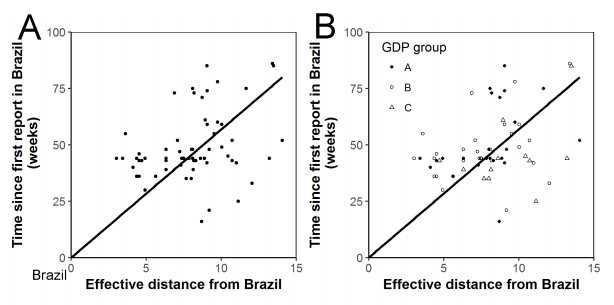
Citation: Nao Yamamoto, Hyojung Lee, Hiroshi Nishiura. Exploring the mechanisms behind the country-specific time of Zika virusimportation[J]. Mathematical Biosciences and Engineering, 2019, 16(5): 3272-3284. doi: 10.3934/mbe.2019163
[1] | Antonios Armaou, Bryce Katch, Lucia Russo, Constantinos Siettos . Designing social distancing policies for the COVID-19 pandemic: A probabilistic model predictive control approach. Mathematical Biosciences and Engineering, 2022, 19(9): 8804-8832. doi: 10.3934/mbe.2022409 |
[2] | Haiyan Wang, Nao Yamamoto . Using a partial differential equation with Google Mobility data to predict COVID-19 in Arizona. Mathematical Biosciences and Engineering, 2020, 17(5): 4891-4904. doi: 10.3934/mbe.2020266 |
[3] | Hai-Feng Huo, Tian Fu, Hong Xiang . Dynamics and optimal control of a Zika model with sexual and vertical transmissions. Mathematical Biosciences and Engineering, 2023, 20(5): 8279-8304. doi: 10.3934/mbe.2023361 |
[4] | Xiaoying Wang . Studying social awareness of physical distancing in mitigating COVID-19 transmission. Mathematical Biosciences and Engineering, 2020, 17(6): 7428-7441. doi: 10.3934/mbe.2020380 |
[5] | Abdelkarim Lamghari, Dramane Sam Idris Kanté, Aissam Jebrane, Abdelilah Hakim . Modeling the impact of distancing measures on infectious disease spread: a case study of COVID-19 in the Moroccan population. Mathematical Biosciences and Engineering, 2024, 21(3): 4370-4396. doi: 10.3934/mbe.2024193 |
[6] | F. S. Vannucchi, S. Boccaletti . Chaotic spreading of epidemics in complex networks of excitable units. Mathematical Biosciences and Engineering, 2004, 1(1): 49-55. doi: 10.3934/mbe.2004.1.49 |
[7] | Huan Rong, Tinghuai Ma, Xinyu Cao, Xin Yu, Gongchi Chen . TEP2MP: A text-emotion prediction model oriented to multi-participant text-conversation scenario with hybrid attention enhancement. Mathematical Biosciences and Engineering, 2022, 19(3): 2671-2699. doi: 10.3934/mbe.2022122 |
[8] | Sherry Towers, Katia Vogt Geisse, Chia-Chun Tsai, Qing Han, Zhilan Feng . The impact of school closures on pandemic influenza: Assessing potential repercussions using a seasonal SIR model. Mathematical Biosciences and Engineering, 2012, 9(2): 413-430. doi: 10.3934/mbe.2012.9.413 |
[9] | Raimund Bürger, Gerardo Chowell, Pep Mulet, Luis M. Villada . Modelling the spatial-temporal progression of the 2009 A/H1N1 influenza pandemic in Chile. Mathematical Biosciences and Engineering, 2016, 13(1): 43-65. doi: 10.3934/mbe.2016.13.43 |
[10] | Mustafa Kamal, Mintodê Nicodème Atchadé, Yves Morel Sokadjo, Sabir Ali Siddiqui, Fathy H. Riad, M. M. Abd El-Raouf, Ramy Aldallal, Eslam Hussam, Huda M. Alshanbari, Hassan Alsuhabi, Ahmed M. Gemeay . Influence of COVID-19 vaccination on the dynamics of new infected cases in the world. Mathematical Biosciences and Engineering, 2023, 20(2): 3324-3341. doi: 10.3934/mbe.2023156 |
Zika virus (ZIKV) is a member of the family Flaviviridae, and is the viral cause of Zika fever, which, when symptomatic, involves only mild symptoms including fever, red eyes, joint pain, headache, and a maculopapular rash; Zika resembles a mild form of dengue fever [1]. The virus is named for the Zika Forest, in Uganda, where the virus was first isolated in 1947 from the serum of a rhesus monkey [2]. Aedes species, especially Aedes aegypti and Aedes albopictus, serve as vectors for ZIKV, allowing transmission between Aedes and humans. The transmission is autochthonous in many South American countries [3,4,5]. Historically, human ZIKV infection is less common than dengue fever, but international spread accelerated the global epidemic, and epidemics were observed in 2007 in Micronesia, in 201314 in French Polynesia, and, since 2015, in Brazil and across the world [6,7,8,9]. Following the detection of a large number of cases in Brazil in late 2015, an excessive number of microcephaly cases was reported approximately 30 weeks later in 2016, in northeastern Brazil, which attracted global attention to this virus [10,11,12]. Although ZIKV infections tend to be mild, infected pregnant women are at risk of delivering babies with microcephaly or other congenital disorders. Additionally, the infection can result in Guillain-Barre syndrome in adults [13,14].
