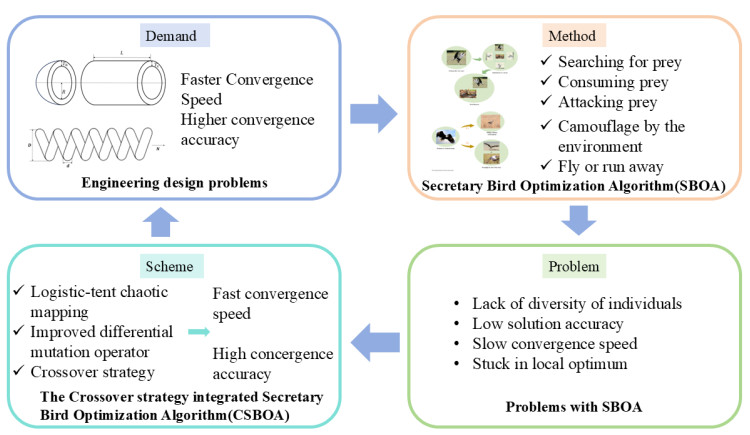
An improved metaheuristic algorithm called the Crossover strategy integrated Secretary Bird Optimization Algorithm (CSBOA) is proposed in this work for solving real optimization problems. This improved algorithm integrated logistic-tent chaotic mapping initialization, an improved differential mutation operator, and crossover strategies with the Secretary Bird Optimization Algorithm (SBOA) for a better quality solution and faster convergence. To evaluate the performance of CSBOA, two sets of a standard benchmark set, CEC2017 and CEC2022, were applied first. The Wilcoxon rank sum test and Friedman test were also used to statistically compare the proposed CSBOA algorithm with seven common metaheuristics. The comparisons demonstrated that CSBOA is more competitive than other metaheuristic algorithms on most benchmark functions. Additionally, the performance of CSBOA was validated for two challenging engineering design case studies. Comparative results showed that CSBOA provides more accurate solutions than the SBOA and the other seven algorithms, suggesting viability in dealing with real global optimization problems.
Citation: Xiongfa Mai, Yan Zhong, Ling Li. The Crossover strategy integrated Secretary Bird Optimization Algorithm and its application in engineering design problems[J]. Electronic Research Archive, 2025, 33(1): 471-512. doi: 10.3934/era.2025023
[1] | Xiaodong Wen, Xiangdong Liu, Cunhui Yu, Haoning Gao, Jing Wang, Yongji Liang, Jiangli Yu, Yan Bai . IOOA: A multi-strategy fusion improved Osprey Optimization Algorithm for global optimization. Electronic Research Archive, 2024, 32(3): 2033-2074. doi: 10.3934/era.2024093 |
[2] | Shiqi Zou, Xiaoping Shi, Shenmin Song . MOEA with adaptive operator based on reinforcement learning for weapon target assignment. Electronic Research Archive, 2024, 32(3): 1498-1532. doi: 10.3934/era.2024069 |
[3] | Tianbao Liu, Lingling Yang, Yue Li, Xiwen Qin . An improved dung beetle optimizer based on Padé approximation strategy for global optimization and feature selection. Electronic Research Archive, 2025, 33(3): 1693-1762. doi: 10.3934/era.2025079 |
[4] | Weishang Gao, Qin Gao, Lijie Sun, Yue Chen . Design of a novel multimodal optimization algorithm and its application in logistics optimization. Electronic Research Archive, 2024, 32(3): 1946-1972. doi: 10.3934/era.2024089 |
[5] | Bo Wei, Xiao Jin, Li Deng, Yanrong Huang, Hongrun Wu . Feature selection via a multi-swarm salp swarm algorithm. Electronic Research Archive, 2024, 32(5): 3588-3617. doi: 10.3934/era.2024165 |
[6] | Yu Shen, Hecheng Li . A multi-strategy genetic algorithm for solving multi-point dynamic aggregation problems with priority relationships of tasks. Electronic Research Archive, 2024, 32(1): 445-472. doi: 10.3934/era.2024022 |
[7] | Hui Li, Rongrong Gong, Pengfei Hou, Libao Xing, Dongbao Jia, Haining Li . Online learning resources recommendation model based on improved NSGA-Ⅱ algorithm. Electronic Research Archive, 2023, 31(5): 3030-3049. doi: 10.3934/era.2023153 |
[8] | Yuhai Zhong, Huashan Feng, Hongbo Wang, Runxiao Wang, Weiwei Yu . A bionic topology optimization method with an additional displacement constraint. Electronic Research Archive, 2023, 31(2): 754-769. doi: 10.3934/era.2023037 |
[9] | Xiaoyan Wu, Guowen Ye, Yongming Liu, Zhuanzhe Zhao, Zhibo Liu, Yu Chen . Application of Improved Jellyfish Search algorithm in Rotate Vector reducer fault diagnosis. Electronic Research Archive, 2023, 31(8): 4882-4906. doi: 10.3934/era.2023250 |
[10] | Ruyang Yin, Jiping Xing, Pengli Mo, Nan Zheng, Zhiyuan Liu . BO-B&B: A hybrid algorithm based on Bayesian optimization and branch-and-bound for discrete network design problems. Electronic Research Archive, 2022, 30(11): 3993-4014. doi: 10.3934/era.2022203 |
An improved metaheuristic algorithm called the Crossover strategy integrated Secretary Bird Optimization Algorithm (CSBOA) is proposed in this work for solving real optimization problems. This improved algorithm integrated logistic-tent chaotic mapping initialization, an improved differential mutation operator, and crossover strategies with the Secretary Bird Optimization Algorithm (SBOA) for a better quality solution and faster convergence. To evaluate the performance of CSBOA, two sets of a standard benchmark set, CEC2017 and CEC2022, were applied first. The Wilcoxon rank sum test and Friedman test were also used to statistically compare the proposed CSBOA algorithm with seven common metaheuristics. The comparisons demonstrated that CSBOA is more competitive than other metaheuristic algorithms on most benchmark functions. Additionally, the performance of CSBOA was validated for two challenging engineering design case studies. Comparative results showed that CSBOA provides more accurate solutions than the SBOA and the other seven algorithms, suggesting viability in dealing with real global optimization problems.
Over the past two decades, metaheuristic algorithms (MAs) have found extensive application in solving complex engineering problems across scientific fields. Particularly when it comes to non-convex, highly nonlinear, non-smooth, and even dynamic real-world problems, MAs are more prevalent than traditional mathematical optimization methods due to their gradient-free nature and simple structure [1,2]. Generally, metaheuristic algorithms are typically divided into three categories: Evolutionary algorithms, physical algorithms, and swarm intelligence algorithms [3]. Among evolutionary algorithms, there are the Genetic Algorithm (GA) [4], Differential Evolution Algorithm (DEA) [5], Imperialist Competitive Algorithm (ICA) [6], Liver Cancer Algorithm (LCA) [7], Partial Reinforcement Optimizer (PRO) [8], Moss Growth Optimization (MGO) [9], and others. Their common feature is that they draw inspiration from natural evolutionary processes. Physical algorithms include Simulated Annealing (SA) [10], the Multi-Verse Optimizer (MVO) [11], Equilibrium Optimizer (EO) [12], Planet Optimization Algorithm (POA)[13], Kepler Optimization Algorithm (KOA) [14], Rime Optimization Algorithm (RIME) [2], Snow Ablation Optimizer (SAO) [15], Newton-Raphson-based Optimizer (NBRO) [16], and so on. Their common feature is the simulation of physical laws and principles. Swarm intelligence optimization algorithms include Particle Swarm Optimization (PSO) [17], Grey Wolf Optimizer (GWO) [18], Artificial Rabbits Optimization (ARO) [19], Snake Optimizer (SO) [20], Crayfish Optimization Algorithm (COA) [21], Crested Porcupine Optimizer (CPO) [22], Hippopotamus Optimization Algorithm (HO) [23], Seagull Optimization Algorithm (SOA)[24], Secretary Bird Optimization Algorithm (SBOA)[25], and so on. Their common characteristic originates from the social behaviors of foraging, reproduction, and hunting within animal groups. Generally, these algorithms start with randomly generated initial solutions and then undergo continuous iterations. The optimal solution gradually approaches the global optimum. When the algorithm reaches the maximum number of iterations, the optimization process stops and the optimal solution is output. Metaheuristic algorithms can handle complex problems flexibly by simulating nature without knowing the details of the problem. Therefore, they are increasingly used and have become a powerful tool for solving real-world problems, such as vehicle scheduling problems [26,27], routing protocols in wireless sensor networks [28], feature selection [29,30], automated UAV trajectory planning [31], and community detection [32].
Due to the "No free lunch theorem" (NFL) proposed by Wolpert and Macready [33], it is always vital to develop new optimization algorithms to solve new and complex optimization problems, which motivates us to propose new optimization methods continually. The improvement of optimization methods is a current research hot spot [34]. For instance, Zheng et al. [35] introduced an enhanced African Vultures Optimization Algorithm (EAVOA). They incorporated a rotating flight mechanism, a representative vulture selection mechanism, and a selection accumulation operator strategy into the original AVOA. The EAVOA was evaluated using the IEEE CEC2005 benchmark suite and the CEC2017 benchmark suite. It demonstrated superior optimization capabilities compared to the original AVOA, showing its strength in continuous optimization problems. For more examples of improved algorithms, please refer to Section 2, "Related work". Similarly, the SBOA algorithm is improved in this study to enhance the search capability and solve complex optimization problems.
The Secretary Bird Optimization Algorithm (SBOA) was proposed by Fu et al. [25] in 2024 to solve real-world optimization problems by exploiting the survival behavior of secretary birds in natural environments. In SBOA, secretary birds need to constantly hunt for prey and escape from predators, for which Fu et al. divided it into an exploration phase and an exploitation phase. The search agent continuously switches between the exploration and exploitation phases to approximate the global optimal solution. Compared with earlier meta-heuristic algorithms, SBOA is characterized by high accuracy and flexible framework. However, SBOA also inevitably has some drawbacks: 1) Since SBOA initializes the population by random initialization, it leads to a lack of diversity of individuals within the population. Therefore, it is important to improve the diversity of the population during the search process of the algorithm. 2) When facing multi-dimensional complex optimization challenges, SBOA has low solution accuracy and slow convergence speed. Thus, there is room for further improvement in SBOA's global search capability. 3) In response to the challenges posed by complex engineering optimization problems in real life, where the algorithm is confronted with a large number of nonlinear constraints, SBOA is inclined to fall into local optima. Therefore, enhancing SBOA's ability to escape from local optimum is of crucial importance. 4) The No Free Lunch (NFL) theorem [33] shows that no algorithm is perfect. Thus, in real engineering optimization problems, appropriate strategies can be employed to facilitate the SBOA to work more effectively.
In light of the above motivation, we propose a Crossover strategy integrated Secretary Bird Optimization Algorithm (CSBOA) for global optimization. The CSBOA algorithm proposed in this paper employs three improvement strategies: first, it is initialized with logistic-tent chaotic mapping to enhance the population diversity; second, it incorporates an improved differential variance operator in the exploratory phase of the secretary bird's search for its prey to improve the algorithm's ability to jump out of the local optimum; and last, it incorporates a crossover strategy after each iteration to accelerate the algorithm's convergence ability. Figure 1 shows the motivation of this study to illustrate the idea and value of the research more intuitively.
To comprehensively evaluate the performance of CSBOA, we perform a series of analyses, including convergence analysis, stability analysis, and statistical tests, using 29 IEEE CEC2017 benchmark functions and 12 IEEE CEC2022 benchmark functions of varying dimensions. CSBOA is compared with several state-of-the-art metaheuristics, including seven advanced optimizers: The original SBOA algorithm, four well-established algorithms, and two cutting-edge algorithms. Additionally, CSBOA is applied to solve two engineering optimization problems to demonstrate its practical applicability in real-world scenarios.
The following are the key ideas and contributions of this study.
1) Integration of improvement strategies: A logistic-tent chaotic mapping strategy is integrated into the population initialization process, enhancing the uniformity of population distribution and increasing the randomness and diversity of the algorithm. During the exploration phase, the differential mutation operator is improved to help avoid local optima. Furthermore, a crossover strategy is employed after each iteration to enhance both the convergence speed and accuracy. CSBOA effectively addresses issues such as delayed convergence and the tendency to get trapped in local optima in SBOA.
2) Evaluation of strategy performance: The individual and combined effects of the three improvement strategies on SBOA's performance are evaluated. The optimal mean and convergence speed of CSBOA are compared with six prominent state-of-the-art algorithms and the original SBOA. Statistical analyses, including the Wilcoxon rank sum test and Friedman ranking test, demonstrate that CSBOA outperforms the other algorithms.
3) Time complexity and balance analysis: The time complexity of CSBOA and the comparative algorithms is analyzed. The balance between exploration and exploitation is also examined. Several convergence plots are generated to visualize CSBOA's convergence ability. The results indicate that CSBOA maintains acceptable time complexity, achieves a good balance between exploration and exploitation, and exhibits faster convergence speed and higher accuracy.
4) Real-world application: To validate the ability of CSBOA in solving practical problems, it is applied to a nonlinear constrained optimization engineering problem. The results, compared with various other algorithms, show that CSBOA consistently ranks first in all cases.
The rest of the paper is organized as follows. In Section 2, we introduce related work. In Section 3, we provide a comprehensive overview of the SBOA algorithm. In Section 4, we elaborate on the logistic-tent chaotic mapping initialization strategy, the improved differential operator strategy, and the crossover strategy, and presents CSBOA, a multi-strategy enhanced Secretary Bird Optimization Algorithm. We also calculate the time complexity of the CSBOA algorithm and other algorithms. In Section 5, we depict the experimental settings, results, and discussions. In Section 6, we showcase the effectiveness of CSBOA in practical applications through two constrained engineering design examples. Last, in Section 7, we summarize the conclusion and potential directions for future research.
Despite the growing use of metaheuristic algorithms, the "No Free Lunch" (NFL) theorem [33] suggests that they cannot consistently offer superior performance across all optimization problems. Different algorithms exhibit varying performance on different issues. As a result, researchers continuously propose new algorithms or enhance existing ones to strike a balance between exploration and exploitation within the algorithms. The problems of easily getting stuck in local optima, slow convergence speed, lack of robustness, and high computational cost have always been challenges faced by metaheuristic algorithms. To address this issue, Taheri et al.[8] introduced the Partial Reinforcement Optimizer (PRO) based on the Partial Reinforcement Effect (PRE) theory, and applied to optimize a Federated Deep Learning Electrocardiography (ECG) classifier. Experimental results demonstrate that the PRO algorithm outperforms existing meta-heuristic optimization algorithms by providing a more accurate and robust solution. Yuan et al. [36] proposed the Polar Lights Optimization (PLO) algorithm, which integrates rotational motion and aurora oval walking strategies to balance local exploitation and global exploration. The rotational motion helps with local development, while the aurora oval walking strategy promotes global exploration. In August 2024, Truong and Chou [37] proposed the Enterprise Development Optimization (EDO) algorithm. This algorithm integrates four types of variables: task, structure, technology, and personnel to form a complex enterprise development system. The algorithm shows excellent robustness in global optimization and structural engineering problems and has wide universality. In September 2024, Ji et al. [38] proposed the Neural Population Dynamics Optimization Algorithm (NPDOA), a metaheuristic algorithm inspired by neuroscience. The algorithm simulates various activities of the brain through three strategies: attractor trending strategy, coupling disturbance strategy, and information projection strategy. These strategies effectively balance exploration and exploitation. To address the shortcomings of the Remora Optimization Algorithm (ROA) [39], such as getting trapped in local optima and having a slow convergence rate, Jia et al. [40] integrated a random restart mechanism, an information entropy evaluation mechanism, and a visual perception mechanism, and proposed the MSROA algorithm. This algorithm has been evaluated on the IEEE CEC2005 benchmark suite and the CEC2017 benchmark suite. The results indicate that MSROA has stronger optimization capabilities than the ROA. Zheng et al. [35] introduced an enhanced African Vultures Optimization Algorithm (EAVOA). They incorporated a rotating flight mechanism, a representative vulture selection mechanism, and a selection accumulation operator strategy into the original AVOA. The EAVOA was evaluated using the IEEE CEC2005 benchmark suite and the CEC2017 benchmark suite. It demonstrated superior optimization capabilities compared to the original AVOA, showing its strength in continuous optimization problems. Hashim et al. [41] proposed an improved Seahorse Optimizer (mSHO). This algorithm integrates three search operators: neighborhood-based local search, global search, and walking within the existing search to achieve a more robust development trend. The mSHO algorithm exhibits strong competitiveness in solving 10 unconstrained CEC2020 benchmark problems and 9 constrained real-world engineering tasks. Qin et al. [42] combined three strategies, namely the feedback regulation mechanism, the golden sinusoidal guidance strategy, and the cooperative camouflage strategy, to improve the Secretary Bird Optimization Algorithm, which was named MISBOA. Based on the benchmark test function of CEC2022, the algorithm has demonstrated good performance, and MISBOA has been applied to solve 5 engineering problems and the shape optimization problem of combined curves. Qin et al. [43] integrated the Circle Chaotic Mapping, the differential evolution strategy, and the population diversity strategy into the Secretary Bird Optimization Algorithm, naming it ISBOA, which achieves the optimal convergence speed and optimization accuracy. Zheng et al. [44] proposed a multi hop routing protocol based on the Secretary Bird Optimization Algorithm (SBOA) to address the issues of limited node energy and imbalanced energy consumption in 3D Bridge Wireless Sensor Networks (BWSN). Pan et al. [45] proposed a Knowledge-based Twin-Population Optimization (KTPO) algorithm to minimize total energy consumption and total delay simultaneously, and the experimental results proved the effectiveness and advantages of KTPO in solving DEPMSP. Zhao et al. [46] proposed a hyperheuristic with Q-learning (HHQL) to solve the energy-efficient distributed blocking flow shop scheduling problem (EEDBFSP). The experimentation that the HHQL outperforms the other comparison algorithm regarding efficiency and significance in solving EEDBFSP. Zhao et al. [47] used the Variable Neighborhood Descent algorithm (VNDA) and the Q-learning mechanism to Improved Iterative Greedy (IIG) algorithm for solving the multiobjective distributed no-idle permutation flowshop scheduling problem without idle alignments. The algorithm optimizes both makespan and total tardiness. The experiments show that the IIG algorithm obtained more satisfactory results. Truong et al. [48] proposed a Quasi-Opposition-Based Learning (QOBL) and Chaotic Local Search (CLS) strategies based on the Symbiotic Organisms Search (SOS), called Quasi-Oppositional Chaotic Symbiotic Organisms Search (QOCSOS), for optimizing the placements for Distributed Generation (DG) units in radial distribution networks. Comparative results showed that QOCSOS provided more accurate solutions than SOS and other methods. Chen et al.[49] proposed a Multi-Strategy Optimizer (MSO) that defines various population search functions and employs superior neighborhood methods for population updates to minimize the energy consumption of a multi-UAV-assisted Mobile Edge Computing (MEC) system. Lan and Wang[50] introduced a novel modifed African vultures optimization algorithm method called OLAVOA based on the opposition learning of African Vultures Optimization Algorithm (AVOA), combining predatory memory and logarithmic spiral, which is used for solving multi-level threshold image segmentation problems. The experimental results show that OLAVOA has better performance and can be efectively utilized to segment medical images. In summary, this paper improves the SBOA based on a logistic-tent chaotic mapping strategy, an improved differential variance operator, and a crossover strategy, and the experimental results show that the idea is reasonable. Table 1 Provides a concise list of some of the latest MAs.
Category | Algorithm | year | Inspiration/Description |
Evolutionary | Liver Cancer Algorithm (LCA) [7] | 2023 | Inspired by the ability of tumors to replicate and spread to other organs, an MA is introduced. |
Partial Reinforcement Optimizer (PRO)[8] | 2024 | Inspired by the partial reinforcement effect (PRE) theory, an evolutionary learning/training theory in psychology, a learner is intermittently reinforced to learn or strengthen a specific behavior based on a schedule. | |
Moss Growth Optimization (MGO)[9] | 2024 | Inspired by the growth of moss in the natural environment, an MA is introduced. | |
Physic-based | Planet Optimization Algorithm (POA) [13] | 2022 | Inspired by the laws of cosmic motion and starting from the interaction of the gravitational forces among planets, an MA is introduced. |
Kepler Optimization Algorithm (KOA) [14] | 2023 | Inspired by Kepler's laws of planetary motion, which can predict the position and velocity of the planets at any given time, an MA is introduced. | |
Rime Optimization Algorithm (RIME)[2] | 2023 | Inspired by the natural frost and ice growth mechanism, algorithms are developed by simulating the motion of soft frost particles for algorithmic search, and by simulating the crossover behavior between hard frost agents. | |
Snow ablation optimizer (SAO) [15] | 2023 | Inspired by the simulation of the sublimation and melting behavior of snow, an MA is introduced. | |
Newton-Raphson-based optimizer (NBRO) [16] | 2024 | The algorithm searches the solution space by defining the solution space using the set of vectors and employing operators such as Natural Resource Strategy (NRSR) as well as Trap Avoidance Operator (TAO). | |
Swarm intelligence | Crested Porcupine Optimizer (CPO)[22] | 2024 | Simulation of four different protective mechanisms in crown porcupines: sight, hearing, odor and physical attack. |
Hippopotamus optimization algorithm (HO) [23] | 2024 | Inspired by the behavioral patterns of hippos in the natural environment, an MA is introduced. | |
Seagull optimization algorithm (SOA)[24] | 2024 | Inspired by the migration and predatory behaviors of seagulls in the natural world, an MA is introduced. | |
enhanced African Vultures Optimization Algorithm (EAVOA) [35] | 2023 | They incorporated a rotating flight mechanism, a representative vulture selection mechanism, and a selection accumulation operator strategy into the original AVOA. It demonstrated superior optimization capabilities compared to the original AVOA. | |
an improved Seahorse Optimizer (mSHO) [41] | 2024 | This algorithm integrates three search operators: neighborhood-based local search, global search, and walking within the existing search to achieve a more robust development trend. | |
multi-strategy improvement Secretary Bird Optimization Algorithm (MISBOA) [42] | 2024 | The algorithm improves the Secretary Bird Optimization Algorithm by combining three strategies: feedback regulation mechanism, golden sinusoidal guidance, and population diversity, and the results show that MISBOA has demonstrated good performance. | |
Improved Secretary Bird Optimization Algorithm [43] | 2024 | ISBOA adds the Circle Chaotic Mapping, the differential evolution strategy, and the population diversity strategy into the SBOA, which achieves the optimal convergence speed and optimization accuracy. optimal convergence speed and optimization accuracy. | |
Crossover strategy-based Secretary Bird Optimization Algorithm (CSBOA) | 2024 | Under the SBOA algorithm's framework, CSBOA innovatively adds the initialization strategy of logical chaos mapping, improved differential operator strategy, and crossover strategy, which greatly enhances the algorithm's efficiency. |
Similar to these works, the CSBOA proposed in this paper further enhances the global search capability of the SBOA, accelerates its convergence speed, and effectively avoids local optima by logistic-tent chaotic mapping, an improved differential mutation operator, and a crossover strategy. Compared to existing optimization algorithms, CSBOA demonstrates superior performance in the IEEE CEC2017 and IEEE CEC2022 benchmark suites and engineering applications. Experimental results show that CSBOA exhibits faster convergence and higher accuracy in optimization problems across multiple dimensions, outperforming both the original SBOA and other state-of-the-art algorithms, thereby further demonstrating its potential for solving complex engineering optimization problems.
In this section, we review the mathematical model of the original Secretary Bird Optimization Algorithm (SBOA). Proposed by Fu et al. in 2024 [25], SBOA is a novel population-based metaheuristic algorithm inspired by the survival behavior of secretary birds in their natural environment. The survival process of secretary birds involves continuous hunting for prey and evading predators. Based on these behaviors, SBOA is structured into three phases: The initialization phase, the exploration phase, and the exploitation phase. In the exploration phase, the algorithm simulates secretary birds hunting for prey, while in the exploitation phase, it models their evasion of predators.
As with other MAs, the iteration procedure of SBOA commences with a randomly generated population. Assuming that the dimensional size of the optimization problem is Dim, the lower and upper boundaries of the search domain are Lb and Ub, respectively, and the number of search agents is N, then the initial position of the entire population can be modeled as a matrix with N rows and Dim columns.
X=Lb+rand×(Ub−Lb), | (3.1) |
where rand indicates a random number in [0,1]. Usually, the position vector of the ith search agent is expressed as: Xi=(Xi,1,Xi,2,...,Xi,j,...,Xi,Dim),i=1,2,...,N;j=1,2,...,Dim.
The hunting behavior of secretary birds when feeding on snakes is typically divided into three stages: Searching for prey, consuming prey, and attacking prey.
Based on the biological statistics of the secretary bird's hunting phases and the time durations for each phase, we have divided the entire hunting process into three equal time intervals, namely t<13T, 13T<t<23T, and 23T<t<T, the modeling of each phase in SBOA is as follows:
Stage 1 (Searching for prey): In this stage, a differential evolution strategy is employed. The introduction of differential mutation operations can help prevent being trapped in local optima thanks to its diversity.
whilet<13T,xP1i,j=xi,j+(xrandom1−xrandom2)×R1, | (3.2) |
Xi={XP1i,ifFP1i<FiXi,else, | (3.3) |
where t represents the current iteration number, T represents the maximum iteration number, Xp1i represents the new state of the ith secretary bird in the first stage, and xrandom1 and xrandom2 are the random candidate solutions in the first stage iteration. R1 represents a randomly generated array of dimension 1×Dim from the interval [0,1], where Dim is the dimensionality of the solution space. xP1i,j represents its value of the jth dimension, and FP1i represents its fitness value of the objective function.
Stage 2 (Consuming prey): The exploration phase of this algorithm introduces individual optimality in the second step to better explore the surrounding solution space. Moreover Brownian is used to simulate the random position of the secretary bird.
RB=randn(1,dim), | (3.4) |
while13T<t<23T,xP1i,j=xbest+exp((tT)4)×(RB−0.5)×(xbest−xi,j), | (3.5) |
Xi={XP1i,ifFP1i<FiXi,else, | (3.6) |
where randn(1,Dim) represents a randomly generated array of dimension 1×Dim from a standard normal distribution (mean 0, standard deviation 1), and xbest represents the current best value.
Stage 3 (Attacking prey): At this stage, the algorithm introduced Levy flight simulation of secretary bird attacking prey, while introducing a nonlinear perturbation factor represented as (1−tT)(2×tT), t represents the current iteration number, T represents the maximum iteration number.
whilet>23T,xP1i,j=xbest+((1−tT)2×tT)×xi,j×RL, | (3.7) |
Xi={XP1i,ifFP1i<FiXi,else, | (3.8) |
RL=0.5×Levy(dim). | (3.9) |
When secretary birds encounter natural enemies, they often adopt two strategies to protect themselves or their food. One is camouflage by the environment, and the other is to fly or run away. The probability of adopting these two strategies is equal.
XP2i,j={C1:Xbest+(2×RB−1)×(1−tT)2×xi,j,ifrand<0.5C2:xi,j+R2×(xrandom−K×xi,j),else, | (3.10) |
Xi={XP2i,ifFP1i<FiXi,else, | (3.11) |
K=round(1+rand(1,1)). | (3.12) |
Here, R2 represents the random generation of an array of dimension (1×Dim) from the normal distribution, xrandom represents the random candidate solution of the iteration, and K represents the random selection of integer 1 or 2.
In this section, a detailed model of the three strategies for enhancing SBOA is given and the complexity of the proposed algorithm CSBOA is calculated.
When the SBOA randomly generates solutions within the solution space according to Eq (3.1), it fails to guarantee a uniform distribution. This lack of uniformity results in poor diversity and an ineffective global exploration capability, which in turn reduces the search efficiency of the algorithm. Therefore, logistic-tent chaotic mapping is introduced in the initialization phase to improve the diversity of the population.
In recent years, the chaos mechanism has been widely applied to meta-heuristic algorithms. The logistic-tent chaotic mapping exhibits robust randomness and ergodicity. Despite its simple structure, it can produce intricate chaotic dynamic behaviors. It is indicated that the abundant diversity of the initial population can considerably improve the performance of the algorithm both in terms of convergence rate and accuracy. Therefore, in this paper, the logistic-tent chaotic mapping is selected to replace random search, and this chaotic system combines the complex chaotic dynamics of logistics with a faster iteration speed, more autocorrelation, and suitability for a large number of sequences of tent chaotic systemS. Its mathematical formula is defined as follows, Eq (4.1):
Xn+1={(rXn(1−Xn)+(4−r)Xn/2)mod1,Xn<0.5(rXn(1−Xn)+(4−r)(1−Xn)/2)mod1,Xn≥0.5. | (4.1) |
The value range of r is (0, 4), and in this experiment, r=0.5 [51].
The Differential Evolution Algorithm (DEA) makes use of the diversity among individuals to guide its exploration in the solution space. DE advances by employing mutation, crossover, and selection operations to refine the population until the predefined termination conditions are met. The mutation operator brings in variability by perturbing a group of target vectors, leading to the creation of a new mutated vector. In this article, the original DE/rand/1 mutation strategy is improved to the DE/rand-rand/1 mutation strategy. Furthermore, the nonlinear perturbation factor CF is used to replace the original random number R1. Three distinct individuals (excluding the ith one) are randomly chosen from the population. Subsequently, the difference between two randomly selected individuals is minimized, along with the gap between the third random individual and the ith one. By integrating the position of the ith individual, a new mutated individual is then generated. The purpose of this is to improve the randomness of individual exploration and help enhance the algorithm's ability to escape from local optima. Its mathematical expression is shown in the following Eq (4.2).
X(i+1)=X(i)+CF×(Xrandom1−Xrandom2)+CF×(Xrandom3−X(i)). | (4.2) |
Among them, CF is the nonlinear disturbance factor (consistent with the disturbance factor in the original paper), expressed as (1−tT)(2×tT), where t represents the current iteration number and T represents the maximum iteration number.
During the iterative process of the SBOA, the birds progressively home in on the direction of the global optimum within the current population. However, if the global optimum leader becomes trapped in a local optimum, it can precipitate a "premature convergence" phenomenon within the algorithm. As iterations accumulate, there is a tendency for the diversity within the population to diminish gradually. The position updates of the secretary birds rely heavily on the experiences of global leaders, local leaders, and individual members, which may not exhaustively explore the entire solution space, potentially leading to unexplored areas and reduced algorithmic precision. In response to the above shortcomings, we introduce the crossover strategy [52] and propose a Secretary Bird Optimization Algorithm to integrate the crossover strategy.
Crossover strategies include both horizontal and vertical crossovers. The horizontal crossover refers to the arithmetic crossover of operations between two different secretary birds in all dimensions, enabling individuals to learn from each other, increase the global search ability, prevente premature population convergence, and improve convergence speed and search accuracy. Horizontal crossover first randomly pairs individuals in the population, and then horizontally crossovers the two paired individuals. Assuming that Xi1 and Xi2 are individuals of paired parents, their offspring individuals Xhci1 and Xhci2 are generated by the following Eqs (4.3) and (4.4):
Xhci1j=r1×Xi1j+(1−r1)×Xi2j+c1×(Xi1j−Xi2j), | (4.3) |
Xhci2j=r2×Xi1j+(1−r2)×Xi1j+c2×(Xi2j−Xi1j). | (4.4) |
Among them, Xi1j and Xi2j represent the jth dimension of Xi1 and Xi2 respectively, j=1,2,3...dim, Xhci1j and Xhci2j represent the jth dimension where Xi1 and Xi2 cross horizontally to produce offspring on the jth dimension, r1 and r2 represent uniformly distributed random numbers within the range of (0,1), and c1 and c2 are uniformly distributed random numbers within the range of (−1,1). The generated offspring compete with their parents to ultimately retain the optimal individual.
Vertical crossover can help some stagnant dimensions of the population break free from premature convergence, thus enabling the algorithm to jump out of local optima. Furthermore, the crossover operation can increase the diversity of the population. Vertical crossover is an arithmetic crossover where all individuals operate on two different dimensions. Each undergoes a vertical crossover that only updates one dimension while keeping the other dimensions unchanged. This gives stagnant dimensions a chance to jump out of local optima without destroying the other potentially optimal dimension. Now, two dimensions j1 and j2 are randomly selected, and the j1 dimension of their descendant Xvci is obtained by the following Eq (4.5), while the other dimensions remain the same as those of the parent.
Xvci1j=r×Xi,j1+(1−r1)×Xi,j2 | (4.5) |
r=rand; |
competition between generated offspring and parents, retaining the optimal individual. This strategy helps the algorithm to escape premature convergence and improve convergence accuracy.
The detailed procedural steps of CSBOA are outlined below algorithm 1. Based on the biological statistics of the secretary bird's hunting phases and the time durations for each phase, we have divided the entire hunting process into three equal time intervals, namely t<13T, 13T<t<23T, and 23T<t<T. When secretary birds encounter natural enemies, they often adopt two strategies to protect themselves or their food. One is camouflage by the environment, and the other is to fly or run away. The probability of adopting these two strategies is equal [25].
Algorithm 1 Pseudocode of the CSBOA |
Input: Population size N, current iteration t, maximum iterations T, dimension size Dim, supremum ub, infimum lb; Output: Global optimal solution Xbest; 1: set N=100,T=500; 2: Initializing populations with logical-tent chaotic mapping; 3: for t=1:T do 4: Update Secretary Bird Xbest; 5: for i=1:N do 6: Exploration: 7: if t<13T then 8: % Searching for prey % 9: Calculate new status of the ith Secretary Bird using Eq (4.2); 10: else if 13T<t<23T then 11: %Consuming prey % 12: Calculate new status of the ith Secretary Bird using Eq (3.5); 13: Update the ith individual's current position using Eq (3.6); 14: else 15: %Attacking prey% 16: Calculate new status of the ith Secretary Bird using Eq (3.7); 17: Update the ith individual's current position using Eq (3.8); 18: end if 19: end for 20: Exploitation: 21: for i=1:N do 22: if r<0.5 then 23: %C1: Camoufage by environment% 24: Calculate new status of the ith Secretary Bird using C1 in Eq (3.10); 25: else 26: % C2: Fly or run away% 27: Calculate new status of the ith Secretary Bird using C2 in Eq (3.10); 28: end if 29: Update the ith individual's current position using Eq (3.11); 30: end for 31: Calculate new status of the ith Secretary Bird using in Eqs (4.3)–(4.5); 32: Save best candidate solution so far. 33:end for |
Optimizing identical problems with different algorithms entails varying durations, and evaluating an algorithm's computational complexity is crucial for assessing its execution efficiency. The computational complexity of the CSBOA is influenced by four primary components: The initialization of the population, the updating of individual positions within the population, the application of horizontal and vertical crossover strategies for position updates, and the evaluation of fitness values.
