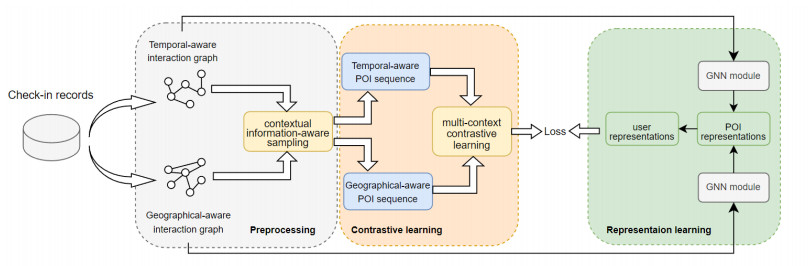
This paper focused on the point-of-interest (POI) recommendation task. Recently, graph representation learning-based POI recommendation models have gained significant attention due to the powerful modeling capacity of graph structural data. Despite their effectiveness, we have found that recent methods struggle to effectively utilize information from POIs that have not been checked in, which could limit their performance. Hence, in this paper, we proposed a new model, named the multi-contextual graph contrastive learning (MCGCL) model, which introduces the contrastive learning into graph representation learning-based methods. First, MCGCL extracts interactions between POIs under different contextual factors from user check-in records using predefined graph structure information. Next, it samples important POI sets from different contextual factors using a random walk-based method. Then, it introduces a new contrastive learning loss that incorporates contextual information into traditional contrastive learning to enhance its ability to capture contextual information. Finally, MCGCL employs a graph neural network (GNN) model to learn representations of users and POIs. Extensive experiments on real-world datasets have demonstrated the effectiveness of MCGCL on the POI recommendation task compared to representative POI recommendation approaches.
Citation: Xueping Han, Xueyong Wang. MCGCL: A multi-contextual graph contrastive learning-based approach for POI recommendation[J]. Electronic Research Archive, 2024, 32(5): 3618-3634. doi: 10.3934/era.2024166
[1] | Jing Chen, Weiyu Ye, Shaowei Kang . Learning user preferences from Multi-Contextual Sequence influences for next POI recommendation. Electronic Research Archive, 2024, 32(1): 486-504. doi: 10.3934/era.2024024 |
[2] | Xia Liu, Liwan Wu . FAGRec: Alleviating data sparsity in POI recommendations via the feature-aware graph learning. Electronic Research Archive, 2024, 32(4): 2728-2744. doi: 10.3934/era.2024123 |
[3] | Yunfei Tan, Shuyu Li, Zehua Li . A privacy preserving recommendation and fraud detection method based on graph convolution. Electronic Research Archive, 2023, 31(12): 7559-7577. doi: 10.3934/era.2023382 |
[4] | Shuwei Zhu, Hao Liu, Meiji Cui . Efficient multi-omics clustering with bipartite graph subspace learning for cancer subtype prediction. Electronic Research Archive, 2024, 32(11): 6008-6031. doi: 10.3934/era.2024279 |
[5] | Rui Wang, Haiqiang Li, Chen Hu, Xiao-Jun Wu, Yingfang Bao . Deep Grassmannian multiview subspace clustering with contrastive learning. Electronic Research Archive, 2024, 32(9): 5424-5450. doi: 10.3934/era.2024252 |
[6] | Hui Li, Rongrong Gong, Pengfei Hou, Libao Xing, Dongbao Jia, Haining Li . Online learning resources recommendation model based on improved NSGA-Ⅱ algorithm. Electronic Research Archive, 2023, 31(5): 3030-3049. doi: 10.3934/era.2023153 |
[7] | Zhiyuan Feng, Kai Qi, Bin Shi, Hao Mei, Qinghua Zheng, Hua Wei . Deep evidential learning in diffusion convolutional recurrent neural network. Electronic Research Archive, 2023, 31(4): 2252-2264. doi: 10.3934/era.2023115 |
[8] | Jicheng Li, Beibei Liu, Hao-Tian Wu, Yongjian Hu, Chang-Tsun Li . Jointly learning and training: using style diversification to improve domain generalization for deepfake detection. Electronic Research Archive, 2024, 32(3): 1973-1997. doi: 10.3934/era.2024090 |
[9] | Chuang Ma, Helong Xia . A one-step graph clustering method on heterogeneous graphs via variational graph embedding. Electronic Research Archive, 2024, 32(4): 2772-2788. doi: 10.3934/era.2024125 |
[10] | Bingjie Zhang, Junchao Yu, Zhe Kang, Tianyu Wei, Xiaoyu Liu, Suhua Wang . An adaptive preference retention collaborative filtering algorithm based on graph convolutional method. Electronic Research Archive, 2023, 31(2): 793-811. doi: 10.3934/era.2023040 |
This paper focused on the point-of-interest (POI) recommendation task. Recently, graph representation learning-based POI recommendation models have gained significant attention due to the powerful modeling capacity of graph structural data. Despite their effectiveness, we have found that recent methods struggle to effectively utilize information from POIs that have not been checked in, which could limit their performance. Hence, in this paper, we proposed a new model, named the multi-contextual graph contrastive learning (MCGCL) model, which introduces the contrastive learning into graph representation learning-based methods. First, MCGCL extracts interactions between POIs under different contextual factors from user check-in records using predefined graph structure information. Next, it samples important POI sets from different contextual factors using a random walk-based method. Then, it introduces a new contrastive learning loss that incorporates contextual information into traditional contrastive learning to enhance its ability to capture contextual information. Finally, MCGCL employs a graph neural network (GNN) model to learn representations of users and POIs. Extensive experiments on real-world datasets have demonstrated the effectiveness of MCGCL on the POI recommendation task compared to representative POI recommendation approaches.
Location-based social networks are widely prevalent in real-world applications, such as platforms like Yelp for food check-ins and Foursquare for social sharing. As one of the essential services in location-based social networks, POI recommendation [1,2] aims to suggest unvisited POIs that users may have the interest to visit, bringing significant economic benefits to various social platforms. To further enhance the quality of service provided by social platforms to users, researchers have been studying how to improve the accuracy of POI recommendation models in recent years.
Early POI recommendation models [3,4,5,6] were based on methods like matrix factorization, collaborative filtering, or topic modeling. Although these methods can intuitively model the interactions between users and POIs, they are limited by the sparsity of data in POI recommendation scenarios, leaving much room for improvement in performance.
In recent years, recommendation models based on graph representation learning [7,8,9] have gained significant attention from researchers. The core idea of these methods is to transform user check-in records into graph-structured data and enhance the model's ability to mine user preferences [10,11] through the relationships between nodes in the graph.
