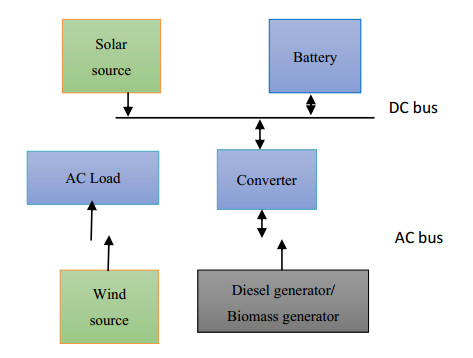
Renewable energy has grown in popularity in recent years as a solution to combat the effects of pollution on the environment. The main purpose of this research is to design a microgrid system in Lakshadweep Island to determine the cost and dependability of a solar photovoltaic system that is combined with biomass, wind energy and diesel generator. Two types of hybrid systems like solar/biomass generator/wind turbine and Solar/diesel generator/biomass are investigated to get an optimal solution using HOMER Pro software. The hybrid microgrid system is optimized with low cost of energy (COE) and less environmental pollution. The reliability indice like unmet load is determined for each case to access the performance of the system. The influence of different Weibull shape parameter in solar/biomass generator/wind turbine hybrid system with sensitive variation of solar irradiation and wind speed are discussed. The scheduling of diesel generator in solar/diesel generator/biomass generator with various scenarios are analyzed based on minimum net present cost. The optimization results shows that the solar/diesel generator/biomass hybrid system has low net present cost of 432513 $ and cost of energy of 0.215 $/kWh as compared to solar/biomass/wind turbine for the selected site location. The proposed solar/diesel generator/biomass system produces emission of 7506 kg/yr. The emission produced in Lakshadweep Island using the proposed model is reduced since this Island currently produces electricity mainly with diesel generators. The optimal sizing of various components in microgrid system is performed to get the optimal solution.
Citation: S. Vinoth John Prakash, P.K. Dhal. Cost optimization and optimal sizing of standalone biomass/diesel generator/wind turbine/solar microgrid system[J]. AIMS Energy, 2022, 10(4): 665-694. doi: 10.3934/energy.2022032
[1] | Aaron St. Leger . Demand response impacts on off-grid hybrid photovoltaic-diesel generator microgrids. AIMS Energy, 2015, 3(3): 360-376. doi: 10.3934/energy.2015.3.360 |
[2] | Mulualem T. Yeshalem, Baseem Khan . Design of an off-grid hybrid PV/wind power system for remote mobile base station: A case study. AIMS Energy, 2017, 5(1): 96-112. doi: 10.3934/energy.2017.1.96 |
[3] | Rashid Al Badwawi, Mohammad Abusara, Tapas Mallick . Speed control of synchronous machine by changing duty cycle of DC/DC buck converter. AIMS Energy, 2015, 3(4): 728-739. doi: 10.3934/energy.2015.4.728 |
[4] | Yassine Charabi, Sabah Abdul-Wahab . The optimal sizing and performance assessment of a hybrid renewable energy system for a mini-gird in an exclave territory. AIMS Energy, 2020, 8(4): 669-685. doi: 10.3934/energy.2020.4.669 |
[5] | Nadwan Majeed Ali, Handri Ammari . Design of a hybrid wind-solar street lighting system to power LED lights on highway poles. AIMS Energy, 2022, 10(2): 177-190. doi: 10.3934/energy.2022010 |
[6] | T S S Senarathna, K T M Udayanga Hemapala . Review of adaptive protection methods for microgrids. AIMS Energy, 2019, 7(5): 557-578. doi: 10.3934/energy.2019.5.557 |
[7] | Abshir Ashour, Taib Iskandar Mohamad, Kamaruzzaman Sopian, Norasikin Ahmad Ludin, Khaled Alzahrani, Adnan Ibrahim . Performance optimization of a photovoltaic-diesel hybrid power system for Yanbu, Saudi Arabia. AIMS Energy, 2021, 9(6): 1260-1273. doi: 10.3934/energy.2021058 |
[8] | Tilahun Nigussie, Wondwossen Bogale, Feyisa Bekele, Edessa Dribssa . Feasibility study for power generation using off- grid energy system from micro hydro-PV-diesel generator-battery for rural area of Ethiopia: The case of Melkey Hera village, Western Ethiopia. AIMS Energy, 2017, 5(4): 667-690. doi: 10.3934/energy.2017.4.667 |
[9] | Mohamed G Moh Almihat . An overview of AC and DC microgrid energy management systems. AIMS Energy, 2023, 11(6): 1031-1069. doi: 10.3934/energy.2023049 |
[10] | Navid Majdi Nasab, Jeff Kilby, Leila Bakhtiaryfard . Integration of wind and tidal turbines using spar buoy floating foundations. AIMS Energy, 2022, 10(6): 1165-1189. doi: 10.3934/energy.2022055 |
Renewable energy has grown in popularity in recent years as a solution to combat the effects of pollution on the environment. The main purpose of this research is to design a microgrid system in Lakshadweep Island to determine the cost and dependability of a solar photovoltaic system that is combined with biomass, wind energy and diesel generator. Two types of hybrid systems like solar/biomass generator/wind turbine and Solar/diesel generator/biomass are investigated to get an optimal solution using HOMER Pro software. The hybrid microgrid system is optimized with low cost of energy (COE) and less environmental pollution. The reliability indice like unmet load is determined for each case to access the performance of the system. The influence of different Weibull shape parameter in solar/biomass generator/wind turbine hybrid system with sensitive variation of solar irradiation and wind speed are discussed. The scheduling of diesel generator in solar/diesel generator/biomass generator with various scenarios are analyzed based on minimum net present cost. The optimization results shows that the solar/diesel generator/biomass hybrid system has low net present cost of 432513 $ and cost of energy of 0.215 $/kWh as compared to solar/biomass/wind turbine for the selected site location. The proposed solar/diesel generator/biomass system produces emission of 7506 kg/yr. The emission produced in Lakshadweep Island using the proposed model is reduced since this Island currently produces electricity mainly with diesel generators. The optimal sizing of various components in microgrid system is performed to get the optimal solution.
In today's world, every country's energy needs are a critical consideration. The People can manage without electricity for some hours in earlier days. But now it is difficult for the people to sustain without electricity for some minutes. The increase in electronic devices, home appliances, industries etc., made it necessary to have a reliable supply to the customer. So, the electricity is necessary for every residential and commercial application. The renewable energies recently used to have a green environment. In Island remote areas, constructing transmission and distribution system is difficult for such a small amount of energy requirement. Power transmission to remote areas is difficult due to unviability of extending the grid, high investment cost during transmission and distribution, has resulted in a huge budget burden and total dependence on imported fossil fuels is economically unaffordable. The stand-alone microgrids system have emerged as the most efficient energy solution to electrify remote sites where expanding the utility grid is not economically feasible. The diesel price and emissions are increasing in Island since this Island mainly rely on diesel generator. Hence, the economical alternatives are to use solar/biomass/wind energy to decrease the maximum dependency on traditional fuels. The disposal of biomass (coconut shell/husk) in selected island is one among the environmental issue and it can be effectively solved by using biomass for generating power at minimum cost. In this study, the microgrid system is simulated in remote areas where there is no grid connectivity using HOMER software.
The Solar, biomass, wind energy and diesel generator are used for cost optimization process. The cost of energy 'COE' is evaluated based on total cost of the system considered, capacity recovery factor and energy demand over a year. The cost of the system is evaluated depending on different component costs of the system involved in optimization process. The COE will help the researcher to choose the optimized system consisting of different sources with reasonable cost of energy. The system will not fail to operate for a particular time when the system is more reliable. The system reliability also maintained with less cost. The power balance equation is maintained in order to operate the system without failure. The microgrid system is allowed to run till the maximum number of system components reached. The maximum number of components considered as constraints are solar panels, wind turbine and batteries. The battery is essential in operating the renewable microgrid system due to intermittent nature. The minimum state of charge (SOC) of the battery is considered as constraints so that battery will not get discharged below the minimum SOC level. The optimization process runs with different components in solar/biomass/wind source and diesel generator system with battery, rectifier and inverter to track the feasible less cost path.
There are different optimization approaches done in recent years for reducing cost of energy. The solar energy incorporation in generation system is one of the challenging parts in optimization process since the output of solar energy is erratic in nature. A practical mathematical model for solar system was developed in [1]. The application of solar energy system which can fulfil the load [2]. The future will be depending only on renewable energies like Solar because it won't emit any harmful gases [3]. There are several studies done for reliability analysis of solar power system since the forced outage is the main problem for getting reduced renewable output [4,5,6,7,8]. Due to unavailability of Solar which is occur due to environmental effect there is outage in the solar output. The total installed capacity of solar, wind and biomass in India are 50303.58 MW, 40100.93 MW and 10609.72 as per the recent data dated 31st Jan 2022. [9]. The conventional energy sources will have more pollution which will affect many human lives. Due to increase in non-conventional energy sources, the pollution is controlled. In recent years, the renewable energy like solar energy has increased solar utilization to satisfy the load demand for every time [10].