The 2015 global epidemic was initially concentrated in northeastern Brazil; however, the infection rapidly spread throughout Latin America and the Caribbean and subsequently to many countries around the world [15,16,17]. More than 45 American countries reported ZIKV importation [18,19], and, of these, all countries except Canada and the US reported ZIKV importation after the Brazil epidemic. While many European countries have reported ZIKV importation from Brazil since the first imported case was reported in 2015, only a few African countries reported importation after the 2015 Brazil epidemic [20]. There are two possible explanations for the timing and the presence of imported cases in a given country, including why some countries did not experience importation. First, due to a countrys effective distance from Brazil or any other epidemic locations, importation may not actually occur. Second, due to limited laboratory capacity, imported cases could have gone undetected, resulting in reporting delays and underreporting. The status and quality of ZIKV diagnostic capacity are known to be highly variable, even among European countries [21], to say nothing of the limitations healthcare workers navigate in low-income countries.
Previous mathematical models were developed to describe vector-borne disease transmission dynamics [22,23], and, after the 2015 Brazil epidemic, mathematical models were applied to ZIKV to describe both local [24,25,26,27] and international transmission [28,29,30]. Both deterministic and stochastic models for ZIKV were formulated to fit data from Colombia, El Salvador, and Suriname in [5]. Massad et.al [31] identified high-risk countries in Europe by using mathematical models to estimate the importation risk from Brazil according to important factors, such as travel volume. In fact, there is an associated question with respect to the predictability of importation time for ZIKV: real-time forecasting for emerging infectious disease epidemics can be achieved by employing meta-population models [32,33], and, as a possible short cut, the so-called "effective distance, " which can be computed from airline network data, has been proposed [34,35]. Use of effective distance indicates that the relative arrival time for imported cases is determined by the path length and adjacency matrix from the origin of the epidemic to the destination. However, in the case of ZIKV, effective distance alone is not necessarily an adequate predictor. Figure 1A shows arrival time as a function of the effective distance from Brazil. While a positive correlation is certainly observed, the resulting importation times were highly variable. Such variation was not identified during severe acute respiratory syndrome (SARS) epidemic and the pandemic of H1N1 influenza in 2009 [34]. Figure 1B classifies countries into three different groups by gross domestic product (GDP) per capita. It seems that the variation in arrival time and deviation from the linear predictor in Figure 1 were partly explainable by GDP or other variables that influence the underlying diagnostic capacity in a given country.
To explore the mechanisms of ZIKV importation, we argued that it is crucial to account for possible inter-country variations in testing, diagnostics, and reporting ZIKV. In this study, we statistically estimated the actual arrival time of ZIKV importation in each country using airline network data, while adjusting for reporting delays. Describing the data generating process of imported cases, we mathematically explored mechanisms behind observed variations in ZIKV arrival time.
The time from Brazil to "reported" importation of ZIKV is defined as the time interval from the first notification of ZIKV infection in Brazil to the time at which each country reported the first ZIKV-infected case. The observed (reported) arrival time was collected from publicly available data sources for a total of 219 countries. Following a recent similar study that was conducted elsewhere [36], we updated our arrival time data. As of September 25, 2018,110 countries reported ZIKV importation (see below for non-imported countries). Of these, we excluded 40 countries that experienced importation before the 2015 Brazil epidemic. In addition to arrival time data, airline transportation data were obtained from the OpenFlights database [37], which uses the Global Flights Network (2016) that includes 230 airports and 4600 flight routes. We selected datasets that can be considered to reflect the diagnostic and reporting capacity of affected countries [38,39]. Specifically, we collected datasets of GDP per capita and several types of health expenditure metrics, including government expenditure on health, per capita government expenditure on health, and private expenditure on health. Moreover, we categorized countries into geographic regions using two different classification systems: first, by World Health Organization (WHO) region, and, second, by continent. Other variables that we examined included religion (Christian, Muslim, and others) and language (English, Spanish, and others). See Otsuki and Nishiura [40] for data collection methods for those explanatory variables.
