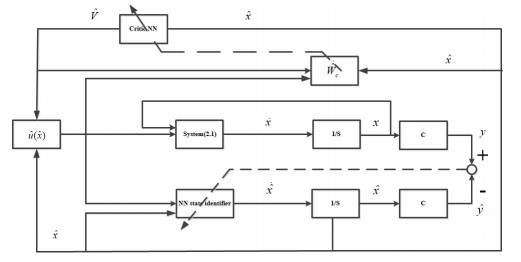
Steel is a versatile metal, got a wide range of applications in all the fields of engineering and technology. Generally, low carbon steels are tough and high alloy carbon steels are hard in nature. Certain applications demand both properties in the same steel. Carburization is one such technique that develops hard and wear resistant surfaces with a soft core. The objective of this work is to study the influence of post carburizing treatment (normalizing) on three grades of steels (EN 3, 20MnCr5, and EN 353). Post carburizing treatments are necessary to overcome the adverse effects of carburization alone. Here carburization was carried out in the propane atmosphere by heating the gas carburizing furnace to 930 ℃ for more than a day. Normalizing was carried out at 870 ℃ for 1 h and cooled in air. Tensile, hardness, Charpy impact tests along with SEM (scanning electron microscopy) and EDAX (energy dispersive X-ray analysis) were conducted to analyze the phase transformation, failure mode analysis in all the samples. Carburized steels displayed the formation of ferrite, pearlite, and sometimes bainite phases in the core and complete coarse pearlite in the case regions, whereas in the post carburized steels, increased amount of ferrite, fine pearlite, and bainite in the core and fine pearlite with traces of bainite in the case region was observed. Normalizing also refines the grain with increased UTS (ultimate tensile strength), hardness, and impact resistance. EN 353 showed higher UTS among the steels with 898 MPa after carburization and 1370 MPa after normalizing treatment. Maximum hardness of 48 HRC was observed in 20MnCr5 and toughness was superior in EN 3 with energy absorbed during test i.e., 8 and 12 J before and after normalizing treatment. Based on the fracture surface analysis, in EN 353 steel, a finer array of dimples with voids and elongated bigger clustered dimples containing ultrafine dimples array are observed in the core and case respectively during carburizing whereas, more density of river pattern and cleavage failure (brittle) are observed in the core and case respectively after post carburizing (normalizing) treatment. There is a reduction in the ductility of the steels after post carburizing treatment. It was observed that normalizing treatment produces superior mechanical properties in the carburized steels by grain refinement and strong microstructures like bainite. Normalizing as post carburizing treatment can be recommended for engineering applications where ductile core and hard surface are of great importance.
Citation: Pavan Hiremath, Gowrishankar M. C., Manjunath Shettar, Sathyashankara Sharma, Jayashree P. K., Suhas Kowshik. Influence of normalizing post carburizing treatment on microstructure, mechanical properties and fracture behavior of low alloy gear steels[J]. AIMS Materials Science, 2021, 8(5): 836-851. doi: 10.3934/matersci.2021051
[1] | Yuhang Yao, Jiaxin Yuan, Tao Chen, Xiaole Yang, Hui Yang . Distributed convex optimization of bipartite containment control for high-order nonlinear uncertain multi-agent systems with state constraints. Mathematical Biosciences and Engineering, 2023, 20(9): 17296-17323. doi: 10.3934/mbe.2023770 |
[2] | Zichen Wang, Xin Wang . Fault-tolerant control for nonlinear systems with a dead zone: Reinforcement learning approach. Mathematical Biosciences and Engineering, 2023, 20(4): 6334-6357. doi: 10.3934/mbe.2023274 |
[3] | Vladimir Djordjevic, Hongfeng Tao, Xiaona Song, Shuping He, Weinan Gao, Vladimir Stojanovic . Data-driven control of hydraulic servo actuator: An event-triggered adaptive dynamic programming approach. Mathematical Biosciences and Engineering, 2023, 20(5): 8561-8582. doi: 10.3934/mbe.2023376 |
[4] | Dongxiang Gao, Yujun Zhang, Libing Wu, Sihan Liu . Fixed-time command filtered output feedback control for twin-roll inclined casting system with prescribed performance. Mathematical Biosciences and Engineering, 2024, 21(2): 2282-2301. doi: 10.3934/mbe.2024100 |
[5] | Na Zhang, Jianwei Xia, Tianjiao Liu, Chengyuan Yan, Xiao Wang . Dynamic event-triggered adaptive finite-time consensus control for multi-agent systems with time-varying actuator faults. Mathematical Biosciences and Engineering, 2023, 20(5): 7761-7783. doi: 10.3934/mbe.2023335 |
[6] | Yuhan Su, Shaoping Shen . Adaptive predefined-time prescribed performance control for spacecraft systems. Mathematical Biosciences and Engineering, 2023, 20(3): 5921-5948. doi: 10.3934/mbe.2023256 |
[7] | K. Renee Fister, Jennifer Hughes Donnelly . Immunotherapy: An Optimal Control Theory Approach. Mathematical Biosciences and Engineering, 2005, 2(3): 499-510. doi: 10.3934/mbe.2005.2.499 |
[8] | Tianqi Yu, Lei Liu, Yan-Jun Liu . Observer-based adaptive fuzzy output feedback control for functional constraint systems with dead-zone input. Mathematical Biosciences and Engineering, 2023, 20(2): 2628-2650. doi: 10.3934/mbe.2023123 |
[9] | Siyu Li, Shu Li, Lei Liu . Fuzzy adaptive event-triggered distributed control for a class of nonlinear multi-agent systems. Mathematical Biosciences and Engineering, 2024, 21(1): 474-493. doi: 10.3934/mbe.2024021 |
[10] | Yong Xiong, Lin Pan, Min Xiao, Han Xiao . Motion control and path optimization of intelligent AUV using fuzzy adaptive PID and improved genetic algorithm. Mathematical Biosciences and Engineering, 2023, 20(5): 9208-9245. doi: 10.3934/mbe.2023404 |