In the initialization phase, the computational complexity required to generate the initial position of each individual using the logistic-tent chaotic mapping is O(N×Dim), where N denotes the number of search agents and Dim is the dimension. Subsequently, the complexity of evaluating the determination of the optimal position and the updating of all solutions is O(N×Dim)+O(T×N)+3×O(N×Dim×T) for each iteration, where T denotes the maximum number of iterations allowed and 2×O(N×Dim×T) is the complexity associated with updating the position for crossover strategy. Therefore, the total computational complexity of the proposed CSBOA can be expressed as O(N×(Dim+T+3×T×Dim)).
Additionally, all experiments are performed on a laptop computer with an Intel(R) Core(TM) i5-10210U CPU @1.60 GHz 2.11 GHz, and all programs are coded on MATLAB R2018B and later versions.
In the research field of intelligent algorithms, comparing computation times is vital for algorithm assessment. Table 2 shows the average CPU time of algorithms like GWO, AVAO, and the proposed CSBOA on twelve CEC2022 functions. Some algorithms, such as GWO and AVAO, have shorter computation times but major flaws. They exhibit slow convergence, often prematurely converging or getting stuck in local optima during complex optimizations. Consequently, for intricate problems, their solution accuracy is poor. In contrast, CSBOA, despite a marginally longer computation time in some situations, has notable strengths. Endowed with enhanced search and a refined optimization mechanism, it can comprehensively explore the search space, especially where others falter in complex function landscapes. This yields solutions of higher precision and reliability. The moderate increase in computational time is outweighed by the enhanced accuracy. In applications where precision is crucial, this trade-off is justifiable. Moreover, as the complexity of CEC2022 functions rises, CSBOA's superiority becomes more evident. It maintains performance while others decline, demonstrating robustness and suitability for handling complex optimization tasks. Thus, while computation time matters, CSBOA's advantages in solution quality and complexity handling make it a significant contender in the intelligent algorithm domain.
Function | GWO | AVAO | GTO | DE | COA | PSO | SBOA | CSBOA |
F1 | 0.2163120 | 0.3335453 | 0.6284790 | 1.1332032 | 0.1795724 | 0.1761100 | 0.3141203 | 1.5787525 |
F2 | 0.2100052 | 0.3018989 | 0.6607184 | 1.1743020 | 0.1837386 | 0.1733390 | 0.3190766 | 1.7292872 |
F3 | 0.2985672 | 0.3746466 | 0.8275277 | 1.3605336 | 0.3303046 | 0.2590517 | 0.5342557 | 2.1225204 |
F4 | 0.2221101 | 0.3152409 | 0.7127616 | 1.1873779 | 0.2305653 | 0.1963042 | 0.3707470 | 1.8089242 |
F5 | 0.2342974 | 0.3237152 | 0.6934132 | 1.2154550 | 0.2350611 | 0.2044259 | 0.4110868 | 1.7652453 |
F6 | 0.2150608 | 0.3068646 | 0.6739646 | 1.1734847 | 0.1979094 | 0.1757798 | 0.3328941 | 1.7567508 |
F7 | 0.3356384 | 0.4250690 | 0.9167834 | 1.3618838 | 0.4035385 | 0.2982822 | 0.5812446 | 2.3796544 |
F8 | 0.3715650 | 0.4781056 | 1.0045935 | 1.4647472 | 0.4844369 | 0.3593346 | 0.6875874 | 2.5092880 |
F9 | 0.3040718 | 0.3946582 | 0.8335377 | 1.3782160 | 0.3375005 | 0.2658512 | 0.5529398 | 2.1785161 |
F10 | 0.2883326 | 0.3836225 | 0.8240736 | 1.3242494 | 0.3325307 | 0.2494263 | 0.5050225 | 2.0998797 |
F11 | 0.3608691 | 0.4689512 | 0.9485607 | 1.4617516 | 0.4222041 | 0.3393067 | 0.6414698 | 2.3714879 |
F12 | 0.3713333 | 0.4656035 | 0.9925775 | 1.4338215 | 0.4992448 | 0.3349514 | 0.6788769 | 2.6054365 |
In this section, to evaluate the effectiveness of the CSBOA algorithm in terms of optimization and its ability to provide optimal solutions, an initial analysis of its exploration-exploitation balance is conducted. Following this, a performance comparison is made between CSBOA and seven other algorithms using the benchmark test functions from CEC2017 and CEC2022. Subsequently, the Wilcoxon rank-sum test and Friedman test are applied to assess whether significant differences exist between CSBOA and the other algorithms.
In this section, to validate the effectiveness of SBOA, we conduct comparisons with 7 advanced algorithms on the CEC2017 and CEC2022 test functions. The compared algorithms fall into three categories: 1) Original algorithm: Secretary Bird Optimization Algorithm (SBOA)[25]; 2) Highly-Cited Algorithms: Differential Evolution Algorithm (DEA)[5], Grey Wolf Optimizer (GWO)[18], Particle Swarm Optimization (PSO)[17] and African Vultures Optimization Algorithm (AVOA) [53]; 3) Advanced Algorithms: Artificial Gorilla Troops Optimizer (GTO)[54], Crayfish Optimization Algorithm (COA)[21]. The parameter configurations for each method align with the respective references, as indicated in Table 3. We set the maximum number of iterations and population size for all algorithms to 500 and 100, respectively. Each algorithm is independently run 30 times, and the experimental results are presented in the following text.
Algorithms | Reference | Name of the parameter | Value of the parameter |
GWO | [18] | a | [0, 2] |
AVAO | [53] | L1, L2, w, p1, p2, p3 | 0.8, 0.2, 2.5, 0.6, 0.4, 0.6 |
GTO | [54] | P, r1, r2, r3 | P, r1, r2, r3 ∈ [0, 1] |
DE | [5] | F, CR | 0.8, 0.1 |
COA | [21] | temp | [20, 35] |
PSO | [17] | w, c1, c2 | [0.9, 0.4], 0.2, 0.2 |
SBOA | [25] | R1, RB, r, R2 | [0, 1], [0, 1], [0, 1], [0, 1] |
Among metaheuristic algorithms, exploration can be defined as a global search that aims to discover better solutions in the global domain. In contrast, exploitation refers to local search that focuses on finding better solutions in a known smaller area. Balancing exploration and exploitation is of crucial importance for the adaptability and robustness of the algorithm. Therefore, Hussain et al. [55] proposed a method for measuring dimensional diversity. Equations (5.1) and (5.2) calculate the percentages of exploration and exploitation, respectively. Moreover, Div(t) is a measure of dimensional diversity calculated from Eq (5.3).
Exploration(%)=Div(t)Divmax×100, | (5.1) |
Exploitation(%)=|Div(t)−Divmax|Divmax×100, | (5.2) |
Div(t)=1DimDim∑d=11NN∑i=1|median(xd(t))−xid(t)|. | (5.3) |
Here, xid represents the dth dimension of the ith position, and Divmax denotes the maximum diversity throughout the iteration process, and median(xd(t)) indicates the median of the dth dimension.
Figure 2 presents the simulation results of CSBOA on six CEC2022 benchmark functions with 10 dimensions. According to research [56], the optimal balance between exploration and exploitation in the search process is 10% exploration and 90% exploitation. When this balance is achieved, the algorithm performs at its best. As shown in Figure 2, the intersection of the exploration and exploitation ratios in CSBOA primarily occurs during the early iterations of the search process. In the later stages, exploration drops below 10%, while exploitation rises above 90%, significantly enhancing the convergence accuracy of the algorithm. Therefore, CSBOA demonstrates a well-balanced trade-off between exploration and exploitation.
To boost the optimization performance of the conventional SBOA algorithm, we employ three improvement strategies, namely logistic-tent chaotic mapping, improved differential mutation operator, and crossover strategy. To confirm the effectiveness of each component, six CSBOA-derived algorithms with one or more fusion strategies are designed as shown in Table 4, where 1 represents that the strategy is embedded; and, 0 indicates that the strategy is not embedded. The performance of SBOA, CSBOA, and multiple CSBOA-derived variants are simultaneously evaluated for ablation in the CEC2022 test set, and the findings are documented in Table 5.
Strategy | CSBOA-1 | CSBOA-2 | CSBOA-3 | CSBOA-4 | CSBOA-5 | CSBOA-6 | CSBOA |
Logistic-tent chaotic mapping | 1 | 0 | 0 | 1 | 1 | 0 | 1 |
Improved differential mutation operator | 0 | 1 | 0 | 1 | 0 | 1 | 1 |
Crossover strategy | 0 | 0 | 1 | 0 | 1 | 1 | 1 |
Function | Metric | SBOA | CSBOA-1 | CSBOA-2 | CSBOA-3 | CSBOA-4 | CSBOA-5 | CSBOA-6 | CSBOA |
F1 | Ave | 468.0984 | 462.0113 | 360.6446 | 354.0001 | 387.1188 | 342.2765 | 300.0422 | 300.0315 |
Std | 152.9298 | 168.8314 | 58.5821 | 45.8167 | 73.5351 | 41.1544 | 0.1029 | 0.0485 | |
Rank | 8 | 7 | 5 | 4 | 6 | 3 | 2 | 1 | |
F2 | Ave | 454.3443 | 453.9698 | 450.5164 | 454.2942 | 448.7348 | 449.7043 | 448.4292 | 445.9545 |
Std | 11.3625 | 12.6751 | 8.4090 | 14.9607 | 17.7389 | 14.2097 | 14.6531 | 14.5031 | |
Rank | 8 | 6 | 5 | 7 | 3 | 4 | 2 | 1 | |
F3 | Ave | 600.0028 | 600.0102 | 600.0734 | 600.0090 | 600.0460 | 600.0024 | 600.0135 | 600.0185 |
Std | 0.0043 | 0.0473 | 0.2534 | 0.0389 | 0.1770 | 0.0036 | 0.0534 | 0.0816 | |
Rank | 2 | 4 | 8 | 3 | 7 | 1 | 5 | 6 | |
F4 | Ave | 832.8866 | 831.9954 | 835.5805 | 834.0843 | 833.6276 | 836.8336 | 833.9302 | 834.4919 |
Std | 9.9938 | 9.1814 | 14.3468 | 9.9029 | 14.4475 | 9.6940 | 11.8680 | 9.1798 | |
Rank | 2 | 1 | 7 | 5 | 3 | 8 | 4 | 6 | |
F5 | Ave | 902.4884 | 901.9341 | 901.9577 | 900.8848 | 902.2329 | 900.8906 | 901.1584 | 900.7389 |
Std | 2.7654 | 2.6170 | 3.2391 | 1.2353 | 2.0574 | 1.3218 | 1.7129 | 1.0435 | |
Rank | 8 | 5 | 6 | 2 | 7 | 3 | 4 | 1 | |
F6 | Ave | 7828.2811 | 6654.5031 | 6912.6022 | 7017.1025 | 6639.8939 | 7405.0108 | 1864.9143 | 1858.5483 |
Std | 6388.4941 | 5805.7743 | 5662.3248 | 5100.5059 | 4777.2112 | 6057.2718 | 23.3027 | 24.1598 | |
Rank | 8 | 4 | 5 | 6 | 3 | 7 | 2 | 1 | |
F7 | Ave | 2032.9970 | 2033.8804 | 2028.0807 | 2029.6145 | 2027.3489 | 2029.7563 | 2025.9835 | 2024.3204 |
Std | 9.8793 | 10.5050 | 7.0884 | 6.1269 | 4.6764 | 7.4310 | 5.7183 | 5.2719 | |
Rank | 7 | 8 | 4 | 5 | 3 | 6 | 2 | 1 | |
F8 | Ave | 2224.0843 | 2224.0779 | 2224.1301 | 2222.0094 | 2224.1719 | 2222.4307 | 2221.0820 | 2221.7982 |
Std | 1.9078 | 2.0098 | 1.9208 | 0.8741 | 2.0107 | 0.9763 | 2.4445 | 0.7588 | |
Rank | 6 | 5 | 7 | 3 | 8 | 4 | 1 | 2 | |
F9 | Ave | 2480.7814 | 2480.7813 | 2480.7813 | 2480.7813 | 2480.7813 | 2480.7813 | 2480.7813 | 2480.7813 |
Std | 0.0002 | 5.61E-05 | 8.45E-06 | 2.13E-07 | 1.67E-05 | 2.84E-07 | 4.19E-08 | 3.38E-08 | |
Rank | 8 | 7 | 6 | 4 | 5 | 3 | 2 | 1 | |
F10 | Ave | 2539.0264 | 2536.6607 | 2559.7474 | 2526.3202 | 2553.5768 | 2526.9783 | 2504.4556 | 2509.1785 |
Std | 60.6819 | 57.9123 | 166.4333 | 53.3955 | 174.2956 | 53.9692 | 39.6923 | 33.1152 | |
Rank | 6 | 5 | 8 | 3 | 7 | 4 | 1 | 2 | |
F11 | Ave | 2908.6735 | 2903.3341 | 2903.3341 | 2903.3333 | 2900.0008 | 2906.6667 | 2900.0000 | 2900.0000 |
Std | 69.4118 | 18.2574 | 18.2575 | 18.2574 | 0.0005 | 25.3708 | 6.60E-07 | 5.18E-07 | |
Rank | 8 | 5 | 6 | 4 | 3 | 7 | 2 | 1 | |
F12 | Ave | 2942.3513 | 2941.4095 | 2939.2758 | 2939.0545 | 2940.0607 | 2941.6061 | 2940.6537 | 2942.5940 |
Std | 6.2628 | 5.3666 | 3.9849 | 3.8635 | 4.5492 | 6.7650 | 4.8318 | 5.2684 | |
Rank | 7 | 5 | 2 | 1 | 3 | 6 | 4 | 8 |
As shown in Table 5, the full-fledged CSBOA with three strategies outperforms other comparison methods, yielding the most satisfactory mean fitness in 7 out of the 12 test cases, followed by CSBOA-6 in 3, CSBOA-5 in 1, and CSBOA-1 in 1. In terms of standard deviation, CSBOA had the most satisfactory standard deviation in 7 of the 12 test cases, followed by CSBOA-2 in 1, CSBOA-3 in 1, CSBOA-5 in 1, and CSBOA-6 in 1. According to the final Friedman mean ranking values, each CSBOA-derived variant benefiting from one single strategy addition shows a great improvement in overall optimization performance compared with the original SBOA, but lacks robustness, and the contribution effect of each strategy can be ranked as follows: A crossover strategy > improved differential mutation operator > logistic-tent chaotic mapping. For unimodal functions (F1), the solutions provided by CSBOA-6 is the closest to the theoretical value except for CSBOA, indicating that the crossover strategy and improved differential mutation operator are helpful to enhance the local search capability of SBOA. For multimodal functions (F2–F5) with many local minima, CSBOA-1 performs best on F4, CSBOA-5 provides the best performance on F3, and CSBOA is the winner on the rest of the benchmark functions. For hybrid and composition functions (F6–F12), the combination of crossover strategy and improved differential mutation operator remains powerful, CSBOA-6 performs best on F8 and F10, followed by CSBOA-6 on F6, F7, F9, and F11. These results demonstrate that the improvement strategy introduced in this paper can balance the advantages and drawbacks. The significance of the crossover strategy in complex optimization challenges cannot be overstated; it plays a pivotal role in balancing exploration and exploitation. Moreover, the improved differential mutation operator and logistic-tent chaotic mapping also contribute to enhancing the global exploration trend and expanding the search horizon respectively. The impact of a single strategy may be ambiguous, but the synergistic implementation of these three strategies is significant for boosting the overall performance of SBOA.
In this section, to comprehensively evaluate the performance of CSBOA, we conduct a comparative validation using the CEC2017 test functions [57]. As depicted in Table 6, the CEC2017 test functions are classified into four categories: Unimodal functions, multimodal functions, hybrid functions, and composite functions. Unimodal functions possess only a single global optimum and no local optima, rendering them suitable for evaluating the exploitation performance of an algorithm. Multimodal test functions contain multiple local optima and are primarily utilized to assess the algorithm's ability to find the global optimum and escape from local optima. Hybrid and composite functions are employed to gauge the algorithm's capability in handling complex and continuous problems. During the validation process using the CEC2017 test suite, tests were conducted in dimensions of 30 and 100. The corresponding results under different types and dimensions are presented in Tables 7–10, respectively. The three symbols (W|T|L) within the table signify the quantities of wins, draws, and losses of CSBOA when contrasted with the global optimum and standard deviation of its competitors. The best results for each test function and its corresponding dimension are highlighted in bold. Convergence curves for some of the functions can be observed in Figures 3 and 4.
Type | ID | CEC2017 function name | Dimension | fmin |
Unimodal | F1 | Shifted and rotated bent cigar function | 30/100 | 100 |
F2 | Shifted and rotated sum of different power function* | 30/100 | 200 | |
F3 | Shifted and rotated Zakharow function | 30/100 | 300 | |
Multimodal | F4 | Shifted and rotated Rosenbrock's function | 30/100 | 400 |
F5 | Shifted and rotated Rastrigin's Function | 30/100 | 500 | |
F6 | Shifted and rotated expanded Scaffer's F6 Function | 30/100 | 600 | |
F7 | Shifted and rotated Lunacek Bi-Rastrigin finc-tion | 30/100 | 700 | |
F8 | Shifted and rotated non-continuous Rastrigin's function | 30/100 | 800 | |
F9 | Shifted and rotated levy function | 30/100 | 900 | |
F10 | Shifted and rotated Schwefel's function | 30/100 | 1000 | |
Hybrid | F11 | Hybrid function 1 (N = 3) | 30/100 | 1100 |
F12 | Hybrid function 2 (N = 3) | 30/100 | 1200 | |
F13 | Hybrid function 3 (N = 3) | 30/100 | 1300 | |
F14 | Hybrid function 4 (N = 4) | 30/100 | 1400 | |
F15 | Hybrid function 5 (N = 4) | 30/100 | 1500 | |
F16 | Hybrid function 6 (N = 4) | 30/100 | 1600 | |
F17 | Hybrid function 7 (N = 5) | 30/100 | 1700 | |
F18 | Hybrid function 8 (N = 5) | 30/100 | 1800 | |
F19 | Hybrid function 9 (N = 5) | 30/100 | 1900 | |
F20 | Hybrid function 10 (N = 6) | 30/100 | 2000 | |
Composition | F21 | Composition function 1 (N = 3) | 30/100 | 2100 |
F22 | Composition function 2 (N = 3) | 30/100 | 2200 | |
F23 | Composition function 3 (N = 4) | 30/100 | 2300 | |
F24 | Composition function 4 (N = 4) | 30/100 | 2400 | |
F25 | Composition function 5 (N = 5) | 30/100 | 2500 | |
F26 | Composition function 6 (N = 5) | 30/100 | 2600 | |
F27 | Composition function 7 (N = 6) | 30/100 | 2700 | |
F28 | Composition function 8 (N = 6) | 30/100 | 2800 | |
F29 | Composition function 9 (N = 3) | 30/100 | 2900 | |
F30 | Composition function 10 (N = 3) | 30/100 | 3000 | |
Search Range [−100,100]D | ||||
F2 has been officially removed due to its unstable behavior on high-dimensional issues. |
Function | GWO | AVAO | GTO | DE | COA | PSO | SBOA | CSBOA | |
F1 | Ave | 1.18E+09 | 3.98E+03 | 4.73E+03 | 1.87E+06 | 5.17E+06 | 9.01E+08 | 6.67E+03 | 2.26E+03 |
Std | 9.70E+08 | 4.64E+03 | 6.14E+03 | 5.62E+05 | 9.56E+06 | 1.20E+09 | 6.76E+03 | 3.48E+03 | |
F3 | Ave | 4.26E+04 | 2.28E+04 | 1.92E+03 | 1.48E+05 | 8.38E+04 | 2.51E+04 | 5.12E+03 | 9.70E+02 |
Std | 9.88E+03 | 4.91E+03 | 1.70E+03 | 1.75E+04 | 1.88E+04 | 1.14E+04 | 2.70E+03 | 4.02E+02 | |
F4 | Ave | 5.71E+02 | 5.05E+02 | 4.96E+02 | 5.12E+02 | 5.29E+02 | 5.60E+02 | 5.00E+02 | 4.88E+02 |
Std | 4.53E+01 | 3.26E+01 | 2.94E+01 | 8.35E+00 | 2.78E+01 | 9.74E+01 | 3.35E+01 | 2.04E+01 | |
F5 | Ave | 5.96E+02 | 7.01E+02 | 6.89E+02 | 6.83E+02 | 7.18E+02 | 5.79E+02 | 5.68E+02 | 5.69E+02 |
Std | 3.54E+01 | 3.52E+01 | 4.64E+01 | 1.17E+01 | 6.03E+01 | 2.43E+01 | 1.55E+01 | 1.91E+01 | |
F6 | Ave | 6.06E+02 | 6.46E+02 | 6.36E+02 | 6.00E+02 | 6.40E+02 | 6.03E+02 | 6.00E+02 | 6.00E+02 |
Std | 2.13E+00 | 1.01E+01 | 9.25E+00 | 2.21E-02 | 1.46E+01 | 2.52E+00 | 4.12E-01 | 2.76E-01 | |
F7 | Ave | 8.65E+02 | 1.11E+03 | 1.02E+03 | 9.17E+02 | 1.12E+03 | 8.15E+02 | 8.11E+02 | 8.22E+02 |
Std | 4.65E+01 | 5.90E+01 | 6.59E+01 | 1.03E+01 | 1.55E+02 | 3.03E+01 | 2.84E+01 | 2.84E+01 | |
F8 | Ave | 8.93E+02 | 9.56E+02 | 9.43E+02 | 9.85E+02 | 9.67E+02 | 8.71E+02 | 8.59E+02 | 8.72E+02 |
Std | 4.51E+01 | 3.09E+01 | 2.73E+01 | 9.75E+00 | 3.34E+01 | 1.99E+01 | 1.54E+01 | 1.56E+01 | |
F9 | Ave | 1.42E+03 | 4.71E+03 | 3.59E+03 | 1.91E+03 | 6.17E+03 | 1.02E+03 | 9.62E+02 | 9.39E+02 |
Std | 3.24E+02 | 7.28E+02 | 9.82E+02 | 2.11E+02 | 2.17E+03 | 1.14E+02 | 8.84E+01 | 4.90E+01 | |
F10 | Ave | 4.48E+03 | 5.38E+03 | 5.30E+03 | 7.31E+03 | 5.65E+03 | 4.54E+03 | 3.99E+03 | 3.70E+03 |
Std | 1.17E+03 | 6.76E+02 | 9.07E+02 | 3.41E+02 | 6.68E+02 | 7.32E+02 | 7.51E+02 | 4.41E+02 | |
(W|T|L) | Ave | (9|0|0) | (9|0|0) | (9|0|0) | (9|0|0) | (9|0|0)) | (9|0|0) | (6|0|3) | |
Std | (9|0|0) | (9|0|0) | (9|0|0) | (3|0|6) | (9|0|0) | (9|0|0) | (7|0|2) |
Function | GWO | AVAO | GTO | DE | COA | PSO | SBOA | CSBOA | |
F11 | Ave | 1.75E+03 | 1.26E+03 | 1.23E+03 | 1.59E+03 | 1.36E+03 | 1.27E+03 | 1.18E+03 | 1.15E+03 |
Std | 7.82E+02 | 5.77E+01 | 4.11E+01 | 2.01E+02 | 7.20E+01 | 7.05E+01 | 3.30E+01 | 2.68E+01 | |
F12 | Ave | 4.62E+07 | 3.60E+06 | 3.59E+05 | 3.96E+07 | 4.71E+06 | 2.68E+07 | 7.42E+05 | 1.32E+05 |
Std | 6.14E+07 | 3.10E+06 | 3.49E+05 | 1.08E+07 | 4.78E+06 | 5.92E+07 | 8.17E+05 | 1.35E+05 | |
F13 | Ave | 5.14E+06 | 7.58E+04 | 1.54E+04 | 3.29E+06 | 1.07E+05 | 5.27E+06 | 2.56E+04 | 1.94E+03 |
Std | 2.31E+07 | 3.94E+04 | 1.46E+04 | 1.58E+06 | 9.63E+04 | 1.81E+07 | 2.26E+04 | 3.34E+02 | |
F14 | Ave | 1.43E+05 | 1.34E+05 | 2.00E+03 | 2.06E+05 | 1.84E+05 | 3.20E+04 | 1.81E+04 | 1.45E+03 |
Std | 2.09E+05 | 1.24E+05 | 1.00E+03 | 1.17E+05 | 3.55E+05 | 3.88E+04 | 1.75E+04 | 8.55E+00 | |
F15 | Ave | 4.67E+05 | 2.42E+04 | 8.67E+03 | 5.30E+05 | 1.72E+04 | 2.32E+04 | 1.15E+04 | 1.60E+03 |
Std | 1.12E+06 | 1.67E+04 | 7.31E+03 | 2.81E+05 | 1.52E+04 | 2.21E+04 | 1.17E+04 | 3.92E+01 | |
F16 | Ave | 2.46E+03 | 2.89E+03 | 2.76E+03 | 2.80E+03 | 2.79E+03 | 2.51E+03 | 2.23E+03 | 2.13E+03 |
Std | 2.44E+02 | 3.81E+02 | 3.02E+02 | 2.02E+02 | 4.31E+02 | 2.71E+02 | 2.44E+02 | 1.97E+02 | |
F17 | Ave | 2.00E+03 | 2.48E+03 | 2.26E+03 | 2.09E+03 | 2.19E+03 | 2.00E+03 | 1.87E+03 | 1.83E+03 |
Std | 1.56E+02 | 2.29E+02 | 2.27E+02 | 7.95E+01 | 1.98E+02 | 1.74E+02 | 1.14E+02 | 7.07E+01 | |
F18 | Ave | 1.23E+06 | 1.19E+06 | 4.10E+04 | 1.76E+06 | 1.38E+06 | 6.57E+05 | 3.17E+05 | 1.99E+03 |
Std | 1.19E+06 | 1.17E+06 | 2.43E+04 | 7.29E+05 | 1.94E+06 | 6.89E+05 | 2.54E+05 | 5.32E+01 | |
F19 | Ave | 4.85E+05 | 2.42E+04 | 6.04E+03 | 3.48E+05 | 1.70E+04 | 2.44E+04 | 1.70E+04 | 1.93E+03 |
Std | 5.64E+05 | 5.06E+04 | 5.81E+03 | 2.02E+05 | 1.85E+04 | 4.03E+04 | 1.87E+04 | 9.76E+00 | |
F20 | Ave | 2.35E+03 | 2.64E+03 | 2.47E+03 | 2.41E+03 | 2.58E+03 | 2.33E+03 | 2.22E+03 | 2.16E+03 |
Std | 1.30E+02 | 2.56E+02 | 1.85E+02 | 1.04E+02 | 2.07E+02 | 1.32E+02 | 9.27E+01 | 7.40E+01 | |
F21 | Ave | 2.39E+03 | 2.50E+03 | 2.41E+03 | 2.48E+03 | 2.41E+03 | 2.38E+03 | 2.36E+03 | 2.35E+03 |
Std | 2.55E+01 | 4.99E+01 | 7.71E+01 | 1.04E+01 | 3.25E+01 | 2.54E+01 | 1.73E+01 | 1.16E+01 | |
F22 | Ave | 4.22E+03 | 6.13E+03 | 2.69E+03 | 4.97E+03 | 2.97E+03 | 4.01E+03 | 2.52E+03 | 2.55E+03 |
Std | 1.90E+03 | 2.00E+03 | 1.22E+03 | 6.54E+02 | 1.48E+03 | 1.65E+03 | 8.57E+02 | 9.48E+02 | |
F23 | Ave | 2.75E+03 | 2.96E+03 | 2.83E+03 | 2.83E+03 | 2.79E+03 | 2.83E+03 | 2.70E+03 | 2.71E+03 |
Std | 4.36E+01 | 7.30E+01 | 6.56E+01 | 1.14E+01 | 3.94E+01 | 6.25E+01 | 1.36E+01 | 1.84E+01 | |
F24 | Ave | 2.93E+03 | 3.11E+03 | 3.01E+03 | 3.03E+03 | 2.95E+03 | 2.99E+03 | 2.87E+03 | 2.88E+03 |
Std | 6.71E+01 | 8.18E+01 | 6.85E+01 | 9.70E+00 | 4.15E+01 | 5.85E+01 | 1.40E+01 | 1.93E+01 | |
F25 | Ave | 2.96E+03 | 2.91E+03 | 2.91E+03 | 2.90E+03 | 2.92E+03 | 2.92E+03 | 2.89E+03 | 2.89E+03 |
Std | 1.94E+01 | 3.02E+01 | 2.04E+01 | 3.13E+00 | 2.22E+01 | 5.43E+01 | 1.53E+01 | 1.02E+01 | |
F26 | Ave | 4.54E+03 | 6.51E+03 | 4.78E+03 | 5.46E+03 | 5.60E+03 | 4.69E+03 | 3.99E+03 | 3.98E+03 |
Std | 3.24E+02 | 8.85E+02 | 1.60E+03 | 1.10E+02 | 1.47E+03 | 4.10E+02 | 5.79E+02 | 7.09E+02 | |
F27 | Ave | 3.24E+03 | 3.27E+03 | 3.29E+03 | 3.23E+03 | 3.25E+03 | 3.25E+03 | 3.21E+03 | 3.21E+03 |
Std | 1.91E+01 | 4.26E+01 | 4.77E+01 | 3.53E+00 | 2.63E+01 | 3.60E+01 | 9.44E+00 | 1.10E+01 | |
F28 | Ave | 3.37E+03 | 3.26E+03 | 3.23E+03 | 3.30E+03 | 3.26E+03 | 3.32E+03 | 3.22E+03 | 3.22E+03 |
Std | 6.42E+01 | 2.76E+01 | 2.11E+01 | 1.67E+01 | 3.31E+01 | 1.28E+02 | 2.11E+01 | 2.01E+01 | |
F29 | Ave | 3.77E+03 | 4.31E+03 | 4.16E+03 | 4.07E+03 | 3.96E+03 | 3.67E+03 | 3.49E+03 | 3.52E+03 |
Std | 1.39E+02 | 2.55E+02 | 4.02E+02 | 1.20E+02 | 2.09E+02 | 1.65E+02 | 1.12E+02 | 9.41E+01 | |
F30 | Ave | 9.71E+06 | 2.05E+05 | 1.37E+04 | 3.37E+05 | 1.96E+05 | 4.89E+04 | 2.39E+04 | 1.16E+04 |
Std | 6.16E+06 | 1.19E+05 | 6.95E+03 | 1.20E+05 | 2.06E+05 | 7.51E+04 | 2.09E+04 | 2.22E+03 | |
(W|T|L) | Ave | (20|0|0) | (20|0|0) | (20|0|0) | (20|0|0) | (20|0|0)) | (20|0|0) | (15|0|5) | |
Std | (20|0|0) | (20|0|0) | (20|0|0) | (12|0|8) | (20|0|0) | (20|0|0) | (20|0|0) |
Function | GWO | AVAO | GTO | DE | COA | PSO | SBOA | CSBOA | |
F1 | Ave | 3.23E+10 | 1.49E+08 | 1.39E+09 | 7.42E+09 | 2.58E+10 | 2.64E+10 | 2.17E+09 | 1.71E+09 |
Std | 7.93E+09 | 1.63E+08 | 1.26E+09 | 9.99E+08 | 8.15E+09 | 1.11E+10 | 1.80E+09 | 2.21E+09 | |
F3 | Ave | 3.54E+05 | 3.09E+05 | 1.68E+05 | 7.65E+05 | 6.21E+05 | 4.88E+05 | 2.59E+05 | 1.52E+05 |
Std | 3.95E+04 | 1.86E+04 | 2.15E+04 | 6.83E+04 | 9.67E+04 | 1.02E+05 | 2.50E+04 | 1.78E+04 | |
F4 | Ave | 3.02E+03 | 1.13E+03 | 1.38E+03 | 2.31E+03 | 3.02E+03 | 2.51E+03 | 1.14E+03 | 1.05E+03 |
Std | 8.11E+02 | 3.93E+02 | 1.83E+02 | 1.80E+02 | 7.87E+02 | 9.18E+02 | 1.19E+02 | 1.25E+02 | |
F5 | Ave | 1.14E+03 | 1.32E+03 | 1.34E+03 | 1.65E+03 | 1.42E+03 | 1.07E+03 | 1.04E+03 | 1.09E+03 |
Std | 1.04E+02 | 7.27E+01 | 5.05E+01 | 2.60E+01 | 3.38E+01 | 1.23E+02 | 6.25E+01 | 8.70E+01 | |
F6 | Ave | 6.32E+02 | 6.62E+02 | 6.62E+02 | 6.25E+02 | 6.68E+02 | 6.31E+02 | 6.26E+02 | 6.23E+02 |
Std | 4.44E+00 | 3.89E+00 | 4.71E+00 | 1.03E+00 | 1.94E+00 | 8.38E+00 | 5.90E+00 | 1.11E+01 | |
F7 | Ave | 1.87E+03 | 3.08E+03 | 2.89E+03 | 2.16E+03 | 3.18E+03 | 1.78E+03 | 1.92E+03 | 1.88E+03 |
Std | 1.38E+02 | 1.64E+02 | 2.12E+02 | 3.51E+01 | 2.82E+02 | 1.97E+02 | 1.21E+02 | 2.26E+02 | |
F8 | Ave | 1.44E+03 | 1.73E+03 | 1.70E+03 | 1.92E+03 | 1.90E+03 | 1.34E+03 | 1.37E+03 | 1.37E+03 |
Std | 1.09E+02 | 8.81E+01 | 1.01E+02 | 2.76E+01 | 5.28E+01 | 7.79E+01 | 6.87E+01 | 9.42E+01 | |
F9 | Ave | 3.37E+04 | 2.52E+04 | 2.45E+04 | 5.78E+04 | 3.99E+04 | 4.28E+04 | 2.10E+04 | 2.07E+04 |
Std | 9.40E+03 | 2.20E+03 | 2.31E+03 | 5.07E+03 | 9.91E+03 | 1.81E+04 | 3.13E+03 | 3.49E+03 | |
F10 | Ave | 1.72E+04 | 1.70E+04 | 1.72E+04 | 3.15E+04 | 2.22E+04 | 1.73E+04 | 1.62E+04 | 1.52E+04 |
Std | 4.32E+03 | 1.69E+03 | 2.31E+03 | 5.22E+02 | 2.78E+03 | 2.27E+03 | 1.35E+03 | 1.24E+03 | |
(W|T|L) | Ave | (9|0|0) | (8|0|1) | (8|0|1) | (9|0|0) | (9|0|0) | (7|0|2) | (7|0|2) | |
Std | (9|0|0) | (8|0|1) | (9|0|0) | (4|0|5) | (9|0|0) | (8|0|1) | (9|0|0) |
Function | GWO | AVAO | GTO | DE | COA | PSO | SBOA | CSBOA | |
F11 | Ave | 5.83E+04 | 3.30E+04 | 8.73E+03 | 2.32E+05 | 2.39E+05 | 2.88E+04 | 1.45E+04 | 4.64E+03 |
Std | 1.51E+04 | 8.89E+03 | 2.86E+03 | 2.95E+04 | 6.36E+04 | 8.29E+03 | 4.90E+03 | 9.91E+02 | |
F12 | Ave | 5.23E+09 | 2.73E+08 | 1.41E+08 | 5.44E+09 | 1.66E+09 | 8.98E+09 | 1.19E+08 | 7.16E+07 |
Std | 2.90E+09 | 1.34E+08 | 5.74E+07 | 5.88E+08 | 2.11E+09 | 6.09E+09 | 5.65E+07 | 3.34E+07 | |
F13 | Ave | 6.64E+08 | 8.03E+04 | 2.70E+04 | 1.61E+06 | 9.58E+06 | 9.76E+08 | 1.51E+04 | 9.33E+03 |
Std | 6.53E+08 | 2.99E+04 | 1.09E+04 | 1.47E+06 | 2.81E+07 | 1.41E+09 | 6.61E+03 | 8.33E+03 | |
F14 | Ave | 5.72E+06 | 3.40E+06 | 4.74E+05 | 3.48E+07 | 4.81E+06 | 3.63E+06 | 2.56E+06 | 7.00E+04 |
Std | 3.40E+06 | 1.84E+06 | 2.07E+05 | 8.23E+06 | 3.11E+06 | 2.39E+06 | 1.45E+06 | 7.12E+04 | |
F15 | Ave | 1.10E+08 | 6.39E+04 | 1.15E+04 | 4.84E+06 | 2.02E+05 | 3.20E+08 | 5.27E+03 | 6.93E+03 |
Std | 1.21E+08 | 2.91E+04 | 5.91E+03 | 3.02E+06 | 1.76E+05 | 5.03E+08 | 4.20E+03 | 6.48E+03 | |
F16 | Ave | 5.81E+03 | 6.62E+03 | 6.40E+03 | 1.11E+04 | 7.15E+03 | 6.00E+03 | 5.16E+03 | 5.16E+03 |
Std | 6.15E+02 | 8.47E+02 | 8.64E+02 | 2.39E+02 | 8.58E+02 | 7.22E+02 | 7.38E+02 | 6.78E+02 | |
F17 | Ave | 4.93E+03 | 6.07E+03 | 5.79E+03 | 7.74E+03 | 6.00E+03 | 6.12E+03 | 4.57E+03 | 4.46E+03 |
Std | 5.39E+02 | 5.95E+02 | 6.79E+02 | 2.37E+02 | 7.65E+02 | 1.15E+03 | 5.45E+02 | 4.77E+02 | |
F18 | Ave | 5.63E+06 | 3.77E+06 | 8.51E+05 | 6.95E+07 | 4.77E+06 | 8.01E+06 | 3.25E+06 | 2.54E+05 |
Std | 2.73E+06 | 2.01E+06 | 4.73E+05 | 1.79E+07 | 2.19E+06 | 5.02E+06 | 1.45E+06 | 1.26E+05 | |
F19 | Ave | 1.14E+08 | 3.35E+05 | 1.08E+04 | 7.41E+06 | 2.13E+06 | 1.78E+08 | 6.98E+03 | 8.21E+03 |
Std | 1.26E+08 | 2.00E+05 | 7.47E+03 | 4.91E+06 | 1.07E+06 | 2.94E+08 | 7.52E+03 | 9.33E+03 | |
F20 | Ave | 4.94E+03 | 5.98E+03 | 5.39E+03 | 7.51E+03 | 6.91E+03 | 5.26E+03 | 4.65E+03 | 4.29E+03 |
Std | 9.73E+02 | 5.55E+02 | 5.02E+02 | 2.76E+02 | 5.58E+02 | 6.72E+02 | 5.72E+02 | 5.12E+02 | |
F21 | Ave | 2.95E+03 | 3.54E+03 | 3.27E+03 | 3.47E+03 | 3.45E+03 | 3.04E+03 | 2.81E+03 | 2.86E+03 |
Std | 7.02E+01 | 1.42E+02 | 1.15E+02 | 3.18E+01 | 1.69E+02 | 1.01E+02 | 5.89E+01 | 8.82E+01 | |
F22 | Ave | 2.06E+04 | 2.03E+04 | 2.25E+04 | 3.39E+04 | 2.85E+04 | 1.96E+04 | 1.92E+04 | 1.85E+04 |
Std | 4.97E+03 | 1.48E+03 | 3.57E+03 | 5.13E+02 | 2.68E+03 | 2.03E+03 | 1.95E+03 | 1.32E+03 | |
F23 | Ave | 3.54E+03 | 4.09E+03 | 4.14E+03 | 3.79E+03 | 4.02E+03 | 4.30E+03 | 3.27E+03 | 3.24E+03 |
Std | 8.75E+01 | 1.64E+02 | 2.28E+02 | 2.88E+01 | 1.66E+02 | 2.29E+02 | 5.96E+01 | 7.85E+01 | |
F24 | Ave | 4.12E+03 | 5.08E+03 | 4.94E+03 | 4.33E+03 | 4.87E+03 | 5.26E+03 | 3.83E+03 | 3.87E+03 |
Std | 1.44E+02 | 2.97E+02 | 4.27E+02 | 3.06E+01 | 2.36E+02 | 4.11E+02 | 7.23E+01 | 8.76E+01 | |
F25 | Ave | 5.53E+03 | 3.75E+03 | 3.97E+03 | 6.69E+03 | 5.21E+03 | 4.47E+03 | 3.76E+03 | 3.74E+03 |
Std | 5.44E+02 | 8.51E+01 | 1.51E+02 | 3.70E+02 | 5.42E+02 | 7.75E+02 | 9.88E+01 | 1.22E+02 | |
F26 | Ave | 1.36E+04 | 2.37E+04 | 2.30E+04 | 1.68E+04 | 2.63E+04 | 1.89E+04 | 1.41E+04 | 1.33E+04 |
Std | 8.25E+02 | 2.17E+03 | 3.20E+03 | 2.86E+02 | 4.30E+03 | 3.77E+03 | 2.88E+03 | 1.61E+03 | |
F27 | Ave | 3.94E+03 | 4.12E+03 | 4.06E+03 | 4.36E+03 | 4.15E+03 | 4.00E+03 | 3.57E+03 | 3.50E+03 |
Std | 1.18E+02 | 2.91E+02 | 3.15E+02 | 9.78E+01 | 2.02E+02 | 2.92E+02 | 7.28E+01 | 7.00E+01 | |
F28 | Ave | 7.12E+03 | 4.01E+03 | 4.08E+03 | 1.29E+04 | 6.65E+03 | 6.55E+03 | 4.03E+03 | 3.80E+03 |
Std | 1.38E+03 | 1.40E+02 | 1.72E+02 | 8.70E+02 | 8.33E+02 | 1.89E+03 | 1.81E+02 | 1.19E+02 | |
F29 | Ave | 7.99E+03 | 8.57E+03 | 8.20E+03 | 1.03E+04 | 9.29E+03 | 7.48E+03 | 6.53E+03 | 6.45E+03 |
Std | 6.02E+02 | 6.07E+02 | 9.11E+02 | 2.17E+02 | 8.70E+02 | 6.33E+02 | 4.69E+02 | 3.67E+02 | |
F30 | Ave | 7.02E+08 | 8.35E+06 | 1.03E+06 | 1.50E+07 | 3.99E+07 | 1.12E+09 | 1.88E+05 | 5.82E+04 |
Std | 7.56E+08 | 4.85E+06 | 7.01E+05 | 3.11E+06 | 2.20E+07 | 1.00E+09 | 1.05E+05 | 3.13E+04 | |
(W|T|L) | Ave | (20|0|0) | (20|0|0) | (20|0|0) | (11|0|9) | (20|0|0) | (20|0|2) | (15|0|5) | |
Std | (20|0|0) | (19|0|1) | (19|0|1) | (16|0|14) | (20|0|0) | (20|0|0) | (13|0|7) |
In the analysis of the CEC2017 algorithm testing, the function results across dimensions illustrate the performance of the CSBOA algorithm. As shown in Table 7 (30-dimensional unimodal and multimodal functions), for the unimodal functions F1 and F3, CSBOA significantly outperforms other algorithms in terms of both the global optimal mean and standard deviation, demonstrating its precise optimization capabilities. In the multimodal functions (F4 to F10), CSBOA performs better in terms of the mean for functions F4, F6, F9, and F10. For functions F5, F7, and F8, its performance ranks second only to SBOA. Only on the F9 function, the standard deviation of CSBOA is slightly worse than DE; moreover, the difference is relatively small. Overall, in the 30-dimensional unimodal and multimodal function tests, CSBOA shows advantages in both mean performance and result stability.
