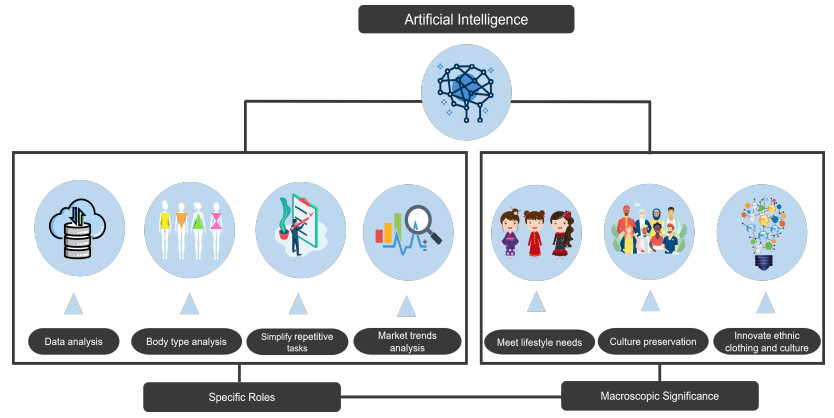
This study delves into the innovative application of Artificial Intelligence (AI) and machine learning algorithms in the realm of ethnic fashion design, with a specific emphasis on the Miao women's apparel. We introduce an AI-powered approach that strategically bridges modern technology with traditional elements, denoting a significant stride in the field of fashion design. Our research underscores three major aspects: customization of body shape, fabric selection, and innovative design. An AI-driven statistical methodology was utilized to accurately adapt to the unique body characteristics of Miao women, demonstrating an application of machine learning in pattern recognition. Furthermore, the AI's capacity to analyze the fabric properties was harnessed to optimize material selection, creating a balance between aesthetics and comfort. The innovative use of the Multimodal Unsupervised Image-to-Image Translation (MUNIT) algorithm, an AI tool, generated diverse and trendy designs, thereby enriching the distinctiveness of ethnic apparel. Our study accentuates the synergistic blend of traditional crafting methods and modern technological applications, highlighting the role of AI in the sustainable development of ethnic fashion. Additionally, we also demonstrate the advantages of Made-to-Measure (MTM) approaches, emphasizing the importance of individual customization in contemporary fashion design. This research presents a pioneering exploration at the nexus of AI, pattern recognition, and ethnic fashion design, which has the potential to transform the future of the fashion industry.
Citation: Meizhen Deng, Yimeng Liu, Ling Chen. AI-driven innovation in ethnic clothing design: an intersection of machine learning and cultural heritage[J]. Electronic Research Archive, 2023, 31(9): 5793-5814. doi: 10.3934/era.2023295
[1] | Mengke Lu, Shang Gao, Xibei Yang, Hualong Yu . Improving performance of decision threshold moving-based strategies by integrating density-based clustering technique. Electronic Research Archive, 2023, 31(5): 2501-2518. doi: 10.3934/era.2023127 |
[2] | Kai Huang, Chang Jiang, Pei Li, Ali Shan, Jian Wan, Wenhu Qin . A systematic framework for urban smart transportation towards traffic management and parking. Electronic Research Archive, 2022, 30(11): 4191-4208. doi: 10.3934/era.2022212 |
[3] | Ilyоs Abdullaev, Natalia Prodanova, Mohammed Altaf Ahmed, E. Laxmi Lydia, Bhanu Shrestha, Gyanendra Prasad Joshi, Woong Cho . Leveraging metaheuristics with artificial intelligence for customer churn prediction in telecom industries. Electronic Research Archive, 2023, 31(8): 4443-4458. doi: 10.3934/era.2023227 |
[4] | Dong-hyeon Kim, Se-woon Choe, Sung-Uk Zhang . Recognition of adherent polychaetes on oysters and scallops using Microsoft Azure Custom Vision. Electronic Research Archive, 2023, 31(3): 1691-1709. doi: 10.3934/era.2023088 |
[5] | Qing Tian, Heng Zhang, Shiyu Xia, Heng Xu, Chuang Ma . Cross-view learning with scatters and manifold exploitation in geodesic space. Electronic Research Archive, 2023, 31(9): 5425-5441. doi: 10.3934/era.2023275 |
[6] | Nihar Patel, Nakul Vasani, Nilesh Kumar Jadav, Rajesh Gupta, Sudeep Tanwar, Zdzislaw Polkowski, Fayez Alqahtani, Amr Gafar . F-LSTM: Federated learning-based LSTM framework for cryptocurrency price prediction. Electronic Research Archive, 2023, 31(10): 6525-6551. doi: 10.3934/era.2023330 |
[7] | Xiaoyan Wu, Guowen Ye, Yongming Liu, Zhuanzhe Zhao, Zhibo Liu, Yu Chen . Application of Improved Jellyfish Search algorithm in Rotate Vector reducer fault diagnosis. Electronic Research Archive, 2023, 31(8): 4882-4906. doi: 10.3934/era.2023250 |
[8] | Shizhen Huang, Enhao Tang, Shun Li, Xiangzhan Ping, Ruiqi Chen . Hardware-friendly compression and hardware acceleration for transformer: A survey. Electronic Research Archive, 2022, 30(10): 3755-3785. doi: 10.3934/era.2022192 |
[9] | Youqun Long, Jianhui Zhang, Gaoli Wang, Jie Fu . Hierarchical federated learning with global differential privacy. Electronic Research Archive, 2023, 31(7): 3741-3758. doi: 10.3934/era.2023190 |
[10] | Duhui Chang, Yan Geng . Distributed data-driven iterative learning control for multi-agent systems with unknown input-output coupled parameters. Electronic Research Archive, 2025, 33(2): 867-889. doi: 10.3934/era.2025039 |
This study delves into the innovative application of Artificial Intelligence (AI) and machine learning algorithms in the realm of ethnic fashion design, with a specific emphasis on the Miao women's apparel. We introduce an AI-powered approach that strategically bridges modern technology with traditional elements, denoting a significant stride in the field of fashion design. Our research underscores three major aspects: customization of body shape, fabric selection, and innovative design. An AI-driven statistical methodology was utilized to accurately adapt to the unique body characteristics of Miao women, demonstrating an application of machine learning in pattern recognition. Furthermore, the AI's capacity to analyze the fabric properties was harnessed to optimize material selection, creating a balance between aesthetics and comfort. The innovative use of the Multimodal Unsupervised Image-to-Image Translation (MUNIT) algorithm, an AI tool, generated diverse and trendy designs, thereby enriching the distinctiveness of ethnic apparel. Our study accentuates the synergistic blend of traditional crafting methods and modern technological applications, highlighting the role of AI in the sustainable development of ethnic fashion. Additionally, we also demonstrate the advantages of Made-to-Measure (MTM) approaches, emphasizing the importance of individual customization in contemporary fashion design. This research presents a pioneering exploration at the nexus of AI, pattern recognition, and ethnic fashion design, which has the potential to transform the future of the fashion industry.
Ethnic costumes serve as living examples of the inheritance and development of ethnic culture, reflecting the aesthetic concepts and lifestyle of ethnic groups throughout history [1]. The creative inheritance and innovative development of such costumes present a significant challenge that has long captivated various sectors. In response to these challenges, the Chinese government has shown explicit commitment since 2017, launching initiatives to promote the protection and dissemination of ethnic minority cultures and their distinctive traditional attire. Programs such as the Chinese Festival and the Etiquette Clothing Program have been implemented to design and produce a series of clothing that showcases the unique cultural charm of the Chinese nation [2].
However, previous research on ethnic clothing has been limited and primarily concentrated on exploring its cultural significance and identifying innovative paths for development in the new era. While the cultural interpretation and innovative inheritance of Miao ethnic clothing have been strongly emphasized, research on innovative design practices has been sparse [3]. It is precisely this type of research that aligns with the concept of "creative inheritance and innovative development".
