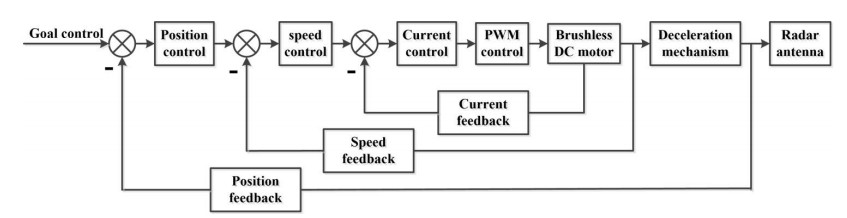
This paper proposes a PID controller optimized by a reformative artificial bee colony algorithm (RABC-PID) for the radar servo system (RSS). The RABC algorithm is an enhancement of the artificial bee colony (ABC) algorithm by introducing the best-positioned food source and modifying the food source probability. The RABC algorithm is validated by simulation with six benchmark functions, and the results show that the RABC algorithm is superior to the other variants of the ABC algorithm in terms of convergence speed and accuracy. The RABC-PID controller is then used for the RSS. The RSS is presented to illustrate the application of the RABC-PID controller. The simulation results, which are also compared to PID optimized by particle swarm optimization, differential evolution, and genetic algorithm (PSO-PID, DE-PID, and GA-PID) respectively, are shown to illustrate the effectiveness and robustness of the RABC-PID controller.
Citation: Hualong Du, Qiuyu Cui, Pengfei Liu, Xin Ma, He Wang. Reformative artificial bee colony algorithm based PID controller for radar servo system[J]. Electronic Research Archive, 2022, 30(8): 2941-2963. doi: 10.3934/era.2022149
[1] | Chunhua Chu, Yijun Chen, Qun Zhang, Ying Luo . Joint linear array structure and waveform design for MIMO radar under practical constraints. Electronic Research Archive, 2022, 30(9): 3249-3265. doi: 10.3934/era.2022165 |
[2] | Mingtao Cui, Min Pan, Jie Wang, Pengjie Li . A parameterized level set method for structural topology optimization based on reaction diffusion equation and fuzzy PID control algorithm. Electronic Research Archive, 2022, 30(7): 2568-2599. doi: 10.3934/era.2022132 |
[3] | Qianqian Zhang, Mingye Mu, Heyuan Ji, Qiushi Wang, Xingyu Wang . An adaptive type-2 fuzzy sliding mode tracking controller for a robotic manipulator. Electronic Research Archive, 2023, 31(7): 3791-3813. doi: 10.3934/era.2023193 |
[4] | Ilyоs Abdullaev, Natalia Prodanova, Mohammed Altaf Ahmed, E. Laxmi Lydia, Bhanu Shrestha, Gyanendra Prasad Joshi, Woong Cho . Leveraging metaheuristics with artificial intelligence for customer churn prediction in telecom industries. Electronic Research Archive, 2023, 31(8): 4443-4458. doi: 10.3934/era.2023227 |
[5] | Tongtong Yu, Zhizhou Zhang, Yang Li, Weilong Zhao, Jinchu Zhang . Improved active disturbance rejection controller for rotor system of magnetic levitation turbomachinery. Electronic Research Archive, 2023, 31(3): 1570-1586. doi: 10.3934/era.2023080 |
[6] | Rajakumar Ramalingam, Saleena B, Shakila Basheer, Prakash Balasubramanian, Mamoon Rashid, Gitanjali Jayaraman . EECHS-ARO: Energy-efficient cluster head selection mechanism for livestock industry using artificial rabbits optimization and wireless sensor networks. Electronic Research Archive, 2023, 31(6): 3123-3144. doi: 10.3934/era.2023158 |
[7] | Jingshi Li, Jiachuan Zhang, Guoliang Ju, Juntao You . A multi-mode expansion method for boundary optimal control problems constrained by random Poisson equations. Electronic Research Archive, 2020, 28(2): 977-1000. doi: 10.3934/era.2020052 |
[8] | Shizhen Huang, Enhao Tang, Shun Li, Xiangzhan Ping, Ruiqi Chen . Hardware-friendly compression and hardware acceleration for transformer: A survey. Electronic Research Archive, 2022, 30(10): 3755-3785. doi: 10.3934/era.2022192 |
[9] | Mengke Lu, Shang Gao, Xibei Yang, Hualong Yu . Improving performance of decision threshold moving-based strategies by integrating density-based clustering technique. Electronic Research Archive, 2023, 31(5): 2501-2518. doi: 10.3934/era.2023127 |
[10] | Xiaoyan Wu, Guowen Ye, Yongming Liu, Zhuanzhe Zhao, Zhibo Liu, Yu Chen . Application of Improved Jellyfish Search algorithm in Rotate Vector reducer fault diagnosis. Electronic Research Archive, 2023, 31(8): 4882-4906. doi: 10.3934/era.2023250 |
This paper proposes a PID controller optimized by a reformative artificial bee colony algorithm (RABC-PID) for the radar servo system (RSS). The RABC algorithm is an enhancement of the artificial bee colony (ABC) algorithm by introducing the best-positioned food source and modifying the food source probability. The RABC algorithm is validated by simulation with six benchmark functions, and the results show that the RABC algorithm is superior to the other variants of the ABC algorithm in terms of convergence speed and accuracy. The RABC-PID controller is then used for the RSS. The RSS is presented to illustrate the application of the RABC-PID controller. The simulation results, which are also compared to PID optimized by particle swarm optimization, differential evolution, and genetic algorithm (PSO-PID, DE-PID, and GA-PID) respectively, are shown to illustrate the effectiveness and robustness of the RABC-PID controller.
Radar servo systems (RSS) are widely used in various fields, such as meteorology, satellites, rockets, etc. [1]. As the diversity of tasks and the complexity of the environment in these fields increase, the requirements for the stability and tracking accuracy of the RSS are further increased [2]. However, compared with other servo systems, the RSS is a complex system with uncertainties. First, parameters in the RSS, such as drag, and viscosity, change with the environment. Second, the radar antenna orientation will be deflected under interference. In addition, the position change of the tracking target is random [3]. Since the tracking performance of the RSS is affected by many uncertainties, it is necessary to design a complex robust controller for it [4].
Current controllers for servo systems are sliding mode controllers (SMC), the neural network controller (NNC), and the proportional-integral-derivative controller (PID) [5]. SMC is widely used for servo systems. Integrations of SMC with intelligent methods, such as SMC with fuzzy [6], SMC with neural network [7], SMC with genetic algorithm (GA) [8], and SMC with adaptive backstepping [9,10] were proposed to reduce the jitter phenomenon and ensure stability. NNC is another promising controller. Cao et al. [11] designed a composite neural network adaptive controller for RSS. Lu et al. [12] designed an adaptive neural network controller to achieve accurate control of an RSS with time-varying state constraints. Huang et al. [13] developed an NNC based on a sliding mode variable structure for improving the stability of the missile servo system. He et al. [14] proposed a neural network parallel self-learning tracking controller that was used in large-scale radar control. Gong et al. [15] proposed a monitoring NNC in which feedback is used in the initial stage to further improve the tracking capability of the radar.