Despite their effectiveness, we find that such methods struggle to effectively utilize information from POIs that have not been checked in. Previous studies have shown that, in the field of POI recommendation, each user checks in to only a few POIs, leaving a large number unchecked. Fully leveraging the information from these unchecked POIs can help improve the model's ability to capture user preferences. However, existing graph-based representation learning methods struggle to exploit this aspect. On the other hand, in the computer vision field, contrastive learning methods are widely used to improve the generalization of models. By introducing contrastive loss regarding positive and negative samples, models can effectively learn target data features from limited data. Inspired by this, we have introduced contrastive learning into graph-based representation learning models in this paper.
In this paper, we propose a new model, named the multi-contextual graph contrastive learning (MCGCL) model, which consists of three core modules. First, the contextual information-aware sampling allows MCGCL to collect key nodes corresponding to each POI in different context scenarios, leveraging the defined graph structure data. Second, the contrastive loss based on context information enhances the model's ability to capture context information by incorporating the context information's influence into the original contrastive loss, based on the sampling results in different context environments. Finally, the representation learning module based on graph representation learning utilizes GNN to learn preference representations of POIs and users for the final recommendation task. We conducted extensive experiments on datasets from real scenarios. The results demonstrate that, compared to representative methods, MCGCL performs superiorly in the POI recommendation task.
The contributions of this paper are summarized as follows:
● We develop a context-aware sampling operation to construct the input sequences containing related POIs based on different contextual information for the target POIs.
● We propose a new POI recommendation method called MCGCL, which introduces multi-level contrastive learning by constructing two-level positive and negative POI sequences to comprehensively leverage the constructed POI sequences to learn distinguishable representations of POIs.
● MCGCL develops a graph attention-based module to adaptively learn final representations of POIs from different contextual information and leverage a time-delay strategy to estimate user preferences.
● We conduct extensive experiments on real-world datasets to validate the effectiveness of the proposed method for the POI recommendation task, compared to recent representative methods.
In this section, we provide a comprehensive review of recent studies from two perspectives: POI recommendation and contrastive learning recommendation.
POI recommendation [3], one of the most important tasks in location-based social services, has attracted great attention in the recent decade. In the past decade, many approaches involving matrix factorization-based [4], topic model-based [5], and graph representation learning-based [6] methods have been developed to improve the performance for the POI recommendation. Here, we briefly review recent efforts for this task.
Wang et al. [4] introduce a novel successive POI recommendation method named SQPMF, a novel POI recommendation method that integrates user preferences, social relationships, and POI transition data for more precise recommendations. Ji et al. [5] propose a social period-aware topic model (SPATM), which automatically learns the influence weights of user interests and social preferences for each check-in time to address the issue in distinguishing between individual user interests and social preferences. Wang et al. [7] propose the intent-aware graph neural network-based model (IAGNN), which first represents user check-in sequences as graphs and utilizes graph neural networks to learn POI embeddings. Gan et al. [8] introduce a unique hypersphere interest model for modeling user preferences. Qin et al. [9] propose a disentangled representation learning model called DIG, which employs a geo-constrained negative sampling strategy to find reliable negative samples for geographical and user interest factors, and a geo-enhanced soft-weighted loss function to balance these factors in loss computation. Cai et al. [12] propose a griends-aware graph collaborative filtering method (FG-CF) that incorporates social information into a user-POI graph by estimating a user-POI correlation matrix and updating user embeddings accordingly. Chen et al. [13] propose a novel POI recommendation method, deep navigator (DeNavi), which incorporates time and distance irregularities into learning sequential transitions. Lang et al. [14] propose a POI recommendation method based on a multiple bipartite graph network model (MBR) to make dynamic recommendations over time. Chang et al. [15] propose a multi-attention n etwork (MANC), which first utilizes a multi-attention network to learn the contextual influence of users and their friends and then employs a feature-level attention network to capture latent features of neighborhood POIs and a POI-level attention network to capture geographical influence among POIs. Zhang et al. [16] propose GNN-POI to leverage GNNs to learn node representations from node information and topological structure, improving recommendation accuracy. Christoforidis et al. [17] propose a new recommendation method with multiple network embeddings termed RELINE, a unified model that jointly learns user and POI dynamics. RELINE incorporates social, geographical, and temporal influences, as well as user preference dynamics by embedding eight relational graphs into one shared latent space.
Contrastive learning, whose goal is to learn distinct representations of input objects, has been widely used in the field of recommender systems [18], such as sequential-based recommendation [19] and session-based recommendation [20].
Yang et al. [21] propose a contrastive learning-based method called supervised contrastive learning (SCL), which employs self-supervised learning on the user-item graph to enhance the robustness of graph convolutional network (GCN) but highlights its shortcomings in fully addressing the specificity and uncertainty of user-item interactions. Wang et al. [22] propose a contrastive graph self-attention network for session-based recommendation which includes three distinct graph encoders to capture various item transition patterns and utilizes an attention-based fusion module to aggregate item representations related to the current session. Li et al. [23] propose a new contrastive learning method called dual-view co-contrastive learning (DCL), which aims to enhance multi-behavior recommendation by leveraging contrastive learning to mine additional supervision signals from raw data. Zhang et al. [24] propose contrastive learning with frequency domain for sequential recommendation (CLF4SRec), which introduces a learnable Fourier layer to provide frequency-based self-supervised signals. Xiao et al. [25] introduce a method based on a novel framework that combines graph contrastive embedding and multi-head cross-attention (GCE-MCAT), which combines graph contrastive embedding and multi-head cross-attention transfer to improve cross-domain recommendation. He et al. [26] propose a meta-path-based graph contrastive learning network (MPGCL) for learning more meaningful user and video embeddings, which uses a well-designed meta-path-based random walk strategy to create homogeneous graphs for user and video types, allowing better capture of heterogeneity. Ji et al. [27] develop a model-agnostic contrastive learning framework called ReACL, which leverages relationship homophily among data to achieve a uniform distribution for nodes and align relevant features to preserve personalized features. Tang et al. [28] discuss two issues with contrastive learning loss in recommender systems and propose a ranking-based contrastive loss (RCL) function to address these issues. Zhuang et al. [29] create a contrastive learning-based graph convolution network for social recommendation that integrates information from both social and interaction graphs.
Recently, there are also studies [30,31] that leverage contrastive learning to enhance the model performance in the POI recommendation task. The main difference between the proposed MCGCL method and previous studies is that MCGCL develops a contextual-aware contrastive learning framework that comprehensively leverages the information from different contextual feature spaces to develop the strategy of generating positive and negative sequences and designs a new contextual-aware loss function to fully preserve the influence of different contextual information.
