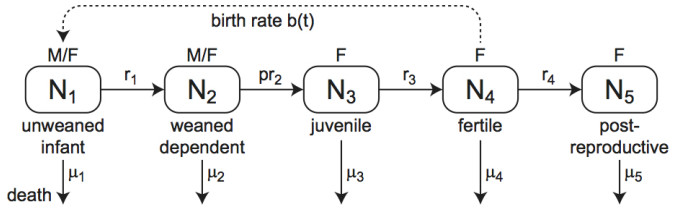
Although females in human and the great ape populations reach the end of fertility at similar ages (approximately 45 years), female humans often live well beyond their post-fertile years, while female primates typically die before or shortly after the end of fertility. The grandmother hypothesis proposes that the care-giving role provided by post-fertile females contributed to the evolution of longevity in human populations. When post-fertile females provide care for weaned infants, mothers are able to have their next baby sooner without compromising the chances of survival of their previous offspring. Thus, the post-menopausal longevity that is unique to human populations may be an evolutionary adaptation. In this work, we construct, simulate, and analyze an ordinary differential equations mathematical model to study the grandmother hypothesis. Our model describes the passage of the individuals of a population through five life stages in the cases with and without grandmothering. We demonstrate via numerical simulation of the mathematical model that grandmothering care is sufficient to significantly increase adult life expectancy. We also investigate the relationship between the number of weaned infants that a post-fertile female can care for at a given time and the steady-state age distributions of a population.
Citation: Dana Paquin, David Kato, Peter Kim. A mathematical model for the effects of grandmothering on human longevity[J]. Mathematical Biosciences and Engineering, 2020, 17(4): 3175-3189. doi: 10.3934/mbe.2020180
[1] | Karen R. Ríos-Soto, Baojun Song, Carlos Castillo-Chavez . Epidemic spread of influenza viruses: The impact of transient populations on disease dynamics. Mathematical Biosciences and Engineering, 2011, 8(1): 199-222. doi: 10.3934/mbe.2011.8.199 |
[2] | H. Thomas Banks, W. Clayton Thompson, Cristina Peligero, Sandra Giest, Jordi Argilaguet, Andreas Meyerhans . A division-dependent compartmental model for computing cell numbers in CFSE-based lymphocyte proliferation assays. Mathematical Biosciences and Engineering, 2012, 9(4): 699-736. doi: 10.3934/mbe.2012.9.699 |
[3] | Süleyman Cengizci, Aslıhan Dursun Cengizci, Ömür Uğur . A mathematical model for human-to-human transmission of COVID-19: a case study for Turkey's data. Mathematical Biosciences and Engineering, 2021, 18(6): 9787-9805. doi: 10.3934/mbe.2021480 |
[4] | Najat Ziyadi . A male-female mathematical model of human papillomavirus (HPV) in African American population. Mathematical Biosciences and Engineering, 2017, 14(1): 339-358. doi: 10.3934/mbe.2017022 |
[5] | Thanh Nam Nguyen, Jean Clairambault, Thierry Jaffredo, Benoît Perthame, Delphine Salort . Adaptive dynamics of hematopoietic stem cells and their supporting stroma: a model and mathematical analysis. Mathematical Biosciences and Engineering, 2019, 16(5): 4818-4845. doi: 10.3934/mbe.2019243 |
[6] | Andrei Korobeinikov, Conor Dempsey . A continuous phenotype space model of RNA virus evolution within a host. Mathematical Biosciences and Engineering, 2014, 11(4): 919-927. doi: 10.3934/mbe.2014.11.919 |
[7] | Jun Chen, Gloria DeGrandi-Hoffman, Vardayani Ratti, Yun Kang . Review on mathematical modeling of honeybee population dynamics. Mathematical Biosciences and Engineering, 2021, 18(6): 9606-9650. doi: 10.3934/mbe.2021471 |
[8] | Dominik Wodarz . Computational modeling approaches to studying the dynamics of oncolytic viruses. Mathematical Biosciences and Engineering, 2013, 10(3): 939-957. doi: 10.3934/mbe.2013.10.939 |
[9] | Maria Vittoria Barbarossa, Christina Kuttler, Jonathan Zinsl . Delay equations modeling the effects of phase-specific drugs and immunotherapy on proliferating tumor cells. Mathematical Biosciences and Engineering, 2012, 9(2): 241-257. doi: 10.3934/mbe.2012.9.241 |
[10] | Azmy S. Ackleh, Mark L. Delcambre, Karyn L. Sutton, Don G. Ennis . A structured model for the spread of Mycobacterium marinum: Foundations for a numerical approximation scheme. Mathematical Biosciences and Engineering, 2014, 11(4): 679-721. doi: 10.3934/mbe.2014.11.679 |
Although females in human and the great ape populations reach the end of fertility at similar ages (approximately 45 years), female humans often live well beyond their post-fertile years, while female primates typically die before or shortly after the end of fertility. The grandmother hypothesis proposes that the care-giving role provided by post-fertile females contributed to the evolution of longevity in human populations. When post-fertile females provide care for weaned infants, mothers are able to have their next baby sooner without compromising the chances of survival of their previous offspring. Thus, the post-menopausal longevity that is unique to human populations may be an evolutionary adaptation. In this work, we construct, simulate, and analyze an ordinary differential equations mathematical model to study the grandmother hypothesis. Our model describes the passage of the individuals of a population through five life stages in the cases with and without grandmothering. We demonstrate via numerical simulation of the mathematical model that grandmothering care is sufficient to significantly increase adult life expectancy. We also investigate the relationship between the number of weaned infants that a post-fertile female can care for at a given time and the steady-state age distributions of a population.
