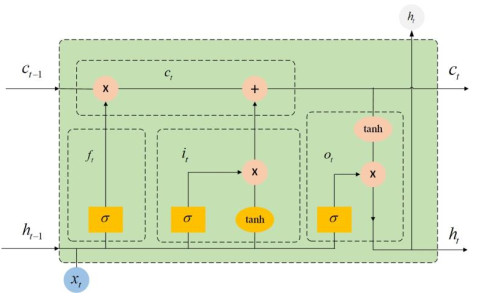
Citation: Silpak Biswas, Rintu Das, Ena Ray Banerjee. Role of free radicals in human inflammatory diseases[J]. AIMS Biophysics, 2017, 4(4): 596-614. doi: 10.3934/biophy.2017.4.596
[1] | Xu Chen, Wenbing Chang, Yongxiang Li, Zhao He, Xiang Ma, Shenghan Zhou . Resnet-1DCNN-REA bearing fault diagnosis method based on multi-source and multi-modal information fusion. Electronic Research Archive, 2024, 32(11): 6276-6300. doi: 10.3934/era.2024292 |
[2] | Shuang Cai, Jiwang Zhang, Cong Li, Zequn He, Zhimin Wang . A RUL prediction method of rolling bearings based on degradation detection and deep BiLSTM. Electronic Research Archive, 2024, 32(5): 3145-3161. doi: 10.3934/era.2024144 |
[3] | Yu Chen, Qingyang Meng, Zhibo Liu, Zhuanzhe Zhao, Yongming Liu, Zhijian Tu, Haoran Zhu . Research on filtering method of rolling bearing vibration signal based on improved Morlet wavelet. Electronic Research Archive, 2024, 32(1): 241-262. doi: 10.3934/era.2024012 |
[4] | Chunpeng Tian, Zhaoyang Xu, Yunjie Liu, Lukun Wang, Pu Sun . SunSpark: Fusion of time-domain and frequency-domain transformer for accurate identification of DC arc faults. Electronic Research Archive, 2024, 32(1): 332-353. doi: 10.3934/era.2024016 |
[5] | Xiaoyan Wu, Guowen Ye, Yongming Liu, Zhuanzhe Zhao, Zhibo Liu, Yu Chen . Application of Improved Jellyfish Search algorithm in Rotate Vector reducer fault diagnosis. Electronic Research Archive, 2023, 31(8): 4882-4906. doi: 10.3934/era.2023250 |
[6] | Zhizhou Zhang, Yueliang Pan, Weilong Zhao, Jinchu Zhang, Zheng Zi, Yuan Xie, Hehong Zhang . Frequency analysis of a discrete-time fast nonlinear tracking differentiator algorithm based on isochronic region method. Electronic Research Archive, 2024, 32(9): 5157-5175. doi: 10.3934/era.2024238 |
[7] | Zhuanzhe Zhao, Mengxian Wang, Yongming Liu, Zhibo Liu, Yuelin Lu, Yu Chen, Zhijian Tu . Adaptive clustering algorithm based on improved marine predation algorithm and its application in bearing fault diagnosis. Electronic Research Archive, 2023, 31(11): 7078-7103. doi: 10.3934/era.2023359 |
[8] | Nan Xu, Longbin Yang, Andrea Lazzaretto, Massimo Masi, Zhenyu Shen, YunPeng Fu, JiaMeng Wang . Fault location in a marine low speed two stroke diesel engine using the characteristic curves method. Electronic Research Archive, 2023, 31(7): 3915-3942. doi: 10.3934/era.2023199 |
[9] | Chang Yu, Qian Ma, Jing Li, Qiuyang Zhang, Jin Yao, Biao Yan, Zhenhua Wang . FF-ResNet-DR model: a deep learning model for diabetic retinopathy grading by frequency domain attention. Electronic Research Archive, 2025, 33(2): 725-743. doi: 10.3934/era.2025033 |
[10] | Abul Bashar . Employing combined spatial and frequency domain image features for machine learning-based malware detection. Electronic Research Archive, 2024, 32(7): 4255-4290. doi: 10.3934/era.2024192 |
Bearings are critical components in rotating machinery and are the primary cause of system faults. In the field of machine health monitoring, diagnosing faults in bearings is crucial. It is estimated that 45–55% of equipment faults are due to issues with bearings [1]. For example, bearings are critical components whose reliable operation is essential for wind turbines. In severe cases, bearing faults can result in downtime, damage, injuries, and significant economic losses [2]. The early detection of weak faults in bearings is paramount for ensuring continuous energy production and preventing potential disruptions or hazards. However, diagnosing faults in bearings poses formidable challenges due to the complexity of operating environments and the subtlety of fault symptoms [3]. Traditional fault diagnosis methods based on signal analysis often fall short in accurately capturing weak fault signatures amidst intricate vibration patterns and environmental noise [4]. Therefore, to guarantee the steady functioning of rotating machinery, the development of efficient fault diagnosis models and the use of advanced intelligent algorithms are crucial [5].
