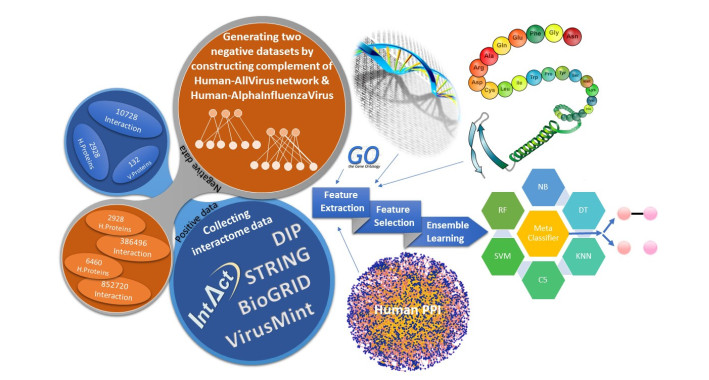
More than ten million deaths make influenza virus one of the deadliest of history. About half a million sever illnesses are annually reported consequent of influenza. Influenza is a parasite which needs the host cellular machinery to replicate its genome. To reach the host, viral proteins need to interact with the host proteins. Therefore, identification of host-virus protein interaction network (HVIN) is one of the crucial steps in treating viral diseases. Being expensive, time-consuming and laborious of HVIN experimental identification, force the researches to use computational methods instead of experimental ones to obtain a better understanding of HVIN. In this study, several features are extracted from physicochemical properties of amino acids, combined with different centralities of human protein-protein interaction network (HPPIN) to predict protein-protein interactions between human proteins and Alphainfluenzavirus proteins (HI-PPIs). Ensemble learning methods were used to predict such PPIs. Our model reached 0.93 accuracy, 0.91 sensitivity and 0.95 specificity. Moreover, a database including 694522 new PPIs was constructed by prediction results of the model. Further analysis showed that HPPIN centralities, gene ontology semantic similarity and conjoint triad of virus proteins are the most important features to predict HI-PPIs.
Citation: Babak Khorsand, Abdorreza Savadi, Javad Zahiri, Mahmoud Naghibzadeh. Alpha influenza virus infiltration prediction using virus-human protein-protein interaction network[J]. Mathematical Biosciences and Engineering, 2020, 17(4): 3109-3129. doi: 10.3934/mbe.2020176
[1] | Jinmiao Song, Shengwei Tian, Long Yu, Qimeng Yang, Qiguo Dai, Yuanxu Wang, Weidong Wu, Xiaodong Duan . RLF-LPI: An ensemble learning framework using sequence information for predicting lncRNA-protein interaction based on AE-ResLSTM and fuzzy decision. Mathematical Biosciences and Engineering, 2022, 19(5): 4749-4764. doi: 10.3934/mbe.2022222 |
[2] | Peter Hinow, Edward A. Rietman, Sara Ibrahim Omar, Jack A. Tuszyński . Algebraic and topological indices of molecular pathway networks in human cancers. Mathematical Biosciences and Engineering, 2015, 12(6): 1289-1302. doi: 10.3934/mbe.2015.12.1289 |
[3] | Linlu Song, Shangbo Ning, Jinxuan Hou, Yunjie Zhao . Performance of protein-ligand docking with CDK4/6 inhibitors: a case study. Mathematical Biosciences and Engineering, 2021, 18(1): 456-470. doi: 10.3934/mbe.2021025 |
[4] | Hanyu Zhao, Chao Che, Bo Jin, Xiaopeng Wei . A viral protein identifying framework based on temporal convolutional network. Mathematical Biosciences and Engineering, 2019, 16(3): 1709-1717. doi: 10.3934/mbe.2019081 |
[5] | Yutong Man, Guangming Liu, Kuo Yang, Xuezhong Zhou . SNFM: A semi-supervised NMF algorithm for detecting biological functional modules. Mathematical Biosciences and Engineering, 2019, 16(4): 1933-1948. doi: 10.3934/mbe.2019094 |
[6] | Zhihong Zhang, Yingchun Luo, Meiping Jiang, Dongjie Wu, Wang Zhang, Wei Yan, Bihai Zhao . An efficient strategy for identifying essential proteins based on homology, subcellular location and protein-protein interaction information. Mathematical Biosciences and Engineering, 2022, 19(6): 6331-6343. doi: 10.3934/mbe.2022296 |
[7] | Yongyin Han, Maolin Liu, Zhixiao Wang . Key protein identification by integrating protein complex information and multi-biological features. Mathematical Biosciences and Engineering, 2023, 20(10): 18191-18206. doi: 10.3934/mbe.2023808 |
[8] | Sathyanarayanan Gopalakrishnan, Swaminathan Venkatraman . Prediction of influential proteins and enzymes of certain diseases using a directed unimodular hypergraph. Mathematical Biosciences and Engineering, 2024, 21(1): 325-345. doi: 10.3934/mbe.2024015 |
[9] | Lu Yuan, Yuming Ma, Yihui Liu . Protein secondary structure prediction based on Wasserstein generative adversarial networks and temporal convolutional networks with convolutional block attention modules. Mathematical Biosciences and Engineering, 2023, 20(2): 2203-2218. doi: 10.3934/mbe.2023102 |
[10] | Jiahui Wen, Haitao Gan, Zhi Yang, Ran Zhou, Jing Zhao, Zhiwei Ye . Mutual-DTI: A mutual interaction feature-based neural network for drug-target protein interaction prediction. Mathematical Biosciences and Engineering, 2023, 20(6): 10610-10625. doi: 10.3934/mbe.2023469 |
More than ten million deaths make influenza virus one of the deadliest of history. About half a million sever illnesses are annually reported consequent of influenza. Influenza is a parasite which needs the host cellular machinery to replicate its genome. To reach the host, viral proteins need to interact with the host proteins. Therefore, identification of host-virus protein interaction network (HVIN) is one of the crucial steps in treating viral diseases. Being expensive, time-consuming and laborious of HVIN experimental identification, force the researches to use computational methods instead of experimental ones to obtain a better understanding of HVIN. In this study, several features are extracted from physicochemical properties of amino acids, combined with different centralities of human protein-protein interaction network (HPPIN) to predict protein-protein interactions between human proteins and Alphainfluenzavirus proteins (HI-PPIs). Ensemble learning methods were used to predict such PPIs. Our model reached 0.93 accuracy, 0.91 sensitivity and 0.95 specificity. Moreover, a database including 694522 new PPIs was constructed by prediction results of the model. Further analysis showed that HPPIN centralities, gene ontology semantic similarity and conjoint triad of virus proteins are the most important features to predict HI-PPIs.
Influenza is the major cause of medically-attended acute respiratory diseases [1,2], and as an infectious disease it is caused by the influenza virus [3] which has three types: A, B, and C. The most significant human influenza pathogens are Alphainfluenza viruses (IAV), which can be further classified into subtypes by combining one of the 16 hemagglutinin (HA: H1– H16) with one of the 9 neuraminidase (NA: N1–N9) surface antigens [4]. Generally, most influenza viruses (e.g., subtypes H5N1, H9N2, H7N7, and H7N3) are avian and have low pathogenicity due to being inefficient at binding to sialic acid receptors of human upper airways [4]. However, some like H7N9, broke out in China and caused 44 deaths [5], cross species from poultry [6] due to the mutations in their HA proteins which enabled them to bind to human-like receptors.