Currently, the creative inheritance and innovative development of ethnic costumes face new challenges in our contemporary society. Traditional garments, which are predominantly designed in black, no longer meet the aesthetic preferences and dressing requirements of modern individuals [4]. Furthermore, the practicality and material selection of traditional ensembles do not align with the diverse fashion aesthetics prevalent today [5]. Moreover, even in geographically remote villages, the instantaneous and widespread connectivity of the internet, coupled with the sustained development of the tourism industry, has exposed people to a myriad of colorful clothing, breaking away from the previous monotony. Furthermore, the impacts of industrialization [6] and urbanization have resulted in increased foreign cultural influences on the Miao culture. The yearning for external cultures and the pursuit of economic interests have led an increasing number of laborers from Miao-inhabited areas to leave their hometowns to either study or work in cities. Consequently, they have gradually abandoned their long-standing practice of wearing Miao ethnic costumes, reserving them primarily for special occasions such as ethnic festivals, weddings, and funerals [7]. This phenomenon of "de-ethnicization" [8] is not unique to the Miao people, and can also be observed among other ethnic groups.
The traditional costumes of the Biasha Miao ethnic minority have encountered challenges in meeting the aesthetic needs of contemporary women, particularly in terms of color matching, body modification, and practicality [9]. Body shape is a reflection of an individual's physical features and the proportional ratios of different body structures. The structure of clothing [10] is derived from the external form of the human body, and its functionality should align with the physiological and motion functions, as well as structural characteristics. However, in the "era of mechanical reproduction", ethnic costumes have lost their so-called authenticity and uniqueness, transforming into a series of homogenized items [11] lacking individuality and internal differences. These types of clothing products are mostly designed and mass-produced based on national standard sizes, disregarding the diversity in body shapes among Biasha Miao women. Consequently, the produced garments struggle to accommodate the realistic needs of different body types. To address these challenges, we conducted a comprehensive study focusing on the specific body characteristics of Biasha Miao women. Through a body data collection and analysis of significant reference measurement points, we aimed to understand the diversity in body shapes among the local female villagers. Based on this information, we adopted Made-to-Measure (MTM) design practices [12] for their ethnic costumes. This approach maximizes the preservation of locally distinctive ethnic costumes and their culture in the new era, while meeting the aesthetic and lifestyle needs of Biasha Miao women. By embracing individuality and cultural authenticity, we envision a future where ethnic costumes thrive as living expressions [13] of identity and cultural heritage.
Artificial Intelligence (AI) has carved an indelible footprint across a multitude of sectors, including healthcare, finance, and transportation, creating paradigm shifts in traditional task executions [14,15,16,17]. With its prowess in processing massive data sets, discerning intricate patterns, and facilitating informed decision-making, AI has heralded groundbreaking advancements, reshaping traditional methods [18,19]. Within the creative contours of design, particularly in the burgeoning field of AI-augmented fashion design, AI stands as a transformative catalyst [20]. It not only amplifies creativity but also injects unprecedented precision into processes, thus refining outcomes. By employing AI, designers are equipped with invaluable insights encompassing consumer inclinations, emerging market trajectories, and innovative material applications. Crucially, AI has revolutionized design by automating mundane tasks, thereby liberating the creative bandwidth for more intricate and pioneering projects. From conceptualizing avant-garde design blueprints to orchestrating virtual prototyping and immersive fitting experiences [21], AI has imbued the design world with efficiency and amplified efficacy. Moreover, embracing AI within the design ethos opens avenues for leveraging its capabilities in data-fueled design fine-tuning. Consequently, by riding the wave of AI innovations, designers are primed to lead with impactful designs, resonating with the dynamic predilections of contemporary consumers [22]. Notably, our study delineates the nuanced application of AI in the niche of ethnic costume design, a significance illustrated in Figure 1.
Diving into our methodological approach, the research journey begins with a rigorous literature review, meticulously weaving a theoretical tapestry that melds the prowess of AI in fashion design with the nuanced concept of MTM [23,24]. Transitioning to empirical insights, we then concentrate on gathering data that spans body metrics, fabric nuances, and design sensibilities pertinent to the target ethnic populace. Culminating our approach, we deploy advanced AI techniques, including machine learning and image processing, to decipher the amassed data. This culminates in extracting pivotal insights that inform personalized fit evaluations, judicious fabric assortments, and trailblazing design methodologies.
This paper is organized as follows. In Section 2, we provide a theoretical framework. Section 3 delves into the core of our research, focusing on AI-driven MTM in ethnic clothing design. Moving forward, Section 4 presents a detailed case study on AI-driven MTM ethnic clothing design practice. A reflection and discussion on the design process are offered in Section 5. Lastly, Section 6 concludes the paper.
AI has had a profound impact on the fashion design industry, revolutionizing various aspects of the field [25,26,27,28]. It has been widely applied in intelligent design assistants, fashion style analysis, creative generation, and size customization, leading to more efficient and innovative approaches to garment design [29,30]. Notably, in the domain of intelligent design assistants, our research explores the significance of AI in enhancing the recommendation process and generating personalized images for ethnic fashion. By integrating advanced image representation and training techniques, our AI-powered design assistants contribute to the development of novel products and extend beyond traditional item recommendations. This underscores the potential of intelligent design assistance in ethnic fashion, setting our study apart in its specific focus on addressing the needs of ethnic clothing design. Regarding fashion style analyses, Ma et al. [31] tackled the challenges of understanding clothing fashion styles, proposing a Fashion Semantic Space (FSS) based on Kobayashi's aesthetics theory. The innovative Bimodal Correlative Deep Autoencoder (BCDA) model captured the relationship between visual features and fashion styles. Experimental results outperformed baselines (+13% in MSE), highlighting the AI-driven advancements in clothing fashion style analyses and predictions. Case studies on brand fashion trends and clothing collocation recommendations further illustrated the model's advantages. Liu et al. [32] highlighted the utilization of AI in a fashion style analysis, focusing on the development of a novel framework. By incorporating multidiscriminators and introducing Attribute generative adversarial networks (Attribute-GAN) and CA generative adversarial networks (CA-GAN) models, the study demonstrated the significant application of AI in advancing fashion style analysis. In the realm of creative generation, Cui et al. [33] introduced an end-to-end virtual garment display method based on Conditional Generative Adversarial Networks. It quickly generated high-quality virtual garment images that matched the input fashion sketch and fabric image, improving fashion design efficiency and reuse rate. In terms of size customization, Bhagyalakshmi et al. [34] proposed a novel pose-guided virtual try-on technique using GANs. It enabled modifications to both the garment and model's posture, creating photorealistic try-on images with intricate garment details. This method outperformed state-of-the-art models in image-based virtual try-on tasks.
MTM is a production method that caters to individual customer needs by creating custom-designed products based on specific measurements, preferences, and specifications. This approach ensures personalized solutions and a tailored fit, enhancing the user experience. In the context of ethnic clothing design, MTM presents a unique and innovative approach that harmoniously combines traditional crafting methods with modern technological applications, as showcased in Figure 2. MTM is a versatile approach that extends to various industries, including automotive, technology, furniture, fashion and apparel. In the automotive industry, MTM is utilized to manufacture vehicles with customized features and instructions. Customers have the opportunity to select interior trims, color schemes, upholstery materials, and additional traits to generate a personalized driving experience [35]. Additionally, MTM extends to the technology sector, where it is employed in the production of devices like computers and smartphones. Customers can configure storage capacity, display size and other attributes to create devices that satisfy their specific requirements. By offering this degree of personalization, users can have technology products that are meticulously tailored to meet their individual needs, leading to an exceptional user experience characterized by optimal usability and functionality. In the furniture sector, MTM is utilized to fabricate customized pieces such as sofas, chairs, and beds. Customers have the flexibility to choose the dimensions, materials, finishes, and other details to suit their particular preferences and spatial constraints. This level of customization allows for the creation of furniture that perfectly matches individual needs and enhances the overall aesthetic appeal of living spaces [36]. In the fashion and apparel industry, MTM enables the creation of individualized clothing items such as suits, dresses, shirts, and trousers. According to the provided unique measurements and selected fabric, style, and details, designers can produce garments that are catered to the customer's exact demands. This customization enhances subscriber satisfaction and offers a superior fit compared to ready-to-wear alternatives [37].