Steel is a versatile metal, got a wide range of applications in all the fields of engineering and technology. Generally, low carbon steels are tough and high alloy carbon steels are hard in nature. Certain applications demand both properties in the same steel. Carburization is one such technique that develops hard and wear resistant surfaces with a soft core. The objective of this work is to study the influence of post carburizing treatment (normalizing) on three grades of steels (EN 3, 20MnCr5, and EN 353). Post carburizing treatments are necessary to overcome the adverse effects of carburization alone. Here carburization was carried out in the propane atmosphere by heating the gas carburizing furnace to 930 ℃ for more than a day. Normalizing was carried out at 870 ℃ for 1 h and cooled in air. Tensile, hardness, Charpy impact tests along with SEM (scanning electron microscopy) and EDAX (energy dispersive X-ray analysis) were conducted to analyze the phase transformation, failure mode analysis in all the samples. Carburized steels displayed the formation of ferrite, pearlite, and sometimes bainite phases in the core and complete coarse pearlite in the case regions, whereas in the post carburized steels, increased amount of ferrite, fine pearlite, and bainite in the core and fine pearlite with traces of bainite in the case region was observed. Normalizing also refines the grain with increased UTS (ultimate tensile strength), hardness, and impact resistance. EN 353 showed higher UTS among the steels with 898 MPa after carburization and 1370 MPa after normalizing treatment. Maximum hardness of 48 HRC was observed in 20MnCr5 and toughness was superior in EN 3 with energy absorbed during test i.e., 8 and 12 J before and after normalizing treatment. Based on the fracture surface analysis, in EN 353 steel, a finer array of dimples with voids and elongated bigger clustered dimples containing ultrafine dimples array are observed in the core and case respectively during carburizing whereas, more density of river pattern and cleavage failure (brittle) are observed in the core and case respectively after post carburizing (normalizing) treatment. There is a reduction in the ductility of the steels after post carburizing treatment. It was observed that normalizing treatment produces superior mechanical properties in the carburized steels by grain refinement and strong microstructures like bainite. Normalizing as post carburizing treatment can be recommended for engineering applications where ductile core and hard surface are of great importance.
The research on optimal control for nonlinear systems plays a significant role in industry and military fields. Due to the influence of the environment and the limitations of the engineering system, it is very tough to maximize or minimize the performance index of the controlled system in practical. Therefore, optimization problem is a difficult problem in current control field, and has gradually been the focus of attention. The optimal control problems of nonlinear systems are finally transformed into the solutions of Hamilton-Jacobi-Bellman (HJB) partial differential equations.
However, because the HJB equation is a nonlinear partial differential equation, it is difficult to obtain an analytical solution. Therefore, how to obtain the analytical solution of HJB equation then realize the optimization performance index of the system, is the key points to resolve the optimization issue.
In order to avoid the problems encountered in solving HJB equation, Kalman [1] proposed the inverse-optimal-based control method for the first time. On the basis of [1], Freeman and Kokotovic [2] studied inverse optimization control of nonlinear systems. The basic idea of inverse optimization control is not to minimize the cost function by designing the controller, but to minimize the cost function by designing the appropriate control Lyapunov function(CLF). Therefore, the solution of HJB equation comes down to seeking the CLF of the controlled system, thus avoiding the shortcoming of directly solving HJB equation.
Besides, aiming at the above problems, Bellman [3] proposed the theory of dynamic programming (DP). However, the issue of "dimension disaster" would be caused in the process of DP control design, that is, the complexity of space storage and computation increases exponentially with the increase of the dimension of control vectors and states. Therefore, in order to overcome the phenomenon of "dimension disaster" in the process of optimal control design, an adaptive optimal control design method combining NNs is proposed by Werbos [4], which is called RL or adaptive/ approximate dynamic programming (ADP). In the control field, RL can effectively solve the "dimension disaster" problem in DP. In [5], Werbos retrospected the classic econometric approach and proposed a robust method. In [6], Werbos defined a more limited design called "brain-like intelligent control", it discusses the brain as a member of intelligent control, which implies a property to be sought in future research.
Since then, inspired by [4], large amounts of optimal control methods via APD have been developed, see [7,8]. Among them, for continuous-time (CT) systems, in offline situations, Abu-Khalaf and Lewis [9] presented an offline algorithm via RL to solve the optimal control issue of CT nonlinear systems. Since the offline control algorithms cannot be adopted to adjust online in real time, thus, to overcome this disadvantage, Vamvoudak and Lewis [10] proposed an online adaptive method via policy iteration. In [11], Li et al. investigated the Lyapunov stability problem for impulsive systems via event-triggered impulsive control. In [12], Li et al. considerd a class of nonlinear impulsive systems with delayed impulses, based on impulsive control theory and the ideas of average dwell-time (ADT), a set of Lyapunov-based sufficient conditions for globally exponential stability were obtained. However, we need to know the accurate knowledge of CT nonlinear systems in [9,10]. Since nonlinear systems usually contain uncertain nonlinear functions, it it difficult to acquire the analytical solution of the HJB equation.