Influenza A can cause major outbreaks and pandemics[7,8]. An estimated three to five million cases of severe illnesses and about 250,000 to 500,000 deaths are reported annually. Once the human population has low immunity against newly emerged influenza sequences, a pandemic happens [9,10].
Three influenza pandemics arose in the 20th century: Spanish influenza in 1918, Asian influenza in 1958, and Hong Kong influenza in 1968, each of which caused more than a million deaths [11]. All the seasonal influenza A epidemics from 1968 to 2009 were dominated by A/H3N2 virus variants produced by antigenic drift [12,13] except A/H1N1 viruses which reappeared in 1977. In fact, the pandemic of 1918 was caused by an H1N1 IAV as well. In March and early April 2009, a new type of Influenza A (H1N1) virus which was of swine origin (S-OIV) came out in Mexico and California [14]. It caused considerable fear and several deaths worldwide. This virus was antigenically distinct from human seasonal influenza viruses. However, it was genetically related to viruses recognized to circulate in pigs. With respect to its similar swine origin, it is often known as ‘swine-origin influenza virus’ (S-OIV) A/H1N1, or pandemic influenza A (H1N1) 2009 virus [15].
Viruses are parasites which need host cellular machinery for their genome replication. For reaching the host, viral proteins need to interact with host proteins. Therefore, identification of host-virus protein-protein interaction network (HVIN) can help to predict the behavior of that virus and lead to design antiviral drugs.
There are many experimental methods for detecting host-virus protein-protein interaction (HV-PPI) such as co-immunoprecipitation [16], bimolecular fluorescence complementation [17], label transfer and yeast two-hybrid. All of these methods are expensive, time-consuming and laborious. So, a series of computational methods have been proposed in recent years to predict HV-PPIs.
While Sprinzak [18] applied sequence-signature pairs, Kim [19] and Ng [20] used protein domain profiles and Yu [21] used sequence homology in order to predict HV-PPIs. Zhang [22] took advantage of decision trees in predicting co-complexed protein pairs using genomic and proteomic data integration. For predicting HV-PPIs via genomic data, Jansen [23] utilized Bayesian networks. Qi [24] used support vector machines and random forest to predict HV-PPIs. Dyer [25] achieved 516 new HV-PPIs by applying Bayesian statistics on every pair of functional domains of human–plasmodium falciparum. Zahiri [26] employed four well-established diverse learners as base classifiers of an ensemble learning model and a variety of features including pseudo amino acid composition and post translation modification to predict HV-PPI between homo sapiens and HCV (hepatitis C) proteins. Tastan [27] applied random forest as a classifier accompanied by a variety of features including co-occurrence of functional motifs and their interaction domains, tissue distributions and gene expression profiles to predict PPIs between HIV (human immunodeficiency) and human proteins. Qi [28] identified novel PPIs among HIV and human proteins by taking advantage of semi-supervised multi task learning while Barnes [29] constructed a protein-protein interaction prediction engine (PIPE) to identify new PPIs between HIV and homo sapiens proteins. Alguwaizani [30] used repeated patterns of amino acids and amino acid composition to predict PPIs among HIV, H1N1, SARS (sever acute respiratory syndrome), HCV, HPV (human papillomavirus) and human.
Zhang [31] constructed a graph by human proteins which share gene ontology terms [32] with H7N9 proteins, then calculated the shortest path of the constructed graph and sorted its proteins based on betweenness score. The top 20 proteins with the highest betweenness score interacting with H7N9 were reported as potential proteins. Eng [33] extracted the physicochemical properties of amino acids of IAV and human proteins and used them as input features of a random forest to predict PPIs between IAV and human proteins.
Nanni [34] used position specific scoring matrix of the proteins (PSSM), substitution matrix representation, wavelet image, physicochemical property response matrix, amino acid composition, pseudo amino acid composition, dipeptide, tripeptide and tetrapeptide composition to improve prediction performance up to two percent in 25 different datasets. Zacharaki [35] with extracting torsion angles density and density of amino acid distances learned a deep convolutional neural network to achieve 90% accuracy in predicting structure-based protein function.
In prediction problems, some of papers combined different classifiers to make an ensemble learning model which improve the accuracy of their model. Saha [36] used support vector machine (SVM), random forest (RF), Naïve Bayes (NB) and decision tree to build an ensemble learning method based on majority voting to improve its prediction accuracy to 90%. Emamjomeh [26] used SVM, RF, NB and multilayer perceptron (MLP) to build and ensemble learning method based on a meta learner combiner to improve its prediction accuracy to 84%. Nanni [37] used SVM, random subspace of adaboost, gaussian process classifier, deep learning and random subspace of rotation boosting to build and ensemble learning model based on normalized summation score of its classifiers to outperform the other methods.
In the present study, 1800 different features were extracted from physicochemical properties of amino acids, different centralities of HPPIN, human and virus proteins’ sequence and gene ontology to predict HI-PPIs between human and AlphaInfluenzavirus. We used KNN, cart tree, NB and SVM as the base learners and RF as a meta-classifier to build an ensemble learning method for predicting HI-PPIs using the extracted features. All these processes are depicted in Figure 1. Our ensemble learning method reached the accuracy of 93% in detecting HI-PPIs according to the experimental data.
Moreover, with running the trained model on 694522 possible HI-PPIs, a database was created which is publicly accessible at http://bioinf.modares.ac.ir/software/complexnet/Influenza.
Finally, feature importance analysis revealed that human PPI network centralities, gene ontology semantic similarity and codon usage are the most informative descriptors for HI-PPIs prediction.
We have constructed two datasets for evaluating the proposed method: A positive dataset and a negative one.
In order to construct positive HI-PPIs, all IAV interactions were extracted from Intact [38], Virus Mint [39], DIP [40], STRING [41] and BioGRID [42] databases. Then, interactions between IAV proteins and other organism proteins except with human proteins were removed. At last, 10775 interactions that annotated as ‘physical association’ or ‘direct interaction’ were considered as the positive interaction set (PS). Constructed PPI network consists of 125 IAV proteins from 10 IAV genes and 2794 HPs from 2498 genes. As it is shown in Figure 2, ten distinct influenza genes interact with 2498 distinct human genes of which non-structural gene (NS) have the most interactions.
As there isn’t any negative data in databases, selecting appropriate negative PPIs is very challenging among the PPI prediction problems [43]. HPPIN has 250038 interactions among 20050 HPs. By using CD-HIT[44], sequence similarity is calculated between 2794 HPs of HI-PPIs and 17256 other HPs of HPPIN. HPs with sequence similarity less than 20% is used as negative HPs and interaction between each of negative HPs and all IAV proteins considered as negative dataset. Final negative dataset consists of 236875 interactions.
