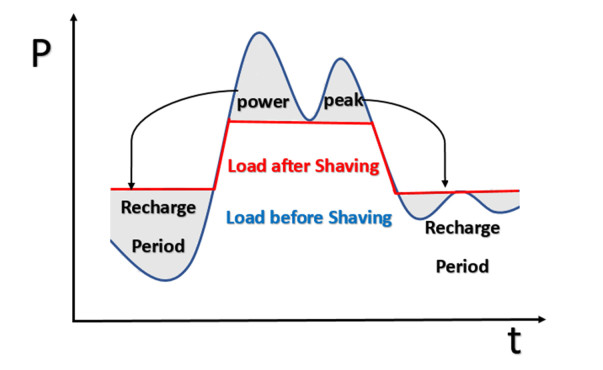
Peak shaving techniques have become increasingly important for managing peak demand and improving the reliability, efficiency, and resilience of modern power systems. In this review paper, we examine different peak shaving strategies for smart grids, including battery energy storage systems, nuclear and battery storage power plants, hybrid energy storage systems, photovoltaic system installations, the real-time scheduling of household appliances, repurposed electric vehicle batteries, uni- and bi-directional electric vehicle charging, the demand response, the time-of-use pricing, load shedding systems, distributed generation systems and energy-efficient management. We analyze the potential of each strategy to reduce peak demand and shift energy consumption to off-peak hours, as well as identify the key themes critical to the success of peak shaving for smart grids, including effective coordination and communication, data analytics and predictive modeling and clear policy and regulatory frameworks. Our review highlights the diverse range of innovative technologies and techniques available to utilities and power system operators and it emphasizes the need for continued research and development in emerging areas such as blockchain technology and artificial intelligence. Overall, the implementation of peak shaving strategies represents a significant step toward a more sustainable, reliable and efficient power system. By leveraging the latest technologies and techniques available, utilities and power system operators can better manage peak demand, integrate renewable energy sources, and create a more reliable and secure grid for the future. By discussing cutting-edge technologies and methods to effectively manage peak demand and incorporate renewable energy sources, this review paper emphasizes the significance of peak shaving strategies for smart grids as a crucial pathway towards realizing a more sustainable, dependable and efficient power system.
Citation: Syed Sabir Hussain Rizvi, Krishna Teerth Chaturvedi, Mohan Lal Kolhe. A review on peak shaving techniques for smart grids[J]. AIMS Energy, 2023, 11(4): 723-752. doi: 10.3934/energy.2023036
[1] | K. M. S. Y. Konara, M. L. Kolhe, Arvind Sharma . Power dispatching techniques as a finite state machine for a standalone photovoltaic system with a hybrid energy storage. AIMS Energy, 2020, 8(2): 214-230. doi: 10.3934/energy.2020.2.214 |
[2] | Dalong Guo, Chi Zhou . Potential performance analysis and future trend prediction of electric vehicle with V2G/V2H/V2B capability. AIMS Energy, 2016, 4(2): 331-346. doi: 10.3934/energy.2016.2.331 |
[3] | Sulabh Sachan . Integration of electric vehicles with optimum sized storage for grid connected photo-voltaic system. AIMS Energy, 2017, 5(6): 997-1012. doi: 10.3934/energy.2017.6.997 |
[4] | Ryuto Shigenobu, Oludamilare Bode Adewuyi, Atsushi Yona, Tomonobu Senjyu . Demand response strategy management with active and reactive power incentive in the smart grid: a two-level optimization approach. AIMS Energy, 2017, 5(3): 482-505. doi: 10.3934/energy.2017.3.482 |
[5] | Hassan Shirzeh, Fazel Naghdy, Philip Ciufo, Montserrat Ros . Stochastic energy balancing in substation energy management. AIMS Energy, 2015, 3(4): 810-837. doi: 10.3934/energy.2015.4.810 |
[6] | Virendra Sharma, Lata Gidwani . Optimistic use of battery energy storage system to mitigate grid disturbances in the hybrid power system. AIMS Energy, 2019, 7(6): 688-709. doi: 10.3934/energy.2019.6.688 |
[7] | Mohamed Hamdi, Hafez A. El Salmawy, Reda Ragab . Optimum configuration of a dispatchable hybrid renewable energy plant using artificial neural networks: Case study of Ras Ghareb, Egypt. AIMS Energy, 2023, 11(1): 171-196. doi: 10.3934/energy.2023010 |
[8] | Aaron St. Leger . Demand response impacts on off-grid hybrid photovoltaic-diesel generator microgrids. AIMS Energy, 2015, 3(3): 360-376. doi: 10.3934/energy.2015.3.360 |
[9] | François KREMER, Stéphane RAEL, Matthieu URBAIN . 1D electrochemical model of lithium-ion battery for a sizing methodology of thermal power plant integrated storage system. AIMS Energy, 2020, 8(5): 721-748. doi: 10.3934/energy.2020.5.721 |
[10] | Khuthadzo Kgopana, Olawale Popoola . Improved utilization of hybrid energy for low-income houses based on energy consumption pattern. AIMS Energy, 2023, 11(1): 79-109. doi: 10.3934/energy.2023005 |
Peak shaving techniques have become increasingly important for managing peak demand and improving the reliability, efficiency, and resilience of modern power systems. In this review paper, we examine different peak shaving strategies for smart grids, including battery energy storage systems, nuclear and battery storage power plants, hybrid energy storage systems, photovoltaic system installations, the real-time scheduling of household appliances, repurposed electric vehicle batteries, uni- and bi-directional electric vehicle charging, the demand response, the time-of-use pricing, load shedding systems, distributed generation systems and energy-efficient management. We analyze the potential of each strategy to reduce peak demand and shift energy consumption to off-peak hours, as well as identify the key themes critical to the success of peak shaving for smart grids, including effective coordination and communication, data analytics and predictive modeling and clear policy and regulatory frameworks. Our review highlights the diverse range of innovative technologies and techniques available to utilities and power system operators and it emphasizes the need for continued research and development in emerging areas such as blockchain technology and artificial intelligence. Overall, the implementation of peak shaving strategies represents a significant step toward a more sustainable, reliable and efficient power system. By leveraging the latest technologies and techniques available, utilities and power system operators can better manage peak demand, integrate renewable energy sources, and create a more reliable and secure grid for the future. By discussing cutting-edge technologies and methods to effectively manage peak demand and incorporate renewable energy sources, this review paper emphasizes the significance of peak shaving strategies for smart grids as a crucial pathway towards realizing a more sustainable, dependable and efficient power system.
The transition toward a sustainable energy future has placed significant demands on the electric power grid, with the need to manage peak demand and integrate renewable energy sources posing new challenges for utilities and power system operators [1]. Smart grids, which leverage advanced sensing, communication and control technologies, offer a promising solution for improving grid reliability, efficiency and resilience.
Peak shaving is a key strategy for smart grids that aims to reduce peak demand and shift energy consumption to off-peak hours [2]. This can help to reduce the need for expensive peak power generation and infrastructure upgrades, while also increasing the integration of renewable energy sources into the grid.
In recent years, there has been significant research and development in the area of peak shaving strategies for smart grids, with a range of innovative technologies and techniques emerging [3]. In this review paper, we explore 10 different peak shaving strategies that have demonstrated some potential to reduce peak demand, including the use of battery energy storage systems (BESSs), nuclear and battery storage power plants, hybrid energy storage systems, photovoltaic (PV) system installations, real-time scheduling of household appliances, repurposed electric vehicle (EV) batteries, uni- and bi-directional EV charging, a demand response, time-of-use pricing, load shedding, distributed generation and energy-efficient management [4]. Through a comprehensive analysis of the latest research and case studies, we aim to provide a comprehensive overview of the strengths and limitations of each peak shaving strategy and identify areas for further research and development. By understanding the potential of these strategies, we can better equip utilities and power system operators to meet the challenges of the modern energy landscape and create a more sustainable, resilient and efficient power system.
In order to increase the dependability, effectiveness, and resilience of contemporary power systems, our goal for this review paper was to give a thorough examination of alternative peak shaving tactics for smart grids. The following crucial actions were part of the research roadmap:
1. Literature review: We did a thorough evaluation of the body of knowledge regarding peak shaving techniques for smart grids. To assemble a diverse range of thoughts and opinions, we have included academic papers, research articles, technical reports and industrial publications in this review.
2. Strategy identification: We identified and chose a broad range of peak shaving tactics for investigation from the literature review. In addition to applying a demand response system, time-of-use pricing, load shedding, distributed generation and energy-efficient management, these strategies also include BESSs, nuclear and battery storage power plants, hybrid energy storage systems, PV system installations, the real-time scheduling of home appliances, repurposed EV batteries and uni- and bi-directional EV charging.
3. Evaluation metrics: We created a set of evaluation parameters to gauge each peak shaving strategy's potential. These measures center on how well they worked to lower peak demand, move energy use to off-peak times and improve the grid's overall efficiency and reliability.
4. Data collection and analysis: We gathered pertinent data and case studies for each technique that was selected in order to assess how well it worked in practice. To evaluate the benefits and drawbacks of each option, we made comparisons between the outcomes and those of conventional methods in practice.