In order to solve this problem, by choosing an appropriate cost function to reflect uncertainty regulation, the authors in [13] proposed a robust optimization controller design strategy based on an online strategy iterative algorithm for a class of continuous nonlinear systems with nonlinearities. Zhang et al. [14] designed a new data-driven robust identified optimization tracking controller via the acquired data-driven model for a kind of nonlinear CT systems.
Consider a class of affine nonlinear system as:
˙x(t)=g(x(t))u(t)+f(x(t)),x(0)=x0 | (2.1) |
where f(x) and g(x) are the uncertain smooth functions, which satisfy that f(0)=0, g(0)=0. u is the control input, x∈Rn is the state vector. For the above system, some adaptive optimization control strategies have been proposed.
In [15], for a class of affine nonlinear CT systems with unknown internal dynamics, Liu and Wang et al. developed an online method based on ADP, which constructed a critic neural network to facilitate the solution of the modified HJB equation. In [16], Liu et al. developed an online optimization control algorithm for CT affine nonlinear systems with infinite horizon cost. And in [17], Wen and Chen et al. studied an adaptive optimized tracking control method via RL algorithm and NNs.
For [16,17], value function is selected as:
V(z)=∫∞tr(z(r),u(z))dτ | (2.2) |
where r(z,u)=zT(t)Q(x)z(t)+uTu is the value function, and Q(x)=q(x)qT(x)∈Rn×n is a positive definite matrix. The HJB equation is defined as:
H(z,u,Vz)=VTz(z)z(t)+r(z,u)=VTz(f(x)+g(x)u−yd(t))+zTQ(x)z(t)+uTu | (2.3) |
where Vz∈Rn is the partial gradient of Vz, yd(t)∈Rn is the ideal tracking trajectory, z(t)=x(t)−yd(t) is the tracking error. When considering the input constraint control and saturation constraints control issue, Liu and Yang developed a robust optimal adaptive optimal control method via RL for a kind of uncertain nonlinear systems. In [18,19], there exists a symmetric definite matrix Q, and the value function is selected as:
V(x(t))=∫∞t[xTQx+ϖ(u)]ds,(s≥t) | (2.4) |
where ϖ(u) is positive. For the sake of solving the constraint control issue, define ϖ(u) as:
ϖ(u)=2κ∫u0(ψ−1(υ/κ))TRdv=2κm∑i=1∫u0(ψ−1(υi/κ))TRidv | (2.5) |
where R=diag[r1,⋯,rn] with ri>0, (i=1,⋯,m), ψ(⋅) is a bounded one-to-one function with |ψ(⋅)|≤1, ψ∈Rm, ψ−1=(ψ−1)T, ψ−1(υ/κ)=[ψ−1(υ1/κ),⋯,ψ−1(υm/κ)]T, u(x)∈Ξ, Ξ={u|u∈Rm,|ui|≤κ,i=1,2,⋯,m}, κ>0 is a constant. Define the HJB equation and the value function as:
H(x,Vx,u)=VTx(f(x)+g(x)u)+r(x,u) | (2.6) |
where Vx∈Rn is the partial derivative of V(x) with respect to x.
In the previous article, since there exist the unknown nonlinear functions f(x) and g(x), the analytic solution of the equation cannot be received when resolving the HJB equation. Because of their properties and fault tolerance, attributes of nonlinearity, adaptivity, the identified solution of the HJB equation can be obtained symmetric via NNs.
Because the system (2.1) contains unknown dynamics, we can identify the system for receiving the optimal control. In [20], Yang et al. presented identifier-actor-critic (IAC) structure, where the actor NN is carried out control actions, and critic NN is employed to estimated these actions, and then returns the evaluations to actor, and the dynamics of uncertain system robust dynamic can be approximate by NN identifiers. From system (2.1), we have that:
˙x=g(x)u+f(x)=g(x)u+Ax+ϝ(x) | (2.7) |
where A∈Rn×n is a certain constant matrix, ϝ(x)=f(x)−Ax.
A NN is applied to identify ϝ(x) as follows:
ϝ(x)=WT1σ(x)+ε1(x) | (2.8) |
where ε1(x)∈Rn is the NN function reestablishment error, σ(x) is the activation function, WT1∈Rn×n is the NN weight. By using (2.8), (2.7) can be developed by:
˙x(t)=g(x)u+ε1(x)+Ax+WT1σ(x) | (2.9) |
The NN identifier is designed as:
˙ˆx(t)=g(ˆx)u+v(t)+Aˆx+ˆWT1σ(ˆx) | (2.10) |
where ˆx∈Rn is the identifier NN state, ˆW1∈Rn×n is weight estimation, and v(t) is the robust feedback term.