As the number of positive and negative interactions, which is used for training the model, needs to be equal to prevent training biased classifiers, inverse random under sampling (IRUS) [45] is used to balance the benchmark dataset.
As it is shown in Figure 3, we used five different schemes to encode features for human and Influenza A proteins: Amino acid sequence-based feature, nucleotide sequence-based features, physicochemical properties, gene ontology semantic similarities and network-based features.
a. Amino acid composition (AAC): Eight categories [26] were defined by clustering twenty naive amino acids using k-means algorithm, according to 514 physicochemical index of amino acids, which exist in the AAindex database [46]. Frequency distribution of each group in the desired sequence is considered as AAC.
b. Dipeptide Composition (DC): Dipeptide composition is defined as the percentage of two consecutive amino acids which will construct a feature vector with the length of 20 × 20 × 2 = 800. But for avoiding the side effect of curse of dimensionality [47], we clustered 20 amino acids into eight groups [26] and subsequently the size of feature vector reduced to 8*8*2 = 128. DC is calculated as below:
DC(AiAj)=N(AiAj)L−1 |
which N(AiAj) is the number of occurrences of jth amino acid group followed by ith amino acid group in the sequence and L is the length of sequence.
c. Conjoint Triad (CT): Percentage of three consecutive amino acids which will construct a feature vector with length of 20 × 20 × 20 × 2 = 16000. Again, we clustered 20 amino acids into eight groups[26] and subsequently the size of feature vector reduced to 8 × 8 × 8 × 2 = 1024. TC is defined as:
TC(GiGjGk)=N(GiGjGk)L−2 |
which N(GiGjGk) is the number of occurrences of kth group of amino acids followed by jth group of amino acids followed by ith group of amino acids and L is the length of the sequence.
d. Biosynthesis energy: Pyruvate, 3-phosphoglycerate and several other metabolic precursors were combined and formed amino acids. Total cost of this procedure is called biosynthesis energy and calculated by Wagner method [48]. We used it as a feature calculated by:
BE=(∑20i=1fi∗ei)/n |
where n is the length of protein, fi is the frequency of ith amino acid and ei is the biosynthesis energy of ith amino acid.
a. GC content: GC Content stands for Guanine-Cytosine content and represents the percentage of nitrogenous bases on a DNA molecule, which may be either guanine or cytosine. As the bond between guanine and cytosine is a triple bond compared to a double bond between adenine and thymine, the sequences with higher GC content are more stable.
b. Codon usage: Codon usage represents the frequency of occurrence of synonymous codons in coding DNA. By considering fi as frequency of ith codon of jth amino acid and nj as the sum of the occurrence of that amino acid in the desired sequence, codon usage of ith codon is calculated by:
CUi=fi/nj |
c. Relative synonymous codon usage (RSCU): Frequency of each codon divided by frequency of that codon with assumption of equal distribution of codons of the related amino acid [49] and is calculated by:
RSCUij=fij(1/ni)∑nij=1fij |
where fij is the frequency of jth codon of ith amino acid in the protein sequence, ni is the number of codons of ith amino acid.
d. Codon adaption index (CAI): An effective, simple measure of RSCU bias[50] which is calculated by:
CAI=(∏ni=1RSCUi)1/n/(∏ni=1RSCUimax)1/n |
where n is the length of protein, RSCUi is the RSCU value of the ith codon and the RSCUimax is the maximum RSCU value among codons of amino acid related to ith codon.
e. Stacking energy: The nearest-neighbor (NN) model of nucleic acids assumes that the identity and orientation of neighboring base pairs of a particular base pair affect the stability of the base pair [51].
Stacking Energy is calculated by:
∇Gtotal=∑(ni∗∇Gi)+∇Ginit+∇Gend+∇Gsym |
where ∇G for init, i and end is acquired by unified nearest-neighbor (NN) free energy parameter. If the duplex is self-complementary, its symmetry is conserved by setting ∇Gsym to +0.43 (kcal/mol) and zero if it is non-self-complementary.
f. Interaction energy: Dispersion and repulsion energies between a codon and its complement is called interaction energy [52] and calculated by:
IE=∑20i=1(ei∗ni)/n |
where n is the length of protein, ni is the frequency of ith amino acid and ei is the interaction energy of ith amino acid.
a. Hydrophobicity: Repletion tendency of an amino acid from a mass of water.
b. Hydrophilicity: Attraction tendency of an amino acid to a mass of water.
c. Polarity: The degree to which a molecule has a dipole moment.
e. Polarizability: The influencing amount of an external electric field on the electron clouds of a molecule.
f. Side chain volume: Sum of volume of side chain atoms of an amino acid.
g. Solvent-accessible surface area: The surface area of a biomolecule that is accessible to a solvent [53].
h. Net charge index of residue side chains [54]
To add the effect of certain distance neighbors of each amino acid, Auto covariance is used [55] and the mentioned physicochemical properties were assumed as interaction mode. AC is calculated by the following equation:
AC(d.k)=1L−dL−d∑i=1(Pi,k−1LL∑j=1Pj.k)∗(Pi+d,k−1LL∑j=1Pj,k) |
Where i, j are ith and jth residue and k is the index of the physicochemical properties. Pi.k is kth physicochemical property of ith amino acid. L is length of the sequence and d is the distance between the current residue and its neighbor. As an example, d = 1 is the first neighbor which is regarded to the next residue while d = 2 is the second neighbor and so on.
Gene ontology (GO) [32] is a comprehensive set of ontologies for molecular biology domains developed for gene annotations of all organisms as a hierarchy. It uses a shared language to achieve a mutual understanding of the definition and meaning of any word used. There are three classes in GO:
a. Cellular compartment (CC): Where a gene product is located such as inner and outer membrane.
b. Molecular function (MF): An element activity, task or job such as protein kinase activity or insulin receptor activity.
c. Biological process (BP): A commonly recognized series of events such as cell division or transcription.
The similarity between these GO terms are achieved from the frequencies of two GO terms involved and their closest common ancestor term in a specific corpus of GO annotations[56].
GO terms have a hierarchical structure. Each of them is a node in a tree and may have parents and children. Frequency of each GO term is calculated by dividing the total number of its children over all number of GO terms which is called Fc for cth term. The information content (IC) of a GO term is computed by the negative log frequency of that term. A rarely used term contains a greater amount of information [57]. IC of a concept is given by the following formula:
IC(c)=−log(Fc) |
The most informative common ancestor (MICA) is the largest IC of all common ancestors of two concepts and calculated by the following formula:
MICA(c1⋅c2)=max{IC(a)|a∈CommonAncestors(c1,c2)} |
Resnik [58] defined largest information content of all common ancestors as the semantic similarity between the concepts.
simRes(c1⋅c2)=MICA(c1,c2) |
Jiang [59] defined the semantic similarity as the inverse of difference between their information content and the largest information content of all common ancestors.
SimJia(c1⋅c2)=1IC(c1)+IC(c2)−2∗MICA(c1,c2)+1 |
Lin [60] considered MICA over their information content as the semantic similarity.