The grandmother hypothesis is a theory in evolutionary anthropology that stipulates that the care-giving role played by human grandmothers is, at least in part, responsible for the unique post-fertile longevity that occurs in human populations. Although the age of the end of female fertility is similar for humans and the great apes, long postmemopausal survival is a characteristic that is unique to modern human populations [1,2]. Indeed, it is common in other primate populations for females to die before or just after reaching the age of terminal fertility [3,4]. Post-menopausal women comprise about one-third of the number of adult females in human hunter-gatherer populations, and women often live for decades after the total loss of fertility [5,6], but only about 5% of the number of adult females in chimpanzee and baboon populations are post-menopausal [3,7]. The grandmother hypothesis, first discussed by Kristen Hawkes and colleagues following their study of the Hazda, a hunter-grather society in Tanzania, proposes that older, infertile females play a unique evolutionary role in human populations by caring and provisioning for young juveniles that cannot acquire food effectively for themselves, thus allowing earlier weaning from the juveniles' mothers and shorter intervals between births [8,9].
Proponents of the grandmother hypothesis argue that, as a result of these fitness benefits due to grandmothering, natural selection processes would have favored longer longevity, slowing senescence and increasing the number of years that human females live beyond the age of fertility. Although researchers have often found evidence of such contributions by grandmothers in human populations [10], the grandmother hypothesis has only recently been studied formally from a mathematical perspective. In Kachel et al. [11], the authors constructed an evolutionary agent-based simulation to study the effects of grandmothering on life spans, and concluded that, while grandmothering may not explain human-like longevity, it may have played an important role in shaping other aspects of human life history, as compared to great ape populations, such as age at first birth and shorter reproductive periods for females. In Kim et al. [12], the authors used that model as the foundation to construct a probabilistic agent-based model to simulate the effects of grandmothering. In that work, the authors began with a model population with an ape-like equilibrium life span of 16–27 years, and demonstrated that grandmothering effects alone are sufficient to propel the model population into lifespans in the range of human longevity (approximately 43 years) in less than sixty thousand years. In [13], the authors present a modified agent-based probabilistic model, using the Gillespie algorithm of determining times to next events, and demonstrated that populations with grandmothering may shift from an equilibrium with life spans consistent with those of the great apes to life spans consistent with human-like averages, though the transition takes longer than in their previous models in [12]. In [14], the authors build on the agent-based model of [13] and present a two-sex partial differential equations model based on the grandmother hypothesis. In that work, similarly to [13], the authors show that grandmothering enables populations to transition from an equilibrium with great ape-like longevities to human-like longevities. In this work, we develop, simulate, and analyze an ordinary differential equations model based on the agent-based model of Kim et al. in [12], and we use the mathematical model to study the effects of grandmothering on the life expectancy of a simulated population.
In this section, we describe an ordinary differential equations model for the passage of the individuals of a population through five life stages. The populations of the different stages at time t are denoted as follows:
● N1(t), unweaned infant;
● N2(t), weaned dependents;
● N3(t), female (non-fertile) juveniles;
● N4(t), fertile females;
● N5(t), post-reproductive females.
