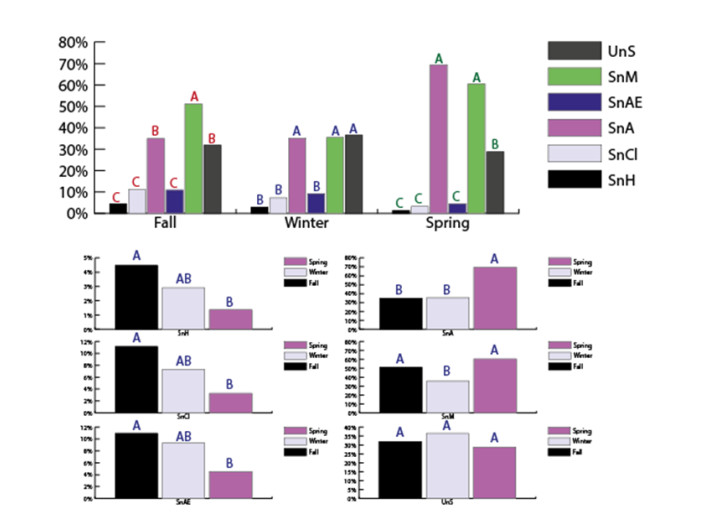
In this paper, a three-dimensional nonlinear delay differential system including Tumour cells, cytotoxic-T lymphocytes, T-helper cells is constructed to investigate the effects of intrinsic recruitment delay and chemotherapy. It is found that the introduction of chemotherapy and time delay can generate richer dynamics in tumor-immune system. In particular, there exists bistable phenomenon and the tumour cells would be cleared if the effect of chemotherapy on depletion of the tumour cells is strong enough or the side effect of chemotherapy on the hunting predator cells is under a threshold. It is also shown that a branch of stable periodic solutions bifurcates from the coexistence equilibrium when the intrinsic recruitment delay of tumor crosses the threshold which is new mechanism, which can help understand the short-term oscillations in tumour sizes as well as long-term tumour relapse. Numerical simulations are presented to illustrate that larger intrinsic recruitment delay of tumor leads to larger amplitude and longer period of the bifurcated periodic solution, which indicates that there exists longer relapse time and then contributes to the control of tumour growth.
Citation: Qingfeng Tang, Guohong Zhang. Stability and Hopf bifurcations in a competitive tumour-immune system with intrinsic recruitment delay and chemotherapy[J]. Mathematical Biosciences and Engineering, 2021, 18(3): 1941-1965. doi: 10.3934/mbe.2021101
[1] | Nicolas Nagahama, Bruno Gastaldi, Michael N. Clifford, María M. Manifesto, Renée H. Fortunato . The influence of environmental variations on the phenolic compound profiles and antioxidant activity of two medicinal Patagonian valerians (Valeriana carnosa Sm. and V. clarionifolia Phil.). AIMS Agriculture and Food, 2021, 6(1): 106-124. doi: 10.3934/agrfood.2021007 |
[2] | María Cámara-Ruiz, José María García Beltrán, Francisco Antonio Guardiola, María Ángeles Esteban . In vitro and in vivo effects of purslane (Portulaca oleracea L.) on gilthead seabream (Sparus aurata L.). AIMS Agriculture and Food, 2020, 5(4): 799-824. doi: 10.3934/agrfood.2020.4.799 |
[3] | Patrick A. Blamo Jr, Hong Ngoc Thuy Pham, The Han Nguyen . Maximising phenolic compounds and antioxidant capacity from Laurencia intermedia using ultrasound-assisted extraction. AIMS Agriculture and Food, 2021, 6(1): 32-48. doi: 10.3934/agrfood.2021003 |
[4] | Nelson Mpumi, Revocatus L. Machunda, Kelvin M. Mtei, Patrick A. Ndakidemi . Insecticidal Efficacy of Syzygium aromaticum, Tephrosia vogelii and Croton dichogamus Extracts against Plutella xylostella and Trichoplusia ni on Brassica oleracea crop in Northern Tanzania. AIMS Agriculture and Food, 2021, 6(1): 185-202. doi: 10.3934/agrfood.2021012 |
[5] | Clarissa Trindade, Carla S.S. Gouveia, Maria Cristina O. Oliveira, Humberto Nóbrega, José G.R. de Freitas, Miguel A.A. Pinheiro de Carvalho . Inhibitory activity of the peel and seed of Annona cherimola Mill. against Fusarium and Aspergillus pathogens. AIMS Agriculture and Food, 2025, 10(1): 155-176. doi: 10.3934/agrfood.2025009 |
[6] | Tarik Ainane, Fatouma Mohamed Abdoul-Latif, Asmae Baghouz, Zineb El Montassir, Wissal Attahar, Ayoub Ainane, Angelo Maria Giuffrè . Correction: Essential oils rich in pulegone for insecticide purpose against legume bruchus species: Case of Ziziphora hispanica L. and Mentha pulegium L.. AIMS Agriculture and Food, 2023, 8(1): 236-238. doi: 10.3934/agrfood.2023013 |
[7] | Oksana B. Polivanova, Mikhail Yu. Cherednichenko, Elena A. Kalashnikova, Rima N. Kirakosyan . In vitro antibacterial effect of silver nanoparticles synthetized using Agastache foeniculum plant and callus extracts. AIMS Agriculture and Food, 2021, 6(2): 631-643. doi: 10.3934/agrfood.2021037 |
[8] | Rita Khathir, Murna Muzaifa, Yunita, Marai Rahmawati . Study on the physicochemical and sensory profile of pliek-u: A traditional dried fermented coconut endosperm from Aceh, Indonesia. AIMS Agriculture and Food, 2023, 8(2): 534-549. doi: 10.3934/agrfood.2023028 |
[9] | Dody Dwi Handoko, Anisa Maharani Kaseh, Laras Cempaka, Wahyudi David, Bram Kusbiantoro, Afifah Zahra Agista, Yusuke Ohsaki, Hitoshi Shirakawa, Ardiansyah . Effects of household-scale cooking on volatile compounds, sensory profile, and hypotensive effect of Kenikir (Cosmos caudatus). AIMS Agriculture and Food, 2023, 8(1): 198-213. doi: 10.3934/agrfood.2023011 |
[10] | Francyelli Regina Costa-Becheleni, Enrique Troyo-Diéguez, Alan Amado Ruiz-Hernández, Fernando Ayala-Niño, Luis Alejandro Bustamante-Salazar, Alfonso Medel-Narváez, Raúl Octavio Martínez-Rincón, Rosario Maribel Robles-Sánchez . Determination of bioactive compounds and antioxidant capacity of the halophytes Suaeda edulis and Suaeda esteroa (Chenopodiaceae): An option as novel healthy agro-foods. AIMS Agriculture and Food, 2024, 9(3): 716-742. doi: 10.3934/agrfood.2024039 |
In this paper, a three-dimensional nonlinear delay differential system including Tumour cells, cytotoxic-T lymphocytes, T-helper cells is constructed to investigate the effects of intrinsic recruitment delay and chemotherapy. It is found that the introduction of chemotherapy and time delay can generate richer dynamics in tumor-immune system. In particular, there exists bistable phenomenon and the tumour cells would be cleared if the effect of chemotherapy on depletion of the tumour cells is strong enough or the side effect of chemotherapy on the hunting predator cells is under a threshold. It is also shown that a branch of stable periodic solutions bifurcates from the coexistence equilibrium when the intrinsic recruitment delay of tumor crosses the threshold which is new mechanism, which can help understand the short-term oscillations in tumour sizes as well as long-term tumour relapse. Numerical simulations are presented to illustrate that larger intrinsic recruitment delay of tumor leads to larger amplitude and longer period of the bifurcated periodic solution, which indicates that there exists longer relapse time and then contributes to the control of tumour growth.