SimLin(c1⋅c2)=2∗MICA(c1,c2)IC(c1)+IC(c2) |
We used all of the mentioned methods for calculating semantic similarities for each pair of HVIN separately for each class. So we gained nine GO features: MFSimRes, MFSimJia, MFSimLin, BPSimRes, BPSimJia, BPSimLin, CCSimRes, CCSimJia, CCSimLin. By evaluating the models which are trained by these features, three features gained by Jiang similarity are chosen as the final GO semantic similarity features.
a. Degree (connectivity): Is defined as the number of partners that are interacting with a protein p.
b. Neighborhood connectivity: Neighborhood connectivity is based on degree (connectivity) measure. In fact, the average connectivity of all neighbors of p represents the neighborhood connectivity of p.
c. Shortest paths: The length of a path is the number of edges forming it. The pass with minimum length between each two proteins i and j is considered as the shortest path. For each protein as shown in the following formula, the shortest path centrality is the summation of shortest path between that protein and all the other proteins divided by the number of proteins. (∑nm=1Sp⋅m)/n
d. Shared neighbors: This topological measure represents the number of interacting partners shared between proteins i and j, i.e., proteins which are neighbors of both i and j.
e. Stress centrality: The number of the shortest paths between all protein pairs in the HPPIN passing through a given protein p stands for the stress centrality of p. This centrality is representative of the workload the protein carries in a network. If a protein is traversed by a high number of shortest paths, then it has a high stress.
f. Topological coefficients: A relative measure for the extent to which a protein shares neighbors with others. Proteins that have no or one neighbor are assigned a topological coefficient of 0 (zero). The chart of the topological coefficients can be used to estimate the tendency of the proteins in the HPPIN to have shared neighbors. Topological coefficient is defined as follows: Tp = avg(Jp.m)/kp, where Jp.m is defined for all proteins m that share at least one neighbor with protein p and the value Jp.m is the number of neighbors shared between the proteins p and m, plus one if there is a direct link between proteins p and m. However, Kp is the number of neighbors of protein p.
g. Closeness centrality: The closeness centrality Cc(p) of a protein p defines the reciprocal of the average shortest path length. Actually, it is a number between 0 and 1 which is computed as:
Cc(p)=1/avg(Lp,m) |
where Lp.m is the length of the shortest path between two proteins p and m.
The closeness centrality of isolated proteins is equal to 0. This measure shows how fast information spreads from a given protein to other reachable ones in the HPPIN.
h. Clustering coefficients: The clustering coefficient for a protein p is the number of triangles (3-loops) that pass through p, relative to the maximum number of triangles that could pass through p.
Cp=2ep/(kp(kp−1)) |
where kp is the number of neighbors of p and ep is the number of connected pairs between all neighbors of p.
i. Betweenness centrality: The betweenness centrality of a protein p represents the amount of control that p exerts over the interactions of others in the HPPIN and it is defined as follows: Cb(p)=∑σst(p)σst, where s and t are proteins in the HPPIN different from p, σst shows the number of the shortest paths from s to t, and σst (p) is the number of the shortest paths from s to t that p lies on.
j. Radiality: The radiality of a protein is calculated by subtracting the average shortest path between that protein and all other proteins in the HPPIN from the value of the diameter. Hence, proteins with higher radiality are usually closer to the other nodes, whereas, proteins with lower radiality are peripheral.
Five different categories of features were used each in a separate model. Combination of these features were performed by choosing random features among all existing features for training 10 other models. All these 15 models were constructed by different classifiers to obtain divers base classifiers.
The results of predictions of 10 most popular classifiers were combined by stacked generalization [61]. In stacked generalization the outputs of the base classifiers were given to a meta-learner which combines the outputs to get the final output.
We used two different models as meta learners including random forest and majority voting. In majority voting, we used three different thresholds for accepting the votes: 30%, 40% and 50%. By this definition we get more sensitivity through sacrificing the specificity in 30% model while in 50% model, we get more specificity through sacrificing sensitivity.
The prediction performance of the proposed method was evaluated by four major measures of evaluation measure package [62], calculated based on the number of interactions predicted correctly (TP), the number of non-interactions which are predicted correctly (TN), the number of non-interactions which are predicted as interaction (FP) and the number of interactions which are predicted as non-interactions (FN). Some of the formulas of these measures are listed below:
Specificity=TNTN+FP,Sensitivity=TPTP+FNAccuracy=TP+TNTP+TN+FP+FN,FMeasure=2TP2TP+FP+FN |
To predict new HV-PPI, five different categories of features were extracted from physicochemical properties of amino acids, network topology of HPPIN, protein sequences, subcellular localization of human proteins and GO semantic similarities (all human and virus proteins’ extracted features are available at http://bioinf.modares.ac.ir/software/complexnet/Influenza/HumanFeatures.rar and http://bioinf.modares.ac.ir/software/complexnet/Influenza/VirusFeatures.rar, respectively).
Several models were constructed by choosing different features from these categories. Five models are made by choosing all the features of one category. Moreover, 10 more models are made by choosing random features from all the exiting features. Among these models, the best results belonged to the models which had more diverse features.
To estimate the proposed model’s performance, a 10-fold cross validation procedure is used. The dataset is partitioned into 10 equal parts (all 10 partitioned train and test datasets are available at http://bioinf.modares.ac.ir/software/complexnet/Influenza/10FoldCrossValidation.rar). Each time nine partitions are used for training and one remaining partition is used for testing the model. Average of the obtained performance in each evaluation measure of the ten testing sets is reported as the final performance in that evaluation measure which is shown in Figure 4 for each of the classifiers.
Finally, we sent the results to a meta learner. If either the sensitivity or specificity measures are more important for the researcher, majority voting with 30% or 50% positive voter is used. Otherwise, random forest is used as a meta learner. The results are shown in Figure 5.
A database containing the predicted interactome of HVPPI has been constructed, which is publicly accessible at http://bioinf.modares.ac.ir/software/complexnet/Influenza as it is shown in Figure 6. To do this end, all possible interactions (812,625 interactions) between each of 6501 HPs of HVIN with each of 125 IAV proteins were examined by the trained model. Mean of the prediction probability of all the models is reported as the final interaction probability (InPr) of the interaction between each pair. The results are shown in Figure 7 as a heat map in which colors show the probability of interaction between the pairs. Columns and rows represent human and virus proteins respectively. Pairs with a score of one could be good candidates for researchers to do experimental test.
Among all the 812,625 pairs, 6919 pairs have the score 1 (which is available at http://bioinf.modares.ac.ir/software/complexnet/Influenza/Novel6919Interactions.rar). By investigating the human partners of these 6919 pairs, 76 human proteins with a degree larger than 5 are selected (human proteins targeted by more than five virus proteins) and their interaction network are gained by STRING [41] as it is depicted in Figure 7. The constructed network has 256 edges with an average node degree of 6.75 in the HPPIN and an average local clustering coefficient of 0.47. Color of edges determine the type of interaction between the nodes. Cyan edges are interaction extracted from curated databases, while pink ones are experimentally determined. Blue, Green and red edges are predicted interactions gained by gene neighborhood, gene fusion and gene co-occurrence respectively. Light green edges are extracted by text mining, while violet edges are gained by protein homology, and finally, the nodes connected by black edges are co-expressed.