5. Identifying key themes: We paid close attention to find the important themes that were essential to peak shaving in smart grids throughout the investigation. These topics include efficient coordination and communication across grid elements, the use of data analytics and predictive modeling to enhance peak shaving tactics and the significance of clear legislative and regulatory frameworks to ease their integration.
6. Technology and innovation focus: We highlight the examination of cutting-edge tools and methods accessible to utilities and power system operators as a key component of the research agenda. This included a look at new fields like artificial intelligence and blockchain technology in the context of peak shaving applications.
7. Future research opportunities: We highlighted topics that merit additional research and suggest future research directions in the review paper's conclusion. These include investigating new peak shaving applications, maximizing their use with renewable energy sources and creating more advanced and flexible peak shaving technologies.
We set out to give a thorough and informative analysis of peak shaving tactics for smart grids by adhering to this research roadmap, highlighting their importance in attaining sustainable, dependable and effective power systems. We want to provide useful information for utilities and power system operators, policymakers and researchers in their quest for a more robust and secure grid for the future through a thorough review of alternative methodologies and major issues.
A load profile is a visual representation of your energy consumption that shows daily or seasonal variations as energy use varies throughout the year. By looking at this graph, you can see trends in your electricity use, including peak and off-peak hours, such as higher use at night when you are at home and lower usage during the day when you are at work. You can find possibilities to lower your use of electricity by analyzing the load profile, which could enable you to reduce the amount you pay for electricity.
The cyclical nature of load or demand over time is described by a load profile. It can be seen as a graph that depicts the peaks and valleys of demand over the course of a day, a week, or a year. Load profiles come in a variety of forms. Consumption, or the overall amount of electricity utilized, is represented by the area under the demand line. Demand varies within each day of the daily load profiles; however, daily load profiles for most days are the same. Demand changes from season to season dominate any daily or weekly variations in a seasonal load profile. Demand varies more from one day to the next than it does from week to week in a weekly load profile [5].
Load profiles serve as crucial blueprints for effective peak shaving strategies, aiding in pinpointing peak demand periods, optimizing energy consumption schedules, and facilitating demand response actions. By analyzing these profiles, organizations can strategically implement load shifting, energy storage sizing, and renewable energy integration to curtail peak demand during critical periods. Moreover, load profiles enable data-driven decision-making, guiding the development of optimal control algorithms and facilitating the evaluation of strategy effectiveness over time, ultimately contributing to more efficient energy usage and grid stability.
Now that we understand what constitutes demand charges and why they exist, let us examine how organizations might limit them. As previously stated, peak shaving offers the most efficient method of managing power expenses for users that incur demand charges. Peak shaving lowers and mellows peak loads, which reduces or eliminates the short-term demand jump that creates additional demand costs. There are several strategies for peak shaving, but some are superior to others, and the approaches adopted should be optimal for the load profile and electricity demands of the enterprise [6]. Figure 1 shows a load curve transformed by peak shaving reduce peak demand.
In electrical engineering, a load profile is a graph of the variation in the electrical load versus time. A load profile will vary according to customer type (typical examples include residential, commercial and industrial), temperature and holiday season. Power producers use this information to plan how much electricity they will need to make available at any given time [7].
In the context of this review paper, the future scope of peak shaving emerges as a promising landscape characterized by technological advancements and evolving energy dynamics. The integration of smart grid technologies, real-time data analytics and machine learning presents the potential for more precise peak demand forecasting, enabling organizations to optimize peak shaving strategies proactively. Advanced energy storage solutions, including next-generation batteries and innovative thermal storage systems, offer the promise of heightened efficiency and cost-effectiveness in peak shaving efforts. The incorporation of demand response programs into broader energy markets holds the prospect of enabling the development of dynamic and responsive peak shaving practices. As renewable energy adoption gains momentum, the coupling of renewable generation with peak shaving strategies emerges as a conduit not only for reducing peak demand but also for facilitating the seamless integration of intermittent renewables into the grid. The synergy between Internet of Things (IoT) devices and energy-management systems could drive automated real-time load management and adaptive peak shaving techniques. Delving into the socioeconomic underpinnings influencing user engagement and participation in demand-side management programs enriches the potential for effective implementation. Ultimately, the future scope of peak shaving encapsulates an innovative trajectory, embracing advanced technologies and holistic approaches to fortify energy efficiency, cost reduction, grid stability, and a sustainable energy future.
Peak shaving with a BESS involves using stored energy in batteries to offset peak electricity demand. The BESS charges during low-demand periods, like off-peak hours or when renewable energy is abundant, and it discharges during peak periods to reduce strain on the grid and minimize electricity costs. By flattening the demand curve and providing grid support, the BESS enhances grid stability, improves energy reliability and supports the integration of renewable energy sources. This approach offers economic benefits, environmental advantages and promotes efficient and sustainable energy management in a smart grid [8].
Uddin et al. [9] describe a peak shaving technique using a BESS. Peak shaving is an effective method for demand management, which involves charging the BESS during periods of low demand and discharging it during peak demand hours. They applied a decision tree to control peak demand. The algorithm was tested on a genuine microgrid. This paper sheds light on an islanded microgrid with a BESS for peak shaving. This study shows that a decision tree-based algorithm may lower peak demand for isolated microgrids. Figure 2 Shows a peak shaving scheme with a BESS.
This method can handle many load conditions in real-world scenarios, proving its usefulness [10]. Comparative peak shaving approaches reveal that the recommended strategy outperforms standard strategies when just one generator is running. It shows how effectively the recommended algorithm works to offer peak shaving for an islanded microgrid, when typical techniques have restrictions. In this work, the appropriate BESS size has not been explored, which may increase the recommended technique's capital costs. Future studies might include the optimal BESS size.
The integration of renewable energy sources and sustainability are greatly aided by electrochemical batteries; yet, battery deterioration might limit energy flexibility and the uptake of renewable energy sources. The operation of integrated thermal storage and intelligent battery charging and discharging methods within integrated multi-energy systems has not yet been thoroughly explored, despite the fact that existing research has extensively examined ways to slow down battery degradation, focusing on electrode materials, electrolytes, and temperature control. This is especially true given the nonlinear degradation mechanism of batteries [11]. BESSs can store excess electricity generated during periods of low demand or high renewable energy production. By charging the batteries during these times and discharging them during peak demand hours, BESSs can effectively level out the load on the grid. Peaks in electricity demand can strain the power grid, potentially leading to voltage fluctuations and frequency imbalances. By deploying a BESS for peak shaving, the grid's stability can be improved, as the batteries act as a buffer to absorb excess power during low-demand periods and release it when demand surges.
The future scope of research on peak shaving with a BESS involves refining control algorithms and predictive models for tailored efficiency, exploring emerging battery technologies for enhanced energy density, assessing environmental impacts, and evaluating scalability across different contexts. Integrating a BESS with renewables, understanding the economic and regulatory factors and addressing user engagement are also crucial dimensions. This multifaceted scope encompasses technical, environmental, economic, regulatory and behavioral considerations for advancing energy-management strategies.
Achieving peak shaving via a combination of nuclear and battery energy storage power plants involves using excess electricity generated by nuclear power plants during low-demand periods to charge BESSs. The stored energy is then discharged during peak demand periods, supplementing the nuclear power plant's capacity and reducing the need for expensive peaker plants or fossil fuel-based generation. This approach optimizes electricity supply, enhances grid stability, and promotes a cleaner and more sustainable energy system by efficiently managing peak demand periods [12].
Chen et al. [13] conducted a case study of Hainan, where nuclear and battery energy storage power plants are working in collaboration to store surplus power generated during off-peak hours and supply it during high-demand periods, their research explores the economic feasibility of a battery-based energy storage power station working collaboratively with nuclear power for peak shaving. It proposes an innovative feasible solution framework for battery type selection and construction scale estimation, which can be applied to other stability problems as well. The combined operating mode was found to enhance the safety and flexibility of nuclear power significantly.
Economic comparison results have revealed that the 270-MW lithium iron phosphate battery energy storage power station performs the best in terms of internal rate of return, payback period, levelized cost of electricity and overall benefit. Sensitivity analysis indicates that a lithium iron phosphate battery energy storage power station's benefits become more stable under uncertain parameters, while the impact of cost parameters on economic indicators becomes more noticeable. This suggests that the difference in opinion between energy storage price and the power market is becoming more evident [14,15].
The proposed solution framework will be further refined in future studies to address frequency management and reactive power regulation constraints, as well as residual rate and financing ratio issues. Figure 3 shows the flow of energy in nuclear and battery storage plants.
By capitalizing on the energy storing capacity of batteries, the proposed optimization process offers a technically sound solution to enhance the grid integration of nuclear power plants. The ability to store surplus power generated during off-peak hours and supply it during high-demand periods maximizes revenue for power system operators and improves the overall power system capacity and grid stability. This research paves the way for a more sustainable and economically viable energy future.
The future scope of research on peak shaving with nuclear and battery energy storage power plants encompasses optimizing load-following strategies, exploring hybrid systems, assessing environmental impacts and safety, evaluating economic feasibility and regulatory aspects, studying grid services and renewable integration potential and understanding societal acceptance. This multifaceted scope addresses technical, environmental, economic, regulatory and societal dimensions, highlighting the potential of this innovative energy-management strategy.
The development of technology to regulate wind power and deal with its variations in the electrical grid has recently attracted the attention of researchers and power system operators. Peak shaving with a hybrid energy storage system has emerged as a promising solution. Integrating energy storage systems with wind energy is one efficient technique [16]. In order to implement this plan, electricity supply and demand must be carefully balanced during peak times.