This section introduces several notations and definitions used in this paper.
Given a location-based social network, we have the user set U={u1,…,un} and the POI set P={p1,…,pm}, where n and m are the number of users and POIs. A users' check-in record is represented as cu={p,l,t}, where l denotes the location information of the check-in record and t denotes the corresponding check-in timestamp. Given the above notations, we have the following definitions:
Definition 1 (Temporal-aware interaction graph). A temporal-aware interaction graph GT=(V,ET) describes the interactions between POIs under the temporal contextual information, where V denotes the node set containing all POIs and ET denotes the edge set. If two POIs are checked by the same user in the same timestamp or adjacent timestamps, there will be an edge between them in GT.
Definition 2 (Geographical-aware interaction graph). A geographical-aware interaction graph GL=(V,EL) describes the interactions between POIs under the geographical contextual information, where V denotes the node set containing all POIs and EL denotes the edge set. If the locations of two POIs are in the same or adjacent districts, there will be an edge between them inGL.
Definition 3 (POI recommendation task). Given all check-in records of a user Cu={c1u,…,cnuu}, the goal of the POI recommendation task is to generate the recommended POI list Ru={p1,…,pk}, where k is the length of the recommendation list.
This section introduces MCGCL in detail. Specifically, MCGCL contains three main stages: contextual information-aware sampling, multi-context contrastive learning, and recommendation score generation. Figure 1 shows the overall framework of the proposed method.
Different contextual information can reflect various associations between POIs. Effectively capturing the associations between POIs from different contextual information is key to enhancing POI recommendation services. In this paper, we construct two types of POI association graphs based on time and geography contextual information GTand GL. These two graphs depict the associations between different POIs from the perspectives of temporal and geographical contexts, respectively. The connectivity in the graphs indicates the strength of associations between POIs, where POIs with strong associations often appear in the vicinity of the target POI. Thus, the topological structure of the graph can express the importance of different POIs.
To capture the different interactions of POIs under different contextual scenarios, we propose a contextual information-aware sampling strategy. Specifically, after generating the context-aware graphs, we obtain the adjacency matrices associated with them.
Taking the temporal context aware graph GT as an example, its adjacency matrix is AT. Subsequently, we employ a personalized PageRank-based sampling method to sample associated POIs from GT:
s=α⋅Ms+(1−α)⋅q, | (1) |
where M is the transition matrix. In this paper, we utilize the normalized adjacency matrix to represent M=D−1AT. s denotes the personalized vector and q denotes the restart vector. α is the hyper-parameter to control the probability of the restart process. In this paper, we adopt the power iteration strategy to approximately calculate the values of the personalized vector s. The rationale of PageRank sampling is that the constructed contextual information-aware graphs preserve the relations between POIs in different contextual feature spaces. Hence, conducting a random walk-based sampling operation on the contextual-aware graph can effectively extract the related POIs in the corresponding contextual feature space for the target POI. Due to the popularity of PageRank sampling in the random walk-based approaches, we adopt this strategy to sample POIs from the constructed graph.
After running the personalized PageRank sampling operation, we obtain an n-dimensional vector sTv for the node v. The values in the vector reflect the degree of topological association between node v and other nodes in the graph. Therefore, to select highly associated nodes, we employ a top-k selection strategy. Specifically, we select the top nk nodes with the highest values in the vector sTvto construct the important node set for node v in the graph:
NTv={vi|vi∈Top(sTv)}, | (2) |
where Top(⋅) denotes the top-k selection strategy. Similar to GT, we can obtain the important node set NLv of the node v.
After constructing the important node set for each POI, we further use a contrastive learning approach to learn the representations of POIs. The key point of contrastive learning lies in the construction of negative sample sets. Different from traditional contrastive learning strategies, which directly consider POIs not appearing in the important node set as negative samples, we propose a multi-context contrastive learning strategy in this paper. Specifically, for each node v, we first extract the intersection of the two important node sets, NTvand NLv, to create a new node set NHv:
NHv={vi|vi∈NTvandvi∈NLv}. | (3) |
Intuitively, if a POI appears in both NTv and NLv, then this POI is considered to be one of the most important POIs to the target node. Then, for each POI vj in NHv, our proposed multi-context contrastive learning strategy is defined as follows:
Lmc=−logexp(Hv⋅Hvj/τ)∑vk∈NTvexp(Hv⋅Hvk/τ)−β⋅logexp(Hv⋅Hvj/τ)∑vk∈NLvexp(Hv⋅Hvk/τ), | (4) |
where H is the initial features of the POIs. In practice, we first utilize a linear layer to obtain the hidden representations of the POIs for model training. τ is the temperature coefficient and β is the trade-off weight.
Equation (4) indicates that we select the intersection of the POI lists sampled from temporal and geographical contexts as positive samples, while the remaining POIs are considered negative samples. The key idea of Eq (4) is to generate the positive POI sequences based on the constructed sequences from different contextual features. The rationale of Eq (4) is that if a POI appears simultaneously in two sequences, it will be more important compared to other POIs in the sampling sequences. The representation of the target POI should be more similar to these POIs than to others only appearing in one sequence. Additionally, we introduce weighted coefficients to control the influence of different contextual features on the representation of the POIs.
Moreover, we also introduce the standard loss function of contrastive learning, i.e., noise contrastive estimation, to leverage the information of other POIs to learn representations:
Lnc=−logexp(Hv⋅Hvj/τ)∑vk∉NTvexp(Hv⋅Hvk/τ). | (5) |
Equation (5) denotes the contrastive loss via the sampled NTv set. We can obtain the similar loss function via the NLv set. The rationale of Eq (5) is that POIs appearing in one sequence are more important to other POIs which have not been sampled. Hence, we consider these POIs in one sequence as positive samples and other POIs as negative samples in Eq (5). By combining Eqs (4) and (5), MCGCL established a two-level contrastive learning that comprehensively leverages the information of check-in records under different contextual information to learn distinguishable representations of POIs.
Estimating user preferences is also a key step in POI recommendation. Intuitively, user preferences are reflected by the POIs they have checked in. The representations of POIs are deeply related to contextual factors. Therefore, in this paper, we first calculate the representations of POIs through different contextual information, and then calculate the user preferences.
To calculate the representations of POIs from different contextual factors, we introduce graph neural networks to compute the representation of POIs from the constructed context-aware interaction graph. Take the temporal-aware interaction graph as an example. We use the following strategy to calculate the representations of POIs on GT:
HTP(l+1)=σ(ATHTP(l)W(l)), | (6) |
where HTP(l) denotes the representations of POIs learned from GT at the l-th neural network layer and HTP(0)=H are the initial features of the POIs. W(l) is the corresponding learnable parameter matrix. Similar to HTP, we can obtain the representations of POIs HLP from GL.