In Table 8 (30-dimensional hybrid and composition functions), CSBOA surpasses the comparative algorithms in both the global optimal means and standard deviations for the hybrid functions (F11 to F20). For the composition functions (F21 to F30), CSBOA and SBOA show comparable advantages, ranking among the top algorithms. Although CSBOA slightly lags behind DE in terms of the stability of the standard deviation, it outperforms DE in the optimal mean. Among the 20 functions, CSBOA excels in the means of 15 functions and the standard deviations of 11 functions, demonstrating its effectiveness and robustness.
Table 9 (100-dimensional unimodal and multimodal functions) shows that CSBOA outperforms the comparative algorithms in terms of the optimal means for 5 functions, with standard deviations within a reasonable range. Table 10 (100-dimensional hybrid and composition functions) reveals that, among the 20 hybrid and composition functions, CSBOA achieves the best means for 15 functions, with the standard deviations performing well.
Figures 3 and 4 show the partial convergence curves for the CEC2017 test functions in 30 and 100 dimensions, respectively. From the graphs, it is evident that, in most test cases, CSBOA converges to the optimal solution within 500 consecutive iterations, demonstrating its outstanding exploration and exploitation capabilities. For unimodal functions (F1–F3), CSBOA exhibits superior convergence speed and accuracy compared to other algorithms. In the later stages, while most comparative algorithms experience stagnation at local optima, CSBOA continues progressing towards higher accuracy, showcasing its robust exploitation abilities. For multimodal functions (F4–F10), CSBOA initially lags behind GWO and AVAO in terms of convergence speed and accuracy during the first few dozen iterations. However, as iterations progress, CSBOA exhibits significant advantages, leading to both convergence accuracy and speed in the later stages. For mixed functions (F11–F20), CSBOA reaches the optimal position around the 200th iteration and maintains this advantage until the end. In the case of composite functions (F21–F30), despite the high complexity, CSBOA maintains strong exploration capabilities and rapid convergence.
These results indicate that the strategies introduced in this paper enhance the convergence rate and overall search performance of the basic SBOA in solving the CEC2017 functions, while preventing stagnation at local optima. The crossover strategy accelerates CSBOA's convergence towards the global optimum by iteratively updating the optimal solution dimension by dimension. Therefore, it is reasonable to conclude that CSBOA can provide superior convergence patterns compared to other algorithms when addressing complex numerical optimization challenges in the future.
In this section, to evaluate the scalability of CSBOA, a comparative analysis is conducted between the improved CSBOA algorithm and seven other well-established benchmark algorithms. The comparison is implemented using the CEC2022 testing suite, which includes a diverse set of 10- and 20-dimensional problems. The test functions in CEC2022 consist of a combination of unimodal, multimodal, mixed, and composite functions [58], totaling twelve functions. For further details, please refer to Table 11. The test results for CSBOA and other seven algorithms on the CEC2022 test suites are provided in Tables 12 and 13, and the convergence curves are presented in Figures 5 and 6.
Type | ID | CEC2022 function name | Dimension | fmin |
Unimodal | F1 | Shifted and full rotated Zakharov function | 10/20 | 300 |
Multimodal | F2 | Shifted and full rotated Rosenbrock's function | 10/20 | 400 |
F3 | Shifted and full rotated Rastrigin's function | 10/20 | 600 | |
F4 | Shifted and full rotated non-continuous Rastrigin's function | 10/20 | 800 | |
F5 | Shifted and full rotated levy function | 10/20 | 900 | |
Hybrid | F6 | Hybrid function 1 (N = 3) | 10/20 | 1800 |
F7 | Hybrid function 2 (N = 6) | 10/20 | 2000 | |
F8 | Hybrid function 3 (N = 5) | 10/20 | 2200 | |
Composition | F9 | Composition function 1 (N = 5) | 10/20 | 2300 |
F10 | Composition function 2 (N = 4) | 10/20 | 2400 | |
F11 | Composition function 3 (N = 5) | 10/20 | 2600 | |
F12 | Composition function 4 (N = 6) | 10/20 | 2700 | |
Search Range [−100,100]D |
Function | GWO | AVAO | GTO | DE | COA | PSO | SBOA | CSBOA | |
F1 | Ave | 1.10E+03 | 3.00E+02 | 3.00E+02 | 4.55E+03 | 1.01E+03 | 3.00E+02 | 3.00E+02 | 3.00E+02 |
Std | 1.35E+03 | 1.13E-07 | 4.31E-13 | 1.44E+03 | 6.70E+02 | 2.01E-06 | 5.46E-04 | 4.72E-14 | |
F2 | Ave | 4.23E+02 | 4.10E+02 | 4.05E+02 | 4.08E+02 | 4.06E+02 | 4.13E+02 | 4.05E+02 | 4.05E+02 |
Std | 2.34E+01 | 1.75E+01 | 3.12E+00 | 4.75E-01 | 3.30E+00 | 1.82E+01 | 3.71E+00 | 3.21E+00 | |
F3 | Ave | 6.01E+02 | 6.13E+02 | 6.03E+02 | 6.00E+02 | 6.03E+02 | 6.00E+02 | 6.00E+02 | 6.00E+02 |
Std | 8.63E-01 | 1.02E+01 | 3.89E+00 | 1.16E-07 | 5.46E+00 | 4.17E-01 | 2.60E-07 | 9.15E-06 | |
F4 | Ave | 8.13E+02 | 8.30E+02 | 8.22E+02 | 8.25E+02 | 8.32E+02 | 8.12E+02 | 8.10E+02 | 8.07E+02 |
Std | 7.78E+00 | 1.11E+01 | 7.75E+00 | 4.15E+00 | 5.72E+00 | 4.70E+00 | 4.60E+00 | 2.74E+00 | |
F5 | Ave | 9.05E+02 | 1.14E+03 | 9.16E+02 | 9.00E+02 | 9.33E+02 | 9.00E+02 | 9.00E+02 | 9.00E+02 |
Std | 1.45E+01 | 2.06E+02 | 1.44E+01 | 8.38E-02 | 1.06E+02 | 9.49E-02 | 3.70E-10 | 3.21E-13 | |
F6 | Ave | 6.35E+03 | 4.18E+03 | 1.88E+03 | 6.66E+03 | 4.57E+03 | 5.02E+03 | 3.76E+03 | 1.80E+03 |
Std | 2.30E+03 | 2.21E+03 | 6.15E+01 | 3.48E+03 | 2.22E+03 | 2.59E+03 | 1.60E+03 | 4.26E-01 | |
F7 | Ave | 2.03E+03 | 2.03E+03 | 2.02E+03 | 2.01E+03 | 2.02E+03 | 2.01E+03 | 2.01E+03 | 2.00E+03 |
Std | 1.01E+01 | 9.55E+00 | 1.07E+01 | 2.10E+00 | 8.42E+00 | 9.82E+00 | 9.51E+00 | 3.68E+00 | |
F8 | Ave | 2.22E+03 | 2.22E+03 | 2.22E+03 | 2.22E+03 | 2.23E+03 | 2.22E+03 | 2.21E+03 | 2.20E+03 |
Std | 6.43E+00 | 4.08E+00 | 5.86E+00 | 4.08E+00 | 5.18E+00 | 8.21E+00 | 9.57E+00 | 6.11E+00 | |
F9 | Ave | 2.55E+03 | 2.53E+03 | 2.53E+03 | 2.53E+03 | 2.53E+03 | 2.53E+03 | 2.53E+03 | 2.53E+03 |
Std | 3.17E+01 | 2.68E+01 | 1.41E-01 | 2.46E-12 | 4.35E-05 | 2.68E+01 | 8.44E-14 | 8.44E-14 | |
F10 | Ave | 2.53E+03 | 2.55E+03 | 2.51E+03 | 2.50E+03 | 2.53E+03 | 2.55E+03 | 2.51E+03 | 2.52E+03 |
Std | 5.21E+01 | 6.53E+01 | 3.01E+01 | 1.24E-01 | 5.12E+01 | 5.72E+01 | 3.68E+01 | 4.34E+01 | |
F11 | Ave | 2.92E+03 | 2.70E+03 | 2.62E+03 | 2.88E+03 | 2.77E+03 | 2.68E+03 | 2.75E+03 | 2.71E+03 |
Std | 8.73E+01 | 1.52E+02 | 7.71E+01 | 3.99E+01 | 1.35E+02 | 1.42E+02 | 1.55E+02 | 1.47E+02 | |
F12 | Ave | 2.86E+03 | 2.87E+03 | 2.86E+03 | 2.86E+03 | 2.86E+03 | 2.87E+03 | 2.86E+03 | 2.86E+03 |
Std | 1.71E+00 | 4.47E+00 | 1.75E+00 | 9.88E-01 | 1.64E+00 | 1.30E+01 | 1.68E+00 | 1.67E+00 | |
(W|T|L) | Ave | (12|0|0) | (12|0|0) | (10|0|2) | (11|0|1) | (12|0|0) | (12|0|0) | (10|2|0) | |
Std | (12|0|0) | (11|0|1) | (11|0|1) | (7|0|5) | (12|0|0) | (12|0|0) | (12|0|0) |
Function | GWO | AVAO | GTO | DE | COA | PSO | SBOA | CSBOA | |
F1 | Ave | 9.15E+03 | 3.50E+03 | 3.01E+02 | 3.63E+04 | 2.94E+04 | 1.11E+03 | 4.68E+02 | 3.00E+02 |
Std | 4.52E+03 | 2.47E+03 | 1.92E+00 | 6.83E+03 | 7.90E+03 | 3.35E+02 | 1.41E+02 | 1.06E-01 | |
F2 | Ave | 4.85E+02 | 4.62E+02 | 4.51E+02 | 4.49E+02 | 4.66E+02 | 4.59E+02 | 4.54E+02 | 4.45E+02 |
Std | 3.14E+01 | 2.28E+01 | 1.53E+01 | 1.42E-01 | 1.86E+01 | 1.89E+01 | 1.18E+01 | 1.79E+01 | |
F3 | Ave | 6.03E+02 | 6.38E+02 | 6.23E+02 | 6.00E+02 | 6.20E+02 | 6.01E+02 | 6.00E+02 | 6.00E+02 |
Std | 1.65E+00 | 1.02E+01 | 8.07E+00 | 1.48E-03 | 1.46E+01 | 1.30E+00 | 2.80E-03 | 6.13E-02 | |
F4 | Ave | 8.59E+02 | 8.93E+02 | 8.73E+02 | 9.16E+02 | 8.81E+02 | 8.42E+02 | 8.32E+02 | 8.34E+02 |
Std | 2.89E+01 | 2.49E+01 | 2.00E+01 | 6.54E+00 | 1.52E+01 | 1.57E+01 | 8.71E+00 | 1.18E+01 | |
F5 | Ave | 9.90E+02 | 2.37E+03 | 1.68E+03 | 1.07E+03 | 2.06E+03 | 9.23E+02 | 9.02E+02 | 9.00E+02 |
Std | 6.19E+01 | 3.56E+02 | 3.66E+02 | 4.88E+01 | 6.81E+02 | 3.83E+01 | 1.65E+00 | 8.08E-01 | |
F6 | Ave | 2.30E+06 | 6.21E+03 | 3.76E+03 | 3.84E+06 | 7.61E+03 | 5.85E+04 | 7.28E+03 | 1.86E+03 |
Std | 6.06E+06 | 6.03E+03 | 2.42E+03 | 1.48E+06 | 5.60E+03 | 2.70E+05 | 5.83E+03 | 2.39E+01 | |
F7 | Ave | 2.06E+03 | 2.16E+03 | 2.09E+03 | 2.05E+03 | 2.07E+03 | 2.04E+03 | 2.03E+03 | 2.02E+03 |
Std | 3.29E+01 | 5.51E+01 | 2.91E+01 | 7.37E+00 | 2.98E+01 | 1.98E+01 | 8.77E+00 | 5.18E+00 | |
F8 | Ave | 2.25E+03 | 2.24E+03 | 2.23E+03 | 2.23E+03 | 2.25E+03 | 2.27E+03 | 2.22E+03 | 2.22E+03 |
Std | 4.61E+01 | 2.32E+01 | 3.13E+01 | 1.52E+00 | 3.57E+01 | 6.61E+01 | 2.06E+00 | 9.52E-01 | |
F9 | Ave | 2.50E+03 | 2.48E+03 | 2.48E+03 | 2.48E+03 | 2.48E+03 | 2.50E+03 | 2.48E+03 | 2.48E+03 |
Std | 1.25E+01 | 7.91E-01 | 2.20E-02 | 3.34E-03 | 9.05E-02 | 2.77E+01 | 3.40E-05 | 3.70E-08 | |
F10 | Ave | 3.01E+03 | 3.01E+03 | 2.94E+03 | 2.52E+03 | 3.40E+03 | 2.74E+03 | 2.53E+03 | 2.50E+03 |
Std | 6.22E+02 | 6.28E+02 | 9.52E+02 | 9.19E+00 | 1.19E+03 | 3.43E+02 | 5.69E+01 | 5.72E+01 | |
F11 | Ave | 3.37E+03 | 2.94E+03 | 2.96E+03 | 2.90E+03 | 3.01E+03 | 2.90E+03 | 2.91E+03 | 2.90E+03 |
Std | 1.87E+02 | 4.98E+01 | 8.97E+01 | 2.48E-02 | 4.65E+02 | 8.49E-04 | 7.73E-04 | 1.83E+01 | |
F12 | Ave | 2.96E+03 | 2.98E+03 | 3.00E+03 | 2.95E+03 | 2.98E+03 | 2.98E+03 | 2.94E+03 | 2.94E+03 |
Std | 9.07E+00 | 3.01E+01 | 4.55E+01 | 1.87E+00 | 1.88E+01 | 3.22E+01 | 8.79E+00 | 6.48E+00 | |
(W|T|L) | Ave | (12|0|0) | (12|0|0) | (12|0|0) | (11|0|1) | (12|0|0) | (12|0|0) | (9|2|1) | |
Std | (12|0|0) | (12|0|0) | (12|0|0) | (7|0|5) | (12|0|0) | (12|0|0) | (11|0|1) |
As shown in Table 12, when the dimension is set to 10, CSBOA has achieved highly satisfactory results. Among them, the global optimal fitness function values of five test functions, namely F1, F3, F5, F7, and F8, can attain the theoretical optimal state. The rest of the functions are also mostly at a relatively better level among all the compared algorithms. In terms of standard deviation, CSBOA presents a smaller standard deviation than other algorithms on F1, F4, F5, F6, and F9, indicating that the three introduced strategies are effective on SBOA and can have a relatively stable advantage on hybrid functions and composition functions. In summary, CSBOA exhibits exceptional performance on the 10-dimensional CEC2022 test function, strongly validating the excellent performance and strong robust optimization capability of the combination of the three strategies added to SBOA.
As presented in Table 13, within the 20-dimensional CEC2022 benchmark test set, the global optimal fitness values for the functions F1 and F3 reach the theoretical optimal values. Notably, the function F1 exhibits a single global optimum, highlighting the robust local exploitation capabilities of CSBOA. Additionally, the global optimal fitness values for eight functions—F2, F5, F6, F7, F8, F9, F11, and F12—are closer to the theoretical optimal values compared to other competing algorithms. The optimal values for functions F3 and F11 are comparable to those obtained by SBOA. In terms of standard deviation, for five functions (F2, F3, F4, F10, and F12), CSBOA's performance is inferior to that of the DE algorithm. However, CSBOA significantly outperforms DE in terms of convergence. Furthermore, CSBOA exhibits the smallest standard deviations for six functions—F1, F5, F6, F7, F8, and F9—demonstrating the highest stability among all comparison algorithms for these functions.
Tests comparing CSBOA with the SBOA algorithm and six other state-of-the-art algorithms on the 12 benchmark functions of CEC2022 demonstrate that CSBOA, enhanced by the logistic-tent chaotic mapping strategy, the improved differential mutation operator, and the crossover strategy, significantly improves optimization performance. It achieves a faster convergence rate, greater accuracy in convergence, and the ability to avoid local optima. These results further highlight that the integration of these three strategies substantially boosts the performance of SBOA.
Additionally, the partial convergence curves of the CEC2022 test functions in 10 and 20 dimensions are presented in Figures 5 and 6. For the unimodal function (F1), when the dimension is set to 10, CSBOA reaches its optimal value after the 100th iteration and outperforms other algorithms, maintaining this advantage throughout the remaining iterations. In the 20-dimensional case, CSBOA continues to optimize and progress until the 200th iteration, demonstrating strong exploitation capabilities. For the multimodal functions (F2–F5), CSBOA exhibits exceptional convergence speed, quickly locating the global optimum and effectively escaping local optima when confronted with multiple local minima. The convergence curve indicates that the algorithm performs excellently in multimodal functions. For the mixed functions (F6–F8) and the composite functions (F9–F12), which involve more complex environments, CSBOA achieves faster convergence and higher accuracy compared to other algorithms, highlighting its robust problem-solving ability in complex continuous optimization problems. In summary, the convergence characteristics in Figures 5 and 6 clearly demonstrate the effectiveness of the introduced mechanism, enabling CSBOA to strike an optimal balance between exploration and exploitation.
In this section, we carry out the Wilcoxon test and the Friedman test on CSBOA and other comparative algorithms. Subsequently, we evaluate the experimental results to conduct a quantitative analysis of the differences between CSBOA and other comparison algorithms.
To comprehensively demonstrate the superiority of the improved algorithm, in this section, we will use the Wilcoxon rank sum test to assess whether the results of each run of CSBOA significantly differ from those of other algorithms at a significance level of 5% [59]. The null hypothesis, denoted as H0, suggests that there is no significant difference between the two algorithms. If the significance probability is less than 0.05, we reject the null hypothesis, indicating a significant difference between the two algorithms. If the significance probability is greater than 0.05, we fail to reject the null hypothesis, suggesting no significant difference between the two algorithms, which implies that the operations performed by the algorithms are similar. The "NaN" value indicates that the two are incomparable and have similar performance. The test results of the CSBOA algorithm and the comparison algorithms on the 30 and 100 dimensions of the CEC2017 benchmark functions are shown in Tables 14 and 15, respectively. Additionally, the results for 10 and 20 dimensions in CEC2022 can be found in Tables 16 and 17. To highlight the comparative results, values exceeding the 5% significance level are presented in bold.