Individuals progress through the various stages in the following way. New unweaned infants are born into the population at rate b(t). Unweaned infants progress to become unweaned weaned dependents at rate r1, and die at rate μ1. Weaned dependents progress to become female juveniles at rate pr2 and die at rate μ2, where p represents the probability of being female. We do not consider males after they become independent in this model, as we assume that they do not contribute to grandmothering. Female juveniles progress to become fertile females at rate r3 and die at rate μ3. Fertile females progress to become post-reproductive females at rate r4 and die at rate μ4. Post-reproductive females die at rate μ5. The ODE system corresponding to the diagram is given below, and the model is illustrated schematically in Figure 1.
dN1dt=b(t)−(r1+μ1)N1,dN2dt=r1N1−(r2+μ2)N2,dN3dt=pr2N2−(r3+μ3)N3,dN4dt=r3N3−(r4+μ4)N4,dN5dt=r4N4−μ5N5. |
For simplicity, we assume that mortality rates are constant, and set μi=1L for i=1,2,3,4,5, where L is the expected adult life span from any age, including the beginning of adulthood. In other words, L is the inverse of the annual mortality rate and does not change with age. We refer to L as the expected adult life span (or adult life expectancy), and our main interest is in studying the relationship between grandmothering effects and L. In particular, we are interested in determining mathematically whether or not grandmothering effects could have significantly increased L for human populations as compared to the great ape populations.
Instead of setting values for the rates of transition ri, we will consider the expected lengths of time spent in each life stage, τi. We let ri=1τi for i=1,2,3,4. The parameters τi are scaled in terms of L as follows. The average duration in the unweaned infant stage, τ1, is 2 years. The average age at independence is L/6, so the average duration in the weaned dependent stage is τ2=L6−τ1. Females reach sexual maturity at age L2.5, so the average duration in the female juvenile stage is τ3=L2.5+τ1−L6. Female fertility ends at age 45, so the average duration in the female fertile state is τ4=45−L2.5−τ1. These parameter scalings are taken from [12], in which the parameters are based on empirical scaling patterns [15] and demographic data on apes, primarily chimpanzees [7,16,17,18].
These scalings describe a basic longevity trade-off that works in the following way. As L increases, greater expected life expectancy increases the probability that a given female will live throughout her fertile years, and therefore give birth to more offspring. However, since the age at independence is L/6, females with greater longevity have offspring that take longer to reach independence, and the time interval between offspring thus increases [15]. There should be an optimal value of the expected adult life expectancy L that produces the highest net population growth rate, and we shall investigate how grandmothering effects this optimal life expectancy.
To determine the birth rate b(t), we consider the cases without grandmothering help and with grandmothering help separately. Without grandmothering help, we assume that every unweaned infant and weaned dependent is attached to a mother, who cannot give birth to another child until the dependent progresses to become an independent juvenile. In the case without grandmothering, we thus set
b(t)=β(N4−(N1+N2)), |
where β is the conception rate for females without dependent children.
Next, we determine the birth rate b(t) in the case with grandmothering help. We assume that all post-reproductive females, N5 can offer help as grandmothers, and that grandmothers can care for c weaned dependents at a time. This grandmothering model allows fertile mothers to have another child sooner than without help, without risking the survival of previous offspring. With grandmothering, fertile mothers need only care for unweaned dependents until they are weaned, at which point eligible grandmothers can take over. In the case with grandmothering, we thus set
b(t)=β(N4−(N1+max{(N2−c⋅N5),0})), |
where β is the conception rate for females without dependent children and c is the number of weaned juveniles that a grandmother can take care of at once. Note that this model produces a more generalized form of grandmothering care than literal grandmothering, as post-fertile females eligible to grandmother can assume care of any weaned juvenile in the population, not only maternal grandchildren.
We thus have an ODE model that describes the progression of individuals through the five life stages in the cases with and without grandmothering help. Numerical values of the model parameters are summarized in Table 1. We will use this ODE model to study the question of whether grandmother effects can increase longevity. In particular, we will numerically simulate the ODE model in the cases with and without grandmothering to determine the adult life expectancy L that results in the optimal long-term population growth rate.