The structure of the SMC is not fixed but is constantly changing as the system state changes, resulting in a range of parameter variations and disturbances. The disadvantages of NCC are slow parameter updates and poor control performance. Therefore, designing a good controller for RSS is still a challenge.
The PID controller is still receiving attention in various fields due to its simplicity and ease of implementation [16]. Chen et al. [17] designed a neuronal adaptive PID controller to improve the response speed of the RSS. However, optimization of the PID controller poses a challenging problem for selecting the right parameters. Swarm intelligence (SI) algorithms have been applied in the real world [18,19,20,21]. SI algorithms have attracted a lot of attention for the optimization of PID controllers in recent years. Özdemir et al. [22] proposed the optical-inspired optimization (OIO) algorithm to optimize the PID controller. The proposed controller has better performance in terms of maximum overshoots and stabilization time. Chen et al. [23] proposed a fuzzy PID controller optimized by an improved ant colony (IACO) algorithm. Nonlinear incremental evaporation rates and pheromone incremental updates were employed in the IACO algorithm to improve the quality of the solution. Hekimoğlu et al. [24]. proposed the atomic search optimization (ASO) algorithm to determine the optimal parameters of fractional-order proportional-integral-derivative (FOPID) controller. Although many SI algorithms have been applied to PID controller optimization, some SI algorithms exhibit local convergence or achieve global solutions at the cost of a high computational burden. Therefore, other SI algorithms are further tried to achieve faster convergence and globally optimal solutions.
The ABC algorithm is a SI algorithm proposed by Karaboga by simulating the characteristics of honey bee colony honey harvesting behavior [25], which is easy to implement with few parameters. The motivation for this paper was to develop an ABC-PID controller for improving the tracking accuracy of the RSS. This paper substantially extends the previous work on PID controllers based on SI algorithms, and can be distinguished from it from three aspects as follows:
● The application of PID controller based on ABC algorithm in RSS has been rarely reported. Here is an addition to the PID-SI controller application. The PID controller based on the RABC algorithm (RABC-PID) is proposed to apply to the RSS.
● A RABC optimization algorithm is conducted in this work. In the RABC algorithm, the solution search equation and select probability are modified to balance its explorative and exploitative capabilities.
● The superiority of the RABC-PID controller is confirmed against various input tracking signals and the RSS parameters uncertainties.
The rest of this paper is organized as follows. Section 2 introduces the position loop model of the RSS, and Section 3 introduces the basic ABC algorithm, the RABC algorithm, and the superiority of the RABC algorithm verified by six benchmark functions. The principle of optimized PID controller parameters and the whole optimization process of the RABC algorithm is introduced. Experimental simulations are performed in Section 4 to verify the superiority of the RABC algorithm by comparing it with three other SI algorithms. Section 5 concludes the full paper.
The RSS is a multi-loop feedback controls system, which is composed of three closed loops: current loop, speed loop, and position loop [26]. The current, speed and position feedback are completed by corresponding sensors [27]. The structure of the RSS is shown in Figure 1.
The structure of the current loop is shown in Figure 2. Low-pass filters are added to the feedback loop and input to improve the quality of the feedback signal.
In Figure 2, U1(s) is the current loop input; ACR is the current controller; Toi is the current filtering time constant; α is the current feedback coefficient.
Since the inverter timed constant TPWM and the filter time constant Toi is much smaller than T1. Thus multiple small inertia links are approximated as one inertia link, and the following expression is given by Eq (1).
TΣi=TPWM+Toi | (1) |
When Eq (2) is satisfied, the transfer function of the current loop can be given by Eq (3)
ωoi⩽13√1TPWMToi | (2) |
Gico=αKPWM/R(T1s+1)(TΣis+1) | (3) |
where KPWM is the amplification factor; T1 is the electromagnetic time constant, R is the average resistance of armature winding, ωoi and is the cut-off frequency for the current loop.
The PI controller is used for the current loop, and its transfer function can be given by the following equation (4)
WACR(S)=Ki(τis+1)τis | (4) |
where Ki is the proportional coefficient of the PI controller; τi is the overrun time constant of the PI controller.
If τi = T1, then the open-loop transfer function of the current loop is expressed as Eq (5)
Gio=KIs(TΣis+1) | (5) |
where KI is a constant and KI=KiKPWMα/τiR.
The transfer function of the closed-loop of the current loop can be given by equation(6)
Gic=KIs(TΣis+1)1+KIs(TΣis+1)=1TΣiKIs2+1KIs+1 | (6) |
When Eq (7) is satisfied, The transfer function of the closed-loop of the current loop can be simplified as Eq (8)
ωcn⩽13√KITΣi | (7) |
Gic(s)=1s/KI+1 | (8) |
where ωcn is the cut-off frequency for the speed loop.
If Eq (8) is considered as a link in the speed loop, then the structure of the speed loop is shown in Figure 3.
In Figure 3, Un(s) is the speed loop input; ASR is the speed controller; Ton is the speed filter time constant; β is the speed feedback coefficient.
Multiple small inertia links are approximated as one inertia link, and the following expression is given by Eq (9)
TΣn=2TΣi+Ton | (9) |
The PI controller is used for the speed loop, and its transfer function can be given by the following Eq (10)
WASR(s)=Kn(τns+1)τns | (10) |
where Kn is the proportional coefficient of the PI controller; τn is the overrun time constant of the PI controller. The open-loop transfer function of the speed loop can be given by
Gno(s)=Kn(τns+1)τnsβRCeTms(TΣns+1)=KnβR(τns+1)τnCeTms2(TΣns+1) | (11) |
Equation (11) can be simplified as Eq (12)
Gno(s)=KN(τns+1)s2(TΣns+1) | (12) |
where KN is a constant and KN=KnβR/τnCeTm.
If higher-order terms are ignored, according to Eq (12) the transfer function of the closed-loop of the speed loop can be given by Eq (13)
Gnc(s)=1(τns+1)(TΣns+1) | (13) |
If Eq (13) is considered as a link in the position loop, then the structure of the position loop is shown in Figure 4.
In Figure 4, UP(s) is the position loop input; ART stands for the position controller; Top is the position filter time constant; γ is the angle feedback coefficient.
The open-loop transfer function of the position loop can be given by Eq (14).