Considering that different contextual information has varying impacts on the representation of POIs, in this paper, we adopt a weighted aggregation method to compute the final representations of POIs from different contextual factors:
HP=γ⋅HTP+(1−γ)⋅HLP, | (7) |
where HP represents the final representations of the POIs and γ is the aggregation weight.
Finally, user preferences are calculated as follows:
HUu=∑(u,p)∈Cu1δ(t−tp)⋅HPp, | (8) |
where t,tp are the timestamps of the current time and the checked time.δ(⋅) denotes the sigmoid function.
After obtaining the user's preference features, we need to calculate the recommendation score between the user and the POIs. In this paper, we use the dot product strategy to calculate the recommendation score between the user and the POI:
Score(u,p)=HUu⋅HPpT. | (9) |
When generating a recommendation list for the user, we calculate the recommendation score between the user and all unvisited POIs, then sort them in descending order of the recommendation score, and recommend the top k POIs to the user.
As for the model training, the loss function of the proposed method contains two parts: recommendation-based loss and contrastive learning-based loss. In this paper, we adopt the widely used Bayesian personalized ranking (BPR) to calculate the recommendation-based loss:
LBPR=−∑(u,vi,vj)∈Dlnσ(Score(u,vi)−Score(u,vj)), | (10) |
where vi,vj represent the positive and negative samples, and D denotes the training set. The total loss function of the proposed method is defined as follows:
LMCGCL=LBPR+λ1⋅LMC+λ2⋅Lnc, | (11) |
where λ1 and λ2 are the regularization coefficients.
Here, we discuss the computational analysis of the proposed method. The computation of MCGCL could be divided into two parts: pre-processing and model training. In the pre-processing, MCGCL utilizes the PageRank-based approach to sample POIs, resulting in O(E) computational complexity, where E denotes the number of edges in the generated contextual graph. In the model training stage, MCGCL leverages the contrastive learning-based methods and GNN-based method to learn representations of POIs, resulting in O(nnk) + O(E) computational complexity, respectively. Here, we use nk to denote the total sampling size of POIs for simplified description.
In this section, we evaluate the performance of our proposed MCGCL through extensive experiments on real-world datasets. We start by discussing the experimental settings, including the datasets, baselines, and evaluation metrics, in Sections 5.1–5.3. Then, we compare the model performance of all methods on the POI recommendation task in Section 5.4. Finally, we investigate how the performance of MCGCL is affected by different design hyperparameters in Section 5.5.
We adopt two real-world datasets, Foursquare and Yelp, which are extracted from the famous location-based social networks. The Foursquare dataset covers check-ins within the United States from April 2012 to September 2013. We remove users with fewer than 20 check-in POIs and POIs with fewer than 20 visitors. The Yelp dataset comprises a large number of POIs and corresponding review information. Similarly, we filter out users with fewer than 20 check-ins and POIs with fewer than 20 visitors. Table 1 provides a summary of the statistics for these datasets.
Foursquare | Yelp | |
#Users | 24,941 | 30,887 |
#POIs | 28,593 | 18,995 |
#Records | 1,196,248 | 860,888 |
Sparsity | 99.83% | 99.85% |
We sort the check-in records of each dataset according to the check-in timestamps. Then, each dataset is divided into three subsets: training, validation, and test sets. Specifically, the earliest 60% of check-ins are regarded as the training set, while the most recent 20% are the test data. The remaining 20% are used as the validation set.
In this paper, we adopt the following representative approaches as baselines:
GeoSoCa [32] incorporates three types of influence—geographical, social, and categorical—to estimate the representations of POIs and users.
POI2Vec [33] employs a latent representation model that incorporates geographical influence, which uses a binary tree to cluster nearby POIs into the same region, enhancing their spatial influence.
STGCN [34] is an augmented long short-term memory (LSTM) spatiotemporal gating network, which introduces spatiotemporal gates to capture the relationships between POIs.
GPR [35] is a graph-based geographic latent representation model designed to capture highly nonlinear geographic influences from interactions between users and POIs.
GSTN [36] is a novel module that focuses on explicitly capturing complex geographical influences by utilizing graph embedding techniques.
ST-RNN [37] utilizes RNN to model a user's previous check-in sequence, which captures both spatial and temporal contexts using time and distance transition matrices.
SLS-REC [38] leverages the spatiotemporal Hawkes attention hypergraph neural network to capture users' dynamic preferences in different contextual feature spaces.
LTPM-TRSP [39] utilizes the temporal recency (TR) measure in the visits along with the location-aware recommendation based on spatial proximity (SP) to the user's location.
In this paper, we adopt two widely used evaluation metrics, Precision@k and Recall@k, to evaluate the performance of all methods on the POI recommendation task. The calculations of the above metrics are as follows:
Precision@k=1|Dtest|∑u∈Dtest|T(u)∩R(u)|k, | (12) |
Recall@k=1|Dtest|∑u∈Dtest|T(u)∩R(u)|T(u), | (13) |
where Dtest denotes the test set. T(u) denotes the POI set that the user u has visited. R(u) represents the recommendation POI set. k is the length of the recommendation list. In this paper, we vary k from 5 to 15.
In this subsection, we compare the performance of the proposed MCGCL with other POI recommendation methods on real-world datasets. Specifically, for each model, we conduct 10 runs on each dataset and report the average results. Tables 2–5 present all of the experimental results.