The integration of AI and MTM techniques has revolutionized the field of clothing customization. By harnessing the power of AI, MTM has been able to provide individualized solutions and heighten the quality of customer interactions in the fashion industry, as illustrated by accurate size recommendations, customized style suggestions, and virtual try-on experiences. One notable application of AI in the integration of MTM is tailored style recommendations. Through AI algorithms, customer preferences, style inclinations and historical data can be analyzed to provide personalized proposals for clothing styles [31]. For example, AI can analyze a customer's past purchases, browsing history, and feedback to suggest clothing styles that align with their unique tastes. Another significant aspect is the rapid prototyping and sample garment creation process. In conjunction with computer-aided design (CAD) technology, enables for the quick generation of design blueprints and digital models for sample garments, which expedites the sample production process and reduces time and costs. Designers can digitally make adjustments and modifications, visualize the final product, and generate virtual prototypes before moving on to physical production [38]. While there is limited specific research in the context of ethnic clothing design, exploring the feasibility of AI within the MTM framework holds great potential. Ethnic clothing embodies unique cultural and traditional elements, offering an opportunity for AI algorithms to analyze and learn from vast amounts of ethnic clothing designs and patterns. Leveraging AI in this domain could facilitate the provision of personalized designs and guidance that align with the cultural heritage and individual preferences of customers [22]. By combining the power of AI with MTM techniques, the fashion industry can offer an enhanced and immersive customer experience, tailoring clothing to each individual's specific needs and preferences. As the interest in AI applications continues to grow across various domains, exploring the integration of AI in ethnic clothing design will undoubtedly unlock new possibilities and contribute to the sustainable development of the fashion industry. In addition to the immediate applications of AI in innovative design and customization, there lies a profound potential for AI in the realm of long-term cultural preservation. As we venture further into the digital age, the risk of fading cultural memories and disappearing traditional craftsmanship becomes more pronounced. Artificial Intelligence offers a bridge to this looming gap. Beyond merely creating trendy designs, AI can be harnessed to digitize and meticulously archive traditional patterns, motifs, and indigenous crafting techniques. By doing so, not only do we ensure that these precious cultural artifacts remain accessible and adaptable to modern-day aesthetics, but we also ensure their longevity, safeguarding them for the appreciation and enrichment of future generations. Thus, the integration of AI in ethnic fashion design isn't just a matter of contemporary relevance; it's a pivotal step towards preserving the tapestry of our cultural heritage, thereby emphasizing its significance far beyond immediate fashion industry applications.
To ensure a personalized fit in ethnic clothing design, we utilized an AI-based body analysis, specifically focusing on Miao women. Furthermore, we employed machine learning algorithms, wherein we use the K-means clustering algorithm to classify the multi-dimensional body measurements. The K-means algorithm can be expressed as follows:
C=argmink∑i=1∑M∈Ci|M−μi|2 | (3.1) |
where C are the clusters, k is the number of clusters, M denotes the measurements, Ci is the ith cluster, and μi is the centroid of Ci.
To augment our understanding of Miao women's unique physical features, we incorporate image processing methods to extract intricate details of their physique. In this case, we employ the Canny edge detection algorithm, which helps us outline the silhouettes in a binary image I. The Canny algorithm applies Gaussian filter and gradient calculations to detect the edges, which can be summarized as follows:
E=Canny(I) | (3.2) |
where E is the set of detected edges.
These insights form the foundation for our MTM designs, allowing us to effectively capture the unique physical characteristics of Miao women. Through Al, we can design more personalized, comfortable, and culturally aware clothing.
Fabric selection plays a significant role in capturing the essence of tradition in modern ethnic clothing design. In our research, we leverage AI to extract fabric characteristics, such as texture, color, and pattern, and predict their suitability in specific designs.
The convolutional layer of a CNN is utilized to extract feature maps from fabric images. Given an image I, the convolution operation can be represented as follows:
Fij=∑m∑nI(i−m)(j−n)∗Kmn | (3.3) |
where Fij is the feature map, I(i−m)(j−n) is the image, and Kmn is the kernel. Then, the extracted feature maps Fij are fed into an MLP model to predict the fabric's suitability score S in a design. The MLP operation can be represented as follows:
S=σ(W⋅F+b) | (3.4) |
where σ is the activation function, W is the weight matrix, F is the input feature map, and b is the bias.
By integrating AI, we provide accurate fabric selection advice that not only enhances the aesthetic appeal, but also aligns with the traditional elements, thereby ensuring the design's cultural integrity.
AI plays a pivotal role in driving innovative design in the realm of Miao ethnic clothing, bridging traditional elements with modern aesthetics. GANs and Natural Language Processing (NLP) serve as primary instruments in our design process.
A GAN is deployed to generate novel patterns and design elements. Given a noise vector z, a generator G creates a new data instance xnew, which can be mathematically represented as follows:
xnew=G(z) | (3.5) |
These new patterns are evaluated against existing ethnic designs by a discriminator D, whose goal is to maximize its ability to distinguish real samples from generated ones. This can be represented as follows:
maxDV(D)=Ex∼pdata(x)[logD(x)]+Ez∼pz(z)[log(1−D(G(z)))] | (3.6) |
where x∼pdata(x) are samples from the real data, z∼pz(z) are the noise samples, and V(D) is the value function to be maximized.
On the other hand, NLP algorithms process textual design briefs, deciphering and translating them into design attributes to guide the generation process.
By incorporating AI, we expand the creative spectrum, marrying tradition with innovation. This approach enables the creation of designs that are not only unique and marketable but also culturally resonant, reaffirming the critical role of AI in ethnic clothing design.
Recognizing the importance of firsthand experiences in reinforcing the validity of our AI-driven MTM approach, we conducted in-depth user studies. We actively sought out Miao women who have engaged with the AI-assisted design process. Through surveys and detailed interviews, we gathered insights into their level of satisfaction, their specific preferences in design alterations, and any challenges or obstacles they faced during the procedure. Their perspectives have significantly enriched our study, offering a holistic understanding of the real-world implications and practicality of our approach in the context of ethnic clothing design. The field survey focused on adult females aged 18 to 45 from the Biasha Miao community, aiming to collect and analyze their body shape data. A total of 132 samples were collected, representing 20.3% of the Biasha Miao adult female population. To ensure accuracy and consistency, manual measurement methods were employed using various measuring tools such as height-weight scales, rulers, protractors, and nylon ropes. These measurements followed the guidelines outlined in the "Body Measurement for Clothing Construction" standard (GB/T16160-2008), encompassing 25 body dimensions.
To address the complexity of the data and to ensure its validity, standardized protocols were followed during measurements. Each body dimension was independently measured by three trained individuals, and the average values were considered as valid measurements. To further enhance data integrity, the collected data underwent organization, classification, and statistical analysis using the SPSS software. Specifically tailored statistical methodologies were employed to analyze the unique body shape characteristics of Miao women in Biasha.
Due to the time-consuming manual measurement process, to ensure the validity of the data and mitigate the potential occurrence of either random measurement deviations or statistical errors during recording or other stages, the collected data underwent analysis using the SPSS software. Through this analysis, the data was thoroughly screened to identify any exceptional cases or outliers that could have an impact on the analysis results. Appropriate measures, such as data correction or exclusion, were taken to address these anomalies and to ensure the integrity of the analysis. From Figure 3, the box plot reveals the presence of three outliers at the lower edge. Upon examining the raw data, it was found that individuals with ID 95, 32, and 123 exhibited distinct characteristics in terms of their height measurements. Although these observations were unique cases, they were excluded from the dataset to preserve the integrity of the subsequent data analysis and statistics. As a result, a total of 129 valid samples were retained, resulting in a sample validity rate of 97.73%.
In the body frequency analysis conducted on adult women of the Biasha Miao ethnic group in the Southeastern Guizhou Province (as depicted in Figure 4), it is evident that their height values conform to a basic normal distribution. The majority of them have heights ranging from 147.00 to 155.00 cm, accounting for a total of 94 individuals, representing 72.86% of the overall measurement sample. The average height is 150.07 cm, with a minimum value of 139.00 cm. On average, their height falls approximately 10.00 cm lower than the height values specified in the Standard Sizing Systems for Garments-Women (GB/T1335.2-2008). Based on the computed data (as shown in Table 1), the skewness value for height is -0.290, indicating a slightly right-skewed distribution with a weak left tail. The kurtosis value is -0.230, suggesting that the kurtosis of the distribution curve is lower than that of the standard normal distribution. Given these height characteristics, it is essential to consider the contemporary minority women's visual pursuit of height when designing traditional ethnic clothing. This will ensure that the designed garments can achieve the desired effect of visually elongating the wearer to a certain extent.