Fouquieria splendens, commonly called Ocotillo [1] is, together with the Boojum tree (F. columnaris), the most representative member of the thirteen species of the Fouquieriaceae family [2,3]. It is a shallow-rooted, ligneous succulent plant with non-xeromorphic leaves; the stems have water-storing cells [4]. It is endemic to the arid and semi-arid zones of North-ern Mexico and the Southwestern United States [5,6], typically found in exposed rocky slopes or calcareous soil plains with high calcium carbonate contents selenite of the Mojave, Sonora, and Chihuahua deserts [3]. Nearly 80% of the human population – approximately four billion people – uses plants as the primary remedy for many diseases [7]. A notable example is the use of fresh stems of F. splendens, used in Mexican traditional medicine to help in the treatment of pelvic circulation, congestion, abdominal swelling, hemorrhoids, and prostatic hyperplasia, among others. Applications are also associated with fat assimilation, glucose metabolism regulation, and alleviation of coughing, varicose veins, urinary infections, menstrual cramps, and pelvic pain [8]. Some authors suggest that ocotillo may prevent and treat chronic diseases such as ulcerative colitis, Crohn's disease, Alzheimer's disease, diabetes, liver disorders and cancer [9,10]. It has been reported that secondary metabolites play a significant role in plants' defense mechanism [11]; that is the reason for the interest in understanding how secondary metabolites allow different plant species and populations to adapt and defend from these changing conditions and for determining which factors to attribute the increase in concentration, production, or inhibition of phytotherapeutic compounds [12]. Antimicrobial activity of ocotillo methanol extracts has been determined against bacteria such as B. cereus, B. subtilis, E. coli and S. aureus; in addition to this, the antifungal efficiency of these extracts against M. gypseum, M. nanum, M. cookie and M. canis fungi was demonstrated at a 125 mg/ mL concentration, and antiparasitic activity against E. histolytica trophozoites was demonstrated at a 0.25 mg/ mL concentration [13,14]. Likewise, the use of Ocotillol type terpenoid antiseptic molecules from the ocotillo plant has been identified and their ability to improve the susceptibility of some antibiotics has been determined, showing strong effects against bacteria such as S. aureus, B. subtilis and even P. aeruginosa because these molecules may exert their antibacterial effect by damaging the bacterial cell membranes [15,16]. It has also been reported that methanol extracts of the ocotillo root show phytotoxic activity against L. sativa and L. perenne and herbicide activity against the green peach aphid M. persicae [11]. This information reveals the need to research the abundance, diversity, and toxicological effects of secondary metabolites from F. splendens that allow considering it as a phytotherapeutic alternative. The aim of this study is therefore to determine volatile compounds present in F. splendens stems using three different extraction methods and solvents with different polarities, as well as the extracts obtained against Artemia salina. No prior studies have been conducted using solvents with different polarities in different seasons of the year.
Ocotillo stems were collected during the fall, winter, and spring of 2019. The coordinates of the collection sites were 28°36′27.846″N, 105°51′38.923″W in the state of Chihuahua, Mexico. AbaTax taxonomic key database (www.Abatax.abaco2.org) was used using the key for families of flowering plants (Magnoliophyta) present in Mexico for the authentication of collected ocotillo plants. For the collection of plant material, the largest plants and the highest number of stems were selected. 1-meter-long ocotillo rods were cut, and the apical end (10 cm) was removed. The material was crushed and stored at 0 ℃ for later use.
Extracts from the F. splendens stems were obtained by three extraction methods:
Hydrodistillation: A modified Schilcher hydrodistillation devise was used (10 L capacity), where a portion of plant material corresponding to each season of the year was placed with distilled water at a 1:10 ratio (500 g/5 L); then it was boiled (model 301-10 SEV Mexico) for 6 hours [17].
Soxhlet solid-liquid extraction: Organic solvents with different polarities were selected: hexane (99.9% No. CAS 110-54-3 J.T. Baker), methylene chloride (99.6% No. CAS 75-09-02 J.T. Baker), ethyl acetate (99.9% No. CAS 141-78-6 J.T. Baker), acetone (99.6% No. CAS 67-64-1 J.T. Baker) and methanol (99.9% No. CAS 67-56-1 J.T. Baker). The plant material (40 g) was placed in the extractor with the solvent (200 mL) and the mixture was boiled (Corning Lab model PC-400D Mexico) for 5 hours. The samples were then filtered and concentrated by rotary evaporation (DLAB Scientific Instrument Inc. model RE100 Pro Ontario, CA United States) [18].
Ultrasound solid-liquid extraction: 50 g of plant material were placed with 125 mL water acidified with methanol, acetone, and 1% acetic acid at a 50:35:15 ratio in a beaker, which in turn was submerged in iced water inside of an ultrasound bath (Branson 5800, model CX5800H, Danbury, USA) for 30 minutes. Extraction was conducted at 40 kHz power. Once the sample was obtained, it was filtered and evaporated with the solvent mixture [19].
A total of twenty-one extracts were obtained from the three seasons analyzed, including three repetitions for each experiment. Extracts were identified with the following nomenclature: hexane (SnH), methyl chloride (SnCl), ethyl acetate (SnAE), methanol (SnM), acetone (SnA), hydrodistillation (HnC) and ultrasound (UnS) where n represents the season (1 = Fall; 2 = Winter; 3 = Spring).
Identification of the extract volatile components was conducted with a gas chromatographer (Agilent Technologies 7890B, Palo Alto, CA, USA) coupled with a mass spectrometer (Agilent Technologies 5975C, Palo Alto, CA, USA) equipped with an HP- INNOWax 19091N–133I column (30 m × 0.25 mm × 0.25 µm with a temperature range from 40 ℃ to 270 ℃, Agilent Inc., Santa Clara, CA, USA). The oven was set at 40 ℃, ramp at 4 ℃/min until 260 ℃, maintaining this temperature for 15 minutes. The injection temperature was 260 ℃ with 1 µL/min helium flow. The temperature at the source at 200 ℃ and a mass range of 40 to 600 a.m.u. was detected. Three repetitions were performed by injecting 1 µL of each of the samples with a 25:1 Split. Retention indices were calculated by the retention times of C6-C26 n-alkanes that was injected under the same chromatographic conditions. Identification of major chemical compounds was conducted by comparing the mass spectrums with the NIST 17 MS Demo ver.2.4, AMDIS_32 libraries (NIST, Washington, DC, USA).
For the toxicity analysis, the method of Meyer et al. [20] was followed with slight modifications. For the incubation of Artemia salina eggs (White Mountain, Great Salt Lake, Utah, USA), a 3.5% NaCl (Golden Bell, 99.9%, CAS No. 7647-14-5) solution was prepared as follows: 35 g of iodine-free sodium chloride and 0.006 g of baker's yeast were weighed and adjusted to one liter of water purified. The procedure was conducted by incubating 0.1 g of A. salina eggs in the saline solution and placing them in a 1 L beaker under dark and oxygenated conditions. At 24 h, the nauplii were transferred with the help of a sterile glass micropipette to another container and kept under oxygenation, lighting, and temperature conditions of 25 ℃ for 24 h. Ocotillo extracts were dissolved in a solution of 30% DMSO (ACS reagent, 99.9%, CAS No. 67-68-5) in 3.5% NaCl, they were tested at concentrations of 1000,750,500,250,100, 50, 10 and 1 mg/L. With a micropipette, ten nauplii (48 hours after hatching) were taken in sterile glass vials. Subsequently, 3 mL of the dilutions of the extracts to be evaluated are added. Potassium dichromate (Sigma Aldrich, 99%, CAS No. 7778-50-9) and 30% DMSO in 3.5% NaCl were used as a negative control and positive control. After applying the extracts, they were incubated for 24 hours at 25 ℃ and the dead Artemias were counted with the help of a stereoscopic microscope. Using the Probit method, the LD50 was determined [22,22].