The cost should be reduced by an appropriate optimization process. The solar, wind, biomass and diesel generator (DG) hybrid system was intended to achieve the feasible operation at lower cost and to produce energy with less pollution. The system will produce emission when DG source is used during unavailability of solar and battery backup. When the system components are decreased then the overall system cost also decreases. The optimal sizing performed during operation with low cost for load demand for each time period in a year.
The solar panel is simulated under different operating condition [11]. The grid and standalone system are compared in this study. The emission and the energy cost were simulated using different optimization techniques like Particle Swarm optimization [12]. The system is simulated based on the mono and multi-objective function. The fitness value evaluated after initialization process. The sizing optimization obtained to extract good performance of the system with minimum cost, the timeline of optimization process was discussed [13]. The energy storage plays a main role in developing a hybrid system model. The system operated with Solar/Wind/DG source. During the operation for the simulated year, the diesel operated less hours in a year [14]. The availability of the source, size of different components and total cost in PV/Wind system is optimized using genetic algorithm [15]. The economic system operation considered with battery is investigated. The system operated with 200kW diesel generator, 180 watts solar, 30 kW wind and 2 V/1000 Ah battery [16]. This system is simulated when there is shortage in solar/wind source. During this case diesel generator is allowed to operate. To overcome the sizing optimization complexity of renewable system, suitable studies were conducted [17]. The reliability indices and cost for hybrid green energy sources for different decision variables by Genetic analysis were discussed [18]. The biomass source incorporated with other non-conventional sources with wind, solar, fuel cell and the result shows that the COE is 0.214 $/kWh [19]. The biomass is developing in non-conventional energy sources [20]. The best solution determined based on the objective function like cost and reliability factor. The system overall cost will reduce by reducing the system components. The reliability indices are determined for solar/wind system during optimization process [21,22]. The losses present in the microgrid system which will affects the system performance. The stability of proposed optimization model considering solar/wind system was tested [23]. The uncertainty of solar power taken in consideration to evaluate the system optimization [24]. The effect on sizing of different components in the system on reliability indices on the system is investigated [25]. The effect of unavailability of solar and reliability indices is evaluated. The solar fail to operate due to outage in generation [26]. The modelling of Solar PV system is formulated [27]. The solar system capacity can be analyzed by solar radiation data. The solar radiation is different for every time period, which is the main thing for producing solar output [28]. The energy storage is necessary to deliver the power to the system during unavailability of power from other sources like Solar/Wind system [29]. The size and capacity of the batteries can be utilized with feasible cost and less numbers of battery. It is noted that the annual solar irradiation is 1900 kWh/m2 in Bangladesh [30]. The installed capacity of Solar PV system increased rapidly during last decades [31]. The Solar PV system is simple in operation since there is no transmission cost [32]. The control of renewable sources in microgrid is discussed in [33]. The hybrid system consists of solar PV, WT and biomass are optimized and modelled to minimize the COE, NPC, unmet load and CO2 emission in [34]. The influence of Weibull shape factor on hybrid system consist of DG and wind are analyzed using HOMER software [35]. In [36], the new control technique developed to extract more power from the solar photovoltaic system. In [37], the optimal sizing of isolated microgrid was analyzed. Apart from optimal sizing, the environmental effect on hybrid system with minimum cost also highlighted. The performance of wind turbine is analyzed based on Weibull shape factor and discussed about the advantages of using wind energy in hybrid system compared to diesel generator [38]. In [39], the Weibull probability density function for different values of wind speed is discussed in Algerian region. In [40,41] investigated about the Weibull density function in wind power system. The average wind speed for a particular site location will differ from other location and the corresponding Weibull density function is estimated. The renewable sources like solar and wind are intermittent in nature. Addressing this problem is very difficult. So, the planning model for microgrid is implemented to address this problem [42]. This model helps to maintain the reliability of the system. It is difficult to improve efficiency of renewable energies like solar/wind and reducing cost of the microgrid. The dynamic optimal scheduling is considered to achieve the efficiency and cost [43]. The operational interval of multi-microgrid can reduce the operating cost of the renewable system and the fluctuation in the renewable sources can be stabilize [44]. The economic and environmental constraints like cost and emission for producing power is considered as major issue. This issue is solved by using the optimization process [45]. The uncertainties of wind power are solved by robust optimization model [46]. To achieve coordination between cost and reliability, the flexible robust optimization model is implemented [47]. Integrating battery energy storage system with renewable energies like solar and wind system requires reliability and environmental factors. This optimization problem can be solved by using genetic algorithm [48]. To overcome the fluctuations in microgrids, the batteries are implemented [49]. The size optimization of renewable sources with and without grid is simulated in the location of Bangladesh using HOMER software [50].
The novelty of the proposed work is that the biomass and wind system are used in this work for power generation but it is not currently used in Lakshadweep Island. Currently, this Island depends only on Solar and diesel generator. The diesel generator produces more emission which will affects the environment. There are lot of coconut waste available in Island which is disposal as waste. To use those waste in a useful manner, the biomass power plant is implemented in Lakshadweep Island. The biomass power plant uses coconut waste as a fuel for running the power plant for generating power. The wind turbine also produces power in this Island without emission. This analysis shows the possibility of installing biomass and wind power plant with solar/DG in Lakshadweep Island.
The solar panel is connected to DC bus. The battery is then connected to the DC bus, which can be used to provide energy when solar is unavailable due to environmental conditions. When battery and solar fail to fulfil the demand, the diesel generator (DG)/biomass is included as a source of demand power. The DG generator is connected with AC bus. The converter is a combination of rectifier and inverter. The rectifier is a device that transforms an AC source to DC. AC loads were used in both residential and commercial applications. Inverter circuits included in order to convert electricity to AC load from battery or solar. The solar, biomass and wind energy can be used to meet load demand instead of using a diesel generator so that a green environments created when optimal solution reached. Figure 1 shows a hybrid system block diagram.
The objective is to investigate a standalone hybrid microgrid system with minimum optimized cost for solar/wind/biomass and solar/biomass/diesel generator configurations and to analyze unmet load reliability indice and environmental pollutions for proposed microgrid system
The scope of the research work is,
● To propose a hybrid standalone microgrid system in remote areas where extension of grid is more cost.
● The use of renewable energies in the Island area reduces the emission when the system operated with non-conventional sources and diesel generator.
In the optimization problem, the objective function formulation is given below Eq (3.1),
MinF(cost)=Min(NPC) | (3.1) |
The net present cost 'NPC' is calculated from the Eq (3.2),
NPC=(Cann,TCRF(d,lt)) | (3.2) |
where CRF (d, lt) is capital recovery factor and Cann, T is the total annualized cost.
The capital recovery factor CRF (d, lt) is estimated from the Eq (3.3),
CRF(d,lt)=(d∗(d+1)lt(d+1)lt−1) | (3.3) |
where
d=dr−fidr+fi | (3.4) |
where lt is lifetime, d is the interest rate, fi is the inflation rate and dr is the discount rate.
The total annualized cost is evaluated based on adding replacement cost, capital cost and operation and maintenance cost of each component in hybrid microgrid system.
The cost of energy (COE) is evaluated based on the Eq (3.5),
COE=NPC∑8760t=1(Energyconsumedbyload)∗CRF | (3.5) |
In this study, the reliability indice like unmet load is estimated for each hybrid system. Mathematically, it is represented as shown in Eq (3.6),
Unmetload=Loaddemandduration∗∑Nt=0(Paccessible(t)<Pneeded(t)) | (3.6) |
The total generated power from different hybrid configurations (solar/WT/biomass generator (BG), solar/diesel generator (DG)/biomass generator (BG)) should satisfy load demand every point of time. Eqs (3.7) and (3.8) shows the power balance constraints.
For solar/WT/BG hybrid system,
PS(t)+PWT(t)+PBG(t)+PB,dis(t)−PB,ch(t)≥PL(t) | (3.7) |
For solar/DG/BG hybrid system,
PS(t)+PDG(t)+PBG(t)+PB,dis(t)−PB,ch(t)≥PL(t) | (3.8) |
where,
PS (t) - Solar power generation for each time period,
PWT (t) - Wind power generation for each time period,
PDG (t) - Diesel generator power generation for each time period,
PBG (t) - Biomass generator power generation for each time period,
PB, ch(t) - Battery charging power for each time period,
PB, dis (t) - Battery discharging power for each time period
PL (t) - Load power for each time period
For battery state of charge (SOC), the constraints followed during optimization processis given in Eq (3.9),
SOCmin<SOC(t)<SOCmax | (3.9) |
where, SOCmin is 20% and SOCmax is 100%.