Function | GWO | AVAO | GTO | DE | COA | PSO | SBOA |
F1 | 3.02E-11 | 2.77E-01 | 8.77E-02 | 3.02E-11 | 3.02E-11 | 4.08E-05 | 1.17E-03 |
F3 | 3.02E-11 | 3.02E-11 | 1.95E-03 | 3.02E-11 | 3.02E-11 | 3.02E-11 | 6.70E-11 |
F4 | 2.61E-10 | 1.17E-02 | 1.09E-01 | 1.78E-04 | 1.16E-07 | 5.09E-06 | 6.15E-02 |
F5 | 2.39E-04 | 3.02E-11 | 3.69E-11 | 3.02E-11 | 3.34E-11 | 6.79E-02 | 9.71E-01 |
F6 | 3.02E-11 | 3.02E-11 | 3.02E-11 | 2.53E-04 | 3.02E-11 | 3.47E-10 | 1.26E-01 |
F7 | 1.25E-05 | 3.02E-11 | 3.02E-11 | 2.37E-10 | 4.08E-11 | 1.58E-01 | 2.01E-01 |
F8 | 3.51E-02 | 5.49E-11 | 6.07E-11 | 3.02E-11 | 5.49E-11 | 8.88E-01 | 2.89E-03 |
F9 | 5.49E-11 | 3.02E-11 | 3.02E-11 | 3.02E-11 | 3.02E-11 | 1.41E-04 | 8.77E-02 |
F10 | 3.56E-04 | 6.70E-11 | 1.41E-09 | 3.02E-11 | 3.34E-11 | 3.83E-06 | 3.27E-02 |
F11 | 3.02E-11 | 2.44E-09 | 3.50E-09 | 3.02E-11 | 3.02E-11 | 1.07E-09 | 2.39E-04 |
F12 | 3.02E-11 | 3.02E-11 | 2.25E-04 | 3.02E-11 | 3.02E-11 | 6.07E-11 | 1.70E-08 |
F13 | 3.02E-11 | 3.02E-11 | 3.34E-11 | 3.02E-11 | 3.02E-11 | 3.02E-11 | 2.03E-09 |
F14 | 3.02E-11 | 3.02E-11 | 3.02E-11 | 3.02E-11 | 3.02E-11 | 3.02E-11 | 3.02E-11 |
F15 | 3.02E-11 | 3.02E-11 | 3.34E-11 | 3.02E-11 | 3.02E-11 | 3.02E-11 | 3.02E-11 |
F16 | 2.15E-06 | 8.15E-11 | 2.87E-10 | 1.96E-10 | 1.10E-08 | 4.44E-07 | 6.57E-02 |
F17 | 5.46E-06 | 3.69E-11 | 1.46E-10 | 8.15E-11 | 3.47E-10 | 2.00E-05 | 3.55E-01 |
F18 | 3.02E-11 | 3.02E-11 | 3.02E-11 | 3.02E-11 | 3.02E-11 | 3.02E-11 | 3.02E-11 |
F19 | 3.02E-11 | 3.02E-11 | 3.02E-11 | 3.02E-11 | 3.02E-11 | 3.02E-11 | 3.16E-10 |
F20 | 3.35E-08 | 4.98E-11 | 1.17E-09 | 2.37E-10 | 1.17E-09 | 3.01E-07 | 3.03E-02 |
F21 | 4.44E-07 | 3.02E-11 | 2.20E-07 | 3.02E-11 | 2.67E-09 | 3.18E-04 | 2.75E-03 |
F22 | 3.20E-09 | 5.00E-09 | 3.32E-06 | 7.12E-09 | 5.97E-09 | 6.52E-09 | 1.25E-04 |
F23 | 9.83E-08 | 3.02E-11 | 1.61E-10 | 3.02E-11 | 7.39E-11 | 4.08E-11 | 3.11E-01 |
F24 | 2.00E-05 | 3.02E-11 | 4.50E-11 | 3.02E-11 | 4.62E-10 | 6.70E-11 | 1.02E-01 |
F25 | 3.69E-11 | 4.42E-06 | 1.19E-06 | 1.07E-07 | 6.53E-08 | 2.15E-06 | 1.38E-02 |
F26 | 5.87E-04 | 4.62E-10 | 1.22E-02 | 3.02E-11 | 9.51E-06 | 5.86E-06 | 9.59E-01 |
F27 | 5.97E-09 | 6.70E-11 | 3.69E-11 | 8.10E-10 | 5.07E-10 | 7.12E-09 | 1.49E-01 |
F28 | 6.07E-11 | 2.57E-07 | 7.62E-03 | 3.02E-11 | 1.60E-07 | 1.07E-09 | 1.33E-01 |
F29 | 9.26E-09 | 3.02E-11 | 6.07E-11 | 3.34E-11 | 2.87E-10 | 1.11E-04 | 2.34E-01 |
F30 | 3.02E-11 | 3.02E-11 | 7.06E-01 | 3.02E-11 | 3.02E-11 | 3.16E-05 | 2.13E-04 |
Function | GWO | AVAO | GTO | DE | COA | PSO | SBOA |
F1 | 3.02E-11 | 9.83E-08 | 2.51E-02 | 1.17E-09 | 3.02E-11 | 3.02E-11 | 9.82E-01 |
F3 | 3.02E-11 | 3.02E-11 | 2.81E-02 | 3.02E-11 | 3.02E-11 | 3.02E-11 | 3.34E-11 |
F4 | 3.02E-11 | 1.16E-07 | 2.23E-09 | 3.02E-11 | 3.02E-11 | 3.02E-11 | 1.03E-06 |
F5 | 3.79E-01 | 6.07E-11 | 4.20E-10 | 3.02E-11 | 3.02E-11 | 3.27E-02 | 1.77E-03 |
F6 | 5.09E-08 | 3.02E-11 | 3.02E-11 | 1.00E-03 | 3.02E-11 | 1.43E-05 | 9.07E-03 |
F7 | 4.92E-01 | 3.02E-11 | 3.69E-11 | 7.66E-05 | 3.34E-11 | 5.94E-02 | 6.41E-01 |
F8 | 6.52E-01 | 3.02E-11 | 3.69E-11 | 3.02E-11 | 3.02E-11 | 6.38E-03 | 1.78E-04 |
F9 | 1.56E-02 | 5.53E-08 | 4.11E-07 | 3.02E-11 | 3.02E-11 | 3.35E-08 | 3.33E-01 |
F10 | 1.17E-03 | 1.78E-04 | 1.86E-03 | 3.02E-11 | 3.02E-11 | 2.88E-06 | 8.77E-02 |
F11 | 3.02E-11 | 3.02E-11 | 1.61E-10 | 3.02E-11 | 3.02E-11 | 3.02E-11 | 3.34E-11 |
F12 | 3.02E-11 | 2.67E-09 | 8.20E-07 | 3.02E-11 | 3.02E-11 | 3.02E-11 | 7.60E-07 |
F13 | 3.02E-11 | 6.72E-10 | 2.83E-08 | 1.09E-10 | 6.07E-11 | 3.69E-11 | 1.00E-03 |
F14 | 3.02E-11 | 3.02E-11 | 4.50E-11 | 3.02E-11 | 3.02E-11 | 3.02E-11 | 3.02E-11 |
F15 | 3.02E-11 | 3.02E-11 | 1.17E-04 | 3.02E-11 | 3.02E-11 | 6.70E-11 | 4.64E-01 |
F16 | 1.25E-05 | 1.46E-10 | 1.07E-07 | 3.02E-11 | 1.55E-09 | 1.04E-04 | 4.64E-01 |
F17 | 2.05E-03 | 1.46E-10 | 1.69E-09 | 3.02E-11 | 1.46E-10 | 5.00E-09 | 1.44E-02 |
F18 | 3.02E-11 | 3.02E-11 | 1.56E-08 | 3.02E-11 | 3.02E-11 | 3.02E-11 | 6.07E-11 |
F19 | 3.02E-11 | 3.34E-11 | 1.00E-03 | 3.02E-11 | 3.02E-11 | 4.98E-11 | 6.73E-01 |
F20 | 3.18E-03 | 2.03E-09 | 2.38E-07 | 3.02E-11 | 2.37E-10 | 8.12E-04 | 7.28E-01 |
F21 | 2.68E-04 | 3.02E-11 | 3.02E-11 | 3.02E-11 | 3.02E-11 | 5.49E-11 | 7.73E-02 |
F22 | 2.61E-02 | 8.88E-06 | 7.12E-09 | 3.02E-11 | 3.02E-11 | 8.29E-06 | 1.81E-01 |
F23 | 3.02E-11 | 3.02E-11 | 3.02E-11 | 3.02E-11 | 3.02E-11 | 3.02E-11 | 3.99E-04 |
F24 | 6.12E-10 | 3.02E-11 | 3.02E-11 | 3.02E-11 | 3.02E-11 | 3.02E-11 | 5.79E-01 |
F25 | 3.02E-11 | 2.34E-01 | 2.57E-07 | 3.02E-11 | 3.02E-11 | 5.07E-10 | 7.01E-02 |
F26 | 3.55E-01 | 2.23E-09 | 4.20E-10 | 1.11E-06 | 3.69E-11 | 4.11E-07 | 3.79E-01 |
F27 | 6.07E-11 | 6.07E-11 | 4.50E-11 | 3.02E-11 | 4.08E-11 | 3.82E-10 | 2.51E-02 |
F28 | 3.02E-11 | 1.61E-06 | 2.19E-08 | 3.02E-11 | 3.02E-11 | 3.69E-11 | 8.35E-08 |
F29 | 6.12E-10 | 2.61E-10 | 1.46E-10 | 3.02E-11 | 4.50E-11 | 8.56E-04 | 7.39E-01 |
F30 | 3.02E-11 | 3.02E-11 | 3.02E-11 | 3.02E-11 | 3.02E-11 | 3.02E-11 | 1.17E-09 |
Function | GWO | AVAO | GTO | DE | COA | PSO | SBOA |
F1 | 1.45E-11 | 1.45E-11 | 5.17E-07 | 1.45E-11 | 1.45E-11 | 1.45E-11 | 1.45E-11 |
F2 | 1.69E-09 | 1.31E-01 | 1.68E-01 | 7.64E-05 | 1.25E-04 | 2.61E-04 | 3.10E-02 |
F3 | 1.26E-11 | 1.26E-11 | 1.26E-11 | 6.83E-03 | 1.91E-11 | 1.17E-03 | 5.43E-03 |
F4 | 3.00E-07 | 3.31E-11 | 4.55E-10 | 4.05E-11 | 4.40E-11 | 3.30E-03 | 5.54E-03 |
F5 | 3.35E-11 | 2.47E-11 | 4.68E-10 | 4.09E-11 | 5.00E-11 | 1.45E-01 | 2.74E-06 |
F6 | 3.02E-11 | 3.02E-11 | 3.02E-11 | 3.02E-11 | 3.02E-11 | 3.02E-11 | 3.02E-11 |
F7 | 2.87E-10 | 7.39E-11 | 9.92E-11 | 3.32E-06 | 2.03E-09 | 3.57E-06 | 3.50E-03 |
F8 | 4.98E-11 | 4.08E-11 | 1.61E-10 | 1.07E-09 | 1.96E-10 | 6.53E-08 | 2.16E-03 |
F9 | 1.21E-12 | 5.42E-09 | 3.34E-01 | 3.12E-04 | 1.21E-12 | 3.34E-01 | NAN |
F10 | 3.96E-08 | 2.67E-09 | 1.60E-07 | 8.48E-09 | 3.20E-09 | 3.96E-08 | 8.31E-03 |
F11 | 2.98E-09 | 2.95E-05 | 1.29E-01 | 3.90E-11 | 1.46E-07 | 4.87E-04 | 1.10E-05 |
F12 | 3.01E-11 | 8.94E-11 | 1.10E-09 | 2.15E-03 | 6.70E-10 | 4.46E-09 | 8.76E-02 |
Function | GWO | AVAO | GTO | DE | COA | PSO | SBOA |
F1 | 3.02E-11 | 3.02E-11 | 6.77E-05 | 3.02E-11 | 3.02E-11 | 3.02E-11 | 3.02E-11 |
F2 | 1.47E-07 | 7.30E-04 | 3.64E-02 | 1.95E-03 | 2.53E-04 | 1.76E-01 | 7.24E-02 |
F3 | 3.02E-11 | 3.02E-11 | 3.02E-11 | 1.11E-04 | 3.02E-11 | 3.20E-09 | 8.65E-01 |
F4 | 6.36E-05 | 4.50E-11 | 8.15E-11 | 3.02E-11 | 4.08E-11 | 1.86E-03 | 9.00E-01 |
F5 | 3.34E-11 | 3.02E-11 | 3.02E-11 | 3.02E-11 | 3.02E-11 | 1.70E-08 | 3.55E-01 |
F6 | 3.02E-11 | 3.02E-11 | 3.69E-11 | 3.02E-11 | 3.02E-11 | 3.02E-11 | 4.50E-11 |
F7 | 6.07E-11 | 3.02E-11 | 3.34E-11 | 3.02E-11 | 7.39E-11 | 2.49E-06 | 2.16E-03 |
F8 | 8.99E-11 | 6.70E-11 | 1.68E-04 | 3.02E-11 | 3.02E-11 | 1.29E-06 | 6.91E-04 |
F9 | 3.02E-11 | 3.02E-11 | 1.04E-04 | 3.02E-11 | 3.02E-11 | 3.00E-11 | 3.02E-11 |
F10 | 4.57E-09 | 1.69E-09 | 8.84E-07 | 1.19E-06 | 1.47E-07 | 8.20E-07 | 5.49E-01 |
F11 | 3.02E-11 | 7.12E-09 | 3.02E-11 | 3.02E-11 | 3.02E-11 | 3.02E-11 | 3.02E-11 |
F12 | 2.92E-09 | 1.09E-10 | 1.96E-10 | 1.87E-05 | 2.15E-10 | 1.55E-09 | 2.77E-01 |
Tables 14 and 15 illustrate that for most functions, the significance probability of the Wilcoxon rank sum test between CSBOA and other optimization algorithms is less than 0.05, indicating a statistically significant difference at the 5% significance level. However, in the 30-dimensional CEC2017 test set, there are 13 benchmark functions where the significance probability between CSBOA and SBOA is greater than 0.05, suggesting no significant difference between the two algorithms for these functions. Similarly, in the 100-dimensional test set, the same condition applies to 14 functions of SBOA, indicating no significant performance difference between CSBOA and SBOA for these functions. This is understandable, as CSBOA is an enhanced variant of SBOA. While retaining the core strengths of the original algorithm, three improvement strategies are incorporated, resulting in no significant differences in performance for some test functions.
Tables 16 and 17 present the Wilcoxon rank sum test results for CSBOA and the comparative algorithms on the 10-dimensional and 20-dimensional CEC2022 benchmark functions, respectively. As shown in Table 16, the differences between CSBOA and the majority of comparison algorithms are statistically significant across various test functions. In Table 17, the Wilcoxon rank sum test results reveal significant differences between CSBOA and most comparison algorithms, except for SBOA. However, for six functions among the CEC2022 test functions, the significance probability between CSBOA and SBOA exceeds 0.05, indicating that, at the 5% significance level, there is no significant difference between CSBOA and SBOA in these functions. In conclusion, CSBOA demonstrates significant performance improvements compared to other state-of-the-art algorithms. However, it shows only marginal differences when compared to SBOA. This can be attributed to the fact that CSBOA introduces only three enhancements—a logistic-tent chao mapping, an improved differential mutation operator, and a crossover strategy—while preserving the core strengths of the original SBOA algorithm, thereby accounting for the observed minor differences.
When delving into the performance of the CSBOA algorithm, we employ the non-parametric Friedman average rank test to rank the experimental results of CSBOA against other algorithms on the CEC2017 and CEC2022 benchmark test suites. This method is favored for its lack of reliance on assumptions about data distribution, making it particularly suitable for assessing the performance of optimization algorithms across a diverse set of test suites.
Through Tables 18 and 19, we present the Friedman test ranking results for each algorithm, with CSBOA securing the top position among all the compared algorithms. This outcome not only confirms the superior performance of CSBOA across the selected test suites but also highlights its efficiency and reliability in solving complex optimization problems. Moreover, the significant ranking advantage of CSBOA underscores its adaptability and robustness across test functions of varying dimensions and characteristics, enabling it to maintain stable optimization performance in a wide range of application scenarios.
Dimensions | 30 | 100 | |||
Algorithms | Mean rank | Overall rank | Mean rank | Overall rank | |
GWO | 5.43 | 5 | 5 | 5 | |
AVOA | 6.03 | 7 | 4.73 | 4 | |
GTO | 4.17 | 3 | 4.17 | 3 | |
DE | 6.17 | 8 | 6.67 | 8 | |
COA | 5.9 | 6 | 6.33 | 7 | |
PSO | 4.7 | 4 | 5.43 | 6 | |
SBOA | 2.23 | 2 | 2.23 | 2 | |
CSBOA | 1.37 | 1 | 1.43 | 1 |
Dimensions | 10 | 20 | |||
Algorithms | Mean rank | Overall rank | Mean rank | Overall rank | |
GWO | 6.25 | 8 | 5.91 | 6 | |
AVOA | 6.17 | 7 | 6.17 | 7 | |
GTO | 3.83 | 3 | 4.91 | 5 | |
DE | 4.33 | 4 | 4.17 | 3 | |
COA | 5.92 | 6 | 6.25 | 8 | |
PSO | 4.92 | 5 | 4.67 | 4 | |
SBOA | 2.79 | 2 | 2.25 | 2 | |
CSBOA | 1.79 | 1 | 1.67 | 1 |
Following the experimental investigations and subsequent analyses presented in Section 5, it has become clear that the CSBOA demonstrates superior performance in optimizing benchmark test functions compared to its counterparts. Yet, the ultimate measure of a metaheuristic algorithm's merit is its capacity to skillfully address and unravel the intricacies of real-world problems. Bearing this in mind, the subsequent section is devoted to validating the practical effectiveness and versatility of CSBOA by integrating it into scenarios that mirror real-world challenges. To thoroughly assess the algorithm's preparedness for practical application and its scalability, it has been meticulously deployed in two quintessential engineering problems and added the high-performance algorithm QOCSOS algorithm to the comparison[48], thereby illustrating its proficiency in handling complex, real-world scenarios with finesse.
The Pressure Vessel Design problem[60], illustrated in Figure 7, entails crafting a structure that optimizes cost efficiency without compromising on its functional requirements. The crux of this design endeavor revolves around four key optimization parameters: the vessel thickness (Ts), head thickness (Th), inner radius (R), and head length (L). These parameters are the linchpins of the design, directly affecting the performance and cost of pressure vessels. The equation that encapsulates the mathematical framework for this optimization undertaking is detailed in Eq (6.1). This equation is the cornerstone for navigating the complex trade-offs between material usage, structural integrity, and economic viability, ensuring that the vessel is not only robust but also cost-effective.
Consider→x=[x1x2x3x4]=[TSThRL],Minimizef(→x)=0.6224x1x3x4+1.7781x2x23+3.1661x21x4+19.84x21x3,Subjecttog1(→x)=−x1+0.0193x3≤0,g2(→x)=−x2+0.00954x3≤0,g3(→x)=−πx23x4−43πx33+1296000≤0,g4(→x)=x4−240≤0,Parmeterrange0≤x1,x2≤99,10≤x3,x4≤200. | (6.1) |
As seen from the results in Table 20, CSBOA outperforms its competitors in optimizing Pressure Vessel Design problems. The optimal value of the CSBOA algorithm proposed in this study is 5885.332773, and the theoretical optimal value is 5.8853E+03. Thus, the CSBOA algorithm is slightly better than QOCSOS. This not only showcases its excellent optimization capabilities but also reflects its efficiency in solving practical engineering problems and its stability and reliability when facing complex design challenges. Additionally, CSBOA has demonstrated remarkable control ability and a precise understanding of design objectives while adjusting optimization parameters in multiple dimensions, such as container thickness (Ts), head thickness (Th), inner diameter (R), and head length (L). The optimization of these parameters directly influences the functional characteristics and cost-effectiveness of pressure vessels. The successful application of CSBOA provides a new and efficient tool for engineering design.
Algorithm | Optimal values for variable | Optimal value | Ranking | |||
Ts | Th | R | L | |||
GWO | 0.785779 | 0.388407 | 40.702147 | 194.754644 | 5900.291027 | 4 |
AVOA | 0.989300 | 0.489012 | 51.259077 | 88.659593 | 6352.998707 | 8 |
GTO | 1.258847 | 0.622249 | 65.225232 | 10.000000 | 7319.000702 | 9 |
DE | 0.781083 | 0.386270 | 40.339766 | 200.000000 | 5914.483351 | 5 |
COA | 0.968743 | 0.480559 | 50.114317 | 97.637567 | 6319.415834 | 7 |
PSO | 0.973894 | 0.481396 | 50.460847 | 94.730452 | 6311.096260 | 5 |
QOCSOS | 0.778238 | 0.384893 | 40.322081 | 199.966711 | 5885.332774 | 2 |
SBOA | 0.779067 | 0.385093 | 40.3661670 | 199.352987 | 5886.870272 | 3 |
CSBOA | 0.778169 | 0.384649 | 40.319619 | 199.999999 | 5885.332773 | 1 |
This design problem[61,62,63] aims to minimize the weight of tension/compression springs by optimizing three critical parameters: Wire diameter (d), coil diameter (D), and the number of coils (N). The structure of this engineering problem is illustrated in Figure 8, with the mathematical model presented in Eq (6.2).
Consider→x=[x1x2x3]=[dDN],Minimizef(→x)=(x3+2)x2x21,Subjecttog1(→x)=1−x32x371785x41≤0,g2(→x)=4x22−x1x212566(x2x31−x41)+15108x21≤0,g3(→x)=1−140.45x1x22x3≤0,g4(→x)=x1+x21.5−1≤0,Parmeterrange0.05≤x1≤2,0.25≤x2≤1.3,2≤x3≤15. | (6.2) |
Table 21 offers a comprehensive comparison of the optimization results of CSBOA and eight distinct competitive algorithms in a tension/compression spring design. Evident from the data in the table is that CSBOA surpasses other algorithms, attaining a value of 0.012665235 with a theoretical optimal value of 1.2665E-02. This outcome showcases the advanced optimization capability of CSBOA, enabling it to proficiently navigate the complex search space of spring design problems and determine solutions that minimize the objective function. The exceptional performance of CSBOA is ascribed to its innovative strategy. These elements collaborate to enhance the exploration and exploitation capabilities of the algorithm, enabling it to avoid local optima more effectively than competing algorithms and converge to the global optimum. CSBOA's attainment of optimal values not only demonstrates its proficiency in handling tension/compression spring design problems but also underscores its potential for application in various complex engineering optimization problems where finding the most cost-effective and efficient design is crucial. The comparison presented in Table 21 solidifies the position of CSBOA as a leading optimization algorithm in mechanical design and other fields.
Algorithm | Optimal values for variable | Optimal value | Ranking | ||
d | D | N | |||
GWO | 0.0523516599 | 0.372775760 | 10.415319625 | 0.012684229 | 4 |
AVOA | 0.050000000 | 0.317425416 | 14.027769769 | 0.012719054 | 6 |
GTO | 0.053325095 | 0.397367912 | 9.250854836 | 0.012712811 | 5 |
DE | 0.053135792 | 0.392469906 | 9.485223289 | 0.012726826 | 7 |
COA | 0.056774591 | 0.491795419 | 6.277155317 | 0.013121202 | 9 |
PSO | 0.054998195 | 0.441675285 | 7.622863618 | 0.012855954 | 8 |
QOCSOS | 0.055130816 | 0.444775663 | 7.544716912 | 0.012665282 | 2 |
SBOA | 0.052219133 | 0.369604350 | 10.571609914 | 0.012670312 | 3 |
CSBOA | 0.051700822 | 0.3570007342 | 11.272393937 | 0.012665235 | 1 |
We introduce a crossover strategy integrated Secretary Bird Optimization Algorithm, dubbed CSBOA, aimed at addressing numerical optimization problems and practical engineering optimization challenges. CSBOA significantly enhances the performance of the original SBOA through three key strategic improvements. First, we employ a logistic-tent chaotic mapping strategy to initialize the population, replacing the conventional random initialization method. This results in a more uniform distribution of individuals within the defined interval, thereby enhancing the population's global search capability. Second, we reinforce the original DE/rand/1 mutation operator by introducing the DE/rand-rand/1 operator, which broadens the algorithm's search scope and increases the randomness of population exploration, effectively aiding the algorithm in escaping local optima. Last, to address the issues of slow convergence speed and low precision inherent in the original algorithm, we integrate a crossover strategy in CSBOA that not only accelerates the convergence rate but also enhances the precision of the final solution. The combined effect of these improvements makes CSBOA more effective and reliable in handling complex optimization problems.
To verify the effectiveness of CSBOA, a series of numerical experiments were conducted using 29 CEC2017 and 12 CEC2022 benchmark functions. The results show that, in comparison to advanced metaheuristic algorithms, CSBOA can offer improved solution accuracy, convergence speed, and stability in most test cases. Moreover, the performance of CSBOA in addressing engineering optimization problems is also quite competitive, which further underscores the practicality of CSBOA in real-world applications.
Although CSBOA has demonstrated outstanding optimization capabilities, there is room for improvement in its performance. Currently, the combination of various enhancement strategies has led to an increase in the computation time and insufficient stability of CSBOA, which has become the main challenge to overcome in its future development. To address this issue, there is a plan to introduce parallel computing technology or integrate it with advanced algorithms to deeply optimize the structure of CSBOA, expecting to effectively reduce computational costs without sacrificing convergence accuracy. In addition, to enhance the robustness of the algorithm, the integration of advanced operators such as dynamic population evolution and quantum rotation gates into CSBOA is being considered. The introduction of these operators will make CSBOA more flexible and powerful in handling a wider range of optimization problems. Given the excellent results achieved by CSBOA in preliminary tests, it is believed that it can be applied to practical optimization problems in various disciplines, such as predictive modeling, image segmentation, and feature selection. Moreover, the development of a multi-objective version of CSBOA is being explored to address more complex multi-objective optimization challenges, thereby further expanding its application scope and influence in the field of computational optimization.
The authors declare they have not used Artificial Intelligence (AI) tools in the creation of this article.
This research was funded by the Guangxi science and technology plan project (AD23023003) and the Fifth Cultivation Program for Thousands of Young and Middle-aged Backbone Teachers in Guangxi Higher Education Institutions.
The authors declare there is no conflict of interest.
[1] |
Q. Li, S. Y. Liu, X. S. Yang, Influence of initialization on the performance of metaheuristic optimizers, Appl. Soft Comput., 91 (2020), 106193. https://doi.org/10.1016/j.asoc.2020.106193 doi: 10.1016/j.asoc.2020.106193
![]() |
[2] |
H. Su, D. Zhao, A. A. Heidari, L. Liu, X. Zhang, M. Mafarja, et al., RIME: A physics-based optimization, Neurocomputing, 532 (2023), 183–214. https://doi.org/10.1016/j.neucom.2023.02.010 doi: 10.1016/j.neucom.2023.02.010
![]() |
[3] |
X. Yu, N. Jiang, X. Wang, M. Li, A hybrid algorithm based on Grey Wolf Optimizer and differential evolution for UAV path planning, Expert Syst. Appl., 215 (2023), 119327. https://doi.org/10.1016/j.eswa.2022.119327 doi: 10.1016/j.eswa.2022.119327
![]() |
[4] | J. H. Holland, Genetic algorithms, Sci. Am., 267 (1992) 66–73. http://www.jstor.org/stable/24939139 |
[5] |
R. Storn, K. Price, Differential evolution–a simple and efficient heuristic for global optimization over continuous spaces, J. Glob. Optim., 11 (1997), 341–359. https://doi.org/10.1023/A:1008202821328 doi: 10.1023/A:1008202821328
![]() |
[6] | E. Atashpaz-Gargari, C. Lucas, Imperialist competitive algorithm: An algorithm for optimization inspired by imperialistic competition, in 2007 IEEE Congress on Evolutionary Computation, (2007), 4661–4667. https://doi.org/10.1109/CEC.2007.4425083 |
[7] |
E. H. Houssein, D. Oliva, N. A. Samee, N. F. Mahmoud, M. M. Emam, Liver Cancer Algorithm: A novel bio-inspired optimizer, Comput. Biol. Med., 165 (2023), 107389. https://doi.org/10.1016/j.compbiomed.2023.107389 doi: 10.1016/j.compbiomed.2023.107389
![]() |
[8] |
A. Taheri, K. RahimiZadeh, A. Beheshti, J. Baumbach, R. V. Rao, S. Mirjalili, et al., Partial reinforcement optimizer: An Evolutionary Optimization Algorithm, Expert Syst. Appl., 238 (2024), 122070. https://doi.org/10.1016/j.eswa.2023.122070 doi: 10.1016/j.eswa.2023.122070