G(S)=γs(τns+1)(TΣns+1)(Tops+1) | (14) |
When Eq (15) is satisfied, the transfer function of the closed-loop of the position loop can be simplified as Eq (16)
ωcp⩽13√1τnTΣn+τnTop+TΣnTop | (15) |
G(S)=γs(TΣps+1) | (16) |
where TΣp=TΣn+Top+τn
In this paper, the PID controller is optimized by the RABC algorithm (RABC-PID) as the ART controller. The RABC-PID controller is described in detail in the next section.
In the basic ABC algorithm, the bees are divided into three types: employed bees, follower bees, and scout bees. A search phrase is set for one type of bees, namely: employed bees phase, follow bees phase, and scout bees phase [28]. In the beginning, the algorithm uses a random initialization method to generate the initial population, and its generation method is shown in the following Eq (17)
Xji=Xjmin+rand(0,1)(Xjmax−Xjmin) | (17) |
where i = 1, ..., SN, n = 1, ..., D. SN represents the population size, D represents the dimension of the problem, rand(0, 1) is a random number between 0-1, Xjmax and Xjmin represents the upper and lower bounds of the individual jth dimension, respectively.
In the stage of the employed bees, the employed bees search for a food source according to Eq (18)
Vji=Xji+φji(Xji−Xjk) | (18) |
where k∈(1, ..., SN) and k ≠i; j∈(1, ..., D). φji is a uniformly distributed random number on [−1, 1].
In the following bees stage, the follower bees use the information that the employed bees share, to select the food source according to the probability calculated by the following equation (19). The search process for following bees still proceeds according to Eq (18)
Pi=fitiΣSNj=1fitj | (19) |
where fiti represents the fitness value of the ith food source.
In the scout bee stage, a food source, the quality that has not been getting better, will be discarded. The following bee searching for this food source will turn into the scout bee. In the ABC algorithm, the food source corresponds to the candidate solution of the optimization problem, and the quality of the food source represents the good or bad candidate solution.
The exploration and the exploitation conflict with each other. Enhancement of the exploration will inevitably affect the exploitation reducing the convergence speed of the algorithm and vice versa. ABC algorithm has a strong exploration ability and weak exploitation ability [29]. This paper proposes an RABC algorithm to balance exploration and exploitation. The search equation in the RABC algorithm is added to the best-positioned food source so that the bees search around better quality food sources. Using the optimal food source as a reference improves the searchability of the algorithm to some extent. The search equation in the RABC algorithm can be given by Eq (20)
Vji=Xji, best+φji(Xji, best−Xji) | (20) |
where Xji, best is the best-positioned food source.
The selection probability of bees is determined by the proportion of fitness of the current food source among all food sources. Little difference between the better food source and the optimal food source leads to a lower probability of selection of the optimal food source. The chance to search for some bad food source results in slowing down the speed of finding the optimal food source due to the random selectivity in the ABC algorithm. In this paper, we propose to use the current optimal fitness as a reference to improve the speed of the colony in finding the optimal food source. Thus the selection probability can be given by Eq (21)
Pi=0.8fitifitmax+0.2 | (21) |
where fitmax is the fitness value for the optimal solution.
Using Eq (20) to update the food source location ensures that the bees are not disturbed by the locally optimal bees, but also that the bees move to the best-positioned food source. Equation (21) enhances the probability of exploitation of high-quality food sources.
To investigate the solution performance of the RABC algorithm, comparisons with ABC, GABC [30], and GBABC [31] algorithms were performed using six benchmark functions that are shown in Table 1. The parameters of the four algorithms were set as follows: D = 50, SN = 100, limit = 50, MaxCycle = 5000. Each algorithm runs independently 30 times. The results are presented in Table 2, Figures 5 and 6.
Function | Name | Definition Domain | Optimal value |
F1 | Ackley | (-15, 30) | 0 |
F2 | Griewank | (-600,600) | 0 |
F3 | Zakharo | (-5, 10) | 0 |
F4 | Sphere | (-100,100) | 0 |
F5 | Sumpower | (-1.1) | 0 |
F6 | Sumsquare | (-10, 10) | 0 |
Function | ABC | GABC | GBABC | RABC | |
F1 | Mean Std |
6.33345e-13+ 4.89751e-14+ |
5.07136e-13+ 2.58946e-14+ |
9.85936e-14+ 8.65746e-15+ |
8.26357e-14 4.29453e-15 |
F2 | Mean Std |
4.01418e-12+ 3.77788e-12+ |
1.03324e-13+ 1.16972e-14+ |
8.13825e-14+ 8.65482e-15+ |
5.10703e-15 5.80934e-15 |
F3 | Mean Std |
8.04328e-15+ 7.47394e-15+ |
2.8544e-15+ 4.4677e-16+ |
1.14164e-15+ 5.13749e-17+ |
1.07917e-15 8.23919e-17 |
F4 | Mean Std |
7.29812e-15+ 8.75926e-17+ |
4.28892e-15+ 3.60087e-17+ |
1.34151e-15+ 1.24779e-17+ |
1.10285e-15 1.03897e-17 |
F5 | Mean Std |
3.48822e-09+ 3.43749e-09+ |
1.57483e-9+ 5.15095e-10+ |
3.42314e-16+ 6.90644e-17+ |
1.48323e-16 3.40782e-17 |
F6 | Mean Std |
5.17476e-15+ 2.52029e-15+ |
3.30387e-15+ 1.26809e-15+ |
1.47304e-15+ 1.95032e-16+ |
1.11128e-15 2.73786e-17 |
Table 2 gives the accuracy results of four algorithms for the six benchmark functions. In Table 2, the mean represents the mean of the error and std represents the standard deviation of the error. The results of the Wilcoxon statistical test at a 5% confidence level are also given, with "+, = , -" indicating that the RABC algorithm is better, the same, or worse than the other algorithms, respectively. From Table 2, it can be seen that the RABC outperforms the ABC, the GABC, and the GBABC in terms of solution accuracy.
Figure 5 shows the convergence curve of four algorithms on the benchmark function. From Figure 5, it can be seen that the RABC is superior to the ABC, the GABC, and the GBABC in terms of convergence speed. Figure 6 shows a boxplot of iterative data for four algorithms. The top and bottom edges of the boxplot indicate the 75th percentile and 25th percentile, respectively. From Figure 6, it can be further seen that the top and bottom edges of the boxplot from the RABC almost overlap, which further illustrates the fast convergence of the RABC.
The schematic of the RABC-PID controller is shown in Figure 7. The essence of the RABC-PID controller is to find the optimal values of Kp, Ki, and Kd using the RABC with the objective function as the criterion, which results in the best control performance of RSS. In Figure 7, r(t) is the RSS input, y(t) is the RSS output, and e(t) is the error between input and output.
It is also important for the system to choose an objective function for calculating the performance index (PI). The objective function used in this paper is the following equation (22)
PI=∫t0[J1t|e(t)|+J2tr(t)2+J3|e(t)|]dt | (22) |
where J1, J2, and J3 are weighting factors, which are 0.999, 0.001, and 100, respectively, in this paper. t is the time.