Signal-based methods, a common approach for fault diagnosis [6], are extensively employed in bearing fault diagnosis. Sensors collect time-domain signals, representing vibration signals as data waveforms evolving over time. Time-domain analysis assesses rolling bearing vibration signals using various indexes like mean square value, variance, and kurtosis [7] to determine the occurrence of faults. Nevertheless, the operating environment of the bearing is frequently complicated, and external vibration sources affect the precision of time-domain index computations, causing it difficult to pinpoint the exact location or kind of bearing fault and resulting in potential misjudgments. Consequently, researchers frequently employ Fourier Transform (FT) [8,9] to convert the original signal into the frequency domain, providing a better representation of the bearing's periodic and frequency characteristics. Nevertheless, in real-world situations, the vibration signals produced by spindle equipment operations are not smooth, rendering it difficult to resolve the problem with straightforward time or frequency domain analyses. Consequently, time-frequency analysis techniques for non-linear and non-smooth data decomposition have gained prominence in recent fault diagnosis research. Traditional methods for handling non-linear and non-smooth sequences, such as Wavelet Transform (WT) [10,11], Variational Mode Decomposition (VMD) [12,13], Empirical Mode Decomposition (EMD) [14,15], Ensemble Empirical Mode Decomposition (EEMD) [16,17], and Complementary Ensemble Empirical Mode Decomposition (CEEMD) [18], are widely employed for denoising and feature extraction in bearing fault diagnosis. Yang et al. [19] proposed an IMF prediction method which added all the prediction results of IMF decomposed by EMD, the nonlinear response of cracked rotor can be predicted. The IMF prediction is more advantageous than the Lyapunov prediction method through validation. Ming et al. [20] proposed an optimal wavelet filtering method which is developed by maximizing the L-kurtosis through the genetic algorithm to extract the bearing weak repetitive transients and validated the feasibility of this method. Guo et al. [21] proposed an iterative morphological difference product wavelet and achieved higher diagnosis accuracy. However, when it comes to selecting the Intrinsic Mode Functions (IMF) post-decomposition, the majority of approaches rely on human experience or consider only one aspect, either from the time or frequency domain perspective. This process is often insufficiently comprehensive, making it challenging to accurately identify the IMF that encapsulates the most informative details about early-stage weak faults.
In the realm of deep learning, bearing fault diagnosis methods based on models such as Convolutional Neural Networks (CNN) [22], Long Short-Term Memory (LSTM) [23], Bi-LSTM [24], and Deep Belief Networks (DBN) have gained widespread adoption. Li et al. [25] proposed a connection network constructed by resonance-based sparse signal decomposition and broad learning system without the need for deep architecture for rolling element bearing weak fault and verified the effectiveness of this method. Zhou et al. [26] proposed a novel end-to-end deep network-based sparse denoising framework based on a model-data-collaborative linkage framework for bearing weak fault diagnosis. Jin et al. [27] proposed a weak fault diagnosis method for train axle box bearing based on parameter optimization VMD and improved Deep Belief Network (DBN) for train axle box bearing. Zhong et al. [28] proposed exponential excitation networks, which improves diagnosing performance by amplifying important information, that is, the discriminative information between normal and weak fault conditions. For deep learning models, the choice of hyperparameters directly affects the performance of the model; however, the hyperparameter optimization process often relies on the accumulation of professional knowledge and long-term experience. Manual tuning is cumbersome, costly, and inefficient, and the performance of neural network models is limited by human experience. Therefore, automatically finding the optimal parameters and thus determining the optimal model is essential for efficiently improving the model performance.