The optimal value function can be expressed by NN as:
V∗(ˆx)=WTϕ(ˆx)+εv(ˆx) | (2.11) |
The optimal control can be expressed by NN as:
u∗(ˆx)=−12R−1gT(ˆx)(ϕ′(ˆx)TW+ε′v(ˆx)T) | (2.12) |
where εv(⋅)∈R is the function reestablishment error, ϕ(ˆx)=[ϕ1(ˆx),ϕ2(ˆx),⋯,ϕN(ˆx)]T∈RN, ϕ′(ˆx)=△∂ϕ(ˆx)∂ˆx and W∈RN are uncertain desired NN weights, N is the number of neurons.
The critic-actor ˆV(ˆx) and ˆu, which can learn the optimization value function and adjust the optimization control online, is expressed as:
ˆV(ˆx)=ˆWTcϕ(ˆx) | (2.13) |
ˆu(ˆx)=−12R−1gT(ˆx)ϕ′T(ˆx)^Wa | (2.14) |
where ˆWc(t)∈RN and ˆWa(t)∈RN estimate the ideal weights of the critic-actor NNs. Whereas the system dynamics are estimated online by using the identification error ˜x(t)=x(t)−ˆx(t). The overall planning diagram of the control algorithm is given in Figure 1.
Besides Bhasin et al. [21] proposed an online adaptive solution via RL for the unbounded optimization control nonlinear systems with CT uncertain problem. The advantage of using the IAC structure is that the learning of critics, actors, and identifiers is successive and simultaneous, removing the knowledge of system drift dynamics.
However, the above proposed control design algorithm for the affine nonlinear systems cannot be used to solve the optimal control issues for unmatching condition nonlinear systems, because it cannot guarantee the optimization of each subsystem.
The above research methods on affine nonlinear systems cannot be applied to nonlinear systems with unmatching conditions and the optimality of each subsystem can not be guaranteed. In order to solve the problem of unmatching conditions, we used the backstepping technology, which can also optimize each subsystem.
Consider the following strict feedback nonlinear systems as:
{˙xi=fi(¯xi)+xi+1,i=1,2,⋯,n−1˙xn=fn(¯xn)+uy=x1 | (3.1) |
where u and y are the control input and output, x is the state, ¯xi=[x1,x2,⋯,xi], is the system state vector. fi(⋅) is the uncertain nonlinear function, which satisfies f(0)=0.
In 1995, Kristic [22] firstly proposed the backstepping technology. The design idea of the backstepping algorithm is as follows: for systems that satisfy strict feedback control structures, via the backstepping algorithm, the Lyapunov function and controller are constructed in a systematic way. Then, for each subsystem, local Lyapunov function and intermediate control function are designed successively until the design of the whole controller is completed.
For the sake of solving the control issue for unmatching nonlinear systems (3.1), Wen et al. [23] first proposed an optimized backstepping control technology, under the backstepping framework, we can ensure that each subsystem can be optimized. Based on [23], for a kind of nonlinear large-scale systems with strict-feedback structure, Tong et al. [24] proposed the fuzzy decentralized adaptive optimal control, and used FLS to identify the uncertain nonlinear function of the systems. And in [25], for a quarter of the car active electric suspension systems, Li et al. addressed the output-feedback adaptive NN optimization control issue.
Because there are unknown nonlinear functions, the updating laws and learning laws designed for the above systems are very complex. In order to solve this problem, in [26], Wen et al. proposed a simplified RL algorithm, which generates a negative gradient of a simple positive function from the partial derivative of HJB equation, and derives a new law from the negative gradient.
Define the Hamiltonian's approximation error as:
E=H(ˆz,u,ˆV∗ˆz)−H(z,u∗,V∗ˆz)=H(ˆz,u,ˆV∗ˆz) | (3.2) |
where V∗ˆz(ˆz) is the gradient of V∗(ˆz), u∗ is the optimal control. Since V∗ˆz(ˆz) and u∗ contain the unknown part V0ˆz(ˆz), which can be approximated on a compact set by NNs as:
V0ˆz(ˆz)=Θ∗TVφV(ˆz)+εV(ˆz) | (3.3) |
Since Θ∗V is an uncertain constant vector, it is not available in practical control, RL algorithm is implemented by both critic-actor NNs.
The learning law of critic NN is designed as:
˙ˆΘVc(t)=−kcφV(ˆz)φTV(ˆz)ˆΘVc(t) | (3.4) |
where kc is the critic network learning rate, ˆΘVc(t) is the critic NN weight.
The learning law of actor NN is designed as:
˙ˆΘVa(t)=−φV(ˆz)φTV(ˆz)(ka(ˆΘVa(t)−ˆΘVc(t))+kcˆΘVc(t)) | (3.5) |
where ka is the actor network learning rate, ˆΘVa(t) is the actor NN weight, ka>kc>0.
In accordance with the above description, the optimal solution ˆα(ˆz) is supposed to meet E(t)=H(ˆz,u,ˆV∗ˆz)→0.