To determine the median lethal dose (LD50), a Probit analysis was performed using a significance level of 0.05. Then, in the yield of the extracts and the lethal concentration, a 7 × 3 factorial analysis was conducted in which the factors extraction technique (heating, hexane, acetone, ethyl acetate, methanol, dichloromethane, the hydrodistillation technique, and the ultrasound technique) and the harvesting season of the ocotillo plants (fall, winter, and spring). After the above, an analysis of variance (ANOVA) was performed with mean differences through Tukey's analysis.
F. splendens is a deciduous plant in a response to the evolutionary effect of the environmental conditions that allow water to be conserved; therefore, it is possible that ocotillo and all plants exposed to changing conditions may generate changes in their chemical composition [4]. In addition to this, exposure to drought or stress caused by elevated levels of salt in ocotillo's endemic zones causes many common reactions in plants such as deficient secondary metabolite production and reduced yield percentage, less than 10% of the plant weight [12]. Using a 7 × 3 factorial analysis in which the conditions that arise from the extraction yield are evaluated. It was possible to conclude with 95% confidence that both the extraction techniques with different solvents, the harvesting season of the plants, and their interactions affected the extraction yields (P ˂ 0.05). being the extracts obtained by the soxhlet technique using acetone and methanol the ones that acquired the best yields. Results indicated that in the fall, higher extraction yields were obtained with S1M (51.12% ± 2.77); in the winter, with S2A (35.15% ± 4.77) and U2S (36.48% ± 2.42); in the spring with S3A (69.40% ± 4.82) and S3M (60.48% ± 4.59) as shown in Figure 1. Such polar extracts do not show significant differences in the yield percentage in the winter, unlike in the fall and spring, when methanol and acetone are the most effective solvents. Extracts obtained by ultrasound, if they were compared, would not show significant differences in the 3 seasons; this may be related to F. splendens characteristic of being highly adapted to extreme temperature conditions. It must be mentioned that extraction yields may be affected by the individual extraction conditions during each of the seasons analyzed [9].
In samples HnC (corresponding to essential oil) it was observed that it was not possible to obtain yield values, suggesting that F. splendens cannot synthetize enough essential oil in stems to be extracted by hydrodistillation in any of the evaluated seasons. This behavior in the plant may be related to the small number of single-cell trichrome present in the stems of this plant genus [23,24]. These structures are secreting glands that allow storing and expelling volatile compounds. Plants synthetize a wide range of metabolites and for a long time, it was thought that defensive chemicals were high priced for plants due to the resources consumed in their biosynthesis [25]. However, when plants are stressed or in changing weather conditions such as in the Chihuahua desert, the production of secondary metabolites may increase because growth is often inhibited more than photosynthesis, and fixed carbon is assigned to secondary metabolites [12]. Such variations in these metabolites may be observed in major eluents of each of the extracts obtained by GC-MS and compared with the AMDI-IS_32 y MestReNova databases with a 1% minimum area threshold for each peak; a total of thirty different volatile compounds were identified. As shown in Table 1 and Figure 2, the most abundant compounds present in extracts isolated by non-polar solvents were bis (2-Ethylhexyl) phthalate (DEHP) and bis (2-Ethylhexyl) ester (DEHA), palmitic acid, and myristic acid. On the other hand, in polar extracts, the most abundant compounds are butyl acetate, diacetone alcohol, Butyl acrylate, and 2-butoxyethanol. It is important to mention that phthalate esters have been extensively applied in the chemical industry as plasticizers or dissolvents, such as DEHP, considered an extremely toxic compound and extremely polluting to the environment. In addition to this, the US Environmental Protection Agency (EPA) classified DEHP as a probable human carcinogen; it has also been reported as an apoptosis inhibitor, a progesterone receptor agonist, and it alters insulin transduction, which exacerbates type 2 diabetes mellitus [26,27]. On the other hand, it is known that the presence of cold-tolerant compounds is associated with higher levels of phenolic molecules as reported by Wollenweber et al [3] and as determined by Monreal-García et al. [9], as well as linear chain molecules such as octacosane, octadecanol, and eicosane that were determined in this study and can be observed in Figure 2 in the winter [3,9,12].
No. | RT* (min) | Compounds | Relative peak area (%)b | ||||
IKCAL | Fall | Winter | Spring | ||||
HnC | |||||||
1 | 43.7 | DEHA | 1892 | 4.92± 0.62 | 95.88 ± 0.2 | tr | |
2 | 45.4 | palmitic acid | 2280 | Tr | tr | 12.72 ± 8.47 | |
3 | 51.5 | DEHP | 3104 | 95.08 ± 0.62 | 95.88 ± 0.2 | 86.1 ± 11.62 | |
SnH | |||||||
4 | 37.3 | tridecane | 1366 | Tr | tr- | 7.4 ± 1.8 | |
5 | 39 | pentadecanol | 1776 | Tr | 13.95 ± 2.25 | tr | |
6 | 41.3 | pentadecane, 2-methyl- | 1565 | Tr | tr | 8.48 ± 2.85 | |
7 | 41.7 | heptadecanol | 2287 | 1.61 ± 1.39 | 5.23 ± 0.74 | 22.39 ± 1.94 | |
8 | 43 | 4-methylpentadecane | 1559 | Tr | tr | 10.35 ± 2.32 | |
1 | 43.5 | DEHA | 1892 | Tr | 3.16 ± 1.63 | tr | |
9 | 44.5 | diethylene glycol hexyl ether | 1363 | Tr | 1.12 ± 1.47 | tr | |
2 | 45.6 | palmitic acid | 2280 | Tr | 1.62 ± 0.82 | 16.42 ± 4.06 | |
10 | 47.9 | 2-methylundecanoic acid | 1506 | Tr | 7.51 ± 4.35 | tr | |
11 | 48.6 | octacosane | 461 | 0.53 ± 0.83 | tr | tr | |
12 | 49.4 | eicosane | 2056 | 2.25 ± 1.19 | tr | tr | |
3 | 51.5 | DEHP | 3104 | 88.49 ± 2.16 | 67.41 ± 3.6 | 34.95 ± 8.24 | |
13 | 54 | methyl stearate | 2245 | 1.72 ±0.89 | tr | tr | |
SnCL | |||||||
14 | 23 | 2-Ethylhexyl glycidyl ether | 1363 | Tr | 3.66 ± 0.58 | 8.17 ± 2.02 | |
15 | 38.8 | octadecanol | 1846 | Tr | 9.93 ± 0.11 | tr | |
7 | 39.1 | heptadecanol | 2286 | 10.2 ± 0.36 | tr | 6.8 ± 0.36 | |
2 | 45.1 | palmitic acid | 2280 | Tr | 4.28 ± 0.44 | 8.81 ± 2.84 | |
12 | 49.3 | eicosane | 2056 | 9.24 ± 0.33 | 15.38 ± 0.17 | Tr | |
3 | 51.1 | DEHP | 3104 | 73.63 ± 1.71 | 66.75 ± 1.08 | 76.22 ± 1.18 | |
13 | 54 | methyl stearate | 2245 | 6.94 ± 2.32 | tr | tr | |
SnAE | |||||||
