upper bound of parameter | |
lb | The lower bound of parameter | |
t | The current iteration | |
T | The maximum of iterations | |
I | The ratio of boundary contraction | |
Time complexity | O | The complexity notation |
PSNR | MSE | The mean square error |
SSIM | R | The original image |
I | The segmented image | |
μR,μI | The average gray values of image | |
σ2R,σ2I | The variance of image | |
σRI | The covariance of image | |
FSIM | PC | Phase congruency |
GM | Gradient magnitude | |
Ω | The entire domain of image | |
SL(x) | The similarity value of each position x | |
PCm(x) | The phase consistency measure | |
SPC(x) | The similarity measure of phase consistency | |
SG(x) | The similarity measure of gradient magnitude |
IMAGE | K | SSA | MVO | DA | FPA | PSO | SCA | ALO | MALO |
Cactus | 4 | 19.1730 | 19.1730 | 19.1730 | 18.7263 | 19.1730 | 18.8974 | 19.1344 | 19.1901 |
6 | 21.4834 | 21.4806 | 21.4819 | 20.6216 | 21.0808 | 20.2685 | 21.4733 | 21.4849 | |
8 | 22.9129 | 23.0452 | 22.5947 | 21.8482 | 22.5738 | 23.0573 | 22.9754 | 23.7403 | |
10 | 23.8232 | 24.1443 | 23.9252 | 23.1394 | 23.9100 | 23.8865 | 24.0865 | 24.1499 | |
12 | 24.6895 | 25.1475 | 24.8778 | 24.9636 | 24.5394 | 25.1646 | 25.0007 | 25.2868 | |
Kangaroo | 4 | 22.0643 | 22.0643 | 22.0643 | 20.7955 | 22.0643 | 20.4905 | 22.0429 | 22.0797 |
6 | 24.8251 | 24.8350 | 24.5043 | 23.2999 | 24.4744 | 23.1015 | 24.8199 | 24.8836 | |
8 | 26.8199 | 27.0457 | 26.8403 | 24.3867 | 26.3434 | 24.2914 | 26.9047 | 27.0860 | |
10 | 28.1619 | 28.8131 | 28.5707 | 25.1187 | 28.1677 | 25.2235 | 28.4114 | 28.8186 | |
12 | 29.1767 | 30.0207 | 29.8385 | 27.1879 | 29.8151 | 26.9052 | 30.1672 | 30.3411 | |
Temple | 4 | 17.4713 | 17.4707 | 17.4713 | 19.7885 | 17.4736 | 17.1516 | 17.4005 | 17.6125 |
6 | 20.7143 | 19.8002 | 20.7137 | 20.7876 | 19.7566 | 18.2250 | 20.7661 | 21.4589 | |
8 | 22.0492 | 23.5836 | 22.5695 | 20.7664 | 22.5250 | 23.8550 | 23.4862 | 23.9977 | |
10 | 23.2619 | 25.2421 | 24.2747 | 23.4468 | 23.3367 | 22.7675 | 24.8846 | 25.7059 | |
12 | 26.8631 | 26.8151 | 25.5113 | 24.5639 | 24.7817 | 26.2330 | 26.4459 | 27.5232 | |
Flower | 4 | 22.6832 | 22.6832 | 22.6832 | 21.9099 | 22.6832 | 22.2661 | 22.6832 | 22.6916 |
6 | 25.1802 | 25.5484 | 25.5446 | 23.9499 | 25.2590 | 24.5962 | 25.5240 | 25.5506 | |
8 | 27.5586 | 27.3846 | 27.6921 | 25.3172 | 27.3576 | 26.1643 | 27.5337 | 27.6960 | |
10 | 28.6654 | 28.8544 | 29.0249 | 26.6791 | 28.8757 | 26.7323 | 29.1768 | 29.2169 | |
12 | 29.9882 | 30.1775 | 29.8010 | 28.1115 | 29.6455 | 27.8780 | 30.6120 | 30.7280 | |
Mountain | 4 | 19.7307 | 19.7307 | 19.7307 | 19.1368 | 19.7307 | 19.2754 | 19.7277 | 19.7307 |
6 | 21.8240 | 22.4882 | 22.3866 | 20.9344 | 22.1794 | 21.8654 | 22.3347 | 22.5039 | |
8 | 24.8724 | 24.1574 | 24.2907 | 22.8823 | 24.0400 | 23.4094 | 24.7870 | 24.9053 | |
10 | 25.9744 | 26.1368 | 26.1295 | 24.6068 | 25.8506 | 23.8109 | 26.0493 | 26.3373 | |
12 | 27.3911 | 26.9209 | 26.9014 | 25.3515 | 26.9991 | 25.7124 | 26.8751 | 27.4991 | |
Tree | 4 | 19.9284 | 19.9265 | 19.9284 | 19.3506 | 19.9206 | 19.0453 | 19.9284 | 19.9364 |
6 | 22.9219 | 22.9184 | 22.9126 | 21.8322 | 22.4339 | 22.4819 | 22.9276 | 22.9388 | |
8 | 25.1296 | 25.0004 | 25.0881 | 22.6562 | 24.4342 | 22.7555 | 25.1489 | 25.1704 | |
10 | 26.7206 | 26.6709 | 26.6389 | 24.1790 | 26.1891 | 24.3475 | 26.5608 | 26.7213 | |
12 | 27.5331 | 28.0382 | 27.7147 | 26.1871 | 27.2252 | 24.9152 | 27.8812 | 28.0724 | |
Horse | 4 | 19.1095 | 19.1095 | 19.1543 | 18.4918 | 19.1095 | 17.8797 | 19.1095 | 19.1569 |
6 | 22.6175 | 22.6343 | 22.6083 | 22.4411 | 22.6441 | 20.0880 | 22.5318 | 22.8301 | |
8 | 24.8257 | 24.6203 | 24.7383 | 23.2636 | 23.7326 | 22.2812 | 24.5913 | 24.8543 | |
10 | 26.7272 | 26.7701 | 26.3299 | 23.8301 | 26.2501 | 23.7805 | 26.4699 | 26.8845 | |
12 | 28.0067 | 27.6999 | 28.1864 | 25.0419 | 28.0623 | 24.4077 | 27.5665 | 28.3129 | |
Bridge | 4 | 18.7684 | 18.7684 | 18.7684 | 18.1883 | 18.7684 | 18.3216 | 18.7562 | 18.7684 |
6 | 21.0341 | 21.5192 | 21.5142 | 20.6458 | 21.5142 | 19.4138 | 21.0529 | 21.5346 | |
8 | 23.5582 | 22.9642 | 23.4355 | 21.6761 | 23.3575 | 20.6855 | 23.3521 | 23.7010 | |
10 | 25.0709 | 24.8332 | 25.2117 | 22.7421 | 24.8889 | 23.3572 | 25.3971 | 25.4265 | |
12 | 26.3616 | 26.8858 | 26.3203 | 25.5030 | 26.4849 | 25.2994 | 26.1879 | 27.0756 | |
Pilot | 4 | 16.9918 | 16.9918 | 16.9918 | 16.9571 | 16.9915 | 16.9918 | 17.0028 | 17.6971 |
6 | 19.4806 | 19.6603 | 19.3935 | 18.8999 | 19.4660 | 19.4742 | 19.4398 | 21.5230 | |
8 | 22.1137 | 21.7970 | 21.8114 | 19.8872 | 20.9936 | 20.1921 | 21.9259 | 22.4562 | |
10 | 23.3937 | 23.4926 | 22.5532 | 23.4677 | 22.5761 | 22.8645 | 23.6086 | 24.1912 | |
12 | 24.6032 | 24.8457 | 25.4417 | 23.3403 | 23.4735 | 24.3050 | 25.6425 | 25.8213 | |
Dog | 4 | 19.9346 | 19.9569 | 19.9346 | 20.2544 | 19.9346 | 18.6387 | 19.9734 | 19.9346 |
6 | 22.5690 | 22.6725 | 22.3348 | 21.6363 | 21.7981 | 20.9528 | 22.5891 | 22.6726 | |
8 | 24.3105 | 24.3265 | 24.3176 | 23.0185 | 22.9836 | 22.5716 | 24.3533 | 24.3548 | |