To determine actual ZIKV arrival times, we constructed a mathematical model that convolutes two functions (Figure 2). For the first function, we modeled the time from Brazil to actual importation, in which we exploited the relationship between arrival time and effective distance. We derived the effective distance, which was created by Brockmann and Helbing [34], from the abovementioned flight network, and we calculated it as the minimum of the summation of all possible path lengths and the logarithm of the product of transition probabilities from the origin country (i.e., Brazil) to each destination country i. A key feature of effective distance is that, as long as we handle emerging infectious diseases, it has shown a very strong correlation with arrival time, demonstrating itself to be an excellent predictor of arrival time. The validity of the predictive performance of effective distance has been described elsewhere [34], and has been used in studies exploring data from the 2003 SARS epidemic and the influenza A(H1N1-2009) pandemic, and it has been widely used to analyze a variety of global infectious disease pandemics [36,40,41]. Let mi be the effective distance from Brazil to country i=1,2⋯,170. Due to the linear relationship between arrival time and effective distance, the hazard function of importation for country i is modeled as an inverse of the effective distance:
λi=kmi | (2.1) |
where k is a constant parameter (to be estimated below). The country-specific hazard function yields the probability density function for ZIKV importation from Brazil to a country i at time t, written as
fi(t,t0;k)=λie−λi(t−t0) | (2.2) |
where t0 is the time at which the global epidemic started at the origin, i.e., Brazil. In this study, we set t0 as 48 weeks prior to the date that Brazil reported their first ZIKV importation, referring to Faria et al.s molecular clock analysis [18].
For the second function, we modeled the time from importation to reporting, accounting for reporting delays that differ by country (Figure 2). The country-specific mean reporting delay μi was modeled with a linear predictor using a combination of two variables: (1) financial capacity for diagnosis and reporting (x1), which was either modeled by GDP per capita or health expenditure (per capita government expenditure on health), and (2) region x2 according to the WHO classification. For x1, we divided 170 countries into 3 groups i.e., G1={A,B,and C} based on tertiles of high, intermediate, and low, respectively. Three-group discretization was adopted because we did not observe GDP per capita as having precise predictor performance when handled as a continuous variable. Additionally, we classified countries into three groups based on WHO region for the variable x2: region of the Americas (AMR), African region (AFR), and countries which belong to neither AMR nor AFR (Others), i.e., G2={AMR,AFR,and Others}. Again, we employed discrete grouping to approximately capture the observed patterns, including the tendency that countries in the AMR reported ZIKV early, while those in the AFR reported relatively late. The linear predictor of μi for country i is
μi=β0+β1G1(J1(i))x1,G1(J1(i))+β2G2(J2(i))x2,G2(J2(i)) | (2.3) |
where βs are coefficients to be estimated, and xy,Gy(j) represents the dummy variable xy that belongs to group j, defined as
x1,G1(j)={0,if j=11,if j=21,if j=3,x2,G2(j)={1,if j=11,if j=20,if j=3 | (2.4) |
That is, for x1,G1(j), j=1 represents high income group, j=2 represents intermediate, and j=3 low income. For x2,G2(j), j=1 represents countries that belong to AMR, j=2 indicates countries that belong to AFR, and j=3 represents other countries. The country grouping j is determined according to country i. Let Jy(i) be the group of variables xy for country i. Then, j corresponds to Jy(i). It shoud be noted that, while AMR is a mix of North America and other countries, the USA and Canada imported ZIKV case prior to Brazil and were excluded from our analysis. We calculated the correlations between x1,G1 and x2,G2 using the Kendall rank correlation coefficient. If significant correlations p<0.05 were identified, we attempted to address the interaction using the interaction term from the linear predictor. However, if the interaction was not adjusted by the interaction-term alone, we removed the candidate model with two correlating variables prior to model comparisons.
For a country i, groups of two variables can be described as gi={G1(J1(i)),G2(J2(i))}. We assumed that the reporting delay followed a gamma distribution. Supposing that country i belongs to group gi, the reporting delay was assumed to be the result of an independent random sampling from the probability density function g(t;μi,σ2i,gi) with a country-specific mean μi and variance σ2i,gi. To obtain group specific variance σ2i,gi, we first estimated the coefficient of variation in two different wayseither using a group-specific standard deviation σgi (i.e., cvgi=σgiμgi) or a constant standard deviation over all the groups σ (i.e., cv′gi=σμgi). Consequently, group-specific variance for gamma distribution obeyed the formula σ2i,gi=cvgiμi or σ2i,gi=cv′giμi.