To better clarify our controller, referred to as RABC-PID, its pseudocode is described in the following Table 3.
1:Set the number of solution parameters (D), the number of populations (SN), the maximum number of cycles (MaxCycle), and the "limit" value; randomly generate candidate solutions (X=[Xji]SN×D); Choose PI as the performance index. |
2: Calculate the fitness value of the initial solution fitx using equation (22), Record the optimal solution Xbest 3: cycle=0, count =0, 4: while (cycle < = MaxCycle) 5: *Employed bee stage* 6: for i=1 to SN 7:Generated the new candidate solution V using equation (20). Calculate the fitness value of the new solution fitv using equation (22). 8: if fitv > fitx, then X= V, count=0; 9: else count=count +1; 10: end if 11: Record the optimal solution Xbest; 12: end for 13: Calculate the fitness values of nectar sources and probability Pi by using equation (21) 14: *Follower bee stage* 15: m =0, n=0 16: while (m < SN) 17: Generate a random number rand between 0 and 1 18: if rand < Pi, then m = m +1; repeat employed bee stage; 19: end if 20: n=n+1 21: if n > SN then n=1; 22: end if 23: end while 24: * Scout bee stage * 25: for i=1 to SN 26: if count > = limit, then Generated the new candidate solution X using equation (17). 27: end if 28: Record the optimal solution Xbest = [Kp, Ki, Kd]; 29: end for 30: cycle = cycle+1; 31: end while 32: output Xbest = [Kp, Ki, Kd] |
In this section RABC-PID controller is applied to the position loop of RSS for simulation verification. In the simulation experiments, the parameters of the RSS are set as follows: γ = 100, TΣp = 0.25. With the above parameters, the transfer function of the closed-loop of the position loop can be given by Eq (23)
G(S)=1000.25s2+s | (23) |
To fully evaluate the performance of the RABC-PID controller, three SI-PID controllers were used for comparison. The basic SI algorithms are particle swarm optimization (PSO) [32], differential evolution (DE) [33], and genetic algorithm (GA) [34], respectively. In the simulation experiments, the specific parameters of four controllers are given below:
RABC-PID settings: population number SN is set 50, the limit is set 50, and the maximum number of cycles MaxCycle is set 50.
PSO-PID settings: acceleration constant c1 and c2 are set 2, inertia weight W is set 0.9, maximum particle flight speed Vmax is set 1, minimum particle flight speed Vmin is set −1, population number SN is set 50, the maximum number of cycles MaxCycle is set 50.
DE-PID settings: crossover probability Pcr, scaling factor F, population number SN, and the maximum number of cycles MaxCycle are set at 0.8, 0.85, 50, and 50, respectively.
GA-PID settings: crossover probability Pc, mutation probability Pm, population number SN and the maximum number of cycles MaxCycle are set at 0.7, 0.3, 50, and 50, respectively.
To simulate different tracking targets, the inputs of the RSS are set as a square wave, triangle wave, and sine wave, respectively. The experimental results, including PI, optimum value, mean, and std are presented in Tables 4–7, respectively. Figures 8–13 show the convergence speed of the four controllers for different inputs.
PI | Kp | Ki | Kd | |
RABC-PID | 582.6 | 47.9215 | -0.680688 | 0.515515 |
PSO-PID | 668.3 | 32.9551 | -4.120694 | 0.168747 |
DE-PID | 665.8 | 20.6111 | -3.454611 | 0.163633 |
GA-PID | 755 | 27.8895 | -4.856542 | 0.280775 |
PI | Kp | Ki | Kd | |
RABC-PID | 483.3 | 24.277 | 2.38791 | 1.08791 |
PSO-PID | 490 | 14.7872 | 2.87741 | 0.82875 |
DE-PID | 489.8 | 12.966 | 2.01063 | 0.63332 |
GA-PID | 507 | 16.5482 | 2.0578 | 0.94521 |
PI | Kp | Ki | Kd | |
RABC-PID | 101.1 | 39.586 | -5.8 | 0.758091 |
PSO-PID | 103.5 | 30.986 | -4.58 | 0.75938 |
DE-PID | 104 | 35.972 | -4.23 | 0.889 |
GA-PID | 103.8 | 32.675 | -4.09 | 0.956 |
GA-PID | DE-PID | PSO-PID | RABC-PID | ||
Square wave | Mean Std |
0.3256 0.5100 |
0.2603 0.5011 |
0.2696 0.4833 |
0.1683 0.4687 |
Triangle wave | Mean Std |
0.1120 0.1588 |
0.0877 0.1640 |
0.0874 0.1627 |
0.0570 0.1451 |
Sine wave | Mean Std |
0.0050 0.0027 |
0.0045 0.0024 |
0.0045 0.0024 |
0.0011 8.6818e-04 |
For square wave input, in Table 4, it is clear that our controller achieves the minimum PI value among four controllers, which indicates that the performance of our controller is better than that of the other three controllers. From Figure 8, the GA-PID controller has the fastest convergence, but its PI is the largest. Although our controller converges slightly slower than PSO-PID and DE-PID, its PI is nearly 15% smaller than theirs. Figure 11 shows a tracking performance comparison for square wave input. From Figure 11(a), the tracking curve obtained by our controller is closer to the input curve than that obtained by the other three controllers. The tracking errors of the four controllers are indistinguishable from each other as seen in Figure 11(b), but the mean and std of the tracking error of our controllers are smaller than those of the other three controllers as seen in Table 7.
For triangular wave input, in Table 5, it can be seen that the PI value of RABC-PID is still the smallest, but all PI values do not differ much. Figure 9 shows convergence that is similar to that shown in Figure 8. From Figure 12, the tracking curves of all controllers are almost coincident in the simulation time except for the first 0.2 s. In Table 7, the tracking performance of our controllers is still ahead of the other three controllers, but that leading trend is decreasing.
For sine wave input, in Table 6, all PI values are similar. The convergence curves in Figure 10 almost overlap except for the curve of GA-PID, resulting in the inability to identify the convergence speed of RABC-PID, PSO-PID, and DE-PID. From Figure 13, the tracking curves of all controllers overlap, but our controller maintains a slight lead given mean and std as shown in Table 7. It is important to note that our controller performs well regardless of the input.
For RSS, the controller is required to have not only good tracking performance but also good robustness to cope with sudden changes in parameters of RSS. In the simulation experiments, the parameter TΣp of RSS varied to 0.5, 0.75, and 1, respectively. The simulation results are presented in Figures 14–22 and Tables 8–10. From Figures 14–22, as the parameter TΣp of RSS increases, the tracking performance of all controllers becomes worse, especially under square wave input. From Tables 8–10, it can be seen that a similar conclusion can be drawn as in Figures 14–22. It can be observed that, although the uncertainty of RSS increases with its parameter, the performance of our controller is not always affected. In summary, our controller has good robustness.