10 | 24.9193 | 26.0109 | 25.7454 | 24.4410 | 25.5648 | 23.1901 | 25.8904 | 26.4961 | |
12 | 26.7167 | 28.0094 | 26.3712 | 24.9053 | 26.4391 | 25.6498 | 27.6947 | 28.1328 |
IMAGE | K | SSA | MVO | DA | FPA | PSO | SCA | ALO | MALO |
Cactus | 4 | 17.1463 | 17.1849 | 17.1849 | 16.6326 | 17.1605 | 16.5963 | 17.1918 | 17.2250 |
6 | 18.7054 | 19.5152 | 18.8374 | 17.7723 | 18.9734 | 18.9692 | 18.9353 | 20.9242 | |
8 | 20.2196 | 21.8061 | 21.8129 | 20.2720 | 20.7186 | 20.1477 | 20.8937 | 23.1098 | |
10 | 21.6755 | 23.5236 | 23.5035 | 22.9740 | 23.3426 | 23.5817 | 23.6783 | 25.6436 | |
12 | 24.5328 | 25.7488 | 24.2717 | 25.8229 | 25.6387 | 22.2231 | 25.5971 | 26.7329 | |
Kangaroo | 4 | 18.7559 | 18.7559 | 18.7529 | 16.9273 | 18.7030 | 17.9415 | 18.7714 | 18.8067 |
6 | 21.2093 | 21.9397 | 21.7227 | 20.3904 | 21.8062 | 20.1191 | 22.1098 | 22.3708 | |
8 | 23.8181 | 24.5562 | 23.9062 | 20.5802 | 24.5744 | 22.1074 | 24.4808 | 24.6322 | |
10 | 25.1196 | 26.0125 | 25.7934 | 25.0967 | 25.7309 | 22.8701 | 25.9331 | 26.1974 | |
12 | 26.6277 | 27.4952 | 27.4380 | 24.5722 | 27.3277 | 24.0530 | 27.2209 | 28.0349 | |
Temple | 4 | 16.7418 | 18.6069 | 17.9079 | 17.7575 | 17.8108 | 16.4613 | 18.5579 | 18.6105 |
6 | 20.3140 | 20.4895 | 20.3140 | 20.7483 | 20.5577 | 21.6953 | 20.6648 | 23.1506 | |
8 | 24.9708 | 24.0553 | 23.2965 | 22.2220 | 24.5715 | 23.2979 | 25.0443 | 25.1273 | |
10 | 25.8405 | 25.8930 | 26.0409 | 23.4840 | 26.5620 | 21.8980 | 26.5010 | 26.7757 | |
12 | 27.0259 | 26.4958 | 27.5263 | 24.8266 | 27.3391 | 24.0635 | 27.5766 | 28.4027 | |
Flower | 4 | 21.4262 | 21.4353 | 21.4262 | 21.0771 | 21.4030 | 21.7291 | 21.3824 | 21.4046 |
6 | 23.9954 | 24.0070 | 24.0260 | 22.9845 | 23.9935 | 23.7137 | 24.0223 | 24.2923 | |
8 | 25.0019 | 25.3825 | 25.3936 | 24.4758 | 25.6350 | 25.5636 | 25.5517 | 26.3947 | |
10 | 26.1749 | 27.0175 | 26.8094 | 25.4329 | 26.9897 | 26.1739 | 26.8538 | 27.6016 | |
12 | 26.8429 | 28.2241 | 27.8388 | 25.9058 | 27.9865 | 27.9984 | 27.8198 | 29.2750 | |
Mountain | 4 | 15.7308 | 16.1695 | 16.1695 | 15.1291 | 14.6360 | 17.2397 | 14.6863 | 17.7501 |
6 | 19.1293 | 19.7275 | 19.3198 | 20.0548 | 19.8595 | 18.9344 | 19.2375 | 20.2658 | |
8 | 22.2621 | 22.4482 | 22.2780 | 20.3443 | 22.2996 | 20.2389 | 22.1739 | 23.7160 | |
10 | 20.8677 | 25.1887 | 23.4748 | 22.1128 | 24.6158 | 22.2042 | 24.5972 | 26.2667 | |
12 | 25.4993 | 26.3821 | 26.2452 | 24.7312 | 26.5979 | 22.1953 | 26.4684 | 26.6949 | |
Tree | 4 | 19.3878 | 19.4098 | 19.3878 | 19.4510 | 19.3660 | 19.0814 | 19.3485 | 19.3878 |
6 | 21.7283 | 21.9982 | 21.9410 | 20.7787 | 21.7496 | 20.5382 | 21.9919 | 22.0355 | |
8 | 23.5510 | 23.6198 | 23.4812 | 22.0054 | 23.5766 | 22.2770 | 23.5404 | 24.2661 | |
10 | 24.8234 | 25.5643 | 25.4307 | 23.3183 | 25.4128 | 22.1914 | 25.4025 | 25.7360 | |
12 | 26.1558 | 26.7973 | 26.3433 | 23.9995 | 26.5906 | 24.8922 | 26.5045 | 26.8461 | |
Horse | 4 | 18.9208 | 19.7557 | 19.0344 | 18.9208 | 19.6738 | 19.4317 | 19.5801 | 19.7788 |
6 | 21.2581 | 21.6962 | 21.6519 | 19.7265 | 21.3967 | 19.8505 | 21.6610 | 21.6974 | |
8 | 23.8699 | 23.6078 | 24.2314 | 22.0431 | 23.2680 | 21.4611 | 23.4581 | 24.2427 | |
10 | 25.5023 | 24.5116 | 25.5495 | 23.2916 | 25.8501 | 22.6324 | 26.0431 | 26.1268 | |
12 | 26.4346 | 25.3050 | 27.0205 | 24.1636 | 27.1689 | 24.0270 | 26.9024 | 27.4180 | |
Bridge | 4 | 17.7375 | 17.7101 | 17.7433 | 17.6562 | 17.7064 | 17.2277 | 17.7680 | 18.3564 |
6 | 21.0395 | 21.0300 | 21.0468 | 20.0621 | 21.0612 | 19.8616 | 21.0352 | 21.1004 | |
8 | 22.8402 | 23.4100 | 23.4820 | 21.0220 | 23.3430 | 21.3364 | 23.4706 | 23.6124 | |
10 | 24.6074 | 24.9201 | 24.1982 | 23.1209 | 24.7082 | 23.6599 | 24.6209 | 25.3739 | |
12 | 25.4895 | 26.0580 | 26.3086 | 23.1938 | 26.7399 | 24.1204 | 26.4986 | 27.4320 | |
Pilot | 4 | 15.9241 | 15.8597 | 15.9653 | 15.5965 | 15.8932 | 15.9792 | 15.9532 | 16.0238 |
6 | 19.9635 | 19.1615 | 20.4713 | 19.5345 | 20.6783 | 20.2829 | 19.8317 | 20.7123 | |
8 | 22.5976 | 22.0836 | 22.9972 | 22.6697 | 23.0674 | 22.7085 | 22.8982 | 23.1598 | |
10 | 24.5888 | 20.7618 | 24.7292 | 23.9268 | 24.7256 | 24.0374 | 24.5571 | 24.8228 | |
12 | 25.6096 | 24.6046 | 26.7532 | 24.9263 | 25.9880 | 25.6817 | 26.4241 | 27.1095 | |
Dog | 4 | 18.3708 | 18.4828 | 17.6137 | 16.0679 | 18.2476 | 18.2657 | 18.4844 | 18.2605 |
6 | 20.0477 | 20.7865 | 20.6360 | 19.9191 | 20.6773 | 18.4516 | 20.7274 | 21.9672 | |
8 | 22.3956 | 22.5129 | 22.5608 | 22.6757 | 22.5483 | 22.5758 | 22.3802 | 24.5205 | |
10 | 23.4554 | 25.3079 | 24.3555 | 24.4406 | 25.4561 | 21.6361 | 24.8465 | 26.3186 | |
12 | 25.8139 | 26.8848 | 26.0117 | 23.2895 | 26.5957 | 23.0829 | 26.4860 | 27.2748 |
IMAGE | K | SSA | MVO | DA | FPA | PSO | SCA | ALO | MALO |
Cactus | 4 | 0.6001 | 0.6001 | 0.6001 | 0.5854 | 0.6015 | 0.6001 | 0.5984 | 0.6063 |
6 | 0.6955 | 0.6961 | 0.6950 | 0.6716 | 0.6801 | 0.6568 | 0.6956 | 0.6963 | |
8 | 0.7438 | 0.7515 | 0.7268 | 0.7278 | 0.7361 | 0.7507 | 0.7487 | 0.7904 | |