A total of 219 countries were divided into three different groups:
(ⅰ) 56 countries that reported ZIKV importation after the 2015 Brazil epidemic; the country i in group gi is defined by i∈CRPgi where RP stands for "reported."
(ⅱ) 89 countries that reported no ZIKV importations by the end of 2017; the country i in group gi is defined by i∈CNRPgi where NRP stands for "never reported."
(ⅲ) 74 excluded countries, including 40 countries that reported ZIKV importation before the 2015 Brazil epidemic and 34 countries for which there are no available data for either GDP per capita or health expenditure.
For the countries in group (ⅰ), the probability density function of the reporting time hi(t,t0;k,μi,σ2i,gi) obeys the formula:
hi(t,t0;k,μi,σ2i,gi)=∫t0g(t−s,t0;μi,σ2i,gi)fi(s,t0;k)ds | (2.5) |
For simplicity, let hi(t) be the simpler notation of hi(t,t0;k,μi,σ2i,gi). The likelihood for observing ti in this group is
L1(k,μi,σ2i,gi;ti,t0)=∏i∈CRPgihi(ti) | (2.6) |
where ti is the time of reported ZIKV importation for each country i. For the countries in group (ⅱ), the probability density function of the reporting time wi(t,t0;k,μi,σ2i,gi) is calculated as
wi(t,t0;k,μi,σ2i,gi)=1−∫t0hi(y)dy | (2.7) |
As was done for group (ⅱ), let wi(t) be the simpler notation of wi(t,t0;k,μi,σ2i,gi). The likelihood function for observing censoring for this group is
L2(k,μi,σ2i,gi;tc,t0)=∏i∈CNRPgiwi(tc) | (2.8) |
where tc is the substituted time of reported ZIKV importation into country i, which we assumed was long enough to represent no reported cases. Finally the full likelihood function is
L(k,μi,σ2i,gi;ti,tc,t0)=L1L2 | (2.9) |
Profile-likelihood based confidence intervals were computed to obtain the 95% confidence interval (CI). We computed the second order Akaike information criterion (AICc) for model comparison.
Herein, we only analyzed publicly available data. As such, the datasets were de-identified and fully anonymized in advance, and the analysis of publicly available data without identifying information does not require ethical approval.
This study fully relies on published data, essential components of which we made available as supplementary material.
Models with variable combinations of explanatory parameters for describing the time from importation to reporting were optimized using the same datasets, yielding a total of 56 imported and 89 non-imported countries during the study period. We compared 10 models and selected the best according to which generated the lowest AICc value. Among the financial variables that influenced diagnostic and reporting capacities, GDP per capita and health expenditure were correlated, indicating that they could not jointly explain the reporting delay. Accordingly, when either GDP per capita or health expenditure was included, the other variable was excluded from the final model. Table 1 compares the goodness-of-fit of those 10 different models. Of these, the model with GDP and WHO region yielded the lowest AICc value of 681.7 (Table 1). We compared the mean reporting delay according to GDP per capita tertiles (Figure 3) using the best model (i.e. Model 1 in Table 1). We found that countries with the highest GDPs yielded the shortest reporting delays. It can be interpreted that countries with relatively high GDPs were more likely to report ZIKV infection earlier than countries with lower GDPs. The median reporting delays for first, second, and third GDP tertiles were 12 weeks (95% CI: 1222), 30 weeks (95% CI: 2030), and 35 weeks (95% CI: 2435), respectively. On average, approximately 4 months after the countries in group A reported a first case, countries in group B reported ZIKV importation, and countries in group C subsequently reported ZIKV importation approximately 1 month later. Figure 4 shows the linear relationship between the effective distance and the time from actual importation in Brazil to actual arrival in each country, according to GDP tertile grouping. As the effective distance became longer, the time required for importation increased. Moreover, comparing the time since actual arrival by GDP groups, the countries in the highest GDP tertile (A) experienced a longer time to actual arrival, even up to 75 weeks, since initial importation in Brazil. The majority of countries in the lowest tertile group experienced importation within 25 weeks of actual importation in Brazil.