GA-PID | DE-PID | PSO-PID | RABC-PID | ||
Square wave | Mean Std |
0.3522 0.5455 |
0.3497 0.5503 |
0.3517 0.5504 |
0.2293 0.5313 |
Triangle wave | Mean Std |
0.1450 0.1679 |
0.1067 0.1650 |
0.1061 0.1643 |
0.0458 0.1342 |
Sine wave | Mean Std |
0.0073 0.0055 |
0.0069 0.0051 |
0.0069 0.0051 |
0.0026 0.0033 |
GA-PID | DE-PID | PSO-PID | RABC-PID | ||
Square wave | Mean Std |
0.3270 0.5515 |
0.4144 0.5832 |
0.4124 0.5830 |
0.2780 0.5188 |
Triangle wave | Mean Std |
0.1665 0.1682 |
0.1334 0.1720 |
0.1328 0.1710 |
0.0503 0.1377 |
Sine wave | Mean Std |
0.0089 0.0073 |
0.0087 0.0072 |
0.0087 0.0072 |
0.0038 0.0035 |
GA-PID | DE-PID | PSO-PID | RABC-PID | ||
Square wave | Mean Std |
0.5834 0.4766 |
0.4724 0.6065 |
0.4749 0.6062 |
0.3193 0.3008 |
Triangle wave | Mean Std |
0.1879 0.1739 |
0.1579 0.1776 |
0.1580 0.1775 |
0.0560 0.1419 |
Sine wave | Mean Std |
0.0115 0.0091 |
0.0107 0.0088 |
0.0107 0.0088 |
0.0050 0.0044 |
The purpose of the current study was to design an RABC-PID controller to improve the tracking performance of RSS. In the RABC-PID controller, a novel RABC algorithm is proposed to enhance the basic ABC algorithm by introducing the best-positioned food source and modifying the food source probability. With a set of six benchmark functions, RABC is proven to have better performance than ABC, GABC, and GBABC. The simulation studies, which conduct on RSS, show that the RABC-PID controller obtains statistical better than the PSO-PID, DE-PID, and GA-PID controllers in terms of tracking performance and robustness. The significance of our work lies in the application of the RABC-PID controller to improve the tracking performance of RSS and enriches the application of SI in RSS.
This study was fully supported by Liaoning Provincial Department of Education Basic Research Projects for Higher Education Institutions, China (No. LJKZ0301); The Scientific Research Foundation of the Education Department of Liaoning Province, China (No. 2017LNQN22); Young Teachers Foundation of University of Science and Technology Liaoning, China (No. 2017QN04).
The authors declare no conflict of interest.
[1] |
A. Bhardwaj, T. K. Pant, R. K. Choudhary, D. Nandy, P. K. Manoharan, Space weather research: Indian perspective, Space Weather, 14 (2016), 1082–1094. https://doi.org/10.1002/2016SW001521 doi: 10.1002/2016SW001521
![]() |
[2] |
H. Y. Xue, Y. J. Li, K. Zhang, Variable structure control of radar servo system based on IMM, 2008 ISECS Int. Colloquium Comput. Commun. Control Manag., 2008. https://doi.org/10.1109/CCCM.2008.249 doi: 10.1109/CCCM.2008.249
![]() |
[3] |
X. Liu, Q. Huang, Y. Chen, Robust adaptive controller with disturbance observer for vehicular radar servo system, Int. J. Control. Autom., 9 (2011), 169–175. https://doi.org/10.1007/s12555-011-0122-6 doi: 10.1007/s12555-011-0122-6
![]() |
[4] |
K. D. Young, V. I. Utkin, U. Ozguner, A control engineer's guide to sliding mode control, IEEE. T. Contr. Syst. T., 7 (1999), 328–342. https://doi.org/10.1109/87.761053 doi: 10.1109/87.761053
![]() |
[5] |
Z. K. Xiong, T. F. Chen, Research on Precise Aiming Control Technology, High Power Laser and Particle Beams, 2012. https://doi.org/10.2514/3.44674 doi: 10.2514/3.44674
![]() |
[6] |
Q. P. Ha, Q. H. Nguyen, D. C. Rye, H. F. Durrant-Whyte, Fuzzy sliding-mode controllers with applications, IEEE. T. Ind. Electron., 48 (2001), 38–46. https://doi.org/10.1109/41.904548 doi: 10.1109/41.904548
![]() |
[7] |
M. Ertugrul, O. Kaynak, Neuro sliding mode control of robotic manipulators, Mechatronics, 10 (2000), 239–263. https://doi.org/10.1016/S0957-4158(99)00057-4 doi: 10.1016/S0957-4158(99)00057-4
![]() |
[8] |
F. J. Lin, W. D. Chou, An induction motor servo drive using sliding-mode controller with geneticalgorithm, Electr. Pow. Syst. Res., 64 (2003), 93–108. https://doi.org/10.1016/S0378-7796(02)00156-6 doi: 10.1016/S0378-7796(02)00156-6
![]() |
[9] |
F. J. Lin, P. H. Shen, S. P. Hsu, Adaptive backstepping sliding mode control for linear induction motordrive, IEE. Procee. Electr. Power. Appl., 149 (2002), 184–194. https://doi.org/10.1049/ip-epa:20020138 doi: 10.1049/ip-epa:20020138
![]() |
[10] |
M. Smaoui, X. Brun, D. Thomasset, Systematic control of an electropneumatic system: integrator backstepping and sliding mode control, IEEE. Trans. Control Syst. Technol., 14 (2006), 905–913. https://doi.org/10.1109/TCST.2006.880183 doi: 10.1109/TCST.2006.880183
![]() |
[11] | F. Cao, Y. Liu, X. Yang, Y. Peng, D. Miao, Neural-network-based sliding mode control for missile electro-hydraulic servo mechanism, In Int. Confer. Neural Inf. Process., Springer, Berlin, Heidelberg, 2006. https://doi.org/10.1007/11893295_66 |
[12] |
S. M. Lu, D. J. Li, Adaptive neural network control for nonlinear hydraulic servo-system with time-varying state constraints, Complexity, 2017. https://doi.org/10.1155/2017/6893521 doi: 10.1155/2017/6893521
![]() |
[13] |