If H(ˆz,u,ˆV∗ˆz) is held and exist the unique solution, then it is equivalent to the following equation holds:
∂H(ˆz,u,ˆV∗ˆz)∂ˆΘVa=φVφTV(ˆΘTVa(t)−ˆΘTVc(t))=0 | (3.6) |
The positive definite function is designed as:
P(t)=(ˆΘVa(t)−ˆΘVc(t))T(ˆΘVa(t)−ˆΘVc(t)) | (3.7) |
Clearly, the Eq (3.6) is the equivalent to P(t)=0. Since ∂P(t)∂ˆΘVa(t)=−∂P(t)∂ˆΘVc(t)=2(ˆΘVa(t)−ˆΘVc(t)), we can get
∂P(t)dt=∂P(t)∂ˆΘVc(t)⋅ˆΘVc(t)+∂P(t)∂ˆΘVa(t)⋅ˆΘVa(t)=−kc∂P(t)∂ˆΘVc(t)φVφTVˆΘTVc(t)−∂P(t)∂ˆΘVc(t)φVφTV[ka(ˆΘVa(t)−ˆΘVc(t))+kcˆΘVc(t)]=−ka2∂P(t)∂ˆΘa(t)φVφTV∂P(t)∂ˆΘa(t)≤0 | (3.8) |
In [27], for nonlinear lithium battery systems, Pei et al. addressed adaptive NN output feedback optimization control problem, and the stability of the nonlinear lithium battery is proved.
On the basis of [26], under the frame of backstepping control, some simplified-based adaptive optimization control algorithms have been proposed, which require construct all intermediate control functions and the actual control function of backstepping to be the optimization controls, hence, RL is performed in each subsystem (see Figure 2).
In [28], Wen et al. addressed optimization control method for nonlinear strict-feedback systems with unknown functions. In [29], for second-order unknown nonlinear multiagent systems, Lan et al. proposed a distributed time-varying optimization formation protocol based on an adaptive NN state observer. In [30], Xiao et al. addressed the distributed optimization containment control issue for multiple nonholonomic mobile robots differential game.
It is worth mentioning that system states usually need to be confined within some preselected compact sets due to the physical limitations of actual systems. For real systems, in [31], Jiang and Lou considered the input-to-state stability (ISS) of delayed systems with bounded-delay impulses. In [32], for a hydraulic servo actuator (HSA) with sensor faults, Vladimir and Ljubisa investigated the mechanism for the fault estimation (FE) problem. However, methods in [29,30] could not solve the actual constraint problem. To solve this problem, various state-constrained control methodologies is discussed.
Aiming at strict-feedback nonlinear systems, which contain immeasurable states and internal dynamics, Li et al. [33] proposed an output-feedback adaptive NN optimization control design. Under the backstepping control design, there will be coupling terms or cross terms at each step, which will lead to that each subsystem is not optimal. Therefore, state constraints should be introduced to make the coupling terms bounded to ensure that each subsystem is optimal. And all the states are limited in the compact sets, that is, |xi|<kci, where kci>0.
The neoteric barrier optimization performance index functions for subsystems are designed to ensure that the system state does not violate the constraint bounds and achieves the optimization control objective, which is selected as:
J(z(t))=limτ→∞1τ∫τtq(z((t),α(z)))dz | (3.9) |
where τ is the terminal time, q(z,α)=ξlog[k4b/(k4b−z4)]+r(α)2, ξ>0 is a constant, α is the intermediate control function, The following Hamiltonian can be derived as:
H(ˆz,u,ˆV∗ˆz)=ξlogk4b(k4b−z4)+r(α)2+dV∗(z)dz(α∗+g(x)−yr) | (3.10) |
According to the algorithm presented in [34], for power systems with stochastic character, Li et al. designed the adaptive NN optimal tracking control to resolve the issue of state constraints and uncertain nonlinear dynamics. In [35], Li et al. put forward an adaptive NN optimized output-feedback control method to solve the issue of unknown nonlinear dynamics and input saturation. In [36], for uncertain nonlinear systems with time-varying full state constraints, input saturation and unknown control direction, Wu and Xie employed asymmetric barrier Lyapunov functions, the auxiliary subsystem and the Nussbaum gain technique.
Based on the above published works, some adaptive optimal control methods via backstepping control have also been applied to practical systems, for example, see [37,38]. In [37], Li et al. presented an adaptive NN optimized control strategy for full vehicle active suspension system. And in [38], Li et al. studied adaptive optimal formation control approach for second-order stochastic multi-agent system, which contains unknown nonlinear dynamics.
Based on the inverse optimization control method in [1], Ezal et al. [38] proposed a new robust backstepping inverse optimal control design, which achieved both local optimization and global inverse optimization. For a class of nonlinear uncertain strict feedback systems, Li et al. [39] designed adaptive fuzzy inverse optimization control by establishing an equivalent system and an auxiliary system.
System (2.1) can be rewritten as the following nonlinear system:
˙x=G(x)u+F(x)+q(x) | (3.11) |
where u∈R is the control input, x is the state vector, x=0 is the equilibrium point of system. q(x) is an uncertain bounded function vector, G(x) and F(x) are smooth function vectors.
Define γ is a class K∞ function, then the derivative of γ exists and it is also a class K∞ function. An auxiliary system is constructed for the nonlinear system (2.1):
˙x=lγ(2|LΔV|R(x))×R−2(x)(LΔV)T(LΔV)2+F(x)+G(x)u | (3.12) |
where V(x) is the control Lyapunov function. L△V=∂△V/∂x, LFVn=∂Vn/∂xF(x), LGVn=∂Vn/∂xG(x).
The cost functional is selected as:
J(u)=supd∈D{limt→∞[∫t0(l(x))+uTRu−γ(d))dτ+E(x)]} | (3.13) |
where D is a set of locally bounded functions of x, R(x) is matrix-valued function, which satisfied that R(x)=R(x)T>0. E(x) and l(x) are positive definite radially unbounded functions.