N | Mean | Standard Deviation | Variance | Skewness | Kurtosis |
129 | 150.07 | 4.72 | 22.23 | -0.29 0.21 | -0.23 0.42 |
Despite the significantly lower average height compared to the standard body, results of the measurement and statistical analysis conducted in this study (as shown in Table 2) reveal that adult women exhibit higher values in bust height and underbust girth when compared to the standard body. Through actual surveys and interactions with the local population, it was discovered that the majority of women engage in household chores and lead a relaxed lifestyle, especially among married and childbearing women who do not wear bras regularly. This is a major contributing factor to the pronounced occurrence of breast sagging. Additionally, the initial larger bust girth value leads to a displacement of the bust high point and an increase in bust height values, with most values concentrated between 25.00 and 29.00 cm. Furthermore, due to the relatively overweight figure and high body weight among the subjects, the underbust girth values also increase and surpass those found in the national standard body. Therefore, in the practice of MTM design for ethnic clothing, careful attention should be given to the shaping of women's bust contours in order to align with the aesthetic preferences of contemporary women.
Control Site | Height | Bust Girth | Bust Height | Underbust Girth |
National Standard Body for Adult Women | 160.00 | 84.00 | 25.00 | 75.00 |
Biasha Miao Adult Women | 150.07 | 87.86 | 26.10 | 75.70 |
The comparison of mean values for key body measurements between adult females of the Biasha Miao ethnic group in the Southeastern Guizhou Province and the national standard body measurements (as shown in Table 3) reveals significant differences. {Notably}, the average height of Biasha Miao adult females is significantly lower than that of the national standard body measurement. Additionally, their shoulder width is smaller by approximately 0.66 cm. Conversely, measurements for bust, waist, and hip girth display contrasting results, with all three values being larger than those of the standard body measurements. Specifically, the waist girth differs by approximately 5.00 to 7.00 cm, while the difference in hip girth is around 3.00 cm. These data suggest that adult females of the Biasha Miao ethnic group have a larger body size and a tendency towards curvaceous body contours.
Measurement Site | Height | Shoulder Width | Bust Girth | Waist Girth | Waist Height |
National Standard Body for Adult Women | 160.00 | 39.40 | 84.00 | 68.20 | 98.10 |
Body Shape of Adult Biasha Miao Women | 150.07 | 38.74 | 87.86 | 75.69 | 94.81 |
Analysis of frequency of body obesity types based on bust-to-height ratio
Through the preliminary data analysis, it was observed that there is not a significant difference in height among adult women of the Biasha Miao ethnic group [39]. In order to distinguish variations in the obese body type among individuals with the same height, the distribution of body fat types among them was analyzed based on the ratio of bust girth to height (refer to Table 4). It can be observed that the majority of adult women fall into the categories of the moderate, slightly overweight, and obese in terms of body fatness. However, there is also a small percentage of individuals whose bust-to-height ratio falls outside the provided classification ranges, accounting for 18.6% of the surveyed population. Upon further examination of the raw data, it became evident that these individuals exhibit a distinct body type characterized by lower height values and larger bust girth values. This finding reinforces the notion of the diverse and unique body shapes present among adult women of the Biasha Miao ethnic group.
Body Obesity Type Distribution | Slender | Thin | Moderate | Slightly Chubby | Obese | Extremely Obese | "Special Body" |
Code | 0 | 1 | 2 | 3 | 4 | 5 | |
HBR | 2.1 | 2 | 1.9 | 1.8 | 1.7 | 1.6 | 1.5 1.4 |
Frequency Distribution | 0 | 3 | 25 | 17 | 42 | 15 | 23 4 |
HBR: Height/Bust Ratio |
Body type classification
In the Standard Sizing Systems for Garments-Women (GB/T 1335.2-2008), female body types are classified into four categories based on the difference between bust and waist measurements: Y (19–24 cm), A (14–18 cm), B (9–13 cm), and C (4–8 cm). Considering the particularity of the adult women's body shape characteristics, their body shapes were classified, with the most representative parts selected as the division basis among the reference measurement points through the principal component analysis method.
Due to the minimal variation in height differences, it was not considered as a reference measurement for classification. Based on the variance of the reference measurement points, waist girth and waist-to-hip ratio were determined as the primary components, while abdominal girth and bust girth were considered as secondary components. Using a clustering analysis process that groups a collection of physical or abstract objects into multiple classes consisting of similar objects, the body types were classified into four categories using the k-means algorithm (randomly selecting K initial cluster centroids, assigning samples to the cluster represented by the nearest centroid, recalculating the centroids of K clusters, repeating the process until the decrease in error falls below a certain threshold). The body types were labeled as MA, MB, MC, and MD (refer to Table 5).
Body Type | MA | MB | MC | MD |
Frequency | 36 | 45 | 24 | 24 |
Measurement Site |
BG WG AG WHR |
BG WG AG WHR |
BG WG AG WHR |
BG WG AG WHR |
Cluster Center Values |
78.67 64.00 72.58 22.25 |
86.40 73.47 81.67 17.53 |
93.88 81.75 88.63 15.25 |
98.38 91.38 97.75 10.00 |
BG: Bust Girth WG: Waist Girth AG: Abdominal girth WHR: Waist-Hip Ratio |
When classifying new sample data into body types, each body measurement value is inputted for calculation.
For example, here is a given sample with a bust girth of B1, waist girth of H1, abdominal girth of AC1, and waist-to-hip ratio of X1 :
1) |B1−78.67|+|H1−64.00|+|AC1−72.58|+|X1−22.25|=Y1
2) |B1−86.40|+|H1−73.47|+|AC1−81.67|+|X1−17.53|=Y2
3) |B1−93.88|+|H1−81.75|+|AC1−88.63|+|X1−15.25|=Y3
4) |B1−98.38|+|H1−91.38|+|AC1−97.75|+|X1−10.00|=Y4 5) If Y4>Y1>Y3>Y2, the sample belongs to MB body type.
When inferring the body type category of a new experimental sample, the values of its bust girth, waist girth, abdominal girth, and waist-to-hip ratio are subtracted from the cluster center values of the four body type categories. The category with the smallest sum of absolute differences is considered the closest match for the experimental sample's body type classification.
Reasonable classification of body types serves as the foundation for expanding the coverage of clothing sizes. In our efforts to establish a comprehensive sizing system for adult female clothing in the Biasha Miao ethnic group, we identified key reference measurement points. These reference measurement points were based on the guidelines provided in the Standard Sizing Systems for Garments-Women, while taking into consideration the specific body characteristics of the Biasha Miao population and the existing clothing production process. The identified reference measurement points include shoulder width, neck girth, bust girth, bust height, waist girth, abdominal girth, hip girth, arm length, and leg length.
The intermediate values of each reference measurement point represent the average levels of various body types, providing a certain level of representativeness. Upon comparison, we observed that the reference measurement points for different body types exhibit significant variations and a wide distribution. On the other hand, the intermediate values for the four body types show minimal changes, indicating less distinct patterns in body shape variations. These findings are summarized in Table 6.
Body Type | MA | MB | MC | MD | ||||
Classification | RV | Intermediate Value | RV | Intermediate Value | RV | Intermediate Value | RV | Intermediate Value |
SW | 36–41 | 39 | 36–42 | 39 | 37–40 | 39 | 34–42 | 39 |
NG | 29–34 | 32 | 29–37 | 32 | 31–35 | 32 | 30–35 | 32 |
BG | 74–95 | 87 | 71–101 | 88 | 77–95 | 87 | 80–103 | 88 |
BH | 22–29 | 26 | 22–34 | 26 | 23–28 | 26 | 25–29 | 29 |
WG | 60–87 | 75 | 63–93 | 76 | 60–82 | 75 | 70–97 | 76 |
AL | 46–54 | 49 | 43–54 | 49 | 44–54 | 49 | 45–51 | 49 |
AG | 61–95 | 82 | 64–100 | 83 | 70–93 | 83 | 76–105 | 83 |
HG | 80–97 | 92 | 82–106 | 93 | 86–100 | 93 | 87–107 | 93 |
LL | 58–68 | 63 | 60–71 | 64 | 59–66 | 63 | 56–68 | 63 |
RV: Range Value SW: Shoulder Width NG: Neck Girth BH: Bust Height AL: Arm Length HG: Hip Girth LL: Leg Length |
In our AI-driven MTM design process, two main types of algorithms were considered: CNN for body shape analysis and GAN for innovative design generation.