To enhance the effectiveness of fault diagnosis using deep learning models, numerous studies have explored the integration of optimization algorithms to improve model performance. Commonly employed algorithms include the particle swarm algorithm [29], cuckoo search algorithm [30], among others. Additionally, to prevent the overlooking of optimal solutions, Gao et al. [31] introduced an adaptive convolutional neural network based on Nesterov momentum for rolling bearing fault diagnosis. The traditional momentum method is replaced by Nesterov momentum, and an adaptive rule dynamically adjusting the learning rate is incorporated to enhance the network's generalization ability. Li et al. [32] devised an automated hyperparameter selection algorithm for motor bearing fault diagnosis. It exhibits superior noise immunity and achieves 100% model accuracy, a notable improvement over other deep learning method.
Early detection of weak faults is crucial for rotating machinery operation, preventing downtime, reducing maintenance costs, extending equipment lifespan, and enhancing safety. However, early weak fault signals in rotating machinery bearings exhibit non-linearity and non-stationarity, posing challenges for effective fault feature extraction. Additionally, traditional diagnostic models often require manual parameter tuning, introducing subjectivity and impacting diagnostic performance. We address these challenges by proposing the adaptive feature extraction algorithm, considering both time-domain and frequency-domain modalities (AFETF), coupled with a GWO-optimized Bi-LSTM algorithm for early weak fault diagnosis in rotating machinery bearings. The effectiveness of the proposed algorithm is demonstrated through comparative analysis. The major contributions are:
1) Utilization of SVD for signal denoising and CEEMDAN for adaptive signal decomposition to obtain Intrinsic Mode Functions (IMFs), beneficial for extracting weak fault features under noisy conditions.
2) Application of the AFETF algorithm to process IMFs, integrating time-domain and frequency-domain modalities information to enhance weak fault signal characterization.
3) Adoption of the GWO-optimized Bi-LSTM algorithm for bearing weak fault diagnosis, improving robustness, and generalization capability by automating hyperparameter optimization.
Singular Value Decomposition (SVD) is a commonly used matrix decomposition method in mathematics [33,34], which has been widely used in signal processing, noise control, and data dimensionality reduction in recent years [35]. In decomposing a matrix using SVD, a matrix of order is assumed, where all the elements belong to the actual or complex domain, and there exists a decomposition equation:
Q=U∑VT, | (1) |
whereU: a∗a unitary matrix, V: b∗b unitary matrix, satisfies UUT=I, VVT=I, T: transpose, ∑: semi-positive definite diagonal matrix, where the elements are zero except the diagonal elements.
The bearing vibration signal is non-smooth and non-linear whereas the Wavelet Transform (WT) and the Empirical Modal Decomposition (EMD) are commonly used methods, the former will produce different effects when dealing with different basis functions, which relies more on people's subjective experience. Complete Ensemble Empirical Mode Decomposition with Adaptive Noise (CEEMDAN) is a further optimized algorithm based on the EMD, which can decompose the signal into several IMFs of a smooth single mode and a cosine term according to the characteristics of the signal itself [36].