The number of solar photovoltaic panels, wind turbines (WT) and batteries should follow the constraints shown in Eqs (3.10) and (3.11),
0≤NSPV≤NSPV,max | (3.10) |
0≤NWT≤NWT,max | (3.11) |
0≤NBattery≤NBattery,max | (3.12) |
where, NSPV, NWT, NBattery are number of solar Photovoltaic panels, wind turbine and batteries. NSPV, max, NWT, max, NBattery, max are the maximum number of solar PV panels, wind turbine and batteries allowed for optimization respectively.
The methodology adopted in the research work is depicted in Figure 2.
A detailed literature of standalone microgrid system is conducted. Various data that includes the description of the proposed site with meteorological data, estimated average daily load consumption, average solar irradiation and wind speed data for Lakshadweep Island was downloaded from NASA surface meteorology and database of solar energy, biomass availability for selected site, proposed standalone microgrid system configurations with solar/wind turbine/biomass/diesel generator for selected remote area to satisfy the load demand. The different electrical factors like solar PV penetration, capacity of solar, wind and biomass are evaluated. The different cost of various components in microgrid system are given as input to HOMER where the lifetime of project is considered as 25. The reliability, electrical factors like capacity factor, renewable fraction and solar penetration are highlighted. The mathematical model of each component in microgrid system is formulated. Then optimization results are performed using HOMER software to minimize the COE and NPC. Sensitivity analysis is also performed for different cases. The minimized COE and NPC are noted for each hybrid system configuration. Then reliability indices unmet load and different emissions in various hybrid system are analyzed.
The above Figure 3 shows the frequency of occurrence of different capacities of load consumption in a year. The load demand of 10 kW–12.5 kW, 31.24% occurred which is the highest occurrence over the year. Then the load demand of 72.5 kW–75 kW, 0.05% occurred which is the lowest occurrence over the year. Similarly, other load demand occurrence are shown in Figure 3. Based on above load demand over a year, the system is simulated with cost of various components to evaluate the system cost. The average solar radiation considered is 5680 Wh/m2/day.
The solar panel, wind turbine and biomass generator need solar irradiation, wind speed and biomass to generate power. The average solar irradiation and speed of wind for Jan-Dec is shown in Figure 4. The biomass availability is given as 1 tons/day in HOMER software to perform optimization.
Table 1, shows the specification of biomass and diesel generator. The diesel generator emits 2.61 liter/Kg CO2 emission. The DG emits other emission like carbon monoxide (16.5 g/L) and nitrogen oxides (15.5 g/L). The cost of diesel generator is 220 $/kWh and the 0.020 $/ op. hours is considered as maintenance and operation cost. The cost of biomass generator is 300$ for 1 kW capacity.
Life (hrs) | Price | Fuel type |
10000 | 1$/L | Diesel |
20000 | 50$/ton | Biogas |
The wind turbine output 'OPWT' depends mainly on speed of wind and it is evaluated by the following Eq (3.13),
OPWT=(u3ρair2)∗AsPwinwatts | (3.13) |
where u is speed of wind of selected site in m/s, ρair is the density of air, AS is the swept area of wind turbine rotor in m2 and PW is wind turbine coefficient of power.
The solar power output 'OPsolar' mainly depends on solar irradiation and evaluated based on the Eq (3.14),
OPSolar=[α(TSC−TSSC)+1]FDPRated∗r(1rs) | (3.14) |
where, α is PV power coefficient in %/℃, TSC is the temperature of PV panel in ℃ and TSSC is the standard temperature of PV panel. 'r' is the solar irradiation in kW/m2, rs is the standard radiation in kW/m2 (1 kW/m2), FD is derating factor (80%) and PRated is the PV rated power in kW.
The cost of 1 kW wind turbine and 1 kW solar panel are 2000$ and 640$ respectively. The solar panel will operate for entire lifetime but the wind turbine will operate only for 80% of the lifetime.
The power output of converter is represented by the Eq (3.15),
Poutput(t)=Pinput(t)∗ηconv | (3.15) |
where Pinput(t) is the power input of converter and ηconv represents the efficiency of the converter.
The battery capacity 'BC' is evaluated using Eq (3.16),
BC=bad∗LdNbattery∗ηb∗DOD | (3.16) |
where Ld is the load demand in kW, bad is the autonomy battery hours, ηb is the efficiency in %, DOD is the depth of discharge and Nbattery is the total No. of batteries.
The state of charge (SOC) during discharging and charging condition depends on the previous time SOC, total generation and total load demand.
The cost of battery and converter are considered as 140$ and 300$ respectively.
For modelling the wind speed, the Weibull distribution is a suitable one. The variation of Weibull shape factor 'p' shows different Weibull probability density function. The variation of the factor 'p' tends to change in different parameters of hybrid microgrid system consisting of solar/wind turbine/biomass generator. The Weibull distribution with two different parameters like Weibull shape factor 'p' and Weibull scale parameter 'q' are used to characterize wind regimes with measured wind speed data, to provide a suitable fit in a suitable site. The Weibull probability density function is shown in Eq (3.17),
f(u)=exp[−(uq)p].pq(uq)p−1. | (3.17) |
where, 'u' is the speed of wind in m/s, 'p' is the Weibull shape factor and 'q' is the Weibull scale parameter (m/s).
The Weibull shape factor 'p' is initially assumed as 2 as initial guess. Then the Weibull scale parameter 'q' can be solved using the Eq (3.18),
q=(1n∑ni=1upi)1╱p | (3.18) |
where, 'n' is the hourly speed of wind which is not equal to zero, 'ui' is the wind speed for each hour and 'p' is the Weibull shape factor.
From the above Eq (3.18), Weibull scale parameter 'q' is evaluated for different values of Weibull shape factor 'p' based on selected site average wind speed. The various Weibull shape factor 'p' considered in this study are 1.5, 1.8, 2, 2.2, 2.5
The above Table 2 shows that, for different Weibull shape factors 'p', Weibull scale parameter 'q' changes according to the Eq (3.18). These two parameters, 'p' and 'q' are important parameters for Weibull probability distribution function.
p | 1.5 | 1.8 | 2 | 2.2 | 2.5 |
q | 5.65 | 5.96 | 6.12 | 6.25 | 6.48 |
The Figure 5, shows the Weibull probability density function of wind speed for various value of Weibull shape factor 'p' considering average wind speed at 5.38m/s.
There is a small variation of distributing wind speed for a higher value of Weibull shape factor 'p'.
The system with biomass, wind turbine and solar resources is simulated for various iterations in HOMER software to minimize the net present cost. After several combinations of hybrid system with various capacities, the Table 3, shows the optimal result for biomass/wind/PV.
System parameters | Values | System parameters | Values |
BG capacity | 30 kW | Battery capacity | 433 kWh |
BG Production | 51717 kWh/yr | Battery energy out | 86,200 kWh/yr |
PV capacity | 96.8 kW | Unmet load | 0.0424% |
PV Production | 216,688 kWh/yr | Unmet load | 66.1 kWh/yr |
PV Penetration | 139% | COE | 0.240 $/kWh |
PV capacity factor | 25.5% | NPC | 484,267$ |
From the Table 3, it is noted that the photovoltaic panel produces 216688 kWh/yr with optimal sizing of 96.8 kW to satisfy the load demand. Additionally, 30 kW biomass generator (BG) source added in the system for continuous supply to the load because of the intermittent nature of solar generation system. This biomass produces 51717 kWh/yr. The wind turbine is not included in the optimization result of hybrid system with minimized cost. The COE is more than 0.240 $/kWh when wind turbine is included in hybrid microgrid system. The battery is also operated as a storage device with an optimal sizing of 433 kWh during operation of the system annually. The battery charges when there is an excess power flow and discharges when solar source is not available especially during night time. The battery produces total energy of 86200 kWh/yr. The PV penetration and PV capacity factor are 139% and 25.5% respectively which is evaluated based on output of photovoltaic system, capacity of photovoltaic system and average load demand. The reliability factor like unmet load is evaluated based on load demand and hourly output of energy sources like biomass and solar. The unmet load is 61.6 kWh/yr and 0.0424%. Thus, the microgrid system can't meet 61.6 kWh load demand in a year. Due to intermittent nature of the renewable sources, this situation happened. Depending on the above operation, the COE and NPC for overall system is 0.240 $/kWh and 484267 $ respectively.