Parameter | Description | Value |
β | Birth rate | 1 |
p | Probability of being female | 1/2 |
L | Life expectancy | varies from 15 to 70 |
τ1 | Average duration in unweaned state | 2 |
τ2 | Average duration in dependent state | max{L6−τ1,0} |
τ3 | Average duration in juvenile state | L2.5+τ1−L6 |
τ4 | Average duration in fertile state | max{45−L2.5−τ1,0} |
μ1 | Death rate of unweaned population | 1/L |
μ2 | Death rate of dependent population | 1/L |
μ3 | Death rate of juvenile population | 1/L |
μ4 | Death rate of fertile population | 1/L |
μ5 | Death rate of post-reproductive population | 1/L |
c | Number of weaned dependents that an eligible | varies from 1 to 3 |
grandmother can take care of at one time |
We simulated the ODE model with the parameters described in Table 1 in MATLAB using the ode45 six-stage, fifth-order, Runge-Kutta solver. The commented MATLAB code is freely available upon request. We conducted simulations with adult life expectancies L ranging from 10 to 60 years, and for c (the number of weaned juveniles that an eligible grandmother can take care of at once) ranging from 1 to 3. For starting populations, we assumed that the system begins with 1000 individuals, all of whom have the same expected adult life expectancy L. For convenience, we specified that the initial 1000 individuals are distributed uniformly throughout the five life stages. From this distribution, the population converges to a steady-state age distribution within several generations. For each simulation, we numerically calculated the net growth rate r of the population at a steady-state age distribution. For each value of c, there is exactly one optimal net growth rate r of the population corresponding to one optimal value of adult life expectancy L, and there is a unique steady state population distribution.
To demonstrate the model convergence to a steady state population distribution, in Figure 2 we plot the total population vs. time (upper left), the logarithm of the total population vs. time (upper right) to, and the proportion of the total population that each of the sub-populations N1, N2, N3, N4, and N5 comprises vs. time (lower). These initial plots are the result of simulating the mathematical model in the case without grandmothering (c=0). As stated previously, we conducted simulations for a range of values of L; to illustrate the general results, we show here the plots for L=20.
In Figure 3, we plot the optimal adult life expectancy L vs. the net growth rate r for the cases without grandmothering (c=0), grandmothering with c=1, grandmothering with c=1.5, and grandmothering with c=2. Local maxima on these plots correspond to local maxima of reproductive output for females. The upper left plot in Figure 3 shows that without grandmothering, the optimal net growth rate of the population is 0.29%, and it occurs at L=14.5 years. Without grandmothering, the net growth rate decreases past L=14.5 years until it falls below 0 at L=25 years, at which point the population cannot sustain itself. With grandmothering with c=1 (i.e. each grandmother can take care of 1 weaned dependent at a time), the growth rate rises until L=20.5 years, reaching a maximum growth rate of 2.09%, and stays above 0 for all values of L that fall within the range of human life expectancy. Thus, even if each grandmother can take care of only 1 dependent at a time, the effect of grandmothering is to increase the optimal expected adult life expectancy by approximately 6 years. With grandmothering with c=1.5, the optimal life expectancy occurs at L=24.5 years, an increase of 10 years compared to the case without grandmothering, and with c=2, the optimal life expectancy occurs at L=31 years. In both of these cases, the growth rate stays above 0 for all values of L that fall within the range of human life expectancy. These results are summarized in Table 2.
Case | Optimal Adult Life Expectancy | Percent Increase (compared to the case without grandmothering) |
Without grandmothering | 14.5 years | N/A |
Grandmothering with c=1 | 20.5 years | 43.1% |
Grandmothering with c=1.5 | 24.5 years | 69.0% |
Grandmothering with c=2 | 31 years | 113.8% |
For each value of c, the number of weaned infants that a grandmother can care for at a given time, there is exactly one maximum growth rate r corresponding to one optimal value of L, and a unique steady-state population distribution that follows from these values. In Figure 4, we plot the optimal adult life expectancy L (left) and the optimal net growth rate r (right) vs. c. We observe that L initially increases as c increases, reaching a maximum of 31.1 years when c=1.5, and we observe that r initially increases as c increases, reaching a maximum of 2.45% when c=1.5. Thus, the model predicts that the optimal adult life expectancy and corresponding optimal net growth rate occur when each grandmother can care for c=1.5 weaned infants at a given time. In fact, we observe that there is a plateau for both L and r that starts at c=1.5, so our model indicates that there is no fitness benefit for grandmothers caring for more than 1.5 infants each.
Next, we consider the dependence of the optimal value of L on β, the conception rate for females without dependent children. The motivation for this is as follows. Consider the total population
N=5∑i=1Ni. |
Then we can show that
dNdt=b(t)−NL+6(p−1)N2L−12, |
where b(t) is the birth rate (and depends on β, c, N1, N2, N4, and N5). Thus, in addition to exploring the relationship between L and c, as in Figure 4, we consider the relationship between the optimal value of L and β. In Figure 5, we plot the the net growth rate r vs. the optimal adult life expectancy L for β=1 (upper left), β=1.5 (upper right), β=2 (lower left), and β=2.5 (lower right). All of these plots are with c=1.5, as our model suggests that (with β=1), the optimal life expectancy and growth rates occur when each grandmother can care for c=1.5 infants at a time.