Add N times Gaussian white noise zi(t)(t=1,2,…,N) to the original signal y(t). This is shown in the following equation:
yi(t)=y(t)+zi(t). | (2) |
Each original signal y(t) with Gaussian white noise added is decomposed using EMD to obtain a number of IMFs. Assuming that there are M IMFs, and the overall averaging is calculated as shown in the following equation:
¯cm(t)=1M∑Mi=1cm,i(t). | (3) |
According to the Eq (3), the first order IMF can be calculated as shown below:
c1=¯c1(t)=1M∑Mi=1c1,i(t). | (4) |
The residual can be calculated from c1 as:
h1(t)=y(t)−c1. | (5) |
Define yEk(⋅) as the k th IMF after EMD decomposition, pk(t) denotes the signal residual after the k th addition of noise, and A denotes the amplitude of the added noise. Gaussian white noise is added on top of the residual from the first stage of decomposition, and the EMD decomposition is continued for the processed signal residual. The IMFs generated from the first stage residuals are averaged together to obtain the second stage IMF c2:
pk(t)=hk(t)+AyEK(zi(t)), | (6) |
c2=1M∑Mi=1yE1(p1(t)). | (7) |
Repeat the steps above for each subsequent stage of the residual to finally obtain the m th signal residual, as shown in the following equation:
hm(t)=hm−1(t)−cm. | (8) |
Multiple EMD decompositions are performed on the signal until the signal residuals cannot be further decomposed to complete the CEEMDAN process, and finally, after M decompositions, all CEEMDAN decompositions of the signal are as follows:
y(t)=∑Mm=1IMFm+hM(t). | (9) |
The Grey Wolf Optimization algorithm (GWO) is based on the collaboration mechanism of grey wolves and simulates a wolf pack hunting to achieve the goal. Compared with other intelligent optimization algorithms, it has the characteristics of solid convergence, few parameters, easiness to implement, and can achieve the global optimal solution [37]. A certain number of grey wolves are divided into α,β,δ and ω according to their duties. α is the sole leader and is responsible for the group decision-making of the wolf pack; β is the auxiliary, assisting the leader in managing the wolf pack and also acting as the replacement of the leader; δ needs to follow the orders of α and β, and is responsible for scouting, hunting, and guarding, etc.; and ω is the lowest level of the wolf pack, which is responsible for following the orders of the superiors and maintaining the equilibrium of the pack races.
The steps of GWO include searching, tracking, encircling, hunting, and attacking. The principle of the algorithm is that, in the encirclement, each wolf represents a potential solution, and α,β,δ, ω gradually decreases according to their levels of priority. According to the positions of the first three wolves, the other individuals calculate the distances and constantly approach them to update the position to complete the search of optimality.
In the Q-dimensional space, a single wolf is denoted as Wi=(wi,1,wi,2,…,wi,q), and the constituent wolves are denoted as Wi=(W1,W2,…,WN). The calculation is shown in the following equations:
Dm=Ck⋅Wm−Wik(t),m=α,β,δ;i=1,2,3…,n, | (10) |
Ck=2r1,k=1,2,3, | (11) |
W'ik=Wm−Ak⋅Dm, | (12) |
Wi(t+1)=13∑W'ik, | (13) |
Ak=2ar2−a,k=1,2,3, | (14) |
where a=2−t/tmax, a: convergence factor, which decreases linearly with the number of iterations and takes values in the range [0, 2], Dm: distance of grey wolf Wi from wolves α,β,δ at iteration t, Ck: coefficient vector, Ak: coefficient vector, r1: random values of [0, 1], r2: random values of [0, 1], W'ik: vector of the grey wolf's movement as it approaches α,β,δ, Wi(t+1): move value of the grey wolf Wi for the next iteration.
Bidirectional Long Short-Term Memory (Bi-LSTM) is composed of forward LSTM and backward LSTM. Bi-LSTM is connected to one output for forward and backward propagation, respectively, but forward and backward propagations are not associated. The hidden layers of both propagations are independent of each other, and their confidential information layers can be propagated in both directions. The composition of Bi-LSTM makes itself more suitable for diagnosing early-stage weak faults of bearings by fully using the information of timing features [38].
The LSTM consists of a forget gate ft, an input gate it, a cell state ct and an output gate ot, and σ is an activation function-sigmoid. The composition of LSTM is shown in Figure 1.
Forward LSTM operates memory cells using input gates, output gates, and forget gates. In this computational process, the forget gate decides to remember or forget the hidden state of the previous layer according to the probability. The error term propagation of the backward LSTM is concentrated in the direction along the time axis and in the direction of the previous layer of the network.
During the actual training process of LSTM, each output is compared with the corresponding labels to update the weight parameters of the network. Each label is coded using one-hot coding, and its coded result needs to match the output of softmax, which takes the value range of [0, 1]. Using this function allows the LSTM to judge the probability of the labels. The value of the softmax function is calculated as shown in the following equation:
Si=eZi∑Jj=1eZj, | (15) |
where Zi: the i th element of the array Z, J: number of categories of the array Z, e: natural number.