By using DAVID [63] tools, gene ontology enrichment analysis was done on these 76 proteins (results are available at enrichment tab of http://bioinf.modares.ac.ir/software/complexnet/Influenza). Furthermore, by using REVIGO [64], the whole enriched biological process (BP), cellular component (CC) and molecular function (MF) terms are depicted and available at enrichment tab of http://bioinf.modares.ac.ir/software/complexnet/Influenza.
In this study, heterogeneous descriptors were used to predict HV-PPI. Contribution of the different descriptors were measured by removing each feature type in turn and recalculating the evaluation measures of the proposed prediction model; the higher the loss of measures, the more important the feature type. As shown in Table 1, HPPIN topology is the most important feature type in predicting HI-PPIs. GO semantic similarity is another important feature with considering the number of features in each feature type (three features of GO semantic similarity make 0.029 loss of sensitivity in contrast with 1414 features of sequence-based feature type which make only 0.005 more loss of sensitivity).
Feature type | Loss of Sensitivity | Loss of Specificity | Loss of Accuracy |
Nucleotide sequence-based | 0.027 | 0.012 | 0.019 |
GO semantic similarity | 0.022 | 0.011 | 0.018 |
Amino acid sequence-based | 0.008 | 0.003 | 0.005 |
Physicochemical properties | 0.025 | 0.013 | 0.018 |
HPPIN topology | 0.035 | 0.023 | 0.029 |
We also extracted the most important features by four ways:
a. Tree models: In tree models, after the split, the percentage of training samples fallen into all terminal nodes determine the feature importance. In this method, since all samples are affected by the first predictor of the first split, it has an importance measurement of 1. Other predictors will be scored in range of zero to one.
b. Rule-based models: The number of rules involving the predictor determines the importance of features.
c. PCA: Sum of the Loading coefficients of the 10 first Principal Components[65] (PCs) are considered as a score for determining the feature importance.
d. GA-PLS: The selection of the best subset of variables is one of the most popular usage of Genetic Algorithms(GA), Especially in variable selection of Partial Least Squares(PLS) models[66]. For this purpose, we made an initial population by selecting part of variables randomly and fit a PLS model on them. In this method, each variable is considered as a gene and each variable set is considered as a chromosome. Every chromosome consists of 1800 genes, in which each gene is on with probability of 0.2 and so the approximate length of the chromosome is 360 variables. By generating 100 chromosomes, initial population was created. ROC value divided by the number of variables is considered as fitness value. This strategy was performed hundreds of times and the top 30 percent of variable sets with higher fitness values were sent to the next generation. In the new generation we made mutations by changing the variable’s value with probability of 0.05 and also performed a crossover between the variable sets and repeated the previous steps. Finally, the variables with the lowest prediction error were reported as the most important features. As the result of genetic algorithm changed with each run, we repeated the previous step 100 times and the variables with the most frequency in these 100 runs were reported as the most important variables.
Feature importance was calculated by all mentioned models. Sum of the scores of the top 10 percent features is depicted in Figure 9. Panel (a) shows the score distribution of each type while Panel (b) shows the features distribution of each type. For the top one percent of features with highest score Panel (c) shows the score of features with size and type of the circles by putting the features with the same category in one circle, whereas Panel (d) compares the features mean for positive and negative samples.
Figure 9(d) reveals that GO semantic similarity-based features of positive samples have apparently higher values in comparison with negative samples while features extracted from physicochemical properties of amino acids of negative samples have higher values in comparison with positive samples.
It seems that network topology of HPPI network plays the most important role in exposing the important features. The gene ontology semantic similarity-based features play the second most important role in determining the important variables. Furthermore, conjoint triad of virus proteins has a higher chance of being a candidate as important features.
In this study, we proposed a computational method for predicting HIPPI. Five different categories of descriptors including physicochemical properties of amino acids, nucleotide sequence-based descriptors, gene ontology similarities, protein sequence-based features and network centrality measures were used to encode protein pairs. Several different classifiers such as C5, RF, SVM, NB, KNN are used as base classifiers. Ensemble learning was used to combine the classifiers. The final model achieved an accuracy of 0.93, a specificity of 0.95, and a sensitivity of 0.91 in a 10-fold cross validation analysis on our benchmark dataset.
In addition, all of possible pairs between all of the human proteins and IAV proteins are given as input to our constructed model to design a new database which is available via the following link http://bioinf.modares.ac.ir/software/complexnet/Influenza. Among all of the predicted pairs, 6919 pairs have score 1 which could be good candidates for experimental research or drug targets purpose.
Moreover, Enrichment analysis is reported on 76 human proteins targeted by more than five virus proteins of these 6919 pairs.
According to our analysis, network topology of HPPI network, gene ontology semantic similarity and conjoint triad of virus proteins contribute most in predicting HI-PPIs.
The proposed method can be extended to predict other HV-PPIs.
The authors declared that they have no conflicts of interest to this work.