Foursquare | Precision@5 | Precision@10 | Precision@15 |
GeoSoCa POI2Vec ST-RNN STGCN GPR GSTN |
0.021 0.061 0.066 0.074 0.078 0.085 |
0.018 0.052 0.058 0.064 0.072 0.078 |
0.016 0.043 0.051 0.063 0.066 0.071 |
SLS-REC | 0.083 | 0.074 | 0.068 |
LTPM-TRSP | 0.069 | 0.061 | 0.059 |
MCGCL | 0.089 | 0.086 | 0.082 |
Foursquare | Recall@5 | Recall@10 | Recall@15 |
GeoSoCa POI2Vec ST-RNN STGCN GPR GSTN |
0.025 0.077 0.095 0.091 0.099 0.122 |
0.033 0.086 0.112 0.124 0.131 0.138 |
0.042 0.091 0.121 0.149 0.158 0.164 |
SLS-REC | 0.118 | 0.135 | 0.161 |
LTPM-TRSP | 0.092 | 0.119 | 0.128 |
MCGCL | 0.126 | 0.149 | 0.171 |
Yelp | Precision@5 | Precision@10 | Precision@15 |
GeoSoCa POI2Vec ST-RNN STGCN GPR GSTN |
0.016 0.054 0.056 0.065 0.105 0.098 |
0.015 0.046 0.051 0.061 0.092 0.082 |
0.013 0.041 0.046 0.054 0.077 0.074 |
SLS-REC | 0.101 | 0.084 | 0.075 |
LTPM-TRSP | 0.052 | 0.048 | 0.043 |
MCGCL | 0.108 | 0.095 | 0.083 |
Yelp | Recall@5 | Recall@10 | Recall@15 |
GeoSoCa POI2Vec ST-RNN STGCN GPR GSTN |
0.027 0.084 0.087 0.124 0.134 0.128 |
0.042 0.091 0.098 0.138 0.147 0.139 |
0.049 0.099 0.105 0.159 0.166 0.162 |
SLS-REC | 0.131 | 0.142 | 0.163 |
LTPM-TRSP | 0.079 | 0.095 | 0.102 |
MCGCL | 0.151 | 0.164 | 0.181 |
From the experimental results in the tables, it can be observed that MCGCL outperforms all baseline methods on two datasets, demonstrating the effectiveness of the proposed approach. Moreover, compared to other models utilizing graph representation learning methods, such as STGCN, GPR, SLS-REC, etc., MCGCL exhibits superior performance. This is attributed to the incorporation of a contrastive learning approach based on contextual factors in MCGCL, enabling it to effectively learn representations of POIs and users from the sparse check-in records. We can also observe that sequential model-based methods, such as POI2Vec and ST-RNN, obtain poor performance. This is because sequential model-based methods require successive check-in records to learn user preferences. However, these methods can suffer from the data sparsity issue, which further hurts the model performance.
Contextual information-aware contrastive learning is the key module of the proposed MCGCL. To validate the gain of this module on model performance, we develop a variant of MCGCL, called MCGCL-C. In this version, the contextual information-aware contrastive learning is removed. Only the BPR loss and the normal contrastive learning loss are preserved. We run this variant model on two datasets. The results are summarized in Tables 6–9.
Foursquare | Precision@5 | Precision@10 | Precision@15 |
MCGCL-C | 0.076 | 0.068 | 0.065 |
MCGCL | 0.089 | 0.086 | 0.082 |
Gain | +0.013 | +0.018 | +0.017 |
Foursquare | Recall@5 | Recall@10 | Recall@15 |
MCGCL-C | 0.095 | 0.128 | 0.152 |
MCGCL | 0.126 | 0.149 | 0.171 |
Gain | +0.031 | +0.021 | 0.019 |
Yelp | Precision@5 | Precision@10 | Precision@15 |
MCGCL-C | 0.092 | 0.078 | 0.069 |
MCGCL | 0.108 | 0.095 | 0.083 |
Gain | +0.016 | +0.017 | +0.014 |
Yelp | Recall@5 | Recall@10 | Recall@15 |
MCGCL-C | 0.122 | 0.134 | 0.159 |
MCGCL | 0.151 | 0.164 | 0.181 |
Gain | +0.029 | +0.030 | +0.022 |
The experimental results demonstrate that our proposed contextual information-aware contrastive learning model consistently improves the model performance across different datasets. This phenomenon indicates the effectiveness of our design in the POI recommendation scenario.
As mentioned before, learning the representations of POIs is the key step of POI recommendation. In this paper, we extract the representations of POIs from temporal and geographical factors. The value of γ is the key parameter to determine the final representations of POIs. Hence, we analyze the influence of γ on model performance. Specifically, we vary γ in {0,0.2,0.4,0.6,0.8,1} and observe the model performance. Figures 2 and 3 show the experimental results.
The graph clearly shows that the model performs poorly when the value of γ is at two endpoints. This suggests that learning the representation of POIs solely from one piece of contextual information is not accurate enough. The model achieves optimal performance when γ is set to 0.8, indicating that, on the Foursquare dataset, the temporal factor has a greater impact on the representation of POIs than the geographical factor.
The regularization coefficient weights λ1 and λ2 in Eq (11) control the contributions of different contrastive learning items to the final loss functions, further influencing the learning of representations of users and POIs. To explore the influence of coefficient weights on the model performance, we vary λ1 and λ2 in {0.5,1,1.5} and observe the changes in the model performance. The results are shown in Figures 4 and 5.
From Figures 4 and 5, we can observe that the model achieves the worst performance when two coefficient weights are set at 0.5. This situation indicates that the contrastive learning loss items are important for learning representations of POIs and users. Moreover, we can observe that the model achieves the best performance when λ1 and λ2 are 1.5 and 1. This situation means that the contextual-aware contrastive learning item is more important than the normal contrastive learning.
The sampling size k is also an important parameter that determines the model input. Here, we explore the influence of k on the model performance. Specifically, we vary k in {3,5,7,9,11} and evaluate the model performance. Table 10 reports the corresponding results.
k | 3 | 5 | 7 | 9 | 11 |
Precision@10 | 0.066 | 0.072 | 0.078 | 0.086 | 0.075 |
Recall@10 | 0.125 | 0.132 | 0.138 | 0.149 | 0.136 |
We can observe that the model performance first increases and then decreases with the increase of k. This situation indicates that increasing the size of the sampling sequence can effectively enhance the model performance. However, a large value of k can hurt the model performance since a long sampling sequence can involve several irrelevant POIs that affect the learning of the POIs' representations.
In this paper, we proposed a novel POI recommendation model called MCGCL. First, it extracts the interactions between POIs in different contextual factors from user check-in records using predefined graph structure information. Next, it samples important POI sets from different contextual factors using a random walk-based method. Then, MCGCL introduces a new contrastive learning loss, which incorporates contextual information into traditional contrastive learning to enhance its ability to capture contextual information. Finally, MCGCL employs a GNN model to learn representations of users and POIs. Extensive experiments on real-world datasets demonstrated the effectiveness of MCGCL on the POI recommendation task, compared to representative POI recommendation approaches.
The authors declare they have not used Artificial Intelligence (AI) tools in the creation of this article.
The authors declare that there are no conflicts of interest.