Y. Huang, Y. Zhang, P. Min, Indirect dynamic recurrent fuzzy neural network and its application in identification and control of electro-hydraulic servo system, Int. Symposium Intell. Comput. Appl., 10 (2009), 295–304. https://doi.org/10.1007/978-3-642-04962-0_34 doi: 10.1007/978-3-642-04962-0_34
![]() |
[14] | S. He, N. Sepehri, Modeling and prediction of hydraulic servo actuators with neural networks, Proc. Am. Control Conf. (Cat. No. 99CH36251), 1999. https://doi.org/10.1109/ACC.1999.782458 |
[15] |
M. Gong, D. Zhao, W. Gong, T. Ni, D. Ding, The Position Control of Electrohydraulic Servo Manipulator Based on Neural Network, J. Jilin. Univ. Technol., 32 (2002). https://doi.org/10.13229/j.cnki.jdxbgxb2002.03.004 doi: 10.13229/j.cnki.jdxbgxb2002.03.004
![]() |
[16] |
H. X. Zheng, M. H. Huang, L. H. Zhan, Y. Zhu, P. Liu, Research on High Precision Servo System of Actuator Based on PID Parameter Stability Domain Under Mixed Sensitivity Constraint, J. Electr. Eng. Technol., 16 (2021), 1651–1665. https://doi.org/10.1007/s42835-021-00686-9 doi: 10.1007/s42835-021-00686-9
![]() |
[17] | S. Chen, L. Yang, Y. Liu, Research on Radar Servo Control System Based on Neuron Adaptive PID Control, J. Phys. Conference Series, IOP Publishing, 2020. https://doi:10.1088/1742-6596/1550/6/062002 |
[18] | S. Ozturk, B. Akdemir, Automatic leaf segmentation using grey wolf optimizer based neural network, 2017 Electronics, IEEE, 2017, 1–6. https://doi.org/10.1109/ELECTRONICS.2017.7995228 |
[19] |
Ş. Öztürk, R. Ahmad, N. Akhtar, Variants of Artificial Bee Colony algorithm and its applications in medical image processing, Appl Soft Comput., 97 (2020), 106799. https://doi.org/10.1016/j.asoc.2020.106799 doi: 10.1016/j.asoc.2020.106799
![]() |
[20] |
E. D. P. Puchta, H. V. Siqueira, M. dos Santos Kaster, Optimization tools based on metaheuristics for performance enhancement in a Gaussian adaptive PID controller, IEEE Trans. Cybern., 50 (2019), 1185–1194. https://doi.org/10.1109/TCYB.2019.2895319 doi: 10.1109/TCYB.2019.2895319
![]() |
[21] | E. D. Puchta, R. Lucas, F. R. Ferreira, H. V. Siqueira, M. S. Kaster, Gaussian adaptive PID control optimized via genetic algorithm applied to a step-down DC-DC converter, 2016 12th IEEE Int. Conf. Ind. Appl. (INDUSCON), IEEE, 2016, 1–6. https://doi.org/10.1109/INDUSCON.2016.7874509 |
[22] |
M. T. Özdemi̇r, D. Öztürk, Comparative performance analysis of optimal PID parameters tuning based on the optics inspired optimization methods for automatic generation control, Energies., 10 (2017), 2134. https://doi.org/10.3390/en10122134 doi: 10.3390/en10122134
![]() |
[23] |
G. Chen, Z. Li, Z. Zhang, S. Li, An improved ACO algorithm optimized fuzzy PID controller for load frequency control in multi area interconnected power systems, IEEE Access, 8 (2019), 6429–6447. https://doi.org/10.1109/ACCESS.2019.2960380 doi: 10.1109/ACCESS.2019.2960380
![]() |
[24] |
B. Hekimoğlu, Optimal tuning of fractional order PID controller for DC motor speed control via chaotic atom search optimization algorithm, IEEE Access, 7 (2019), 38100–38114. https://doi.org/10.1109/ACCESS.2019.2905961 doi: 10.1109/ACCESS.2019.2905961
![]() |
[25] |
S. F. Hussain, A. Pervez, M. Hussain, Co-clustering optimization using Artificial Bee Colony (ABC)algorithm, Appl. Soft. Comput., 97 (2020), 106725. https://doi.org/10.1016/j.asoc.2020.106725 doi: 10.1016/j.asoc.2020.106725
![]() |
[26] | G. Wu, X. Xiao, Speed Controller of Servo System Based on Self-tuning Control, Electric. Drive., 39 (2009), 47–50. https://doiorg/10.19457/j.1001 -2095.2009.10.011 |
[27] |
H. Ji, Z. Li, K. Pan, Z. Zhang, Shipborne Radar Servo Control based on Neural Sliding Mode Variable Structure, 2018 IEEE 3rd Adv. Inf. Technol. Electron. Automation Control Conf. (IAEAC), 2018. https://doi.org/10.1109/IAEAC.2018.8577549 doi: 10.1109/IAEAC.2018.8577549
![]() |
[28] |
Karaboga, B. Akay, A comparative study of artificial bee colony algorithm, Appl. Math. Comput., 214 (2009), 108–132. https://doi.org/10.1016/j.amc.2009.03.090 doi: 10.1016/j.amc.2009.03.090
![]() |
[29] |
X. Zhou, H. Wang, M. Wang, J. Wan, Enhancing the modified artificial bee colony algorithm with neighborhood search, Soft Comput., 21 (2017), 2733–2743. https://doi.org/10.1007/s00500-015-1977-x doi: 10.1007/s00500-015-1977-x
![]() |
[30] |
G. Zhu, S. Kwong, Gbest-guided artificial bee colony algorithm for numerical function optimization, Appl. Math. Comput., 217 (2010), 3166–3173. https://doi.org/10.1016/j.amc.2010.08.049 doi: 10.1016/j.amc.2010.08.049
![]() |
[31] |
X. Zhou, Z. Wu, H. Wang, S. Rahnamayan, Gaussian bare-bones artificial bee colony algorithm, Soft. Comput., 20 (2016), 907–924. https://doi.org/10.1007/s00500-014-1549-5 doi: 10.1007/s00500-014-1549-5
![]() |
[32] |
H. Feng, W. Ma, C. Yin, D. Cao, Trajectory control of electro-hydraulic position servo system using improved PSO-PID controller, Autom. Constr., 127 (2021), 103722. https://doi.org/10.1016/j.autcon.2021.103722 doi: 10.1016/j.autcon.2021.103722