In Table 3, we illustrate the optimal life expectancy L obtained for different values of the conception rate β, all in the case of grandmothering with c=1.5.
Conception Rate β | Optimal Adult Life Expectancy L | Optimal Growth Rate r |
1 | 31 years | 2.44% per year |
1.5 | 24 years | 3.02% per year |
2 | 21 years | 3.39% per year |
2.5 | 20 years | 3.65 % per year |
In Figure 6, we plot the long-term population distribution of the groups N1,N2,N3,N4,N5 vs optimal adult life expectancy L with c=0, i.e. no grandmothering (left), and c=1 (right). The plots in Figure 6 illustrate the effects of grandmothering on the long-term distributions of the population demographics as a function of L. Recall that when c=0 (corresponding to no grandmothering), the optimal value of L is 14.5 years, and when c=1, the optimal value of L is 20.5 years. The corresponding points on the population distribution curves are marked in Figure 6 with an X. In particular, we observe that when c=1, the sub-populations N2 and N5 comprise an identical proportion of the population at the optimal value L=20.5 years. This can be explained from the perspective of the mathematical model by noting that each member of sub-population N5 can care for exactly one member of sub-population N2 when c=1, so the optimal growth rate r (and thus optimal corresponding adult life expectancy L) should occur when N2 and N5 are equal to one another.
In particular, since each grandmother can care for c weaned infants at a given time, we expect that the long-term steady state population distributions corresponding to the optimal value of L for each value of c should be such that N2 and c⋅N5 are equal to one another. For each value of c between 0 and 2.5, we compute the optimal adult life expectancy L, and then determine the steady-state age distributions, and compute the proportion of the total population comprised by each of the sub-populatios N1,N2,N3,N4,N5. In Figure 7 we plot the proportions comprised by N2 and c⋅N5 vs. c. These numerical observations and results suggest that the optimal steady state growth rate r indeed occurs when N2 and c⋅N5 are equivalent. Comparing Figure 7 and Figure 4, we observe that the optimal L increases most rapidly with respect to c over the interval of c-values for which the steady-state proportions comprised by N2 and c⋅N5 overlap. We also observe that increasing the care-giving capacity of grandmothers (i.e. increasing c) increases optimal life spans only insofar as grandmothers are fully utilized. Specifically, when c⋅N5 exceeds N2, optimal life expectancy no longer increases as c increases. Again, we observe that the model does not predict that there will be a fitness benefit for grandmothers caring for more than 1.5 infants at a time.
In this work, we have developed a continuous dynamical systems mathematical model to study the effects of grandmothering on human longevity. Our model demonstrates that even weak grandmothering effects in which each member of the post-fertile female population can care for at most 1 weaned infant at a time are sufficient to increase adult life expectacy by 43% as compared to the case without grandmothering. Without grandmothering, our model predicts that populations have a positive net growth rate only when adult life expectancy is less than 25 years, and with grandmothering, populations have a positive net growth rate for all adult life expectancy values within the current range of human life expectancies. Our model also predicts that grandmother effects have an impact on the long-term steady-state age distributions of a population. In particular, the optimal steady-state age distributions corresponding to optimum life expectancy are such that the number of weaned infants and c (the number of weaned infants that a grandmother can take care of at a given time) times the number of grandmothers are equal.
Additionally, our model suggests that there is no fitness benefit for grandmothers caring for more than c=1.5 infants at a time, and in future work, we plan to explore modifications of this model that may favor grandmother care for larger numbers of events. In this model, though, we have demonstrated that, broadly, the care provided by grandmothers, even for a limited number of infants, may play a role in increased human life expectancy. We observe also that this differential equations model results in apparent discontinuities in the first derivative in the plots of growth rate r vs. adult life expectancy L at the absolute maximum values (L=20.5 for c=1 and L=24.5 for c=1.5), and we plan to investigate this phenomena in more detail in future work. We do not observe these discontinuities for c=1.5 or greater. These discontinuities in the first derivative do not seem to be a numerical artifact related to the simulation technique.
As our model builds on previous mathematical models of the grandmother hypothesis, most notably Kachel et al. [11], Kim et al. [12,13], and Chan et al. [14], we note here the similarities and differences between our model and these other mathematical models.