The hybrid system, which may incorporate components like battery systems, flywheel energy storage, or super capacitors, is used to store excess energy during off-peak hours when wind energy production exceeds demand [17]. The stored energy is then released during times of high demand to augment the grid, effectively reducing volatility and stabilizing the supply of electricity. The grid operator can maximize the use of renewable wind energy while providing a stable and dependable power supply thanks to the integration of several energy storage technologies inside the hybrid system. As a result, the grid operates more effectively, using less traditional electricity during peak hours, and successfully integrating renewable energy sources.
Using the harmonic analysis method, Zhao et al. established a preliminary capacity allocation strategy for hybrid systems for energy storage for power system peak shaving. To estimate the capacity allocation of a hybrid energy storage system, four components were dissected from an imbalanced power series: outer-day, intra-day, short-term and very short-term. The results show that the power and energy ratings of hybrid storage systems for electricity are substantially lower in the partial smoothing mode than in the complete smoothing mode, resulting in considerable capital savings [18]. Conventional power plants, for example, may smooth out the disassembled parts more effectively and for a longer duration. By the year 2060, it is anticipated that using renewable energy sources to electrify buildings and transportation will significantly reduce carbon emissions by 30%. In the currently available literature, there are not many state-of-the-art assessments of cutting-edge materials and methods for electrochemical batteries that can help communities to make the transition to carbon-neutral communities [19].
The strategy proposed by Korpaas et al. is an early method for allocating capacity in hybrid systems for energy storage [20]. To further quantify hybrid power storage, more study on economic gain evaluation and power system dynamic stability evaluation can be carried out in the future. Figure 4 demonstrates a peak shaving operation using a hybrid system for energy storage.
Peak shaving with a hybrid energy storage system is a promising approach to address wind power fluctuations and enhance the reliability of the power grid. Future growth in this area depends on technological advancements in energy storage systems, increased wind energy penetration, integration with smart grids, supportive policies and cost reduction of energy storage. Collaborative research efforts and the ability to provide grid flexibility and resilience will also contribute to its success.
The future scope of research on peak shaving with hybrid energy storage systems involves optimizing control strategies, integrating predictive algorithms, assessing environmental impacts, evaluating economic feasibility and regulatory aspects, exploring versatility across applications, enhancing renewable integration and considering user engagement. This comprehensive scope addresses technical, environmental, economic, regulatory and societal dimensions, underscoring the potential of hybrid systems for innovative energy-management strategies.
Peak shaving with a PV system entails carefully controlling solar energy production and consumption to lower the grid's peak electricity demand during times of high usage. Installation of PV systems, solar energy production, load monitoring and potential energy storage are used to achieve this [21]. The PV system's ability to interact with the grid and move non-critical loads allows it to reduce electricity usage. Peak shaving is even more effective when the smart grid is included, and ongoing monitoring helps to ensure a reliable and sustainable power supply.
Ceran et al. [22] investigated how Poland could use PV systems in office buildings to cut down on the amount of electricity used at peak times. An artificial neural network was used to figure out how much power was consumed by office buildings because there was not any history of how much power was used there. The study looked at how PV modules could be tilted and oriented, as well as how they could get old and break down. There was a way to figure out how peak shaving would affect the national power system [23,24]. The monthly balancing factor for peak load was used to figure this out. In addition, the impact of PV installations on the grid infrastructure was investigated by figuring out how long transformers would last. The development of PV thermal systems, which provide on-site generation of both electrical and thermal energy for buildings, has been encouraged by the rising daily energy demand and growing global environmental concerns. However, these systems encounter difficulties because of significant radiative and convective heat losses, which have a negative impact on their total efficiency (which is often below 70%) [25].
Because of Poland's current transition to a decentralized energy system, the proposed method for analyzing the impact of PV installations on peak shaving for the national electricity system is extremely important at this time [26]. It is possible to observe a systematic rise in the proportion of renewable energy sources, which is occurring at the expense of coal-fired power plants, which are being phased out of operation. In the future, researchers will look at how PV systems can cut down on the amount of electricity used at peak times in the grid by taking into account systemic energy storage Figure 5 illustrates how an artificial neural network can be used to predict the electrical load based on weather data in order to realize peak shaving with a PV installation system. Figure 6 shows how the electrical power output of a PV system is estimated to achieve peak shaving with a PV installation system.
As researchers, we think that there are several ways to enhance the peak shaving method with PV system installation. The improvement of solar energy forecasting, the use of dynamic load management techniques, the optimization of energy storage technologies, the investigation of grid-interactive systems, the use of hybrid PV and energy storage setups, the integration of smart grid technologies, the promotion of demand-response initiatives, the promotion of supportive regulations and the implementation of real-world case studies are a few of these. By concentrating on these upgrades, we may achieve peak shaving that is more efficient and long lasting, furthering the integration of PV systems into the energy system.
The future scope of research on peak shaving with PV system installation involves optimizing control strategies, exploring hybrid PV-battery systems, assessing economic viability, integration with microgrids and smart grids, evaluating environmental impact, addressing regulatory considerations and understanding user behavior. This comprehensive scope encompasses technical, economic, regulatory, environmental and user-centric dimensions, underscoring the potential of PV system-based strategies for advancing energy management and sustainability.
Peak shaving is a technique that uses smart technology to shift electricity use from peak to off-peak hours, reducing the overall demand during high-load periods. It involves real-time scheduling of household appliances. This strategy balances the electricity grid, reduces costs, enhances grid stability, and encourages energy efficiency by installing smart meters, recognizing peak hours, and programming appliances accordingly [27]. Additionally, taking part in demand response initiatives may provide customers with financial incentives while advancing sustainability and environmental goals.
Caprino et al. [28] in their paper presented a real-time scheduling-based technique for electric load management that was developed in-house. The reduction of peak loads, as well as the application of service constraints on these controlled loads, have been the primary objectives. In order to assess its performance, the method was been applied to a set of household appliances that were precisely modeled for the scheduling approach in question. In order to satisfy the timing and service constraints, an amalgamation of a level packing strategy, an insubstantial preemption strategy, and a carefully chosen set of timing restrictions was also applied. Simulations of a single apartment demonstrated that the proposed techniques could be applied in a variety of realistic scenario [29]. As a result, the peak load was brought down by 8% on average and by 41% when compared to the worst-case scenario. Cooperative arrangements, i.e., the situations in which any time scheduler is able to control a complex of apartments as a single virtual electricity consumer [30], increase the benefits derived from the arrangement. The scheduling method, when it takes into account the coalition of several apartments, can cut peak load by up to 46%. The findings of the research set the foundation for electrification and clear a path towards sustainability and carbon neutrality while also providing conceptual frameworks, prospects and insights into technological obstacles [31]. Figure 7 shows the general scheme for peak shaving for home energy management systems using real-time control methods.
Investing in cutting-edge smart grid technology, utilizing artificial intelligence and machine learning for precise predictions, integrating renewable energy sources, utilizing IoT devices, providing incentives to consumers, providing education, supporting beneficial policies, establishing interoperability standards, and embracing emerging technologies are all necessary to improve peak shaving through real-time scheduling of home appliances. These improvements are meant to increase energy efficiency, reduce peak demand and build a more resilient and sustainable energy infrastructure.
The future scope of research on peak shaving through the real-time scheduling of household appliances involves developing advanced scheduling algorithms, integrating IoT devices, analyzing user behavior, assessing economic viability, evaluating grid impact, exploring scalability and addressing regulatory aspects. This comprehensive scope covers technical, user-centric, economic, grid-related, and regulatory dimensions, emphasizing the potential of real-time scheduling strategies for optimizing household energy consumption and peak demand reduction.
Peak shaving for a smart grid with repurposed for batteries involves using the stored energy in EV batteries during low-demand periods to supplement the grid's power supply and reduce strain during peak periods. This integration requires a smart grid system for efficient communication and management [32]. The process offers benefits such as cost savings, reduced greenhouse gas emissions, and incentivizing EV owners to participate in peak shaving programs. As technology advances and EV adoption increases, this practice is expected to play a significant role in improving grid reliability and sustainability.
Lee et al. [33] considering the literature reviewed in their article, discarded EV batteries that had been reused without public notice. EV batteries have become the market's most important segment. These batteries are gaining attention for their less demanding uses (second-life applications). Government and policymaker initiatives could help to spread the use of second-life batteries [34]. Encouraging SLB use could reduce EV ownership and operation costs. The existence of a glut of discarded EV batteries is lowering their price. The government should consider a standardization procedure and policies governing SLB processes. Tax exemptions will motivate consumers to use SLBs in second-life applications. Due to SLB's low cost and long lifespan for second-life applications, the market is expected to grow. SLBs will help to lower the cost of EVs and reduce greenhouse gas emissions in the coming decades. EV batteries are a new source of income for battery-repurposing companies and recyclers. Figure 8 showing life cycle of a battery.