10 | 0.7684 | 0.7838 | 0.7732 | 0.7495 | 0.7745 | 0.7814 | 0.7807 | 0.8391 | |
12 | 0.8012 | 0.8123 | 0.8157 | 0.8272 | 0.7927 | 0.8022 | 0.8050 | 0.8456 | |
Kangaroo | 4 | 0.7735 | 0.7735 | 0.7735 | 0.7156 | 0.7738 | 0.7046 | 0.7725 | 0.7735 |
6 | 0.8609 | 0.8615 | 0.8515 | 0.8179 | 0.8525 | 0.8033 | 0.8608 | 0.8627 | |
8 | 0.9018 | 0.9066 | 0.9029 | 0.8415 | 0.8958 | 0.8431 | 0.9041 | 0.9084 | |
10 | 0.9219 | 0.9325 | 0.9288 | 0.8581 | 0.9244 | 0.8658 | 0.9262 | 0.9331 | |
12 | 0.9352 | 0.9466 | 0.9426 | 0.9032 | 0.9449 | 0.9032 | 0.9474 | 0.9495 | |
Temple | 4 | 0.5829 | 0.5829 | 0.5829 | 0.5906 | 0.5825 | 0.5492 | 0.5793 | 0.6798 |
6 | 0.7234 | 0.6884 | 0.7242 | 0.7222 | 0.6836 | 0.6239 | 0.7249 | 0.7263 | |
8 | 0.7702 | 0.8149 | 0.7873 | 0.7265 | 0.7843 | 0.8102 | 0.8121 | 0.8152 | |
10 | 0.8069 | 0.8561 | 0.8329 | 0.7886 | 0.8084 | 0.7752 | 0.8480 | 0.8643 | |
12 | 0.8823 | 0.8883 | 0.8606 | 0.8254 | 0.8453 | 0.8546 | 0.8753 | 0.8917 | |
Flower | 4 | 0.4655 | 0.4655 | 0.4655 | 0.4194 | 0.4655 | 0.4655 | 0.4656 | 0.4916 |
6 | 0.5704 | 0.6806 | 0.6789 | 0.4849 | 0.6696 | 0.6800 | 0.6765 | 0.7406 | |
8 | 0.7883 | 0.7946 | 0.7974 | 0.6136 | 0.7867 | 0.7725 | 0.7911 | 0.8000 | |
10 | 0.8139 | 0.8286 | 0.8219 | 0.7771 | 0.8206 | 0.7406 | 0.8268 | 0.8294 | |
12 | 0.8364 | 0.8550 | 0.8310 | 0.8422 | 0.8372 | 0.8203 | 0.8644 | 0.8682 | |
Mountain | 4 | 0.6987 | 0.6987 | 0.6987 | 0.6715 | 0.6987 | 0.6987 | 0.6976 | 0.7081 |
6 | 0.7471 | 0.7758 | 0.7691 | 0.7237 | 0.7698 | 0.7761 | 0.7705 | 0.7932 | |
8 | 0.8293 | 0.8166 | 0.8127 | 0.7688 | 0.8101 | 0.8206 | 0.8311 | 0.8322 | |
10 | 0.8442 | 0.8536 | 0.8476 | 0.8254 | 0.8524 | 0.8261 | 0.8520 | 0.8603 | |
12 | 0.8682 | 0.8650 | 0.8626 | 0.8273 | 0.8731 | 0.8511 | 0.8617 | 0.8780 | |
Tree | 4 | 0.6406 | 0.6410 | 0.6406 | 0.6162 | 0.6419 | 0.6406 | 0.6412 | 0.6915 |
6 | 0.7535 | 0.7538 | 0.7535 | 0.6682 | 0.7420 | 0.7454 | 0.7538 | 0.7546 | |
8 | 0.7919 | 0.7890 | 0.7901 | 0.7176 | 0.7465 | 0.7515 | 0.7954 | 0.7960 | |
10 | 0.8229 | 0.8272 | 0.8263 | 0.7932 | 0.8190 | 0.8009 | 0.8258 | 0.8281 | |
12 | 0.8372 | 0.8505 | 0.8416 | 0.8307 | 0.8387 | 0.8142 | 0.8555 | 0.8579 | |
Horse | 4 | 0.7206 | 0.7206 | 0.7203 | 0.6791 | 0.7206 | 0.7155 | 0.7201 | 0.7206 |
6 | 0.7543 | 0.7624 | 0.7613 | 0.7617 | 0.7611 | 0.7700 | 0.7591 | 0.7744 | |
8 | 0.8102 | 0.8062 | 0.8103 | 0.7689 | 0.7945 | 0.7622 | 0.8114 | 0.8117 | |
10 | 0.8501 | 0.8510 | 0.8505 | 0.8026 | 0.8454 | 0.8162 | 0.8523 | 0.8534 | |
12 | 0.8709 | 0.8769 | 0.8739 | 0.8233 | 0.8757 | 0.8161 | 0.8686 | 0.8793 | |
Bridge | 4 | 0.7286 | 0.7286 | 0.7286 | 0.7286 | 0.7286 | 0.7293 | 0.7280 | 0.7398 |
6 | 0.8032 | 0.8180 | 0.8180 | 0.8022 | 0.8190 | 0.7728 | 0.8030 | 0.8193 | |
8 | 0.8626 | 0.8512 | 0.8566 | 0.8374 | 0.8604 | 0.8323 | 0.8620 | 0.8691 | |
10 | 0.8830 | 0.8909 | 0.8903 | 0.8447 | 0.8912 | 0.8886 | 0.8956 | 0.8993 | |
12 | 0.9021 | 0.9140 | 0.9031 | 0.8986 | 0.9041 | 0.9063 | 0.9087 | 0.9226 | |
Pilot | 4 | 0.7296 | 0.7296 | 0.7296 | 0.7222 | 0.7296 | 0.7296 | 0.7299 | 0.7399 |
6 | 0.7854 | 0.7857 | 0.7839 | 0.7540 | 0.7852 | 0.7849 | 0.7855 | 0.8224 | |
8 | 0.8420 | 0.8385 | 0.8383 | 0.7887 | 0.8218 | 0.7875 | 0.8390 | 0.8424 | |
10 | 0.8720 | 0.8651 | 0.8607 | 0.8555 | 0.8570 | 0.8401 | 0.8737 | 0.8788 | |
12 | 0.8905 | 0.8996 | 0.8951 | 0.8581 | 0.8726 | 0.8668 | 0.8947 | 0.9017 | |
Dog | 4 | 0.7003 | 0.7031 | 0.7003 | 0.7003 | 0.7003 | 0.6563 | 0.6999 | 0.7428 |
6 | 0.7700 | 0.7755 | 0.7624 | 0.7684 | 0.7514 | 0.7104 | 0.7694 | 0.7756 | |
8 | 0.8188 | 0.8190 | 0.8190 | 0.7735 | 0.7848 | 0.7632 | 0.8213 | 0.8240 | |
10 | 0.8314 | 0.8572 | 0.8510 | 0.8116 | 0.8470 | 0.7804 | 0.8544 | 0.8655 | |
12 | 0.8691 | 0.8928 | 0.8643 | 0.8246 | 0.8654 | 0.8566 | 0.8886 | 0.8948 |
IMAGE | K | SSA | MVO | DA | FPA | PSO | SCA | ALO | MALO |
Cactus | 4 | 0.4811 | 0.4831 | 0.4831 | 0.4466 | 0.4818 | 0.4497 | 0.4835 | 0.4858 |
6 | 0.5552 | 0.5975 | 0.5613 | 0.5064 | 0.5697 | 0.7353 | 0.5657 | 0.5690 | |
8 | 0.6254 | 0.7141 | 0.7143 | 0.6989 | 0.6473 | 0.6168 | 0.6574 | 0.7681 | |
10 | 0.7156 | 0.7719 | 0.7926 | 0.7684 | 0.7661 | 0.8080 | 0.7761 | 0.8677 | |
12 | 0.8238 | 0.8537 | 0.7953 | 0.8778 | 0.8524 | 0.7282 | 0.8510 | 0.8873 | |
Kangaroo | 4 | 0.6080 | 0.6080 | 0.6078 | 0.5032 | 0.6058 | 0.5645 | 0.6083 | 0.6116 |
6 | 0.7320 | 0.7635 | 0.7531 | 0.6689 | 0.7565 | 0.6881 | 0.7690 | 0.7782 | |
8 | 0.8263 | 0.8498 | 0.8304 | 0.7028 | 0.8493 | 0.7534 | 0.8476 | 0.8521 | |
10 | 0.8632 | 0.8867 | 0.8798 | 0.8570 | 0.8798 | 0.7602 | 0.8843 | 0.8913 | |
12 | 0.8976 | 0.9150 | 0.9128 | 0.8380 | 0.9111 | 0.8155 | 0.9096 | 0.9254 | |
Temple | 4 | 0.5367 | 0.6145 | 0.5713 | 0.5753 | 0.5657 | 0.5101 | 0.6117 | 0.6149 |
6 | 0.6933 | 0.6996 | 0.6933 | 0.7056 | 0.6976 | 0.7261 | 0.7014 | 0.7784 | |
8 | 0.8252 | 0.8034 | 0.7796 | 0.7400 | 0.8173 | 0.7918 | 0.8318 | 0.8465 | |
10 | 0.8469 | 0.8719 | 0.8522 | 0.7703 | 0.8665 | 0.7311 | 0.8648 | 0.8787 | |
12 | 0.8733 | 0.8941 | 0.8845 | 0.8153 | 0.8796 | 0.8086 | 0.8915 | 0.9021 | |