16 | 5.3 | butyl acetate | 1078 | 79.24 ± 1.48 | 21.91 ± 2.4 | 58.62 ± 0.17 | |
17 | 7.8 | butyl acrylate | 1189 | 10.07 ± 2.08 | 3.21 ± 1.44 | 8.25 ± 0.01 | |
18 | 13.2 | diacetone alcohol | 1352 | Tr | tr | 5.91 ± 0.01 | |
19 | 14.5 | 2-butoxyethanol | 1406 | 10.69 ± 0.6 | 1.7 ± 0.03 | 8.08 ± 0.3 | |
20 | 43.2 | undecanal | 1609 | Tr | 13.87 ± 0.24 | tr | |
21 | 46.1 | myristic acid | 1660 | Tr | 18.76 ± 0.31 | tr | |
2 | 50 | palmitic acid | 2713 | Tr | 40.54 ± 0.73 | 19.13 ± 0.14 | |
SnA | |||||||
16 | 5.3 | butyl acetate | 1078 | 48.61 ± 0.97 | 55.75 ± 0.1 | 63.77 ± 0.74 | |
17 | 7.8 | butyl acrylate | 1189 | 7.55 ± 0.15 | 7.21 ± 0.06 | 10.35 ± 0.28 | |
18 | 13.2 | diacetone alcohol | 1352 | 11.02 ± 0.75 | 21.79 ± 0.04 | 9.89 ± 0.55 | |
19 | 14.4 | 2-butoxyethanol | 1406 | 4.29 ± 0.89 | 6.95 ± 0.04 | 7.85 ± 0.17 | |
22 | 16.2 | acetic acid | 1460 | 28.53 ± 0.53 | 8.3 ± 0.07 | 8.14 ± 0.17 | |
SnM | |||||||
16 | 5.3 | butyl acetate | 1078 | 76.71 ± 0.1 | 77.93 ± 1.52 | 79.15 ± 1.38 | |
23 | 6.8 | m-xylene | 1146 | 2.95 ± 0.52 | 1.22 ± 0.09 | tr | |
17 | 7.7 | butyl acrylate | 1189 | 10.79 ± 0.25 | 10.54 ± 0.74 | 10.77 ± 0.35 | |
19 | 14.4 | 2-butoxyethanol | 1406 | 9.54 ± 0.37 | 10.31 ± 0.68 | 10.08 ± 1.03 | |
UnS | |||||||
16 | 5.3 | butyl acetate | 1078 | 57.58 ± 0.23 | 49.14 ± 2.33 | 3.99 ± 0.53 | |
17 | 7.7 | butyl acrylate | 1189 | 5.42 ± 0.73 | 6.01 ± 0.28 | tr | |
24 | 11.4 | hydroxyacetone | 1318 | Tr | tr | 4.28 ± 0.29 | |
19 | 14.4 | 2-butoxyethanol | 1406 | 5.7 ± 0.35 | 5.14 ± 0.2 | tr | |
22 | 16.2 | acetic acid | 1460 | 31.29 ± 0.86 | 39.71 ± 1.85 | 42.96 ± 1.55 | |
25 | 16.5 | 3-furaldehyde | 1454 | Tr | tr | 2.92 ± 0.44 | |
26 | 18 | oxalic acid | 1127 | Tr | tr | 5.08 ± 0.69 | |
27 | 22.3 | furfuryl alcohol | 1669 | Tr | tr | 2.76 ± 0.26 | |
28 | 33.3 | dihydroxyacetone | 2075 | Tr | Tr | 8.87 ± 0.46 | |
29 | 37.4 | trehalose | 2816 | Tr | Tr | 13.57 ± 0.51 | |
30 | 42.4 | 1, 3, 5-benzenetriol | 2692 | Tr | Tr | 15.57 ± 0.6 | |
Note: * Retention Time, IKCAL = Retention Index relative n-alkanes (C6 to C26) on a HP-Innowax 19091N133I capillary column. tr = below detection rate. |
In extracts isolated by non-polar solvents of F. splendens, there are high concentrations of DEHP, these values may be related to the results shown in Table 1 and Figure 2, where the high toxicity (LC50 < 100 ppm) of these extracts can be observed. The presence of this molecule in ocotillo stems may be attributed to two phenomena: the first suggests that F. splendens has the capacity for phytoabsorption and compound accumulation because there have been reports of the presence of phthalate esters in nature, such as in sediments, natural waters, soils, and plants. There is evidence of the use of some plants such as L. salicaria that are grown in the course of water in rivers and streams for phytoremediation of wastewaters; however, plants such as Pongamia pinnata, Raphanus salivas, T. Aestivum, Triticum aestivum, Brassica napus and Zea mays that are generally grown for food have also proven to absorb these phthalate esters due to their capacity of receiving nutrients and toxic substances through the roots and the green parts above grade [26,27]. Second, there is the possibility of molecule synthesis and accumulation, as done by Brown algae called sargassum (U. pinnatifida, L. japonica and Ulva sp) which are capable of synthetizing and storing phthalate esters [26,27,28]. Another compound identified in this study was butyl acrylate, commonly used in the elaboration of paints, coatings, sealants, and adhesives, and a blooming compound in plants, attractive for some insects such as Apolygus lucorum [29]. Being an attractant molecule, plants synthetize it when they are in a reproductive state. This can be confirmed with the information in Figure 2 which shows an increase of this compound in polar extracts (SnA, SnAE and SnM) obtained in F. splendens blooming seasons (fall and spring) and a decrease in the winter season, in agreement with the data obtained by Moraru et al. [29], and Yan et al. [30]. Killingbech [4] and Monreal et al. [9] mention this compound, together with the anthocyanins identified in their experiments, maybe the attractant compounds for hummingbirds and carpenter bees to migrate North through the arid regions during migration and cause fertilization of ocotillo seeds in the spring season [4,9]. It has also been discovered that the accumulation of anthocyanins and the metabolites described above is stimulated by diverse environmental stresses, such as high-intensity ultraviolet light, to which ocotillo is exposed when temperatures rise [12]. Three abundant vegetable metabolites are butyl acetate palmitic acid and myristic acid; the former is a characteristic component in apple's aroma [29], the latter two are components of palm, cocoa, and coconut oils in percentages up to 40%, in addition to being skin and eye irritants [31], in some animals developed tumors, a possible indication that exposure to F. splendens extracts may generate the same clinical manifestations [32]. However, these compounds have also been identified in other consumable plants showing phytotherapeutic properties with antioxidant, antidiabetic, and anticarcinogenic effects [10]. The presence of volatile compounds was also determined; those with the highest phytotherapeutic interest are undecanol, pentadecanol, and 2-butoxyethanol. Undecanal has also been identified in Citrus reticulata essential oil; its high antimicrobial potential has been reported [33] as well as its possible pesticide and herbicide effects; Pérez-Leal et al. [11] determined up to 53% of growth inhibition for M persicae and Lactuca sativa. Pentadecanol, is a compound found in vegetable waxes and its relevance in phytotherapy lies in the reduction of plasmatic cholesterol in humans, as well as octacosanol and triacontanol, allowing a reduction of low-density lipoproteins (LDL) and increase in high-density lipoproteins (HDL); this explains the use of ocotillo to reduce cholesterol and fatty acid levels. Trehalose or micose, also identified in this study, is a compound involved in anhydrosis. Although in plants the determined concentration was lower, the levels increase with exposure to abiotic stresses as suggested by Marcorro-Gallardo et al. [34], as shown in Table 2 (U3S = 653.4 ± 34.5). This molecule could be involved in the capacity of F. splendens and other plants to withstand prolonged periods without humidity and to resume cell activity without any damage from extreme conditions; it also regulates efficient water use and stoma movement [34,35].