Peak shaving with repurposed EV batteries for a smart grid offers significant benefits, including reduced strain on the grid, cost savings and less greenhouse gas emissions. However, to optimize its effectiveness, improvements are needed in battery management to improve longevity and performance, as well as for standardization to ensure interoperability, advanced grid stability control and scalability to handle increasing EV adoption. Additionally, robust cybersecurity measures, consumer engagement strategies and sustainable end-of-life approaches for battery recycling are essential. The technology's economic viability must be carefully considered alongside supportive regulatory frameworks and effective grid planning to ensure successful integration and widespread adoption, thus contributing to a more sustainable and reliable energy system.
The future research scope involves confirming the technical feasibility of repurposing EV batteries for peak shaving through rigorous testing, the optimization of battery management strategies, the evaluation of economic viability, the assessment of environmental impact, the introduction of regulatory frameworks and understanding user acceptance. This comprehensive scope encompasses technical, economic, environmental, regulatory and user-centric dimensions, highlighting the potential of repurposed EV batteries as a sustainable solution for efficient power peak shaving.
Peak shaving for EV charging, achieved through model predictive control (MPC) [35], offers cost reduction benefits for both uni-directional and bi-directional charging systems. In uni-directional charging, MPC optimizes the charging process based on forecasted electricity prices and grid demand, allowing EVs to charge during off-peak hours when electricity costs are lower, thereby reducing expenses for EV owners and reducing the strain on the grid during peak periods. In bi-directional charging, MPC enables a dynamic approach, deciding whether to charge or discharge the EV battery based on real-time electricity prices and grid conditions. During peak demand, bi-directional charging can discharge stored energy back to the grid, reducing reliance on traditional power plants and earning incentives for EV owners, which thus results in cost-efficient energy management.
In [36] two different MPC methods for charge scheduling are presented. One method enables peak and cost reduction for the uni-directional and bi-directional charging of EVs. The other algorithm proposes a multiobjective function, which includes bill curtailment as well as a component to improve the power profile. Bi-directional charging strategies for EVs significantly reduce peak power consumption [37]. They also allow peak energy levels to go back to where they were before EVS were added to the local energy system.
The minimum state-of-charge (SOC) has been discovered to be a significant factor in the ability of bi-directional charging to improve the power profile when used in conjunction with other technologies. Increasing the minimum SOC makes it more difficult to charge, which means less peak power reduction.
MPCs can be used to conduct a thorough investigation into the impact of smart unidirectional and bidirectional charging on asset size (such as solar PV systems) and cost estimates [38]. Future research might focus on increasing the optimizability of the MPCs in order to enable the operation of a greater number of EVs. User-acceptance studies can help us to figure out if EV drivers want to use smart charge scheduling or not.
A novel life-cycle battery carbon intensity criterion has been introduced to measure the overall carbon footprint of batteries throughout their lifespan. The criterion is employed to evaluate the decarbonization potential of various renewable energy sources and the interactions between battery-reusing and vehicle-to-building (V2B) and building-to-vehicle applications. The findings indicate that repurposing retired EV batteries for buildings increases the demand coverage ratio from 0.480 to 0.504 and the renewable penetration ratio from 0.502 to 0.531 [39]. Figure 9 presents a schematic of the uni- and bi-directional charging of EVs.
To improve peak shaving via MPC for EV charging, advanced algorithms for better forecasting of electricity prices and grid demand are needed, along with real-time data integration from various sources. Implementing smart grid infrastructure, V2G technology and flexible energy pricing mechanisms will enhance grid flexibility and encourage EV owners to participate. Consumer engagement through education and incentives, coupled with advanced battery-management systems and scalable solutions, will optimize charging schedules and extend battery life. Additionally, supportive policies and robust cybersecurity measures are essential to ensure the widespread adoption and success of this technique, ultimately leading to more efficient energy consumption, reduced costs and improved grid stability.
The future research scope involves optimizing MPC algorithms for both uni- and bi-directional EV charging, exploring bi-directional charging integration, assessing economic feasibility, evaluating environmental impact, addressing regulatory aspects and understanding user engagement. This comprehensive scope encompasses technical, economic, environmental, regulatory and user-centric dimensions, underscoring the potential of advanced charging strategies for peak reduction, cost savings, and grid support.
Demand response-based peak shaving for a smart grid involves proactive demand management through programs and incentives that encourage consumers to reduce electricity usage during peak periods [40]. Real-time communication, time-of-use pricing and automated control systems are utilized to inform and assist consumers in adjusting their energy consumption. Integrating distributed energy resources further optimizes the grid's stability, while load shifting shifts energy-intensive activities to off-peak hours. This strategy benefits both utilities and consumers, as it efficiently manages demand, reduces costs and contributes to a more sustainable and reliable energy system.
Shen et al. [41] presented research on an operational scheduling issue for a microgrid with a variety of operational incentives, rewards and restrictions. The optimal scheduling of batteries and diesel generators is part of the issue formulation, which also incorporates peak time rebate (PTR) incentives and demand response programming for suitable loads.
According to a previous study, PTR programming may significantly reduce the peak load on the grid tie line and the microgrid can actively adapt to the main grid [42]. Peak shaving of the main grid is also significantly aided by demand response programming of controllable loads. The study also examined PSQI and PSAI, two peak-shaving indices, and revealed that the suggested approach can successfully reduce peak loads on the grid tie line while maintaining the microgrids ideal schedule. The study's conclusions act as cutting-edge recommendations for system designers, planners, price policymakers and energy managers, facilitating cooperative efforts to create a smart grid area that is transformed in terms of sustainability [43]. Figure 10 shows the interactive connection between the microgrid and grid.
In conclusion, the study emphasizes the significance of using incentives and demand response programming to realize peak shaving for smart grids, and it shows the effectiveness of these techniques in terms of reducing peak demand and enhancing smart grid performance. Two important pillars in the path to zero-energy buildings are energy-efficient structures and renewable energy sources, both of which are essential for accelerating the energy paradigm shift toward carbon neutrality in the construction industry. Building envelopes have considerable potential to reduce cooling and heating loads by enhancing thermal insulation performance and utilizing solar radiation through the use of PVs, which produce renewable energy because of their significant heat loss and large surface areas [44].
The future research scope involves optimizing demand response algorithms, integrating IoT devices and real-time data analytics, assessing economic viability, evaluating environmental benefits, addressing regulatory aspects, understanding user behavior, and enhancing smart grid functionalities. This comprehensive scope encompasses technical, economic, environmental, regulatory, and user-centric dimensions, underscoring the potential of demand response strategies for efficient peak shaving, sustainable energy management, and smart grid optimization.
The time-of-use strategy for peak shaving for a smart grid involves varying electricity prices based on the time of day to encourage consumers to shift their energy consumption away from peak hours and toward off-peak periods. By dividing the day into different pricing tiers and using smart meters for real-time information, consumers are incentivized to adjust their energy-intensive activities to off-peak hours when electricity costs are lower. Through consumer awareness, load management, and the use of smart appliances, the time-of-use strategy helps utilities operators to manage demand, reduce strain on the grid during peak period, and optimize the use of available energy resources [45]. This benefits consumers by offering potential cost savings on electricity bills, and it contributes to a more efficient and sustainable energy system overall.
The authors of [46] suggest a multimarket strategy for lowering the daily peak demand for distribution systems in smart grid architecture. The strategy makes use of market mechanisms and communication resources. Based on the time-of-use pricing trends and the quantity of market entities, several situations were investigated. The findings suggest that time-of-use schemes may not always be effective in reducing peak demand, and that there is a need for judicious pricing. The authors successfully reduced the overall system peak without any peak rebound by implementing an optimization scheme to choose the right time-of-use prices.
In addition to the time-of-use strategy, one article suggests a multimarket solution [47] that makes use of various market entities with improved peak shaving price patterns. In order to reduce peak simultaneity, this method divides loads into numerous sets and isolates each set's peak. The outcomes demonstrate that this strategy affords system operators more control, leading to gentler prices and less demand-side pressure.
The study makes the case for more research to be done in order to optimize the number of market entities, load distribution among markets, and other objectives including reducing feeder phase imbalance. Overall, the multimarket strategy outlined in the study offers a practical way to lower the daily peak demand for distribution systems in smart grid architecture. Figure 11 shows the real-time pricing and real-time consumption scheme for smart grids.
To improve the time-of-use strategy for peak shaving for a smart grid, various measures can be implemented. This includes introducing more granular and dynamic pricing options to offer precise and real-time signals to consumers, utilizing automation and artificial intelligence to automate energy-intensive devices based on time-of-use pricing and integrating time-of-use pricing with demand response programs. Providing personalized feedback, offering consumer incentives, and enhancing grid stability and forecasting can increase consumer engagement and overall effectiveness. Educating consumers and ensuring regulatory support also contribute to a more efficient, engaging and successful time-of-use strategy for managing electricity demand and creating a sustainable energy system. Additionally, integrating energy storage solutions can optimize cost savings and grid resilience.
The future research scope involves optimizing time-of-use algorithms, integrating real-time data analytics, assessing economic viability, evaluating environmental benefits, addressing regulatory aspects, understanding user behavior and enhancing smart grid functionalities. This comprehensive scope encompasses technical, economic, environmental, regulatory and user-centric dimensions, emphasizing the potential of time-of-use strategies for efficient peak shaving, sustainable energy management, and smart grid optimization.