Flower | 4 | 0.3948 | 0.3985 | 0.3948 | 0.3969 | 0.3976 | 0.3976 | 0.3950 | 0.3994 |
6 | 0.4916 | 0.5129 | 0.4916 | 0.4830 | 0.4925 | 0.4923 | 0.4917 | 0.5247 | |
8 | 0.5171 | 0.5986 | 0.5385 | 0.5047 | 0.5538 | 0.5388 | 0.5387 | 0.6431 | |
10 | 0.5589 | 0.6425 | 0.5845 | 0.5280 | 0.5968 | 0.5964 | 0.5885 | 0.6606 | |
12 | 0.5737 | 0.6445 | 0.6168 | 0.5823 | 0.6299 | 0.7444 | 0.6410 | 0.7643 | |
Mountain | 4 | 0.4651 | 0.5563 | 0.5563 | 0.4348 | 0.4186 | 0.5804 | 0.4192 | 0.6240 |
6 | 0.6590 | 0.6916 | 0.6673 | 0.7108 | 0.6961 | 0.6653 | 0.6674 | 0.7131 | |
8 | 0.7628 | 0.7797 | 0.7716 | 0.6980 | 0.7612 | 0.7505 | 0.7695 | 0.8259 | |
10 | 0.7035 | 0.8433 | 0.7862 | 0.7690 | 0.8408 | 0.8062 | 0.8135 | 0.8792 | |
12 | 0.8537 | 0.8681 | 0.8645 | 0.8367 | 0.8718 | 0.7863 | 0.8668 | 0.9085 | |
Tree | 4 | 0.5888 | 0.5906 | 0.5888 | 0.6081 | 0.5874 | 0.5888 | 0.5866 | 0.6264 |
6 | 0.6716 | 0.6898 | 0.6728 | 0.6909 | 0.6708 | 0.6817 | 0.6770 | 0.7219 | |
8 | 0.7080 | 0.7157 | 0.7126 | 0.6873 | 0.7171 | 0.7503 | 0.7129 | 0.7737 | |
10 | 0.7271 | 0.7594 | 0.7631 | 0.7740 | 0.7656 | 0.6862 | 0.7653 | 0.8214 | |
12 | 0.7646 | 0.7871 | 0.7772 | 0.7040 | 0.7822 | 0.7977 | 0.8101 | 0.8792 | |
Horse | 4 | 0.6486 | 0.6486 | 0.6637 | 0.6911 | 0.6976 | 0.6675 | 0.6835 | 0.7070 |
6 | 0.7287 | 0.7486 | 0.7478 | 0.6954 | 0.7414 | 0.7463 | 0.7487 | 0.7802 | |
8 | 0.7789 | 0.7930 | 0.7922 | 0.7668 | 0.8010 | 0.7618 | 0.8035 | 0.8335 | |
10 | 0.8221 | 0.8388 | 0.8247 | 0.7824 | 0.8276 | 0.7849 | 0.8376 | 0.8697 | |
12 | 0.8410 | 0.8699 | 0.8567 | 0.7938 | 0.8691 | 0.8089 | 0.8598 | 0.8979 | |
Bridge | 4 | 0.6914 | 0.6927 | 0.6902 | 0.6790 | 0.6918 | 0.6897 | 0.6946 | 0.7176 |
6 | 0.7909 | 0.7974 | 0.7957 | 0.7433 | 0.7926 | 0.7813 | 0.7979 | 0.8053 | |
8 | 0.8336 | 0.8532 | 0.8522 | 0.7775 | 0.8505 | 0.8427 | 0.8564 | 0.8820 | |
10 | 0.8688 | 0.8812 | 0.8598 | 0.8144 | 0.8717 | 0.8636 | 0.8878 | 0.9160 | |
12 | 0.8836 | 0.9044 | 0.9271 | 0.8634 | 0.9124 | 0.8837 | 0.9116 | 0.9393 | |
Pilot | 4 | 0.7676 | 0.7675 | 0.7665 | 0.7440 | 0.7644 | 0.7686 | 0.7688 | 0.7512 |
6 | 0.8059 | 0.8241 | 0.8201 | 0.7920 | 0.8220 | 0.8259 | 0.7979 | 0.8317 | |
8 | 0.8584 | 0.8617 | 0.8621 | 0.8436 | 0.8620 | 0.8585 | 0.8619 | 0.8672 | |
10 | 0.8859 | 0.8857 | 0.8839 | 0.8781 | 0.8852 | 0.8787 | 0.8908 | 0.8937 | |
12 | 0.9031 | 0.9045 | 0.9100 | 0.8703 | 0.9036 | 0.8707 | 0.9009 | 0.9124 | |
Dog | 4 | 0.6534 | 0.6534 | 0.6218 | 0.5749 | 0.6510 | 0.6471 | 0.6582 | 0.6599 |
6 | 0.7021 | 0.7305 | 0.7252 | 0.7381 | 0.7333 | 0.6472 | 0.7290 | 0.7797 | |
8 | 0.7768 | 0.7801 | 0.7867 | 0.8020 | 0.7815 | 0.7875 | 0.7823 | 0.8486 | |
10 | 0.8039 | 0.8538 | 0.8278 | 0.8369 | 0.8574 | 0.7654 | 0.8338 | 0.9002 | |
12 | 0.8576 | 0.8860 | 0.8706 | 0.8378 | 0.8754 | 0.8326 | 0.8720 | 0.8889 |
IMAGE | K | SSA | MVO | DA | FPA | PSO | SCA | ALO | MALO |
Cactus | 4 | 0.8104 | 0.8104 | 0.8104 | 0.7856 | 0.8104 | 0.7991 | 0.8103 | 0.8104 |
6 | 0.8679 | 0.8673 | 0.8676 | 0.8305 | 0.8585 | 0.8280 | 0.8676 | 0.8681 | |
8 | 0.8967 | 0.8978 | 0.8936 | 0.8625 | 0.8912 | 0.8869 | 0.8974 | 0.8980 | |
10 | 0.9133 | 0.9153 | 0.9143 | 0.8868 | 0.9137 | 0.9029 | 0.9148 | 0.9153 | |
12 | 0.9250 | 0.9261 | 0.9264 | 0.9001 | 0.9218 | 0.9111 | 0.9272 | 0.9287 | |
Kangaroo | 4 | 0.8293 | 0.8293 | 0.8293 | 0.8005 | 0.8297 | 0.7913 | 0.8284 | 0.8293 |
6 | 0.8998 | 0.8999 | 0.8962 | 0.8585 | 0.8904 | 0.8642 | 0.9000 | 0.9001 | |
8 | 0.9325 | 0.9328 | 0.9325 | 0.8860 | 0.9246 | 0.8884 | 0.9330 | 0.9335 | |
10 | 0.9474 | 0.9521 | 0.9534 | 0.8914 | 0.9454 | 0.9055 | 0.9504 | 0.9541 | |
12 | 0.9607 | 0.9649 | 0.9638 | 0.9358 | 0.9594 | 0.9352 | 0.9630 | 0.9653 | |
Temple | 4 | 0.7391 | 0.7391 | 0.7391 | 0.7435 | 0.7388 | 0.7130 | 0.7374 | 0.7840 |
6 | 0.8178 | 0.7984 | 0.8181 | 0.8137 | 0.7968 | 0.7606 | 0.8189 | 0.8203 | |
8 | 0.8420 | 0.8693 | 0.8497 | 0.8270 | 0.8491 | 0.8671 | 0.8709 | 0.8722 | |
10 | 0.8628 | 0.8929 | 0.8805 | 0.8614 | 0.8615 | 0.8481 | 0.8888 | 0.8994 | |
12 | 0.9185 | 0.9135 | 0.8967 | 0.8820 | 0.8854 | 0.8994 | 0.9085 | 0.9301 | |
Flower | 4 | 0.7838 | 0.7838 | 0.7838 | 0.7604 | 0.7838 | 0.7726 | 0.7838 | 0.7841 |
6 | 0.8402 | 0.8413 | 0.8406 | 0.8177 | 0.8356 | 0.8142 | 0.8404 | 0.8418 | |
8 | 0.8829 | 0.8810 | 0.8864 | 0.8461 | 0.8790 | 0.8524 | 0.8828 | 0.8874 | |
10 | 0.9041 | 0.9100 | 0.9110 | 0.8639 | 0.9092 | 0.8791 | 0.9114 | 0.9120 | |
12 | 0.9246 | 0.9269 | 0.9191 | 0.8891 | 0.9195 | 0.8912 | 0.9333 | 0.9347 | |
Mountain | 4 | 0.8006 | 0.8006 | 0.8006 | 0.7658 | 0.8006 | 0.7918 | 0.7999 | 0.8006 |
6 | 0.8426 | 0.8561 | 0.8550 | 0.8225 | 0.8494 | 0.8335 | 0.8552 | 0.8563 | |
8 | 0.8893 | 0.8892 | 0.8866 | 0.8570 | 0.8824 | 0.8672 | 0.8901 | 0.8903 | |
10 | 0.9074 | 0.9094 | 0.9083 | 0.8789 | 0.9042 | 0.8793 | 0.9078 | 0.9142 | |
12 | 0.9217 | 0.9217 | 0.9214 | 0.8919 | 0.9211 | 0.8941 | 0.9182 | 0.9253 | |
Tree | 4 | 0.8055 | 0.8056 | 0.8055 | 0.7873 | 0.8060 | 0.8055 | 0.8058 | 0.8166 |