Extract | LC50 | Classification |
H1C | 467.8 ±41.9 | Moderately toxic |
H2C | 440.3 ± 8.9 | Moderately toxic |
H3C | 924.6 ± 46.7 | Moderately toxic |
S1H | ND | Extremely toxic |
S2H | ND | Extremely toxic |
S3H | 92.6 ± 6.2 | Toxic |
S1Cl | 5.3 ± 0.0 | Extremely toxic |
S2Cl | ND | Extremely toxic |
S3Cl | 15.5 ± 10.5 | Toxic |
S1AE | 49.2 ± 4.9 | Toxic |
S2AE | ND | Extremely toxic |
S3AE | 30.2 ± 3.3 | Toxic |
S1A | 986.3 ± 123.2 | Moderately toxic |
S2A | 701.3 ± 37.1 | Moderately toxic |
S3A | 1324.0 ± 61.0 | Nontoxic |
S1M | 1274.7 ± 116.8 | Nontoxic |
S2M | 659.3 ±115.5 | Moderately toxic |
S3M | 876.9 ± 105.7 | Moderately toxic |
U1S | 523.4 ± 28.8 | Moderately toxic |
U2S | 519.2 ±58.8 | Moderately toxic |
U3S | 653.4 ± 34.5 | Moderately toxic |
Note: ND (Not defined for LC50 as is very close to 0). |
Figure 3 shows the results obtained in the determination of median lethal concentration (LC50) of the F. splendens stem extracts using the crustacean Artemia salina. K2Cr2O7 5% was used as positive control and saline solution in DMSO was used as a negative control. In this section, a factorial analysis was also conducted, which showed that both the extraction technique with different solvents, the season of the year of harvesting of the ocotillo plants, and their interaction affected the concentration at which the population of A. salina appeared in 50%.
With the results obtained it is possible to classify the extracts according to their level of toxicity, showing that the polarity of the molecule is related to the concentration required to kill 50% of the Artemia population.
According to the factor analysis and as can be seen in the previous figure. The extracts that appeared with a higher LD50 (less toxic) were those obtained with acetone, and methanol using the soxhlet technique and those obtained by the hydrodistillation technique (floral water) and by the ultrasound technique. On the contrary, the extracts that presented the highest toxicity were those obtained with ethyl acetate, dichloromethane, and hexane as extraction solvents. Similarly, concerning the season of the year, the extracts obtained in the spring season presented less toxicity while those obtained in winter presented greater toxicity. The polar extracts (SnM, UnS and SnA) show a higher LC50, with values ranging from 500 ppm (nontoxic) to over 1000 ppm (innocuous). These results are similar to the report of Serrano-Gallardo et al. [35], who obtained LC50 values close to 1000 ppm for methanolic extracts, in contrast with extracts isolated by non-polar solvents (SnH, SnCL, and SnAE) which recorded values under 100 ppm (toxic). This toxicity may be attributed to the presence of DEHA and DEHP in such extracts.
Probit analysis revealed that extracts isolated by non-polar solvents of F. splendens are not suitable to be used in phytotherapy because their high content of DEHA and DEHP and the LC50 values under 100 ppm make them extremely toxic. However, in polar extracts, there are molecules such as 2-butoxyethanol, pentadecanol, and undecanal that allow justify the use of ocotillo in traditional medicine, because these molecules have proven insecticide capacity, antimicrobial, and cholesterol-reducing activity
The authors wish to thank the Mexican Council of Science and Technology (CONACYT) and the Autonomous University of Chihuahua (UACH) for the support and infrastructure.
The authors declare no conflict of interest.
[1] |
L. M. F. Merlo, J. W. Pepper, B. J. Reid, C. C. Maley, Cancer as an evolutionary and ecological process, Nat. Rev. Cancer, 6 (2006), 924–935. doi: 10.1038/nrc2013
![]() |
[2] | A. Jemal, F. Bray, M. M. Center, Global cancer statistics, Ca. Cancer. J. Clin., 61 (2011), 69–90. |
[3] |
R. R. Sarkar, S. Banerjee, Cancer self remission and tumor stability-a stochastic approach, Math. Biosci., 196 (2005), 65–81. doi: 10.1016/j.mbs.2005.04.001
![]() |
[4] | K. Subhas, J. J. Nieto, Mathematical modeling of tumor-immune competitive system, considering the role of time delay, Appl. Math. Comput., 340 (2019), 180–205. |
[5] |
D. Kirschner, J. Panetta, Modelling immunotherapy of the tumor-immune interaction, J. Math. Biol., 37 (1998), 235–252. doi: 10.1007/s002850050127
![]() |
[6] | L. G. de Pillis, A. Radunskaya, The dynamics of an optimally controlled tumor model: A case study, Math. Comput. Model., 37 (2003), 1221–1244. |
[7] |
N. Tsur, Y. Kogana, M. Rehmc, Z. Agur, Response of patients with melanoma to immune checkpoint blockade-insights gleaned from analysis of a new mathematical mechanistic model, J. Theor. Biol., 485 (2020), 110033. doi: 10.1016/j.jtbi.2019.110033
![]() |
[8] |
M. A. Owen, J. A. Sherratt, Mathematical modelling macrophage dynamics in tumors, Math. Model. Meth. Appl. Sci., 9 (1999), 513–539. doi: 10.1142/S0218202599000270
![]() |
[9] | J. L. Yu, S. R. J. Jang, A mathematical model of tumor-immune interactions with an immune checkpoint inhibitor, Appl. Math. Comput., 362 (2019), 124532. |
[10] |
R. A. Ku-Carrillo, S. E. Delgadillo, B. M. Chen-Charpentier, A mathematical model for the effect of obesity on cancer growth and on the immune system response, Appl. Math. Model., 40 (2016), 4908–4920. doi: 10.1016/j.apm.2015.12.018
![]() |
[11] |