[1] |
J. M. Langley, M. E. Faughnan, Prevention of influenza in the general population, Can. Med. Assoc. J., 171 (2004), 1213-1222. doi: 10.1503/cmaj.1031737
![]() |
[2] |
W. W. Thompson, D. K. Shay, E. Weintraub, L. Brammer, C. B. Bridges, et al., Influenza-associated hospitalizations in the United States, J. Am. Med. Assoc., 292 (2004), 1333-1340. doi: 10.1001/jama.292.11.1333
![]() |
[3] |
J. K. Taubenberger, D. M. Morens, The pathology of influenza virus infections, Annu. Rev. Pathol. Mech. Dis., 3 (2008), 499-522. doi: 10.1146/annurev.pathmechdis.3.121806.154316
![]() |
[4] |
A. Nagy, L. Černíková, V. Křivda, J. Horníčková, Digital genotyping of avian influenza viruses of H7 subtype detected in central Europe in 2007-2011, Virus Res., 165 (2012), 126-133. doi: 10.1016/j.virusres.2012.02.005
![]() |
[5] | Q Li, L Zhou, M Zhou, Z Chen, F Li, H Wu, et al., Preliminary report: Epidemiology of the avian influenza A (H7N9) outbreak in China, N. Engl. J. Med., 24 (2013), xi-xii. |
[6] |
Y. Hu, S. Lu, Z. Song, W. Wang, P. Hao, J. Li, et al., Association between adverse clinical outcome in human disease caused by novel influenza A H7N9 virus and sustained viral shedding and emergence of antiviral resistance, Lancet, 381 (2013), 2273-2279. doi: 10.1016/S0140-6736(13)61125-3
![]() |
[7] |
G. Neumann, T. Noda, Y. Kawaoka, Emergence and pandemic potential of swine-origin H1N1 influenza virus, Nature, 459 (2009), 931-939. doi: 10.1038/nature08157
![]() |
[8] | G. Lu, K. Buyyani, N. Goty, R. Donis, Z. Chen, Influenza a virus informatics: Genotype-centered database and genotype annotation, Second International Multi-Symposiums on Computer and Computational Sciences (IMSCCS 2007), 2007. Available from: https://ieeexplore.ieee.org/abstract/document/4392583. |
[9] |
A. Melidou, G. Gioula, M. Exindari, D. Chatzidimitriou, E. Diza, N. Malisiovas, Molecular and phylogenetic analysis of the haemagglutinin gene of pandemic influenza H1N1 2009 viruses associated with severe and fatal infections, Virus Res., 151 (2010), 192-199. doi: 10.1016/j.virusres.2010.05.005
![]() |
[10] |
E. D. Kilbourne, Influenza pandemics of the 20th century, Emerg. Infect. Dis., 12 (2006), 9. doi: 10.3201/eid1201.051254
![]() |
[11] | W. H. Organization, Ten things you need to know about pandemic influenza (update of 14 October 2005), Wkly. Epidemiol. Rec., 80 (2005), 428-431. |
[12] |
D. J. Smith, A. S. Lapedes, J. C. de Jong, T. M. Bestebrore, G. F. Rimmelzwaan, A. D. Osterhaus, et al., Mapping the antigenic and genetic evolution of influenza virus, Science, 305 (2004), 371-376. doi: 10.1126/science.1097211
![]() |
[13] |
J. K. Taubenberger, D. M. Morens, 1918 Influenza: the mother of all pandemics, Rev. Biomed., 17 (2006), 69-79. doi: 10.32776/revbiomed.v17i1.440
![]() |
[14] | A Patient, Swine influenza A (H1N1) infection in two children-Southern California, March-April 2009, Morb. Mortal. Wkly. Rep., 58 (2009), 400-402. |
[15] |
M. P. Girard, J. S. Tam, O. M. Assossou, M. P. Kieny, The 2009 A (H1N1) influenza virus pandemic: A review, Vaccine, 28 (2010), 4895-4902. doi: 10.1016/j.vaccine.2010.05.031
![]() |
[16] | E. Golemis, Protein-protein interactions: A molecular cloning manual, CSHL Press, (2005). |
[17] |
C. D. Hu, Y. Chinenov, T. K. Kerppola, Visualization of interactions among bZIP and Rel family proteins in living cells using bimolecular fluorescence complementation, Mol. Cell, 9 (2002), 789-798. doi: 10.1016/S1097-2765(02)00496-3
![]() |
[18] |
E. Sprinzak, H. Margalit, Correlated sequence-signatures as markers of protein-protein interaction11Edited by G. von Heijne, J. Mol. Biol., 311 (2001), 681-692. doi: 10.1006/jmbi.2001.4920
![]() |
[19] | W. K. Kim, J. Park, J. K. Suh, Large scale statistical prediction of protein-protein interaction by potentially interacting domain (PID) pair., Genome Inform., 13 (2002) 42-50. |
[20] |
S. K. Ng, Z. Zhang, S. H. Tan, Integrative approach for computationally inferring protein domain interactions, Bioinformatics, 19 (2003), 923-929. doi: 10.1093/bioinformatics/btg118
![]() |
[21] |
H. Yu, N. M. Luscombe, H. X. Lu, X. Zhu, Y. Xia, J. D. Han, et al., Annotation transfer between genomes: protein-protein interologs and protein-DNA regulogs, Genome Res., 14 (2004), 1107-1118. doi: 10.1101/gr.1774904
![]() |
[22] |
L. V Zhang, S. L. Wong, O. D. King, F. P. Roth, Predicting co-complexed protein pairs using genomic and proteomic data integration, BMC Bioinformatics, 5 (2004), 38. doi: 10.1186/1471-2105-5-38
![]() |
[23] |
R. Jansen, H. Yu, D. Greenbaum, Y. Kluger, N. J. Krogan, S. Chung, et al., A Bayesian networks approach for predicting protein-protein interactions from genomic data, Science, 302 (2003), 449-453. doi: 10.1126/science.1087361
![]() |
[24] |
Y. Qi, Z. Bar‐Joseph, J. Klein‐Seetharaman, Evaluation of different biological data and computational classification methods for use in protein interaction prediction, Proteins Struct. Funct. Bioinforma., 63 (2006), 490-500. doi: 10.1002/prot.20865
![]() |
[25] |
M. D. Dyer, T. M. Murali, B. W. Sobral, Computational prediction of host-pathogen protein-protein interactions, Bioinformatics, 23 (2007), 159-166. doi: 10.1093/bioinformatics/btm208
![]() |
[26] |
A. Emamjomeh, B. Goliaei, J. Zahiri, R. Ebrahimpour, Predicting protein-protein interactions between human and hepatitis C virus via an ensemble learning method, Mol Biosyst, 10 (2014), 3147-3154. doi: 10.1039/C4MB00410H
![]() |
[27] | O. Tastan, Y. Qi, J. G. Carbonell, J. Klein-Seetharaman, Prediction of interactions between HIV-1 and human proteins by information integration, in Biocomputing, World Scientific, (2009), 516-527. |
[28] |
Y. Qi, O. Tastan, J. G. Carbonell, J. Klein-Seetharaman, J. Weston, Semi-supervised multi-task learning for predicting interactions between HIV-1 and human proteins, Bioinformatics, 26 (2010), i645-i652. doi: 10.1093/bioinformatics/btq394
![]() |
[29] | B. Barnes, M. Karimloo, A. Schoenrock, D. Burnside, E. Cassol, A. Wong, et al., Predicting novel protein-protein interactions between the HIV-1 virus and homo sapiens, 2016 IEEE EMBS International Student Conference (ISC), 2016. Available from: https://ieeexplore.ieee.org/abstract/document/7508598/. |