[1] |
Y. Hwangbo, K. J. Lee, B. Jeong, K. Y. Park, Recommendation system with minimized transaction data, Data Sci. Manage., 4 (2021), 40-45. https://doi.org/10.1016/j.dsm.2022.01.001 doi: 10.1016/j.dsm.2022.01.001
![]() |
[2] |
L. Shi, G. Song, G. Cheng, X. Liu, A user-based aggregation topic model for understanding user's preference and intention in social network, Neurocomputing, 413 (2020), 1-13. https://doi.org/10.1016/j.neucom.2020.06.099 doi: 10.1016/j.neucom.2020.06.099
![]() |
[3] |
W. Ji, X. Meng, Y. Zhang, STARec: Adaptive learning with spatiotemporal and activity influence for POI recommendation, ACM Trans. Inf. Syst., 40 (2021), 1-40. https://doi.org/10.1145/3485631 doi: 10.1145/3485631
![]() |
[4] |
J. Wang, Z. Huang, Z. Liu, SQPMF: successive point of interest recommendation system based on probability matrix factorization, Appl. Intell., 54 (2024), 680-700. https://doi.org/10.1007/s10489-023-05196-x doi: 10.1007/s10489-023-05196-x
![]() |
[5] |
W. Ji, X. Meng, Y. Zhang, SPATM: A social period-aware topic model for personalized venue recommendation, IEEE Trans. Knowl. Data Eng., 34 (2020), 3997-4010. https://doi.org/10.1109/TKDE.2020.3029070 doi: 10.1109/TKDE.2020.3029070
![]() |
[6] |
F. Mo, X. Fan, C. Chen, H. Yamana, Sampling-based epoch differentiation calibrated graph convolution network for point-of-interest recommendation, Neurocomputing, 571 (2024), 127140. https://doi.org/10.1016/j.neucom.2023.127140 doi: 10.1016/j.neucom.2023.127140
![]() |
[7] |
X. Wang, D. Wang, D. Yu, R. Wu, Q. Yang, S. Deng, et al., Intent-aware graph neural network for point-of-interest embedding and recommendation, Neurocomputing, 557 (2023), 126734. https://doi.org/10.1016/j.neucom.2023.126734 doi: 10.1016/j.neucom.2023.126734
![]() |
[8] |
M. Gan, Y. Ma, Mapping user interest into hyper-spherical space: a novel poi recommendation method, Inf. Process. Manage., 60 (2023), 103169. https://doi.org/10.1016/j.ipm.2022.103169 doi: 10.1016/j.ipm.2022.103169
![]() |
[9] |
Y. Qin, C. Gao, Y. Wang, S. Wei, D. Jin, J. Yuan, et al., Disentangling geographical effect for point-of-interest recommendation, IEEE Trans. Knowl. Data Eng., 35 (2023), 7883-7897. https://doi.org/10.1109/TKDE.2022.3221873 doi: 10.1109/TKDE.2022.3221873
![]() |
[10] |
L. Shi, J. Luo, C. Zhu, F. Kou, G. Cheng, X. Liu, A survey on cross-media search based on user intention understanding in social networks, Inf. Fusion, 91 (2023), 566-581. https://doi.org/10.1016/j.inffus.2022.11.017 doi: 10.1016/j.inffus.2022.11.017
![]() |
[11] |
L. Shi, J. P. Du, G. Cheng, X. Liu, Z. G. Xiong, J. Luo, Cross‐media search method based on complementary attention and generative adversarial network for social networks, Int. J. Intell. Syst., 37 (2022), 4393-4416. https://doi.org/10.1002/int.22723 doi: 10.1002/int.22723
![]() |
[12] |
Z. Cai, G. Yuan, S. Qiao, S. Qu, Y. Zhang, R. Bing, FG-CF: Friends-aware graph collaborative filtering for POI recommendation, Neurocomputing, 488 (2022), 107-119. https://doi.org/10.1016/j.neucom.2022.02.070 doi: 10.1016/j.neucom.2022.02.070
![]() |
[13] |
Y. C. Chen, T. Thaipisutikul, T. K. Shih, A learning-based POI recommendation with spatiotemporal context awareness, IEEE Trans. Cybern., 52 (2020), 2453-2466. https://doi.org/10.1109/TCYB.2020.3000733 doi: 10.1109/TCYB.2020.3000733
![]() |
[14] |
C. Lang, Z. Wang, K. He, S. Sun, POI recommendation based on a multiple bipartite graph network model, J. Supercomput., 78 (2022), 9782-9816. https://doi.org/10.1007/s11227-021-04279-1 doi: 10.1007/s11227-021-04279-1
![]() |
[15] |
L. Chang, W. Chen, J. Huang, C. Bin, W. Wang, Exploiting multi-attention network with contextual influence for point-of-interest recommendation, Appl. Intell., 51 (2021), 1904-1917. https://doi.org/10.1007/s10489-020-01868-0 doi: 10.1007/s10489-020-01868-0
![]() |
[16] |
J. Zhang, X. Liu, X. Zhou, X. Chu, Leveraging graph neural networks for point-of-interest recommendations, Neurocomputing, 462 (2021), 1-13. https://doi.org/10.1016/j.neucom.2021.07.063 doi: 10.1016/j.neucom.2021.07.063
![]() |
[17] | G. Christoforidis, P. Kefalas, A. N. Papadopoulos, Y. Manolopoulos, RELINE: point-of-interest recommendations using multiple network embeddings, Knowl. Inf. Syst., 63 (2021), 791-817. |
[18] | Y. Yang, Z. Wu, L. Wu, K. Zhang, R. Hong, Z. Zhang, et al., Generative-contrastive graph learning for recommendation, in Proceedings of the 46th International ACM SIGIR Conference on Research and Development in Information Retrieval, (2023), 1117-1126. https://doi.org/10.1145/3539618.3591691 |
[19] |
L. Guo, J. Zhang, L. Tang, T. Chen, L. Zhu, H. Yin, Time interval-enhanced graph neural network for shared-account cross-domain sequential recommendation, IEEE Trans. Neural Networks Learn. Syst., 35 (2024), 4002-4016. https://doi.org/10.1109/TNNLS.2022.3201533 doi: 10.1109/TNNLS.2022.3201533