![]() |
[33] |
N. Jalali, H. Razmi, H. Doagou-Mojarrad, Optimized fuzzy self-tuning PID controller design based on Tribe-DE optimization algorithm and rule weight adjustment method for load frequency control of interconnected multi-area power systems, Appl. Soft Comput., 93 (2020), 106424. https://doi.org/10.1016/j.asoc.2020.106424 doi: 10.1016/j.asoc.2020.106424
![]() |
[34] |
S. Wang, H. Liang, J. Wang, GA PID control research in inverter motor speed governing system, J. Comput. Methods Sci., 19 (2019), 299–306. https://doi.org/10.3233/JCM-180869 doi: 10.3233/JCM-180869
![]() |
1. | Hui Li, Yan-Bo Hui, Qiao Wang, Hong-Xiao Wang, Lin-Jun Wang, Design of Anti-Swing PID Controller for Bridge Crane Based on PSO and SA Algorithm, 2022, 11, 2079-9292, 3143, 10.3390/electronics11193143 | |
2. | Lu Xiao, Xiao Wang, Fei Chen, Aimin Li, Fuzzy proportional integral differential control of magnetorheological clutch torque based on bee colony algorithm optimization, 2023, 1077-5463, 10.1177/10775463231223585 | |
3. | Yiming Li, Zhongchao Zhang, Mingliang Bai, Guiqiu Song, Composite RISE control for vehicle-mounted servo system with unknown modeling uncertainties and unknown time-varying disturbances, 2024, 147, 00190578, 590, 10.1016/j.isatra.2024.02.017 |
Function | Name | Definition Domain | Optimal value |
F1 | Ackley | (-15, 30) | 0 |
F2 | Griewank | (-600,600) | 0 |
F3 | Zakharo | (-5, 10) | 0 |
F4 | Sphere | (-100,100) | 0 |
F5 | Sumpower | (-1.1) | 0 |
F6 | Sumsquare | (-10, 10) | 0 |
Function | ABC | GABC | GBABC | RABC | |
F1 | Mean Std |
6.33345e-13+ 4.89751e-14+ |
5.07136e-13+ 2.58946e-14+ |
9.85936e-14+ 8.65746e-15+ |
8.26357e-14 4.29453e-15 |
F2 | Mean Std |
4.01418e-12+ 3.77788e-12+ |
1.03324e-13+ 1.16972e-14+ |
8.13825e-14+ 8.65482e-15+ |
5.10703e-15 5.80934e-15 |
F3 | Mean Std |
8.04328e-15+ 7.47394e-15+ |
2.8544e-15+ 4.4677e-16+ |
1.14164e-15+ 5.13749e-17+ |
1.07917e-15 8.23919e-17 |
F4 | Mean Std |
7.29812e-15+ 8.75926e-17+ |
4.28892e-15+ 3.60087e-17+ |
1.34151e-15+ 1.24779e-17+ |
1.10285e-15 1.03897e-17 |
F5 | Mean Std |
3.48822e-09+ 3.43749e-09+ |
1.57483e-9+ 5.15095e-10+ |
3.42314e-16+ 6.90644e-17+ |
1.48323e-16 3.40782e-17 |
F6 | Mean Std |
5.17476e-15+ 2.52029e-15+ |
3.30387e-15+ 1.26809e-15+ |
1.47304e-15+ 1.95032e-16+ |
1.11128e-15 2.73786e-17 |
1:Set the number of solution parameters (D), the number of populations (SN), the maximum number of cycles (MaxCycle), and the "limit" value; randomly generate candidate solutions (X=[Xji]SN×D); Choose PI as the performance index. |
2: Calculate the fitness value of the initial solution fitx using equation (22), Record the optimal solution Xbest 3: cycle=0, count =0, 4: while (cycle < = MaxCycle) 5: *Employed bee stage* 6: for i=1 to SN 7:Generated the new candidate solution V using equation (20). Calculate the fitness value of the new solution fitv using equation (22). 8: if fitv > fitx, then X= V, count=0; 9: else count=count +1; 10: end if 11: Record the optimal solution Xbest; 12: end for 13: Calculate the fitness values of nectar sources and probability Pi by using equation (21) 14: *Follower bee stage* 15: m =0, n=0 16: while (m < SN) 17: Generate a random number rand between 0 and 1 18: if rand < Pi, then m = m +1; repeat employed bee stage; 19: end if 20: n=n+1 21: if n > SN then n=1; 22: end if 23: end while 24: * Scout bee stage * 25: for i=1 to SN 26: if count > = limit, then Generated the new candidate solution X using equation (17). 27: end if 28: Record the optimal solution Xbest = [Kp, Ki, Kd]; 29: end for 30: cycle = cycle+1; 31: end while 32: output Xbest = [Kp, Ki, Kd] |
PI | Kp | Ki | Kd | |
RABC-PID | 582.6 | 47.9215 | -0.680688 | 0.515515 |
PSO-PID | 668.3 | 32.9551 | -4.120694 | 0.168747 |
DE-PID | 665.8 | 20.6111 | -3.454611 | 0.163633 |
GA-PID | 755 | 27.8895 | -4.856542 | 0.280775 |
PI | Kp | Ki | Kd | |
RABC-PID | 483.3 | 24.277 | 2.38791 | 1.08791 |
PSO-PID | 490 | 14.7872 | 2.87741 | 0.82875 |
DE-PID | 489.8 | 12.966 | 2.01063 | 0.63332 |
GA-PID | 507 | 16.5482 | 2.0578 | 0.94521 |
PI | Kp | Ki | Kd | |
RABC-PID | 101.1 | 39.586 | -5.8 | 0.758091 |
PSO-PID | 103.5 | 30.986 | -4.58 | 0.75938 |
DE-PID | 104 | 35.972 | -4.23 | 0.889 |
GA-PID | 103.8 | 32.675 | -4.09 | 0.956 |
GA-PID | DE-PID | PSO-PID | RABC-PID | ||
Square wave | Mean Std |
0.3256 0.5100 |
0.2603 0.5011 |
0.2696 0.4833 |
0.1683 0.4687 |
Triangle wave | Mean Std |
0.1120 0.1588 |
0.0877 0.1640 |
0.0874 0.1627 |
0.0570 0.1451 |
Sine wave | Mean Std |
0.0050 0.0027 |
0.0045 0.0024 |
0.0045 0.0024 |
0.0011 8.6818e-04 |
GA-PID | DE-PID | PSO-PID | RABC-PID | ||
Square wave | Mean Std |
0.3522 0.5455 |
0.3497 0.5503 |
0.3517 0.5504 |
0.2293 0.5313 |
Triangle wave | Mean Std |
0.1450 0.1679 |
0.1067 0.1650 |
0.1061 0.1643 |
0.0458 0.1342 |
Sine wave | Mean Std |