Peak shaving via load shedding for a smart grid is a demand management strategy that reduces electricity consumption during peak demand periods by intelligently shedding non-critical loads. Using real-time data and automation, the smart grid identifies peak periods and automatically prioritizes and sheds non-essential electricity use. Consumers may receive notifications to adjust their consumption during these times [48]. By balancing demand and supply, load shedding helps to maintain grid stability and prevents the need for expensive upgrades while incentivizing consumers to participate in a more resilient and cost-effective energy system.
The authors of [49] investigated frequency load-shedding techniques for a high-voltage network in New England. A hybrid strategy proved to be the best in terms of customer satisfaction, accuracy, and preventing excessive load shedding, as the authors evaluated various centralized and decentralized load-shedding algorithms.
The report also covers the usage of smart home technologies and frequency monitoring tools, which can raise consumer satisfaction levels by allowing the consumers to choose which burdens they desire to shed [50]. The suggested approach achieved high reliability and prevented nodes from making mistakes by using a timer to detect link failure, as well as a predetermined time interval for messages.
Overall, the study seems to indicate that an ideal solution for preserving generation/load balance in a high voltage network while also guaranteeing customer satisfaction and system reliability can be achieved by combining a hybrid approach with frequency load shedding and the use of cutting-edge technology, such as frequency monitoring tools and smart home systems. Figure 12 shows load shedding scheme for smart grids.
To enhance the effects of peak shaving via load shedding for a smart grid, several improvements can be made. These include the use of advanced load prioritization algorithms, real-time data utilization and the use of predictive analytics to optimize load shedding strategies and grid management. Engaging consumers through incentives, rewards and educational campaigns can boost voluntary participation. Integrating load shedding with distributed energy resources and coordinating it with other demand response measures ensures a comprehensive approach to peak demand management. Grid flexibility, efficient load-restoration strategies and reliable load-shedding systems contribute to a more efficient and consumer-friendly technique, fostering a cooperative and resilient energy system with enhanced consumer satisfaction.
The future research scope involves optimizing load shedding algorithms, integrating data analytics and artificial intelligence, assessing economic viability, evaluating environmental benefits, addressing regulatory aspects, understanding user engagement, and enhancing grid resilience. This comprehensive scope encompasses technical, economic, environmental, regulatory and user-centric dimensions, highlighting the potential of load-shedding strategies for efficient energy management and grid stability for smart grids.
Distributed generation in a smart grid involves generating electricity locally from small-scale, decentralized sources like solar panels and wind turbines. It is unlike traditional centralized power generation and can be integrated with advanced technologies for bi-directional power flow, real-time monitoring and control. Distributed generation enhances grid resilience, reduces transmission losses, promotes renewable energy use and fosters a more sustainable and reliable energy system [51].
In [52], the authors show that it is possible to lower the demand for grid electricity during peak hours by using distributed generation. This can be done by offering incentives to clients to build rooftop wind or solar power systems or small-scale combined heat and power systems. In order to release excess energy produced by distributed generation during peak hours, energy storage can be employed to store it during off-peak hours. As a result, the grid may experience less stress during moments of high demand for grid electricity. This showed that the off-peak power shifting approach and V2V technology effectively increased energy flexibility and decreased reliance on the power grid [53].
Power quality control methods can be used by a smart grid to decrease peak loads by increasing the effectiveness of power delivery [54]. This can be done by controlling the voltage and reactive power, which can decrease line losses and boost the grid's ability to supply power during peak hours. Figure 13 shows the mechanism of distributed generation in smart grids.
To optimize distributed generation in a smart grid, several improvements can be implemented. Enhancing integration with advanced control and communication systems, using smart inverters, and integrating energy storage solutions can enable better coordination and bi-directional power flow. Coupling distributed generation with a demand response scheme, improving grid flexibility, and creating microgrids contribute to enhanced grid stability and resilience. Supportive policies, consumer engagement, and accurate forecasting further promote distributed generation adoption. Additionally, ensuring robust cybersecurity safeguards grid security and reliability. These measures collectively foster a more efficient, reliable and sustainable energy system through the widespread adoption of distributed generation in the smart grid.
The future research scope involves optimizing control strategies, integrating advanced technologies, assessing economic viability, evaluating environmental benefits, addressing regulatory aspects, understanding user engagement, and enabling comprehensive grid transformation. This multifaceted scope encompasses technical, economic, environmental, regulatory, and user-centric dimensions, highlighting the potential of distributed generation for efficient energy production, grid stability, and sustainable energy management within smart grids.
Realizing peak shaving by implementing energy-efficient smart grid management involves optimizing electricity consumption during peak demand periods through the use of intelligent energy-management practices. The smart grid utilizes advanced technologies, such as smart meters, sensors and control systems, to monitor and manage electricity usage in real-time [55]. Energy-efficient management strategies include load shifting, the use of demand response and the prioritization of energy-intensive activities during off-peak hours. By actively controlling and reducing electricity usage during peak periods, the smart grid can flatten the demand curve, reduce the strain on the grid and minimize the need for additional power generation capacity. This approach not only reduces the costs for both the utilities operators and consumers, it also promotes sustainability by optimizing energy resources and enhancing overall grid stability.
The authors of [56] incorporated machine learning techniques and environmental factors, and the suggested strategy demonstrated promise as a means to enhance energy management for smart buildings. In a smart grid setting, optimizing energy consumption and demand response techniques may be possible with the use of granular-level electrical consumption data and the Smart Peak algorithm.
Modern approaches to dynamic information interaction and power exchange have been made possible by the latest developments in artificial intelligence, machine learning and the IoT. This has made peer-to-peer energy sharing and trading systems more effective [54].
It is common practice in data analysis [56] to compare the performance of the two well-liked machine learning regression techniques, i.e., multiple linear regression (MLR) and support vector regression (SVR), by using the root mean square error as a performance indicator. SVR surpassed MLR in terms of consumption prediction, which emphasizes the importance of choosing the best machine learning method for a given task.
The suggested Smart Peak algorithm [56] can help to prevent overloading and power failures by scheduling the smart appliances based on overall priorities and the building's threshold load. The algorithm can optimize the use of smart appliances in a building, resulting in energy savings and cost savings, by taking into account both schedulable and non-schedulable devices. Figure 14 shows energy-management system of a smart grid.
To enhance the effects of peak shaving via energy-efficient smart-grid management, several improvements must be made. This includes implementing advanced energy monitoring and analytics, encouraging the use of smart appliances and home energy management systems and utilizing predictive energy management to forecast peak demand accurately. Consumer education and engagement, along with incentives and rewards, can foster active participation and cooperation. Integrating renewable energy sources, enhancing grid flexibility and resilience and implementing dynamic pricing mechanisms can further optimize energy efficiency and demand response strategies. Supportive policies and regulations also play a key role in promoting widespread adoption and achieving a more sustainable and reliable energy system.
Overall, this study makes a significant contribution to the subject of energy management and smart buildings, and it may encourage future research in this area. The future research scope involves optimizing algorithms, integrating advanced technologies, assessing economic viability, evaluating environmental benefits, addressing regulatory aspects, understanding user engagement, and enabling comprehensive grid enhancement. This comprehensive scope encompasses technical, economic, environmental, regulatory, and user-centric dimensions, highlighting the potential of energy-efficient management strategies for sustainable energy practices, grid optimization, and the transition to a greener energy landscape with the use of smart grids.
Comparing different peak shaving strategies is essential for organizations to optimize energy consumption, reduce costs, and ensure grid stability. By identifying the most efficient methods, businesses can achieve substantial savings during high-demand periods, decrease reliance on fossil fuels, and enhance environmental sustainability. Moreover, strategic comparisons enable the adoption of innovative approaches, compliance with regulations and the ability to adapt to changing energy landscapes. This informed decision-making not only ensures energy security and risk management, it also positions organizations to be competitive while fostering long-term planning and resource management.
References | Peak demand reduction methods and technological solutions | Strategies and computational models | Hazard and security | Utilization and final purpose |
[8,9,10,11] | BESS | Decision tree-based algorithm to lower peak demand | Battery size has not been explored; deterioration of BESS needs to be explored | Applicable to microgrids and islanded microgrid |
[12,13,14,15] | Nuclear and battery energy storage power plant | Collaborative working of battery energy storage power plant and nuclear power plant | Combined operating mode enhances the safety and flexibility of nuclear power | Technique can only be Applied to a nuclear power plant |
[16,17,18,19,20] | Hybrid energy storage system | The harmonic analysis method | No evaluation of economic gain and risk of power system dynamics stability problems | Technique can be applied for short-term peak shaving only |
[21,22,23,24,25,26] | PV system | PV systems used in office buildings to reduce peak demand | Energy from the sun is intermittent and unpredictable | Technique can be applied in office buildings with a large roof space |
[27,28,29,30,31] | Real-time scheduling of household appliances | Electric load management technique | Timing and service constraints for use of household devices | Technique applicable to household devices precisely made for scheduling purposes |
[32,33,34]. | Repurposed EV batteries' | Discarded EV batteries could be used in Grid storage system | Reduces EV ownership cost and promotes low carbon footprint | Second life of EV batteries will add capacity to storage power plants |
[35,36,37,38,39] | Uni- and bi-directional EV charging | MPC method | Reduces the overall carbon footprint | Technique can be applied to a grid with a large number of EV owners |
[40,41,42,43,44] | Demand response | Scheduling for microgrid with a variety of operational incentives, rewards and restrictions | Increases sustainability of microgrids | Applicable to microgrids and main grid |
[45,46,47] | Time-of-use strategy | Multimarket strategy for lowering the daily peak demand. | Time-of-use schemes may not always be effective in reducing peak demand | Techniques is applicable to smart grid ecosystem with two-way communication |
[48,49,50] | Load shedding | Implementation of frequency load-shedding techniques | Approach achieves system reliability while also guaranteeing customer satisfaction | Technique applicable for frequency monitoring tools and smart house systems |
[51,52,53,54] | Distributed generation | Offering incentives to clients to build rooftop wind or solar power systems or small-scale combined heat and power | Power quality control methods need to be implemented to decrease peak load | Technique applicable under the condition of customer active participation with generation grid |
[55,56] | Energy efficient management | Incorporating machine learning techniques and Smart Peak algorithm | Algorithm can optimize the use of smart appliances in a building, resulting in energy savings and cost savings | Technique compatible with smart home devices |
In recent years, the demand for energy has been increasing at an unprecedented rate. This has put a strain on the traditional power grid system, leading to several issues such as high peak demand, energy wastage, and reliability problems. To tackle these issues, peak load shaving has emerged as a viable solution that helps utility providers and end-users to maximize their profits while ensuring efficient energy management.