![]() |
[20] |
R. Gao, Y. Tao, Y. Yu, J. Wu, X. Shao, J. Li, et al., Self-supervised dual hypergraph learning with intent disentanglement for session-based recommendation, Knowl. Based Syst., 270 (2023), 110528. https://doi.org/10.1016/j.knosys.2023.110528 doi: 10.1016/j.knosys.2023.110528
![]() |
[21] |
C. Yang, J. Zou, J. Wu, H. Xu, S. Fan, Supervised contrastive learning for recommendation, Knowl. Based Syst., 258 (2022), 109973. https://doi.org/10.1016/j.knosys.2022.109973 doi: 10.1016/j.knosys.2022.109973
![]() |
[22] |
F. Wang, X. Lu, L. Lyu, CGSNet: Contrastive graph self-attention network for session-based recommendation, Knowl. Based Syst., 251 (2022), 109282. https://doi.org/10.1016/j.knosys.2022.109282 doi: 10.1016/j.knosys.2022.109282
![]() |
[23] |
Q. Li, H. Ma, R. Zhang, W. Jin, Z. Li, Dual-view co-contrastive learning for multi-behavior recommendation, Appl. Intell., 53 (2023), 20134-20151. https://doi.org/10.1007/s10489-023-04495-7 doi: 10.1007/s10489-023-04495-7
![]() |
[24] |
Y. Zhang, G. Yin, Y. Dong, L. Zhang, Contrastive learning with frequency domain for sequential recommendation, Appl. Soft Comput., 144 (2023), 110481. https://doi.org/10.1016/j.asoc.2023.110481 doi: 10.1016/j.asoc.2023.110481
![]() |
[25] |
S. Xiao, D. Zhu, C. Tang, Z. Huang, Combining graph contrastive embedding and multi-head cross-attention transfer for cross-domain recommendation, Data Sci. Eng., 8 (2023), 247-262. https://doi.org/10.1007/s41019-023-00226-7 doi: 10.1007/s41019-023-00226-7
![]() |
[26] |
Y. He, G. Wu, D. Cai, X. Hu, Meta-path based graph contrastive learning for micro-video recommendation, Expert Syst. Appl., 222 (2023), 119713. https://doi.org/10.1016/j.eswa.2023.119713 doi: 10.1016/j.eswa.2023.119713
![]() |
[27] |
J. Ji, B. Zhang, J. Yu, X. Zhang, D. Qiu, B. Zhang, Relationship-aware contrastive learning for social recommendations, Inf. Sci., 629 (2023), 778-797. https://doi.org/10.1016/j.ins.2023.02.011 doi: 10.1016/j.ins.2023.02.011
![]() |
[28] |
H. Tang, G. Zhao, Y. He, Y. Wu, X. Qian, Ranking-based contrastive loss for recommendation systems, Knowl. Based Syst., 261 (2023), 110180. https://doi.org/10.1016/j.knosys.2022.110180 doi: 10.1016/j.knosys.2022.110180
![]() |
[29] |
J. Zhuang, S. Meng, J. Zhang, V. S. Sheng, Contrastive learning based graph convolution network for social recommendation, ACM Trans. Knowl. Discov. Data, 17 (2023), 1-21. https://doi.org/10.1145/3587268 doi: 10.1145/3587268
![]() |
[30] | Y. Qin, Y. Wang, F. Sun, W. Ju, X. Hou, Z. Wang, et al., DisenPOI: Disentangling sequential and geographical influence for point-of-interest recommendation, in Proceedings of the Sixteenth ACM International Conference on Web Search and Data Mining, (2023), 508-516. https://doi.org/10.1145/3539597.3570408 |
[31] | W. Ju, Y. Qin, Z. Qiao, X. Luo, Y. Wang, Y. Fu, et al., Kernel-based substructure exploration for next POI recommendation, in Proceedings of the 2022 IEEE International Conference on Data Mining, (2022), 221-230. https://doi.org/10.1109/ICDM54844.2022.00032 |
[32] | J. D. Zhang, C. Y. Chow, Geosoca: Exploiting geographical, social and categorical correlations for point-of-interest recommendations, in Proceedings of the 38th International ACM SIGIR Conference on Research and Development in Information Retrieval, (2015), 443-452. https://doi.org/10.1145/2766462.2767711 |
[33] | S. Feng, G. Cong, B. An, Y. M. Chee, Poi2vec: Geographical latent representation for predicting future visitors, in Proceedings of the AAAI Conference on Artificial Intelligence, (2017), 102-108. https://doi.org/10.1609/aaai.v31i1.10500 |
[34] |
P. Zhao, A. Luo, Y. Liu, J. Xu, Z. Li, F. Zhuang, et al., Where to go next: A spatio-temporal gated network for next poi recommendation, IEEE Trans. Knowl. Data Eng., 34 (2022), 2512-2524. https://doi.org/10.1109/TKDE.2020.3007194 doi: 10.1109/TKDE.2020.3007194
![]() |
[35] | B. Chang, G. Jang, S. Kim, J. Kang, Learning graph-based geographical latent representation for point-of-interest recommendation, in Proceedings of the 29th ACM International Conference on Information & Knowledge Management, (2020), 135-144. https://doi.org/10.1145/3340531.3411905 |
[36] |
Z. Wang, Y. Zhu, Q. Zhang, H. Liu, C. Wang, T. Liu, Graph-enhanced spatial-temporal network for next POI recommendation, ACM Trans. Knowl. Discov. Data, 16 (2022), 1-21. https://doi.org/10.1145/3513092 doi: 10.1145/3513092
![]() |
[37] | Q. Liu, S. Wu, L. Wang, T. Tan, Predicting the next location: A recurrent model with spatial and temporal contexts, in Proceedings of the AAAI Conference on Artificial Intelligence, (2016), 194-200. https://doi.org/10.1609/aaai.v30i1.9971 |