![]() |
[9] |
B. Zheng, Y. Chen, C. Wang, A. A. Heidari, L. Liu, H. Chen, The moss growth optimization (MGO): Concepts and performance, J. Comput. Des. Eng., 11 (2024), 184–221. https://doi.org/10.1093/jcde/qwae080 doi: 10.1093/jcde/qwae080
![]() |
[10] |
S. Kirkpatrick, C. D. Gelatt Jr, M. P. Vecchi, Optimization by simulated annealing, Science, 220 (1983), 671–680. https://doi.org/10.1126/science.220.4598.671 doi: 10.1126/science.220.4598.671
![]() |
[11] |
S. Mirjalili, S. M. Mirjalili, A. Hatamlou, Multi-verse optimizer: A nature-inspired algorithm for global optimization, Neural Comput. Appl., 27 (2016), 495–513. https://doi.org/10.1007/s00521-015-1870-7 doi: 10.1007/s00521-015-1870-7
![]() |
[12] |
A. Faramarzi, M. Heidarinejad, B. Stephens, S. Mirjalili, Equilibrium optimizer: A novel optimization algorithm, Knowl. Based Syst., 191 (2020), 105190. https://doi.org/10.1016/j.knosys.2019.105190 doi: 10.1016/j.knosys.2019.105190
![]() |
[13] |
T. Sang-To, M. Hoang-Le, M. A. Wahab, T. Cuong-Le, An efficient Planet Optimization Algorithm for solving engineering problems, Sci. Rep., 12 (2022), 8362. https://doi.org/10.1038/s41598-022-12030-w doi: 10.1038/s41598-022-12030-w
![]() |
[14] |
M. Abdel-Basset, R. Mohamed, S. A. Abdel Azeem, M. Jameel, M. Abouhawwash, Kepler optimization algorithm: A new metaheuristic algorithm inspired by Kepler's laws of planetary motion, Knowledge-Based Syst., 268 (2023), 110454. https://doi.org/10.1016/j.knosys.2023.110454 doi: 10.1016/j.knosys.2023.110454
![]() |
[15] |
L. Deng, S. Liu, Snow ablation optimizer: A novel metaheuristic technique for numerical optimization and engineering design, Expert Syst. Appl., 225 (2023), 120069. https://doi.org/10.1016/j.eswa.2023.120069 doi: 10.1016/j.eswa.2023.120069
![]() |
[16] |
R. Sowmya, M. Premkumar, P. Jangir, Newton-Raphson-based optimizer: A new population-based metaheuristic algorithm for continuous optimization problems, Eng. Appl. Artif. Intell., 128 (2024), 107532. https://doi.org/10.1016/j.engappai.2023.107532 doi: 10.1016/j.engappai.2023.107532
![]() |
[17] | J. Kennedy, R. Eberhart, Particle swarm optimization, in Proceedings of ICNN'95-international Conference on Neural Networks, (1995), 1942–1948. https://doi.org/10.1109/ICNN.1995.488968 |
[18] |
S. Mirjalili, S. M. Mirjalili, A. Lewis, Grey Wolf Optimizer, Adv. Eng. Softw., 69 (2014), 46–61. https://doi.org/10.1016/j.advengsoft.2013.12.007 doi: 10.1016/j.advengsoft.2013.12.007
![]() |
[19] |
L. Wang, Q. Cao, Z. Zhang, S. Mirjalili, W. Zhao, Artificial rabbits optimization: A new bio-inspired meta-heuristic algorithm for solving engineering optimization problems, Eng. Appl. Artif. Intell., 114 (2022), 105082. https://doi.org/10.1016/j.engappai.2022.105082 doi: 10.1016/j.engappai.2022.105082
![]() |
[20] |
F. A. Hashim, A. G. Hussien, Snake optimizer: A novel Meta-heuristic Optimization Algorithm, Knowledge Based Syst., 242 (2022), 108320. https://doi.org/10.1016/j.knosys.2022.10832 doi: 10.1016/j.knosys.2022.10832
![]() |
[21] |
H. Jia, H. Rao, C. Wen, S. Mirjalili, Crayfish Optimization Algorithm, Artif. Intell. Rev., 56 (2023), 1919–1979. https://doi.org/10.1007/s10462-023-10567-4 doi: 10.1007/s10462-023-10567-4
![]() |
[22] |
M. Abdel-Basset, R. Mohamed, M. Abouhawwash, Crested Porcupine Optimizer: A new nature-inspired metaheuristic, Knowledge Based Syst., 284 (2024), 111257. https://doi.org/10.1016/j.knosys.2023.111257 doi: 10.1016/j.knosys.2023.111257
![]() |
[23] |
M. H. Amiri, N. M. Hashjin, M. Montazeri, S. Mirjalili, N. Khodadadi, Hippopotamus Optimization Algorithm: A novel nature-inspired optimization algorithm, Sci. Rep., 14 (2024), 5032. https://doi.org/10.1038/s41598-024-54910-3 doi: 10.1038/s41598-024-54910-3
![]() |
[24] |
G. Dhiman, V. Kumar, Seagull Optimization Algorithm: Theory and its applications for large-scale industrial engineering problems, Knowledge Based Syst., 165 (2019), 169–196. https://doi.org/10.1016/j.knosys.2018.11.024 doi: 10.1016/j.knosys.2018.11.024
![]() |
[25] |
Y. Fu, D. Liu, J. Chen, L. He, Secretary Bird Optimization Algorithm: A new metaheuristic for solving global optimization problems, Artif. Intell. Rev., 57 (2024), 1–102. https://doi.org/10.1007/s10462-024-10729-y doi: 10.1007/s10462-024-10729-y
![]() |
[26] |
S. Abbasi, A. M. Rahmani, A. Balador, A. Sahafi, A fault-tolerant adaptive genetic algorithm for service scheduling in internet of vehicles, Appl. Soft Comput., 143 (2023), 110413. https://doi.org/10.1016/j.asoc.2023.110413 doi: 10.1016/j.asoc.2023.110413
![]() |
[27] |
B. Zhou, Z. Zhao, An adaptive artificial bee colony algorithm enhanced by deep Q-learning for milk-run vehicle scheduling problem based on supply hub, Knowledge Based Syst., 264 (2023), 110367. https://doi.org/10.1016/j.knosys.2023.110367 doi: 10.1016/j.knosys.2023.110367
![]() |
[28] |
Z. Wang, L. Shao, S. Yang, J. Wang, D. Li, CRLM: A cooperative model based on reinforcement learning and metaheuristic algorithms of routing protocols in wireless sensor networks, Comput. Netw., 236 (2023), 110019. https://doi.org/10.1016/j.comnet.2023.110019 doi: 10.1016/j.comnet.2023.110019
![]() |
[29] |
S. Deng, Y. Li, J. Wang, R. Cao, M. Li, A feature-thresholds guided genetic algorithm based on a multi-objective feature scoring method for high-dimensional feature selection, Appl. Soft Comput., 148 (2023), 110765. https://doi.org/10.1016/j.asoc.2023.110765 doi: 10.1016/j.asoc.2023.110765
![]() |
[30] |
C. Zhong, G. Li, Z. Meng, H. Li, W. He, A self-adaptive quantum equilibrium optimizer with artificial bee colony for feature selection, Comput. Biol. Med., 153 (2023), 106520. https://doi.org/10.1016/j.compbiomed.2022.106520 doi: 10.1016/j.compbiomed.2022.106520
![]() |
[31] |
Y. Jia, L. Qu, X. Li, Automatic path planning of unmanned combat aerial vehicle based on double-layer coding method with enhanced Grey Wolf Optimizer, Artif. Intell. Rev., 56 (2023), 12257–12314. https://doi.org/10.1007/s10462-023-10481-9 doi: 10.1007/s10462-023-10481-9
![]() |
[32] |
M. H. Nadimi-Shahraki, E. Moeini, S. Taghian, S. Mirjalili, Discrete improved Grey Wolf Optimizer for community detection, J. Bionic Eng., 20 (2023), 2331–2358. https://doi.org/10.1007/s42235-023-00387-1 doi: 10.1007/s42235-023-00387-1
![]() |
[33] |
D. H. Wolpert, W. G. Macready, No free lunch theorems for optimization, IEEE Trans. Evol. Comput., 1 (1997), 67–82. https://doi.org/10.1109/4235.585893 doi: 10.1109/4235.585893
![]() |
[34] |
R. Zheng, H. Jia, L. Abualigah, S. Wang, D. Wu, An improved Remora Optimization Algorithm with autonomous foraging mechanism for global optimization problems, Math. Biosci. Eng., 19 (2022), 3994–4037. https://doi.org/10.3934/mbe.2022184 doi: 10.3934/mbe.2022184
![]() |
[35] |
R. Zheng, A. G. Hussien, R. Qaddoura, H. Jia, L. Abualigah, S. Wang, et al., A multi-strategy enhanced African vultures optimization algorithm for global optimization problems, J. Comput. Des. Eng., 10 (2023), 329–356. https://doi.org/10.1093/jcde/qwac135 doi: 10.1093/jcde/qwac135
![]() |
[36] |
C. Yuan, D. Zhao, A. Heidari, L. Liu, Y. Chen, H. Chen, Polar lights optimizer: Algorithm and applications in image segmentation and feature selection, Neurocomputing, 607 (2024), 128427. https://doi.org/10.1016/j.neucom.2024.128427 doi: 10.1016/j.neucom.2024.128427
![]() |
[37] |
D. Truong, J. Chou, Metaheuristic algorithm inspired by enterprise development for global optimization and structural engineering problems with frequency constraints, Eng. Struct., 318 (2024), 118679. https://doi.org/10.1016/j.engstruct.2024.118679 doi: 10.1016/j.engstruct.2024.118679
![]() |
[38] |
J. Ji, T. Wu, C. Yang, Neural population dynamics optimization algorithm: A novel brain-inspired meta-heuristic method, Knowledge Based Syst., 300 (2024), 112194. https://doi.org/10.1016/j.knosys.2024.112194 doi: 10.1016/j.knosys.2024.112194
![]() |
[39] |
H. Jia, X. Peng, C. Lang, Remora Optimization Algorithm, Expert Syst. Appl., 185 (2021), 115665. https://doi.org/10.1016/j.eswa.2021.115665 doi: 10.1016/j.eswa.2021.115665
![]() |
[40] |
H. Jia, Y. Li, D. Wu, H. Rao, C. Wen, L. Abualigah, Multi-strategy Remora Optimization Algorithm for solving multi-extremum problems, J. Comput. Des. Eng., 10 (2023), 1315–1349. https://doi.org/10.1093/jcde/qwad044 doi: 10.1093/jcde/qwad044
![]() |
[41] |
F. A. Hashim, R. R. Mostafa, R. Abu Khurma, R. Qaddoura, P. A. Castillo, A new approach for solving global optimization and engineering problems based on modified sea horse optimizer, J. Comput. Des. Eng., 11 (2024), 73–98. https://doi.org/10.1093/jcde/qwae001 doi: 10.1093/jcde/qwae001
![]() |
[42] |
S. Qin, J. Liu, X. Bai, G. Hu, A multi-strategy improvement Secretary Bird Optimization Algorithm for engineering optimization problems, Biomimetics, 9 (2024), 478, https://doi.org/10.3390/biomimetics9080478 doi: 10.3390/biomimetics9080478
![]() |
[43] | H. Qin, S. Yang, Z. Liu, G. Li, An improved Secretary Bird Optimization Algorithm, in Proceedings of the 2024 5th International Conference on Information Science, Parallel and Distributed Systems (ISPDS), (2024), 442–445. https://doi.org/10.1109/ISPDS62779.2024.10667529 |
[44] |
S. Zheng, J. Huo, J. Yang, F. Cao, An energy-efficient multi-hop routing protocol for 3D bridge wireless sensor network based on secretary bird optimization algorithm, IEEE Sens. J., (2024). https://doi.org/10.1109/JSEN.2024.3464513 doi: 10.1109/JSEN.2024.3464513
![]() |
[45] |
Z. Pan, D. Lei, L. Wang, A knowledge-based two-population optimization algorithm for distributed energy-efficient parallel machines scheduling, IEEE Trans. Cybern., 52 (2022), 5051–5063. https://doi.org/10.1109/TCYB.2020.3026571 doi: 10.1109/TCYB.2020.3026571
![]() |
[46] |
F. Zhao, S. Di, L. Wang, A hyperheuristic with Q-learning for the multiobjective energy-efficient distributed blocking flow shop scheduling problem, IEEE Trans. Cybern., 53 (2023), 3337–3350. https://doi.org/10.1109/TCYB.2022.3192112 doi: 10.1109/TCYB.2022.3192112
![]() |
[47] |
F. Zhao, C. Zhuang, L. Wang, C. Dong, An iterative greedy algorithm with q-learning mechanism for the multiobjective distributed no-idle permutation flowshop scheduling, IEEE Trans. Syst., Man, Cybern. Syst., 54 (2024), 3207–3219. https://doi.org/10.1109/TSMC.2024.3358383 doi: 10.1109/TSMC.2024.3358383
![]() |
[48] |
K. H. Truong, P. Nallagownden, Z. Baharudin, D. N. Vo, A quasi-oppositional-chaotic symbiotic organisms search algorithm for global optimization problems, Appl. Soft Comput., 77 (2019), 567–583. https://doi.org/10.1016/j.asoc.2019.01.043 doi: 10.1016/j.asoc.2019.01.043
![]() |
[49] |
Y. Chen, D. Pi, S. Yang, Y. Xu, B. Wang, Y. Wang, A multi-strategy optimizer for energy minimization of multi-UAV-assisted mobile edge computing, Swarm Evol. Comput., 91 (2024), 101748. https://doi.org/10.1016/j.swevo.2024.101748 doi: 10.1016/j.swevo.2024.101748
![]() |
[50] |
L. Lan, S. Wang, Improved African vultures optimization algorithm for medical image segmentation, Multimedia Tools Appl., 83 (2024), 45241–45290. https://doi.org/10.1007/s11042-023-17189-6 doi: 10.1007/s11042-023-17189-6
![]() |
[51] |
J. Liu, Y. Deng, Y. Liu, L. Chen, Z. Hu, P. Wei, et al., A logistic-tent chaotic mapping Levenberg Marquardt Algorithm for improving positioning accuracy of grinding robot, Sci. Rep., 14 (2024). https://doi.org/10.1038/s41598-024-60402-1 doi: 10.1038/s41598-024-60402-1
![]() |
[52] |
A. B. Meng, Y. C. Chen, H. Yin, S. Z. Chen, Crisscross Optimization Algorithm and its application, Knowledge-Based Syst., 67 (2014), 218–229. https://doi.org/10.1016/j.knosys.2014.05.004 doi: 10.1016/j.knosys.2014.05.004
![]() |
[53] |
B. Abdollahzadeh, F. S. Gharehchopogh, S. Mirjalili, African vultures optimization algorithm: A new nature-inspired metaheuristic algorithm for global optimization problems, Comput. Ind. Eng., 158 (2021), 107408. https://doi.org/10.1016/j.cie.2021.107408 doi: 10.1016/j.cie.2021.107408
![]() |
[54] |
B. Abdollahzadeh, F. S. Gharehchopogh, S. Mirjalili, Artificial gorilla troops optimizer: A new nature-inspired metaheuristic algorithm for global optimization problems, Int. J. Intell. Syst., 36 (2021), 5887–5958. https://doi.org/10.1002/int.22535 doi: 10.1002/int.22535
![]() |
[55] |
K. Hussain, M. N. M. Salleh, S. Cheng, Y. Shi, On the exploration and exploitation in popular swarm-based metaheuristic algorithms, Neural Comput. Appl., 31 (2019), 7665–7683. https://doi.org/10.1007/s00521-018-3592-0 doi: 10.1007/s00521-018-3592-0
![]() |
[56] |
B. Morales-Castañeda, D. Zaldivar, E. Cuevas, F. Fausto, A. Rodríguez, A better balance in metaheuristic algorithms: Does it exist, Swarm Evol. Comput., 54 (2020), 100671. https://doi.org/10.1016/j.swevo.2020.100671 doi: 10.1016/j.swevo.2020.100671
![]() |
[57] | G. Wu, R. Mallipeddi, P. N. Suganthan, Problem definitions and evaluation criteria for the CEC 2017 competition on constrained real-parameter optimization, 2017. |
[58] | A. Kumar, K. V. Price, A. W. Mohamed, A. A. Hadi, Problem definitions and evaluation criteria for the CEC 2022 special session and competition on single objective bound constrained numerical optimization, 2022. |
[59] |
P. B. Dao, On Wilcoxon rank sum test for condition monitoring and fault detection of wind turbines, Appl. Energy, 318 (2022), 119209. https://doi.org/10.1016/j.apenergy.2022.119209 doi: 10.1016/j.apenergy.2022.119209
![]() |
[60] | E. Sandgren, NIDP in mechanical design optimization, J. Mech. Design, 112 (1990), 223–229. |
[61] |
J. S. Arora, Optimum design problem formulation, Introduction to Optimum Design, 2004 (2004), 15–54. https://doi.org/10.1016/B978-012064155-0/50002-1 doi: 10.1016/B978-012064155-0/50002-1
![]() |
[62] |
A. D. Belegundu, J. S. Arora, A study of mathematical programming methods for structural optimization. Part I: Theory, Int. J. Numer. Meth. Eng., 21 (1985), 1583–1599. https://doi.org/10.1002/nme.1620210904 doi: 10.1002/nme.1620210904
![]() |
[63] |
C. A. Coello, E. M. Montes, Constraint-handling in genetic algorithms through the use of dominance-based tournament selection, Adv. Eng. Inform., 16 (2002), 193–203. https://doi.org/10.1016/S1474-0346(02)00011-3 doi: 10.1016/S1474-0346(02)00011-3
![]() |
Category | Algorithm | year | Inspiration/Description |
Evolutionary | Liver Cancer Algorithm (LCA) [7] | 2023 | Inspired by the ability of tumors to replicate and spread to other organs, an MA is introduced. |
Partial Reinforcement Optimizer (PRO)[8] | 2024 | Inspired by the partial reinforcement effect (PRE) theory, an evolutionary learning/training theory in psychology, a learner is intermittently reinforced to learn or strengthen a specific behavior based on a schedule. | |
Moss Growth Optimization (MGO)[9] | 2024 | Inspired by the growth of moss in the natural environment, an MA is introduced. | |
Physic-based | Planet Optimization Algorithm (POA) [13] | 2022 | Inspired by the laws of cosmic motion and starting from the interaction of the gravitational forces among planets, an MA is introduced. |
Kepler Optimization Algorithm (KOA) [14] | 2023 | Inspired by Kepler's laws of planetary motion, which can predict the position and velocity of the planets at any given time, an MA is introduced. | |
Rime Optimization Algorithm (RIME)[2] | 2023 | Inspired by the natural frost and ice growth mechanism, algorithms are developed by simulating the motion of soft frost particles for algorithmic search, and by simulating the crossover behavior between hard frost agents. | |
Snow ablation optimizer (SAO) [15] | 2023 | Inspired by the simulation of the sublimation and melting behavior of snow, an MA is introduced. | |
Newton-Raphson-based optimizer (NBRO) [16] | 2024 | The algorithm searches the solution space by defining the solution space using the set of vectors and employing operators such as Natural Resource Strategy (NRSR) as well as Trap Avoidance Operator (TAO). | |
Swarm intelligence | Crested Porcupine Optimizer (CPO)[22] | 2024 | Simulation of four different protective mechanisms in crown porcupines: sight, hearing, odor and physical attack. |
Hippopotamus optimization algorithm (HO) [23] | 2024 | Inspired by the behavioral patterns of hippos in the natural environment, an MA is introduced. | |
Seagull optimization algorithm (SOA)[24] | 2024 | Inspired by the migration and predatory behaviors of seagulls in the natural world, an MA is introduced. | |
enhanced African Vultures Optimization Algorithm (EAVOA) [35] | 2023 | They incorporated a rotating flight mechanism, a representative vulture selection mechanism, and a selection accumulation operator strategy into the original AVOA. It demonstrated superior optimization capabilities compared to the original AVOA. | |
an improved Seahorse Optimizer (mSHO) [41] | 2024 | This algorithm integrates three search operators: neighborhood-based local search, global search, and walking within the existing search to achieve a more robust development trend. | |
multi-strategy improvement Secretary Bird Optimization Algorithm (MISBOA) [42] | 2024 | The algorithm improves the Secretary Bird Optimization Algorithm by combining three strategies: feedback regulation mechanism, golden sinusoidal guidance, and population diversity, and the results show that MISBOA has demonstrated good performance. | |
Improved Secretary Bird Optimization Algorithm [43] | 2024 | ISBOA adds the Circle Chaotic Mapping, the differential evolution strategy, and the population diversity strategy into the SBOA, which achieves the optimal convergence speed and optimization accuracy. optimal convergence speed and optimization accuracy. | |
Crossover strategy-based Secretary Bird Optimization Algorithm (CSBOA) | 2024 | Under the SBOA algorithm's framework, CSBOA innovatively adds the initialization strategy of logical chaos mapping, improved differential operator strategy, and crossover strategy, which greatly enhances the algorithm's efficiency. |
Function | GWO | AVAO | GTO | DE | COA | PSO | SBOA | CSBOA |
F1 | 0.2163120 | 0.3335453 | 0.6284790 | 1.1332032 | 0.1795724 | 0.1761100 | 0.3141203 | 1.5787525 |
F2 | 0.2100052 | 0.3018989 | 0.6607184 | 1.1743020 | 0.1837386 | 0.1733390 | 0.3190766 | 1.7292872 |
F3 | 0.2985672 | 0.3746466 | 0.8275277 | 1.3605336 | 0.3303046 | 0.2590517 | 0.5342557 | 2.1225204 |
F4 | 0.2221101 | 0.3152409 | 0.7127616 | 1.1873779 | 0.2305653 | 0.1963042 | 0.3707470 | 1.8089242 |
F5 | 0.2342974 | 0.3237152 | 0.6934132 | 1.2154550 | 0.2350611 | 0.2044259 | 0.4110868 | 1.7652453 |
F6 | 0.2150608 | 0.3068646 | 0.6739646 | 1.1734847 | 0.1979094 | 0.1757798 | 0.3328941 | 1.7567508 |
F7 | 0.3356384 | 0.4250690 | 0.9167834 | 1.3618838 | 0.4035385 | 0.2982822 | 0.5812446 | 2.3796544 |
F8 | 0.3715650 | 0.4781056 | 1.0045935 | 1.4647472 | 0.4844369 | 0.3593346 | 0.6875874 | 2.5092880 |
F9 | 0.3040718 | 0.3946582 | 0.8335377 | 1.3782160 | 0.3375005 | 0.2658512 | 0.5529398 | 2.1785161 |
F10 | 0.2883326 | 0.3836225 | 0.8240736 | 1.3242494 | 0.3325307 | 0.2494263 | 0.5050225 | 2.0998797 |
F11 | 0.3608691 | 0.4689512 | 0.9485607 | 1.4617516 | 0.4222041 | 0.3393067 | 0.6414698 | 2.3714879 |
F12 | 0.3713333 | 0.4656035 | 0.9925775 | 1.4338215 | 0.4992448 | 0.3349514 | 0.6788769 | 2.6054365 |
Algorithms | Reference | Name of the parameter | Value of the parameter |
GWO | [18] | a | [0, 2] |
AVAO | [53] | L1, L2, w, p1, p2, p3 | 0.8, 0.2, 2.5, 0.6, 0.4, 0.6 |
GTO | [54] | P, r1, r2, r3 | P, r1, r2, r3 ∈ [0, 1] |
DE | [5] | F, CR | 0.8, 0.1 |
COA | [21] | temp | [20, 35] |
PSO | [17] | w, c1, c2 | [0.9, 0.4], 0.2, 0.2 |
SBOA | [25] | R1, RB, r, R2 | [0, 1], [0, 1], [0, 1], [0, 1] |
Strategy | CSBOA-1 | CSBOA-2 | CSBOA-3 | CSBOA-4 | CSBOA-5 | CSBOA-6 | CSBOA |
Logistic-tent chaotic mapping | 1 | 0 | 0 | 1 | 1 | 0 | 1 |
Improved differential mutation operator | 0 | 1 | 0 | 1 | 0 | 1 | 1 |
Crossover strategy | 0 | 0 | 1 | 0 | 1 | 1 | 1 |
Function | Metric | SBOA | CSBOA-1 | CSBOA-2 | CSBOA-3 | CSBOA-4 | CSBOA-5 | CSBOA-6 | CSBOA |
F1 | Ave | 468.0984 | 462.0113 | 360.6446 | 354.0001 | 387.1188 | 342.2765 | 300.0422 | 300.0315 |
Std | 152.9298 | 168.8314 | 58.5821 | 45.8167 | 73.5351 | 41.1544 | 0.1029 | 0.0485 | |
Rank | 8 | 7 | 5 | 4 | 6 | 3 | 2 | 1 | |
F2 | Ave | 454.3443 | 453.9698 | 450.5164 | 454.2942 | 448.7348 | 449.7043 | 448.4292 | 445.9545 |
Std | 11.3625 | 12.6751 | 8.4090 | 14.9607 | 17.7389 | 14.2097 | 14.6531 | 14.5031 | |
Rank | 8 | 6 | 5 | 7 | 3 | 4 | 2 | 1 | |
F3 | Ave | 600.0028 | 600.0102 | 600.0734 | 600.0090 | 600.0460 | 600.0024 | 600.0135 | 600.0185 |
Std | 0.0043 | 0.0473 | 0.2534 | 0.0389 | 0.1770 | 0.0036 | 0.0534 | 0.0816 | |
Rank | 2 | 4 | 8 | 3 | 7 | 1 | 5 | 6 | |
F4 | Ave | 832.8866 | 831.9954 | 835.5805 | 834.0843 | 833.6276 | 836.8336 | 833.9302 | 834.4919 |
Std | 9.9938 | 9.1814 | 14.3468 | 9.9029 | 14.4475 | 9.6940 | 11.8680 | 9.1798 | |
Rank | 2 | 1 | 7 | 5 | 3 | 8 | 4 | 6 | |
F5 | Ave | 902.4884 | 901.9341 | 901.9577 | 900.8848 | 902.2329 | 900.8906 | 901.1584 | 900.7389 |
Std | 2.7654 | 2.6170 | 3.2391 | 1.2353 | 2.0574 | 1.3218 | 1.7129 | 1.0435 | |
Rank | 8 | 5 | 6 | 2 | 7 | 3 | 4 | 1 | |
F6 | Ave | 7828.2811 | 6654.5031 | 6912.6022 | 7017.1025 | 6639.8939 | 7405.0108 | 1864.9143 | 1858.5483 |
Std | 6388.4941 | 5805.7743 | 5662.3248 | 5100.5059 | 4777.2112 | 6057.2718 | 23.3027 | 24.1598 | |
Rank | 8 | 4 | 5 | 6 | 3 | 7 | 2 | 1 | |
F7 | Ave | 2032.9970 | 2033.8804 | 2028.0807 | 2029.6145 | 2027.3489 | 2029.7563 | 2025.9835 | 2024.3204 |
Std | 9.8793 | 10.5050 | 7.0884 | 6.1269 | 4.6764 | 7.4310 | 5.7183 | 5.2719 | |
Rank | 7 | 8 | 4 | 5 | 3 | 6 | 2 | 1 | |
F8 | Ave | 2224.0843 | 2224.0779 | 2224.1301 | 2222.0094 | 2224.1719 | 2222.4307 | 2221.0820 | 2221.7982 |
Std | 1.9078 | 2.0098 | 1.9208 | 0.8741 | 2.0107 | 0.9763 | 2.4445 | 0.7588 | |
Rank | 6 | 5 | 7 | 3 | 8 | 4 | 1 | 2 | |
F9 | Ave | 2480.7814 | 2480.7813 | 2480.7813 | 2480.7813 | 2480.7813 | 2480.7813 | 2480.7813 | 2480.7813 |
Std | 0.0002 | 5.61E-05 | 8.45E-06 | 2.13E-07 | 1.67E-05 | 2.84E-07 | 4.19E-08 | 3.38E-08 | |
Rank | 8 | 7 | 6 | 4 | 5 | 3 | 2 | 1 | |
F10 | Ave | 2539.0264 | 2536.6607 | 2559.7474 | 2526.3202 | 2553.5768 | 2526.9783 | 2504.4556 | 2509.1785 |
Std | 60.6819 | 57.9123 | 166.4333 | 53.3955 | 174.2956 | 53.9692 | 39.6923 | 33.1152 | |
Rank | 6 | 5 | 8 | 3 | 7 | 4 | 1 | 2 | |
F11 | Ave | 2908.6735 | 2903.3341 | 2903.3341 | 2903.3333 | 2900.0008 | 2906.6667 | 2900.0000 | 2900.0000 |
Std | 69.4118 | 18.2574 | 18.2575 | 18.2574 | 0.0005 | 25.3708 | 6.60E-07 | 5.18E-07 | |
Rank | 8 | 5 | 6 | 4 | 3 | 7 | 2 | 1 | |