N. Bellomo, L. Preziosi, Modelling and mathematical problems related to tumor evolution and its interaction with the immune system, Math. Comput. Model., 32 (2000), 413–452. doi: 10.1016/S0895-7177(00)00143-6
![]() |
[12] |
V. A. Kuznetsov, I. A. Makalkin, M. A. Taylor, A. S. Perelson, Nonlinear dynamics of immunogenic tumors: Parameter estimation and global bifurcation analysis, Bull. Math. Biol., 56 (1994), 295–321. doi: 10.1016/S0092-8240(05)80260-5
![]() |
[13] |
C. Letellier, F. Denis, L. A. Aguirre, What can be learned from a chaotic cancer model?, J. Theor. Biol., 322 (2013), 7–16. doi: 10.1016/j.jtbi.2013.01.003
![]() |
[14] |
A. Zazoua, W. D. Wang, Analysis of mathematical model of prostate cancer with androgen deprivation therapy, Commun. Nonlinear. Sci. Numer. Simul., 66 (2019), 41–60. doi: 10.1016/j.cnsns.2018.06.004
![]() |
[15] |
L. Y. Pang, Z. Zhao, X. Y. Song, Cost-effectiveness analysis of optimal strategy for tumor treatment, Chaos Solitons Fractals, 87 (2016), 293–301. doi: 10.1016/j.chaos.2016.03.032
![]() |
[16] |
S. Khajanchi, S. Banerjee, Quantifying the role of immunotherapeutic drug T11 target structure in progression of malignant gliomas: Mathematical modeling and dynamical perspective, Math. Biosci., 289 (2017), 69–77. doi: 10.1016/j.mbs.2017.04.006
![]() |
[17] |
S. Banerjee, S. Khajanchi, S. Chaudhur, A mathematical model to elucidate brain tumor abrogation by immunotherapy with T11 target structure, PLoS One, 10 (2015), 1–21. doi: 10.1371/journal.pone.0116884
![]() |
[18] | J. Arciero, T. Jackson, D. Kirschner, A mathematical model of tumor-immune evasion and siRNA treatment, Discret. Contin. Dyn. Syst. Ser. B., 4 (2004), 39–58. |
[19] |
L. G. depillis, A. Eladdadi, A. E. Radunskaya, Modeling cancer-immune responses to therapy, J. Pharmacokinet. Pharmacodyn., 41 (2014), 461–0478. doi: 10.1007/s10928-014-9386-9
![]() |
[20] |
M. Itik, M. U. Salamci, S. P. Banks, Optimal control of drug therapy in cancer treatment, Nonlinear Anal., 71 (2009), 1473–1486. doi: 10.1016/j.na.2009.01.214
![]() |
[21] |
X. D. Liu, Q. Z. Li, J. X. Pan, A deterministic and stochastic model for the system dynamics of tumor-immune responses to chemotherapy, Phys. A, 500 (2018), 162–176. doi: 10.1016/j.physa.2018.02.118
![]() |
[22] |
L. G. depillis, W. Gu, K. R. Fister, T. Head, K. Maples, A. Murugan, et al., Chemotherapy for tumors: An analysis of the dynamics and a study of quadratic and linear optimal controls, Math. Biosci., 209 (2007), 292–315. doi: 10.1016/j.mbs.2006.05.003
![]() |
[23] |
P. Rokhforoz, A. A. Jamshidi, N. N. Sarvestani, Adaptive robust control of cancer chemotherapy with extended Kalman filter observer, Inform. Med. Unlock., 8 (2017), 1–7. doi: 10.1016/j.imu.2017.03.002
![]() |
[24] |
L. G. de Pillis, W. Gu, A. E. Radunskaya, Mixed immunotherapy and chemotherapy of tumors: modeling, applications and biological interpretations, J. Theor. Biol., 238 (2006), 841–862. doi: 10.1016/j.jtbi.2005.06.037
![]() |
[25] |
R. Eftimie, J. J. Gillard, D. A. Cantrell, Mathematical models for immunology: Current state of the art and future research directions, Bull. Math. Biol., 78 (2016), 2091–2134. doi: 10.1007/s11538-016-0214-9
![]() |
[26] |
M. Villasana, A. Radunskaya, A delay differential equation model for tumor growth, J. Math. Biol., 47 (2003), 270–294. doi: 10.1007/s00285-003-0211-0
![]() |
[27] |
Y. Radouane, Hopf bifurcation in differential equations with delay for tumor-immune system competition model, SIAM J. Appl. Math., 67 (2007), 1693–1703. doi: 10.1137/060657947
![]() |
[28] | F. A. Rihan, D. H. Abdel Rahman, S. Lakshmanan, A. S. Alkhajeh, A time delay model of tumour-immune system interactions: Global dynamics, parameter estimation, sensitivity analysis, Appl. Math. Comput., 232 (2014), 606–623. |
[29] |
M. Yu, Y. P. Dong, Y. Takeuchi, Dual role of delay effects in a tumour-immune system, J. Biol. Dyn., 11 (2017), 334–347. doi: 10.1080/17513758.2016.1231347
![]() |
[30] | S. Khajanchi, S. Banerjee, Stability and bifurcation analysis of delay induced tumor immune interaction model, Appl. Math. Comput., 248 (2014), 652–671. |
[31] |
S. Khajanchi, Bifurcation analysis of a delayed mathematical model for tumor growth, Chaos Solitons Fractals, 77 (2015), 264–276. doi: 10.1016/j.chaos.2015.06.001
![]() |
[32] |
S. Khajanchi, S. Banerjee, Influence of multiple delays in brain tumor and immune system interaction with T11 target structure as a potent stimulator, Math. Biosci., 302 (2018), 116–130. doi: 10.1016/j.mbs.2018.06.001
![]() |
[33] |
M. J. Piotrowska, M. Bodnar, Influence of distributed delays on the dynamics of a generalized immune system cancerous cells interactions model, Commun. Nonlinear. Sci. Numer. Simul., 54 (2018), 389–415. doi: 10.1016/j.cnsns.2017.06.003
![]() |
[34] |
S. Banerjee, R. R. Sarkar, Delay-induced model for tumor-immune interaction and control of malignant tumor growth, BioSystems, 91 (2008), 268–288. doi: 10.1016/j.biosystems.2007.10.002
![]() |
[35] |
A. E. Gohary, Chaos and optimal control of cancer self-remission and tumor system steady states, Chaos Solitons Fractals, 37 (2008), 1305–1316. doi: 10.1016/j.chaos.2006.10.060
![]() |
[36] |
H. Mayer, K. Zaenker, U. an der Heiden, A basic mathematical model of the immune response, Chaos, 5 (1995), 155–161. doi: 10.1063/1.166098
![]() |
[37] |
H. M. Byrne, The effect of time delay on the dynamics of avascular tumor growth, Math. Biosci., 144 (1997), 83–117. doi: 10.1016/S0025-5564(97)00023-0