Our article has provided a comprehensive literature review on various peak shaving approaches, highlighting their potential to reduce peak demand and flatten the power profile. These approaches include the use of demand response, energy storage, renewable energy integration and load shifting. By adopting these methods, both utility providers and end-users can benefit from reduced energy costs, improved system reliability and lower carbon emissions.
As we move toward a smart grid ecosystem, integrating multiple peak shaving technologies and addressing the associated challenges are becoming increasingly crucial. These challenges include the integration of renewable energy sources, effective communication and control systems, and cybersecurity issues. Our research has uncovered critical challenges in the smart grid system, which provides new research topics to further enhance the efficiency of the smart grid.
Our article can serve as a guide for designing a smart grid system that reduces peak demand while ensuring efficient energy management. By bridging the gap between the existing traditional power grid and the smart grid of the future, we can pave the way for a more sustainable energy future. The techniques discussed in our article can help utility providers and end-users to manage their energy consumption effectively, leading to a more efficient and reliable energy system.
Our review can serve as a guide to inform researchers and policymakers to bring the best possible outcome for a low-carbon-footprint smart grid ecosystem, as well as inform on renewable resources with the implementation of innovative technologies for peak shaving in smart grid ecosystem. Each peak shaving strategy discussed in this paper has its own unique approaches and algorithm, which may not be suitable for application in every smart grid environment.
This review paper provides a comprehensive analysis of diverse peak shaving strategies, revealing their potential to reduce peak demand, enhance grid stability, and foster sustainable energy practices. The future research scope encompasses predictive algorithms, advanced energy storage technologies, regulatory considerations, user engagement, and multidisciplinary collaboration. As experts, we stress the significance of holistic research that bridges technical, economic, environmental and social dimensions, as well as the importance of user-centric strategies and industry partnerships. By embracing these opportunities, we can pave the way for a sustainable energy future driven by intelligent energy management and grid optimization.
Future research directions in the area of peak shaving could include investigating advanced predictive algorithms for more accurate peak demand forecasts, exploring the integration of emerging energy storage technologies to enhance peak shaving effectiveness, examining the potential of artificial intelligence and machine learning for real-time demand response optimization, evaluating the economic and environmental impacts of combining multiple peak shaving strategies in a holistic manner and analyzing the scalability and feasibility of implementing peak shaving techniques in various industries and urban environments. Additionally, exploring the social and behavioral aspects of user engagement and participation in demand-side management programs could provide valuable insights for future implementation strategies.
In conclusion, peak load shaving is a crucial strategy for efficient energy management, and a smart grid ecosystem provides a significant opportunity to achieve this goal. By addressing the identified challenges and adopting the recommended techniques, we can make our power systems more efficient, reliable, and sustainable, paving the way for a greener future.
The authors declare that they have not used artificial intelligence tools in the creation of this article.
The first author would like to express his sincere gratitude to Honorable Vice Chancellor RGPV Bhopal and Professor Mohan Lal Kolhe from the University of Agder, Kristiansand, Norway for their invaluable guidance and support throughout the process of writing his review paper. Their expertise and insights have greatly enriched his work, and he is grateful for their mentorship and encouragement.
Mohan Lal Kolhe is a special issue editor for AIMS Energy and was not involved in the editorial review or the decision to publish this article. All authors declare that there are no competing interests.
Syed Sabir Hussain Rizvi: conceptualization, selection of research papers, formal analysis, writing original draft, writing review and editing.
Krishna Teerth Chaturvedi: conceptualization, selection of research papers, formal analysis, writing original draft, writing review and editing.
Mohan Lal Kolhe: conceptualization, selection of research papers, formal analysis, writing original draft, writing review and editing.
[1] |
Markovic DS, Zivkovic D, Branovic I, et al. (2013) Smart power grid and cloud computing. Renewable Sustainable Energy Rev 24: 566–577. https://doi.org/10.1016/j.rser.2013.03.068 doi: 10.1016/j.rser.2013.03.068
![]() |
[2] | Ozdemir E, Ozdemir S, Erhan K, et al. (2016) Energy storage technologies opportunities and challenges in smart grids. International Smart Grid Workshop and Certificate Program (ISGWCP), Istanbul, Turkey, 1–6. https://doi.org/10.1109/ISGWCP.2016.7548263 |
[3] | Borlase S (2018) Smart grids: Advanced technologies and solutions, second edition (2nd Ed.). CRC Press, Taylor & Francis Group. https://doi.org/10.1201/9781351228480 |
[4] |
Uddin M, Romlie MF, Abdullah MF, et al. (2017) A review on peak load shaving strategies. Renewable Sustainable Energy Rev 82: 3323–3332. https://doi.org/10.1016/j.rser.2017.10.056 doi: 10.1016/j.rser.2017.10.056
![]() |
[5] |
Yılmaz F, Eren Y (2023) A novel load profile generation method based on the estimation of regional usage habit parameters with genetic algorithm. Electric Power Syst Res 217: 1–13. https://doi.org/10.1016/j.epsr.2023.109165 doi: 10.1016/j.epsr.2023.109165
![]() |
[6] | Ideal energy (2023) Peak shaving with solar and energy storage. Available from: https://www.idealenergysolar.com/peak-shaving-solar-storage. |
[7] | Zhang TS, Sheng WX, Song XH, et al. (2013) Probabilistic modelling and simulation of stochastic load for power system studies. UKSim 15th International Conference on Computer Modelling and Simulation, Cambridge, UK, 519–524. https://doi.org/10.1109/UKSim.2013.23 |
[8] | Oudalov A, Cherkaoui R, Beguin A (2007) Sizing and optimal operation of battery energy storage system for peak shaving application. 2007 IEEE Lausanne Power Tech, Lausanne, Switzerland, 621–625. https://doi.org/10.1109/PCT.2007.4538388 |
[9] |
Uddin M, Romlie MF, Abdullah MF, et al. (2020) A novel peak shaving algorithm for islanded microgrid using battery energy storage system. Energy 196: 117084. https://doi.org/10.1016/j.energy.2020.117084 doi: 10.1016/j.energy.2020.117084
![]() |
[10] |
Arcos-Vargas A, Lugo D, Nunez F (2018) Residential peak electricity management. A storage and control systems application taking advantages of smart meters. Int J Electr Power Energy Syst 102: 110–121. https://doi.org/10.1016/j.ijepes.2018.04.016 doi: 10.1016/j.ijepes.2018.04.016
![]() |
[11] |
Evans A, Strezov V, Evans TJ (2012) Assessment of utility energy storage options for increased renewable energy penetration. Renewable Sustainable Energy Rev 16: 4141–4147. https://doi.org/10.1016/j.rser.2012.03.048 doi: 10.1016/j.rser.2012.03.048
![]() |
[12] |
Song A, Zhou YK (2023) A hierarchical control with thermal and electrical synergies on battery cycling ageing and energy flexibility in a multi-energy sharing network. Renewable Energy 212: 1020–1037. https://doi.org/10.1016/j.renene.2023.05.050 doi: 10.1016/j.renene.2023.05.050
![]() |
[13] |
Chen X, Huang L, Liu J (2022) Peak shaving benefit assessment considering the joint operation of nuclear and battery energy storage power stations: Hainan case study. Energy 239: 21897. https://doi.org/10.1016/j.energy.2021.121897 doi: 10.1016/j.energy.2021.121897
![]() |
[14] |
Song D, Chang Q, Zheng S (2021) Adaptive model predictive control for Yaw system of variable-speed wind turbines. J Modern Power Syst Clean Energy 9: 219–224. https://doi.org/10.35833/MPCE.2019.000467 doi: 10.35833/MPCE.2019.000467
![]() |
[15] |
Yang J, Fang LQ, Song DR, et al. (2021) Review of control strategy of large horizontal-axis wind turbines yaw system. Wind Energy 24: 97–115. https://doi.org/10.1002/we.2564 doi: 10.1002/we.2564
![]() |
[16] |
Atawi IE, Al-Shetwi AQ, Magableh AM, et al. (2023) Recent advances in hybrid energy storage system integrated renewable power generation: Configuration, control, applications, and future directions. Batteries 9: 1–35. https://doi.org/10.3390/batteries9010029 doi: 10.3390/batteries9010029