6 | 0.8562 | 0.8566 | 0.8562 | 0.8218 | 0.8479 | 0.8448 | 0.8562 | 0.8568 | |
8 | 0.8826 | 0.8825 | 0.8825 | 0.8408 | 0.8680 | 0.8423 | 0.8833 | 0.8840 | |
10 | 0.9038 | 0.9038 | 0.9041 | 0.8713 | 0.8981 | 0.8729 | 0.9026 | 0.9055 | |
12 | 0.9149 | 0.9203 | 0.9201 | 0.8984 | 0.9098 | 0.8789 | 0.9186 | 0.9208 | |
Horse | 4 | 0.8217 | 0.8217 | 0.8216 | 0.7960 | 0.8217 | 0.8099 | 0.8213 | 0.8217 |
6 | 0.8506 | 0.8504 | 0.8503 | 0.8472 | 0.8505 | 0.8388 | 0.8490 | 0.8506 | |
8 | 0.8849 | 0.8801 | 0.8848 | 0.8555 | 0.8687 | 0.8423 | 0.8827 | 0.8852 | |
10 | 0.9120 | 0.9082 | 0.9053 | 0.8773 | 0.9053 | 0.8708 | 0.9063 | 0.9122 | |
12 | 0.9269 | 0.9240 | 0.9279 | 0.8886 | 0.9254 | 0.8801 | 0.9202 | 0.9303 | |
Bridge | 4 | 0.8399 | 0.8399 | 0.8399 | 0.8276 | 0.8399 | 0.8276 | 0.8397 | 0.8399 |
6 | 0.8894 | 0.8971 | 0.8968 | 0.8706 | 0.8968 | 0.8613 | 0.8894 | 0.8974 | |
8 | 0.9235 | 0.9150 | 0.9223 | 0.8951 | 0.9203 | 0.8849 | 0.9202 | 0.9241 | |
10 | 0.9368 | 0.9350 | 0.9389 | 0.9098 | 0.9346 | 0.9192 | 0.9397 | 0.9398 | |
12 | 0.9478 | 0.9493 | 0.9470 | 0.9332 | 0.9469 | 0.9341 | 0.9453 | 0.9499 | |
Pilot | 4 | 0.7854 | 0.7854 | 0.7854 | 0.7765 | 0.7854 | 0.7736 | 0.7854 | 0.7855 |
6 | 0.8294 | 0.8260 | 0.8290 | 0.8070 | 0.8292 | 0.8290 | 0.8295 | 0.8345 | |
8 | 0.8623 | 0.8623 | 0.8619 | 0.8278 | 0.8531 | 0.8277 | 0.8617 | 0.8625 | |
10 | 0.8890 | 0.8819 | 0.8829 | 0.8710 | 0.8788 | 0.8547 | 0.8898 | 0.8918 | |
12 | 0.9033 | 0.9122 | 0.9079 | 0.8714 | 0.8905 | 0.8787 | 0.9101 | 0.9148 | |
Dog | 4 | 0.7911 | 0.7918 | 0.7911 | 0.7911 | 0.7911 | 0.7584 | 0.7911 | 0.7943 |
6 | 0.8497 | 0.8519 | 0.8467 | 0.8274 | 0.8347 | 0.8092 | 0.8494 | 0.8521 | |
8 | 0.8822 | 0.8793 | 0.8823 | 0.8436 | 0.8571 | 0.8452 | 0.8827 | 0.8834 | |
10 | 0.8932 | 0.9052 | 0.9049 | 0.8720 | 0.9002 | 0.8584 | 0.9050 | 0.9109 | |
12 | 0.9147 | 0.9277 | 0.9127 | 0.8882 | 0.9109 | 0.8965 | 0.9235 | 0.9288 |
IMAGE | K | SSA | MVO | DA | FPA | PSO | SCA | ALO | MALO |
Cactus | 4 | 0.7694 | 0.7700 | 0.7700 | 0.7508 | 0.7701 | 0.7453 | 0.7708 | 0.7711 |
6 | 0.8213 | 0.8263 | 0.8232 | 0.7858 | 0.8269 | 0.8315 | 0.8248 | 0.8363 | |
8 | 0.8598 | 0.8767 | 0.8766 | 0.8257 | 0.8672 | 0.8361 | 0.8674 | 0.8924 | |
10 | 0.8791 | 0.9048 | 0.8995 | 0.8791 | 0.9021 | 0.8787 | 0.9049 | 0.9227 | |
12 | 0.9164 | 0.9290 | 0.9154 | 0.9140 | 0.9278 | 0.8735 | 0.9260 | 0.9375 | |
Kangaroo | 4 | 0.7539 | 0.7539 | 0.7536 | 0.6738 | 0.7522 | 0.7172 | 0.7549 | 0.7574 |
6 | 0.8466 | 0.8609 | 0.8550 | 0.7787 | 0.8574 | 0.8118 | 0.8674 | 0.8713 | |
8 | 0.9112 | 0.9218 | 0.9113 | 0.8341 | 0.9214 | 0.8522 | 0.9196 | 0.9231 | |
10 | 0.9366 | 0.9456 | 0.9430 | 0.9110 | 0.9427 | 0.8605 | 0.9468 | 0.9482 | |
12 | 0.9543 | 0.9617 | 0.9609 | 0.9105 | 0.9617 | 0.8950 | 0.9596 | 0.9632 | |
Temple | 4 | 0.7190 | 0.7590 | 0.7310 | 0.7199 | 0.7282 | 0.6860 | 0.7570 | 0.7591 |
6 | 0.8107 | 0.8139 | 0.8107 | 0.7906 | 0.8129 | 0.7971 | 0.8111 | 0.8537 | |
8 | 0.8954 | 0.8796 | 0.8681 | 0.8172 | 0.8891 | 0.8519 | 0.8990 | 0.9067 | |
10 | 0.9103 | 0.9290 | 0.9146 | 0.8471 | 0.9256 | 0.7991 | 0.9226 | 0.9299 | |
12 | 0.9293 | 0.9389 | 0.9386 | 0.8743 | 0.9315 | 0.8680 | 0.9425 | 0.9501 | |
Flower | 4 | 0.7495 | 0.7505 | 0.7495 | 0.7293 | 0.7507 | 0.7504 | 0.7505 | 0.7508 |
6 | 0.8086 | 0.8097 | 0.8101 | 0.7886 | 0.8096 | 0.8024 | 0.8095 | 0.8202 | |
8 | 0.8317 | 0.8419 | 0.8411 | 0.8211 | 0.8494 | 0.8519 | 0.8464 | 0.8705 | |
10 | 0.8605 | 0.8796 | 0.8741 | 0.8586 | 0.8784 | 0.8538 | 0.8769 | 0.8935 | |
12 | 0.8736 | 0.9073 | 0.8994 | 0.8478 | 0.9015 | 0.8888 | 0.8969 | 0.9255 | |
Mountain | 4 | 0.7490 | 0.7600 | 0.7600 | 0.7470 | 0.7390 | 0.7640 | 0.7385 | 0.7857 |
6 | 0.8040 | 0.8101 | 0.8111 | 0.7933 | 0.8088 | 0.7753 | 0.8046 | 0.8142 | |
8 | 0.8467 | 0.8612 | 0.8539 | 0.8003 | 0.8485 | 0.8084 | 0.8498 | 0.8765 | |
10 | 0.8444 | 0.8899 | 0.8723 | 0.8368 | 0.8834 | 0.8370 | 0.8810 | 0.9131 | |
12 | 0.8988 | 0.9099 | 0.9081 | 0.8771 | 0.9113 | 0.8453 | 0.9067 | 0.9313 | |
Tree | 4 | 0.7803 | 0.7816 | 0.7803 | 0.7881 | 0.7804 | 0.7947 | 0.7800 | 0.7803 |
6 | 0.8303 | 0.8366 | 0.8311 | 0.8372 | 0.8304 | 0.8367 | 0.8340 | 0.8398 | |
8 | 0.8575 | 0.8602 | 0.8583 | 0.8362 | 0.8606 | 0.8490 | 0.8586 | 0.8795 | |
10 | 0.8741 | 0.8850 | 0.8855 | 0.8588 | 0.8862 | 0.8377 | 0.8889 | 0.8985 | |
12 | 0.8962 | 0.8987 | 0.8983 | 0.8545 | 0.9015 | 0.8627 | 0.9035 | 0.9141 | |
Horse | 4 | 0.7725 | 0.7725 | 0.7844 | 0.7942 | 0.8057 | 0.7902 | 0.7952 | 0.8125 |
6 | 0.8344 | 0.8467 | 0.8465 | 0.8092 | 0.8422 | 0.8260 | 0.8479 | 0.8604 | |
8 | 0.8722 | 0.8784 | 0.8781 | 0.8486 | 0.8800 | 0.8444 | 0.8832 | 0.8946 | |
10 | 0.8977 | 0.9053 | 0.9011 | 0.8600 | 0.9005 | 0.8459 | 0.9058 | 0.9087 | |
12 | 0.9126 | 0.9234 | 0.9201 | 0.8674 | 0.9186 | 0.8707 | 0.9207 | 0.9240 | |
Bridge | 4 | 0.8220 | 0.8221 | 0.8219 | 0.8042 | 0.8221 | 0.8083 | 0.8234 | 0.8378 |
6 | 0.8894 | 0.8909 | 0.8908 | 0.8475 | 0.8897 | 0.8495 | 0.8917 | 0.8928 | |
8 | 0.9137 | 0.9215 | 0.9209 | 0.8719 | 0.9208 | 0.8902 | 0.9215 | 0.9224 | |