![]() |
[38] | P. Bi, S. G. Ruan, Bifurcations in delay differential equations and applications to tumor and immune system interaction models, SIAM J. Appl. Dyn., 12 (2014), 1847–1888. |
[39] | S. G. Ruan, Nonlinear dynamics in tumor-immune system interaction models with delays, Discret. Contin. Dyn. Syst. Ser. B., 26 (2021), 541–602. |
[40] |
F. A. Rihan, Sensitivity analysis of dynamic systems with time lags, J. Comput. Appl. Math., 151 (2003), 445–462. doi: 10.1016/S0377-0427(02)00659-3
![]() |
[41] |
K. Gopalsamy, Nonoscillation in a delay-logistic equation, Quart. Appl. Math., 43 (1985), 189–197. doi: 10.1090/qam/793526
![]() |
[42] |
D. Mukherjee, P. C. Bhakta, A. B. Roy, Uniform persistence in Kolmogorov models with convex growth functions, Nonlinear Anal., 34 (1998), 427–432. doi: 10.1016/S0362-546X(97)00605-6
![]() |
[43] | J. J. Wei, S. G. Ruan, Stability and bifurcation in a neural network model with two delays, Phys. D, 130 (1998), 255–272. |
[44] | B. D. Hassard, N. D. Kazarinoff, Y. H. Wan, Theory and Application of Hopf Bifurcation, London Mathematical Society Lecture Note Series, Cambridge University Press, 1981. |
1. | Irma E. Dávila-Rangel, Ana V. Charles-Rodríguez, Julio C. López-Romero, María L. Flores-López, Plants from Arid and Semi-Arid Zones of Mexico Used to Treat Respiratory Diseases: A Review, 2024, 13, 2223-7747, 792, 10.3390/plants13060792 |
No. | RT* (min) | Compounds | Relative peak area (%)b | ||||
IKCAL | Fall | Winter | Spring | ||||
HnC | |||||||
1 | 43.7 | DEHA | 1892 | 4.92± 0.62 | 95.88 ± 0.2 | tr | |
2 | 45.4 | palmitic acid | 2280 | Tr | tr | 12.72 ± 8.47 | |
3 | 51.5 | DEHP | 3104 | 95.08 ± 0.62 | 95.88 ± 0.2 | 86.1 ± 11.62 | |
SnH | |||||||
4 | 37.3 | tridecane | 1366 | Tr | tr- | 7.4 ± 1.8 | |
5 | 39 | pentadecanol | 1776 | Tr | 13.95 ± 2.25 | tr | |
6 | 41.3 | pentadecane, 2-methyl- | 1565 | Tr | tr | 8.48 ± 2.85 | |
7 | 41.7 | heptadecanol | 2287 | 1.61 ± 1.39 | 5.23 ± 0.74 | 22.39 ± 1.94 | |
8 | 43 | 4-methylpentadecane | 1559 | Tr | tr | 10.35 ± 2.32 | |
1 | 43.5 | DEHA | 1892 | Tr | 3.16 ± 1.63 | tr | |
9 | 44.5 | diethylene glycol hexyl ether | 1363 | Tr | 1.12 ± 1.47 | tr | |
2 | 45.6 | palmitic acid | 2280 | Tr | 1.62 ± 0.82 | 16.42 ± 4.06 | |
10 | 47.9 | 2-methylundecanoic acid | 1506 | Tr | 7.51 ± 4.35 | tr | |
11 | 48.6 | octacosane | 461 | 0.53 ± 0.83 | tr | tr | |
12 | 49.4 | eicosane | 2056 | 2.25 ± 1.19 | tr | tr | |
3 | 51.5 | DEHP | 3104 | 88.49 ± 2.16 | 67.41 ± 3.6 | 34.95 ± 8.24 | |
13 | 54 | methyl stearate | 2245 | 1.72 ±0.89 | tr | tr | |
SnCL | |||||||
14 | 23 | 2-Ethylhexyl glycidyl ether | 1363 | Tr | 3.66 ± 0.58 | 8.17 ± 2.02 | |
15 | 38.8 | octadecanol | 1846 | Tr | 9.93 ± 0.11 | tr | |
7 | 39.1 | heptadecanol | 2286 | 10.2 ± 0.36 | tr | 6.8 ± 0.36 | |
2 | 45.1 | palmitic acid | 2280 | Tr | 4.28 ± 0.44 | 8.81 ± 2.84 | |
12 | 49.3 | eicosane | 2056 | 9.24 ± 0.33 | 15.38 ± 0.17 | Tr | |
3 | 51.1 | DEHP | 3104 | 73.63 ± 1.71 | 66.75 ± 1.08 | 76.22 ± 1.18 | |
13 | 54 | methyl stearate | 2245 | 6.94 ± 2.32 | tr | tr | |
SnAE | |||||||
16 | 5.3 | butyl acetate | 1078 | 79.24 ± 1.48 | 21.91 ± 2.4 | 58.62 ± 0.17 | |
17 | 7.8 | butyl acrylate | 1189 | 10.07 ± 2.08 | 3.21 ± 1.44 | 8.25 ± 0.01 | |
18 | 13.2 | diacetone alcohol | 1352 | Tr | tr | 5.91 ± 0.01 | |
19 | 14.5 | 2-butoxyethanol | 1406 | 10.69 ± 0.6 | 1.7 ± 0.03 | 8.08 ± 0.3 | |
20 | 43.2 | undecanal | 1609 | Tr | 13.87 ± 0.24 | tr | |
21 | 46.1 | myristic acid | 1660 | Tr | 18.76 ± 0.31 | tr | |
2 | 50 | palmitic acid | 2713 | Tr | 40.54 ± 0.73 | 19.13 ± 0.14 | |
SnA | |||||||
16 | 5.3 | butyl acetate | 1078 | 48.61 ± 0.97 | 55.75 ± 0.1 | 63.77 ± 0.74 | |
17 | 7.8 | butyl acrylate | 1189 | 7.55 ± 0.15 | 7.21 ± 0.06 | 10.35 ± 0.28 | |
18 | 13.2 | diacetone alcohol | 1352 | 11.02 ± 0.75 | 21.79 ± 0.04 | 9.89 ± 0.55 | |
19 | 14.4 | 2-butoxyethanol | 1406 | 4.29 ± 0.89 | 6.95 ± 0.04 | 7.85 ± 0.17 | |
22 | 16.2 | acetic acid | 1460 | 28.53 ± 0.53 | 8.3 ± 0.07 | 8.14 ± 0.17 | |
SnM | |||||||
16 | 5.3 | butyl acetate | 1078 | 76.71 ± 0.1 | 77.93 ± 1.52 | 79.15 ± 1.38 | |
23 | 6.8 | m-xylene | 1146 | 2.95 ± 0.52 | 1.22 ± 0.09 | tr | |
17 | 7.7 | butyl acrylate | 1189 | 10.79 ± 0.25 | 10.54 ± 0.74 | 10.77 ± 0.35 | |
19 | 14.4 | 2-butoxyethanol | 1406 | 9.54 ± 0.37 | 10.31 ± 0.68 | 10.08 ± 1.03 | |
UnS | |||||||
16 | 5.3 | butyl acetate | 1078 | 57.58 ± 0.23 | 49.14 ± 2.33 | 3.99 ± 0.53 | |
17 | 7.7 | butyl acrylate | 1189 | 5.42 ± 0.73 | 6.01 ± 0.28 | tr | |
24 | 11.4 | hydroxyacetone | 1318 | Tr | tr | 4.28 ± 0.29 | |
19 | 14.4 | 2-butoxyethanol | 1406 | 5.7 ± 0.35 | 5.14 ± 0.2 | tr | |
22 | 16.2 | acetic acid | 1460 | 31.29 ± 0.86 | 39.71 ± 1.85 | 42.96 ± 1.55 | |
25 | 16.5 | 3-furaldehyde | 1454 | Tr | tr | 2.92 ± 0.44 | |
26 | 18 | oxalic acid | 1127 | Tr | tr | 5.08 ± 0.69 | |
27 | 22.3 | furfuryl alcohol | 1669 | Tr | tr | 2.76 ± 0.26 | |
28 | 33.3 | dihydroxyacetone | 2075 | Tr | Tr | 8.87 ± 0.46 | |
29 | 37.4 | trehalose | 2816 | Tr | Tr | 13.57 ± 0.51 | |
30 | 42.4 | 1, 3, 5-benzenetriol | 2692 | Tr | Tr | 15.57 ± 0.6 | |
Note: * Retention Time, IKCAL = Retention Index relative n-alkanes (C6 to C26) on a HP-Innowax 19091N133I capillary column. tr = below detection rate. |
Extract | LC50 | Classification |
H1C | 467.8 ±41.9 | Moderately toxic |
H2C | 440.3 ± 8.9 | Moderately toxic |