0.0073 0.0055 |
0.0069 0.0051 |
0.0069 0.0051 |
0.0026 0.0033 |
GA-PID | DE-PID | PSO-PID | RABC-PID | ||
Square wave | Mean Std |
0.3270 0.5515 |
0.4144 0.5832 |
0.4124 0.5830 |
0.2780 0.5188 |
Triangle wave | Mean Std |
0.1665 0.1682 |
0.1334 0.1720 |
0.1328 0.1710 |
0.0503 0.1377 |
Sine wave | Mean Std |
0.0089 0.0073 |
0.0087 0.0072 |
0.0087 0.0072 |
0.0038 0.0035 |
GA-PID | DE-PID | PSO-PID | RABC-PID | ||
Square wave | Mean Std |
0.5834 0.4766 |
0.4724 0.6065 |
0.4749 0.6062 |
0.3193 0.3008 |
Triangle wave | Mean Std |
0.1879 0.1739 |
0.1579 0.1776 |
0.1580 0.1775 |
0.0560 0.1419 |
Sine wave | Mean Std |
0.0115 0.0091 |
0.0107 0.0088 |
0.0107 0.0088 |
0.0050 0.0044 |
Function | Name | Definition Domain | Optimal value |
F1 | Ackley | (-15, 30) | 0 |
F2 | Griewank | (-600,600) | 0 |
F3 | Zakharo | (-5, 10) | 0 |
F4 | Sphere | (-100,100) | 0 |
F5 | Sumpower | (-1.1) | 0 |
F6 | Sumsquare | (-10, 10) | 0 |
Function | ABC | GABC | GBABC | RABC | |
F1 | Mean Std |
6.33345e-13+ 4.89751e-14+ |
5.07136e-13+ 2.58946e-14+ |
9.85936e-14+ 8.65746e-15+ |
8.26357e-14 4.29453e-15 |
F2 | Mean Std |
4.01418e-12+ 3.77788e-12+ |
1.03324e-13+ 1.16972e-14+ |
8.13825e-14+ 8.65482e-15+ |
5.10703e-15 5.80934e-15 |
F3 | Mean Std |
8.04328e-15+ 7.47394e-15+ |
2.8544e-15+ 4.4677e-16+ |
1.14164e-15+ 5.13749e-17+ |
1.07917e-15 8.23919e-17 |
F4 | Mean Std |
7.29812e-15+ 8.75926e-17+ |
4.28892e-15+ 3.60087e-17+ |
1.34151e-15+ 1.24779e-17+ |
1.10285e-15 1.03897e-17 |
F5 | Mean Std |
3.48822e-09+ 3.43749e-09+ |
1.57483e-9+ 5.15095e-10+ |
3.42314e-16+ 6.90644e-17+ |
1.48323e-16 3.40782e-17 |
F6 | Mean Std |
5.17476e-15+ 2.52029e-15+ |
3.30387e-15+ 1.26809e-15+ |
1.47304e-15+ 1.95032e-16+ |
1.11128e-15 2.73786e-17 |
1:Set the number of solution parameters (D), the number of populations (SN), the maximum number of cycles (MaxCycle), and the "limit" value; randomly generate candidate solutions (X=[Xji]SN×D); Choose PI as the performance index. |
2: Calculate the fitness value of the initial solution fitx using equation (22), Record the optimal solution Xbest 3: cycle=0, count =0, 4: while (cycle < = MaxCycle) 5: *Employed bee stage* 6: for i=1 to SN 7:Generated the new candidate solution V using equation (20). Calculate the fitness value of the new solution fitv using equation (22). 8: if fitv > fitx, then X= V, count=0; 9: else count=count +1; 10: end if 11: Record the optimal solution Xbest; 12: end for 13: Calculate the fitness values of nectar sources and probability Pi by using equation (21) 14: *Follower bee stage* 15: m =0, n=0 16: while (m < SN) 17: Generate a random number rand between 0 and 1 18: if rand < Pi, then m = m +1; repeat employed bee stage; 19: end if 20: n=n+1 21: if n > SN then n=1; 22: end if 23: end while 24: * Scout bee stage * 25: for i=1 to SN 26: if count > = limit, then Generated the new candidate solution X using equation (17). 27: end if 28: Record the optimal solution Xbest = [Kp, Ki, Kd]; 29: end for 30: cycle = cycle+1; 31: end while 32: output Xbest = [Kp, Ki, Kd] |
PI | Kp | Ki | Kd | |
RABC-PID | 582.6 | 47.9215 | -0.680688 | 0.515515 |
PSO-PID | 668.3 | 32.9551 | -4.120694 | 0.168747 |
DE-PID | 665.8 | 20.6111 | -3.454611 | 0.163633 |
GA-PID | 755 | 27.8895 | -4.856542 | 0.280775 |
PI | Kp | Ki | Kd | |
RABC-PID | 483.3 | 24.277 | 2.38791 | 1.08791 |
PSO-PID | 490 | 14.7872 | 2.87741 | 0.82875 |
DE-PID | 489.8 | 12.966 | 2.01063 | 0.63332 |
GA-PID | 507 | 16.5482 | 2.0578 | 0.94521 |
PI | Kp | Ki | Kd | |
RABC-PID | 101.1 | 39.586 | -5.8 | 0.758091 |
PSO-PID | 103.5 | 30.986 | -4.58 | 0.75938 |
DE-PID | 104 | 35.972 | -4.23 | 0.889 |
GA-PID | 103.8 | 32.675 | -4.09 | 0.956 |
GA-PID | DE-PID | PSO-PID | RABC-PID | ||
Square wave | Mean Std |
0.3256 0.5100 |
0.2603 0.5011 |
0.2696 0.4833 |
0.1683 0.4687 |
Triangle wave | Mean Std |
0.1120 0.1588 |
0.0877 0.1640 |
0.0874 0.1627 |
0.0570 0.1451 |
Sine wave | Mean Std |
0.0050 0.0027 |
0.0045 0.0024 |
0.0045 0.0024 |
0.0011 8.6818e-04 |
GA-PID | DE-PID | PSO-PID | RABC-PID | ||
Square wave | Mean Std |
0.3522 0.5455 |
0.3497 0.5503 |
0.3517 0.5504 |
0.2293 0.5313 |
Triangle wave | Mean Std |
0.1450 0.1679 |
0.1067 0.1650 |
0.1061 0.1643 |
0.0458 0.1342 |
Sine wave | Mean Std |
0.0073 0.0055 |
0.0069 0.0051 |
0.0069 0.0051 |
0.0026 0.0033 |
GA-PID | DE-PID | PSO-PID | RABC-PID | ||
Square wave | Mean Std |
0.3270 0.5515 |
0.4144 0.5832 |
0.4124 0.5830 |
0.2780 0.5188 |
Triangle wave | Mean Std |
0.1665 0.1682 |
0.1334 0.1720 |
0.1328 0.1710 |
0.0503 0.1377 |
Sine wave | Mean Std |
0.0089 0.0073 |
0.0087 0.0072 |
0.0087 0.0072 |
0.0038 0.0035 |
GA-PID | DE-PID | PSO-PID | RABC-PID | ||
Square wave | Mean Std |
0.5834 0.4766 |
0.4724 0.6065 |
0.4749 0.6062 |
0.3193 0.3008 |
Triangle wave | Mean Std |
0.1879 0.1739 |
0.1579 0.1776 |
0.1580 0.1775 |
0.0560 0.1419 |
Sine wave | Mean Std |
0.0115 0.0091 |
0.0107 0.0088 |
0.0107 0.0088 |
0.0050 0.0044 |