The fuzzy adaptive inverse optimization control structure is shown in Figure 3.
And in [40], Li et al. studied a fuzzy inverse optimization fuzzy adaptive output feedback control method based on observer for a class of nonlinear strict feedback systems. In [41], Lu et al. addressed a fuzzy adaptive inverse optimization control issue, and a switching inverse optimization controller is constructed by using a single parameter learning mechanism, which confirmed that the method guarantees the input-to-state stability of the control systems.
Inspired by the above theory, the inverse optimization theory is also widely applied to some practical systems. In [42], for vehicle active suspension system with unknown nonlinear dynamics, Li et al. designed an adaptive fuzzy inverse optimal control method via state observer. Long et al. [43] proposed an inverse optimal fuzzy adaptive control approach for the system of flexible spacecraft system with fault-free actuator, which is subjected to input saturation, uncertain parameter and external disturbances.
In addition, people hope the practical engineering will reach the stable in finite time, and use the less control energy when achieving the satisfactory performance indicators simultaneously. Thus, how to achieve the effective balance between control quality and control energy has become a hot research issue. In [44], for nonlinear impulsive systems, Li and Ho studied the problem of finite-time stability (FTS). In [45], Li and Yang developed the Lyapunov–Razumikhin method for finite-time stability (FTS) and finite-time contractive stability (FTCS) of time-delay systems. In [46], via the power integral control approach and backstepping control method, Yang designed a semi-global real finite time controller. Then, according to the basic idea of inverse optimization, an appropriate objective functional is constructed, and the constructed objective functional is minimized by adjusting the parameters of semi-global real finite time controller.
Consider the Lyapunov function as follows:
V1=[r12v−τ]x(2v−τ)r1+12¯ω12 | (3.14) |
where ¯ω1=ω∗1−^ω1, ^ω1 is the estimation of the unknown parameter ω∗1, v=max{r1,p1r2}, j=1,2,⋯,n, pjrj+1=rj+τ, υ=max1≤j≤n{rj,pjrj+1}, j=1,2,⋯,n. rj and pj is the ratio of two positive odd numbers. r1=1, τ is the design parameter.
Based on [46], for a class of interlinked nonlinear systems with powers of positive odd rational numbers, Li et al. [47] developed a series of homogeneous controllers, which are capable of guaranteeing the local finite-time stability of the closed-loop systems by using the adding one power integrator approach and backstepping technique.
Most of the existing optimization finite-time control methods are limited by complicated design and updating process, which vastly affect the ideal property of optimization finite-time control. In order to solve this issue, in [48], Lu et al. first proposed an immediate fuzzy adaptive inverse optimization approach to receive a switching-type inverse optimization controller and a one parameter learning mechanism. The inverse optimal stabilization is solvable, and there exists a matrix-valued function P(x), which satisfied that P(x)=P(x)T>0, then the cost function is defined as:
J(u)=limt→∞∫t0[L(x)+ℏ(|P(x)12u)]dτ} | (3.15) |
where ℏ and its derivative ℏ′ are K∞ functions, L(x) is positive functions, u(x) is away from the origin in succession with u(x)=0.
For a kind of robotic manipulator system, which contains uncertain dynamics and input saturation, the authors in [49] proposed a fixed-time trajectory tracking control approach based on RL. For the sake of guaranteeing that e1 and e2 convergence to diminutive neighborhood around 0 in a uniformly bounded convergence time Ts, where Ts stands alone with the original states. A noval nonsingular fixed-time fast terminal sliding mode is proposed as:
s=K(e1e1)+sigυ1(e2) | (3.16) |
where Ke1=diag[ke11,ke12,⋯,ke1n] is a diagonal matrix. ke1i,i=1,2,⋯,n, are designed as:
ke1i=(α|e1i|p−1/(kυ1)+β|e1i|g−1/(kυ1))kυ1 | (3.17) |
where p and g are positive scalars with gk>1 and 1/υ1<pk<1, α>0, β>0, k>1, υ1>1.
Obviously, the above developed control method can effectively solve the finite/fixed-time optimal control problems and can make the minimize the cost function. Besides, in [50], Hu et al. considered the fixed-time stability of delayed neural networks with impulsive perturbations.
It can be seen from this review that optimization control design for unknown nonlinear systems via RL and ADP has been diffusely studied in control area and has achieved fruitful results. The origin and the development of optimization algorithms have been introduced, the research results of optimization control of affine nonlinear systems have been summarized. Then, under the frame of backstepping control, the adaptive optimal control, finite-time inverse optimal control, constraint control have also been described for strict-feedback nonlinear systems. At the same time, we have summarized the applications development of adaptive optimization control methods. In addition, as a novel hot issue in this field, finite/fixed-time optimal control via backstepping and RL/ADP for nonlinear systems have attracted considerable attentions, both theory and practical applications also need to be further studied in the future.
This work was supported by the National Natural Science Foundation of China under Grant No. 61822307.
The authors declare there is no conflict of interest.