![]() |
[17] | Mohd A, Ortjohann E, Schmelter A, et al. (2008) Challenges in integrating distributed energy storage systems into future smart grid. 2008 IEEE International Symposium on Industrial Electronics, Cambridge, UK, 1627–1632. https://doi.org/10.1109/isie.2008.4676896 |
[18] |
Zhao P, Wang JF, Dai YP (2015) Capacity allocation of a hybrid energy storage system for power system peak shaving at high wind power penetration level. Renewable Energy 75: 541–549. https://doi.org/10.1016/j.renene.2014.10.040 doi: 10.1016/j.renene.2014.10.040
![]() |
[19] |
Zhou YK (2022) Transition towards carbon-neutral districts based on storage techniques and spatiotemporal energy sharing with electrification and hydrogenation. Renewable Sustainable Energy Rev 162: 112444. https://doi.org/10.1016/j.rser.2022.112444 doi: 10.1016/j.rser.2022.112444
![]() |
[20] |
Korpaas M, Holen AT, Hildrum R (2003) Operation and sizing of energy storage for wind power plants in a market system. Int J Electric Power Energy Syst 25: 599–606. https://doi.org/10.1016/s0142-0615(03)00016-4 doi: 10.1016/s0142-0615(03)00016-4
![]() |
[21] |
Müller M, Viernstein L, Truong CN, et al. (2017) Evaluation of grid-level adaptability for stationary battery energy storage system applications. Europe J Energy Storage 9: 1–11. https://doi.org/10.1016/j.est.2016.11.005 doi: 10.1016/j.est.2016.11.005
![]() |
[22] |
Ceran B, Jurasz J, Mielcarek A, et al. (2021) PV systems integrated with commercial buildings for local and national peak load shaving. Poland J Cleaner Product 322: 129076. https://doi.org/10.1016/j.jclepro.2021.129076 doi: 10.1016/j.jclepro.2021.129076
![]() |
[23] |
Shu S, Mo L, Wang Y (2019) Peak shaving strategy of wind-solar-hydro hybrid generation system based on modified differential evolution algorithm. Energy Proc 158: 3500–3505. https://doi.org/10.1016/j.egypro.2019.01.920 doi: 10.1016/j.egypro.2019.01.920
![]() |
[24] | Syafii, Zaini, Juliandri D, et al. (2018) Design of PV system for electricity peak-shaving: A case study of faculty of engineering, Andalas University. 2018 International Conference on Computing, Power and Communication Technologies (GUCON), Greater Noida, India, 294–298. https://doi.org/10.1109/gucon.2018.8675096 |
[25] |
Zheng X, Zhou Y (2023) A three-dimensional unsteady numerical model on a novel aerogel-based PV/T-PCM system with dynamic heat-transfer mechanism and solar energy harvesting analysis. Appl Energy 338: 120899. https://doi.org/10.1016/j.apenergy.2023.120899 doi: 10.1016/j.apenergy.2023.120899
![]() |
[26] |
Jurasz JK, Bronisław P, Campana PE (2019) Can a city reach energy self-sufficiency by means of rooftop photovoltaics? Case study from Poland. J Cleaner Product 245: 118813. https://doi.org/10.1016/j.jclepro.2019.118813 doi: 10.1016/j.jclepro.2019.118813
![]() |
[27] |
Uddin M, Romlie MF, Abdullah MF, et al. (2018) A review on peak load shaving strategies. Renewable Sustainable Energy Rev 82: 3323–3332. https://doi.org/10.1016/j.rser.2017.10.056 doi: 10.1016/j.rser.2017.10.056
![]() |
[28] |
Caprino D, Della Vedova ML, Facchinetti T (2014) Peak shaving through real-time scheduling of household appliances. Energy Build 75: 133–148. https://doi.org/10.1016/j.enbuild.2014.02.013 doi: 10.1016/j.enbuild.2014.02.013
![]() |
[29] |
Lin JT, Chen CM (2015) Simulation optimization approach for hybrid flow shop scheduling problem in semiconductor back-end manufacturing. Simul Model Practice Theory 51: 100–114. https://doi.org/10.1016/j.simpat.2014.10.008 doi: 10.1016/j.simpat.2014.10.008
![]() |
[30] | Vinyals M, Bistaffa F, Farinelli A, et al. (2012) Coalitional energy purchasing in the smart grid. Energy Conference and Exhibition (ENERGYCON), 2012 IEEE International, 848–853. https://doi.org/10.1109/EnergyCon.2012.6348270 |
[31] |
Zhou YK (2022) Low-carbon transition in smart city with sustainable airport energy ecosystems and hydrogen-based renewable-grid-storage-flexibility. Energy Rev 1: 100001. https://doi.org/10.1016/j.enrev.2022.100001 doi: 10.1016/j.enrev.2022.100001
![]() |
[32] | Zhao L, Aravinthan V (2013) Strategies of residential peak shaving with integration of demand response and V2H. 2013 IEEE PES Asia-Pacific Power and Energy Engineering Conference (APPEEC). https://doi.org/10.1109/appeec.2013.6837260 |
[33] |
Lee JW, Haram MHSM, Ramasamy G (2021) Technical feasibility and economics of repurposed electric vehicles batteries for power peak shaving. J Energy Storage 40: 102752. https://doi.org/10.1016/j.est.2021.102752 doi: 10.1016/j.est.2021.102752
![]() |
[34] |
Laserna EM, Gandiaga I, Zabala ES, et al. (2018). Battery second life: Hype, hope or reality? A critical review of the state of the art. Renewable Sustainable Energy Rev 93: 701–718. https://doi.org/10.1016/j.rser.2018.04.035 doi: 10.1016/j.rser.2018.04.035
![]() |
[35] |
Giorgio AD, Liberati F, Canale S (2014). Electric vehicles charging control in a smart grid: A model predictive control approach. Control Eng Pract 22: 147–162. https://doi.org/10.1016/j.conengprac.2013.10.005 doi: 10.1016/j.conengprac.2013.10.005
![]() |
[36] |
Kriekinge GV, Cauwer CD, Sapountzoglou N, et al. (2021) Peak shaving and cost minimization using model predictive control for uni- and bi-directional charging of electric vehicles. Energy Rep 7: 8760–8771. https://doi.org/10.1016/j.egyr.2021.11.207 doi: 10.1016/j.egyr.2021.11.207
![]() |
[37] |
Ito A, Kawashima A, Suzuki T (2018) Model predictive charging control of in-vehicle batteries for home energy management based on vehicle state prediction. IEEE Transactions on Control Systems Technology, 26: 51–64. https://doi.org/10.1109/tcst.2017.2664727 doi: 10.1109/tcst.2017.2664727
![]() |
[38] |
Zhaoxi L, Qiuwei W, Kang M, et al. (2019) Two-stage optimal scheduling of electric vehicle charging based on transactive control. IEEE Trans Smart Grid 10: 2948–2958. https://doi.org/10.1109/TSG.2018.2815593 doi: 10.1109/TSG.2018.2815593
![]() |
[39] |
Song A, Zhou Y (2023) Advanced cycling ageing-driven circular economy with E-mobility-based energy sharing and lithium battery cascade utilisation in a district community. J Cleaner Prod 415: 137797. https://doi.org/10.1016/j.jclepro.2023.137797 doi: 10.1016/j.jclepro.2023.137797
![]() |
[40] |
Lai J, Zhou H, Hu W, et al. (2015) Smart demand response based on smart homes. Math Probl 2015: 912535. Eng https://doi.org/10.1155/2015/912535 doi: 10.1155/2015/912535
![]() |
[41] |
Shen J, Jiang C, Liu Y, et al. (2016) A microgrid energy management system with demand response for providing grid peak shaving. Electr Power Compon Syst 44: 843–852. https://doi.org/10.1080/15325008.2016.1138344 doi: 10.1080/15325008.2016.1138344
![]() |
[42] |
Zhong H, Xie L, Xia Q (2013) Coupon incentive-based demand response: Theory and case study. IEEE Trans Power Syst 28: 1266–1276. https://doi.org/10.1109/TPWRS.2012.2218665 doi: 10.1109/TPWRS.2012.2218665
![]() |
[43] |
Zhou Y (2022) Incentivising multi-stakeholders' proactivity and market vitality for spatiotemporal microgrids in Guangzhou-Shenzhen-Hong Kong Bay Area. Appl Energy 328: 120196. https://doi.org/10.1016/j.apenergy.2022.120196 doi: 10.1016/j.apenergy.2022.120196
![]() |
[44] |
Zhou Y (2022) Demand response flexibility with synergies on passive PCM walls, BIPVs, and active air-conditioning system in a subtropical climate. Renewable Energy 199: 204–225. https://doi.org/10.1016/j.renene.2022.08.128 doi: 10.1016/j.renene.2022.08.128
![]() |
[45] | Papadopoulos V, Delerue T, Ryckeghem JV, et al. (2017) Assessing the impact of load forecasting accuracy on battery dispatching strategies with respect to Peak Shaving and Time-of-Use (TOU) applications for industrial consumers. 52nd International Universities Power Engineering Conference (UPEC), Heraklion, Greece, 1–5. https://doi.org/10.1109/UPEC.2017.8231939 |
[46] | Manoochehri H, Fereidunian A (2016) Peak-shaving using time-of-use pricing and market coordination. 4th Regional Conference on Electricity Distribution (CIRED), Tehran, Iran. https://doi.org/10.1109/ISTEL.2016.7881915 |
[47] | Hassan R, M. Abdallah M, Radman G (2012) Load shedding in smart grid: A reliable efficient Ad-Hoc broadcast algorithm for smart house. Proceedings of IEEE Southeastcon, Orlando, FL, USA, 1–5. https://doi.org/10.1109/SECon.2012.6196919 |
[48] | Mishra S, Palanisamy P (2018) Efficient power flow management and peak shaving in a microgrid-PV system. IEEE Energy Conversion Congress and Exposition (ECCE), Portland, OR, USA, 3792–3798. https://doi.org/10.1109/ECCE.2018.8558312 |
[49] | Hassan R, Abdallah M, Radman G, et al. (2011) Under-Frequency Load Shedding: Towards a smarter smart house with a consumer level controller. Proc IEEE, 73–78. https://doi.org/10.1109/SECON.2011.5752909 |