The Figure 6 shows the net present cost of 1kWh lithium-ion battery, biogas genset, solar photovoltaic system and system converter. The net present cost is the combination of capital cost 'CC', cost of replacement 'CR' and operation and maintenance cost 'COM'. The net present cost of 1kWh battery, biogas genset, solar photovoltaic system and system converter are $ 181929, $ 186545, $ 91923, $ 23868 respectively.
Figure 7 shows that the monthly average electricity production for biomass/PV/wind turbine microgrid system. The solar contributes 80.7% and biomass generator contributes 19.3% during optimization results of biomass/solar/WT. Based on solar irradiation of selected site, the solar contributes more power each month in a year to satisfy the demand and biomass produces more power from March to August as compared to other months in a year. From September to December, the biomass generator produces power to satisfy demand in less capacity.
The above Figures 8 and 9, shows the fuel consumption of biomass for each month and hours in a year respectively. Totally 157 tons feedstock are consumed. The average feedstock per day and average feedstock per hour are 0.431 tons/day and 0.0179 tons/hour respectively.
The system is simulated with minimum cost with wind turbine operated in biomass/solar/wind hybrid system. The wind turbine produces 944 kWh/year to satisfy the load demand as shown in Table 4. But the cost of energy is high (COE = 0.401 $/kWh) when the system is operated only with solar and wind turbine (WT). In this case, the wind turbine produces 1888 kWh/year. The system is operated with high cost (COE = 1.77 $/kWh) when operating with wind turbine and biomass. In this case, the wind turbine produces 808,186 kWh/year with 856 kW capacity and biomass produces 19,370 kWh/year to satisfy load demand for the selected site location.
System parameters | Values | System parameters | Values |
BG capacity | 30 kW | Battery capacity | 428 kW |
BG Production | 51,067 | Battery energy out | 85,986 kWh/yr |
PV capacity | 99.2 kW | Unmet load | 0.0405% |
PV Production | 222,008 | Unmet load | 63.1 kWh/yr |
WT capacity | 1 kW | COE | 0.241 $/kWh |
WT Production | 944 | NPC | $485,641 |
Depending on the load demand data, the system is simulated and after several simulations, the best optimization result is determined with minimum cost.
From Table 5, the photovoltaic panel produces 228913 kWh/yr with a capacity of 123 kW to satisfy the load consumption. So, 123 number of solar panels are used to satisfy the load demand. Since the capacity of each solar panel is 1 kW, the number of solar panels is same as the capacity of solar. The optimal sizing of different sources like 30 kW biomass generator (BG) and 85 kW diesel generator (DG) are added in the system for continuous operation due to intermittent nature of solar generation system as a component optimal sizing. Biomass produces 12434 kWh/yr. The battery also operated as a storage device with an optimal sizing of 440 kWh during operation of the system annually. The battery charges when there is an excess power flow and discharges when solar source is not available especially during night time. The battery produces total energy of 88897 kWh/yr. The PV penetration and PV capacity factor are 147% and 21.2% which is evaluated based on output of photovoltaic system, capacity of photovoltaic system and average load demand. The reliability factor like unmet load is evaluated based on load demand and hourly output of non-conventional energies like biomass and solar. There is no unmet load for this hybrid system consisting of biomass/DG/solar. Since there is no unmet load, this system produces continuous power supply without any interruption. Based on the above operation of the system, the COE and NPC for overall system are 0.215 $/kWh and 432513$ respectively. The net present cost of various components in biomass/DG/solar are shown in Figure 10.
System parameters | Values | System parameters | Values |
BG capacity | 30 kW | Battery capacity | 440 kWh |
BG Production | 12434 kWh/yr | Battery energy out | 88897 kWh/yr |
PV capacity | 123 kW | Unmet load | 0% |
PV Production | 228913 kWh/yr | Unmet load | 0 kWh/yr |
PV Penetration | 147% | COE | 0.215 $/kWh |
PV capacity factor | 21.2% | NPC | 432513 $ |
Production of DG | 8604 kWh/yr | Capacity of DG | 85 kW |
The net present cost is evaluated for each component. After optimization process completed, the result shows that the NPC of diesel generator, Li-ion battery, biogas generator, photovoltaic system and converter are $57440, $184870, $59362, $107055 and $23784 respectively. The total NPC is $432513. It is also found that the total emission in biomass/solar and biomass/DG/solar are 267.74 kg/year and 7506 kg/year respectively.
Figure 11 shows the monthly average electricity production in biomass/solar/diesel generator. The solar panels contribute 91.6%, biomass contributes 4.97%, diesel generator contributes 3.44%. During Jan-Feb and Sep-Dec, almost solar alone operating to meet the load. During Mar-Aug, DG and biomass generator contributes a little amount to balance the intermittent operation of solar panels. During the month of June, the diesel generator and biomass contributes more compared to other months due to less average solar irradiation in this month at 4680 Whr/m2/day.
The different values of Weibull shape factor 'p' in solar/wind turbine/biomass hybrid system has a significant role on operating different sources with optimal solution. Based on NPC and COE the optimal solution is decided to operate with various sources to fulfil the demand. There will be different distribution of wind speed with variation of Weibull shape factor 'p' in wind source. The average wind speed and irradiation of solar is higher in top right of optimal system plot and average speed of wind and irradiation of solar is lower in bottom left of optimal system plot as shown in Figures 12–14. The different values of average solar irradiation (4 kWh/m2/day, 4.5 kWh/m2/day, 5 kWh/m2/day, 5.68 kWh/m2/day and 6 kWh/m2/day) and average wind speed (4.5 m/s, 5 m/s, 5.38 m/s, 6 m/s and 6.5 m/s) are considered as a sensitive case in optimal system plot.
Figure 12 shows the distribution of wind speed according to solar radiation in solar/wind turbine/biomass microgrid system with different Weibull shape factors 'p' is graphical representation by optimal system plot. According to Figure 12 (a), When solar irradiation is 5.05 kWh/m2/day and wind speed is 6.5 m/s, the wind turbine operates with biomass and solar photovoltaic system. The wind turbine is operating in hybrid system when solar irradiation is 5.67 kWh/m2/day and wind speed is 6.17 m/s and also when wind speed is high near to 6.5 m/s and 5 m/sand with less solar irradiation like 4 kWh/m2/day to 4.3 kWh/m2/day. For remaining solar irradiation and wind speed, the solar operates with biomass without wind turbine as shown in Figure 12(a). In Figure 12(b), when solar irradiation varies from 4.6 kWh/m2/day to 5.1 kWh/m2/day and 4 kWh/m2/day to 4.2 kWh/m2/day with average wind speed around 6.5 m/s alone wind turbine is operated with solar and biomass generator. For other values of solar irradiation with wind speed less than 6.4 m/s, only solar and biomass operating with low net present cost. In every case, lithium-ion battery is operating in hybrid system. From the above Figure 12 (a), (b). The biomass fuel used in biomass generator varies from 151 tons to 192 tons during different values of solar irradiation and wind speed when Weibull shape factors 'p' is 1.5 and 1.8. The usage of biomass is less when the solar irradiation is 6 kWh/m2/day with less wind speed. The usage of biomass is more when the solar irradiation is less with more wind speed.
The Figure 13 shows the distribution of wind speed according to solar radiation in solar/wind turbine/biomass microgrid system with different Weibull shape factors 'p' is graphical representation by optimal system plot. The wind turbine is not operated when Weibull shape factors 'p' considered as 2 in hybrid system consisting of solar PV/biomass/WT with minimum cost for different solar irradiation and wind speed. The wind turbine operating with Weibull shape factors 'p' as 2.2 in hybrid system with low cost when solar irradiation varies from 4.2 kWh/m2/day to 4.6 kWh/m2/day and 5.3 kWh/m2/day to 6.0 kWh/m2/day with wind speed almost more than 6 m/s. Thus, according to the Figure 13(b), the wind turbine is not operated for most of sensitive cases due to increase in net present cost. The use of biomass fuel varies from 151 tons to 189 tons for different sensitive case without the wind turbine in operation. The use of biomass is less with just 30 tons when wind turbine is operated with solar and biomass for Weibull shape factors 'p' as 2.2.
Figure 14 shows the distribution of wind speed according to solar irradiation in solar/wind turbine/biomass microgrid system with Weibull shape factors 'p' as 2.5 is graphical representation by optimal system plot. When the Weibull shape factor 'p' is increased to 2.5, the biomass/solar/WT is operated for sensitive cases when compared to the Weibull shape factor 'p' less than 2.5. The optimal system configuration for different solar irradiation and wind speed are considered in the optimal system plot. For every solar irradiation value between 4 kWh/m2/day to 6 kWh/m2/day and with average wind speed at least greater than 6 m/s, this configuration is in operation with minimum cost. The solar and biomass alone is operating with minimum cost when the speed of wind is less than 6 m/s with different values of solar irradiation varies from 4 kWh/m2/day to 6 kWh/m2/day. According to the optimization result, the use of biomass in hybrid system varies between 29 tons and 54 tons when the system is operated with wind turbine. When the hybrid system not operated with wind turbine, the use of biomass increases and ranges between 154 tons to 201 tons.