[1] |
Kajihara M (2009) Numerical analysis for migration of austenite/ferrite interface during carburization of Fe. J Mater Sci 44: 2109-2118. doi: 10.1007/s10853-009-3299-9
![]() |
[2] |
Christ HJ (1998) Experimental characterization and computerbased description of the carburization behaviour of the austenitic stainless steel AISI 304L. Mater Corros 49: 258-265. doi: 10.1002/(SICI)1521-4176(199804)49:4<258::AID-MACO258>3.0.CO;2-U
![]() |
[3] |
Sudha C, Bharasi NS, Anand R, et al. (2010) Carburization behavior of AISI 316LN austenitic stainless steel—Experimental studies and modeling. J Nucl Mater 402: 186-195. doi: 10.1016/j.jnucmat.2010.05.023
![]() |
[4] |
Akiyama M, Oki Y, Nagai M (2012) Steam reforming of ethanol over carburized alkali-doped nickel on zirconia and various supports for hydrogen production. Catal Today 181:4-13. doi: 10.1016/j.cattod.2011.09.043
![]() |
[5] |
Boniatti R, Bandeira AL, Crespi  E, et al. (2013) The influence of surface microstructure and chemical composition on corrosion behaviour in fuel-grade bio-ethanol of low-alloy steel modified by plasma nitro-carburizing and post-oxidizing. Appl Surf Sci 280: 156-163. doi: 10.1016/j.apsusc.2013.04.116
![]() |
[6] |
Yin L, Ma X, Tang G, et al. (2019) Characterization of carburized 14Cr14Co13Mo4 stainless steel by low pressure carburizing. Surf Coat Technol 358: 654-660. doi: 10.1016/j.surfcoat.2018.11.090
![]() |
[7] |
Roy S, Sundararajan S (2019) Effect of retained austenite on spalling behavior of carburized AISI 8620 steel under boundary lubrication. Int J Fatigue 119: 238-246. doi: 10.1016/j.ijfatigue.2018.10.001
![]() |
[8] |
Lødeng R, Ranga C, Rajkhowa T, et al. (2017) Hydrodeoxygenation of phenolics in liquid phase over supported MoO3 and carburized analogues. Biomass Convers Biorefin 7: 343-359. doi: 10.1007/s13399-017-0252-z
![]() |
[9] |
Kvryan A, Efaw C, Higginbotham K, et al. (2019) Corrosion initiation and propagation on carburized martensitic stainless steel surfaces studied via advanced scanning probe microscopy. Materials 12: 940. doi: 10.3390/ma12060940
![]() |
[10] |
McLeod AC, Bishop CM, Stevens KJ, et al. (2015) Microstructure and carburization detection in HP alloy pyrolysis tubes. Metallogr Microstruct Anal 4: 273-285. doi: 10.1007/s13632-015-0210-8
![]() |
[11] |
Gupta A, Singla G, Pandey OP (2016) Effect of synthesis parameters on structural and thermal properties of NbC/C nano composite synthesized via in-situ carburization reduction route at low temperature. Ceram Int 42: 13024-13034. doi: 10.1016/j.ceramint.2016.05.081
![]() |
[12] |
Niu L, Liu X, Liu X, et al. (2017) In situ XRD study on promotional effect of potassium on carburization of spray-dried precipitated Fe2O3 catalysts. ChemCatChem 9: 1691-1700. doi: 10.1002/cctc.201601665
![]() |
[13] |
Hegde A, Sharma S, Sadanand RV (2019) Mechanical characterization and optimization of heat treatment parameters of manganese alloyed austempered ductile iron. J Mech Eng Sci 13: 4356-4367. doi: 10.15282/jmes.13.1.2019.01.0371
![]() |
[14] |
Ahmed HM, Seetharaman S (2010) Reduction-carburization of NiO-WO3 under isothermal conditions using H2-CH4 gas mixture. Metall Mater Trans B 41: 173-181. doi: 10.1007/s11663-009-9307-2
![]() |
[15] |
Gutierrez-Urrutia I, Zaefferer S, Raabe D (2010) The effect of grain size and grain orientation on deformation twinning in a Fe-22wt.% Mn-0.6wt.% C TWIP steel. Mat Sci Eng A-Struct 527: 3552-3560. doi: 10.1016/j.msea.2010.02.041
![]() |
[16] |
Gramlich A, Schmiedl T, Schönborn S, et al. (2020) Development of air-hardening martensitic forging steels. Mat Sci Eng A-Struct 784: 139321. doi: 10.1016/j.msea.2020.139321
![]() |
[17] |
Faccoli M, Ghidini A, Mazzù A (2019) Changes in the microstructure and mechanical properties of railway wheel steels as a result of the thermal load caused by shoe braking. Metall Mater Trans A 50: 1701-1714. doi: 10.1007/s11661-019-05135-x
![]() |
[18] |
Medyński D, Janus A (2018) Effect of heat treatment parameters on abrasive wear and corrosion resistance of austenitic nodular cast iron Ni-Mn-Cu. Arch Civ Mech Eng 18: 515-521. doi: 10.1016/j.acme.2017.08.001
![]() |
[19] |
Zheng B, Shu G, Jiang Q (2019) Experimental study on residual stresses in cold rolled austenitic stainless steel hollow sections. J Constr Steel Res 152: 94-104. doi: 10.1016/j.jcsr.2018.04.021
![]() |
[20] |
Sajid HU, Kiran R (2018) Influence of stress concentration and cooling methods on post-fire mechanical behavior of ASTM A36 steels. Constr Build Mater 186: 920-945. doi: 10.1016/j.conbuildmat.2018.08.006