Model (a) | Variable* | n∗∗σ | n†a | AICc‡a | Δ AICc‡a |
1 | GDP, WHO region | 1 | 6 | 681.7 | 0 |
2 | Constant | 3 | 2 | 685.0 | 3.3 |
3 | GDP | 1 | 4 | 685.5 | 3.8 |
4 | HEALTH, WHO region | 3 | 6 | 686.3 | 4.6 |
5 | GDP | 3 | 4 | 687.8 | 6.1 |
6 | GDP, WHO region | 3 | 6 | 688.7 | 7.0 |
7 | HEALTH | 3 | 4 | 688.8 | 7.1 |
8 | HEALTH, WHO region | 1 | 6 | 688.9 | 7.2 |
9 | Constant | 1 | 2 | 690.6 | 8.9 |
10 | HEALTH | 1 | 4 | 690.7 | 9.0 |
* Variables of ten models: GDP - three groups based on gross domestic product per capita, HEALTHthree groups based on per capita government expenditure on health, WHO regionthree groups based on WHO region; Region of the Americas (AMR), African region (AFR), and Others. ∗∗ : nσ is the number of standard deviations, σ in model a. † : na is the number of parameters for model a. ‡ : AIC for each model a is calculated as AICa=−2ln(La)+2na and subsequently, AICca is calculated as AICa+2n2a+2nam−na−1 where m is the sample size.ΔAICca=AICca−min(AICc1), where La is the likelihood value for model a. |
We statistically estimated ZIKV arrival time around the world after it first appeared in Brazil in 2015. Taking a similar approach to our previous study that we conducted in real time [36], we modeled inter-country infection spread using effective distance, but, as Figure 1 indicates, there was substantial variation among observed importation times, and we determined that effective distance alone was not an adequate predictor of ZIKV arrival time. To better decipher the importation mechanisms, we also accounted for reporting delay, which we regressed by plausible indicators of country-specific laboratory capacity and reporting, including GDP per capita, health expenditure per capita, and geographic regions. We found that high GDP is a good predictor of short reporting delays. Additionally, reporting delay was dependent on WHO geographic region. ZIKV infection is generally mild and, without substantial laboratory capacity, cases can be underestimated. Herein, we successfully highlighted this feature and identified several variables as important to the data generating process of time from Brazil to reported importation around the world.
There are two major findings from our regression models of reporting delay, which yielded smaller AICs than models using a constant to explain delay. First, the reporting delays were shorter in countries with higher GDP per capita. We chose GDP per capita to reflect the capacity of laboratory testing and surveillance. For similar reasons, we also evaluated health expenditure per capita. Because GDP and health expenditure were correlated, the weaker predictorhealth expenditurewas not included in the final model. Because of this finding, the estimated actual arrival time from the actual start of the Brazil epidemic was longest in countries in the highest tertile of GDP per capita. To the best of our knowledge, this study is the first to identify and decipher country-dependent mechanisms behind reporting delays.
Second, we found that South American countries had shorter reporting delays than other countries. Owing to their geographic proximity to Brazil and an elevated awareness of the virus among South American countries, this is an intuitive finding. We found longer reporting delays among the African countries. This is also in line with our expectation that the laboratory capacity in the African region may be lower relative to other countries. Moreover, owing to the mild nature of ZIKV infection, which is historically endemic in African countries [13], it is probable that there is a high rate of underreporting in this region.
As shown in Figure 4, our study does not fully explain the global variation in arrival time. Rather, owing to the discrete grouping of GDP per capita and other variables, the estimated variation in actual arrival time was escalated compared with the observed (reported) arrival time. As a response to country-specific variations, herein, we showed that laboratory capacity for testing and surveillance, as well as elevated illness awareness and geographic proximity to the origin, are likely to drive diagnosis and reporting rates. Rather than reducing the arrival-time variance, our contribution with this study was to identify the effect of reporting delays on country-specific observed arrival times of the ZIKV epidemic. A critical lesson learned from modeling the 201516 ZIKV pandemic is that the use of effective distance alone is not sufficiently precise to capture the observed patterns of country-specific arrival times; rather, we need to additionally account for case ascertainment, laboratory capacity, and virus awareness to address possible variations in reporting delays and laboratory coverage.
Several technical limitations of our study should be noted. First, even though our linear regression model explored reporting delay by country, ZIKV epidemics include asymptomatic infections; thus, it is possible that several importation events were missed. Second, even though country-specific variation was regressed, our approach adopted discrete classification of GDP per capita into three groups, but this approach could not fully explain variations in observed arrival times. Additional mechanisms need to be identified via country-specific analyses. Third, we used static network data to capture human mobility patterns, but it is possible that global spread in 2016 was enhanced due to the Olympic Games, which were held in Rio de Janeiro that year [42]. Lastly, we estimated the ZIKV importation risk in each country as a risk of importation from Brazil. Importations from other areas that experienced a contemporaneous ZIKV epidemic (e.g., South Pacific) were ignored.
Despite these limitations, we argue that, with this study, we have successfully elucidated key importation mechanisms, including diffusion by human migration and reporting delay. The time required for ZIKV to arrive and be reported in a country was determined not only by global airline travel patterns, but also by other factors, most notably heterogeneous laboratory and reporting capacities.