F12 | Ave | 2942.3513 | 2941.4095 | 2939.2758 | 2939.0545 | 2940.0607 | 2941.6061 | 2940.6537 | 2942.5940 |
Std | 6.2628 | 5.3666 | 3.9849 | 3.8635 | 4.5492 | 6.7650 | 4.8318 | 5.2684 | |
Rank | 7 | 5 | 2 | 1 | 3 | 6 | 4 | 8 |
Type | ID | CEC2017 function name | Dimension | fmin |
Unimodal | F1 | Shifted and rotated bent cigar function | 30/100 | 100 |
F2 | Shifted and rotated sum of different power function* | 30/100 | 200 | |
F3 | Shifted and rotated Zakharow function | 30/100 | 300 | |
Multimodal | F4 | Shifted and rotated Rosenbrock's function | 30/100 | 400 |
F5 | Shifted and rotated Rastrigin's Function | 30/100 | 500 | |
F6 | Shifted and rotated expanded Scaffer's F6 Function | 30/100 | 600 | |
F7 | Shifted and rotated Lunacek Bi-Rastrigin finc-tion | 30/100 | 700 | |
F8 | Shifted and rotated non-continuous Rastrigin's function | 30/100 | 800 | |
F9 | Shifted and rotated levy function | 30/100 | 900 | |
F10 | Shifted and rotated Schwefel's function | 30/100 | 1000 | |
Hybrid | F11 | Hybrid function 1 (N = 3) | 30/100 | 1100 |
F12 | Hybrid function 2 (N = 3) | 30/100 | 1200 | |
F13 | Hybrid function 3 (N = 3) | 30/100 | 1300 | |
F14 | Hybrid function 4 (N = 4) | 30/100 | 1400 | |
F15 | Hybrid function 5 (N = 4) | 30/100 | 1500 | |
F16 | Hybrid function 6 (N = 4) | 30/100 | 1600 | |
F17 | Hybrid function 7 (N = 5) | 30/100 | 1700 | |
F18 | Hybrid function 8 (N = 5) | 30/100 | 1800 | |
F19 | Hybrid function 9 (N = 5) | 30/100 | 1900 | |
F20 | Hybrid function 10 (N = 6) | 30/100 | 2000 | |
Composition | F21 | Composition function 1 (N = 3) | 30/100 | 2100 |
F22 | Composition function 2 (N = 3) | 30/100 | 2200 | |
F23 | Composition function 3 (N = 4) | 30/100 | 2300 | |
F24 | Composition function 4 (N = 4) | 30/100 | 2400 | |
F25 | Composition function 5 (N = 5) | 30/100 | 2500 | |
F26 | Composition function 6 (N = 5) | 30/100 | 2600 | |
F27 | Composition function 7 (N = 6) | 30/100 | 2700 | |
F28 | Composition function 8 (N = 6) | 30/100 | 2800 | |
F29 | Composition function 9 (N = 3) | 30/100 | 2900 | |
F30 | Composition function 10 (N = 3) | 30/100 | 3000 | |
Search Range [−100,100]D | ||||
F2 has been officially removed due to its unstable behavior on high-dimensional issues. |
Function | GWO | AVAO | GTO | DE | COA | PSO | SBOA | CSBOA | |
F1 | Ave | 1.18E+09 | 3.98E+03 | 4.73E+03 | 1.87E+06 | 5.17E+06 | 9.01E+08 | 6.67E+03 | 2.26E+03 |
Std | 9.70E+08 | 4.64E+03 | 6.14E+03 | 5.62E+05 | 9.56E+06 | 1.20E+09 | 6.76E+03 | 3.48E+03 | |
F3 | Ave | 4.26E+04 | 2.28E+04 | 1.92E+03 | 1.48E+05 | 8.38E+04 | 2.51E+04 | 5.12E+03 | 9.70E+02 |
Std | 9.88E+03 | 4.91E+03 | 1.70E+03 | 1.75E+04 | 1.88E+04 | 1.14E+04 | 2.70E+03 | 4.02E+02 | |
F4 | Ave | 5.71E+02 | 5.05E+02 | 4.96E+02 | 5.12E+02 | 5.29E+02 | 5.60E+02 | 5.00E+02 | 4.88E+02 |
Std | 4.53E+01 | 3.26E+01 | 2.94E+01 | 8.35E+00 | 2.78E+01 | 9.74E+01 | 3.35E+01 | 2.04E+01 | |
F5 | Ave | 5.96E+02 | 7.01E+02 | 6.89E+02 | 6.83E+02 | 7.18E+02 | 5.79E+02 | 5.68E+02 | 5.69E+02 |
Std | 3.54E+01 | 3.52E+01 | 4.64E+01 | 1.17E+01 | 6.03E+01 | 2.43E+01 | 1.55E+01 | 1.91E+01 | |
F6 | Ave | 6.06E+02 | 6.46E+02 | 6.36E+02 | 6.00E+02 | 6.40E+02 | 6.03E+02 | 6.00E+02 | 6.00E+02 |
Std | 2.13E+00 | 1.01E+01 | 9.25E+00 | 2.21E-02 | 1.46E+01 | 2.52E+00 | 4.12E-01 | 2.76E-01 | |
F7 | Ave | 8.65E+02 | 1.11E+03 | 1.02E+03 | 9.17E+02 | 1.12E+03 | 8.15E+02 | 8.11E+02 | 8.22E+02 |
Std | 4.65E+01 | 5.90E+01 | 6.59E+01 | 1.03E+01 | 1.55E+02 | 3.03E+01 | 2.84E+01 | 2.84E+01 | |
F8 | Ave | 8.93E+02 | 9.56E+02 | 9.43E+02 | 9.85E+02 | 9.67E+02 | 8.71E+02 | 8.59E+02 | 8.72E+02 |
Std | 4.51E+01 | 3.09E+01 | 2.73E+01 | 9.75E+00 | 3.34E+01 | 1.99E+01 | 1.54E+01 | 1.56E+01 | |
F9 | Ave | 1.42E+03 | 4.71E+03 | 3.59E+03 | 1.91E+03 | 6.17E+03 | 1.02E+03 | 9.62E+02 | 9.39E+02 |
Std | 3.24E+02 | 7.28E+02 | 9.82E+02 | 2.11E+02 | 2.17E+03 | 1.14E+02 | 8.84E+01 | 4.90E+01 | |
F10 | Ave | 4.48E+03 | 5.38E+03 | 5.30E+03 | 7.31E+03 | 5.65E+03 | 4.54E+03 | 3.99E+03 | 3.70E+03 |
Std | 1.17E+03 | 6.76E+02 | 9.07E+02 | 3.41E+02 | 6.68E+02 | 7.32E+02 | 7.51E+02 | 4.41E+02 | |
(W|T|L) | Ave | (9|0|0) | (9|0|0) | (9|0|0) | (9|0|0) | (9|0|0)) | (9|0|0) | (6|0|3) | |
Std | (9|0|0) | (9|0|0) | (9|0|0) | (3|0|6) | (9|0|0) | (9|0|0) | (7|0|2) |
Function | GWO | AVAO | GTO | DE | COA | PSO | SBOA | CSBOA | |
F11 | Ave | 1.75E+03 | 1.26E+03 | 1.23E+03 | 1.59E+03 | 1.36E+03 | 1.27E+03 | 1.18E+03 | 1.15E+03 |
Std | 7.82E+02 | 5.77E+01 | 4.11E+01 | 2.01E+02 | 7.20E+01 | 7.05E+01 | 3.30E+01 | 2.68E+01 | |
F12 | Ave | 4.62E+07 | 3.60E+06 | 3.59E+05 | 3.96E+07 | 4.71E+06 | 2.68E+07 | 7.42E+05 | 1.32E+05 |
Std | 6.14E+07 | 3.10E+06 | 3.49E+05 | 1.08E+07 | 4.78E+06 | 5.92E+07 | 8.17E+05 | 1.35E+05 | |
F13 | Ave | 5.14E+06 | 7.58E+04 | 1.54E+04 | 3.29E+06 | 1.07E+05 | 5.27E+06 | 2.56E+04 | 1.94E+03 |
Std | 2.31E+07 | 3.94E+04 | 1.46E+04 | 1.58E+06 | 9.63E+04 | 1.81E+07 | 2.26E+04 | 3.34E+02 | |
F14 | Ave | 1.43E+05 | 1.34E+05 | 2.00E+03 | 2.06E+05 | 1.84E+05 | 3.20E+04 | 1.81E+04 | 1.45E+03 |
Std | 2.09E+05 | 1.24E+05 | 1.00E+03 | 1.17E+05 | 3.55E+05 | 3.88E+04 | 1.75E+04 | 8.55E+00 | |
F15 | Ave | 4.67E+05 | 2.42E+04 | 8.67E+03 | 5.30E+05 | 1.72E+04 | 2.32E+04 | 1.15E+04 | 1.60E+03 |
Std | 1.12E+06 | 1.67E+04 | 7.31E+03 | 2.81E+05 | 1.52E+04 | 2.21E+04 | 1.17E+04 | 3.92E+01 | |
F16 | Ave | 2.46E+03 | 2.89E+03 | 2.76E+03 | 2.80E+03 | 2.79E+03 | 2.51E+03 | 2.23E+03 | 2.13E+03 |
Std | 2.44E+02 | 3.81E+02 | 3.02E+02 | 2.02E+02 | 4.31E+02 | 2.71E+02 | 2.44E+02 | 1.97E+02 | |
F17 | Ave | 2.00E+03 | 2.48E+03 | 2.26E+03 | 2.09E+03 | 2.19E+03 | 2.00E+03 | 1.87E+03 | 1.83E+03 |
Std | 1.56E+02 | 2.29E+02 | 2.27E+02 | 7.95E+01 | 1.98E+02 | 1.74E+02 | 1.14E+02 | 7.07E+01 | |
F18 | Ave | 1.23E+06 | 1.19E+06 | 4.10E+04 | 1.76E+06 | 1.38E+06 | 6.57E+05 | 3.17E+05 | 1.99E+03 |
Std | 1.19E+06 | 1.17E+06 | 2.43E+04 | 7.29E+05 | 1.94E+06 | 6.89E+05 | 2.54E+05 | 5.32E+01 | |
F19 | Ave | 4.85E+05 | 2.42E+04 | 6.04E+03 | 3.48E+05 | 1.70E+04 | 2.44E+04 | 1.70E+04 | 1.93E+03 |
Std | 5.64E+05 | 5.06E+04 | 5.81E+03 | 2.02E+05 | 1.85E+04 | 4.03E+04 | 1.87E+04 | 9.76E+00 | |
F20 | Ave | 2.35E+03 | 2.64E+03 | 2.47E+03 | 2.41E+03 | 2.58E+03 | 2.33E+03 | 2.22E+03 | 2.16E+03 |
Std | 1.30E+02 | 2.56E+02 | 1.85E+02 | 1.04E+02 | 2.07E+02 | 1.32E+02 | 9.27E+01 | 7.40E+01 | |
F21 | Ave | 2.39E+03 | 2.50E+03 | 2.41E+03 | 2.48E+03 | 2.41E+03 | 2.38E+03 | 2.36E+03 | 2.35E+03 |
Std | 2.55E+01 | 4.99E+01 | 7.71E+01 | 1.04E+01 | 3.25E+01 | 2.54E+01 | 1.73E+01 | 1.16E+01 | |
F22 | Ave | 4.22E+03 | 6.13E+03 | 2.69E+03 | 4.97E+03 | 2.97E+03 | 4.01E+03 | 2.52E+03 | 2.55E+03 |
Std | 1.90E+03 | 2.00E+03 | 1.22E+03 | 6.54E+02 | 1.48E+03 | 1.65E+03 | 8.57E+02 | 9.48E+02 | |
F23 | Ave | 2.75E+03 | 2.96E+03 | 2.83E+03 | 2.83E+03 | 2.79E+03 | 2.83E+03 | 2.70E+03 | 2.71E+03 |
Std | 4.36E+01 | 7.30E+01 | 6.56E+01 | 1.14E+01 | 3.94E+01 | 6.25E+01 | 1.36E+01 | 1.84E+01 | |
F24 | Ave | 2.93E+03 | 3.11E+03 | 3.01E+03 | 3.03E+03 | 2.95E+03 | 2.99E+03 | 2.87E+03 | 2.88E+03 |
Std | 6.71E+01 | 8.18E+01 | 6.85E+01 | 9.70E+00 | 4.15E+01 | 5.85E+01 | 1.40E+01 | 1.93E+01 | |
F25 | Ave | 2.96E+03 | 2.91E+03 | 2.91E+03 | 2.90E+03 | 2.92E+03 | 2.92E+03 | 2.89E+03 | 2.89E+03 |
Std | 1.94E+01 | 3.02E+01 | 2.04E+01 | 3.13E+00 | 2.22E+01 | 5.43E+01 | 1.53E+01 | 1.02E+01 | |
F26 | Ave | 4.54E+03 | 6.51E+03 | 4.78E+03 | 5.46E+03 | 5.60E+03 | 4.69E+03 | 3.99E+03 | 3.98E+03 |
Std | 3.24E+02 | 8.85E+02 | 1.60E+03 | 1.10E+02 | 1.47E+03 | 4.10E+02 | 5.79E+02 | 7.09E+02 | |
F27 | Ave | 3.24E+03 | 3.27E+03 | 3.29E+03 | 3.23E+03 | 3.25E+03 | 3.25E+03 | 3.21E+03 | 3.21E+03 |
Std | 1.91E+01 | 4.26E+01 | 4.77E+01 | 3.53E+00 | 2.63E+01 | 3.60E+01 | 9.44E+00 | 1.10E+01 | |
F28 | Ave | 3.37E+03 | 3.26E+03 | 3.23E+03 | 3.30E+03 | 3.26E+03 | 3.32E+03 | 3.22E+03 | 3.22E+03 |
Std | 6.42E+01 | 2.76E+01 | 2.11E+01 | 1.67E+01 | 3.31E+01 | 1.28E+02 | 2.11E+01 | 2.01E+01 | |
F29 | Ave | 3.77E+03 | 4.31E+03 | 4.16E+03 | 4.07E+03 | 3.96E+03 | 3.67E+03 | 3.49E+03 | 3.52E+03 |
Std | 1.39E+02 | 2.55E+02 | 4.02E+02 | 1.20E+02 | 2.09E+02 | 1.65E+02 | 1.12E+02 | 9.41E+01 | |
F30 | Ave | 9.71E+06 | 2.05E+05 | 1.37E+04 | 3.37E+05 | 1.96E+05 | 4.89E+04 | 2.39E+04 | 1.16E+04 |
Std | 6.16E+06 | 1.19E+05 | 6.95E+03 | 1.20E+05 | 2.06E+05 | 7.51E+04 | 2.09E+04 | 2.22E+03 | |
(W|T|L) | Ave | (20|0|0) | (20|0|0) | (20|0|0) | (20|0|0) | (20|0|0)) | (20|0|0) | (15|0|5) | |
Std | (20|0|0) | (20|0|0) | (20|0|0) | (12|0|8) | (20|0|0) | (20|0|0) | (20|0|0) |
Function | GWO | AVAO | GTO | DE | COA | PSO | SBOA | CSBOA | |
F1 | Ave | 3.23E+10 | 1.49E+08 | 1.39E+09 | 7.42E+09 | 2.58E+10 | 2.64E+10 | 2.17E+09 | 1.71E+09 |
Std | 7.93E+09 | 1.63E+08 | 1.26E+09 | 9.99E+08 | 8.15E+09 | 1.11E+10 | 1.80E+09 | 2.21E+09 | |
F3 | Ave | 3.54E+05 | 3.09E+05 | 1.68E+05 | 7.65E+05 | 6.21E+05 | 4.88E+05 | 2.59E+05 | 1.52E+05 |
Std | 3.95E+04 | 1.86E+04 | 2.15E+04 | 6.83E+04 | 9.67E+04 | 1.02E+05 | 2.50E+04 | 1.78E+04 | |
F4 | Ave | 3.02E+03 | 1.13E+03 | 1.38E+03 | 2.31E+03 | 3.02E+03 | 2.51E+03 | 1.14E+03 | 1.05E+03 |
Std | 8.11E+02 | 3.93E+02 | 1.83E+02 | 1.80E+02 | 7.87E+02 | 9.18E+02 | 1.19E+02 | 1.25E+02 | |
F5 | Ave | 1.14E+03 | 1.32E+03 | 1.34E+03 | 1.65E+03 | 1.42E+03 | 1.07E+03 | 1.04E+03 | 1.09E+03 |
Std | 1.04E+02 | 7.27E+01 | 5.05E+01 | 2.60E+01 | 3.38E+01 | 1.23E+02 | 6.25E+01 | 8.70E+01 | |
F6 | Ave | 6.32E+02 | 6.62E+02 | 6.62E+02 | 6.25E+02 | 6.68E+02 | 6.31E+02 | 6.26E+02 | 6.23E+02 |
Std | 4.44E+00 | 3.89E+00 | 4.71E+00 | 1.03E+00 | 1.94E+00 | 8.38E+00 | 5.90E+00 | 1.11E+01 | |
F7 | Ave | 1.87E+03 | 3.08E+03 | 2.89E+03 | 2.16E+03 | 3.18E+03 | 1.78E+03 | 1.92E+03 | 1.88E+03 |
Std | 1.38E+02 | 1.64E+02 | 2.12E+02 | 3.51E+01 | 2.82E+02 | 1.97E+02 | 1.21E+02 | 2.26E+02 | |
F8 | Ave | 1.44E+03 | 1.73E+03 | 1.70E+03 | 1.92E+03 | 1.90E+03 | 1.34E+03 | 1.37E+03 | 1.37E+03 |
Std | 1.09E+02 | 8.81E+01 | 1.01E+02 | 2.76E+01 | 5.28E+01 | 7.79E+01 | 6.87E+01 | 9.42E+01 | |
F9 | Ave | 3.37E+04 | 2.52E+04 | 2.45E+04 | 5.78E+04 | 3.99E+04 | 4.28E+04 | 2.10E+04 | 2.07E+04 |
Std | 9.40E+03 | 2.20E+03 | 2.31E+03 | 5.07E+03 | 9.91E+03 | 1.81E+04 | 3.13E+03 | 3.49E+03 | |
F10 | Ave | 1.72E+04 | 1.70E+04 | 1.72E+04 | 3.15E+04 | 2.22E+04 | 1.73E+04 | 1.62E+04 | 1.52E+04 |
Std | 4.32E+03 | 1.69E+03 | 2.31E+03 | 5.22E+02 | 2.78E+03 | 2.27E+03 | 1.35E+03 | 1.24E+03 | |
(W|T|L) | Ave | (9|0|0) | (8|0|1) | (8|0|1) | (9|0|0) | (9|0|0) | (7|0|2) | (7|0|2) | |
Std | (9|0|0) | (8|0|1) | (9|0|0) | (4|0|5) | (9|0|0) | (8|0|1) | (9|0|0) |
Function | GWO | AVAO | GTO | DE | COA | PSO | SBOA | CSBOA | |
F11 | Ave | 5.83E+04 | 3.30E+04 | 8.73E+03 | 2.32E+05 | 2.39E+05 | 2.88E+04 | 1.45E+04 | 4.64E+03 |
Std | 1.51E+04 | 8.89E+03 | 2.86E+03 | 2.95E+04 | 6.36E+04 | 8.29E+03 | 4.90E+03 | 9.91E+02 | |
F12 | Ave | 5.23E+09 | 2.73E+08 | 1.41E+08 | 5.44E+09 | 1.66E+09 | 8.98E+09 | 1.19E+08 | 7.16E+07 |
Std | 2.90E+09 | 1.34E+08 | 5.74E+07 | 5.88E+08 | 2.11E+09 | 6.09E+09 | 5.65E+07 | 3.34E+07 | |
F13 | Ave | 6.64E+08 | 8.03E+04 | 2.70E+04 | 1.61E+06 | 9.58E+06 | 9.76E+08 | 1.51E+04 | 9.33E+03 |
Std | 6.53E+08 | 2.99E+04 | 1.09E+04 | 1.47E+06 | 2.81E+07 | 1.41E+09 | 6.61E+03 | 8.33E+03 | |
F14 | Ave | 5.72E+06 | 3.40E+06 | 4.74E+05 | 3.48E+07 | 4.81E+06 | 3.63E+06 | 2.56E+06 | 7.00E+04 |
Std | 3.40E+06 | 1.84E+06 | 2.07E+05 | 8.23E+06 | 3.11E+06 | 2.39E+06 | 1.45E+06 | 7.12E+04 | |
F15 | Ave | 1.10E+08 | 6.39E+04 | 1.15E+04 | 4.84E+06 | 2.02E+05 | 3.20E+08 | 5.27E+03 | 6.93E+03 |
Std | 1.21E+08 | 2.91E+04 | 5.91E+03 | 3.02E+06 | 1.76E+05 | 5.03E+08 | 4.20E+03 | 6.48E+03 | |
F16 | Ave | 5.81E+03 | 6.62E+03 | 6.40E+03 | 1.11E+04 | 7.15E+03 | 6.00E+03 | 5.16E+03 | 5.16E+03 |
Std | 6.15E+02 | 8.47E+02 | 8.64E+02 | 2.39E+02 | 8.58E+02 | 7.22E+02 | 7.38E+02 | 6.78E+02 | |
F17 | Ave | 4.93E+03 | 6.07E+03 | 5.79E+03 | 7.74E+03 | 6.00E+03 | 6.12E+03 | 4.57E+03 | 4.46E+03 |
Std | 5.39E+02 | 5.95E+02 | 6.79E+02 | 2.37E+02 | 7.65E+02 | 1.15E+03 | 5.45E+02 | 4.77E+02 | |
F18 | Ave | 5.63E+06 | 3.77E+06 | 8.51E+05 | 6.95E+07 | 4.77E+06 | 8.01E+06 | 3.25E+06 | 2.54E+05 |
Std | 2.73E+06 | 2.01E+06 | 4.73E+05 | 1.79E+07 | 2.19E+06 | 5.02E+06 | 1.45E+06 | 1.26E+05 | |
F19 | Ave | 1.14E+08 | 3.35E+05 | 1.08E+04 | 7.41E+06 | 2.13E+06 | 1.78E+08 | 6.98E+03 | 8.21E+03 |
Std | 1.26E+08 | 2.00E+05 | 7.47E+03 | 4.91E+06 | 1.07E+06 | 2.94E+08 | 7.52E+03 | 9.33E+03 | |
F20 | Ave | 4.94E+03 | 5.98E+03 | 5.39E+03 | 7.51E+03 | 6.91E+03 | 5.26E+03 | 4.65E+03 | 4.29E+03 |
Std | 9.73E+02 | 5.55E+02 | 5.02E+02 | 2.76E+02 | 5.58E+02 | 6.72E+02 | 5.72E+02 | 5.12E+02 | |
F21 | Ave | 2.95E+03 | 3.54E+03 | 3.27E+03 | 3.47E+03 | 3.45E+03 | 3.04E+03 | 2.81E+03 | 2.86E+03 |
Std | 7.02E+01 | 1.42E+02 | 1.15E+02 | 3.18E+01 | 1.69E+02 | 1.01E+02 | 5.89E+01 | 8.82E+01 | |
F22 | Ave | 2.06E+04 | 2.03E+04 | 2.25E+04 | 3.39E+04 | 2.85E+04 | 1.96E+04 | 1.92E+04 | 1.85E+04 |
Std | 4.97E+03 | 1.48E+03 | 3.57E+03 | 5.13E+02 | 2.68E+03 | 2.03E+03 | 1.95E+03 | 1.32E+03 | |
F23 | Ave | 3.54E+03 | 4.09E+03 | 4.14E+03 | 3.79E+03 | 4.02E+03 | 4.30E+03 | 3.27E+03 | 3.24E+03 |
Std | 8.75E+01 | 1.64E+02 | 2.28E+02 | 2.88E+01 | 1.66E+02 | 2.29E+02 | 5.96E+01 | 7.85E+01 | |
F24 | Ave | 4.12E+03 | 5.08E+03 | 4.94E+03 | 4.33E+03 | 4.87E+03 | 5.26E+03 | 3.83E+03 | 3.87E+03 |
Std | 1.44E+02 | 2.97E+02 | 4.27E+02 | 3.06E+01 | 2.36E+02 | 4.11E+02 | 7.23E+01 | 8.76E+01 | |
F25 | Ave | 5.53E+03 | 3.75E+03 | 3.97E+03 | 6.69E+03 | 5.21E+03 | 4.47E+03 | 3.76E+03 | 3.74E+03 |
Std | 5.44E+02 | 8.51E+01 | 1.51E+02 | 3.70E+02 | 5.42E+02 | 7.75E+02 | 9.88E+01 | 1.22E+02 | |
F26 | Ave | 1.36E+04 | 2.37E+04 | 2.30E+04 | 1.68E+04 | 2.63E+04 | 1.89E+04 | 1.41E+04 | 1.33E+04 |
Std | 8.25E+02 | 2.17E+03 | 3.20E+03 | 2.86E+02 | 4.30E+03 | 3.77E+03 | 2.88E+03 | 1.61E+03 | |
F27 | Ave | 3.94E+03 | 4.12E+03 | 4.06E+03 | 4.36E+03 | 4.15E+03 | 4.00E+03 | 3.57E+03 | 3.50E+03 |
Std | 1.18E+02 | 2.91E+02 | 3.15E+02 | 9.78E+01 | 2.02E+02 | 2.92E+02 | 7.28E+01 | 7.00E+01 | |
F28 | Ave | 7.12E+03 | 4.01E+03 | 4.08E+03 | 1.29E+04 | 6.65E+03 | 6.55E+03 | 4.03E+03 | 3.80E+03 |
Std | 1.38E+03 | 1.40E+02 | 1.72E+02 | 8.70E+02 | 8.33E+02 | 1.89E+03 | 1.81E+02 | 1.19E+02 | |
F29 | Ave | 7.99E+03 | 8.57E+03 | 8.20E+03 | 1.03E+04 | 9.29E+03 | 7.48E+03 | 6.53E+03 | 6.45E+03 |
Std | 6.02E+02 | 6.07E+02 | 9.11E+02 | 2.17E+02 | 8.70E+02 | 6.33E+02 | 4.69E+02 | 3.67E+02 | |
F30 | Ave | 7.02E+08 | 8.35E+06 | 1.03E+06 | 1.50E+07 | 3.99E+07 | 1.12E+09 | 1.88E+05 | 5.82E+04 |
Std | 7.56E+08 | 4.85E+06 | 7.01E+05 | 3.11E+06 | 2.20E+07 | 1.00E+09 | 1.05E+05 | 3.13E+04 | |
(W|T|L) | Ave | (20|0|0) | (20|0|0) | (20|0|0) | (11|0|9) | (20|0|0) | (20|0|2) | (15|0|5) | |
Std | (20|0|0) | (19|0|1) | (19|0|1) | (16|0|14) | (20|0|0) | (20|0|0) | (13|0|7) |
Type | ID | CEC2022 function name | Dimension | fmin |
Unimodal | F1 | Shifted and full rotated Zakharov function | 10/20 | 300 |
Multimodal | F2 | Shifted and full rotated Rosenbrock's function | 10/20 | 400 |
F3 | Shifted and full rotated Rastrigin's function | 10/20 | 600 | |
F4 | Shifted and full rotated non-continuous Rastrigin's function | 10/20 | 800 | |
F5 | Shifted and full rotated levy function | 10/20 | 900 | |
Hybrid | F6 | Hybrid function 1 (N = 3) | 10/20 | 1800 |
F7 | Hybrid function 2 (N = 6) | 10/20 | 2000 | |
F8 | Hybrid function 3 (N = 5) | 10/20 | 2200 | |
Composition | F9 | Composition function 1 (N = 5) | 10/20 | 2300 |
F10 | Composition function 2 (N = 4) | 10/20 | 2400 | |
F11 | Composition function 3 (N = 5) | 10/20 | 2600 | |
F12 | Composition function 4 (N = 6) | 10/20 | 2700 | |
Search Range [−100,100]D |
Function | GWO | AVAO | GTO | DE | COA | PSO | SBOA | CSBOA | |
F1 | Ave | 1.10E+03 | 3.00E+02 | 3.00E+02 | 4.55E+03 | 1.01E+03 | 3.00E+02 | 3.00E+02 | 3.00E+02 |
Std | 1.35E+03 | 1.13E-07 | 4.31E-13 | 1.44E+03 | 6.70E+02 | 2.01E-06 | 5.46E-04 | 4.72E-14 | |
F2 | Ave | 4.23E+02 | 4.10E+02 | 4.05E+02 | 4.08E+02 | 4.06E+02 | 4.13E+02 | 4.05E+02 | 4.05E+02 |
Std | 2.34E+01 | 1.75E+01 | 3.12E+00 | 4.75E-01 | 3.30E+00 | 1.82E+01 | 3.71E+00 | 3.21E+00 | |
F3 | Ave | 6.01E+02 | 6.13E+02 | 6.03E+02 | 6.00E+02 | 6.03E+02 | 6.00E+02 | 6.00E+02 | 6.00E+02 |
Std | 8.63E-01 | 1.02E+01 | 3.89E+00 | 1.16E-07 | 5.46E+00 | 4.17E-01 | 2.60E-07 | 9.15E-06 | |
F4 | Ave | 8.13E+02 | 8.30E+02 | 8.22E+02 | 8.25E+02 | 8.32E+02 | 8.12E+02 | 8.10E+02 | 8.07E+02 |
Std | 7.78E+00 | 1.11E+01 | 7.75E+00 | 4.15E+00 | 5.72E+00 | 4.70E+00 | 4.60E+00 | 2.74E+00 | |
F5 | Ave | 9.05E+02 | 1.14E+03 | 9.16E+02 | 9.00E+02 | 9.33E+02 | 9.00E+02 | 9.00E+02 | 9.00E+02 |
Std | 1.45E+01 | 2.06E+02 | 1.44E+01 | 8.38E-02 | 1.06E+02 | 9.49E-02 | 3.70E-10 | 3.21E-13 | |
F6 | Ave | 6.35E+03 | 4.18E+03 | 1.88E+03 | 6.66E+03 | 4.57E+03 | 5.02E+03 | 3.76E+03 | 1.80E+03 |
Std | 2.30E+03 | 2.21E+03 | 6.15E+01 | 3.48E+03 | 2.22E+03 | 2.59E+03 | 1.60E+03 | 4.26E-01 | |
F7 | Ave | 2.03E+03 | 2.03E+03 | 2.02E+03 | 2.01E+03 | 2.02E+03 | 2.01E+03 | 2.01E+03 | 2.00E+03 |
Std | 1.01E+01 | 9.55E+00 | 1.07E+01 | 2.10E+00 | 8.42E+00 | 9.82E+00 | 9.51E+00 | 3.68E+00 | |
F8 | Ave | 2.22E+03 | 2.22E+03 | 2.22E+03 | 2.22E+03 | 2.23E+03 | 2.22E+03 | 2.21E+03 | 2.20E+03 |
Std | 6.43E+00 | 4.08E+00 | 5.86E+00 | 4.08E+00 | 5.18E+00 | 8.21E+00 | 9.57E+00 | 6.11E+00 | |
F9 | Ave | 2.55E+03 | 2.53E+03 | 2.53E+03 | 2.53E+03 | 2.53E+03 | 2.53E+03 | 2.53E+03 | 2.53E+03 |
Std | 3.17E+01 | 2.68E+01 | 1.41E-01 | 2.46E-12 | 4.35E-05 | 2.68E+01 | 8.44E-14 | 8.44E-14 | |
F10 | Ave | 2.53E+03 | 2.55E+03 | 2.51E+03 | 2.50E+03 | 2.53E+03 | 2.55E+03 | 2.51E+03 | 2.52E+03 |
Std | 5.21E+01 | 6.53E+01 | 3.01E+01 | 1.24E-01 | 5.12E+01 | 5.72E+01 | 3.68E+01 | 4.34E+01 | |
F11 | Ave | 2.92E+03 | 2.70E+03 | 2.62E+03 | 2.88E+03 | 2.77E+03 | 2.68E+03 | 2.75E+03 | 2.71E+03 |
Std | 8.73E+01 | 1.52E+02 | 7.71E+01 | 3.99E+01 | 1.35E+02 | 1.42E+02 | 1.55E+02 | 1.47E+02 | |
F12 | Ave | 2.86E+03 | 2.87E+03 | 2.86E+03 | 2.86E+03 | 2.86E+03 | 2.87E+03 | 2.86E+03 | 2.86E+03 |
Std | 1.71E+00 | 4.47E+00 | 1.75E+00 | 9.88E-01 | 1.64E+00 | 1.30E+01 | 1.68E+00 | 1.67E+00 | |
(W|T|L) | Ave | (12|0|0) | (12|0|0) | (10|0|2) | (11|0|1) | (12|0|0) | (12|0|0) | (10|2|0) | |
Std | (12|0|0) | (11|0|1) | (11|0|1) | (7|0|5) | (12|0|0) | (12|0|0) | (12|0|0) |
Function | GWO | AVAO | GTO | DE | COA | PSO | SBOA | CSBOA | |
F1 | Ave | 9.15E+03 | 3.50E+03 | 3.01E+02 | 3.63E+04 | 2.94E+04 | 1.11E+03 | 4.68E+02 | 3.00E+02 |
Std | 4.52E+03 | 2.47E+03 | 1.92E+00 | 6.83E+03 | 7.90E+03 | 3.35E+02 | 1.41E+02 | 1.06E-01 | |
F2 | Ave | 4.85E+02 | 4.62E+02 | 4.51E+02 | 4.49E+02 | 4.66E+02 | 4.59E+02 | 4.54E+02 | 4.45E+02 |
Std | 3.14E+01 | 2.28E+01 | 1.53E+01 | 1.42E-01 | 1.86E+01 | 1.89E+01 | 1.18E+01 | 1.79E+01 | |
F3 | Ave | 6.03E+02 | 6.38E+02 | 6.23E+02 | 6.00E+02 | 6.20E+02 | 6.01E+02 | 6.00E+02 | 6.00E+02 |
Std | 1.65E+00 | 1.02E+01 | 8.07E+00 | 1.48E-03 | 1.46E+01 | 1.30E+00 | 2.80E-03 | 6.13E-02 | |
F4 | Ave | 8.59E+02 | 8.93E+02 | 8.73E+02 | 9.16E+02 | 8.81E+02 | 8.42E+02 | 8.32E+02 | 8.34E+02 |
Std | 2.89E+01 | 2.49E+01 | 2.00E+01 | 6.54E+00 | 1.52E+01 | 1.57E+01 | 8.71E+00 | 1.18E+01 | |
F5 | Ave | 9.90E+02 | 2.37E+03 | 1.68E+03 | 1.07E+03 | 2.06E+03 | 9.23E+02 | 9.02E+02 | 9.00E+02 |
Std | 6.19E+01 | 3.56E+02 | 3.66E+02 | 4.88E+01 | 6.81E+02 | 3.83E+01 | 1.65E+00 | 8.08E-01 | |
F6 | Ave | 2.30E+06 | 6.21E+03 | 3.76E+03 | 3.84E+06 | 7.61E+03 | 5.85E+04 | 7.28E+03 | 1.86E+03 |
Std | 6.06E+06 | 6.03E+03 | 2.42E+03 | 1.48E+06 | 5.60E+03 | 2.70E+05 | 5.83E+03 | 2.39E+01 | |
F7 | Ave | 2.06E+03 | 2.16E+03 | 2.09E+03 | 2.05E+03 | 2.07E+03 | 2.04E+03 | 2.03E+03 | 2.02E+03 |
Std | 3.29E+01 | 5.51E+01 | 2.91E+01 | 7.37E+00 | 2.98E+01 | 1.98E+01 | 8.77E+00 | 5.18E+00 | |
F8 | Ave | 2.25E+03 | 2.24E+03 | 2.23E+03 | 2.23E+03 | 2.25E+03 | 2.27E+03 | 2.22E+03 | 2.22E+03 |
Std | 4.61E+01 | 2.32E+01 | 3.13E+01 | 1.52E+00 | 3.57E+01 | 6.61E+01 | 2.06E+00 | 9.52E-01 | |
F9 | Ave | 2.50E+03 | 2.48E+03 | 2.48E+03 | 2.48E+03 | 2.48E+03 | 2.50E+03 | 2.48E+03 | 2.48E+03 |
Std | 1.25E+01 | 7.91E-01 | 2.20E-02 | 3.34E-03 | 9.05E-02 | 2.77E+01 | 3.40E-05 | 3.70E-08 | |
F10 | Ave | 3.01E+03 | 3.01E+03 | 2.94E+03 | 2.52E+03 | 3.40E+03 | 2.74E+03 | 2.53E+03 | 2.50E+03 |
Std | 6.22E+02 | 6.28E+02 | 9.52E+02 | 9.19E+00 | 1.19E+03 | 3.43E+02 | 5.69E+01 | 5.72E+01 | |
F11 | Ave | 3.37E+03 | 2.94E+03 | 2.96E+03 | 2.90E+03 | 3.01E+03 | 2.90E+03 | 2.91E+03 | 2.90E+03 |
Std | 1.87E+02 | 4.98E+01 | 8.97E+01 | 2.48E-02 | 4.65E+02 | 8.49E-04 | 7.73E-04 | 1.83E+01 | |
F12 | Ave | 2.96E+03 | 2.98E+03 | 3.00E+03 | 2.95E+03 | 2.98E+03 | 2.98E+03 | 2.94E+03 | 2.94E+03 |
Std | 9.07E+00 | 3.01E+01 | 4.55E+01 | 1.87E+00 | 1.88E+01 | 3.22E+01 | 8.79E+00 | 6.48E+00 | |
(W|T|L) | Ave | (12|0|0) | (12|0|0) | (12|0|0) | (11|0|1) | (12|0|0) | (12|0|0) | (9|2|1) | |
Std | (12|0|0) | (12|0|0) | (12|0|0) | (7|0|5) | (12|0|0) | (12|0|0) | (11|0|1) |
Function | GWO | AVAO | GTO | DE | COA | PSO | SBOA |
F1 | 3.02E-11 | 2.77E-01 | 8.77E-02 | 3.02E-11 | 3.02E-11 | 4.08E-05 | 1.17E-03 |
F3 | 3.02E-11 | 3.02E-11 | 1.95E-03 | 3.02E-11 | 3.02E-11 | 3.02E-11 | 6.70E-11 |
F4 | 2.61E-10 | 1.17E-02 | 1.09E-01 | 1.78E-04 | 1.16E-07 | 5.09E-06 | 6.15E-02 |
F5 | 2.39E-04 | 3.02E-11 | 3.69E-11 | 3.02E-11 | 3.34E-11 | 6.79E-02 | 9.71E-01 |
F6 | 3.02E-11 | 3.02E-11 | 3.02E-11 | 2.53E-04 | 3.02E-11 | 3.47E-10 | 1.26E-01 |
F7 | 1.25E-05 | 3.02E-11 | 3.02E-11 | 2.37E-10 | 4.08E-11 | 1.58E-01 | 2.01E-01 |
F8 | 3.51E-02 | 5.49E-11 | 6.07E-11 | 3.02E-11 | 5.49E-11 | 8.88E-01 | 2.89E-03 |
F9 | 5.49E-11 | 3.02E-11 | 3.02E-11 | 3.02E-11 | 3.02E-11 | 1.41E-04 | 8.77E-02 |
F10 | 3.56E-04 | 6.70E-11 | 1.41E-09 | 3.02E-11 | 3.34E-11 | 3.83E-06 | 3.27E-02 |
F11 | 3.02E-11 | 2.44E-09 | 3.50E-09 | 3.02E-11 | 3.02E-11 | 1.07E-09 | 2.39E-04 |
F12 | 3.02E-11 | 3.02E-11 | 2.25E-04 | 3.02E-11 | 3.02E-11 | 6.07E-11 | 1.70E-08 |
F13 | 3.02E-11 | 3.02E-11 | 3.34E-11 | 3.02E-11 | 3.02E-11 | 3.02E-11 | 2.03E-09 |
F14 | 3.02E-11 | 3.02E-11 | 3.02E-11 | 3.02E-11 | 3.02E-11 | 3.02E-11 | 3.02E-11 |
F15 | 3.02E-11 | 3.02E-11 | 3.34E-11 | 3.02E-11 | 3.02E-11 | 3.02E-11 | 3.02E-11 |
F16 | 2.15E-06 | 8.15E-11 | 2.87E-10 | 1.96E-10 | 1.10E-08 | 4.44E-07 | 6.57E-02 |
F17 | 5.46E-06 | 3.69E-11 | 1.46E-10 | 8.15E-11 | 3.47E-10 | 2.00E-05 | 3.55E-01 |
F18 | 3.02E-11 | 3.02E-11 | 3.02E-11 | 3.02E-11 | 3.02E-11 | 3.02E-11 | 3.02E-11 |
F19 | 3.02E-11 | 3.02E-11 | 3.02E-11 | 3.02E-11 | 3.02E-11 | 3.02E-11 | 3.16E-10 |
F20 | 3.35E-08 | 4.98E-11 | 1.17E-09 | 2.37E-10 | 1.17E-09 | 3.01E-07 | 3.03E-02 |
F21 | 4.44E-07 | 3.02E-11 | 2.20E-07 | 3.02E-11 | 2.67E-09 | 3.18E-04 | 2.75E-03 |
F22 | 3.20E-09 | 5.00E-09 | 3.32E-06 | 7.12E-09 | 5.97E-09 | 6.52E-09 | 1.25E-04 |
F23 | 9.83E-08 | 3.02E-11 | 1.61E-10 | 3.02E-11 | 7.39E-11 | 4.08E-11 | 3.11E-01 |
F24 | 2.00E-05 | 3.02E-11 | 4.50E-11 | 3.02E-11 | 4.62E-10 | 6.70E-11 | 1.02E-01 |