Figure 15, shows the summary of NPC when Weibull shape factor 'p' is 2 in hybrid microgrid system when average solar irradiation and wind speed are 6 kWh/m2/day and 6.5 m/s respectively. The NPC is the main parameter in optimization problem which should be minimized in every hybrid microgrid configurations. When the hybrid system with solar, biomass and wind turbine is considered, there would be different combinations. The different combinations consist of solar/biomass, solar/WT/biomass, solar/wind turbine, solar alone, biomass/WT and wind turbine alone. For all above configurations, the net present cost is less in solar/biomass hybrid system with net present cost of 473824.9$ when the average irradiation of solar and speed of wind are 6 kWh/m2/day and 6.5 m/s. The net present cost is high when the system has wind turbine alone. Thus, according to Figure 15, the solar/biomass is the suitable hybrid system with low cost.
Initially, the maximum annual capacity shortage is set to 0% in HOMER software. In that case, the unmet load will be negligible due to very less value. But when the maximum capacity shortage is allowed to some extent other than 0%, there will be an increase in unmet load. In that case, the microgrid system can't able to supply power to the load demand every period of time. This optimal system plot shown in Figure 16, helps to understand the consequences of changing the maximum annual capacity shortage in microgrid system. In that Figure 16, a two-dimensional plot with different sensitive values of solar irradiation and wind speed are highlighted in optimal system plot. As a base case, the Weibull shape factor 'p' is considered as 2 during the analysis of changing the maximum annual capacity shortage. Different sources like solar PV/WT/biomass generator may operate while the environmental condition changes. While the average solar irradiation and speed of wind changes, there will be different optimal solution with suitable combinations of hybrid system.
The Figure 16 shows the different variation in maximum annual capacity shortage 'CSmax' in microgrid system. The 'CSmax' is considered as 10% and 12% in this study. In Figure 16 (a), optimal system plot for 10% maximum annual capacity shortage for solar/WT/biomass hybrid system is considered with different average solar irradiation and wind speed. The optimization results show that, when solar irradiation is between 4 kWh/m2/day and 4.8 kWh/m2/day and different wind speed variation from 4.5 m/s to 6.5 m/s, the optimal solution is the system that operates with solar and biomass. When solar irradiation is between4.8 kWh/m2/day to 6 kWh/m2/day and wind speed ranges from 4.5 m/s to 5.8 m/s and 5.8 m/s and 6.5 m/s, only solar operates with minimum cost. The solar and wind turbine operating with minimum cost when the solar irradiation is from 4.8 kWh/m2/day to 5.1 kWh/m2/day and wind speed varies from 5.8 m/s to 6.5 m/s. Similarly, during very high wind speed and solar irradiation, this solar/Wind turbine is operated. The unmet load is the reliability indice in which the generation will not satisfy the demand for particular period of time. The unmet load is represented by kWh/year. Since the maximum annual capacity shortage is allowed for 10%, the unmet load is high and it varies from 7496 kWh/year to 11553 kWh/year in the optimal system plot.
According to the Figure 16(b), almost always solar alone operates in hybrid system for different sensitivity case with minimum cost. When average solar irradiation varies from 4.08 kWh/m2/day to 6 kWh/m2/day, the solar source operating with wind speed varies from 4.5 m/s to 6 m/s. When average wind speed is greater than 6 m/s in optimal system plot and solar irradiation varies from 4.1 kWh/m2/day to 4.9 kWh/m2/day, the optimal solution based on solar and wind source. The biomass and solar source are operated in hybrid microgrid system for various sensitive values of wind speed and when the solar irradiation is less than 4.08 kWh/m2/day. The unmet load varies from 7374 kWh/year to 13654 kWh/year in optimal system plot as shown in Figure 16(b). The system operating with solar and biomass has less unmet load compared to other combinations of sensitive values.
The comparison of different diesel generator scheduling is presented to determine optimal solution in each scheduling. When the diesel generator (DG) is scheduled for particular period of time, there will be a change in optimization result with net present cost variation. The diesel generator is allowed to forced ON/OFF during particular period depending on the circumstance. The main drawback in renewable sources is that the output of solar/wind are intermittent in nature. So, the output is varying for every period of time. There is a chance in unsatisfying the load at a particular time when a system is operated only with solar/wind with battery. When number of batteries more, it will increase the net present cost of the system. Thus, the scheduling of diesel generator has an impact on net present cost of microgrid system.
Figure 17 shows the diesel generator (DG) scheduling (Scenario-1). The scenario-1is the base case, where no scheduling of generator takes place. Depending on the availability of different sources, each source operates in solar/DG/BG system for each time period in a year with minimum cost. During this case, the auto size diesel generator will operate based on the load requirement for each hour in a year. Whenever the solar irradiation is highly available, the system operates only with solar at a minimum cost.
Figure 18 shows the diesel generator (DG) scheduling (Scenario-2). From 7 a.m. to 6 p.m., the diesel generator is allowed to forced OFF for the entire year. The solar irradiation is available in this period and the solar panels will operate during the day. At night time when sun light is not available, there will be no output power of solar panel. With the intermittent operation of solar during day time, mainly the lithium-ion battery used as a supporting source to satisfy the load demand. At night, from 6 p.m–7 a.m., the auto size diesel generator will operate with sources like battery and biomass generator.
Figure 19 shows the diesel generator (DG) scheduling (Scenario-3). This generator scheduling is based on forced OFF of the diesel generator during day time from February to May. The diesel generator is not allowed to operate from 7 a.m. and 6 p.m. for these 4 months in a year. The reason behind this scheduling is that, the average solar irradiation is more during February, March, April, May months for this selected site location. Thus, to operate the solar panel with battery in an effective manner, this scheduling takes place to get an optimal solution. The average solar irradiation during the month of February, March, April, May are 6.43 kWh/m2/day, 6.92 kWh/m2/day, 6.81 kWh/m2/day and 5.97 kWh/m2/day respectively.
Figure 20 shows the diesel generator (DG) scheduling (Scenario-4). In the time gap between 6 a.m. to 6 p.m., the diesel generator is allowed to forced OFF since the solar irradiation will be available to fulfil the load demand with battery. Aside from forced OFF the diesel generator, the diesel generator is allowed to forced ON on June month from 12 a.m. to 5 a.m. and from 7 p.m. to 11 p.m. when there is no solar irradiation at night. The diesel generator is ON at night in June since average solar irradiation is less on June month as 4.68 kWh/m2/day.
In Solar/BG/DG hybrid system, different parameters in hybrid system is analyzed for different diesel generator scheduling scenario as shown in Table 6. It shows the summary of hybrid system under different generator scheduling.
Parameters | Units | Scenario-1 | Scenario-2 | Scenario-3 | Scenario-4 |
COE | $/kWh | 0.215 | 0.218 | 0.214 | 0.236 |
NPC | $ | 432513 | 439,346 | 431,454 | 475,822 |
Unmet load | % | 0 | 0.00757 | 0 | 0.00662 |
Unmet load | kWh/yr | 0 | 11.8 | 0 | 10.3 |
CO2 emission | Kg/yr | 7396 | 3885 | 7772 | 9095 |
Solar capacity | kW | 123 | 147 | 116 | 160 |
DG capacity | kW | 85 | 85 | 85 | 85 |
BG capacity | kW | 30 | 30 | 30 | 30 |
Capacity of battery | kW | 440 | 499 | 435 | 469 |
Electricity production (PV) | kWh/yr | 228913 | 272,462 | 214,779 | 297,448 |
Electricity production (DG) | kWh/yr | 8604 | 4694 | 9109 | 9543 |
Electricity production (BG) | kWh/yr | 12434 | 8039 | 13837 | 9547 |
Energy out (battery) | kWh/yr | 88897 | 94,350 | 87,752 | 88,311 |
Total feedstock consumed | Tons | 39 | 25.2 | 43.4 | 29.9 |
Total fuel consumed | Litre | 2800 | 1468 | 2941 | 3455 |
The optimization result of Solar/DG/BG configuration shows that the COE and NPC is minimum in DG scheduling scenario-3 when compared to other scenarios. In scenario-1, the diesel generator is not allowed to forced ON/OFF during particular period of time. The different capacities and electricity production of microgrid system components are highlighted in Table 5. Additionally, fuel consumption of diesel generator and biomass generator are also determined. The CO2 emission is 3885 kg/year in scenario-2, which is less when compared to other scenarios. There is no unmet load in scenario-1 and 3. Also in other scenarios, the unmet load also very less. The diesel generators consume 3455-liter fuel in scenario-4, which is more as compared to other scenarios. The biomass generator consumes more fuel in scenario-3, which is more as compared to other scenarios. So, based on electricity produced by diesel and biomass generator, the fuel is consumed.