Table 7 provides a comparative analysis of these algorithms.
Algorithm | Strengths | Weaknesses |
CNN | High accuracy in image recognition | Need large datasets |
GAN | Can generate novel designs | Training can be unstable |
The choice of CNN was driven by its superior performance in image recognition tasks, which is crucial for an accurate body shape analysis. GAN was chosen for its ability to generate novel and realistic designs, which is vital for an innovative design generation. These choices ensure a seamless AI-MTM design process, facilitating an accurate analysis and creative design output.
The custom fitting process commences with a meticulous measurement of the individual's body dimensions. For the surveyed Miao women, the average height was determined to be 150.07 cm, with an average bust girth of 87.86 cm, bust height of 26.10 cm, and underbust measurement of 75.70 cm.
This collected data serves as the input to our CNN model for body shape analysis. The CNN model has been trained to accurately predict the body shape class of a new individual based on their measurements. Then, the prediction from the CNN model is utilized to design clothing that offers an optimal fit for that individual's specific body shape.
A scatter plot visualization of the fitting process is depicted in Figure 5, wherein each data point represents an individual's measurements. The x-axis signifies the height, while the y-axis represents the bust girth. Each individual is color-coded based on their predicted body shape class, showcasing the classification outcomes provided by the CNN model.
This process enables us to systematically account for variations in body shape and to ensure that the resulting clothing designs fit well and enhances the wearer's comfort and aesthetic appeal.
The traditional fabric used in Biasha Miao's clothing is a home-woven shiny cloth, characterized by high gloss and firm texture. While maintaining a sleek, hard appearance, it has limitations such as color fading when wet and a tendency to crease. Given these attributes, an AI could be trained to recognize such limitations and suggest alternatives.
In the case study, we adopted spacer fabric (commonly known as "spacer cotton") as the primary material for our design (Figure 6). The choice is influenced by its excellent plasticity, vibrant visual saturation, and a good balance between glossiness and softness, increasing the clothing's overall comfort. For the chest decoration, we still utilize traditional Miao fabric.
With further advancements in AI, we foresee a future where it could potentially predict the optimum fabric types given the wearer's body measurements and local climate conditions, truly revolutionizing the fabric selection process in ethnic clothing design.
The MUNIT algorithm is a powerful generative model that operates by utilizing content and style encoding concepts to generate multiple translations for a single input image in an unsupervised manner [40]. In the domain of clothing design, we harnessed the capabilities of this algorithm to create diverse and distinct patterns, elements, and styles.
The algorithm works in the following way. Given an input image X from domain A and a random style code from domain B, the algorithm generates an image translation Y. This process can be formulated as follows:
Y=GA→B(X,zB) | (4.1) |
where GA→B is the generator, and zB is a random style drawn from a style code distribution in domain B.
In our case, the input image X is a standard pattern of Miao women's clothing. By applying various style codes zB, which represent different artistic styles, patterns, or color palettes, we generate a variety of unique designs Y, as presented in Figure 7. This approach enables a fusion of traditional Miao motifs with modern aesthetics, maintaining the cultural essence while achieving a contemporary appeal.
By integrating such advanced AI algorithms into the design process, we are not only enhancing the creativity and novelty of designs, but also making strides towards the digital transformation of the ethnic clothing design industry.
In the realm of ethnic costume design, Zedong Mao, the founding chairman of the People's Republic of China, emphasized the importance of tapping into the reservoir of literary and artistic raw materials that exist in people's lives [41]. These raw materials, though natural and rough, are the most vivid, abundant, and fundamental elements that form the basis of artistic creations. In a sense, ethnic costumes are also works of art, and their design should ultimately resonate with the daily lives of the target population, becoming their everyday attire [42]. Specifically, when considering the traditional costumes of the Miao ethnic group in the Southeastern Guizhou Province, their original design and manufacture were driven by practical needs related to production and living [43]. However, with the growth of cultural and tourism industries and the widespread use of mobile internet, the aesthetic consciousness of the Miao people has gradually awakened. As a result, the design and production of Miao ethnic costumes today must not only cater to daily life needs and respect cultural traditions, but also align with current aesthetic culture. Embracing innovation that is rooted in and serves daily life becomes an inevitable path for the modern development of Miao ethnic costume design [44]. In this context, the integration of AI presents a fresh and innovative perspective, igniting a more vibrant approach that combines "life + aesthetics". The incorporation of AI in ethnic costume design adds a new dimension of creativity and possibilities, enriching the connection between cultural heritage and contemporary fashion trends. This paradigm of innovation, significantly embedded in and beneficial for daily life, stands as a crucial trajectory for the evolution and revitalization of Miao ethnic costume design in the modern era.
Most researchers have focused primarily on the aspects of Miao ethnic clothing [45], such as its forms, patterns, colors, and production techniques, paying little attention to the physique of the Miao people. However, the physique is a central consideration in clothing design, as garments must fit well to exhibit both aesthetics and practicality [46]. Amid China's ongoing economic development, human body shape has gradually evolved, though these changes appear less evident among the Miao ethnic group in the Southeastern Guizhou Province. This study demonstrates that adult women, specifically in the Biasha area, have an average height of 150.07 cm, which is 10.00 cm lower than the national standard. Their bust, waist, and hip measurements are higher than the national standard, exhibiting a body physique of a fuller bust, wider waist, and rounder hips. Designing and producing Miao ethnic clothing based on the national standard would clearly not be suitable for their physique characteristics. Given these differences, harnessing AI in the design process offers substantial possibilities. AI, equipped with machine learning algorithms and data analysis, can effectively customize the design and construction of Miao ethnic clothing to better suit the unique body shape of the target population [47]. By harnessing AI's capabilities, clothing can be tailored to enhance comfort and fit while preserving the inherent aesthetic qualities of Miao ethnic clothing.
The continuous advancements in AI technology offer promising prospects for the field of ethnic costumes and clothing design. Among these advancements, GANs emerge as powerful AI tools capable of generating ethnic clothing designs. Through training the model to understand and integrate the distinct styles, patterns, and colors of ethnic costumes, GANs can produce novel and culturally-informed costume designs, serving as a valuable source of creative inspiration for designers.
Furthermore, AI can find applications in material selection, production optimization, and garment quality control, enhancing the efficiency and quality of garment design and manufacturing. The incorporation of AI in the domain of ethnic costumes and clothing design presents an array of opportunities for progress and novelty. It equips designers with invaluable tools for creative support, cultural exploration, and design refinement, thereby fostering innovation and advancement in ethnic costume design. Embracing AI holds the promise of a future where diverse, personalized, and culturally distinctive ethnic apparel pieces abound.
Actually, the risk of cultural appropriation emerges when AI algorithms, devoid of cultural understanding, attempt to generate designs based on datasets. The indiscriminate application might lead to either superficial or incorrect interpretations of ethnic motifs, patterns, or symbols, risking the dilution or misrepresentation of the rich Miao heritage. To mitigate this, our study emphasized the continuous collaboration with Miao artisans and cultural experts, ensuring that the AI algorithms were trained with an authentic and respectful understanding of the Miao culture. Moreover, there's a looming concern about the eclipse of traditional craftsmanship. The heart of ethnic fashion lies in its handcrafted elements, passed down through generations. Introducing AI-driven methods might raise apprehensions about overshadowing these traditional practices. However, our approach is designed not as a replacement but as a complement to these age-old practices. The AI-powered designs aim to enhance the traditional with modern insights, ensuring the preservation of the intrinsic value of handmade ethnic fashion.
In conclusion, the integration of AI technologies in ethnic clothing design has ushered in a new era of advanced and personalized design processes. This research presents a comprehensive approach to designing Miao women's apparel by harnessing the power algorithms, specifically in body shape customization, fabric selection, and innovative design practices.