[50] |
Panda S, Mohanty S, Rout PK, et al. (2022) Residential demand side management model, optimization and future perspective: A review. Energy Rep 8: 3727–3766. https://doi.org/10.1016/j.egyr.2022.02.300 doi: 10.1016/j.egyr.2022.02.300
![]() |
[51] |
Zhou L, Zhou Y (2023) Study on thermo-electric-hydrogen conversion mechanisms and synergistic operation on hydrogen fuel cell and electrochemical battery in energy flexible buildings. Energy Conver Manage 277: 116610. https://doi.org/10.1016/j.enconman.2022.116610 doi: 10.1016/j.enconman.2022.116610
![]() |
[52] | Pudjianto D, Ramsay C, Strbac G (2007) Virtual power plant and system integration of distributed energy resources. Renewable Power Gener 10–16. https://doi.org/10.1049/iet-rpg: 20060023 |
[53] | Behera S, Misra R (2018) SmartPeak: Peak shaving and ambient analysis for energy efficiency in electrical smart grid. Proceedings of the 2018 Artificial Intelligence and Cloud Computing Conference, 157–165. https://doi.org/10.1145/3299819.3299833 |
[54] |
Zhou Y, Lund PD (2023) Peer-to-peer energy sharing and trading of renewable energy in smart communities—Trading pricing models, decision-making and agent-based collaboration. Renewable Energy 207: 177–193. https://doi.org/10.1016/j.renene.2023.02.125 doi: 10.1016/j.renene.2023.02.125
![]() |
[55] |
Molderink A, Bakker V, Bosman MGC, et al. (2010) Management and control of domestic smart grid technology. IEEE Trans Smart Grid 1: 109–119. https://doi.org/10.1109/TSG.2010.2055904 doi: 10.1109/TSG.2010.2055904
![]() |
[56] | Holcomb D, Li W, Seshia SA (2009) Algorithms for green buildings: Learning-based techniques for energy prediction and fault diagnosis. Technical Report No: UCB/EECS-2009-138. Available from: https://digitalassets.lib.berkeley.edu/techreports/ucb/text/EECS-2009-138.pdf. |
1. | Linyun Huang, Yong Zhang, Yujie Su, Youngchul Bae, Multi-Objective Reliable Optimization Dispatch of High-proportion Power System Based on Improved Particle Swarm Algorithm, 2024, 2890, 1742-6588, 012028, 10.1088/1742-6596/2890/1/012028 | |
2. | Shreyas Kulkarni, Sheetal Kumar K R, Akhash Vellandurai, Vinoth Kumar, 2024, Optimization-Based Grid Energy Management System with BESS and EV Charging Load for Peak Shaving, 979-8-3503-6248-0, 5176, 10.1109/BigData62323.2024.10825548 | |
3. | Mohammad Sameer Ahmad, Nurulafiqah Nadzirah Mansor, Hazlie Mokhlis, Kanendra Naidu, Hasmaini Mohamad, Farah Ramadhani, Demand Response Program Toward Sustainable Power Supply: Current Status, Challenges, and Prospects in Malaysia, 2025, 13, 2169-3536, 34706, 10.1109/ACCESS.2025.3541841 |
References | Peak demand reduction methods and technological solutions | Strategies and computational models | Hazard and security | Utilization and final purpose |
[8,9,10,11] | BESS | Decision tree-based algorithm to lower peak demand | Battery size has not been explored; deterioration of BESS needs to be explored | Applicable to microgrids and islanded microgrid |
[12,13,14,15] | Nuclear and battery energy storage power plant | Collaborative working of battery energy storage power plant and nuclear power plant | Combined operating mode enhances the safety and flexibility of nuclear power | Technique can only be Applied to a nuclear power plant |
[16,17,18,19,20] | Hybrid energy storage system | The harmonic analysis method | No evaluation of economic gain and risk of power system dynamics stability problems | Technique can be applied for short-term peak shaving only |
[21,22,23,24,25,26] | PV system | PV systems used in office buildings to reduce peak demand | Energy from the sun is intermittent and unpredictable | Technique can be applied in office buildings with a large roof space |
[27,28,29,30,31] | Real-time scheduling of household appliances | Electric load management technique | Timing and service constraints for use of household devices | Technique applicable to household devices precisely made for scheduling purposes |
[32,33,34]. | Repurposed EV batteries' | Discarded EV batteries could be used in Grid storage system | Reduces EV ownership cost and promotes low carbon footprint | Second life of EV batteries will add capacity to storage power plants |
[35,36,37,38,39] | Uni- and bi-directional EV charging | MPC method | Reduces the overall carbon footprint | Technique can be applied to a grid with a large number of EV owners |
[40,41,42,43,44] | Demand response | Scheduling for microgrid with a variety of operational incentives, rewards and restrictions | Increases sustainability of microgrids | Applicable to microgrids and main grid |
[45,46,47] | Time-of-use strategy | Multimarket strategy for lowering the daily peak demand. | Time-of-use schemes may not always be effective in reducing peak demand | Techniques is applicable to smart grid ecosystem with two-way communication |
[48,49,50] | Load shedding | Implementation of frequency load-shedding techniques | Approach achieves system reliability while also guaranteeing customer satisfaction | Technique applicable for frequency monitoring tools and smart house systems |
[51,52,53,54] | Distributed generation | Offering incentives to clients to build rooftop wind or solar power systems or small-scale combined heat and power | Power quality control methods need to be implemented to decrease peak load | Technique applicable under the condition of customer active participation with generation grid |
[55,56] | Energy efficient management | Incorporating machine learning techniques and Smart Peak algorithm | Algorithm can optimize the use of smart appliances in a building, resulting in energy savings and cost savings | Technique compatible with smart home devices |
References | Peak demand reduction methods and technological solutions | Strategies and computational models | Hazard and security | Utilization and final purpose |
[8,9,10,11] | BESS | Decision tree-based algorithm to lower peak demand | Battery size has not been explored; deterioration of BESS needs to be explored | Applicable to microgrids and islanded microgrid |
[12,13,14,15] | Nuclear and battery energy storage power plant | Collaborative working of battery energy storage power plant and nuclear power plant | Combined operating mode enhances the safety and flexibility of nuclear power | Technique can only be Applied to a nuclear power plant |
[16,17,18,19,20] | Hybrid energy storage system | The harmonic analysis method | No evaluation of economic gain and risk of power system dynamics stability problems | Technique can be applied for short-term peak shaving only |
[21,22,23,24,25,26] | PV system | PV systems used in office buildings to reduce peak demand | Energy from the sun is intermittent and unpredictable | Technique can be applied in office buildings with a large roof space |
[27,28,29,30,31] | Real-time scheduling of household appliances | Electric load management technique | Timing and service constraints for use of household devices | Technique applicable to household devices precisely made for scheduling purposes |
[32,33,34]. | Repurposed EV batteries' | Discarded EV batteries could be used in Grid storage system | Reduces EV ownership cost and promotes low carbon footprint | Second life of EV batteries will add capacity to storage power plants |
[35,36,37,38,39] | Uni- and bi-directional EV charging | MPC method | Reduces the overall carbon footprint | Technique can be applied to a grid with a large number of EV owners |
[40,41,42,43,44] | Demand response | Scheduling for microgrid with a variety of operational incentives, rewards and restrictions | Increases sustainability of microgrids | Applicable to microgrids and main grid |
[45,46,47] | Time-of-use strategy | Multimarket strategy for lowering the daily peak demand. | Time-of-use schemes may not always be effective in reducing peak demand | Techniques is applicable to smart grid ecosystem with two-way communication |
[48,49,50] | Load shedding | Implementation of frequency load-shedding techniques | Approach achieves system reliability while also guaranteeing customer satisfaction | Technique applicable for frequency monitoring tools and smart house systems |
[51,52,53,54] | Distributed generation | Offering incentives to clients to build rooftop wind or solar power systems or small-scale combined heat and power | Power quality control methods need to be implemented to decrease peak load | Technique applicable under the condition of customer active participation with generation grid |
[55,56] | Energy efficient management | Incorporating machine learning techniques and Smart Peak algorithm | Algorithm can optimize the use of smart appliances in a building, resulting in energy savings and cost savings | Technique compatible with smart home devices |