F25 | 3.69E-11 | 4.42E-06 | 1.19E-06 | 1.07E-07 | 6.53E-08 | 2.15E-06 | 1.38E-02 |
F26 | 5.87E-04 | 4.62E-10 | 1.22E-02 | 3.02E-11 | 9.51E-06 | 5.86E-06 | 9.59E-01 |
F27 | 5.97E-09 | 6.70E-11 | 3.69E-11 | 8.10E-10 | 5.07E-10 | 7.12E-09 | 1.49E-01 |
F28 | 6.07E-11 | 2.57E-07 | 7.62E-03 | 3.02E-11 | 1.60E-07 | 1.07E-09 | 1.33E-01 |
F29 | 9.26E-09 | 3.02E-11 | 6.07E-11 | 3.34E-11 | 2.87E-10 | 1.11E-04 | 2.34E-01 |
F30 | 3.02E-11 | 3.02E-11 | 7.06E-01 | 3.02E-11 | 3.02E-11 | 3.16E-05 | 2.13E-04 |
Function | GWO | AVAO | GTO | DE | COA | PSO | SBOA |
F1 | 3.02E-11 | 9.83E-08 | 2.51E-02 | 1.17E-09 | 3.02E-11 | 3.02E-11 | 9.82E-01 |
F3 | 3.02E-11 | 3.02E-11 | 2.81E-02 | 3.02E-11 | 3.02E-11 | 3.02E-11 | 3.34E-11 |
F4 | 3.02E-11 | 1.16E-07 | 2.23E-09 | 3.02E-11 | 3.02E-11 | 3.02E-11 | 1.03E-06 |
F5 | 3.79E-01 | 6.07E-11 | 4.20E-10 | 3.02E-11 | 3.02E-11 | 3.27E-02 | 1.77E-03 |
F6 | 5.09E-08 | 3.02E-11 | 3.02E-11 | 1.00E-03 | 3.02E-11 | 1.43E-05 | 9.07E-03 |
F7 | 4.92E-01 | 3.02E-11 | 3.69E-11 | 7.66E-05 | 3.34E-11 | 5.94E-02 | 6.41E-01 |
F8 | 6.52E-01 | 3.02E-11 | 3.69E-11 | 3.02E-11 | 3.02E-11 | 6.38E-03 | 1.78E-04 |
F9 | 1.56E-02 | 5.53E-08 | 4.11E-07 | 3.02E-11 | 3.02E-11 | 3.35E-08 | 3.33E-01 |
F10 | 1.17E-03 | 1.78E-04 | 1.86E-03 | 3.02E-11 | 3.02E-11 | 2.88E-06 | 8.77E-02 |
F11 | 3.02E-11 | 3.02E-11 | 1.61E-10 | 3.02E-11 | 3.02E-11 | 3.02E-11 | 3.34E-11 |
F12 | 3.02E-11 | 2.67E-09 | 8.20E-07 | 3.02E-11 | 3.02E-11 | 3.02E-11 | 7.60E-07 |
F13 | 3.02E-11 | 6.72E-10 | 2.83E-08 | 1.09E-10 | 6.07E-11 | 3.69E-11 | 1.00E-03 |
F14 | 3.02E-11 | 3.02E-11 | 4.50E-11 | 3.02E-11 | 3.02E-11 | 3.02E-11 | 3.02E-11 |
F15 | 3.02E-11 | 3.02E-11 | 1.17E-04 | 3.02E-11 | 3.02E-11 | 6.70E-11 | 4.64E-01 |
F16 | 1.25E-05 | 1.46E-10 | 1.07E-07 | 3.02E-11 | 1.55E-09 | 1.04E-04 | 4.64E-01 |
F17 | 2.05E-03 | 1.46E-10 | 1.69E-09 | 3.02E-11 | 1.46E-10 | 5.00E-09 | 1.44E-02 |
F18 | 3.02E-11 | 3.02E-11 | 1.56E-08 | 3.02E-11 | 3.02E-11 | 3.02E-11 | 6.07E-11 |
F19 | 3.02E-11 | 3.34E-11 | 1.00E-03 | 3.02E-11 | 3.02E-11 | 4.98E-11 | 6.73E-01 |
F20 | 3.18E-03 | 2.03E-09 | 2.38E-07 | 3.02E-11 | 2.37E-10 | 8.12E-04 | 7.28E-01 |
F21 | 2.68E-04 | 3.02E-11 | 3.02E-11 | 3.02E-11 | 3.02E-11 | 5.49E-11 | 7.73E-02 |
F22 | 2.61E-02 | 8.88E-06 | 7.12E-09 | 3.02E-11 | 3.02E-11 | 8.29E-06 | 1.81E-01 |
F23 | 3.02E-11 | 3.02E-11 | 3.02E-11 | 3.02E-11 | 3.02E-11 | 3.02E-11 | 3.99E-04 |
F24 | 6.12E-10 | 3.02E-11 | 3.02E-11 | 3.02E-11 | 3.02E-11 | 3.02E-11 | 5.79E-01 |
F25 | 3.02E-11 | 2.34E-01 | 2.57E-07 | 3.02E-11 | 3.02E-11 | 5.07E-10 | 7.01E-02 |
F26 | 3.55E-01 | 2.23E-09 | 4.20E-10 | 1.11E-06 | 3.69E-11 | 4.11E-07 | 3.79E-01 |
F27 | 6.07E-11 | 6.07E-11 | 4.50E-11 | 3.02E-11 | 4.08E-11 | 3.82E-10 | 2.51E-02 |
F28 | 3.02E-11 | 1.61E-06 | 2.19E-08 | 3.02E-11 | 3.02E-11 | 3.69E-11 | 8.35E-08 |
F29 | 6.12E-10 | 2.61E-10 | 1.46E-10 | 3.02E-11 | 4.50E-11 | 8.56E-04 | 7.39E-01 |
F30 | 3.02E-11 | 3.02E-11 | 3.02E-11 | 3.02E-11 | 3.02E-11 | 3.02E-11 | 1.17E-09 |
Function | GWO | AVAO | GTO | DE | COA | PSO | SBOA |
F1 | 1.45E-11 | 1.45E-11 | 5.17E-07 | 1.45E-11 | 1.45E-11 | 1.45E-11 | 1.45E-11 |
F2 | 1.69E-09 | 1.31E-01 | 1.68E-01 | 7.64E-05 | 1.25E-04 | 2.61E-04 | 3.10E-02 |
F3 | 1.26E-11 | 1.26E-11 | 1.26E-11 | 6.83E-03 | 1.91E-11 | 1.17E-03 | 5.43E-03 |
F4 | 3.00E-07 | 3.31E-11 | 4.55E-10 | 4.05E-11 | 4.40E-11 | 3.30E-03 | 5.54E-03 |
F5 | 3.35E-11 | 2.47E-11 | 4.68E-10 | 4.09E-11 | 5.00E-11 | 1.45E-01 | 2.74E-06 |
F6 | 3.02E-11 | 3.02E-11 | 3.02E-11 | 3.02E-11 | 3.02E-11 | 3.02E-11 | 3.02E-11 |
F7 | 2.87E-10 | 7.39E-11 | 9.92E-11 | 3.32E-06 | 2.03E-09 | 3.57E-06 | 3.50E-03 |
F8 | 4.98E-11 | 4.08E-11 | 1.61E-10 | 1.07E-09 | 1.96E-10 | 6.53E-08 | 2.16E-03 |
F9 | 1.21E-12 | 5.42E-09 | 3.34E-01 | 3.12E-04 | 1.21E-12 | 3.34E-01 | NAN |
F10 | 3.96E-08 | 2.67E-09 | 1.60E-07 | 8.48E-09 | 3.20E-09 | 3.96E-08 | 8.31E-03 |
F11 | 2.98E-09 | 2.95E-05 | 1.29E-01 | 3.90E-11 | 1.46E-07 | 4.87E-04 | 1.10E-05 |
F12 | 3.01E-11 | 8.94E-11 | 1.10E-09 | 2.15E-03 | 6.70E-10 | 4.46E-09 | 8.76E-02 |
Function | GWO | AVAO | GTO | DE | COA | PSO | SBOA |
F1 | 3.02E-11 | 3.02E-11 | 6.77E-05 | 3.02E-11 | 3.02E-11 | 3.02E-11 | 3.02E-11 |
F2 | 1.47E-07 | 7.30E-04 | 3.64E-02 | 1.95E-03 | 2.53E-04 | 1.76E-01 | 7.24E-02 |
F3 | 3.02E-11 | 3.02E-11 | 3.02E-11 | 1.11E-04 | 3.02E-11 | 3.20E-09 | 8.65E-01 |
F4 | 6.36E-05 | 4.50E-11 | 8.15E-11 | 3.02E-11 | 4.08E-11 | 1.86E-03 | 9.00E-01 |
F5 | 3.34E-11 | 3.02E-11 | 3.02E-11 | 3.02E-11 | 3.02E-11 | 1.70E-08 | 3.55E-01 |
F6 | 3.02E-11 | 3.02E-11 | 3.69E-11 | 3.02E-11 | 3.02E-11 | 3.02E-11 | 4.50E-11 |
F7 | 6.07E-11 | 3.02E-11 | 3.34E-11 | 3.02E-11 | 7.39E-11 | 2.49E-06 | 2.16E-03 |
F8 | 8.99E-11 | 6.70E-11 | 1.68E-04 | 3.02E-11 | 3.02E-11 | 1.29E-06 | 6.91E-04 |
F9 | 3.02E-11 | 3.02E-11 | 1.04E-04 | 3.02E-11 | 3.02E-11 | 3.00E-11 | 3.02E-11 |
F10 | 4.57E-09 | 1.69E-09 | 8.84E-07 | 1.19E-06 | 1.47E-07 | 8.20E-07 | 5.49E-01 |
F11 | 3.02E-11 | 7.12E-09 | 3.02E-11 | 3.02E-11 | 3.02E-11 | 3.02E-11 | 3.02E-11 |
F12 | 2.92E-09 | 1.09E-10 | 1.96E-10 | 1.87E-05 | 2.15E-10 | 1.55E-09 | 2.77E-01 |
Dimensions | 30 | 100 | |||
Algorithms | Mean rank | Overall rank | Mean rank | Overall rank | |
GWO | 5.43 | 5 | 5 | 5 | |
AVOA | 6.03 | 7 | 4.73 | 4 | |
GTO | 4.17 | 3 | 4.17 | 3 | |
DE | 6.17 | 8 | 6.67 | 8 | |
COA | 5.9 | 6 | 6.33 | 7 | |
PSO | 4.7 | 4 | 5.43 | 6 | |
SBOA | 2.23 | 2 | 2.23 | 2 | |
CSBOA | 1.37 | 1 | 1.43 | 1 |
Dimensions | 10 | 20 | |||
Algorithms | Mean rank | Overall rank | Mean rank | Overall rank | |
GWO | 6.25 | 8 | 5.91 | 6 | |
AVOA | 6.17 | 7 | 6.17 | 7 | |
GTO | 3.83 | 3 | 4.91 | 5 | |
DE | 4.33 | 4 | 4.17 | 3 | |
COA | 5.92 | 6 | 6.25 | 8 | |
PSO | 4.92 | 5 | 4.67 | 4 | |
SBOA | 2.79 | 2 | 2.25 | 2 | |
CSBOA | 1.79 | 1 | 1.67 | 1 |
Algorithm | Optimal values for variable | Optimal value | Ranking | |||
Ts | Th | R | L | |||
GWO | 0.785779 | 0.388407 | 40.702147 | 194.754644 | 5900.291027 | 4 |
AVOA | 0.989300 | 0.489012 | 51.259077 | 88.659593 | 6352.998707 | 8 |
GTO | 1.258847 | 0.622249 | 65.225232 | 10.000000 | 7319.000702 | 9 |
DE | 0.781083 | 0.386270 | 40.339766 | 200.000000 | 5914.483351 | 5 |
COA | 0.968743 | 0.480559 | 50.114317 | 97.637567 | 6319.415834 | 7 |
PSO | 0.973894 | 0.481396 | 50.460847 | 94.730452 | 6311.096260 | 5 |
QOCSOS | 0.778238 | 0.384893 | 40.322081 | 199.966711 | 5885.332774 | 2 |
SBOA | 0.779067 | 0.385093 | 40.3661670 | 199.352987 | 5886.870272 | 3 |
CSBOA | 0.778169 | 0.384649 | 40.319619 | 199.999999 | 5885.332773 | 1 |
Algorithm | Optimal values for variable | Optimal value | Ranking | ||
d | D | N | |||
GWO | 0.0523516599 | 0.372775760 | 10.415319625 | 0.012684229 | 4 |
AVOA | 0.050000000 | 0.317425416 | 14.027769769 | 0.012719054 | 6 |
GTO | 0.053325095 | 0.397367912 | 9.250854836 | 0.012712811 | 5 |
DE | 0.053135792 | 0.392469906 | 9.485223289 | 0.012726826 | 7 |
COA | 0.056774591 | 0.491795419 | 6.277155317 | 0.013121202 | 9 |
PSO | 0.054998195 | 0.441675285 | 7.622863618 | 0.012855954 | 8 |
QOCSOS | 0.055130816 | 0.444775663 | 7.544716912 | 0.012665282 | 2 |
SBOA | 0.052219133 | 0.369604350 | 10.571609914 | 0.012670312 | 3 |
CSBOA | 0.051700822 | 0.3570007342 | 11.272393937 | 0.012665235 | 1 |
Category | Algorithm | year | Inspiration/Description |
Evolutionary | Liver Cancer Algorithm (LCA) [7] | 2023 | Inspired by the ability of tumors to replicate and spread to other organs, an MA is introduced. |
Partial Reinforcement Optimizer (PRO)[8] | 2024 | Inspired by the partial reinforcement effect (PRE) theory, an evolutionary learning/training theory in psychology, a learner is intermittently reinforced to learn or strengthen a specific behavior based on a schedule. | |
Moss Growth Optimization (MGO)[9] | 2024 | Inspired by the growth of moss in the natural environment, an MA is introduced. | |
Physic-based | Planet Optimization Algorithm (POA) [13] | 2022 | Inspired by the laws of cosmic motion and starting from the interaction of the gravitational forces among planets, an MA is introduced. |
Kepler Optimization Algorithm (KOA) [14] | 2023 | Inspired by Kepler's laws of planetary motion, which can predict the position and velocity of the planets at any given time, an MA is introduced. | |
Rime Optimization Algorithm (RIME)[2] | 2023 | Inspired by the natural frost and ice growth mechanism, algorithms are developed by simulating the motion of soft frost particles for algorithmic search, and by simulating the crossover behavior between hard frost agents. | |
Snow ablation optimizer (SAO) [15] | 2023 | Inspired by the simulation of the sublimation and melting behavior of snow, an MA is introduced. | |
Newton-Raphson-based optimizer (NBRO) [16] | 2024 | The algorithm searches the solution space by defining the solution space using the set of vectors and employing operators such as Natural Resource Strategy (NRSR) as well as Trap Avoidance Operator (TAO). | |
Swarm intelligence | Crested Porcupine Optimizer (CPO)[22] | 2024 | Simulation of four different protective mechanisms in crown porcupines: sight, hearing, odor and physical attack. |
Hippopotamus optimization algorithm (HO) [23] | 2024 | Inspired by the behavioral patterns of hippos in the natural environment, an MA is introduced. | |
Seagull optimization algorithm (SOA)[24] | 2024 | Inspired by the migration and predatory behaviors of seagulls in the natural world, an MA is introduced. | |
enhanced African Vultures Optimization Algorithm (EAVOA) [35] | 2023 | They incorporated a rotating flight mechanism, a representative vulture selection mechanism, and a selection accumulation operator strategy into the original AVOA. It demonstrated superior optimization capabilities compared to the original AVOA. | |
an improved Seahorse Optimizer (mSHO) [41] | 2024 | This algorithm integrates three search operators: neighborhood-based local search, global search, and walking within the existing search to achieve a more robust development trend. | |
multi-strategy improvement Secretary Bird Optimization Algorithm (MISBOA) [42] | 2024 | The algorithm improves the Secretary Bird Optimization Algorithm by combining three strategies: feedback regulation mechanism, golden sinusoidal guidance, and population diversity, and the results show that MISBOA has demonstrated good performance. | |
Improved Secretary Bird Optimization Algorithm [43] | 2024 | ISBOA adds the Circle Chaotic Mapping, the differential evolution strategy, and the population diversity strategy into the SBOA, which achieves the optimal convergence speed and optimization accuracy. optimal convergence speed and optimization accuracy. | |
Crossover strategy-based Secretary Bird Optimization Algorithm (CSBOA) | 2024 | Under the SBOA algorithm's framework, CSBOA innovatively adds the initialization strategy of logical chaos mapping, improved differential operator strategy, and crossover strategy, which greatly enhances the algorithm's efficiency. |
Function | GWO | AVAO | GTO | DE | COA | PSO | SBOA | CSBOA |
F1 | 0.2163120 | 0.3335453 | 0.6284790 | 1.1332032 | 0.1795724 | 0.1761100 | 0.3141203 | 1.5787525 |
F2 | 0.2100052 | 0.3018989 | 0.6607184 | 1.1743020 | 0.1837386 | 0.1733390 | 0.3190766 | 1.7292872 |
F3 | 0.2985672 | 0.3746466 | 0.8275277 | 1.3605336 | 0.3303046 | 0.2590517 | 0.5342557 | 2.1225204 |
F4 | 0.2221101 | 0.3152409 | 0.7127616 | 1.1873779 | 0.2305653 | 0.1963042 | 0.3707470 | 1.8089242 |
F5 | 0.2342974 | 0.3237152 | 0.6934132 | 1.2154550 | 0.2350611 | 0.2044259 | 0.4110868 | 1.7652453 |
F6 | 0.2150608 | 0.3068646 | 0.6739646 | 1.1734847 | 0.1979094 | 0.1757798 | 0.3328941 | 1.7567508 |
F7 | 0.3356384 | 0.4250690 | 0.9167834 | 1.3618838 | 0.4035385 | 0.2982822 | 0.5812446 | 2.3796544 |
F8 | 0.3715650 | 0.4781056 | 1.0045935 | 1.4647472 | 0.4844369 | 0.3593346 | 0.6875874 | 2.5092880 |
F9 | 0.3040718 | 0.3946582 | 0.8335377 | 1.3782160 | 0.3375005 | 0.2658512 | 0.5529398 | 2.1785161 |
F10 | 0.2883326 | 0.3836225 | 0.8240736 | 1.3242494 | 0.3325307 | 0.2494263 | 0.5050225 | 2.0998797 |
F11 | 0.3608691 | 0.4689512 | 0.9485607 | 1.4617516 | 0.4222041 | 0.3393067 | 0.6414698 | 2.3714879 |
F12 | 0.3713333 | 0.4656035 | 0.9925775 | 1.4338215 | 0.4992448 | 0.3349514 | 0.6788769 | 2.6054365 |
Algorithms | Reference | Name of the parameter | Value of the parameter |
GWO | [18] | a | [0, 2] |
AVAO | [53] | L1, L2, w, p1, p2, p3 | 0.8, 0.2, 2.5, 0.6, 0.4, 0.6 |
GTO | [54] | P, r1, r2, r3 | P, r1, r2, r3 ∈ [0, 1] |
DE | [5] | F, CR | 0.8, 0.1 |
COA | [21] | temp | [20, 35] |
PSO | [17] | w, c1, c2 | [0.9, 0.4], 0.2, 0.2 |
SBOA | [25] | R1, RB, r, R2 | [0, 1], [0, 1], [0, 1], [0, 1] |
Strategy | CSBOA-1 | CSBOA-2 | CSBOA-3 | CSBOA-4 | CSBOA-5 | CSBOA-6 | CSBOA |
Logistic-tent chaotic mapping | 1 | 0 | 0 | 1 | 1 | 0 | 1 |
Improved differential mutation operator | 0 | 1 | 0 | 1 | 0 | 1 | 1 |
Crossover strategy | 0 | 0 | 1 | 0 | 1 | 1 | 1 |
Function | Metric | SBOA | CSBOA-1 | CSBOA-2 | CSBOA-3 | CSBOA-4 | CSBOA-5 | CSBOA-6 | CSBOA |
F1 | Ave | 468.0984 | 462.0113 | 360.6446 | 354.0001 | 387.1188 | 342.2765 | 300.0422 | 300.0315 |
Std | 152.9298 | 168.8314 | 58.5821 | 45.8167 | 73.5351 | 41.1544 | 0.1029 | 0.0485 | |
Rank | 8 | 7 | 5 | 4 | 6 | 3 | 2 | 1 | |
F2 | Ave | 454.3443 | 453.9698 | 450.5164 | 454.2942 | 448.7348 | 449.7043 | 448.4292 | 445.9545 |
Std | 11.3625 | 12.6751 | 8.4090 | 14.9607 | 17.7389 | 14.2097 | 14.6531 | 14.5031 | |
Rank | 8 | 6 | 5 | 7 | 3 | 4 | 2 | 1 | |
F3 | Ave | 600.0028 | 600.0102 | 600.0734 | 600.0090 | 600.0460 | 600.0024 | 600.0135 | 600.0185 |
Std | 0.0043 | 0.0473 | 0.2534 | 0.0389 | 0.1770 | 0.0036 | 0.0534 | 0.0816 | |
Rank | 2 | 4 | 8 | 3 | 7 | 1 | 5 | 6 | |
F4 | Ave | 832.8866 | 831.9954 | 835.5805 | 834.0843 | 833.6276 | 836.8336 | 833.9302 | 834.4919 |
Std | 9.9938 | 9.1814 | 14.3468 | 9.9029 | 14.4475 | 9.6940 | 11.8680 | 9.1798 | |
Rank | 2 | 1 | 7 | 5 | 3 | 8 | 4 | 6 | |
F5 | Ave | 902.4884 | 901.9341 | 901.9577 | 900.8848 | 902.2329 | 900.8906 | 901.1584 | 900.7389 |
Std | 2.7654 | 2.6170 | 3.2391 | 1.2353 | 2.0574 | 1.3218 | 1.7129 | 1.0435 | |
Rank | 8 | 5 | 6 | 2 | 7 | 3 | 4 | 1 | |
F6 | Ave | 7828.2811 | 6654.5031 | 6912.6022 | 7017.1025 | 6639.8939 | 7405.0108 | 1864.9143 | 1858.5483 |
Std | 6388.4941 | 5805.7743 | 5662.3248 | 5100.5059 | 4777.2112 | 6057.2718 | 23.3027 | 24.1598 | |
Rank | 8 | 4 | 5 | 6 | 3 | 7 | 2 | 1 | |
F7 | Ave | 2032.9970 | 2033.8804 | 2028.0807 | 2029.6145 | 2027.3489 | 2029.7563 | 2025.9835 | 2024.3204 |
Std | 9.8793 | 10.5050 | 7.0884 | 6.1269 | 4.6764 | 7.4310 | 5.7183 | 5.2719 | |
Rank | 7 | 8 | 4 | 5 | 3 | 6 | 2 | 1 | |
F8 | Ave | 2224.0843 | 2224.0779 | 2224.1301 | 2222.0094 | 2224.1719 | 2222.4307 | 2221.0820 | 2221.7982 |
Std | 1.9078 | 2.0098 | 1.9208 | 0.8741 | 2.0107 | 0.9763 | 2.4445 | 0.7588 | |
Rank | 6 | 5 | 7 | 3 | 8 | 4 | 1 | 2 | |
F9 | Ave | 2480.7814 | 2480.7813 | 2480.7813 | 2480.7813 | 2480.7813 | 2480.7813 | 2480.7813 | 2480.7813 |
Std | 0.0002 | 5.61E-05 | 8.45E-06 | 2.13E-07 | 1.67E-05 | 2.84E-07 | 4.19E-08 | 3.38E-08 | |
Rank | 8 | 7 | 6 | 4 | 5 | 3 | 2 | 1 | |
F10 | Ave | 2539.0264 | 2536.6607 | 2559.7474 | 2526.3202 | 2553.5768 | 2526.9783 | 2504.4556 | 2509.1785 |
Std | 60.6819 | 57.9123 | 166.4333 | 53.3955 | 174.2956 | 53.9692 | 39.6923 | 33.1152 | |
Rank | 6 | 5 | 8 | 3 | 7 | 4 | 1 | 2 | |
F11 | Ave | 2908.6735 | 2903.3341 | 2903.3341 | 2903.3333 | 2900.0008 | 2906.6667 | 2900.0000 | 2900.0000 |
Std | 69.4118 | 18.2574 | 18.2575 | 18.2574 | 0.0005 | 25.3708 | 6.60E-07 | 5.18E-07 | |
Rank | 8 | 5 | 6 | 4 | 3 | 7 | 2 | 1 | |
F12 | Ave | 2942.3513 | 2941.4095 | 2939.2758 | 2939.0545 | 2940.0607 | 2941.6061 | 2940.6537 | 2942.5940 |
Std | 6.2628 | 5.3666 | 3.9849 | 3.8635 | 4.5492 | 6.7650 | 4.8318 | 5.2684 | |
Rank | 7 | 5 | 2 | 1 | 3 | 6 | 4 | 8 |
Type | ID | CEC2017 function name | Dimension | fmin |
Unimodal | F1 | Shifted and rotated bent cigar function | 30/100 | 100 |
F2 | Shifted and rotated sum of different power function* | 30/100 | 200 | |
F3 | Shifted and rotated Zakharow function | 30/100 | 300 | |
Multimodal | F4 | Shifted and rotated Rosenbrock's function | 30/100 | 400 |
F5 | Shifted and rotated Rastrigin's Function | 30/100 | 500 | |
F6 | Shifted and rotated expanded Scaffer's F6 Function | 30/100 | 600 | |
F7 | Shifted and rotated Lunacek Bi-Rastrigin finc-tion | 30/100 | 700 | |
F8 | Shifted and rotated non-continuous Rastrigin's function | 30/100 | 800 | |
F9 | Shifted and rotated levy function | 30/100 | 900 | |
F10 | Shifted and rotated Schwefel's function | 30/100 | 1000 | |
Hybrid | F11 | Hybrid function 1 (N = 3) | 30/100 | 1100 |
F12 | Hybrid function 2 (N = 3) | 30/100 | 1200 | |
F13 | Hybrid function 3 (N = 3) | 30/100 | 1300 | |
F14 | Hybrid function 4 (N = 4) | 30/100 | 1400 | |
F15 | Hybrid function 5 (N = 4) | 30/100 | 1500 | |
F16 | Hybrid function 6 (N = 4) | 30/100 | 1600 | |
F17 | Hybrid function 7 (N = 5) | 30/100 | 1700 | |
F18 | Hybrid function 8 (N = 5) | 30/100 | 1800 | |
F19 | Hybrid function 9 (N = 5) | 30/100 | 1900 | |
F20 | Hybrid function 10 (N = 6) | 30/100 | 2000 | |
Composition | F21 | Composition function 1 (N = 3) | 30/100 | 2100 |
F22 | Composition function 2 (N = 3) | 30/100 | 2200 | |
F23 | Composition function 3 (N = 4) | 30/100 | 2300 | |
F24 | Composition function 4 (N = 4) | 30/100 | 2400 | |
F25 | Composition function 5 (N = 5) | 30/100 | 2500 | |
F26 | Composition function 6 (N = 5) | 30/100 | 2600 | |
F27 | Composition function 7 (N = 6) | 30/100 | 2700 | |
F28 | Composition function 8 (N = 6) | 30/100 | 2800 | |
F29 | Composition function 9 (N = 3) | 30/100 | 2900 | |
F30 | Composition function 10 (N = 3) | 30/100 | 3000 | |
Search Range [−100,100]D | ||||
F2 has been officially removed due to its unstable behavior on high-dimensional issues. |
Function | GWO | AVAO | GTO | DE | COA | PSO | SBOA | CSBOA | |
F1 | Ave | 1.18E+09 | 3.98E+03 | 4.73E+03 | 1.87E+06 | 5.17E+06 | 9.01E+08 | 6.67E+03 | 2.26E+03 |
Std | 9.70E+08 | 4.64E+03 | 6.14E+03 | 5.62E+05 | 9.56E+06 | 1.20E+09 | 6.76E+03 | 3.48E+03 | |
F3 | Ave | 4.26E+04 | 2.28E+04 | 1.92E+03 | 1.48E+05 | 8.38E+04 | 2.51E+04 | 5.12E+03 | 9.70E+02 |
Std | 9.88E+03 | 4.91E+03 | 1.70E+03 | 1.75E+04 | 1.88E+04 | 1.14E+04 | 2.70E+03 | 4.02E+02 | |
F4 | Ave | 5.71E+02 | 5.05E+02 | 4.96E+02 | 5.12E+02 | 5.29E+02 | 5.60E+02 | 5.00E+02 | 4.88E+02 |
Std | 4.53E+01 | 3.26E+01 | 2.94E+01 | 8.35E+00 | 2.78E+01 | 9.74E+01 | 3.35E+01 | 2.04E+01 | |
F5 | Ave | 5.96E+02 | 7.01E+02 | 6.89E+02 | 6.83E+02 | 7.18E+02 | 5.79E+02 | 5.68E+02 | 5.69E+02 |
Std | 3.54E+01 | 3.52E+01 | 4.64E+01 | 1.17E+01 | 6.03E+01 | 2.43E+01 | 1.55E+01 | 1.91E+01 | |
F6 | Ave | 6.06E+02 | 6.46E+02 | 6.36E+02 | 6.00E+02 | 6.40E+02 | 6.03E+02 | 6.00E+02 | 6.00E+02 |
Std | 2.13E+00 | 1.01E+01 | 9.25E+00 | 2.21E-02 | 1.46E+01 | 2.52E+00 | 4.12E-01 | 2.76E-01 | |
F7 | Ave | 8.65E+02 | 1.11E+03 | 1.02E+03 | 9.17E+02 | 1.12E+03 | 8.15E+02 | 8.11E+02 | 8.22E+02 |
Std | 4.65E+01 | 5.90E+01 | 6.59E+01 | 1.03E+01 | 1.55E+02 | 3.03E+01 | 2.84E+01 | 2.84E+01 | |
F8 | Ave | 8.93E+02 | 9.56E+02 | 9.43E+02 | 9.85E+02 | 9.67E+02 | 8.71E+02 | 8.59E+02 | 8.72E+02 |
Std | 4.51E+01 | 3.09E+01 | 2.73E+01 | 9.75E+00 | 3.34E+01 | 1.99E+01 | 1.54E+01 | 1.56E+01 | |
F9 | Ave | 1.42E+03 | 4.71E+03 | 3.59E+03 | 1.91E+03 | 6.17E+03 | 1.02E+03 | 9.62E+02 | 9.39E+02 |
Std | 3.24E+02 | 7.28E+02 | 9.82E+02 | 2.11E+02 | 2.17E+03 | 1.14E+02 | 8.84E+01 | 4.90E+01 | |
F10 | Ave | 4.48E+03 | 5.38E+03 | 5.30E+03 | 7.31E+03 | 5.65E+03 | 4.54E+03 | 3.99E+03 | 3.70E+03 |
Std | 1.17E+03 | 6.76E+02 | 9.07E+02 | 3.41E+02 | 6.68E+02 | 7.32E+02 | 7.51E+02 | 4.41E+02 | |
(W|T|L) | Ave | (9|0|0) | (9|0|0) | (9|0|0) | (9|0|0) | (9|0|0)) | (9|0|0) | (6|0|3) | |
Std | (9|0|0) | (9|0|0) | (9|0|0) | (3|0|6) | (9|0|0) | (9|0|0) | (7|0|2) |
Function | GWO | AVAO | GTO | DE | COA | PSO | SBOA | CSBOA | |
F11 | Ave | 1.75E+03 | 1.26E+03 | 1.23E+03 | 1.59E+03 | 1.36E+03 | 1.27E+03 | 1.18E+03 | 1.15E+03 |
Std | 7.82E+02 | 5.77E+01 | 4.11E+01 | 2.01E+02 | 7.20E+01 | 7.05E+01 | 3.30E+01 | 2.68E+01 | |
F12 | Ave | 4.62E+07 | 3.60E+06 | 3.59E+05 | 3.96E+07 | 4.71E+06 | 2.68E+07 | 7.42E+05 | 1.32E+05 |
Std | 6.14E+07 | 3.10E+06 | 3.49E+05 | 1.08E+07 | 4.78E+06 | 5.92E+07 | 8.17E+05 | 1.35E+05 | |
F13 | Ave | 5.14E+06 | 7.58E+04 | 1.54E+04 | 3.29E+06 | 1.07E+05 | 5.27E+06 | 2.56E+04 | 1.94E+03 |
Std | 2.31E+07 | 3.94E+04 | 1.46E+04 | 1.58E+06 | 9.63E+04 | 1.81E+07 | 2.26E+04 | 3.34E+02 | |
F14 | Ave | 1.43E+05 | 1.34E+05 | 2.00E+03 | 2.06E+05 | 1.84E+05 | 3.20E+04 | 1.81E+04 | 1.45E+03 |
Std | 2.09E+05 | 1.24E+05 | 1.00E+03 | 1.17E+05 | 3.55E+05 | 3.88E+04 | 1.75E+04 | 8.55E+00 | |
F15 | Ave | 4.67E+05 | 2.42E+04 | 8.67E+03 | 5.30E+05 | 1.72E+04 | 2.32E+04 | 1.15E+04 | 1.60E+03 |
Std | 1.12E+06 | 1.67E+04 | 7.31E+03 | 2.81E+05 | 1.52E+04 | 2.21E+04 | 1.17E+04 | 3.92E+01 | |
F16 | Ave | 2.46E+03 | 2.89E+03 | 2.76E+03 | 2.80E+03 | 2.79E+03 | 2.51E+03 | 2.23E+03 | 2.13E+03 |
Std | 2.44E+02 | 3.81E+02 | 3.02E+02 | 2.02E+02 | 4.31E+02 | 2.71E+02 | 2.44E+02 | 1.97E+02 | |
F17 | Ave | 2.00E+03 | 2.48E+03 | 2.26E+03 | 2.09E+03 | 2.19E+03 | 2.00E+03 | 1.87E+03 | 1.83E+03 |
Std | 1.56E+02 | 2.29E+02 | 2.27E+02 | 7.95E+01 | 1.98E+02 | 1.74E+02 | 1.14E+02 | 7.07E+01 | |
F18 | Ave | 1.23E+06 | 1.19E+06 | 4.10E+04 | 1.76E+06 | 1.38E+06 | 6.57E+05 | 3.17E+05 | 1.99E+03 |
Std | 1.19E+06 | 1.17E+06 | 2.43E+04 | 7.29E+05 | 1.94E+06 | 6.89E+05 | 2.54E+05 | 5.32E+01 | |
F19 | Ave | 4.85E+05 | 2.42E+04 | 6.04E+03 | 3.48E+05 | 1.70E+04 | 2.44E+04 | 1.70E+04 | 1.93E+03 |
Std | 5.64E+05 | 5.06E+04 | 5.81E+03 | 2.02E+05 | 1.85E+04 | 4.03E+04 | 1.87E+04 | 9.76E+00 | |
F20 | Ave | 2.35E+03 | 2.64E+03 | 2.47E+03 | 2.41E+03 | 2.58E+03 | 2.33E+03 | 2.22E+03 | 2.16E+03 |
Std | 1.30E+02 | 2.56E+02 | 1.85E+02 | 1.04E+02 | 2.07E+02 | 1.32E+02 | 9.27E+01 | 7.40E+01 | |
F21 | Ave | 2.39E+03 | 2.50E+03 | 2.41E+03 | 2.48E+03 | 2.41E+03 | 2.38E+03 | 2.36E+03 | 2.35E+03 |
Std | 2.55E+01 | 4.99E+01 | 7.71E+01 | 1.04E+01 | 3.25E+01 | 2.54E+01 | 1.73E+01 | 1.16E+01 | |
F22 | Ave | 4.22E+03 | 6.13E+03 | 2.69E+03 | 4.97E+03 | 2.97E+03 | 4.01E+03 | 2.52E+03 | 2.55E+03 |
Std | 1.90E+03 | 2.00E+03 | 1.22E+03 | 6.54E+02 | 1.48E+03 | 1.65E+03 | 8.57E+02 | 9.48E+02 | |
F23 | Ave | 2.75E+03 | 2.96E+03 | 2.83E+03 | 2.83E+03 | 2.79E+03 | 2.83E+03 | 2.70E+03 | 2.71E+03 |
Std | 4.36E+01 | 7.30E+01 | 6.56E+01 | 1.14E+01 | 3.94E+01 | 6.25E+01 | 1.36E+01 | 1.84E+01 | |
F24 | Ave | 2.93E+03 | 3.11E+03 | 3.01E+03 | 3.03E+03 | 2.95E+03 | 2.99E+03 | 2.87E+03 | 2.88E+03 |
Std | 6.71E+01 | 8.18E+01 | 6.85E+01 | 9.70E+00 | 4.15E+01 | 5.85E+01 | 1.40E+01 | 1.93E+01 | |
F25 | Ave | 2.96E+03 | 2.91E+03 | 2.91E+03 | 2.90E+03 | 2.92E+03 | 2.92E+03 | 2.89E+03 | 2.89E+03 |