Our model is based on an ordinary differential equations model, and we do not distinguish between males and females in the model. The model of Kachel et al. [11] is an evolutionary agent-based model. The model of Kim et al. [12] is an agent-based model, implemented as a deterministic difference equations model. The more sophisticated agent-based model of Kim et al. [13] is a probabilistic agent-based model, and is an improvement over the authors' previous agent-based model in that the model is probabilistic rather than deterministic, and events can happen at any time, rather than at discrete intervals. Finally, the model of Chan et al. [14] is a partial differential equations model that is similar in many ways in terms of assumptions to the model of Kim et al. [13], but allows for evolution in age at last birth, restricts grandmothers to only support their daughters, and uses a more advanced mortality assumption (see below).
In the models of Kachel et al. [11] and Chan et al. [14], the authors allow grandmothers to provide help for their daughters and matrilineal grandchildren only. In the two agent-based models of Kim et al. [12,13], and in this differential equations model, the assumption is that female who are eligible to grandmother can take care of any weaned dependent in the population, not only direct matrilineal descendants.
One of the key differences in the different models is the assumption on how to mathematically model mortality in the population, which has significant impacts on the ultimate results obtained by simulating the models. Our ordinary differential equations model assumes a constant mortality rate, whereas the evolutionary agent-based model of Kachel et al. [11] uses Siler's competing hazards model to model male and female mortality rates as a sum of three components of mortality (immature, residual, and senescent). In both [12] and [13], the authors also assume a constant mortality rate. In Chan et al. [14], the authors model mortality as a rate that depends on both age and longevity, where there are three distinct stages (early, middle, and late). This is a more realistic mortality function than the constant mortality assumption used in this work and in the two models of Kim et al.
The mathematical models of grandmothering discussed here also differ in their ultimate conclusions regarding the possible effects of grandmothering on human longevity. In this work, our ordinary differential equations model suggests that the effects of grandmothering may contribute to increasing human life expectancy by approximately 43%. The authors of Kachel et al. [11] conclude that human longevity is not likely to be an evolutionary consequence of grandmothering, but their model does indicate that the help provided by grandmothers may have played a role in humans having a later age at first birth, as compared to great ape populations. In their agent-based model [12], Kim et al. conclude that grandmother effects may results in doubling human life spans in a span of less than sixty thousand years. In their refined probabilistic agent-based model in [13], Kim et al. again conclude that the fitness benefit provided by grandmothering may lead to increased longevity in human populations, though the time of transition between life expectancy consistent with great apes and life expectancy consistent with human populations is significantly longer in the probabilistic model [13] than in the deterministic model [12]. Using the partial differential equations model of Chan et al. [14], the authors conclude that grandmothering effects contribute to the transition from great ape to human-like longevities in approximately 30,000 years, but they have an insignificant impact on the equilibrium value of the average adult lifespan.
In future work, we plan to investigate in more detail the mathematical relationship between c⋅N5 and N2. We also plan to investigate the effects of incorporating an “age of frailty”, i.e. a maximum age after which grandmothers are no longer able to provide care. Additionally, our model only includes grandmothering effects provided by post-fertile females, so another area of future investigation will include modeling caregiving help provided by fathers [19], and older siblings [20]. We also note that another area of future research will be to incorporate additional evolutionary changes in our mathematical model, including larger brain sizes [21], increased skill learning [22,23], and changing social contexts. Our work here focuses solely on quantifying the effects of grandmothering on the evolution of increased longevity. There are a range of other possible hypotheses for increased human longevity, and the grandmother hypothesis is still subject to discussion and evaluation. Our model simply demonstrates that grandmothering may be, at least in part, responsible for propelling life expectancy from one equilibrium point to another. We note also that the results described in this work are, of course, dependent on the validity of this particular model, and additional work is needed to evaluate this model in the broader context of evolutionary theory. To further evaluate our ordinary differential equations model, we plan, in future work, to conduct a full sensitivity analysis on the parameters involved in the model, so that we may more fully evaluate the role of each parameter in our conclusions here. Additionally, in future work, we plan to analyze the analytic solution of the model in more detail.
DP and DK would like to thank California Polytechnic State University for supporting this work.
There are no conflicts of interest.