In the softmax function, the cross entropy is used as the loss function in this paper, and its expression is shown in the following equation:
L=∑nk=1yilog(Si), | (16) |
where yi: category of the i th data, Si: probability of a category for the i th data, n: total number of categories.
CEEMDAN decomposes the vibration signal according to the frequency. However, there are pseudo-components in the decomposition process, so it is very critical to filter out the IMF that contains the wealthiest information about early-stage weak faults, which directly affects the establishment of the fault diagnosis model and ultimately affects the accuracy of weak fault diagnosis. The time-domain characteristics of the vibration signal are analyzed according to the time history of the signal to obtain its statistical characteristics. Its processing will not cause losses of signal or distortions, and it is not affected by the influence of the measurement points, which renders itself rather suitable for weak fault diagnosis.
We propose an adaptive extraction algorithm of weak fault features considering time-frequency modalities (AFETF) for envelope spectrum analysis. We select the kurtosis, mean square error, and sample entropy as the parameter indexes in the time domain dimension. Kurtosis is more sensitive to shock pulses and pulse-like fault signals, which makes itself applicable for surfacing damage faults and diagnosing early weak faults [39]. The mean square error can reflect the degree of data dispersion and is an essential indicator of accuracy. The early-stage weak faults will be enhanced when the faults appear and change the mean square deviation [40]. The sample entropy can reflect the complexity of the time series, and at the same time, it is less dependent on the data and it is high in consistency [41]. These three indexes are more sensitive to early weak faults.
Kurtosis is affected by the number and the size of signal x waveform shocks, and the formula for calculating the kurtosis value is shown below:
K=E(x−μ)2σ4, | (17) |
where E: expectation, μ: signal mean, σ: signal mean variance.
The formula for calculating the mean square error is shown in the following equation:
σ=√1N∑Ni=1(xi−μ)2, | (18) |
where N: number of sampling points.
The sample entropy equation is shown below:
SampEn(m,r,N)=−ln[Am(r)Bm(r)], | (19) |
where Bm(r): the probability of matching to m points under the distance restriction r, Am(r): the probability of matching to m+1 points.
The specific equation of the metric proposed in this paper used to quantify information of the early-stage weak faults contained in IMFs is shown as follow:
Xi=KiKmax+σiσmax+SiSmax, | (20) |
whereKi: the kurtosis of i th IMF, Kmax: the max kurtosis of IMFs, σi: the mean square error of i th IMF, σmax: the max mean square error of IMFs, Si: the sample entropy of i th IMF, Smax: the max sample entropy of IMFs.
Although time domain modality analysis is suitable for early weak fault diagnosis, its precision must be improved. Frequency domain modality analysis can make up well for this defect. In this paper, the theoretical characteristic frequency of each fault is calculated according to the bearing type, size parameter, and rotational speed. After CEEMDAN has been used to decompose the original signal into several IMFs, assuming that L IMFs are obtained, their envelope signals are obtained using the Hilbert variation. Then, the spectral transformation is performed to obtain the envelope spectrum of the IMF. Given the manufacture and assembly of bearings and the influence of the operating environment, there is often a particular gap between the actual fault characteristic frequency of bearings and the theoretical fault characteristic frequency, and the fluctuation range is usually within 5%. The number of fault characteristic frequencies and that of their multiplicative frequencies are obtained in the envelope spectrum. We define the fault characteristic frequency screening function, as shown in the following equation:
Fi=Numberm(Wi(f)),f∈[f'−γf',f'+γf'],i=1,2,…,L, | (21) |
where f': the theoretical characteristic frequency of fault, f: the range of characteristic frequency for screening, Wi(f): the envelope spectrum of the i th IMF component, γ: the screening factor within 5%, m: the number of selected spectral amplitudes, Number: the number of spectra in the first m frequencies with the highest spectral amplitude that match the screening range.