Std | 1.94E+01 | 3.02E+01 | 2.04E+01 | 3.13E+00 | 2.22E+01 | 5.43E+01 | 1.53E+01 | 1.02E+01 | |
F26 | Ave | 4.54E+03 | 6.51E+03 | 4.78E+03 | 5.46E+03 | 5.60E+03 | 4.69E+03 | 3.99E+03 | 3.98E+03 |
Std | 3.24E+02 | 8.85E+02 | 1.60E+03 | 1.10E+02 | 1.47E+03 | 4.10E+02 | 5.79E+02 | 7.09E+02 | |
F27 | Ave | 3.24E+03 | 3.27E+03 | 3.29E+03 | 3.23E+03 | 3.25E+03 | 3.25E+03 | 3.21E+03 | 3.21E+03 |
Std | 1.91E+01 | 4.26E+01 | 4.77E+01 | 3.53E+00 | 2.63E+01 | 3.60E+01 | 9.44E+00 | 1.10E+01 | |
F28 | Ave | 3.37E+03 | 3.26E+03 | 3.23E+03 | 3.30E+03 | 3.26E+03 | 3.32E+03 | 3.22E+03 | 3.22E+03 |
Std | 6.42E+01 | 2.76E+01 | 2.11E+01 | 1.67E+01 | 3.31E+01 | 1.28E+02 | 2.11E+01 | 2.01E+01 | |
F29 | Ave | 3.77E+03 | 4.31E+03 | 4.16E+03 | 4.07E+03 | 3.96E+03 | 3.67E+03 | 3.49E+03 | 3.52E+03 |
Std | 1.39E+02 | 2.55E+02 | 4.02E+02 | 1.20E+02 | 2.09E+02 | 1.65E+02 | 1.12E+02 | 9.41E+01 | |
F30 | Ave | 9.71E+06 | 2.05E+05 | 1.37E+04 | 3.37E+05 | 1.96E+05 | 4.89E+04 | 2.39E+04 | 1.16E+04 |
Std | 6.16E+06 | 1.19E+05 | 6.95E+03 | 1.20E+05 | 2.06E+05 | 7.51E+04 | 2.09E+04 | 2.22E+03 | |
(W|T|L) | Ave | (20|0|0) | (20|0|0) | (20|0|0) | (20|0|0) | (20|0|0)) | (20|0|0) | (15|0|5) | |
Std | (20|0|0) | (20|0|0) | (20|0|0) | (12|0|8) | (20|0|0) | (20|0|0) | (20|0|0) |
Function | GWO | AVAO | GTO | DE | COA | PSO | SBOA | CSBOA | |
F1 | Ave | 3.23E+10 | 1.49E+08 | 1.39E+09 | 7.42E+09 | 2.58E+10 | 2.64E+10 | 2.17E+09 | 1.71E+09 |
Std | 7.93E+09 | 1.63E+08 | 1.26E+09 | 9.99E+08 | 8.15E+09 | 1.11E+10 | 1.80E+09 | 2.21E+09 | |
F3 | Ave | 3.54E+05 | 3.09E+05 | 1.68E+05 | 7.65E+05 | 6.21E+05 | 4.88E+05 | 2.59E+05 | 1.52E+05 |
Std | 3.95E+04 | 1.86E+04 | 2.15E+04 | 6.83E+04 | 9.67E+04 | 1.02E+05 | 2.50E+04 | 1.78E+04 | |
F4 | Ave | 3.02E+03 | 1.13E+03 | 1.38E+03 | 2.31E+03 | 3.02E+03 | 2.51E+03 | 1.14E+03 | 1.05E+03 |
Std | 8.11E+02 | 3.93E+02 | 1.83E+02 | 1.80E+02 | 7.87E+02 | 9.18E+02 | 1.19E+02 | 1.25E+02 | |
F5 | Ave | 1.14E+03 | 1.32E+03 | 1.34E+03 | 1.65E+03 | 1.42E+03 | 1.07E+03 | 1.04E+03 | 1.09E+03 |
Std | 1.04E+02 | 7.27E+01 | 5.05E+01 | 2.60E+01 | 3.38E+01 | 1.23E+02 | 6.25E+01 | 8.70E+01 | |
F6 | Ave | 6.32E+02 | 6.62E+02 | 6.62E+02 | 6.25E+02 | 6.68E+02 | 6.31E+02 | 6.26E+02 | 6.23E+02 |
Std | 4.44E+00 | 3.89E+00 | 4.71E+00 | 1.03E+00 | 1.94E+00 | 8.38E+00 | 5.90E+00 | 1.11E+01 | |
F7 | Ave | 1.87E+03 | 3.08E+03 | 2.89E+03 | 2.16E+03 | 3.18E+03 | 1.78E+03 | 1.92E+03 | 1.88E+03 |
Std | 1.38E+02 | 1.64E+02 | 2.12E+02 | 3.51E+01 | 2.82E+02 | 1.97E+02 | 1.21E+02 | 2.26E+02 | |
F8 | Ave | 1.44E+03 | 1.73E+03 | 1.70E+03 | 1.92E+03 | 1.90E+03 | 1.34E+03 | 1.37E+03 | 1.37E+03 |
Std | 1.09E+02 | 8.81E+01 | 1.01E+02 | 2.76E+01 | 5.28E+01 | 7.79E+01 | 6.87E+01 | 9.42E+01 | |
F9 | Ave | 3.37E+04 | 2.52E+04 | 2.45E+04 | 5.78E+04 | 3.99E+04 | 4.28E+04 | 2.10E+04 | 2.07E+04 |
Std | 9.40E+03 | 2.20E+03 | 2.31E+03 | 5.07E+03 | 9.91E+03 | 1.81E+04 | 3.13E+03 | 3.49E+03 | |
F10 | Ave | 1.72E+04 | 1.70E+04 | 1.72E+04 | 3.15E+04 | 2.22E+04 | 1.73E+04 | 1.62E+04 | 1.52E+04 |
Std | 4.32E+03 | 1.69E+03 | 2.31E+03 | 5.22E+02 | 2.78E+03 | 2.27E+03 | 1.35E+03 | 1.24E+03 | |
(W|T|L) | Ave | (9|0|0) | (8|0|1) | (8|0|1) | (9|0|0) | (9|0|0) | (7|0|2) | (7|0|2) | |
Std | (9|0|0) | (8|0|1) | (9|0|0) | (4|0|5) | (9|0|0) | (8|0|1) | (9|0|0) |
Function | GWO | AVAO | GTO | DE | COA | PSO | SBOA | CSBOA | |
F11 | Ave | 5.83E+04 | 3.30E+04 | 8.73E+03 | 2.32E+05 | 2.39E+05 | 2.88E+04 | 1.45E+04 | 4.64E+03 |
Std | 1.51E+04 | 8.89E+03 | 2.86E+03 | 2.95E+04 | 6.36E+04 | 8.29E+03 | 4.90E+03 | 9.91E+02 | |
F12 | Ave | 5.23E+09 | 2.73E+08 | 1.41E+08 | 5.44E+09 | 1.66E+09 | 8.98E+09 | 1.19E+08 | 7.16E+07 |
Std | 2.90E+09 | 1.34E+08 | 5.74E+07 | 5.88E+08 | 2.11E+09 | 6.09E+09 | 5.65E+07 | 3.34E+07 | |
F13 | Ave | 6.64E+08 | 8.03E+04 | 2.70E+04 | 1.61E+06 | 9.58E+06 | 9.76E+08 | 1.51E+04 | 9.33E+03 |
Std | 6.53E+08 | 2.99E+04 | 1.09E+04 | 1.47E+06 | 2.81E+07 | 1.41E+09 | 6.61E+03 | 8.33E+03 | |
F14 | Ave | 5.72E+06 | 3.40E+06 | 4.74E+05 | 3.48E+07 | 4.81E+06 | 3.63E+06 | 2.56E+06 | 7.00E+04 |
Std | 3.40E+06 | 1.84E+06 | 2.07E+05 | 8.23E+06 | 3.11E+06 | 2.39E+06 | 1.45E+06 | 7.12E+04 | |
F15 | Ave | 1.10E+08 | 6.39E+04 | 1.15E+04 | 4.84E+06 | 2.02E+05 | 3.20E+08 | 5.27E+03 | 6.93E+03 |
Std | 1.21E+08 | 2.91E+04 | 5.91E+03 | 3.02E+06 | 1.76E+05 | 5.03E+08 | 4.20E+03 | 6.48E+03 | |
F16 | Ave | 5.81E+03 | 6.62E+03 | 6.40E+03 | 1.11E+04 | 7.15E+03 | 6.00E+03 | 5.16E+03 | 5.16E+03 |
Std | 6.15E+02 | 8.47E+02 | 8.64E+02 | 2.39E+02 | 8.58E+02 | 7.22E+02 | 7.38E+02 | 6.78E+02 | |
F17 | Ave | 4.93E+03 | 6.07E+03 | 5.79E+03 | 7.74E+03 | 6.00E+03 | 6.12E+03 | 4.57E+03 | 4.46E+03 |
Std | 5.39E+02 | 5.95E+02 | 6.79E+02 | 2.37E+02 | 7.65E+02 | 1.15E+03 | 5.45E+02 | 4.77E+02 | |
F18 | Ave | 5.63E+06 | 3.77E+06 | 8.51E+05 | 6.95E+07 | 4.77E+06 | 8.01E+06 | 3.25E+06 | 2.54E+05 |
Std | 2.73E+06 | 2.01E+06 | 4.73E+05 | 1.79E+07 | 2.19E+06 | 5.02E+06 | 1.45E+06 | 1.26E+05 | |
F19 | Ave | 1.14E+08 | 3.35E+05 | 1.08E+04 | 7.41E+06 | 2.13E+06 | 1.78E+08 | 6.98E+03 | 8.21E+03 |
Std | 1.26E+08 | 2.00E+05 | 7.47E+03 | 4.91E+06 | 1.07E+06 | 2.94E+08 | 7.52E+03 | 9.33E+03 | |
F20 | Ave | 4.94E+03 | 5.98E+03 | 5.39E+03 | 7.51E+03 | 6.91E+03 | 5.26E+03 | 4.65E+03 | 4.29E+03 |
Std | 9.73E+02 | 5.55E+02 | 5.02E+02 | 2.76E+02 | 5.58E+02 | 6.72E+02 | 5.72E+02 | 5.12E+02 | |
F21 | Ave | 2.95E+03 | 3.54E+03 | 3.27E+03 | 3.47E+03 | 3.45E+03 | 3.04E+03 | 2.81E+03 | 2.86E+03 |
Std | 7.02E+01 | 1.42E+02 | 1.15E+02 | 3.18E+01 | 1.69E+02 | 1.01E+02 | 5.89E+01 | 8.82E+01 | |
F22 | Ave | 2.06E+04 | 2.03E+04 | 2.25E+04 | 3.39E+04 | 2.85E+04 | 1.96E+04 | 1.92E+04 | 1.85E+04 |
Std | 4.97E+03 | 1.48E+03 | 3.57E+03 | 5.13E+02 | 2.68E+03 | 2.03E+03 | 1.95E+03 | 1.32E+03 | |
F23 | Ave | 3.54E+03 | 4.09E+03 | 4.14E+03 | 3.79E+03 | 4.02E+03 | 4.30E+03 | 3.27E+03 | 3.24E+03 |
Std | 8.75E+01 | 1.64E+02 | 2.28E+02 | 2.88E+01 | 1.66E+02 | 2.29E+02 | 5.96E+01 | 7.85E+01 | |
F24 | Ave | 4.12E+03 | 5.08E+03 | 4.94E+03 | 4.33E+03 | 4.87E+03 | 5.26E+03 | 3.83E+03 | 3.87E+03 |
Std | 1.44E+02 | 2.97E+02 | 4.27E+02 | 3.06E+01 | 2.36E+02 | 4.11E+02 | 7.23E+01 | 8.76E+01 | |
F25 | Ave | 5.53E+03 | 3.75E+03 | 3.97E+03 | 6.69E+03 | 5.21E+03 | 4.47E+03 | 3.76E+03 | 3.74E+03 |
Std | 5.44E+02 | 8.51E+01 | 1.51E+02 | 3.70E+02 | 5.42E+02 | 7.75E+02 | 9.88E+01 | 1.22E+02 | |
F26 | Ave | 1.36E+04 | 2.37E+04 | 2.30E+04 | 1.68E+04 | 2.63E+04 | 1.89E+04 | 1.41E+04 | 1.33E+04 |
Std | 8.25E+02 | 2.17E+03 | 3.20E+03 | 2.86E+02 | 4.30E+03 | 3.77E+03 | 2.88E+03 | 1.61E+03 | |
F27 | Ave | 3.94E+03 | 4.12E+03 | 4.06E+03 | 4.36E+03 | 4.15E+03 | 4.00E+03 | 3.57E+03 | 3.50E+03 |
Std | 1.18E+02 | 2.91E+02 | 3.15E+02 | 9.78E+01 | 2.02E+02 | 2.92E+02 | 7.28E+01 | 7.00E+01 | |
F28 | Ave | 7.12E+03 | 4.01E+03 | 4.08E+03 | 1.29E+04 | 6.65E+03 | 6.55E+03 | 4.03E+03 | 3.80E+03 |
Std | 1.38E+03 | 1.40E+02 | 1.72E+02 | 8.70E+02 | 8.33E+02 | 1.89E+03 | 1.81E+02 | 1.19E+02 | |
F29 | Ave | 7.99E+03 | 8.57E+03 | 8.20E+03 | 1.03E+04 | 9.29E+03 | 7.48E+03 | 6.53E+03 | 6.45E+03 |
Std | 6.02E+02 | 6.07E+02 | 9.11E+02 | 2.17E+02 | 8.70E+02 | 6.33E+02 | 4.69E+02 | 3.67E+02 | |
F30 | Ave | 7.02E+08 | 8.35E+06 | 1.03E+06 | 1.50E+07 | 3.99E+07 | 1.12E+09 | 1.88E+05 | 5.82E+04 |
Std | 7.56E+08 | 4.85E+06 | 7.01E+05 | 3.11E+06 | 2.20E+07 | 1.00E+09 | 1.05E+05 | 3.13E+04 | |
(W|T|L) | Ave | (20|0|0) | (20|0|0) | (20|0|0) | (11|0|9) | (20|0|0) | (20|0|2) | (15|0|5) | |
Std | (20|0|0) | (19|0|1) | (19|0|1) | (16|0|14) | (20|0|0) | (20|0|0) | (13|0|7) |
Type | ID | CEC2022 function name | Dimension | fmin |
Unimodal | F1 | Shifted and full rotated Zakharov function | 10/20 | 300 |
Multimodal | F2 | Shifted and full rotated Rosenbrock's function | 10/20 | 400 |
F3 | Shifted and full rotated Rastrigin's function | 10/20 | 600 | |
F4 | Shifted and full rotated non-continuous Rastrigin's function | 10/20 | 800 | |
F5 | Shifted and full rotated levy function | 10/20 | 900 | |
Hybrid | F6 | Hybrid function 1 (N = 3) | 10/20 | 1800 |
F7 | Hybrid function 2 (N = 6) | 10/20 | 2000 | |
F8 | Hybrid function 3 (N = 5) | 10/20 | 2200 | |
Composition | F9 | Composition function 1 (N = 5) | 10/20 | 2300 |
F10 | Composition function 2 (N = 4) | 10/20 | 2400 | |
F11 | Composition function 3 (N = 5) | 10/20 | 2600 | |
F12 | Composition function 4 (N = 6) | 10/20 | 2700 | |
Search Range [−100,100]D |
Function | GWO | AVAO | GTO | DE | COA | PSO | SBOA | CSBOA | |
F1 | Ave | 1.10E+03 | 3.00E+02 | 3.00E+02 | 4.55E+03 | 1.01E+03 | 3.00E+02 | 3.00E+02 | 3.00E+02 |
Std | 1.35E+03 | 1.13E-07 | 4.31E-13 | 1.44E+03 | 6.70E+02 | 2.01E-06 | 5.46E-04 | 4.72E-14 | |
F2 | Ave | 4.23E+02 | 4.10E+02 | 4.05E+02 | 4.08E+02 | 4.06E+02 | 4.13E+02 | 4.05E+02 | 4.05E+02 |
Std | 2.34E+01 | 1.75E+01 | 3.12E+00 | 4.75E-01 | 3.30E+00 | 1.82E+01 | 3.71E+00 | 3.21E+00 | |
F3 | Ave | 6.01E+02 | 6.13E+02 | 6.03E+02 | 6.00E+02 | 6.03E+02 | 6.00E+02 | 6.00E+02 | 6.00E+02 |
Std | 8.63E-01 | 1.02E+01 | 3.89E+00 | 1.16E-07 | 5.46E+00 | 4.17E-01 | 2.60E-07 | 9.15E-06 | |
F4 | Ave | 8.13E+02 | 8.30E+02 | 8.22E+02 | 8.25E+02 | 8.32E+02 | 8.12E+02 | 8.10E+02 | 8.07E+02 |
Std | 7.78E+00 | 1.11E+01 | 7.75E+00 | 4.15E+00 | 5.72E+00 | 4.70E+00 | 4.60E+00 | 2.74E+00 | |
F5 | Ave | 9.05E+02 | 1.14E+03 | 9.16E+02 | 9.00E+02 | 9.33E+02 | 9.00E+02 | 9.00E+02 | 9.00E+02 |
Std | 1.45E+01 | 2.06E+02 | 1.44E+01 | 8.38E-02 | 1.06E+02 | 9.49E-02 | 3.70E-10 | 3.21E-13 | |
F6 | Ave | 6.35E+03 | 4.18E+03 | 1.88E+03 | 6.66E+03 | 4.57E+03 | 5.02E+03 | 3.76E+03 | 1.80E+03 |
Std | 2.30E+03 | 2.21E+03 | 6.15E+01 | 3.48E+03 | 2.22E+03 | 2.59E+03 | 1.60E+03 | 4.26E-01 | |
F7 | Ave | 2.03E+03 | 2.03E+03 | 2.02E+03 | 2.01E+03 | 2.02E+03 | 2.01E+03 | 2.01E+03 | 2.00E+03 |
Std | 1.01E+01 | 9.55E+00 | 1.07E+01 | 2.10E+00 | 8.42E+00 | 9.82E+00 | 9.51E+00 | 3.68E+00 | |
F8 | Ave | 2.22E+03 | 2.22E+03 | 2.22E+03 | 2.22E+03 | 2.23E+03 | 2.22E+03 | 2.21E+03 | 2.20E+03 |
Std | 6.43E+00 | 4.08E+00 | 5.86E+00 | 4.08E+00 | 5.18E+00 | 8.21E+00 | 9.57E+00 | 6.11E+00 | |
F9 | Ave | 2.55E+03 | 2.53E+03 | 2.53E+03 | 2.53E+03 | 2.53E+03 | 2.53E+03 | 2.53E+03 | 2.53E+03 |
Std | 3.17E+01 | 2.68E+01 | 1.41E-01 | 2.46E-12 | 4.35E-05 | 2.68E+01 | 8.44E-14 | 8.44E-14 | |
F10 | Ave | 2.53E+03 | 2.55E+03 | 2.51E+03 | 2.50E+03 | 2.53E+03 | 2.55E+03 | 2.51E+03 | 2.52E+03 |
Std | 5.21E+01 | 6.53E+01 | 3.01E+01 | 1.24E-01 | 5.12E+01 | 5.72E+01 | 3.68E+01 | 4.34E+01 | |
F11 | Ave | 2.92E+03 | 2.70E+03 | 2.62E+03 | 2.88E+03 | 2.77E+03 | 2.68E+03 | 2.75E+03 | 2.71E+03 |
Std | 8.73E+01 | 1.52E+02 | 7.71E+01 | 3.99E+01 | 1.35E+02 | 1.42E+02 | 1.55E+02 | 1.47E+02 | |
F12 | Ave | 2.86E+03 | 2.87E+03 | 2.86E+03 | 2.86E+03 | 2.86E+03 | 2.87E+03 | 2.86E+03 | 2.86E+03 |
Std | 1.71E+00 | 4.47E+00 | 1.75E+00 | 9.88E-01 | 1.64E+00 | 1.30E+01 | 1.68E+00 | 1.67E+00 | |
(W|T|L) | Ave | (12|0|0) | (12|0|0) | (10|0|2) | (11|0|1) | (12|0|0) | (12|0|0) | (10|2|0) | |
Std | (12|0|0) | (11|0|1) | (11|0|1) | (7|0|5) | (12|0|0) | (12|0|0) | (12|0|0) |
Function | GWO | AVAO | GTO | DE | COA | PSO | SBOA | CSBOA | |
F1 | Ave | 9.15E+03 | 3.50E+03 | 3.01E+02 | 3.63E+04 | 2.94E+04 | 1.11E+03 | 4.68E+02 | 3.00E+02 |
Std | 4.52E+03 | 2.47E+03 | 1.92E+00 | 6.83E+03 | 7.90E+03 | 3.35E+02 | 1.41E+02 | 1.06E-01 | |
F2 | Ave | 4.85E+02 | 4.62E+02 | 4.51E+02 | 4.49E+02 | 4.66E+02 | 4.59E+02 | 4.54E+02 | 4.45E+02 |
Std | 3.14E+01 | 2.28E+01 | 1.53E+01 | 1.42E-01 | 1.86E+01 | 1.89E+01 | 1.18E+01 | 1.79E+01 | |
F3 | Ave | 6.03E+02 | 6.38E+02 | 6.23E+02 | 6.00E+02 | 6.20E+02 | 6.01E+02 | 6.00E+02 | 6.00E+02 |
Std | 1.65E+00 | 1.02E+01 | 8.07E+00 | 1.48E-03 | 1.46E+01 | 1.30E+00 | 2.80E-03 | 6.13E-02 | |
F4 | Ave | 8.59E+02 | 8.93E+02 | 8.73E+02 | 9.16E+02 | 8.81E+02 | 8.42E+02 | 8.32E+02 | 8.34E+02 |
Std | 2.89E+01 | 2.49E+01 | 2.00E+01 | 6.54E+00 | 1.52E+01 | 1.57E+01 | 8.71E+00 | 1.18E+01 | |
F5 | Ave | 9.90E+02 | 2.37E+03 | 1.68E+03 | 1.07E+03 | 2.06E+03 | 9.23E+02 | 9.02E+02 | 9.00E+02 |
Std | 6.19E+01 | 3.56E+02 | 3.66E+02 | 4.88E+01 | 6.81E+02 | 3.83E+01 | 1.65E+00 | 8.08E-01 | |
F6 | Ave | 2.30E+06 | 6.21E+03 | 3.76E+03 | 3.84E+06 | 7.61E+03 | 5.85E+04 | 7.28E+03 | 1.86E+03 |
Std | 6.06E+06 | 6.03E+03 | 2.42E+03 | 1.48E+06 | 5.60E+03 | 2.70E+05 | 5.83E+03 | 2.39E+01 | |
F7 | Ave | 2.06E+03 | 2.16E+03 | 2.09E+03 | 2.05E+03 | 2.07E+03 | 2.04E+03 | 2.03E+03 | 2.02E+03 |
Std | 3.29E+01 | 5.51E+01 | 2.91E+01 | 7.37E+00 | 2.98E+01 | 1.98E+01 | 8.77E+00 | 5.18E+00 | |
F8 | Ave | 2.25E+03 | 2.24E+03 | 2.23E+03 | 2.23E+03 | 2.25E+03 | 2.27E+03 | 2.22E+03 | 2.22E+03 |
Std | 4.61E+01 | 2.32E+01 | 3.13E+01 | 1.52E+00 | 3.57E+01 | 6.61E+01 | 2.06E+00 | 9.52E-01 | |
F9 | Ave | 2.50E+03 | 2.48E+03 | 2.48E+03 | 2.48E+03 | 2.48E+03 | 2.50E+03 | 2.48E+03 | 2.48E+03 |
Std | 1.25E+01 | 7.91E-01 | 2.20E-02 | 3.34E-03 | 9.05E-02 | 2.77E+01 | 3.40E-05 | 3.70E-08 | |
F10 | Ave | 3.01E+03 | 3.01E+03 | 2.94E+03 | 2.52E+03 | 3.40E+03 | 2.74E+03 | 2.53E+03 | 2.50E+03 |
Std | 6.22E+02 | 6.28E+02 | 9.52E+02 | 9.19E+00 | 1.19E+03 | 3.43E+02 | 5.69E+01 | 5.72E+01 | |
F11 | Ave | 3.37E+03 | 2.94E+03 | 2.96E+03 | 2.90E+03 | 3.01E+03 | 2.90E+03 | 2.91E+03 | 2.90E+03 |
Std | 1.87E+02 | 4.98E+01 | 8.97E+01 | 2.48E-02 | 4.65E+02 | 8.49E-04 | 7.73E-04 | 1.83E+01 | |
F12 | Ave | 2.96E+03 | 2.98E+03 | 3.00E+03 | 2.95E+03 | 2.98E+03 | 2.98E+03 | 2.94E+03 | 2.94E+03 |
Std | 9.07E+00 | 3.01E+01 | 4.55E+01 | 1.87E+00 | 1.88E+01 | 3.22E+01 | 8.79E+00 | 6.48E+00 | |
(W|T|L) | Ave | (12|0|0) | (12|0|0) | (12|0|0) | (11|0|1) | (12|0|0) | (12|0|0) | (9|2|1) | |
Std | (12|0|0) | (12|0|0) | (12|0|0) | (7|0|5) | (12|0|0) | (12|0|0) | (11|0|1) |
Function | GWO | AVAO | GTO | DE | COA | PSO | SBOA |
F1 | 3.02E-11 | 2.77E-01 | 8.77E-02 | 3.02E-11 | 3.02E-11 | 4.08E-05 | 1.17E-03 |
F3 | 3.02E-11 | 3.02E-11 | 1.95E-03 | 3.02E-11 | 3.02E-11 | 3.02E-11 | 6.70E-11 |
F4 | 2.61E-10 | 1.17E-02 | 1.09E-01 | 1.78E-04 | 1.16E-07 | 5.09E-06 | 6.15E-02 |
F5 | 2.39E-04 | 3.02E-11 | 3.69E-11 | 3.02E-11 | 3.34E-11 | 6.79E-02 | 9.71E-01 |
F6 | 3.02E-11 | 3.02E-11 | 3.02E-11 | 2.53E-04 | 3.02E-11 | 3.47E-10 | 1.26E-01 |
F7 | 1.25E-05 | 3.02E-11 | 3.02E-11 | 2.37E-10 | 4.08E-11 | 1.58E-01 | 2.01E-01 |
F8 | 3.51E-02 | 5.49E-11 | 6.07E-11 | 3.02E-11 | 5.49E-11 | 8.88E-01 | 2.89E-03 |
F9 | 5.49E-11 | 3.02E-11 | 3.02E-11 | 3.02E-11 | 3.02E-11 | 1.41E-04 | 8.77E-02 |
F10 | 3.56E-04 | 6.70E-11 | 1.41E-09 | 3.02E-11 | 3.34E-11 | 3.83E-06 | 3.27E-02 |
F11 | 3.02E-11 | 2.44E-09 | 3.50E-09 | 3.02E-11 | 3.02E-11 | 1.07E-09 | 2.39E-04 |
F12 | 3.02E-11 | 3.02E-11 | 2.25E-04 | 3.02E-11 | 3.02E-11 | 6.07E-11 | 1.70E-08 |
F13 | 3.02E-11 | 3.02E-11 | 3.34E-11 | 3.02E-11 | 3.02E-11 | 3.02E-11 | 2.03E-09 |
F14 | 3.02E-11 | 3.02E-11 | 3.02E-11 | 3.02E-11 | 3.02E-11 | 3.02E-11 | 3.02E-11 |
F15 | 3.02E-11 | 3.02E-11 | 3.34E-11 | 3.02E-11 | 3.02E-11 | 3.02E-11 | 3.02E-11 |
F16 | 2.15E-06 | 8.15E-11 | 2.87E-10 | 1.96E-10 | 1.10E-08 | 4.44E-07 | 6.57E-02 |
F17 | 5.46E-06 | 3.69E-11 | 1.46E-10 | 8.15E-11 | 3.47E-10 | 2.00E-05 | 3.55E-01 |
F18 | 3.02E-11 | 3.02E-11 | 3.02E-11 | 3.02E-11 | 3.02E-11 | 3.02E-11 | 3.02E-11 |
F19 | 3.02E-11 | 3.02E-11 | 3.02E-11 | 3.02E-11 | 3.02E-11 | 3.02E-11 | 3.16E-10 |
F20 | 3.35E-08 | 4.98E-11 | 1.17E-09 | 2.37E-10 | 1.17E-09 | 3.01E-07 | 3.03E-02 |
F21 | 4.44E-07 | 3.02E-11 | 2.20E-07 | 3.02E-11 | 2.67E-09 | 3.18E-04 | 2.75E-03 |
F22 | 3.20E-09 | 5.00E-09 | 3.32E-06 | 7.12E-09 | 5.97E-09 | 6.52E-09 | 1.25E-04 |
F23 | 9.83E-08 | 3.02E-11 | 1.61E-10 | 3.02E-11 | 7.39E-11 | 4.08E-11 | 3.11E-01 |
F24 | 2.00E-05 | 3.02E-11 | 4.50E-11 | 3.02E-11 | 4.62E-10 | 6.70E-11 | 1.02E-01 |
F25 | 3.69E-11 | 4.42E-06 | 1.19E-06 | 1.07E-07 | 6.53E-08 | 2.15E-06 | 1.38E-02 |
F26 | 5.87E-04 | 4.62E-10 | 1.22E-02 | 3.02E-11 | 9.51E-06 | 5.86E-06 | 9.59E-01 |
F27 | 5.97E-09 | 6.70E-11 | 3.69E-11 | 8.10E-10 | 5.07E-10 | 7.12E-09 | 1.49E-01 |
F28 | 6.07E-11 | 2.57E-07 | 7.62E-03 | 3.02E-11 | 1.60E-07 | 1.07E-09 | 1.33E-01 |
F29 | 9.26E-09 | 3.02E-11 | 6.07E-11 | 3.34E-11 | 2.87E-10 | 1.11E-04 | 2.34E-01 |
F30 | 3.02E-11 | 3.02E-11 | 7.06E-01 | 3.02E-11 | 3.02E-11 | 3.16E-05 | 2.13E-04 |
Function | GWO | AVAO | GTO | DE | COA | PSO | SBOA |
F1 | 3.02E-11 | 9.83E-08 | 2.51E-02 | 1.17E-09 | 3.02E-11 | 3.02E-11 | 9.82E-01 |
F3 | 3.02E-11 | 3.02E-11 | 2.81E-02 | 3.02E-11 | 3.02E-11 | 3.02E-11 | 3.34E-11 |
F4 | 3.02E-11 | 1.16E-07 | 2.23E-09 | 3.02E-11 | 3.02E-11 | 3.02E-11 | 1.03E-06 |
F5 | 3.79E-01 | 6.07E-11 | 4.20E-10 | 3.02E-11 | 3.02E-11 | 3.27E-02 | 1.77E-03 |
F6 | 5.09E-08 | 3.02E-11 | 3.02E-11 | 1.00E-03 | 3.02E-11 | 1.43E-05 | 9.07E-03 |
F7 | 4.92E-01 | 3.02E-11 | 3.69E-11 | 7.66E-05 | 3.34E-11 | 5.94E-02 | 6.41E-01 |
F8 | 6.52E-01 | 3.02E-11 | 3.69E-11 | 3.02E-11 | 3.02E-11 | 6.38E-03 | 1.78E-04 |
F9 | 1.56E-02 | 5.53E-08 | 4.11E-07 | 3.02E-11 | 3.02E-11 | 3.35E-08 | 3.33E-01 |
F10 | 1.17E-03 | 1.78E-04 | 1.86E-03 | 3.02E-11 | 3.02E-11 | 2.88E-06 | 8.77E-02 |
F11 | 3.02E-11 | 3.02E-11 | 1.61E-10 | 3.02E-11 | 3.02E-11 | 3.02E-11 | 3.34E-11 |
F12 | 3.02E-11 | 2.67E-09 | 8.20E-07 | 3.02E-11 | 3.02E-11 | 3.02E-11 | 7.60E-07 |
F13 | 3.02E-11 | 6.72E-10 | 2.83E-08 | 1.09E-10 | 6.07E-11 | 3.69E-11 | 1.00E-03 |
F14 | 3.02E-11 | 3.02E-11 | 4.50E-11 | 3.02E-11 | 3.02E-11 | 3.02E-11 | 3.02E-11 |
F15 | 3.02E-11 | 3.02E-11 | 1.17E-04 | 3.02E-11 | 3.02E-11 | 6.70E-11 | 4.64E-01 |
F16 | 1.25E-05 | 1.46E-10 | 1.07E-07 | 3.02E-11 | 1.55E-09 | 1.04E-04 | 4.64E-01 |
F17 | 2.05E-03 | 1.46E-10 | 1.69E-09 | 3.02E-11 | 1.46E-10 | 5.00E-09 | 1.44E-02 |
F18 | 3.02E-11 | 3.02E-11 | 1.56E-08 | 3.02E-11 | 3.02E-11 | 3.02E-11 | 6.07E-11 |
F19 | 3.02E-11 | 3.34E-11 | 1.00E-03 | 3.02E-11 | 3.02E-11 | 4.98E-11 | 6.73E-01 |
F20 | 3.18E-03 | 2.03E-09 | 2.38E-07 | 3.02E-11 | 2.37E-10 | 8.12E-04 | 7.28E-01 |
F21 | 2.68E-04 | 3.02E-11 | 3.02E-11 | 3.02E-11 | 3.02E-11 | 5.49E-11 | 7.73E-02 |
F22 | 2.61E-02 | 8.88E-06 | 7.12E-09 | 3.02E-11 | 3.02E-11 | 8.29E-06 | 1.81E-01 |
F23 | 3.02E-11 | 3.02E-11 | 3.02E-11 | 3.02E-11 | 3.02E-11 | 3.02E-11 | 3.99E-04 |
F24 | 6.12E-10 | 3.02E-11 | 3.02E-11 | 3.02E-11 | 3.02E-11 | 3.02E-11 | 5.79E-01 |
F25 | 3.02E-11 | 2.34E-01 | 2.57E-07 | 3.02E-11 | 3.02E-11 | 5.07E-10 | 7.01E-02 |
F26 | 3.55E-01 | 2.23E-09 | 4.20E-10 | 1.11E-06 | 3.69E-11 | 4.11E-07 | 3.79E-01 |
F27 | 6.07E-11 | 6.07E-11 | 4.50E-11 | 3.02E-11 | 4.08E-11 | 3.82E-10 | 2.51E-02 |
F28 | 3.02E-11 | 1.61E-06 | 2.19E-08 | 3.02E-11 | 3.02E-11 | 3.69E-11 | 8.35E-08 |
F29 | 6.12E-10 | 2.61E-10 | 1.46E-10 | 3.02E-11 | 4.50E-11 | 8.56E-04 | 7.39E-01 |
F30 | 3.02E-11 | 3.02E-11 | 3.02E-11 | 3.02E-11 | 3.02E-11 | 3.02E-11 | 1.17E-09 |
Function | GWO | AVAO | GTO | DE | COA | PSO | SBOA |
F1 | 1.45E-11 | 1.45E-11 | 5.17E-07 | 1.45E-11 | 1.45E-11 | 1.45E-11 | 1.45E-11 |
F2 | 1.69E-09 | 1.31E-01 | 1.68E-01 | 7.64E-05 | 1.25E-04 | 2.61E-04 | 3.10E-02 |
F3 | 1.26E-11 | 1.26E-11 | 1.26E-11 | 6.83E-03 | 1.91E-11 | 1.17E-03 | 5.43E-03 |
F4 | 3.00E-07 | 3.31E-11 | 4.55E-10 | 4.05E-11 | 4.40E-11 | 3.30E-03 | 5.54E-03 |
F5 | 3.35E-11 | 2.47E-11 | 4.68E-10 | 4.09E-11 | 5.00E-11 | 1.45E-01 | 2.74E-06 |
F6 | 3.02E-11 | 3.02E-11 | 3.02E-11 | 3.02E-11 | 3.02E-11 | 3.02E-11 | 3.02E-11 |
F7 | 2.87E-10 | 7.39E-11 | 9.92E-11 | 3.32E-06 | 2.03E-09 | 3.57E-06 | 3.50E-03 |
F8 | 4.98E-11 | 4.08E-11 | 1.61E-10 | 1.07E-09 | 1.96E-10 | 6.53E-08 | 2.16E-03 |
F9 | 1.21E-12 | 5.42E-09 | 3.34E-01 | 3.12E-04 | 1.21E-12 | 3.34E-01 | NAN |
F10 | 3.96E-08 | 2.67E-09 | 1.60E-07 | 8.48E-09 | 3.20E-09 | 3.96E-08 | 8.31E-03 |
F11 | 2.98E-09 | 2.95E-05 | 1.29E-01 | 3.90E-11 | 1.46E-07 | 4.87E-04 | 1.10E-05 |
F12 | 3.01E-11 | 8.94E-11 | 1.10E-09 | 2.15E-03 | 6.70E-10 | 4.46E-09 | 8.76E-02 |
Function | GWO | AVAO | GTO | DE | COA | PSO | SBOA |
F1 | 3.02E-11 | 3.02E-11 | 6.77E-05 | 3.02E-11 | 3.02E-11 | 3.02E-11 | 3.02E-11 |
F2 | 1.47E-07 | 7.30E-04 | 3.64E-02 | 1.95E-03 | 2.53E-04 | 1.76E-01 | 7.24E-02 |
F3 | 3.02E-11 | 3.02E-11 | 3.02E-11 | 1.11E-04 | 3.02E-11 | 3.20E-09 | 8.65E-01 |
F4 | 6.36E-05 | 4.50E-11 | 8.15E-11 | 3.02E-11 | 4.08E-11 | 1.86E-03 | 9.00E-01 |
F5 | 3.34E-11 | 3.02E-11 | 3.02E-11 | 3.02E-11 | 3.02E-11 | 1.70E-08 | 3.55E-01 |
F6 | 3.02E-11 | 3.02E-11 | 3.69E-11 | 3.02E-11 | 3.02E-11 | 3.02E-11 | 4.50E-11 |
F7 | 6.07E-11 | 3.02E-11 | 3.34E-11 | 3.02E-11 | 7.39E-11 | 2.49E-06 | 2.16E-03 |
F8 | 8.99E-11 | 6.70E-11 | 1.68E-04 | 3.02E-11 | 3.02E-11 | 1.29E-06 | 6.91E-04 |
F9 | 3.02E-11 | 3.02E-11 | 1.04E-04 | 3.02E-11 | 3.02E-11 | 3.00E-11 | 3.02E-11 |
F10 | 4.57E-09 | 1.69E-09 | 8.84E-07 | 1.19E-06 | 1.47E-07 | 8.20E-07 | 5.49E-01 |
F11 | 3.02E-11 | 7.12E-09 | 3.02E-11 | 3.02E-11 | 3.02E-11 | 3.02E-11 | 3.02E-11 |
F12 | 2.92E-09 | 1.09E-10 | 1.96E-10 | 1.87E-05 | 2.15E-10 | 1.55E-09 | 2.77E-01 |
Dimensions | 30 | 100 | |||
Algorithms | Mean rank | Overall rank | Mean rank | Overall rank | |
GWO | 5.43 | 5 | 5 | 5 | |
AVOA | 6.03 | 7 | 4.73 | 4 | |
GTO | 4.17 | 3 | 4.17 | 3 | |
DE | 6.17 | 8 | 6.67 | 8 | |
COA | 5.9 | 6 | 6.33 | 7 | |
PSO | 4.7 | 4 | 5.43 | 6 | |
SBOA | 2.23 | 2 | 2.23 | 2 | |
CSBOA | 1.37 | 1 | 1.43 | 1 |
Dimensions | 10 | 20 | |||
Algorithms | Mean rank | Overall rank | Mean rank | Overall rank | |
GWO | 6.25 | 8 | 5.91 | 6 | |
AVOA | 6.17 | 7 | 6.17 | 7 | |
GTO | 3.83 | 3 | 4.91 | 5 | |
DE | 4.33 | 4 | 4.17 | 3 | |
COA | 5.92 | 6 | 6.25 | 8 | |
PSO | 4.92 | 5 | 4.67 | 4 | |
SBOA | 2.79 | 2 | 2.25 | 2 | |
CSBOA | 1.79 | 1 | 1.67 | 1 |
Algorithm | Optimal values for variable | Optimal value | Ranking | |||
Ts | Th | R | L | |||
GWO | 0.785779 | 0.388407 | 40.702147 | 194.754644 | 5900.291027 | 4 |
AVOA | 0.989300 | 0.489012 | 51.259077 | 88.659593 | 6352.998707 | 8 |
GTO | 1.258847 | 0.622249 | 65.225232 | 10.000000 | 7319.000702 | 9 |
DE | 0.781083 | 0.386270 | 40.339766 | 200.000000 | 5914.483351 | 5 |
COA | 0.968743 | 0.480559 | 50.114317 | 97.637567 | 6319.415834 | 7 |
PSO | 0.973894 | 0.481396 | 50.460847 | 94.730452 | 6311.096260 | 5 |
QOCSOS | 0.778238 | 0.384893 | 40.322081 | 199.966711 | 5885.332774 | 2 |
SBOA | 0.779067 | 0.385093 | 40.3661670 | 199.352987 | 5886.870272 | 3 |
CSBOA | 0.778169 | 0.384649 | 40.319619 | 199.999999 | 5885.332773 | 1 |
Algorithm | Optimal values for variable | Optimal value | Ranking | ||
d | D | N | |||
GWO | 0.0523516599 | 0.372775760 | 10.415319625 | 0.012684229 | 4 |
AVOA | 0.050000000 | 0.317425416 | 14.027769769 | 0.012719054 | 6 |
GTO | 0.053325095 | 0.397367912 | 9.250854836 | 0.012712811 | 5 |
DE | 0.053135792 | 0.392469906 | 9.485223289 | 0.012726826 | 7 |
COA | 0.056774591 | 0.491795419 | 6.277155317 | 0.013121202 | 9 |
PSO | 0.054998195 | 0.441675285 | 7.622863618 | 0.012855954 | 8 |
QOCSOS | 0.055130816 | 0.444775663 | 7.544716912 | 0.012665282 | 2 |
SBOA | 0.052219133 | 0.369604350 | 10.571609914 | 0.012670312 | 3 |
CSBOA | 0.051700822 | 0.3570007342 | 11.272393937 | 0.012665235 | 1 |