[1] | S. L. Robson, C. P. van Schaik, K. Hawkes, The derived features of human life history, in The Evolution of Human Life History (eds. K. Hawkes and R. R. Paine), School of American Research Press, (2006), 17-44. |
[2] |
G. C. Williams, Pleiotropy, natural selection, and the evolution of senescence, Evolution, 11 (1957), 398-411. doi: 10.1111/j.1558-5646.1957.tb02911.x
![]() |
[3] |
M. Pavelka, L. Fedigan, Reproductive termination in female Japanese monkeys: A comparative lifehistory perspective, Am. J. Phys. Anthropol., 109 (1999), 455-464. doi: 10.1002/(SICI)1096-8644(199908)109:4<455::AID-AJPA3>3.0.CO;2-Z
![]() |
[4] |
C. Packer, M. Tatar, A. Collins, Reproductive cessation in female mammals, Nature, 392 (1998), 807-811. doi: 10.1038/33910
![]() |
[5] | K. Hawkes, J. F. O'Connell, N. G. Blurton Jones, The evolution of human life histories: Primate tradeoffs, grandmothering socioecology, and the fossil record, in Primate Life Histories and Socioecology (eds. P. Kappeler and M. Pereira), University of Chicago Press, (2003), 204-227. |
[6] | N. Howell, Demography of the Dobe!Kung, Academic Press, New York, 1979. |
[7] |
K. Hill, C. Boesch, J. Goodall, A. Pusey, J. Williams, R. Wrangham, Mortality rates among wild chimpanzees, J. Human Evol., 40 (2001), 437-450. doi: 10.1006/jhev.2001.0469
![]() |
[8] | K. Hawkes, J. F. O'Connell, N. G. B. Jones, Hardworking Hadza grandmothers, in Comparative Socioecology: The Behavioral Ecology of Humans and Other Mammals (eds. K. hawkes, J. F. O'Connell and N. G. B. Jones), UK: Blackwell Scientific Publications, Oxford, (1989), 341-366. |
[9] |
K. Hawkes, Grandmothers and the evolution of human longevity, Am. J. Hum. Biol., 15 (2003), 380-400. doi: 10.1002/ajhb.10156
![]() |
[10] |
R. Sear, D. Coall, How much does family matter? Cooperative breeding and the demongraphic transition, Popul. Dev. Rev., 37 (2011), 81-112. doi: 10.1111/j.1728-4457.2011.00379.x
![]() |
[11] |
A. F. Kachel, L. S. Premo, J. J. Hublin, Grandmothering and natural selection, Proc. R. Soc. B: Biol. Sci., 278 (2011), 384-391. doi: 10.1098/rspb.2010.1247
![]() |
[12] |
P. S. Kim, J. E. Coxworth, K. Hawkes, Increased longevity evolves from grandmothering, Proc. R. Soc. B: Biol. Sci., 279 (2012), 4880-4884. doi: 10.1098/rspb.2012.1751
![]() |
[13] |
P. S. Kim, J. S. McQueen, J. E. Coxworth, K. Hawkes, Grandmothering drives the evolution of longevity in a probabilistic model, J. Theor. Biol., 353 (2014), 84-94. doi: 10.1016/j.jtbi.2014.03.011
![]() |
[14] |
M. H. Chan, K. Hawkes, P. Kim, Evolution of longevity, age at last birth and sexual conflict with grandmothering, J. Theor. Biol., 393 (2016), 145-157. doi: 10.1016/j.jtbi.2015.12.014
![]() |
[15] | E. L. Charnov, Life History Invariants: Some Explorations of Symmetry in Evolutionary Ecology, Oxford University Press, Oxford, 1993. |
[16] |
B. Dyke, T. B. Gage, P. L. Alford, B. Swenson, S. Williams-Blangero, Model life table for captive chimpanzees, Am. J. Primatol., 37 (1995), 25-37. doi: 10.1002/ajp.1350370104
![]() |
[17] |
C. Boesch, C. Bolé, N. Eckhardt, H. Boesch, Altruism in forest chimpanzees: The case of adoption, PLoS ONE, 5 (2010), e8901. doi: 10.1371/journal.pone.0008901
![]() |
[18] |
M. E. Thompson, M. N. Muller, R. W. Wrangham, The energetics of lactation and the return to fecundity in wild chimpanzees, Behav. Ecol., 23 (2012), 1234-1241. doi: 10.1093/beheco/ars107
![]() |
[19] | P. Grey, K. G. Anderson, Fatherhood: Evolution and Human Paternal Behavior, Harvard University Press, Cambridge, 2010. |
[20] |
K. Kramer, The evolution of human parental care and recruitment of juvenile help, Trends Ecol. Evol., 26 (2011), 533-540. doi: 10.1016/j.tree.2011.06.002