In hybrid system solar/DG/BG, the best DG scheduling is identified and discussed based on scheduling the diesel generator in different scenarios. During optimization process, low cost is the main objective of the study. While comparing four types of scheduling of DG's, it is found that the best scheduling is scenario-3 with minimum cost of energy and net present cost.
The diesel generator is not allowed to operate from 7 a.m. and 6 p.m. for March for a year. The above Figure 21 shows the energy balance in solar/DG/biomass system based upon best DG scheduling on 28th march. The diesel generator operated only from 12 a.m. to 3 a.m. at different capacities like 35.94 kW, 42.61 kW, 65.24 kW, 51.11 kW. The remaining hours during night time (4 a.m. to 6 a.m.) power is supplied by biomass generator (BG). The battery gets charged from the excess power of biomass generator compared to load demand for the particular period of time (4 a.m.–6 a.m.). The solar operates dominantly from 8 a.m. to 6 p.m. with different capacities. During this period, lithium-ion battery gets charged when excess power flow from solar panel occurs as compared to load demand for time 't'. The lithium-ion battery gets discharged from 7 p.m to11 p.m. to support the load demand. During discharge of battery, the lithium-ion battery follows the constraints given for SOCmin. Apart from power of battery, the biomass generator supports the load demand of 66.61 kW at 11 p.m.
The optimal sizing and optimization of cost for solar/wind/biomass and solar/diesel generator/biomass are investigated using HOMER software. The optimization results shows that the solar and biomass generator with optimal sizing of 96.8 kW and 30 kW respectively are operated when the system is simulated with solar/wind/biomass. The net present cost and cost of energy for this configuration are 484,267$ and 0.240 $/kWh respectively. Similarly, the optimization results of solar/diesel generator/biomass configuration have the optimal sizing of 123 kW solar, 85 kW diesel generator and 30 kW biomass generator. This configuration has the lowest NPC of 432513$ and cost of energy at 0.215 $/kWh. The impact of Weibull shape factor on solar/wind/biomass system performed with different solar irradiation and wind speed sensitive results. The impact of 10% and 12% capacity shortages on reliability factor for solar/wind/biomass system analyzed. The results shows that the unmet load was high when the system was allowed to capacity shortage. The different diesel generator scheduling was performed in solar/DG/biomass system. The results shows that the proper scheduling of diesel generator has the significant impact on NPC and COE. The best diesel generator scheduling optimization results shows that the system has 431,454 $ net present cost and 0.214 $/kWh cost of energy. The total feedstock consumed for solar/DG/biomass system while considering scheduling of diesel generators is less as 25.2 tons for biomass and 1468 litre for diesel generator in scenario-2. In scenario-2, the diesel generator is allowed to forced OFF during morning time for an entire year. This work exhibits that the standalone microgrid system with solar/DG/biomass is best suited for the selected site with low cost.
The future scope of the work is that the microgrid system with solar/wind/biomass can be analyzed on integrating electric vehicle charging station with microgrid for the selected site according to environmental benefits. Beyond renewable energies like solar PV, WT and biomass, the microgrid system with tidal energy can be analyzed for electrifying various load demands in Island. The maintenance schedule for wind turbine can be considered in microgrid operation for functioning of wind turbine without any failure.
The authors declare no conflicts of interest.
[1] |
Ai B, Yang B, Shen H, et al. (2003) Computer-aided design of PV/Solar hybrid system. Renewable Energy 28: 1491–1512. https://doi.org/10.1016/S0960-1481(03)00011-9 doi: 10.1016/S0960-1481(03)00011-9
![]() |
[2] |
Billinton R, Karki R (2001) Capacity expansion of small isolated power systems using PV and Solar energy. IEEE Trans Power Syst 16: 892–897. https://doi.org/10.1109/59.962442 doi: 10.1109/59.962442
![]() |
[3] |
Fujisaki T (2018) Evaluation of Green Paradox: Case study of Japan. Evergreen: Green Asia Educ Cent 5: 26–31. https://doi.org/10.5109/2174855 doi: 10.5109/2174855
![]() |
[4] |
Ghahderijani MM, Barakati MS, Tavakoli S (2012) Reliability evaluation of stand-alone hybrid microgrid using Sequential Monte Carlo simulation, Proceedings: IEEE Second Iranian conference on renewable energy and distributed Generation, 33–38. https://doi.org/10.1109/ICREDG.2012.6190464 doi: 10.1109/ICREDG.2012.6190464
![]() |
[5] | Aien M, Biglari A, Rashidinejad M (2013) Probabilistic reliability evaluation of hybrid wind-photovoltaic power systems. Proceedings: International Conference on Electrical Engineering, Mashhad, Iran, 1–6. https://doi.org/10.1109/IranianCEE.2013.6599825 |
[6] |
Leite AM, Manso LAF, Mello JCO, et al. (2000) Pseudo-chronological simulation for composite reliability analysis with time varying loads. IEEE Trans Power Syst 15: 73–80. https://doi.org/10.1109/59.852103 doi: 10.1109/59.852103
![]() |
[7] | Karki R, Billinton R (2004) Considering renewable energy in small isolated power system expansion. Proceedings: Canadian Conference on Electrical and Computer Engineering, 367–370. |
[8] |
Wangdee W, Billinton R (2005) Reliability-performance-index probability distribution analysis of bulk electricity systems. Canadian J Electr Comput Eng 30: 189–193. https://doi.org/10.1109/CJECE.2005.1541750 doi: 10.1109/CJECE.2005.1541750
![]() |
[9] | All India installed capacity of power stations. (2022) Available from: https://npp.gov.in/publishedReports. |
[10] |
Rathore A, Patidar NP (2019) Reliability assessment using probabilistic modelling of pumped storage hydro plant with PV-Solar based standalone microgrid. Electr Power Energy Syst 106: 17–32. https://doi.org/10.1016/j.ijepes.2018.09.030 doi: 10.1016/j.ijepes.2018.09.030
![]() |
[11] |
Alktranee M, Bencs P (2021) Simulation study of the photovoltaic panel under different operating conditions. ACTA IMEKO 10: 62–66. http://dx.doi.org/10.21014/acta_imeko.v10i4.1111 doi: 10.21014/acta_imeko.v10i4.1111
![]() |
[12] |
Sengthavy P, Doumbia ML, St-Pierre DL (2020) Review on the cost optimization of microgrids via particle swarm optimization. Int J Energy Environ Eng 11: 73–99. https://doi.org/10.1007/s40095-019-00332-1 doi: 10.1007/s40095-019-00332-1
![]() |
[13] |
Fathima AH, Palanisamy K (2015) Optimization in microgrids with hybrid energy systems—A review. Renewable Sustainable Energy Rev 45: 431–446, https://doi.org/10.1016/j.rser.2015.01.059 doi: 10.1016/j.rser.2015.01.059
![]() |
[14] |
López RD, Bernal JL, Yusta JM, et al. (2011) Multi-objective optimization minimizing cost and life cycle emissions of stand-alone PV-wind-diesel systems with batteries storage. Appl Energy 88: 4033–4041. https://doi.org/10.1016/j.apenergy.2011.04.019 doi: 10.1016/j.apenergy.2011.04.019
![]() |
[15] |
Shadmand MB, Balog RS (2014) Multi-objective optimization and design of photovoltaic-wind hybrid system for community smart DC microgrid. IEEE Trans Smart Grid 5: 2635–2643. https://doi.org/10.1109/TSG.2014.2315043 doi: 10.1109/TSG.2014.2315043