After obtaining Fi for each IMF, its percentage is calculated as shown in the following equation:
Yi=Fi∑Li=1Fi. | (22) |
The index of measuring fault information is obtained in the dimension of time domain, and the number of corresponding fault characteristic frequencies and their multiples are obtained in the dimension of frequency domain. Based on the time-frequency characteristics above, it defines Z as a parameter index which can achieve the most abundant information of early-stage weak faults, and its calculation formula is shown as the following equation:
Zi=max(Xi∗Yi),i=1,2,…,L. | (23) |
LSTM is improved by introducing a flexible and self-control self-loop design on the RNN [42]. LSTM can only propagate in one direction, and in the process of weak fault diagnosis. LSTM misses the part of the weak fault feature information because the weak fault itself is not apparent. Bi-LSTM can realize the bi-directional propagation to extract information of weak fault feature to the maximum extent to improve the accuracy of the model. In weak fault diagnosis, by using Bi-LSTM, the number of neurons in the hidden layer of the model, the initial learning rate, and the regularization coefficient need to be specified subjectively, which cannot guarantee the optimal solution, thus affecting the accuracy of the model [43]. Therefore, we propose a classification algorithm for GWO-BiLSTM, which uses GWO to optimize and adjust the hyperparameters of Bi-LSTM to obtain the optimal solution of the model parameters, thus improving the accuracy of the model.
To improve the accuracy of the Bi-LSTM model, this paper uses the GWO algorithm to optimize the hyperparameters of the Bi-LSTM, including the number of neurons in the hidden layer, the learning rate, and the regularization coefficient. The obtained results of model classification must be infinitely close to the actual results to get the minimum objective function. The fitness function is defined as shown in the following equation:
fitness=−∑SS=1(y'−y)2, | (24) |
wherey': the real class, y: the actual class, S: the length of training.
The flow of the optimized classification algorithm based on GWO-BiLSTM is shown in Figure 2.
First, the number of hidden layer neurons, the learning rate, and the regularization coefficient of the Bi-LSTM are initialized. The random distribution function is used to initialize the grey wolf position, decompose the parameters of the LSTM network, including the weights, etc., and substitute them into the network to iteratively calculate the fitness value until the end of the iteration. The best Wα is returned as the optimal solution. Inputting it into the Bi-LSTM network and inputting the dataset for training can generate the final classification result.
The process of early-stage weak fault diagnosis for bearings proposed in this paper is shown in Figure 3.
We begin by employing SVD to reduce noise in the original bearing signal. The order of the SVD is then determined using the difference spectrum. Next, CEEMDAN decomposes the signal into multiple IMFs. We utilize AFETF algorithm proposed in this paper to screen the IMF, which contains the richest weak fault information. Finally, GWO is employed to optimize the hyperparameters of Bi-LSTM and train the model for final diagnosis.
To verify the effectiveness of the proposed method, a simulation test of bearing fault is performed in the test rig shown in Figure 4. The test rig consists of an electric motor, an inertia wheel for applying radial load, a coupling, a conveyor belt drive mechanism, a conveyor belt, a crank-connecting rod mechanism, a gearbox with the bearing, and a reciprocating mechanism with a spring. The bearing selected for this test is a deep groove ball bearing (MB ER-10K), and the rotational frequency of the shaft is defined as fr. Two acceleration meters as shown in Figure 4, are PCB Model 608A11. The sensitivity of these acceleration meters is 10.2 (mV/ms-2) / 100 (mv/g). The acquisition card model is NI 9234. According to the formula for calculating the fault frequency of the bearing, the characteristic fault frequencies of the outer race, inner race and ball are fo = 3.052fr, fi = 4.948fr, and fb = 3.984fr, respectively.
Vibration tests were conducted on bearings featuring damage to the outer race, inner race, and steel ball components. Localized cracks with a width and a height of 0.2 and 0.4 mm were etched on the outer race grooves, inner race grooves, and steel ball surfaces, respectively. The sampling frequency fs of this test is 25.6 kHz and the motor speed is 900 r/min, corresponding to a rotational frequency fr of 15 Hz.
Table 1 displays the characteristic fault frequency of each component under real measurements.
Component | Outer race | Inner race | Ball |
Measured rotation frequency | 14.33 | 14.76 | 14.12 |
Characteristic fault frequency | 43.75 | 73.03 | 56.25 |
Taking the outer race weak fault with a 0.2 mm crack vibration signal as an example, its time domain and frequency domain are shown in Figure 5.