[30] | S. Alguwaizani, B. Park, X. Zhou, D. S. Huang, K. Han, Predicting Interactions between Virus and Host Proteins Using Repeat Patterns and Composition of Amino Acids, J. Healthc. Eng., 2018 (2018). |
[31] | N. Zhang, M. Jiang, T. Huang, Y. D. Cai, Identification of Influenza A/H7N9 virus infection-related human genes based on shortest paths in a virus-human protein interaction network, Biomed. Res. Int., 2014 (2014). |
[32] |
Gene Ontology Consortium, The Gene Ontology (GO) database and informatics resource, Nucleic Acids Res., 32 (2004), D258-D261. doi: 10.1093/nar/gkh036
![]() |
[33] | C. L. P. Eng, J. C. Tong, T. W. Tan, Predicting host tropism of influenza A virus proteins using random forest, BMC Med. Genomics, 7 (2014), S1. |
[34] | L. Nanni, A. Lumini, S. Brahnam, An Empirical Study of Different Approaches for Protein Classification, Sci. World J., 2014 (2014), 236717. |
[35] |
E. I. Zacharaki, Prediction of protein function using a deep convolutional neural network ensemble, PeerJ Comput. Sci., 3 (2017), e124. doi: 10.7717/peerj-cs.124
![]() |
[36] |
I. Saha, J. Zubek, T. Klingstrom, S. Forsberg, J. Wikander, M. Kierczak, et al., Ensemble learning prediction of protein-protein interactions using proteins functional annotations, Mol. Biosyst., 10 (2014), 820-830. doi: 10.1039/c3mb70486f
![]() |
[37] | L. Nanni, S. Brahnam, S. Ghidoni, A. Lumini, Toward a general-purpose heterogeneous ensemble for pattern classification, Comput. Intell. Neurosci., 2015 (2015). |
[38] | S. Kerrien, B. Aranda, L. Breuza, A. Bridge, F. Broackes-Carter, C. Chen, et al., The IntAct molecular interaction database in 2012, Nucleic Acids Res., 40 (2011), D841-D846. |
[39] |
A. Chatr-aryamontri, A. Ceol, D. Peluso, A. Nardozza, S. Panni, F. Sacco, et al., VirusMINT: A viral protein interaction database, Nucleic Acids Res., 37 (2009), D669-D673. doi: 10.1093/nar/gkn739
![]() |
[40] |
I. Xenarios, L. Salwinski, X. J. Duan, P. Higney, S. M. Kim, D. Eisenberg, DIP, the Database of Interacting Proteins: a research tool for studying cellular networks of protein interactions, Nucleic Acids Res., 30 (2002), 303-305. doi: 10.1093/nar/30.1.303
![]() |
[41] | D. Szklarczyk, A. Franceschini, S. Wyder, K. Forslund, D. Heller, J. Huerta-Cepas, et al., STRING v10: protein-protein interaction networks, integrated over the tree of life, Nucleic Acids Res., 43 (2014), D447-D452. |
[42] |
C. Stark, B. J. Breitkreutz, T. Reguly, L. Boucher, A. Breitkreutz, M. Tyers, BioGRID: A general repository for interaction datasets, Nucleic Acids Res., 34 (2006), D535-D539. doi: 10.1093/nar/gkj109
![]() |
[43] |
J. Yu, M. Guo, C. J. Needham, Y. Huang, L. Cai, D. R. Westhead, Simple sequence-based kernels do not predict protein-protein interactions, Bioinformatics, 26 (2010), 2610-2614. doi: 10.1093/bioinformatics/btq483
![]() |
[44] |
Y. Huang, B. Niu, Y. Gao, L. Fu, W. Li, CD-HIT Suite: A web server for clustering and comparing biological sequences, Bioinformatics, 26 (2010), 680-682. doi: 10.1093/bioinformatics/btq003
![]() |
[45] |
M. A. Tahir, J. Kittler, F. Yan, Inverse random under sampling for class imbalance problem and its application to multi-label classification, Pattern Recognit., 45 (2012), 3738-3750. doi: 10.1016/j.patcog.2012.03.014
![]() |
[46] |
S. Kawashima, P. Pokarowski, M. Pokarowska, A. Kolinski, T. Katayama, M. Kanehisa, AAindex: Amino acid index database, progress report 2008, Nucleic Acids Res, 36 (2008) D202-205. doi: 10.1093/nar/gkn255
![]() |
[47] | R. Bellman, R. Corporation, Dynamic Programming, Princeton University Press, (1957). |
[48] |
A. Wagner, Energy constraints on the evolution of gene expression, Mol. Biol. Evol., 22 (2005), 1365-1374. doi: 10.1093/molbev/msi126
![]() |
[49] |
P. M. Sharp, T. M. Tuohy, K. R. Mosurski, Codon usage in yeast: Cluster analysis clearly differentiates highly and lowly expressed genes., Nucleic Acids Res., 14 (1986), 5125-5143. doi: 10.1093/nar/14.13.5125
![]() |
[50] |
P. M. Sharp, W. H. Li, The codon Adaptation Index--a measure of directional synonymous codon usage bias, and its potential applications, Nucleic Acids Res., 15 (1987), 1281-1295. doi: 10.1093/nar/15.3.1281
![]() |
[51] |
J. SantaLucia, A unified view of polymer, dumbbell, and oligonucleotide DNA nearest-neighbor thermodynamics, Proc. Natl. Acad. Sci., 95 (1998), 1460-1465. doi: 10.1073/pnas.95.4.1460
![]() |
[52] |
P. Claverie, Calculation of interaction energy between triplets in the RNA 11 configuration, J. Mol. Biol., 56 (1971), 75-82. doi: 10.1016/0022-2836(71)90085-4
![]() |
[53] |
B. Lee, F. M. Richards, The interpretation of protein structures: estimation of static accessibility, J. Mol. Biol., 55 (1971), 379-IN4. doi: 10.1016/0022-2836(71)90324-X
![]() |
[54] |
P. Klein, M. Kanehisa, C. DeLisi, Prediction of protein function from sequence properties: Discriminant analysis of a data base, Biochim. Biophys. Acta, Protein Struct. Mol. Enzymol., 787 (1984) 221-226. doi: 10.1016/0167-4838(84)90312-1
![]() |
[55] |
Y. Guo, L. Yu, Z. Wen, M. Li, Using support vector machine combined with auto covariance to predict protein-protein interactions from protein sequences, Nucleic Acids Res., 36 (2008), 3025-3030. doi: 10.1093/nar/gkn159
![]() |
[56] |
X. Wu, E. Pang, K. Lin, Z. M. Pei, Improving the Measurement of Semantic Similarity between Gene Ontology Terms and Gene Products: Insights from an Edge- and IC-Based Hybrid Method, PLoS One, 8 (2013), e66745. doi: 10.1371/journal.pone.0066745
![]() |
[57] |
Y. R. Cho, W. Hwang, M. Ramanathan, A. Zhang, Semantic integration to identify overlapping functional modules in protein interaction networks, BMC Bioinformatics, 8 (2007) 265. doi: 10.1186/1471-2105-8-265
![]() |
[58] | P. Resnik, Using information content to evaluate semantic similarity in a taxonomy, arXiv Prepr. C., 1995 (1995). |
[59] | J. J. Jiang, D. W. Conrath, Semantic similarity based on corpus statistics and lexical taxonomy, arXiv Prepr. C., 1997 (1997). |
[60] | D. Lin, An information-theoretic definition of similarity, Icml, 98 (1998) 296-304. |
[61] |