![]() |
[16] |
Zhao B, Zhang X, Chen J, et al. (2013) Operation optimization of standalone microgrids considering lifetime characteristics of battery energy storage system. IEEE Trans Sustainable Energy 4: 934–943. https://doi.org/10.1109/TSTE.2013.2248400 doi: 10.1109/TSTE.2013.2248400
![]() |
[17] |
Khan FA, Pal N, Saeed SH (2018) Review of solar photovoltaic and wind hybrid energy systems for sizing strategies optimization techniques and cost analysis methodologies. Renewable Sustainable Energy Rev 92: 937–947. https://doi.org/10.1016/j.rser.2018.04.107 doi: 10.1016/j.rser.2018.04.107
![]() |
[18] |
Yang H, Wei Z, Lou C (2009) Optimal design and techno-economic analysis of a hybrid solar-wind power generation system. Appl Energy 86: 163–169. https://doi.org/10.1016/j.apenergy.2008.03.008 doi: 10.1016/j.apenergy.2008.03.008
![]() |
[19] |
Vendoti KS, Muralidhar M, Kiranmayi R (2021) Techno-economic analysis of off grid solar/wind/biogas/biomass/fuel cell/battery system for electrification in a cluster of villages by HOMER software. Environ Dev Sustainability 23: 351–372. https://doi.org/10.1007/s10668-019-00583-2 doi: 10.1007/s10668-019-00583-2
![]() |
[20] |
Bihari SP, Sadhu PK, Sarita K, et al. (2021) A Comprehensive review of microgrid control mechanism and impact assessment for hybrid renewable energy integration. IEEE Access 9: 88942–88958. https://doi.org/10.1109/ACCESS.2021.3090266 doi: 10.1109/ACCESS.2021.3090266
![]() |
[21] |
Bilal BO, Sambou V, Ndiaye PA, et al. (2010) Optimal design of a hybrid solar wind-battery system using the minimization of the annualized cost system and the minimization of the loss of power supply probability (LPSP). Renewable Energy. 35: 2388–2390. https://doi.org/10.1016/j.renene.2010.03.004 doi: 10.1016/j.renene.2010.03.004
![]() |
[22] |
Shi Z, Wang R, Zhang T (2015) Multi-objective optimal design of hybrid renewable energy systems using preference-inspired co evolutionary approach. Sol Energy 118: 96–106. https://doi.org/10.1016/j.solener.2015.03.052 doi: 10.1016/j.solener.2015.03.052
![]() |
[23] |
Diab AAZ, Sultan HM, Mohamed IS, et al. (2019) Application of different optimization algorithms for optimal sizing of PV/wind/diesel/battery storage stand-alone hybrid microgrid. IEEE Access 7: 119223–119245. https://doi.org/10.1109/ACCESS.2019.2936656 doi: 10.1109/ACCESS.2019.2936656
![]() |
[24] | Bashir M, Sadeh J (2012) Optimal sizing of hybrid wind/photovoltaic/battery considering the uncertainty of wind and photovoltaic power using Monte Carlo. International Conference on Environment and Electrical Engineering, Venice, Italy. https://doi.org/10.1109/EEEIC.2012.6221541 |
[25] |
Ma T, Yang H, Lin Lu (2014) Feasibility study of a Stand-Alone Hybrid Solar-Wind-Battery system for a remote island. Appl Energy 121: 149–158. https://doi.org/10.1016/j.apenergy.2014.01.090 doi: 10.1016/j.apenergy.2014.01.090
![]() |
[26] |
Adefarati T, Bansal R (2019) Reliability, economic and environmental analysis of a microgrid system in the presence of renewable energy resources. Appl Energy 236: 1089–1114. https://doi.org/10.1016/j.apenergy.2018.12.050 doi: 10.1016/j.apenergy.2018.12.050
![]() |
[27] | Bansal M, Khatod DK, Saini RP (2014) Modeling and optimization of integrated renewable energy system for a rural site. International Conference on Reliability, Optimization and Information Technology—ICROIT, India, 2014. https://doi.org/10.1109/ICROIT.2014.6798289 |
[28] |
Gami D, Sioshansi R, Denholm P (2017) Data challenges in estimating the capacity value of solar photovoltaics. IEEE J Photovoltaics 7: 1065–1073. https://doi.org/10.1109/JPHOTOV.2017.2695328 doi: 10.1109/JPHOTOV.2017.2695328
![]() |
[29] |
Kamali S, Tyagi VV, Rahim NA, et al. (2013) Emergence of energy storage technologies as the solution for reliable operation of smart power systems: A review. Renewable Sustainable Energy Rev 25: 135–165. https://doi.org/10.1016/j.rser.2013.03.056 doi: 10.1016/j.rser.2013.03.056
![]() |
[30] |
Khananm M, Hasan MF, Miyazaki T, et al. (2018) Key factors of solar energy progress in Bangladesh until 2017. Evergreen 5: 77–85. https://doi.org/10.5109/1936220 doi: 10.5109/1936220
![]() |
[31] |
Kumar M, Saha BB (2015) Energy security and sustainability in Japan. Evergreen 2: 49–56. https://doi.org/10.5109/1500427 doi: 10.5109/1500427
![]() |
[32] |
Gima H, Yoshitake T (2016) Comparative study of energy security in Okinawa Prefecture and the state of Hawaii. Evergreen 3: 36–44. https://doi.org/10.5109/1800870 doi: 10.5109/1800870
![]() |
[33] |
Srivastava A, Bajpai RS (2021) Model predictive control of renewable energy sources in DC microgrid for Power flow control. Int J Energy Convers, 9. https://doi.org/10.15866/irecon.v9i4.20152 doi: 10.15866/irecon.v9i4.20152
![]() |
[34] |
Suresh V, Muralidhar M, Kiranmayi R (2020) Modelling and optimization of an off-grid hybrid renewable energy system for electrification in a rural areas. Energy Rep 6: 594–604. https://doi.org/10.1016/j.egyr.2020.01.013 doi: 10.1016/j.egyr.2020.01.013
![]() |
[35] |
Mariana G, Benevit, Andre G, et al. (2016) Subtle influence of the Weibull Shape Parameter on Homer optimization space of a wind diesel hybrid Gen set for use in southern Brazil. J Power Energy Eng 4: 38–48. http://dx.doi.org/10.4236/jpee.2016.48004 doi: 10.4236/jpee.2016.48004
![]() |
[36] |
Nannam HC, Banerjee A (2021) A novel control technique for a single-phase grid-tied inverter to extract peak power from PV-Based home energy systems. AIMS Energy 9: 414–415. https://doi.org/10.3934/energy.2021021 doi: 10.3934/energy.2021021
![]() |
[37] |
Gamil M, Lotfy M, Ashraf M, et al. (2021) Optimal sizing of a residential microgrid in Egypt under deterministic and stochastic conditions with PV/WG/Biomass Energy integration. AIMS Energy 9: 483–515. https://doi.org/10.3934/energy.2021024 doi: 10.3934/energy.2021024
![]() |
[38] |
Charabi Y, Wahab SA (2020) Wind turbine performance analysis for energy cost minimization. Renewables: Wind, Water Sol, 7. https://doi.org/10.1186/s40807-020-00062-7 doi: 10.1186/s40807-020-00062-7
![]() |
[39] |
Alliche M, Rebhi R, Kaid N, et al. (2021) Estimation of the wind energy potential in various North Algerian regions. Energies 14: 7564. https://doi.org/10.3390/en14227564 doi: 10.3390/en14227564
![]() |
[40] |
Mohammadi K, Alavi O, Mostafaeipour A, et al. (2016) Assessing different parameters estimation methods of Weibull distribution to compute wind power density. Energy Convers Manage 108: 322–335. https://doi.org/10.1016/j.enconman.2015.11.015 doi: 10.1016/j.enconman.2015.11.015
![]() |
[41] |
Celik AN (2003) Energy output estimation for small-scale wind power generators using Weibull-representative wind data. J Wind Eng Indust Aerodynamics 91: 693–707. https://doi.org/10.1016/S0167-6105(02)00471-3 doi: 10.1016/S0167-6105(02)00471-3
![]() |
[42] |
Yang D, Jiang C, Cai G, et al. (2020) Interval method based optimal planning of multi-energy microgrid with uncertain renewable generation and demand. Appl Energy 277: 115491. https://doi.org/10.1016/j.apenergy.2020.115491 doi: 10.1016/j.apenergy.2020.115491
![]() |
[43] |