The study highlighted the significant advantages of combining AI and MTM techniques for achieving individual customization. Through a statistical approach, the research formulated accurate and comfortable fits for Miao women, taking into account their distinct body characteristics. Moreover, AI-driven fabric selection optimized the process, enabling careful analysis of fabric properties and recommending ideal materials for both aesthetic and comfort purposes.
Innovatively, the MUNIT algorithm application has redefined design possibilities, generating an array of distinctive and fashionable designs. This capability to churn unique styles underscores AI's role in reinforcing the uniqueness and modernity of ethnic clothing design. Moreover, this work emphasized the integration of traditional craft elements and modern materials to preserve cultural heritage while enhancing comfort and visual appeal. This balanced approach showcased the significant potential of AI technology in fostering the sustainable development of ethnic costume design.
The findings of this study open up exciting opportunities for further research and development in the intersection of artificial intelligence and fashion design, thereby providing a novel perspective that could reshape the fashion industry's landscape.
The authors declare they have not used Artificial Intelligence (AI) tools in the creation of this article.
This work was supported by the Humanities and Social Research Science Institute of the Ministry of Education under grant number 17YJAZH015.
The authors declare there is no conflicts of interest.
[1] |
E. Cohen, Ethnic tourism in mainland Southeast Asia: the state of the art, Tourism Recreat. Res., 41 (2016), 232–245. https://doi.org/10.1080/02508281.2016.1188485 doi: 10.1080/02508281.2016.1188485
![]() |
[2] | X. N. Agency, Opinions on the implementation of the project for the inheritance and development of excellent traditional chinese culture, 2017. Available from: https://www.gov.cn/gongbao/content/2017/content_5171322.htm. |
[3] |
A. P. Provin, A. R. de A. Dutra, M. M. Machado, A. L. V. Cubas, New materials for clothing: rethinking possibilities through a sustainability approach-a review, J. Cleaner Prod., 282 (2021), 124444. https://doi.org/10.1016/j.jclepro.2020.124444 doi: 10.1016/j.jclepro.2020.124444
![]() |
[4] |
S. E. Palmer, K. B. Schloss, J. Sammartino, Visual aesthetics and human preference, Annu. Rev. Psychol., 64 (2013), 77–107. https://doi.org/10.1146/annurev-psych-120710-100504 doi: 10.1146/annurev-psych-120710-100504
![]() |
[5] |
J. Kim, Collaborative fashion consumption in the sharing economy: philosophical and aesthetic perspectives, J. Global Fashion Mark., 11 (2020), 289–305. https://doi.org/10.1080/20932685.2020.1724815 doi: 10.1080/20932685.2020.1724815
![]() |
[6] |
C. Gu, L. Wu, I. Cook, Progress in research on chinese urbanization, Front. Archit. Res., 1 (2012), 101–149. https://doi.org/10.1016/j.foar.2012.02.013 doi: 10.1016/j.foar.2012.02.013
![]() |
[7] |
V. Greene, Dealing with diversity: milwaukee's multiethnic festivals and urban identity, 1840–1940, J. Urban Hist., 31 (2005), 820–849. https://doi.org/10.1177/0096144205278169 doi: 10.1177/0096144205278169
![]() |
[8] | R. Gill, Cities and ethnicity: a case of de-ethnicization or re-ethnicization? Sociol. Bull., 49 (2000), 211–228. https://doi.org/10.1177/0038022920000203 |
[9] |
C. Hwang, T. L. Chung, E. A. Sanders, Attitudes and purchase intentions for smart clothing: examining us consumers' functional, expressive, and aesthetic needs for solar-powered clothing, Clothing Text. Res. J., 34 (2016), 207–222. https://doi.org/10.1177/0887302X16646447 doi: 10.1177/0887302X16646447
![]() |
[10] |
H. M. K. Ullah, J. Lejeune, A. Cayla, M. Monceaux, C. Campagne, E. Devaux, A review of noteworthy/major innovations in wearable clothing for thermal and moisture management from material to fabric structure, Text. Res. J., 92 (2022), 3351–3386. https://doi.org/10.1177/00405175211027799 doi: 10.1177/00405175211027799
![]() |
[11] |
M. Magri, P. Ciarletta, Mathematics meets the fashion industry on path to product innovation and sustainability, Proc. R. Soc. A, 479 (2023), 20220715. https://doi.org/10.1098/rspa.2022.0715 doi: 10.1098/rspa.2022.0715
![]() |
[12] |
H. S. Han, J. Y. Kim, S. M. Kim, H. S. Lim, C. K. Park, The development of an automatic pattern-making system for made-to-measure clothing, Fibers Polym., 15 (2014), 422–425. https://doi.org/10.1007/s12221-014-0422-6 doi: 10.1007/s12221-014-0422-6
![]() |
[13] |
J. Li, Q. Wang, Z. Zhu, Living inheritance of intangible cultural heritage: experience value system, measurement and inspection, Tourism Tribune, 35 (2020), 78–89. https://doi.org/10.19765/j.cnki.1002-5006.2020.11.011 doi: 10.19765/j.cnki.1002-5006.2020.11.011
![]() |
[14] |
Y. Shi, L. Li, J. Yang, Y. Wang, S. Hao, Center-based transfer feature learning with classifier adaptation for surface defect recognition, Mech. Syst. Signal Process., 188 (2023), 110001. https://doi.org/10.1016/j.ymssp.2022.110001 doi: 10.1016/j.ymssp.2022.110001
![]() |
[15] |
Y. Shi, H. Li, X. Fu, R. Luan, Y. Wang, N. Wang, et al., Self-powered difunctional sensors based on sliding contact-electrification and tribovoltaic effects for pneumatic monitoring and controlling, Nano Energy, 110 (2023), 108339. https://doi.org/10.1016/j.nanoen.2023.108339 doi: 10.1016/j.nanoen.2023.108339
![]() |
[16] |
H. Su, W. Qi, Y. Hu, H. R. Karimi, G. Ferrigno, E. De Momi, An incremental learning framework for human-like redundancy optimization of anthropomorphic manipulators, IEEE Trans. Ind. Inf., 18 (2020), 1864–1872. https://doi.org/10.1109/TII.2020.3036693 doi: 10.1109/TII.2020.3036693
![]() |
[17] |
W. Qi, H. Fan, H. R. Karimi, H. Su, An adaptive reinforcement learning-based multimodal data fusion framework for human–robot confrontation gaming, Neural Networks, 164 (2023), 489–496. https://doi.org/10.1016/j.neunet.2023.04.043 doi: 10.1016/j.neunet.2023.04.043
![]() |
[18] |
D. Liu, Y. Zhang, D. Jia, Q. Zhang, X. Zhao, H. Rong, Toward secure distributed data storage with error locating in blockchain enabled edge computing, Comput. Stand. Interfaces, 79 (2022), 103560. https://doi.org/10.1016/j.csi.2021.103560 doi: 10.1016/j.csi.2021.103560
![]() |
[19] |
W. Qi, H. Su, A cybertwin based multimodal network for ecg patterns monitoring using deep learning, IEEE Trans. Ind. Inf., 18 (2022), 6663–6670. https://doi.org/10.1109/TII.2022.3159583 doi: 10.1109/TII.2022.3159583
![]() |