HN received funding support from the Japan Agency for Medical Research and Development (JP18fk0108050); Japan Society for the Promotion of Science KAKENHI (Grant Numbers 16KT0130, 17H04701, 17H05808 and 18H04895); Health and Labour Sciences Research Grant (H28-AIDS-General-001); the Inamori Foundation; the Telecommunication Advancement Foundation; and the Japan Science and Technology Agency (JST) CREST program (JPMJCR1413). HL received financial support from the Japan Society for the Promotion of Science, Program for Advancing Strategic International Networks to Accelerate the Circulation of Talented Researchers; and the Japan Society for the Promotion of Science KAKENHI (Grant number 18H06385). The funders had no role in the study design, data collection and analysis, decision to publish, or preparation of the manuscript. We also thank Ashley Hazel, PhD, from Edanz Group (www.edanzediting.com/ac) for editing a draft of this manuscript.
The authors declare that they have no conflict of interest.
[1] | G. Benelli and H. Mehlhorn, Declining malaria, rising of dengue and Zika virus: insights for mosquito vector control, Parasitol Res., 115 (2016), 17471754. |
[2] | G. W. Dick, Zika virus. II. Pathogenicity and physical properties, Trans. R. Soc. Trop. Med. Hyg., 46 (1952), 521–534. |
[3] | I. I. Bogoch, O. J. Brady, M. U. G. Kraemer, et al., Anticipating the international spread of Zika virus from Brazil, Lancet, 387 (2016), 335336. |
[4] | E. M. Netto, A. Moreira-Soto, C. Pedroso, et al., High Zika virus seroprevalence in Salvador, Northeastern Brazil limits the potential for further outbreaks, MBio, 8 (2017), e01390–17. |
[5] | D. P. Shutt, C. A. Manore, S. Pankavich, et al., Estimating the reproductive number, total outbreak size, and reporting rates for Zika epidemics in South and Central America, Epidemics, 21 (2017), 63–79. |
[6] | L. Dinh, G. Chowell, K. Mizumoto, et al., Estimating the subcritical transmissibility of the Zika outbreak in the State of Florida, USA, 2016, Theor. Biol. Med. Model., 13 (2016), 20. |
[7] | A. J. Kucharski, S. Funk, R. M. Eggo, et al., Transmission dynamics of Zika virus in island popu- lations: a modelling analysis of the 201314 French Polynesia outbreak, PLoS Negl. Trop. Dis., 10 (2016), e0004726. |
[8] | H. Nishiura, R Kinoshita, K. Mizumoto, et al., Transmission potential of Zika virus infection in the South Pacific, Int. J. Infect. Dis., 45 (2016), 95–97. |
[9] | N. H. Ogden, A. Fazil, D. Safronetz, et al., Risk of travel-related cases of Zika virus infection is predicted by transmission intensity in outbreak-affected countries, Parasit. Vectors, 10 (2017), 41. |
[10] | S. Cauchemez, M. Besnard, P. Bompard, et al., Association between Zika virus and microcephaly in French Polynesia, 20132015: a retrospective study, Lancet, 387 (2016), 2125–2132. |
[11] | J. Mlakar, M. Korva, N. Tul, et al., Zika virus associated with microcephaly, N. Engl. J. Med., 374 (2016), 951–958. |
[12] | V. Sikka, V. K. Chattu, R. K. Popli, et al., The emergence of Zika virus as a global health security threat: A review and a consensus statement of the INDUSEM Joint working Group (JWG). J. Glob. Infect. Dis., 8 (2016), 3–15. |
[13] | R. W. Malone, J. Homan, M. V. Callahan, et al., Zika Virus: Medical Countermeasure Develop- ment Challenges, PLoS Negl. Trop. Dis., 10 (2016), 1–26. |
[14] | S. A. Rasmussen, D. J. Jamieson, M. A. Honein, et al., Zika virus and birth defects reviewing the evidence for causality, N. Engl. J. Med., 374 (2016), 1981–1987. |
[15] | F. J. Colón-González, C. A. Peres, C. S. S˜ ao Bernardo, et al., After the epidemic: Zika virus projections for Latin America and the Caribbean, PLoS Negl. Trop. Dis., 11 (2017), e0006007. |