H3C | 924.6 ± 46.7 | Moderately toxic |
S1H | ND | Extremely toxic |
S2H | ND | Extremely toxic |
S3H | 92.6 ± 6.2 | Toxic |
S1Cl | 5.3 ± 0.0 | Extremely toxic |
S2Cl | ND | Extremely toxic |
S3Cl | 15.5 ± 10.5 | Toxic |
S1AE | 49.2 ± 4.9 | Toxic |
S2AE | ND | Extremely toxic |
S3AE | 30.2 ± 3.3 | Toxic |
S1A | 986.3 ± 123.2 | Moderately toxic |
S2A | 701.3 ± 37.1 | Moderately toxic |
S3A | 1324.0 ± 61.0 | Nontoxic |
S1M | 1274.7 ± 116.8 | Nontoxic |
S2M | 659.3 ±115.5 | Moderately toxic |
S3M | 876.9 ± 105.7 | Moderately toxic |
U1S | 523.4 ± 28.8 | Moderately toxic |
U2S | 519.2 ±58.8 | Moderately toxic |
U3S | 653.4 ± 34.5 | Moderately toxic |
Note: ND (Not defined for LC50 as is very close to 0). |
No. | RT* (min) | Compounds | Relative peak area (%)b | ||||
IKCAL | Fall | Winter | Spring | ||||
HnC | |||||||
1 | 43.7 | DEHA | 1892 | 4.92± 0.62 | 95.88 ± 0.2 | tr | |
2 | 45.4 | palmitic acid | 2280 | Tr | tr | 12.72 ± 8.47 | |
3 | 51.5 | DEHP | 3104 | 95.08 ± 0.62 | 95.88 ± 0.2 | 86.1 ± 11.62 | |
SnH | |||||||
4 | 37.3 | tridecane | 1366 | Tr | tr- | 7.4 ± 1.8 | |
5 | 39 | pentadecanol | 1776 | Tr | 13.95 ± 2.25 | tr | |
6 | 41.3 | pentadecane, 2-methyl- | 1565 | Tr | tr | 8.48 ± 2.85 | |
7 | 41.7 | heptadecanol | 2287 | 1.61 ± 1.39 | 5.23 ± 0.74 | 22.39 ± 1.94 | |
8 | 43 | 4-methylpentadecane | 1559 | Tr | tr | 10.35 ± 2.32 | |
1 | 43.5 | DEHA | 1892 | Tr | 3.16 ± 1.63 | tr | |
9 | 44.5 | diethylene glycol hexyl ether | 1363 | Tr | 1.12 ± 1.47 | tr | |
2 | 45.6 | palmitic acid | 2280 | Tr | 1.62 ± 0.82 | 16.42 ± 4.06 | |
10 | 47.9 | 2-methylundecanoic acid | 1506 | Tr | 7.51 ± 4.35 | tr | |
11 | 48.6 | octacosane | 461 | 0.53 ± 0.83 | tr | tr | |
12 | 49.4 | eicosane | 2056 | 2.25 ± 1.19 | tr | tr | |
3 | 51.5 | DEHP | 3104 | 88.49 ± 2.16 | 67.41 ± 3.6 | 34.95 ± 8.24 | |
13 | 54 | methyl stearate | 2245 | 1.72 ±0.89 | tr | tr | |
SnCL | |||||||
14 | 23 | 2-Ethylhexyl glycidyl ether | 1363 | Tr | 3.66 ± 0.58 | 8.17 ± 2.02 | |
15 | 38.8 | octadecanol | 1846 | Tr | 9.93 ± 0.11 | tr | |
7 | 39.1 | heptadecanol | 2286 | 10.2 ± 0.36 | tr | 6.8 ± 0.36 | |
2 | 45.1 | palmitic acid | 2280 | Tr | 4.28 ± 0.44 | 8.81 ± 2.84 | |
12 | 49.3 | eicosane | 2056 | 9.24 ± 0.33 | 15.38 ± 0.17 | Tr | |
3 | 51.1 | DEHP | 3104 | 73.63 ± 1.71 | 66.75 ± 1.08 | 76.22 ± 1.18 | |
13 | 54 | methyl stearate | 2245 | 6.94 ± 2.32 | tr | tr | |
SnAE | |||||||
16 | 5.3 | butyl acetate | 1078 | 79.24 ± 1.48 | 21.91 ± 2.4 | 58.62 ± 0.17 | |
17 | 7.8 | butyl acrylate | 1189 | 10.07 ± 2.08 | 3.21 ± 1.44 | 8.25 ± 0.01 | |
18 | 13.2 | diacetone alcohol | 1352 | Tr | tr | 5.91 ± 0.01 | |
19 | 14.5 | 2-butoxyethanol | 1406 | 10.69 ± 0.6 | 1.7 ± 0.03 | 8.08 ± 0.3 | |
20 | 43.2 | undecanal | 1609 | Tr | 13.87 ± 0.24 | tr | |
21 | 46.1 | myristic acid | 1660 | Tr | 18.76 ± 0.31 | tr | |
2 | 50 | palmitic acid | 2713 | Tr | 40.54 ± 0.73 | 19.13 ± 0.14 | |
SnA | |||||||
16 | 5.3 | butyl acetate | 1078 | 48.61 ± 0.97 | 55.75 ± 0.1 | 63.77 ± 0.74 | |
17 | 7.8 | butyl acrylate | 1189 | 7.55 ± 0.15 | 7.21 ± 0.06 | 10.35 ± 0.28 | |
18 | 13.2 | diacetone alcohol | 1352 | 11.02 ± 0.75 | 21.79 ± 0.04 | 9.89 ± 0.55 | |
19 | 14.4 | 2-butoxyethanol | 1406 | 4.29 ± 0.89 | 6.95 ± 0.04 | 7.85 ± 0.17 | |
22 | 16.2 | acetic acid | 1460 | 28.53 ± 0.53 | 8.3 ± 0.07 | 8.14 ± 0.17 | |
SnM | |||||||
16 | 5.3 | butyl acetate | 1078 | 76.71 ± 0.1 | 77.93 ± 1.52 | 79.15 ± 1.38 | |
23 | 6.8 | m-xylene | 1146 | 2.95 ± 0.52 | 1.22 ± 0.09 | tr | |
17 | 7.7 | butyl acrylate | 1189 | 10.79 ± 0.25 | 10.54 ± 0.74 | 10.77 ± 0.35 | |
19 | 14.4 | 2-butoxyethanol | 1406 | 9.54 ± 0.37 | 10.31 ± 0.68 | 10.08 ± 1.03 | |
UnS | |||||||
16 | 5.3 | butyl acetate | 1078 | 57.58 ± 0.23 | 49.14 ± 2.33 | 3.99 ± 0.53 | |
17 | 7.7 | butyl acrylate | 1189 | 5.42 ± 0.73 | 6.01 ± 0.28 | tr | |
24 | 11.4 | hydroxyacetone | 1318 | Tr | tr | 4.28 ± 0.29 | |
19 | 14.4 | 2-butoxyethanol | 1406 | 5.7 ± 0.35 | 5.14 ± 0.2 | tr | |
22 | 16.2 | acetic acid | 1460 | 31.29 ± 0.86 | 39.71 ± 1.85 | 42.96 ± 1.55 | |
25 | 16.5 | 3-furaldehyde | 1454 | Tr | tr | 2.92 ± 0.44 | |
26 | 18 | oxalic acid | 1127 | Tr | tr | 5.08 ± 0.69 | |
27 | 22.3 | furfuryl alcohol | 1669 | Tr | tr | 2.76 ± 0.26 | |
28 | 33.3 | dihydroxyacetone | 2075 | Tr | Tr | 8.87 ± 0.46 | |
29 | 37.4 | trehalose | 2816 | Tr | Tr | 13.57 ± 0.51 | |
30 | 42.4 | 1, 3, 5-benzenetriol | 2692 | Tr | Tr | 15.57 ± 0.6 | |
Note: * Retention Time, IKCAL = Retention Index relative n-alkanes (C6 to C26) on a HP-Innowax 19091N133I capillary column. tr = below detection rate. |
Extract | LC50 | Classification |
H1C | 467.8 ±41.9 | Moderately toxic |
H2C | 440.3 ± 8.9 | Moderately toxic |
H3C | 924.6 ± 46.7 | Moderately toxic |
S1H | ND | Extremely toxic |
S2H | ND | Extremely toxic |
S3H | 92.6 ± 6.2 | Toxic |
S1Cl | 5.3 ± 0.0 | Extremely toxic |
S2Cl | ND | Extremely toxic |
S3Cl | 15.5 ± 10.5 | Toxic |
S1AE | 49.2 ± 4.9 | Toxic |
S2AE | ND | Extremely toxic |
S3AE | 30.2 ± 3.3 | Toxic |
S1A | 986.3 ± 123.2 | Moderately toxic |
S2A | 701.3 ± 37.1 | Moderately toxic |
S3A | 1324.0 ± 61.0 | Nontoxic |
S1M | 1274.7 ± 116.8 | Nontoxic |
S2M | 659.3 ±115.5 | Moderately toxic |
S3M | 876.9 ± 105.7 | Moderately toxic |
U1S | 523.4 ± 28.8 | Moderately toxic |
U2S | 519.2 ±58.8 | Moderately toxic |
U3S | 653.4 ± 34.5 | Moderately toxic |
Note: ND (Not defined for LC50 as is very close to 0). |