10 | 0.9317 | 0.9350 | 0.9277 | 0.8925 | 0.9313 | 0.9038 | 0.9353 | 0.9467 | |
12 | 0.9394 | 0.9567 | 0.9453 | 0.9113 | 0.9503 | 0.9190 | 0.9476 | 0.9580 | |
Pilot | 4 | 0.7901 | 0.7894 | 0.7893 | 0.7740 | 0.7887 | 0.7850 | 0.7874 | 0.7912 |
6 | 0.8303 | 0.8409 | 0.8326 | 0.8103 | 0.8337 | 0.8292 | 0.8284 | 0.8343 | |
8 | 0.8726 | 0.8726 | 0.8749 | 0.8554 | 0.8755 | 0.8649 | 0.8751 | 0.8758 | |
10 | 0.8991 | 0.8872 | 0.8983 | 0.8829 | 0.8984 | 0.8782 | 0.9023 | 0.9063 | |
12 | 0.9158 | 0.9158 | 0.9225 | 0.8776 | 0.9177 | 0.8890 | 0.9175 | 0.9237 | |
Dog | 4 | 0.7595 | 0.7594 | 0.7375 | 0.7074 | 0.7580 | 0.7413 | 0.7621 | 0.7625 |
6 | 0.8043 | 0.8208 | 0.8182 | 0.8037 | 0.8205 | 0.7507 | 0.8212 | 0.8490 | |
8 | 0.8582 | 0.8617 | 0.8609 | 0.8404 | 0.8615 | 0.8355 | 0.8609 | 0.8911 | |
10 | 0.8772 | 0.9033 | 0.8918 | 0.8788 | 0.9032 | 0.8278 | 0.8976 | 0.9269 | |
12 | 0.9092 | 0.9254 | 0.9151 | 0.8727 | 0.9178 | 0.8642 | 0.9187 | 0.9275 |
IMAGE | K | PSNR | SSIM | FSIM | |||
Otsu | Kapur | Otsu | Kapur | Otsu | Kapur | ||
Cactus | 4 | 19.1901 | 17.2250 | 0.6063 | 0.4858 | 0.8104 | 0.7711 |
6 | 21.4849 | 20.9242 | 0.6963 | 0.5690 | 0.8681 | 0.8363 | |
8 | 23.7403 | 23.1098 | 0.7904 | 0.7681 | 0.8980 | 0.8924 | |
10 | 24.1499 | 25.6436 | 0.8391 | 0.8677 | 0.9153 | 0.9227 | |
12 | 25.2868 | 26.7329 | 0.8456 | 0.8873 | 0.9287 | 0.9375 | |
Kangaroo | 4 | 22.0797 | 18.8067 | 0.7735 | 0.6116 | 0.8293 | 0.7574 |
6 | 24.8836 | 22.3708 | 0.8627 | 0.7782 | 0.9001 | 0.8713 | |
8 | 27.0860 | 24.6322 | 0.9084 | 0.8521 | 0.9335 | 0.9231 | |
10 | 28.8186 | 26.1974 | 0.9331 | 0.8913 | 0.9541 | 0.9482 | |
12 | 30.3411 | 28.0349 | 0.9495 | 0.9254 | 0.9653 | 0.9632 | |
Temple | 4 | 17.6125 | 18.6105 | 0.6798 | 0.6149 | 0.7840 | 0.7591 |
6 | 21.4589 | 23.1506 | 0.7263 | 0.7784 | 0.8203 | 0.8537 | |
8 | 23.9977 | 25.1273 | 0.8152 | 0.8465 | 0.8722 | 0.9067 | |
10 | 25.7059 | 26.7757 | 0.8643 | 0.8787 | 0.8994 | 0.9299 | |
12 | 27.5232 | 28.4027 | 0.8917 | 0.9021 | 0.9301 | 0.9501 | |
Flower | 4 | 22.6916 | 21.4046 | 0.4916 | 0.3994 | 0.7841 | 0.7508 |
6 | 25.5506 | 24.2923 | 0.7406 | 0.5247 | 0.8418 | 0.8202 | |
8 | 27.6960 | 26.3947 | 0.8000 | 0.6431 | 0.8874 | 0.8705 | |
10 | 29.2169 | 27.6016 | 0.8294 | 0.6606 | 0.9120 | 0.8935 | |
12 | 30.7280 | 29.2750 | 0.8682 | 0.7643 | 0.9347 | 0.9255 | |
Mountain | 4 | 19.7307 | 17.7501 | 0.7081 | 0.6240 | 0.8006 | 0.7857 |
6 | 22.5039 | 20.2658 | 0.7932 | 0.7131 | 0.8563 | 0.8142 | |
8 | 24.9053 | 23.7160 | 0.8322 | 0.8259 | 0.8903 | 0.8765 | |
10 | 26.3373 | 26.2667 | 0.8603 | 0.8792 | 0.9142 | 0.9131 | |
12 | 27.4991 | 26.6949 | 0.8780 | 0.9085 | 0.9253 | 0.9313 | |
Tree | 4 | 19.9364 | 19.3878 | 0.6915 | 0.6264 | 0.8166 | 0.7803 |
6 | 22.9388 | 22.0355 | 0.7546 | 0.7219 | 0.8568 | 0.8398 | |
8 | 25.1704 | 24.2661 | 0.7960 | 0.7737 | 0.8840 | 0.8795 | |
10 | 26.7213 | 25.7360 | 0.8281 | 0.8214 | 0.9055 | 0.8985 | |
12 | 28.0724 | 26.8461 | 0.8579 | 0.8792 | 0.9208 | 0.9141 | |
Horse | 4 | 19.1569 | 19.7788 | 0.7206 | 0.7070 | 0.8217 | 0.8125 |
6 | 22.8301 | 21.6974 | 0.7744 | 0.7802 | 0.8506 | 0.8604 | |
8 | 24.8543 | 24.2427 | 0.8117 | 0.8335 | 0.8852 | 0.8946 | |
10 | 26.8845 | 26.1268 | 0.8534 | 0.8697 | 0.9122 | 0.9087 | |
12 | 28.3129 | 27.4180 | 0.8793 | 0.8979 | 0.9303 | 0.9240 | |
Bridge | 4 | 18.7684 | 18.3564 | 0.7398 | 0.7176 | 0.8399 | 0.8378 |
6 | 21.5346 | 21.1004 | 0.8193 | 0.8053 | 0.8974 | 0.8928 | |
8 | 23.7010 | 23.6124 | 0.8691 | 0.8820 | 0.9241 | 0.9224 | |
10 | 25.4265 | 25.3739 | 0.8993 | 0.9160 | 0.9398 | 0.9467 | |
12 | 27.0756 | 27.4320 | 0.9226 | 0.9393 | 0.9499 | 0.9580 | |
Pilot | 4 | 17.6971 | 16.0238 | 0.7399 | 0.7512 | 0.7855 | 0.7912 |
6 | 21.5230 | 20.7123 | 0.8224 | 0.8317 | 0.8345 | 0.8343 | |
8 | 22.4562 | 23.1598 | 0.8424 | 0.8672 | 0.8625 | 0.8758 | |
10 | 24.1912 | 24.8228 | 0.8788 | 0.8937 | 0.8918 | 0.9063 | |
12 | 25.8213 | 27.1095 | 0.9017 | 0.9124 | 0.9148 | 0.9237 | |
Dog | 4 | 19.9346 | 18.2605 | 0.7428 | 0.6599 | 0.7943 | 0.7625 |
6 | 22.6726 | 21.9672 | 0.7756 | 0.7797 | 0.8521 | 0.8490 | |
8 | 24.3548 | 24.5205 | 0.8240 | 0.8486 | 0.8834 | 0.8911 | |
10 | 26.4961 | 26.3186 | 0.8655 | 0.9002 | 0.9109 | 0.9269 | |
12 | 28.1328 | 27.2748 | 0.8948 | 0.8889 | 0.9288 | 0.9275 |
IMAGE | K | R | G | B |
Cactus | 4 | 48, 79,124,194 | 58, 90,126,187 | 54, 85,123,180 |
6 | 40, 63, 85,115,155,209 | 50, 74, 97,121,159,212 | 46, 67, 88,115,146,192 | |
8 | 36, 54, 71, 89,113,144,181,225 | 45, 64, 82, 99,118,142,177,221 | 41, 58, 74, 91,112,137,165,204 | |
10 | 34, 49, 63, 77, 91,109,133,160,190,227 | 43, 58, 73, 87,101,115,133,159,192,228 | 38, 51, 64, 77, 92,111,131,153,178,214 | |
12 | 33, 46, 58, 70, 82, 95,113,134,156,182,209,235 | 40, 53, 66, 79, 91,102,114,129,148,173,200,232 | 37, 48, 60, 71, 82, 96,112,130,147,166,189,220 | |
Kangaroo | 4 | 54, 87,116,155 | 49, 87,112,143 | 43, 72,103,145 |
6 | 41, 72, 92,113,140,173 | 38, 73, 95,113,134,163 | 32, 58, 77, 99,128,163 | |
8 | 34, 62, 80, 95,111,131,156,186 | 32, 62, 82, 97,111,125,145,175 | 28, 51, 67, 82,100,122,148,180 | |