Cheng Z, Jia D, Li Z, et al. (2022) Multi-time scale dynamic robust optimal scheduling of CCHP microgrid based on rolling optimization. Int J Electr Power Energy Syst 139: 107957. https://doi.org/10.1016/j.ijepes.2022.107957 doi: 10.1016/j.ijepes.2022.107957
![]() |
[44] |
Yang D, Zhang C, Jiang C, et al. (2021) Interval method based optimal scheduling of regional multi-microgrids with uncertainties of renewable energy. IEEE Access 9: 53292–53305. http://10.1109/ACCESS.2021.3070592 doi: 10.1109/ACCESS.2021.3070592
![]() |
[45] |
Tang T, Ding H, Nojavan S, et al. (2020). Environmental and economic operation of wind-PV-CCHP-based energy system considering risk analysis via downside risk constraints technique. IEEE Access 8: 124661–124674. https://doi.org/10.1109/ACCESS.2020.3006159 doi: 10.1109/ACCESS.2020.3006159
![]() |
[46] |
Zhang H, Yue D, Xie X (2016). Robust optimization for dynamic economic dispatch under wind power uncertainty with different levels of uncertainty budget. IEEE Access 4: 7633–7644. https://doi.org/10.1109/ACCESS.2016.2621338 doi: 10.1109/ACCESS.2016.2621338
![]() |
[47] |
Peng C, Xie P, Pan L, et al. (2015) Flexible robust optimization dispatch for hybrid wind/photovoltaic/hydro/thermal power system. IEEE Trans Smart Grid 7: 751–762. https://doi.org/10.1109/TSG.2015.2471102 doi: 10.1109/TSG.2015.2471102
![]() |
[48] |
Mohamad F, Teh J, Abunima H (2019) Multi-objective optimization of solar/wind penetration in power generation systems. IEEE Access 7: 169094–169106. https://doi.org/10.1109/ACCESS.2019.2955112 doi: 10.1109/ACCESS.2019.2955112
![]() |
[49] |
Farrokhabadi M, Solanki BV, Canizares A, et al. (2017) Energy storage in microgrids: Compensating for generation and demand fluctuations while providing ancillary services. IEEE Power Energy Magazine 15: 81–91. https://doi.org/10.1109/MPE.2017.2708863 doi: 10.1109/MPE.2017.2708863
![]() |
[50] |
Nurunnabi M, Roy NK, Hossain E, et al. (2019) Size optimization and sensitivity analysis of hybrid wind/PV micro-grids-a case study for Bangladesh. IEEE Access 7: 150120–150140. https://doi.org/10.1109/ACCESS.2019.2945937 doi: 10.1109/ACCESS.2019.2945937
![]() |
1. | Marcos Tostado-Véliz, Antonio Escámez, Roque Aguado, Daniel Sánchez-Lozano, Francisco Jurado, David Vera, Optimal sizing of hybrid PV–diesel–biomass gasification plants for electrification of off-grid communities: An efficient approach based on Benders’ decomposition, 2024, 458, 09596526, 142360, 10.1016/j.jclepro.2024.142360 | |
2. | Kharisma Bani Adam, Jangkung Raharjo, Desri Kristina Silalahi, Bandiyah Sri Aprilia, , Integrative analysis of diverse hybrid power systems for sustainable energy in underdeveloped regions: A case study in Indonesia, 2024, 12, 2333-8334, 304, 10.3934/energy.2024015 | |
3. | Alvin Henao, Luceny Guzman, Exploration of Alternatives to Reduce the Gap in Access to Electricity in Rural Communities—Las Nubes Village Case (Barranquilla, Colombia), 2024, 17, 1996-1073, 256, 10.3390/en17010256 |
Life (hrs) | Price | Fuel type |
10000 | 1$/L | Diesel |
20000 | 50$/ton | Biogas |
p | 1.5 | 1.8 | 2 | 2.2 | 2.5 |
q | 5.65 | 5.96 | 6.12 | 6.25 | 6.48 |
System parameters | Values | System parameters | Values |
BG capacity | 30 kW | Battery capacity | 433 kWh |
BG Production | 51717 kWh/yr | Battery energy out | 86,200 kWh/yr |
PV capacity | 96.8 kW | Unmet load | 0.0424% |
PV Production | 216,688 kWh/yr | Unmet load | 66.1 kWh/yr |
PV Penetration | 139% | COE | 0.240 $/kWh |
PV capacity factor | 25.5% | NPC | 484,267$ |
System parameters | Values | System parameters | Values |
BG capacity | 30 kW | Battery capacity | 428 kW |
BG Production | 51,067 | Battery energy out | 85,986 kWh/yr |
PV capacity | 99.2 kW | Unmet load | 0.0405% |
PV Production | 222,008 | Unmet load | 63.1 kWh/yr |
WT capacity | 1 kW | COE | 0.241 $/kWh |
WT Production | 944 | NPC | $485,641 |
System parameters | Values | System parameters | Values |
BG capacity | 30 kW | Battery capacity | 440 kWh |
BG Production | 12434 kWh/yr | Battery energy out | 88897 kWh/yr |
PV capacity | 123 kW | Unmet load | 0% |
PV Production | 228913 kWh/yr | Unmet load | 0 kWh/yr |
PV Penetration | 147% | COE | 0.215 $/kWh |
PV capacity factor | 21.2% | NPC | 432513 $ |
Production of DG | 8604 kWh/yr | Capacity of DG | 85 kW |
Parameters | Units | Scenario-1 | Scenario-2 | Scenario-3 | Scenario-4 |
COE | $/kWh | 0.215 | 0.218 | 0.214 | 0.236 |
NPC | $ | 432513 | 439,346 | 431,454 | 475,822 |
Unmet load | % | 0 | 0.00757 | 0 | 0.00662 |
Unmet load | kWh/yr | 0 | 11.8 | 0 | 10.3 |
CO2 emission | Kg/yr | 7396 | 3885 | 7772 | 9095 |
Solar capacity | kW | 123 | 147 | 116 | 160 |
DG capacity | kW | 85 | 85 | 85 | 85 |
BG capacity | kW | 30 | 30 | 30 | 30 |
Capacity of battery | kW | 440 | 499 | 435 | 469 |
Electricity production (PV) | kWh/yr | 228913 | 272,462 | 214,779 | 297,448 |
Electricity production (DG) | kWh/yr | 8604 | 4694 | 9109 | 9543 |
Electricity production (BG) | kWh/yr | 12434 | 8039 | 13837 | 9547 |
Energy out (battery) | kWh/yr | 88897 | 94,350 | 87,752 | 88,311 |
Total feedstock consumed | Tons | 39 | 25.2 | 43.4 | 29.9 |
Total fuel consumed | Litre | 2800 | 1468 | 2941 | 3455 |
Life (hrs) | Price | Fuel type |
10000 | 1$/L | Diesel |
20000 | 50$/ton | Biogas |
p | 1.5 | 1.8 | 2 | 2.2 | 2.5 |
q | 5.65 | 5.96 | 6.12 | 6.25 | 6.48 |
System parameters | Values | System parameters | Values |
BG capacity | 30 kW | Battery capacity | 433 kWh |
BG Production | 51717 kWh/yr | Battery energy out | 86,200 kWh/yr |
PV capacity | 96.8 kW | Unmet load | 0.0424% |
PV Production | 216,688 kWh/yr | Unmet load | 66.1 kWh/yr |
PV Penetration | 139% | COE | 0.240 $/kWh |
PV capacity factor | 25.5% | NPC | 484,267$ |
System parameters | Values | System parameters | Values |
BG capacity | 30 kW | Battery capacity | 428 kW |
BG Production | 51,067 | Battery energy out | 85,986 kWh/yr |
PV capacity | 99.2 kW | Unmet load | 0.0405% |
PV Production | 222,008 | Unmet load | 63.1 kWh/yr |
WT capacity | 1 kW | COE | 0.241 $/kWh |
WT Production | 944 | NPC | $485,641 |
System parameters | Values | System parameters | Values |
BG capacity | 30 kW | Battery capacity | 440 kWh |
BG Production | 12434 kWh/yr | Battery energy out | 88897 kWh/yr |
PV capacity | 123 kW | Unmet load | 0% |
PV Production | 228913 kWh/yr | Unmet load | 0 kWh/yr |
PV Penetration | 147% | COE | 0.215 $/kWh |
PV capacity factor | 21.2% | NPC | 432513 $ |
Production of DG | 8604 kWh/yr | Capacity of DG | 85 kW |
Parameters | Units | Scenario-1 | Scenario-2 | Scenario-3 | Scenario-4 |
COE | $/kWh | 0.215 | 0.218 | 0.214 | 0.236 |
NPC | $ | 432513 | 439,346 | 431,454 | 475,822 |
Unmet load | % | 0 | 0.00757 | 0 | 0.00662 |
Unmet load | kWh/yr | 0 | 11.8 | 0 | 10.3 |
CO2 emission | Kg/yr | 7396 | 3885 | 7772 | 9095 |
Solar capacity | kW | 123 | 147 | 116 | 160 |
DG capacity | kW | 85 | 85 | 85 | 85 |
BG capacity | kW | 30 | 30 | 30 | 30 |
Capacity of battery | kW | 440 | 499 | 435 | 469 |
Electricity production (PV) | kWh/yr | 228913 | 272,462 | 214,779 | 297,448 |
Electricity production (DG) | kWh/yr | 8604 | 4694 | 9109 | 9543 |
Electricity production (BG) | kWh/yr | 12434 | 8039 | 13837 | 9547 |
Energy out (battery) | kWh/yr | 88897 | 94,350 | 87,752 | 88,311 |
Total feedstock consumed | Tons | 39 | 25.2 | 43.4 | 29.9 |
Total fuel consumed | Litre | 2800 | 1468 | 2941 | 3455 |