![]() |
[21] |
K. Isler, C. P. van Schaik, The expensive brain: A framework for explaining evolutionary changes in brain size, J. Hum. Evol., 57 (2009), 392-400. doi: 10.1016/j.jhevol.2009.04.009
![]() |
[22] |
H. Kaplan, K. Hill, J. Lancaster, A. M. Hurtado, A theory of human life history evolution: Diet, intelligence, and longevity, Evol. Anthropol., 9 (2000), 156-185. doi: 10.1002/1520-6505(2000)9:4<156::AID-EVAN5>3.0.CO;2-7
![]() |
[23] |
H. Kaplan, M. Gurven, J. Winking, P. Hooper, J. Stieglitz, Learning, menopause and the human adaptive complex, Ann. NY Acad. Sci., 1204 (2010), 30-42. doi: 10.1111/j.1749-6632.2010.05528.x
![]() |
1. | Hideki Innan, Daniel Vaiman, Reiner A. Veitia, Predictable increase in female reproductive window, 2021, 0265-9247, 2000233, 10.1002/bies.202000233 | |
2. | Aja Watkins, Reevaluating the grandmother hypothesis, 2021, 43, 0391-9714, 10.1007/s40656-021-00455-x | |
3. | Dave Dalton, How evolutionary biology can explain why human and a few marine mammal females are the only ones that are menopausal, 2022, 543, 00225193, 111123, 10.1016/j.jtbi.2022.111123 | |
4. | Robert G. Bednarik, The Domestication of Humans, 2023, 3, 2673-8392, 947, 10.3390/encyclopedia3030067 |
Parameter | Description | Value |
β | Birth rate | 1 |
p | Probability of being female | 1/2 |
L | Life expectancy | varies from 15 to 70 |
τ1 | Average duration in unweaned state | 2 |
τ2 | Average duration in dependent state | max{L6−τ1,0} |
τ3 | Average duration in juvenile state | L2.5+τ1−L6 |
τ4 | Average duration in fertile state | max{45−L2.5−τ1,0} |
μ1 | Death rate of unweaned population | 1/L |
μ2 | Death rate of dependent population | 1/L |
μ3 | Death rate of juvenile population | 1/L |
μ4 | Death rate of fertile population | 1/L |
μ5 | Death rate of post-reproductive population | 1/L |
c | Number of weaned dependents that an eligible | varies from 1 to 3 |
grandmother can take care of at one time |
Case | Optimal Adult Life Expectancy | Percent Increase (compared to the case without grandmothering) |
Without grandmothering | 14.5 years | N/A |
Grandmothering with c=1 | 20.5 years | 43.1% |
Grandmothering with c=1.5 | 24.5 years | 69.0% |
Grandmothering with c=2 | 31 years | 113.8% |
Conception Rate β | Optimal Adult Life Expectancy L | Optimal Growth Rate r |
1 | 31 years | 2.44% per year |
1.5 | 24 years | 3.02% per year |
2 | 21 years | 3.39% per year |
2.5 | 20 years | 3.65 % per year |
Parameter | Description | Value |
β | Birth rate | 1 |
p | Probability of being female | 1/2 |
L | Life expectancy | varies from 15 to 70 |
τ1 | Average duration in unweaned state | 2 |
τ2 | Average duration in dependent state | max{L6−τ1,0} |
τ3 | Average duration in juvenile state | L2.5+τ1−L6 |
τ4 | Average duration in fertile state | max{45−L2.5−τ1,0} |
μ1 | Death rate of unweaned population | 1/L |
μ2 | Death rate of dependent population | 1/L |
μ3 | Death rate of juvenile population | 1/L |
μ4 | Death rate of fertile population | 1/L |
μ5 | Death rate of post-reproductive population | 1/L |
c | Number of weaned dependents that an eligible | varies from 1 to 3 |
grandmother can take care of at one time |
Case | Optimal Adult Life Expectancy | Percent Increase (compared to the case without grandmothering) |
Without grandmothering | 14.5 years | N/A |
Grandmothering with c=1 | 20.5 years | 43.1% |
Grandmothering with c=1.5 | 24.5 years | 69.0% |
Grandmothering with c=2 | 31 years | 113.8% |
Conception Rate β | Optimal Adult Life Expectancy L | Optimal Growth Rate r |
1 | 31 years | 2.44% per year |
1.5 | 24 years | 3.02% per year |
2 | 21 years | 3.39% per year |
2.5 | 20 years | 3.65 % per year |