[20] | J. Zhu, A. Liapis, S. Risi, R. Bidarra, G. M. Youngblood, Explainable AI for designers: a human-centered perspective on mixed-initiative co-creation, in 2018 IEEE Conference on Computational Intelligence and Games (CIG), (2018), 1–8. https://doi.org/10.1109/CIG.2018.8490433 |
[21] |
U. Gültepe, U. Güdükbay, Real-time virtual fitting with body measurement and motion smoothing, Comput. Graphics, 43 (2014), 31–43. https://doi.org/10.1016/j.cag.2014.06.001 doi: 10.1016/j.cag.2014.06.001
![]() |
[22] |
E. Nica, O. M. Sabie, S. Mascu, A. G. Luţan, Artificial intelligence decision-making in shopping patterns: consumer values, cognition, and attitudes, Econ. Manage. Financ. Mark., 17 (2022), 31–43. https://doi.org/10.22381/emfm17120222 doi: 10.22381/emfm17120222
![]() |
[23] |
D. Jia, Z. Xu, Y. Wang, R. Ma, W. Jiang, Y. Qian, et al., Application of intelligent time series prediction method to dew point forecast, Electron. Res. Arch., 31 (2023), 2878–2899. https://doi.org/10.3934/era.2023145 doi: 10.3934/era.2023145
![]() |
[24] |
J. Zhao, Y. Lv, Output-feedback robust tracking control of uncertain systems via adaptive learning, Int. J. Control Autom. Syst., 21 (2023), 1108–1118. https://doi.org/10.1007/s12555-021-0882-6 doi: 10.1007/s12555-021-0882-6
![]() |
[25] |
W. Qi, A. Aliverti, A multimodal wearable system for continuous and real-time breathing pattern monitoring during daily activity, IEEE J. Biomed. Health. Inf., 24 (2019), 2199–2207. https://doi.org/10.1109/JBHI.2019.2963048 doi: 10.1109/JBHI.2019.2963048
![]() |
[26] |
C. Tian, Z. Xu, L. Wang, Y. Liu, Arc fault detection using artificial intelligence: challenges and benefits, Math. Biosci. Eng., 20 (2023), 12404–12432. https://doi.org/10.3934/mbe.2023552 doi: 10.3934/mbe.2023552
![]() |
[27] |
Z. Liu, D. Yang, Y. Wang, M. Lu, R. Li, Egnn: Graph structure learning based on evolutionary computation helps more in graph neural networks, Appl. Soft Comput., 135 (2023), 110040. https://doi.org/10.1016/j.asoc.2023.110040 doi: 10.1016/j.asoc.2023.110040
![]() |
[28] |
Y. Wang, Z. Liu, J. Xu, W. Yan, Heterogeneous network representation learning approach for ethereum identity identification, IEEE Trans. Comput. Social Syst., 10 (2022), 890–899. https://doi.org/10.1109/TCSS.2022.3164719 doi: 10.1109/TCSS.2022.3164719
![]() |
[29] |
H. Su, W. Qi, Y. Schmirander, S. E. Ovur, S. Cai, X. Xiong, A human activity-aware shared control solution for medical human–robot interaction, Assem. Autom., 42 (2021), 388–394. https://doi.org/10.1108/AA-12-2021-0174 doi: 10.1108/AA-12-2021-0174
![]() |
[30] |
W. Qi, S. E. Ovur, Z. Li, A. Marzullo, R. Song, Multi-sensor guided hand gesture recognition for a teleoperated robot using a recurrent neural network, IEEE Rob. Autom. Lett., 6 (2021), 6039–6045. https://doi.org/10.1109/LRA.2021.3089999 doi: 10.1109/LRA.2021.3089999
![]() |
[31] | Y. Ma, J. Jia, S. Zhou, J. Fu, Y. Liu, Z. Tong, Towards better understanding the clothing fashion styles: a multimodal deep learning approach, in Proceedings of the AAAI Conference on Artificial Intelligence, 31 (2017). https://doi.org/10.1609/aaai.v31i1.10509 |
[32] |
L. Liu, H. Zhang, X. Xu, Z. Zhang, S. Yan, Collocating clothes with generative adversarial networks cosupervised by categories and attributes: a multidiscriminator framework, IEEE Trans. Neural Networks Learn. Syst., 31 (2019), 3540–3554. https://doi.org/10.1109/TNNLS.2019.2944979 doi: 10.1109/TNNLS.2019.2944979
![]() |
[33] |
Y. R. Cui, Q. Liu, C. Y. Gao, Z. Su, Fashiongan: Display your fashion design using conditional generative adversarial nets, Comput. Graphics Forum, 37 (2018), 109–119. https://doi.org/10.1111/cgf.13552 doi: 10.1111/cgf.13552
![]() |
[34] | A. Bhagyalakshmi, N. Sanjay, S. Aravindhan, V. Jayakrishnan, Virtual dressing room application using GANs, in 2023 9th International Conference on Advanced Computing and Communication Systems (ICACCS), (2023), 112–116. https://doi.org/10.1109/ICACCS57279.2023.10113074 |
[35] | T. Chen, S. Fritz, K. Shea, Design for mass customization using additive manufacture: case-study of a balloon-powered car, in DS 80-4 Proceedings of the 20th International Conference on Engineering Design (ICED 15), 4 (2015), 245–254. |
[36] | M. T. Feraboli, The made-to-measure house: from an ideal home to a palace between the 19th and 21st centuries, La Casa. Espacios domésticos, modos de habitar, 2019 (2019), 1341–1350. Available from: https://re.public.polimi.it/handle/11311/1084179. |
[37] |
A. R. Harwood, J. Gill, S. Gill, Jblockcreator: An open source, pattern drafting framework to facilitate the automated manufacture of made-to-measure clothing, Software X, 11 (2020), 100365. https://doi.org/10.1016/j.softx.2019.100365 doi: 10.1016/j.softx.2019.100365
![]() |
[38] |
E. Papahristou, N. Bilalis, 3D virtual prototyping traces new avenues for fashion design and product development: a qualitative study, J. Text. Sci. Eng., 7 (2017), 1–6. https://doi.org/10.4172/2165-8064.1000297 doi: 10.4172/2165-8064.1000297
![]() |
[39] | E. A. Gribbin, Body shape and its influence on apparel size and consumer choices, Designing Apparel Consum., (2014), 3–16. https://doi.org/10.1533/9781782422150.1.3 |
[40] | X. Huang, M. Y. Liu, S. Belongie, J. Kautz, Multimodal unsupervised image-to-image translation, in Computer Vision – ECCV 2018, (2018), 179–196. https://doi.org/10.1007/978-3-030-01219-9_11 |
[41] |
X. Zhang, Reflections on the historical context and political philosophical implications of mao zedong's talks at the yan'an forum on literature and art, Front. Lit. Stud. China, 13 (2019), 122–146. https://doi.org/10.3868/s010-007-019-0006-7 doi: 10.3868/s010-007-019-0006-7
![]() |
[42] |
Z. Ji, W. H. Huang, M. Lin, Design mode innovation of local color cultures: a case study of the traditional female costume of Yi nationality, Designs, 4 (2020), 56. https://doi.org/10.3390/designs4040056 doi: 10.3390/designs4040056
![]() |
[43] | G. Tao, The computer-aided design of miao costume patterns based on big data, in MMIA 2020: Application of Intelligent Systems in Multi-modal Information Analytics, (2020), 844–849. https://doi.org/10.1007/978-3-030-51556-0_132 |
[44] |
D. Han, L. Cong, Miao traditional patterns: the origins and design transformation, Visual Stud., 2021 (2021), 1–8. https://doi.org/10.1080/1472586X.2021.1940261 doi: 10.1080/1472586X.2021.1940261
![]() |
[45] |
J. Su, J. Sun, Spatial changes of ethnic communities during tourism development: a case study of basha miao minority community, J. Tourism Cult. Change, 18 (2020), 333–350. https://doi.org/10.1080/14766825.2019.1679159 doi: 10.1080/14766825.2019.1679159