![]() |
[21] |
An JH, Lee J, Kim YS, et al. (2019) Effects of post weld heat treatment on mechanical and electrochemical properties of welded carbon steel pipe. Met Mater Int 25: 304-312. doi: 10.1007/s12540-018-0201-9
![]() |
[22] |
Pandey C, Mahapatra MM, Kumar P, et al. (2018) Homogenization of P91 weldments using varying normalizing and tempering treatment. Mat Sci Eng A-Struct 710: 86-101. doi: 10.1016/j.msea.2017.10.086
![]() |
[23] |
Kusakin P, Belyakov A, Haase C, et al. (2014) Microstructure evolution and strengthening mechanisms of Fe-23Mn-0.3C-1.5Al TWIP steel during cold rolling. Mat Sci Eng A-Struct 617: 52-60. doi: 10.1016/j.msea.2014.08.051
![]() |
[24] |
Senopati G, Sutowo C, Kartika I, et al. (2019) The effect of solution treatment on microstructure and mechanical properties of Ti-6Mo-6Nb-8Sn alloy. Mater Today Proc 13: 224-228. doi: 10.1016/j.matpr.2019.03.218
![]() |
[25] |
Bakhtiari R, Ekrami A (2009) The effect of bainite morphology on the mechanical properties of a high bainite dual phase (HBDP) steel. Mat Sci Eng A-Struct 525: 159-165. doi: 10.1016/j.msea.2009.07.042
![]() |
[26] |
van Bohemen SMC (2012) Bainite and martensite start temperature calculated with exponential carbon dependence. Mater Sci Tech-Lond 28: 487-495. doi: 10.1179/1743284711Y.0000000097
![]() |
[27] |
Yang J, Yu H, Yin J, et al. (2016) Formation and control of martensite in Ti-6Al-4V alloy produced by selective laser melting. Mater Design 108: 308-318. doi: 10.1016/j.matdes.2016.06.117
![]() |
[28] |
Adnan F, Sajuri Z, Omar MZ (2019) Effect of uniaxial load on microstructure and mechanical properties of Thixo-joint AISI D2 tool steel. J Mech Eng Sci 13: 5006-5020. doi: 10.15282/jmes.13.2.2019.17.0414
![]() |
[29] |
Dmitrieva O, Ponge D, Inden G, et al. (2011) Chemical gradients across phase boundaries between martensite and austenite in steel studied by atom probe tomography and simulation. Acta Mater 59: 364-374. doi: 10.1016/j.actamat.2010.09.042
![]() |
[30] |
Odusote K, Adekunle AS, Rabiu AB (2015) Effect of vegetable oil quenchants on the properties of aluminum during solution heat treatment. J Mech Eng Sci 8: 1343-1350. doi: 10.15282/jmes.8.2015.9.0131
![]() |
[31] |
Pandey C, Mahapatra MM, Kumar P, et al. (2017) Microstructure characterization and charpy toughness of P91 weldment for as-welded, post-weld heat treatment and normalizing & tempering heat treatment. Met Mater Int 23: 900-914. doi: 10.1007/s12540-017-6850-2
![]() |
[32] |
Majid MSA, Daud R, Afendi M, et al. (2014) Stress-strain response modelling of glass fibre reinforced epoxy composite pipes under multiaxial loadings. J Mech Eng Sci 6: 916-928. doi: 10.15282/jmes.6.2014.18.0088
![]() |
[33] |
Ghadimi H, Nedjhad Sh, Eghbali B (2013) Enhanced grain refinement of cast aluminum alloy by thermal and mechanical treatment of Al-5Ti-B master alloy. Trans Nonferrous Met Soc China 23: 1563-1569. doi: 10.1016/S1003-6326(13)62631-X
![]() |
[34] |
Wang T, Cao F, Chen Z, et al. (2015) Three dimensional microstructures and wear resistance of Al-Bi immiscible alloys with different grain refiners. Sci China Technol Sci 58: 870-875. doi: 10.1007/s11431-015-5816-1
![]() |
[35] |
Rosip NIM, Ahmad S, Jamaludin KR, et al. (2013) Production of 316L stainless steel (SS316L) foam via slurry method. J Mech Eng Sci 5: 707-712. doi: 10.15282/jmes.5.2013.17.0068
![]() |
[36] |
Crupi V, Epasto G, Guglielmino E, et al. (2015) Analysis of temperature and fracture surface of AISI4140 steel in very high cycle fatigue regime. Theor Appl Fract Mec 80: 22-30. doi: 10.1016/j.tafmec.2015.07.007
![]() |
[37] |
Casati R, Lemke J, Vedani M (2016) Microstructure and fracture behavior of 316L austenitic stainless steel produced by selective laser melting. J Mater Sci Technol 32: 738-744. doi: 10.1016/j.jmst.2016.06.016
![]() |
[38] |
Rafi HK, Starr TL, Stucker BE (2013) A comparison of the tensile, fatigue, and fracture behavior of Ti-6Al-4V and 15-5 PH stainless steel parts made by selective laser melting. Int J Adv Manuf Tech 69: 1299-1309. doi: 10.1007/s00170-013-5106-7
![]() |
[39] |
Pandey C, Saini N, Mahapatra MM, et al. (2017) Study of the fracture surface morphology of impact and tensile tested cast and forged (C & F) Grade 91 steel at room temperature for different heat treatment regimes. Eng Fail Anal 71: 131-147. doi: 10.1016/j.engfailanal.2016.06.012
![]() |