[38] |
J. Fu, R. Gao, Y. Yu, J. Wu, J. Li, D. Liu, et al., Contrastive graph learning long and short-term interests for POI recommendation, Expert Syst. Appl., 238 (2024), 121931. https://doi.org/10.1016/j.eswa.2023.121931 doi: 10.1016/j.eswa.2023.121931
![]() |
[39] |
M. Acharya, K. K. Mohbey, D. S. Rajput, Long-term preference mining with temporal and spatial fusion for point-of-interest recommendation, IEEE Access, 12 (2024), 11584-11596. https://doi.org/10.1109/ACCESS.2024.3354934 doi: 10.1109/ACCESS.2024.3354934
![]() |
Foursquare | Yelp | |
#Users | 24,941 | 30,887 |
#POIs | 28,593 | 18,995 |
#Records | 1,196,248 | 860,888 |
Sparsity | 99.83% | 99.85% |
Foursquare | Precision@5 | Precision@10 | Precision@15 |
GeoSoCa POI2Vec ST-RNN STGCN GPR GSTN |
0.021 0.061 0.066 0.074 0.078 0.085 |
0.018 0.052 0.058 0.064 0.072 0.078 |
0.016 0.043 0.051 0.063 0.066 0.071 |
SLS-REC | 0.083 | 0.074 | 0.068 |
LTPM-TRSP | 0.069 | 0.061 | 0.059 |
MCGCL | 0.089 | 0.086 | 0.082 |
Foursquare | Recall@5 | Recall@10 | Recall@15 |
GeoSoCa POI2Vec ST-RNN STGCN GPR GSTN |
0.025 0.077 0.095 0.091 0.099 0.122 |
0.033 0.086 0.112 0.124 0.131 0.138 |
0.042 0.091 0.121 0.149 0.158 0.164 |
SLS-REC | 0.118 | 0.135 | 0.161 |
LTPM-TRSP | 0.092 | 0.119 | 0.128 |
MCGCL | 0.126 | 0.149 | 0.171 |
Yelp | Precision@5 | Precision@10 | Precision@15 |
GeoSoCa POI2Vec ST-RNN STGCN GPR GSTN |
0.016 0.054 0.056 0.065 0.105 0.098 |
0.015 0.046 0.051 0.061 0.092 0.082 |
0.013 0.041 0.046 0.054 0.077 0.074 |
SLS-REC | 0.101 | 0.084 | 0.075 |
LTPM-TRSP | 0.052 | 0.048 | 0.043 |
MCGCL | 0.108 | 0.095 | 0.083 |
Yelp | Recall@5 | Recall@10 | Recall@15 |
GeoSoCa POI2Vec ST-RNN STGCN GPR GSTN |
0.027 0.084 0.087 0.124 0.134 0.128 |
0.042 0.091 0.098 0.138 0.147 0.139 |
0.049 0.099 0.105 0.159 0.166 0.162 |
SLS-REC | 0.131 | 0.142 | 0.163 |
LTPM-TRSP | 0.079 | 0.095 | 0.102 |
MCGCL | 0.151 | 0.164 | 0.181 |
Foursquare | Precision@5 | Precision@10 | Precision@15 |
MCGCL-C | 0.076 | 0.068 | 0.065 |
MCGCL | 0.089 | 0.086 | 0.082 |
Gain | +0.013 | +0.018 | +0.017 |
Foursquare | Recall@5 | Recall@10 | Recall@15 |
MCGCL-C | 0.095 | 0.128 | 0.152 |
MCGCL | 0.126 | 0.149 | 0.171 |
Gain | +0.031 | +0.021 | 0.019 |
Yelp | Precision@5 | Precision@10 | Precision@15 |
MCGCL-C | 0.092 | 0.078 | 0.069 |
MCGCL | 0.108 | 0.095 | 0.083 |
Gain | +0.016 | +0.017 | +0.014 |
Yelp | Recall@5 | Recall@10 | Recall@15 |
MCGCL-C | 0.122 | 0.134 | 0.159 |
MCGCL | 0.151 | 0.164 | 0.181 |
Gain | +0.029 | +0.030 | +0.022 |
k | 3 | 5 | 7 | 9 | 11 |
Precision@10 | 0.066 | 0.072 | 0.078 | 0.086 | 0.075 |
Recall@10 | 0.125 | 0.132 | 0.138 | 0.149 | 0.136 |
Foursquare | Yelp | |
#Users | 24,941 | 30,887 |
#POIs | 28,593 | 18,995 |
#Records | 1,196,248 | 860,888 |
Sparsity | 99.83% | 99.85% |
Foursquare | Precision@5 | Precision@10 | Precision@15 |
GeoSoCa POI2Vec ST-RNN STGCN GPR GSTN |
0.021 0.061 0.066 0.074 0.078 0.085 |
0.018 0.052 0.058 0.064 0.072 0.078 |
0.016 0.043 0.051 0.063 0.066 0.071 |
SLS-REC | 0.083 | 0.074 | 0.068 |
LTPM-TRSP | 0.069 | 0.061 | 0.059 |
MCGCL | 0.089 | 0.086 | 0.082 |
Foursquare | Recall@5 | Recall@10 | Recall@15 |
GeoSoCa POI2Vec ST-RNN STGCN GPR GSTN |
0.025 0.077 0.095 0.091 0.099 0.122 |
0.033 0.086 0.112 0.124 0.131 0.138 |
0.042 0.091 0.121 0.149 0.158 0.164 |
SLS-REC | 0.118 | 0.135 | 0.161 |
LTPM-TRSP | 0.092 | 0.119 | 0.128 |
MCGCL | 0.126 | 0.149 | 0.171 |
Yelp | Precision@5 | Precision@10 | Precision@15 |
GeoSoCa POI2Vec ST-RNN STGCN GPR GSTN |
0.016 0.054 0.056 0.065 0.105 0.098 |
0.015 0.046 0.051 0.061 0.092 0.082 |
0.013 0.041 0.046 0.054 0.077 0.074 |
SLS-REC | 0.101 | 0.084 | 0.075 |
LTPM-TRSP | 0.052 | 0.048 | 0.043 |
MCGCL | 0.108 | 0.095 | 0.083 |
Yelp | Recall@5 | Recall@10 | Recall@15 |
GeoSoCa POI2Vec ST-RNN STGCN GPR GSTN |
0.027 0.084 0.087 0.124 0.134 0.128 |
0.042 0.091 0.098 0.138 0.147 0.139 |
0.049 0.099 0.105 0.159 0.166 0.162 |
SLS-REC | 0.131 | 0.142 | 0.163 |
LTPM-TRSP | 0.079 | 0.095 | 0.102 |
MCGCL | 0.151 | 0.164 | 0.181 |
Foursquare | Precision@5 | Precision@10 | Precision@15 |
MCGCL-C | 0.076 | 0.068 | 0.065 |
MCGCL | 0.089 | 0.086 | 0.082 |
Gain | +0.013 | +0.018 | +0.017 |
Foursquare | Recall@5 | Recall@10 | Recall@15 |
MCGCL-C | 0.095 | 0.128 | 0.152 |
MCGCL | 0.126 | 0.149 | 0.171 |
Gain | +0.031 | +0.021 | 0.019 |
Yelp | Precision@5 | Precision@10 | Precision@15 |
MCGCL-C | 0.092 | 0.078 | 0.069 |
MCGCL | 0.108 | 0.095 | 0.083 |
Gain | +0.016 | +0.017 | +0.014 |
Yelp | Recall@5 | Recall@10 | Recall@15 |
MCGCL-C | 0.122 | 0.134 | 0.159 |
MCGCL | 0.151 | 0.164 | 0.181 |
Gain | +0.029 | +0.030 | +0.022 |
k | 3 | 5 | 7 | 9 | 11 |
Precision@10 | 0.066 | 0.072 | 0.078 | 0.086 | 0.075 |
Recall@10 | 0.125 | 0.132 | 0.138 | 0.149 | 0.136 |