D. H. Wolpert, Stacked Generalization, Neural Networks, 5 (1992), 241-259. doi: 10.1016/S0893-6080(05)80023-1
![]() |
[62] | B. Khorsand, EvaluationMeasures: Collection of Model Evaluation Measure Functions, CRAN, 2016 (2016). |
[63] |
D. W. Huang, B. T. Sherman, R. A. Lempicki, Systematic and integrative analysis of large gene lists using DAVID bioinformatics resources, Nat. Protoc., 4 (2009), 44-57. doi: 10.1038/nprot.2008.211
![]() |
[64] |
F. Supek, M. Bošnjak, N. Škunca, T. Šmuc, REVIGO summarizes and visualizes long lists of gene ontology terms, PLoS One, 6 (2011), e21800. doi: 10.1371/journal.pone.0021800
![]() |
[65] |
H. Hotelling, Analysis of a complex of statistical variables into principal components, J. Educ. Psychol., 24 (1933), 417. doi: 10.1037/h0071325
![]() |
[66] |
R. Leardi, A. L. Gonzalez, Genetic algorithms applied to feature selection in PLS regression: how and when to use them, Chemom. Intell. Lab. Syst., 41 (1998), 195-207. doi: 10.1016/S0169-7439(98)00051-3
![]() |
1. | Abhigyan Nath, André Leier, Improved cytokine–receptor interaction prediction by exploiting the negative sample space, 2020, 21, 1471-2105, 10.1186/s12859-020-03835-5 | |
2. | Babak Khorsand, Abdorreza Savadi, Mahmoud Naghibzadeh, SARS-CoV-2-human protein-protein interaction network, 2020, 20, 23529148, 100413, 10.1016/j.imu.2020.100413 | |
3. | Babak Khorsand, Abdorreza Savadi, Mahmoud Naghibzadeh, Comprehensive host-pathogen protein-protein interaction network analysis, 2020, 21, 1471-2105, 10.1186/s12859-020-03706-z | |
4. | Shengrong Xia, Yingchun Xia, Chulei Xiang, Hui Wang, Chao Wang, Jin He, Guolong Shi, Lichuan Gu, A virus–target host proteins recognition method based on integrated complexes data and seed extension, 2022, 23, 1471-2105, 10.1186/s12859-022-04792-x | |
5. | Aref Shiralipour, Babak Khorsand, Leila Jafari, Mohammad Salehi, Mahsa Kazemi, Javad Zahiri, Vahid Jajarmi, Bahram Kazemi, Identifying Key Lysosome-Related Genes Associated with Drug-Resistant Breast Cancer Using Computational and Systems Biology Approach, 2022, 21, 1735-0328, 10.5812/ijpr-130342 | |
6. | Maryam Soltanyzadeh, Babak Khorsand, Alireza Ahmadi Baneh, Hamidreza Houri, Clarifying differences in gene expression profile of umbilical cord vein and bone marrow-derived mesenchymal stem cells; a comparative in silico study, 2022, 33, 23529148, 101072, 10.1016/j.imu.2022.101072 | |
7. | Hakimeh Khojasteh, Alireza Khanteymoori, Mohammad Hossein Olyaee, Comparing protein–protein interaction networks of SARS-CoV-2 and (H1N1) influenza using topological features, 2022, 12, 2045-2322, 10.1038/s41598-022-08574-6 | |
8. | Mohammad Rasoul Samandari Bahraseman, Babak Khorsand, Keyvan Esmaeilzadeh-Salestani, Solmaz Sarhadi, Nima Hatami, Banafsheh Khaleghdoust, Evelin Loit, Aneta Agnieszka Koronowicz, The use of integrated text mining and protein-protein interaction approach to evaluate the effects of combined chemotherapeutic and chemopreventive agents in cancer therapy, 2022, 17, 1932-6203, e0276458, 10.1371/journal.pone.0276458 | |
9. | Jie Pan, Li-Ping Li, Chang-Qing Yu, Zhu-Hong You, Yong-Jian Guan, Zhong-Hao Ren, Sequence-Based Prediction of Plant Protein-Protein Interactions by Combining Discrete Sine Transformation With Rotation Forest, 2021, 17, 1176-9343, 117693432110500, 10.1177/11769343211050067 | |
10. | Neda Eskandarzade, Abozar Ghorbani, Samira Samarfard, Jose Diaz, Pietro H. Guzzi, Niloofar Fariborzi, Ahmad Tahmasebi, Keramatollah Izadpanah, Network for network concept offers new insights into host- SARS-CoV-2 protein interactions and potential novel targets for developing antiviral drugs, 2022, 146, 00104825, 105575, 10.1016/j.compbiomed.2022.105575 | |
11. | Yingjun Ma, Junjiang Zhong, Logistic tensor decomposition with sparse subspace learning for prediction of multiple disease types of human–virus protein–protein interactions, 2023, 24, 1467-5463, 10.1093/bib/bbac604 | |
12. | Sho Tsukiyama, Hiroyuki Kurata, Cross-attention PHV: Prediction of human and virus protein-protein interactions using cross-attention–based neural networks, 2022, 20, 20010370, 5564, 10.1016/j.csbj.2022.10.012 | |
13. | Zeynep Banu ÖZGER, Zeynep ÇAKABAY, Computational Prediction of Interactions Between SARS-CoV-2 and Human Protein Pairs by PSSM-Based Images, 2023, 12, 2147-3129, 166, 10.17798/bitlisfen.1220301 | |
14. | Weicheng Sun, Jinsheng Xu, Weihan Zhang, Xuelian Li, Yongbin Zeng, Ping Zhang, Funnel graph neural networks with multi-granularity cascaded fusing for protein–protein interaction prediction, 2024, 257, 09574174, 125030, 10.1016/j.eswa.2024.125030 | |
15. | Jerry Emmanuel, Itunuoluwa Isewon, Grace Olasehinde, Jelili Oyelade, 2024, Current Trend and Performance Evaluation of Machine Learning Methods for Predicting Host-Pathogen Protein-Protein Interactions, 979-8-3503-5815-5, 1, 10.1109/SEB4SDG60871.2024.10629915 | |
16. | Zeynep Banu Ozger, A robust protein language model for SARS-CoV-2 protein–protein interaction network prediction, 2023, 142, 09333657, 102574, 10.1016/j.artmed.2023.102574 | |
17. | Mohamad Hazwan Fikri Khairi, Nor Azlan Nor Muhammad, Hamidun Bunawan, Kauthar Mohd Daud, Suhaila Sulaiman, Zeti-Azura Mohamed-Hussein, Mui-Yun Wong, Ahmad Bazli Ramzi, Current progress on the computational methods for prediction of host-pathogen protein-protein interaction in the Ganoderma boninense-oil palm pathosystem, 2024, 129, 08855765, 102201, 10.1016/j.pmpp.2023.102201 | |
18. | Yasmin Bano, Abhinav Shrivastava, 2024, 9780323958905, 15, 10.1016/B978-0-323-95890-5.00006-5 | |
19. | Jerry Emmanuel, Itunuoluwa Isewon, Grace Olasehinde, Jelili Oyelade, An Extended Feature Representation Technique for Predicting Sequenced-based Host-pathogen Protein-protein Interaction, 2025, 20, 15748936, 229, 10.2174/0115748936286848240108074303 |
Feature type | Loss of Sensitivity | Loss of Specificity | Loss of Accuracy |
Nucleotide sequence-based | 0.027 | 0.012 | 0.019 |
GO semantic similarity | 0.022 | 0.011 | 0.018 |
Amino acid sequence-based | 0.008 | 0.003 | 0.005 |
Physicochemical properties | 0.025 | 0.013 | 0.018 |
HPPIN topology | 0.035 | 0.023 | 0.029 |