![]() |
[46] |
O. Rahman, M. Gong, Sustainable practices and transformable fashion design–chinese professional and consumer perspectives, Int. J. Fashion Des. Technol. Educ., 9 (2016), 233–247. https://doi.org/10.1080/17543266.2016.1167256 doi: 10.1080/17543266.2016.1167256
![]() |
[47] | M. Luo, Y. Liu, P. Li, J. Hu, A clothing design method and system implementation based on virtual measurement of human body shape, in 2021 International Conference on Intelligent Transportation, Big Data & Smart City (ICITBS), (2021), 489–492. https://doi.org/10.1109/ICITBS53129.2021.00125 |
1. | Rufan Lin, Yongkang Chen, Lekai Qiu, Yihan Yu, Fan Xia, The Influence of Interactivity, Aesthetic, Creativity and Vividness on Consumer Purchase of Virtual Clothing: The Mediating Effect of Satisfaction and Flow, 2024, 1044-7318, 1, 10.1080/10447318.2024.2359226 | |
2. | Xubing Xu, Qiong Luo, Tian Zhong, Research on innovative visualization design of miao costume images in Qiandongnan under aesthetic perspective, 2024, 9, 2444-8656, 10.2478/amns-2024-2727 | |
3. | Zhengtang Tan, Shuang Lin, Zebin Wang, Cluster Size Intelligence Prediction System for Young Women’s Clothing Using 3D Body Scan Data, 2024, 12, 2227-7390, 497, 10.3390/math12030497 | |
4. | Gu Xiaoxue, 2024, Research on Application System of Computer Aided Design in Innovative Design of Intangible Cultural Heritage Clothing, 979-8-3503-6024-0, 1993, 10.1109/ICIPCA61593.2024.10709043 | |
5. | Yi Xiang, 2024, Intelligent Clothing Design and Embodied Cognitive System Based on Convolutional Neural Network and Generative Adversarial Network, 979-8-3315-2762-4, 1173, 10.1109/ICEDCS64328.2024.00214 | |
6. | Meizhen Deng, Ling Chen, CDGFD: Cross-Domain Generalization in Ethnic Fashion Design Using LLMs and GANs: A Symbolic and Geometric Approach, 2025, 13, 2169-3536, 7192, 10.1109/ACCESS.2024.3524444 | |
7. | Miao Yu, Geometric modeling and computer-aided creation methods in traditional cultural costume design, 2025, 10, 2444-8656, 10.2478/amns-2025-0717 | |
8. | Jingting Meng, Xingjia Fang, Jian Xu, Ziqi Zhang, Research on the Innovative Application of Song Dynasty Boundary Painting in Interior Soft Decoration Design Based on AIGC, 2025, 15, 2075-5309, 1067, 10.3390/buildings15071067 |
N | Mean | Standard Deviation | Variance | Skewness | Kurtosis |
129 | 150.07 | 4.72 | 22.23 | -0.29 0.21 | -0.23 0.42 |
Control Site | Height | Bust Girth | Bust Height | Underbust Girth |
National Standard Body for Adult Women | 160.00 | 84.00 | 25.00 | 75.00 |
Biasha Miao Adult Women | 150.07 | 87.86 | 26.10 | 75.70 |
Measurement Site | Height | Shoulder Width | Bust Girth | Waist Girth | Waist Height |
National Standard Body for Adult Women | 160.00 | 39.40 | 84.00 | 68.20 | 98.10 |
Body Shape of Adult Biasha Miao Women | 150.07 | 38.74 | 87.86 | 75.69 | 94.81 |
Body Obesity Type Distribution | Slender | Thin | Moderate | Slightly Chubby | Obese | Extremely Obese | "Special Body" |
Code | 0 | 1 | 2 | 3 | 4 | 5 | |
HBR | 2.1 | 2 | 1.9 | 1.8 | 1.7 | 1.6 | 1.5 1.4 |
Frequency Distribution | 0 | 3 | 25 | 17 | 42 | 15 | 23 4 |
HBR: Height/Bust Ratio |
Body Type | MA | MB | MC | MD |
Frequency | 36 | 45 | 24 | 24 |
Measurement Site |
BG WG AG WHR |
BG WG AG WHR |
BG WG AG WHR |
BG WG AG WHR |
Cluster Center Values |
78.67 64.00 72.58 22.25 |
86.40 73.47 81.67 17.53 |
93.88 81.75 88.63 15.25 |
98.38 91.38 97.75 10.00 |
BG: Bust Girth WG: Waist Girth AG: Abdominal girth WHR: Waist-Hip Ratio |
Body Type | MA | MB | MC | MD | ||||
Classification | RV | Intermediate Value | RV | Intermediate Value | RV | Intermediate Value | RV | Intermediate Value |
SW | 36–41 | 39 | 36–42 | 39 | 37–40 | 39 | 34–42 | 39 |
NG | 29–34 | 32 | 29–37 | 32 | 31–35 | 32 | 30–35 | 32 |
BG | 74–95 | 87 | 71–101 | 88 | 77–95 | 87 | 80–103 | 88 |
BH | 22–29 | 26 | 22–34 | 26 | 23–28 | 26 | 25–29 | 29 |
WG | 60–87 | 75 | 63–93 | 76 | 60–82 | 75 | 70–97 | 76 |
AL | 46–54 | 49 | 43–54 | 49 | 44–54 | 49 | 45–51 | 49 |
AG | 61–95 | 82 | 64–100 | 83 | 70–93 | 83 | 76–105 | 83 |
HG | 80–97 | 92 | 82–106 | 93 | 86–100 | 93 | 87–107 | 93 |
LL | 58–68 | 63 | 60–71 | 64 | 59–66 | 63 | 56–68 | 63 |
RV: Range Value SW: Shoulder Width NG: Neck Girth BH: Bust Height AL: Arm Length HG: Hip Girth LL: Leg Length |
Algorithm | Strengths | Weaknesses |
CNN | High accuracy in image recognition | Need large datasets |
GAN | Can generate novel designs | Training can be unstable |
N | Mean | Standard Deviation | Variance | Skewness | Kurtosis |
129 | 150.07 | 4.72 | 22.23 | -0.29 0.21 | -0.23 0.42 |
Control Site | Height | Bust Girth | Bust Height | Underbust Girth |
National Standard Body for Adult Women | 160.00 | 84.00 | 25.00 | 75.00 |
Biasha Miao Adult Women | 150.07 | 87.86 | 26.10 | 75.70 |
Measurement Site | Height | Shoulder Width | Bust Girth | Waist Girth | Waist Height |
National Standard Body for Adult Women | 160.00 | 39.40 | 84.00 | 68.20 | 98.10 |
Body Shape of Adult Biasha Miao Women | 150.07 | 38.74 | 87.86 | 75.69 | 94.81 |
Body Obesity Type Distribution | Slender | Thin | Moderate | Slightly Chubby | Obese | Extremely Obese | "Special Body" |
Code | 0 | 1 | 2 | 3 | 4 | 5 | |
HBR | 2.1 | 2 | 1.9 | 1.8 | 1.7 | 1.6 | 1.5 1.4 |
Frequency Distribution | 0 | 3 | 25 | 17 | 42 | 15 | 23 4 |
HBR: Height/Bust Ratio |
Body Type | MA | MB | MC | MD |
Frequency | 36 | 45 | 24 | 24 |
Measurement Site |
BG WG AG WHR |
BG WG AG WHR |
BG WG AG WHR |
BG WG AG WHR |
Cluster Center Values |
78.67 64.00 72.58 22.25 |
86.40 73.47 81.67 17.53 |
93.88 81.75 88.63 15.25 |
98.38 91.38 97.75 10.00 |
BG: Bust Girth WG: Waist Girth AG: Abdominal girth WHR: Waist-Hip Ratio |
Body Type | MA | MB | MC | MD | ||||
Classification | RV | Intermediate Value | RV | Intermediate Value | RV | Intermediate Value | RV | Intermediate Value |
SW | 36–41 | 39 | 36–42 | 39 | 37–40 | 39 | 34–42 | 39 |
NG | 29–34 | 32 | 29–37 | 32 | 31–35 | 32 | 30–35 | 32 |
BG | 74–95 | 87 | 71–101 | 88 | 77–95 | 87 | 80–103 | 88 |
BH | 22–29 | 26 | 22–34 | 26 | 23–28 | 26 | 25–29 | 29 |
WG | 60–87 | 75 | 63–93 | 76 | 60–82 | 75 | 70–97 | 76 |
AL | 46–54 | 49 | 43–54 | 49 | 44–54 | 49 | 45–51 | 49 |
AG | 61–95 | 82 | 64–100 | 83 | 70–93 | 83 | 76–105 | 83 |
HG | 80–97 | 92 | 82–106 | 93 | 86–100 | 93 | 87–107 | 93 |
LL | 58–68 | 63 | 60–71 | 64 | 59–66 | 63 | 56–68 | 63 |
RV: Range Value SW: Shoulder Width NG: Neck Girth BH: Bust Height AL: Arm Length HG: Hip Girth LL: Leg Length |
Algorithm | Strengths | Weaknesses |
CNN | High accuracy in image recognition | Need large datasets |
GAN | Can generate novel designs | Training can be unstable |