[16] | N. D. Grubaugh, J. T. Ladner, M. U. G. Kraemer, et al., Genomic epidemiology reveals multiple introductions of Zika virus into the United States, Nature, 546 (2017), 401–405. |
[17] | J. P. Messina, M. U. Kraemer, O. J. Brady, et al., Mapping global environmental suitability for Zika virus, Elife, 5 (2016), e15272. |
[18] | R. N. Faria, J. Quick, I. Morales, et al., Establishment and cryptic transmission of Zika virus in Brazil and the Americas, Nature, 546 (2017), 406–410. |
[19] | World Health Organization, Situation ReportZika virus, microcephaly, GuillainBarr syn- drome, 2017. Available from: http://apps.who.int/iris/bitstream/10665/254714/1/ zikasitrep10Mar17-eng.pdf?ua=1. |
[20] | I. I. Bogoch, O. J. Brady, M. U. G. Kraemer, et al., Potential for Zika virus introduction and transmissioninresource-limitedcountriesinAfricaandtheAsia-Pacificregion: amodellingstudy, Lancet Infect. Dis, 16 (2016), 1237–1245. |
[21] | R. Mgling, H. Zeller, J. Revez, et al., ZIKV reference laboratory group and C. Reusken, Status, quality and specific needs of Zika virus (ZIKV) diagnostic capacity and capability in National Reference Laboratories for arboviruses in 30 EU/EEA countries, May 2016, Euro. Surveill., 22 (2017), 30609. |
[22] | J. M. Marshall, S. L. Wu, H. M. Sanchez, et al., Mathematical models of human mobility of relevance to malaria transmission in Africa, Sci. Rep., 8 (2018), 1–12. |
[23] | J. Riou, C. Poletto and P. Y. Bolle, A comparative analysis of Chikungunya and Zika transmission, Epidemics, 19 (2017), 43–52. |
[24] | N. M. Ferguson, Z. M. Cucunub, I. Dorigatti, et al., Countering the Zika epidemic in Latin Amer- ica, Science, 353 (2016), 353–354. |
[25] | K. Sun, Q. Zhang, A. Pastore-Piontti, et al., Quantifying the risk of local Zika virus transmission in the contiguous US during the 2015-2016 ZIKV epidemic, BMC Med., 16 (2018), 195. |
[26] | S. Towers, F. Brauer, C. Castillo-Chavez, et al., Estimate of the reproduction number of the 2015 Zika virus outbreak in Barranquilla, Colombia, and estimation of the relative role of sexual trans- mission, Epidemics, 17 (2016), 50–55. |
[27] | Q. Zhang, K. Sun, M. Chinazzi, et al., Spread of Zika virus in the Americas, Proc. Natl. Acad. Sci. USA, 114 (2017), E4334–E4343. |
[28] | L. T. Keegan, J. Lessler and M. A. Johansson, Quantifying Zika: advancing the epidemiology of Zika with quantitative models, J. Infect. Dis., 216 (2017), S884–S890. |
[29] | S. M. Moghadas, A. Shoukat, A. L. Espindola, et al., Asymptomatic transmission and the dynam- ics of Zika infection, Sci. Rep., 7 (2017), 5829. |
[30] | A. Wiratsudakul, P. Suparit and C. Modchang, Dynamics of Zika virus outbreaks: an overview of mathematical modeling approaches, Peer. J., 6 (2018), e4526. |
[31] | E. Massad, S. H. Tan, K. Khan, et al., Estimated Zika virus importations to Europe by travellers from Brazil, Glob. Health. Action, 9 (2016), 31669. |
[32] | D. Balcan, B. Gonalves, H. Hu, et al., Modeling the spatial spread of infectious diseases: the GLobal Epidemic and Mobility computational model, J. Comput. Sci., 1 (2010), 132–145. |
[33] | D. Balcan, V. Colizza, B. Goncalves, et al., Multiscale mobility networks and the spatial spreading of infectious diseases, Proc. Natl. Acad. Sci. USA, 106 (2009), 21484–21489. |
[34] | D. Brockmann and D. Helbing, The hidden geometry of complex , network-driven contagion phe- nomena, Science, 342 (2013), 1337–1342. |
[35] | M. R. T. Nunes, G. Palacios, N. R. Faria, et al., Air travel is associated with intracontinental spread of dengue virus serotypes 13 in Brazil, PLoS Negl. Trop. Dis., 8 (2014), e2769. |
[36] | K. Nah, K. Mizumoto, Y. Miyamatsu, et al., Estimating risks of importation and local transmission of Zika virus infection, Peer. J., 4 (2016), e1904. |
[37] | J. Patokallio, Openflights data, 2016. Available from: http://openflights.org/. |