Figure 5(a) reveals prominent shock characteristics in the time-domain plot, while Figure 5(b) illustrates a frequency domain plot with a poorly smoothed signal waveform and numerous mutation points, indicating significant background noise. Extracting fault features in this scenario without noise reduction is challenging.
We initially employ SVD for noise reduction of fault signal data to eliminate background noises. However, selecting the appropriate order is crucial, as different singular value orders yield vastly different effects on noise reduction. The distribution of singular values is depicted in Figure 6, where the singular value gradually decreases with increasing order.
Figure 7 displays the singular value difference spectrum. At a singular value order of 8, the maximum difference value reaches 8.157, indicating a clearer distinction between the signal and noise. Hence, a singular value order of 8 is selected for noise reduction in this study.
Figure 8 illustrates the time domain comparison before and after noise reduction, indicating a reduced signal amplitude and a more defined boundary contour. Similarly, Figure 9 displays smoother frequency domains post-noise reduction, with fewer mutation points. Irrelevant noise bands are suppressed, preserving valuable signal bands and enhancing signal amplitude. SVD noise reduction better represents the characteristics of the original signal.
Following noise reduction, the signal undergoes CEEMDAN decomposition, yielding 12 IMFs. These decomposition results are illustrated in Figure 10.
CEEMDAN decomposes the signal into 12 IMFs based on frequency, with the final IMF representing the residual. Throughout the decomposition process, a reduction in the richness of information within each IMF becomes evident.
Figure 11 illustrates the sifting iterations for each IMF during CEEMDAN decomposition. The maximum number of sifting iterations for IMF1 is 159, indicating the rapid signal decomposition capability of CEEMDAN [44].
We propose a AFETF algorithm, which can help filter the IMF containing the richest fault information for diagnosis of early-stage weak faults.
Figure 12 presents the calculation results of mean square error, kurtosis, and sample entropy for each IMF in the time domain.
Figure 12 indicates that the first five IMFs contain the most significant amount of information. Setting L as 5, γ as 0.05, and m as 5, and according to Eq (21), the envelope spectrum of the first five IMFs are plotted so as to find the number of times the fault frequency that will be multiplied.
Figure 13 shows that among the eigenfrequencies of the first five highest amplitudes and their multiples, there are four for IMF1, two for IMF2, one for IMF3, two for IMF4, and one for IMF5.
The fault information contained in each IMF is calculated according to Eq (23) and the results are shown in Figure 14.
According to Figure 14, it can be learned that according to the adaptive feature extraction method proposed in this paper, IMF1 contains the most abundant fault information, so IMF1 is chosen as the feature of weak fault extracted from the outer race.
Initially, the weak fault with a 0.2 mm crack signal is sliced into segments of 0.1 seconds each. Using AFETF proposed in this paper, the optimal IMF is selected and labeled to create a dataset of 1140 × 2560. Subsequently, 30% of the data from each category is extracted as the test set. Next, the strong fault with a 0.4 mm crack signal is processed similarly to form the same 1140 × 2560 dataset.
As an example, for weak fault with a 0.2 mm crack, the IMFs selected for the three types of fault signals are shown in Figure 15.
From Figure 15, it can be seen that according to the AFETF algorithm of this paper, IMF1, IMF2, and IMF3 are the major features to be selected for faults from the outer race, IMF1 for faults from the inner race, and IMF1, IMF2, and IMF3 for the ball faults.
To assess the effectiveness of the proposed method, the weak fault with a 0.2 mm crack is taken as an example. First, noise reduction is performed using SVD, followed by signal decomposition with CEEMDAN. Subsequently, feature extraction is conducted using Minimum Entropy Deconvolution (MED), Maximum Correlated Kurtosis Deconvolution (MCKD), and AFETF proposed in this paper. Additionally, the original signal and the first IMF selected after signal decomposition are included as features for comprehensive comparison. These features are visualized in 2D using t-sne, as depicted in Figure 16.
According to Figure 16, it can be found that the three features extracted by the AFETF algorithm proposed in this paper have more distinct boundaries.
Taking weak fault with a 0.2 mm crack diagnosis as an example, after feature extraction using the different feature extraction algorithms mentioned above, the GWO-BiLSTM algorithm is used for classification, and the curve of its train accuracy is shown in Figure 17.