10 | 31, 56, 72, 85, 97,110,126,145,166,195 | 28, 55, 74, 88,100,111,123,138,156,185 | 23, 44, 59, 71, 84, 99,117,138,161,190 | |
12 | 24, 47, 63, 76, 87, 97,108,122,139,157,177,206 | 24, 47, 65, 79, 91,101,110,120,131,145,162,187 | 19, 37, 51, 63, 73, 84, 96,111,128,147,167,196 | |
Temple | 4 | 80,116,152,207 | 81,111,141,178 | 62, 87,115,145 |
6 | 65, 89,113,137,165,213 | 71, 93,115,138,165,208 | 53, 70, 88,107,127,151 | |
8 | 57, 75, 94,112,130,149,172,216 | 64, 81, 98,115,132,150,172,210 | 47, 61, 74, 89,104,120,138,157 | |
10 | 55, 71, 87,103,118,134,151,172,200,234 | 60, 74, 88,102,115,129,143,160,178,212 | 45, 58, 69, 81, 95,109,124,141,159,239 | |
12 | 51, 65, 78, 92,105,117,130,142,156,174,201,233 | 57, 70, 83, 96,108,120,133,147,162,179,201,229 | 42, 53, 61, 69, 79, 90,101,111,122,135,148,162 | |
Flower | 4 | 51, 98,143,201 | 31, 69,111,168 | 19, 44, 75,119 |
6 | 34, 66, 98,131,163,209 | 22, 47, 77,109,150,202 | 7, 18, 37, 58, 84,126 | |
8 | 30, 55, 79,103,129,155,182,220 | 10, 25, 45, 68, 92,120,159,207 | 6, 14, 26, 42, 59, 78,102,140 | |
10 | 25, 44, 84,104,126,149,169,192,226 | 10, 23, 39, 57, 76, 95,117,144,178,218 | 6, 14, 26, 40, 56, 73, 94,126,176,252 | |
12 | 21, 36, 53, 70, 87,104,122,141,159,177,201,232 | 9, 20, 33, 48, 63, 79, 95,112,133,158,187,221 | 5, 10, 16, 25, 36, 48, 60, 73, 90,109,139,194 | |
Mountain | 4 | 50, 76,111,190 | 58, 91,124,190 | 58, 94,131,192 |
6 | 40, 58, 76,100,135,201 | 48, 69, 94,118,144,204 | 53, 76, 97,125,149,196 | |
8 | 36, 52, 67, 82,103,130,167,221 | 45, 63, 79, 99,118,139,174,224 | 39, 59, 78, 96,121,142,157,199 | |
10 | 34, 48, 60, 71, 84,102,123,148,187,230 | 2, 44, 62, 78, 96,112,124,144,182,229 | 38, 57, 75, 88,105,125,143,156,183,224 | |
12 | 34, 47, 58, 67, 76, 88,103,119,138,161,195,230 | 9, 43, 58, 69, 82, 98,111,122,137,157,189,233 | 37, 53, 68, 79, 92,110,127,141,151,160,183,224 | |
Tree | 4 | 30, 67,103,155 | 51,106,161,207 | 54,120,185,225 |
6 | 13, 35, 65, 96,132,175 | 39, 72,112,152,183,216 | 38, 74,125,173,203,229 | |
8 | 12, 31, 52, 72, 94,116,148,185 | 36, 63, 98,133,161,184,207,231 | 30, 52, 81,119,157,186,208,231 | |
10 | 10, 23, 39, 56, 74, 94,114,139,169,197 | 30, 50, 73,100,127,150,169,187,208,231 | 28, 46, 68, 97,130,160,184,202,215,234 | |
12 | 9, 22, 37, 51, 66, 82, 97,115,136,157,181,203 | 28, 45, 63, 84,107,130,150,166,179,192,210,233 | 24, 37, 51, 69, 92,119,147,171,190,205,217,235 | |
Horse | 4 | 59,100,145,193 | 54, 94,142,190 | 33, 61, 86,147 |
6 | 49, 83,111,143,179,208 | 46, 78,104,139,177,203 | 27, 51, 72, 93,145,193 | |
8 | 42, 70, 93,114,139,169,194,215 | 38, 65, 89,110,137,169,193,212 | 23, 44, 63, 80,101,148,189,200 | |
10 | 34, 56, 77, 95,111,130,155,180,200,218 | 30, 51, 71, 88,103,119,143,172,194,212 | 18, 33, 47, 60, 72, 84, 98,123,162,194 | |
12 | 29, 48, 67, 83, 98,112,127,147,168,187,204,220 | 29, 46, 63, 79, 93,106,120,142,166,185,200,215 | 17, 31, 44, 56, 67, 78, 89,103,128,164,190,201 | |
Bridge | 4 | 73,113,158,216 | 69,109,151,210 | 35, 75,116,159 |
6 | 61, 89,116,145,180,225 | 61, 92,120,148,181,224 | 24, 51, 80,110,139,169 | |
8 | 55, 77, 99,120,142,168,198,233 | 52, 74, 96,118,140,165,197,233 | 17, 36, 58, 81,105,127,148,172 | |
10 | 50, 68, 86,104,121,139,161,184,211,239 | 48, 67, 86,104,122,140,160,183,211,239 | 13, 29, 46, 63, 81,100,119,136,153,174 | |
12 | 48, 64, 79, 95,110,125,140,158,178,199,224,246 | 44, 60, 76, 91,106,122,137,154,172,192,218,243 | 11, 25, 40, 55, 71, 88,105,121,135,149,166,182 | |
Pilot | 4 | 102,149,194,224 | 104,157,205,235 | 83,123,167,220 |
6 | 90,120,155,191,217,234 | 88,117,150,187,217,239 | 78,111,149,181,212,243 | |
8 | 80,102,127,157,187,210,225,238 | 81,103,127,154,185,209,225,241 | 66, 87,109,136,165,188,214,243 | |
10 | 77, 96,116,135,157,180,200,216,228,239 | 76, 95,114,134,158,185,206,220,233,246 | 61, 78, 96,115,137,162,181,195,217,244 | |
12 | 72, 89,105,121,138,158,180,197,212,223,232,242 | 72, 86,100,117,136,159,184,203,216,226,237,248 | 61, 77, 94,112,132,154,172,185,196,214,234,248 | |
Dog | 4 | 50, 84,115,157 | 73,107,145,188 | 52, 83,123,161 |
6 | 38, 66, 89,112,133,168 | 61, 84,108,136,161,193 | 45, 67, 93,125,156,178 | |
8 | 33, 58, 78, 97,115,130,150,184 | 57, 75, 93,112,135,156,171,199 | 42, 60, 79,102,131,157,175,193 | |
10 | 30, 52, 70, 86,102,118,132,151,183,243 | 53, 69, 84,100,117,136,154,167,181,207 | 36, 49, 64, 80, 99,122,145,163,177,196 | |
12 | 24, 42, 57, 71, 84, 97,109,121,132,147,170,197 | 48, 61, 73, 87,101,115,131,147,159,169,183,209 | 33, 44, 57, 70, 83,100,121,141,157,168,179,196 |
IMAGE | K | R | G | B |
Cactus | 4 | 63,103,146,189 | 73,115,151,196 | 68,111,163,206 |
6 | 58, 92,121,153,185,216 | 62, 92,123,151,184,215 | 60, 95,129,162,190,222 | |
8 | 50, 77,102,128,154,180,204,229 | 56, 81,106,129,152,177,202,226 | 19, 53, 80,106,134,163,190,221 | |
10 | 43, 63, 83,103,122,143,164,185,207,230 | 49, 69, 89,109,129,148,169,191,212,233 | 19, 48, 71, 93,115,139,163,186,208,230 | |