[38] | Central Intelligence Agency, The World Factbook 2017. Available from: https://www.cia. gov/library/publications/the-world-factbook/rankorder/2004rank.html. |
[39] | World Health Organization, Global Health Observatory data repository 2017. Avail- able from: http://data.un.org/Data.aspx?q=Health+expenditure&d=WHO&f=MEASURE_ CODE%3aWHS7_108. |
[40] | S. Otsuki and H. Nishiura, Reduced Risk of Importing Ebola Virus Disease because of Travel Restrictions in 2014: A Retrospective Epidemiological Modeling Study, PLoS One, 11 (2016), e0163418. |
[41] | K. Nah, S. Otsuki, G. Chowell, et al., Predicting the international spread of Middle East respiratory syndrome (MERS), BMC Infect. Dis., 16 (2016), 356. |
[42] | M. N. Burattini, F. A. B. Coutinho, L. F. Lopez, et al., Potential exposure to Zika virus for foreign tourists during the 2016 Carnival and Olympic Games in Rio de Janeiro, Brazil, Epidemiol. Infect., 144 (2016), 1904–1906. |
1. | Wei Gao, Behzad Ghanbari, Haci Mehmet Baskonus, New numerical simulations for some real world problems with Atangana–Baleanu fractional derivative, 2019, 128, 09600779, 34, 10.1016/j.chaos.2019.07.037 | |
2. | Clarisse Lins de Lima, Ana Clara Gomes da Silva, Giselle Machado Magalhães Moreno, Cecilia Cordeiro da Silva, Anwar Musah, Aisha Aldosery, Livia Dutra, Tercio Ambrizzi, Iuri V. G. Borges, Merve Tunali, Selma Basibuyuk, Orhan Yenigün, Tiago Lima Massoni, Ella Browning, Kate Jones, Luiza Campos, Patty Kostkova, Abel Guilhermino da Silva Filho, Wellington Pinheiro dos Santos, Temporal and Spatiotemporal Arboviruses Forecasting by Machine Learning: A Systematic Review, 2022, 10, 2296-2565, 10.3389/fpubh.2022.900077 |
Model (a) | Variable* | n∗∗σ | n†a | AICc‡a | Δ AICc‡a |
1 | GDP, WHO region | 1 | 6 | 681.7 | 0 |
2 | Constant | 3 | 2 | 685.0 | 3.3 |
3 | GDP | 1 | 4 | 685.5 | 3.8 |
4 | HEALTH, WHO region | 3 | 6 | 686.3 | 4.6 |
5 | GDP | 3 | 4 | 687.8 | 6.1 |
6 | GDP, WHO region | 3 | 6 | 688.7 | 7.0 |
7 | HEALTH | 3 | 4 | 688.8 | 7.1 |
8 | HEALTH, WHO region | 1 | 6 | 688.9 | 7.2 |
9 | Constant | 1 | 2 | 690.6 | 8.9 |
10 | HEALTH | 1 | 4 | 690.7 | 9.0 |
* Variables of ten models: GDP - three groups based on gross domestic product per capita, HEALTHthree groups based on per capita government expenditure on health, WHO regionthree groups based on WHO region; Region of the Americas (AMR), African region (AFR), and Others. ∗∗ : nσ is the number of standard deviations, σ in model a. † : na is the number of parameters for model a. ‡ : AIC for each model a is calculated as AICa=−2ln(La)+2na and subsequently, AICca is calculated as AICa+2n2a+2nam−na−1 where m is the sample size.ΔAICca=AICca−min(AICc1), where La is the likelihood value for model a. |
Model (a) | Variable* | n∗∗σ | n†a | AICc‡a | Δ AICc‡a |
1 | GDP, WHO region | 1 | 6 | 681.7 | 0 |
2 | Constant | 3 | 2 | 685.0 | 3.3 |
3 | GDP | 1 | 4 | 685.5 | 3.8 |
4 | HEALTH, WHO region | 3 | 6 | 686.3 | 4.6 |
5 | GDP | 3 | 4 | 687.8 | 6.1 |
6 | GDP, WHO region | 3 | 6 | 688.7 | 7.0 |
7 | HEALTH | 3 | 4 | 688.8 | 7.1 |
8 | HEALTH, WHO region | 1 | 6 | 688.9 | 7.2 |
9 | Constant | 1 | 2 | 690.6 | 8.9 |
10 | HEALTH | 1 | 4 | 690.7 | 9.0 |
* Variables of ten models: GDP - three groups based on gross domestic product per capita, HEALTHthree groups based on per capita government expenditure on health, WHO regionthree groups based on WHO region; Region of the Americas (AMR), African region (AFR), and Others. ∗∗ : nσ is the number of standard deviations, σ in model a. † : na is the number of parameters for model a. ‡ : AIC for each model a is calculated as AICa=−2ln(La)+2na and subsequently, AICca is calculated as AICa+2n2a+2nam−na−1 where m is the sample size.ΔAICca=AICca−min(AICc1), where La is the likelihood value for model a. |