12 | 17, 42, 64, 83,101,120,139,159,178,197,215,234 | 46, 63, 80, 97,115,132,148,165,183,201,219,237 | 19, 42, 58, 76, 94,112,130,148,166,187,208,231 | |
Kangaroo | 4 | 52,113,158,203 | 51, 90,138,188 | 40, 97,150,203 |
6 | 43, 75,113,148,184,215 | 47, 83,123,153,186,220 | 36, 68,102,142,185,220 | |
8 | 32, 58, 84,113,141,169,198,227 | 35, 61, 86,113,139,168,194,220 | 31, 57, 84,108,136,165,193,220 | |
10 | 24, 45, 66, 90,115,138,162,186,208,231 | 21, 42, 63, 86,109,133,153,175,195,220 | 25, 47, 69, 91,114,138,161,185,207,231 | |
12 | 20, 40, 58, 78, 98,117,136,157,178,198,215,234 | 17, 38, 56, 75, 94,114,132,152,171,190,210,229 | 18, 36, 55, 74, 94,115,135,154,174,194,214,233 | |
Temple | 4 | 74,114,153,192 | 82,120,158,195 | 46, 79,113,145 |
6 | 64, 96,128,160,194,225 | 72,103,134,163,196,226 | 25, 49, 80,113,144,178 | |
8 | 40, 65, 91,116,142,166,194,225 | 62, 89,116,143,169,196,216,236 | 23, 44, 67, 89,111,133,155,178 | |
10 | 39, 60, 82,103,126,149,170,194,214,235 | 46, 68, 89,109,130,151,173,196,216,235 | 18, 31, 47, 64, 81, 99,118,137,156,183 | |
12 | 35, 51, 69, 88,106,125,142,159,176,194,213,238 | 41, 55, 73, 90,107,123,141,159,178,196,216,235 | 18, 31, 46, 63, 80, 97,111,127,144,158,178,187 | |
Flower | 4 | 53, 99,143,187 | 49,106,153,202 | 27, 75,118,175 |
6 | 42, 75,108,143,177,206 | 32, 69,106,140,175,211 | 20, 51, 82,113,145,175 | |
8 | 37, 65, 94,121,149,177,202,227 | 25, 54, 83,112,140,169,196,223 | 20, 47, 75,102,127,150,175,197 | |
10 | 28, 50, 73, 95,116,137,159,183,205,228 | 22, 45, 68, 92,115,138,161,184,207,230 | 17, 36, 55, 76, 97,116,135,155,175,197 | |
12 | 26, 46, 66, 84,102,121,140,158,176,195,214,233 | 20, 39, 58, 78, 98,116,136,155,175,196,216,236 | 14, 27, 42, 56, 71, 87,103,118,135,155,175,198 | |
Mountain | 4 | 53, 89,124,156 | 87,138,177,218 | 88,132,173,215 |
6 | 53, 91,129,168,199,232 | 73,103,138,172,207,241 | 19, 63, 95,132,173,215 | |
8 | 52, 81,107,134,162,188,216,245 | 51, 77,102,136,160,185,213,241 | 19, 46, 65, 87,109,133,173,210 | |
10 | 22, 51, 74, 97,123,147,168,192,218,245 | 51, 76,101,119,138,160,181,203,223,244 | 19, 45, 65, 87,109,131,151,173,199,227 | |
12 | 21, 40, 60, 82,103,124,145,167,185,205,224,247 | 48, 64, 82,101,120,137,154,172,188,206,224,244 | 19, 45, 65, 89,111,132,151,172,185,200,218,236 | |
Tree | 4 | 51, 92,134,182 | 63,112,157,206 | 56, 99,148,192 |
6 | 29, 63, 97,132,168,206 | 45, 77,110,141,170,207 | 44, 78,113,151,190,225 | |
8 | 25, 55, 83,110,134,162,190,218 | 39, 66, 94,123,152,180,206,230 | 38, 65, 91,118,145,170,196,225 | |
10 | 22, 43, 65, 88,111,133,155,178,198,219 | 33, 54, 75, 96,117,139,160,183,206,229 | 33, 55, 77, 99,119,140,160,182,203,225 | |
12 | 21, 42, 63, 84,108,131,149,167,183,200,218,235 | 29, 47, 65, 83,100,117,134,152,168,187,206,230 | 28, 46, 63, 81, 99,119,138,156,174,190,206,225 | |
Horse | 4 | 52, 91,136,187 | 68,124,176,235 | 53,117,174,214 |
6 | 43, 77,111,142,177,213 | 49, 86,126,161,193,235 | 35, 67,103,134,173,214 | |
8 | 35, 62, 88,115,141,169,194,222 | 38, 67, 96,127,156,184,211,235 | 30, 59, 89,114,142,173,192,214 | |
10 | 31, 55, 77, 99,123,144,167,191,213,235 | 32, 55, 78,102,127,150,172,193,213,235 | 22, 44, 64, 86,105,125,148,173,192,214 | |
12 | 27, 46, 66, 85,104,123,142,160,178,198,219,239 | 28, 46, 64, 82,100,120,136,156,176,195,215,235 | 19, 35, 51, 70, 87,103,119,136,155,173,190,214 | |
Bridge | 4 | 70,113,156,199 | 77,129,180,227 | 49, 96,142,194 |
6 | 63, 98,133,167,201,236 | 60, 93,126,159,193,230 | 32, 62, 92,122,155,194 | |
8 | 54, 80,106,132,158,184,209,236 | 52, 78,105,132,158,185,210,236 | 26, 49, 73, 97,121,146,171,195 | |
10 | 48, 70, 92,113,134,156,177,198,218,239 | 47, 68, 90,111,133,155,177,198,219,238 | 24, 45, 68, 90,112,133,155,176,194,209 | |
12 | 45, 62, 79, 97,114,132,149,167,184,201,220,239 | 44, 62, 80, 99,116,134,152,169,186,204,222,240 | 22, 40, 58, 74, 89,105,121,139,157,176,194,209 | |
Pilot | 4 | 94,134,169,204 | 100,146,191,220 | 74,106,139,173 |
6 | 81,110,139,168,197,221 | 81,108,135,163,192,220 | 41, 85,125,168,203,235 | |
8 | 72, 96,119,143,168,194,216,238 | 74, 98,121,145,168,191,210,231 | 34, 67, 92,118,144,171,203,235 | |
10 | 69, 87,106,125,144,164,183,202,220,238 | 70, 89,108,127,146,164,182,200,218,236 | 28, 42, 68, 91,115,139,163,182,205,235 | |
12 | 55, 70, 86,103,120,136,151,168,185,203,220,238 | 67, 82, 98,113,129,145,160,175,191,206,221,238 | 28, 42, 67, 88,110,130,149,168,185,205,227,245 | |
Dog | 4 | 43, 92,146,187 | 74,112,149,192 | 62,102,149,200 |
6 | 38, 73,110,145,174,206 | 62, 90,119,150,184,215 | 56, 91,124,157,195,217 | |
8 | 29, 55, 84,113,144,167,190,214 | 61, 87,113,138,159,184,206,233 | 46, 72, 97,124,152,175,196,217 | |
10 | 21, 40, 60, 80,100,120,144,167,190,214 | 55, 76, 99,120,140,159,182,198,215,233 | 18, 45, 68, 91,114,137,157,176,196,217 | |
12 | 20, 39, 57, 75, 92,110,127,144,160,179,198,217 | 49, 66, 82, 99,117,133,149,165,182,197,215,233 | 18, 39, 56, 72, 89,105,122,141,159,178,196,217 |