The GWO-BiLSTM classification results of confusion matrixes are shown in Figure 18.
The features are extracted using MED, MCKD, the first IMF, the original signal, and AFETF algorithm proposed in this paper, and then classified using CNN, Wide and Deep Convolutional Neural Network (WDCNN), Residual Neural Network (ResNet), Support Vector Machine (SVM), Random Forest (RF), and GWO-BiLSTM algorithms, respectively, adopted in this paper. The classification results for the weak fault with a 0.2 mm crack and for the strong fault with a 0.4 mm crack are shown in Figure 19.
The accuracy of strong fault with a 0.4 mm crack diagnosis is shown in Table 2.
Methods | MED | MCKD | First IMF | Original signal | AFETF |
CNN | 88.88 | 86.35 | 91.33 | 61.76 | 92.65 |
WDCNN | 92.36 | 88.74 | 86.63 | 74.52 | 94.26 |
ResNet | 93.12 | 90.32 | 83.75 | 74.26 | 95.98 |
SVM | 90.67 | 91.93 | 85.96 | 73.68 | 92.27 |
RF | 88.49 | 80.06 | 84.53 | 76.12 | 89.34 |
GWO-BiLSTM | 93.64 | 92.25 | 92.81 | 82.32 | 96.82 |
The diagnosis accuracy in the case of weak fault with a 0.2 mm crack is shown in Table 3.
Methods | MED | MCKD | First IMF | Original signal | AFETF |
CNN | 78.98 | 76.63 | 71.23 | 62.26 | 82.26 |
WDCNN | 82.43 | 83.74 | 79.63 | 70.25 | 89.03 |
ResNet | 83.36 | 70.12 | 76.25 | 68.36 | 85.94 |
SVM | 80.17 | 71.13 | 75.36 | 61.98 | 82.20 |
RF | 78.46 | 60.26 | 64.47 | 70.12 | 89.27 |
GWO-BiLSTM | 84.65 | 82.28 | 82.89 | 72.20 | 96.93 |
According to Figure 19, Tables 2 and 3, regarding feature extraction, the diagnosis algorithms are unified using GWO-BiLSTM. AFETF algorithm proposed in this paper improves the accuracy respectively by 3.18, 4.57, 4.01, and 14.5% in the diagnosis of strong fault with a 0.4 mm crack. In the diagnosis of weak fault with a 0.2 mm crack, the accuracy is respectively improved by 12.28, 14.65, 14.04, and 24.73%. Regarding diagnosis algorithms, approaches of feature extraction are unified using AFETF algorithm proposed in this paper. In the diagnosis of strong fault with a 0.4 mm crack, compared with other algorithms, the accuracy of GWO-BiLSTM proposed in this paper is respectively improved by 4.17, 2.56, 0.84, 4.55, and 7.48%. In the diagnosis of weak fault with a 0.2 mm crack, the accuracy is respectively improved by 14.67, 7.9, 10.99, 14.73, and 7.66%.
We present an adaptive feature extraction and diagnosis method for detecting early-stage weak faults in bearings. Following SVD noise reduction, CEEMDAN decomposes the signal into modal components. Subsequently, the proposed algorithm, AFETF extracts fault features rich in information. GWO-BiLSTM, adept at identifying timing features, conduct adaptive fault diagnosis. Experimental results demonstrate superior diagnostic accuracy for both 0.4 and 0.2 mm faults compared to traditional methods. While effective for both strong and weak faults, the method exhibits particularly significant accuracy improvement for weak faults. This study underscores the suitability of the proposed diagnosis method for detecting early-stage weak faults in bearings.
We focus on the early-stage weak fault diagnosis of bearings under constant speed conditions. Future work will extend this research to weak fault diagnosis under variable speed conditions.
The authors declare they have not used Artificial Intelligence (AI) tools in the creation of this article.
This research was funded by the National Natural Science Foundation of China under Grant 11372080.
The authors declare there is no conflict of interest.
[1] | Stohs SJ (1995) The role of free radicals in toxicity and disease. J Basic Clin Physiol Pharmacol 6: 205–228. |
[2] |
Florence TM (1995) The role of free radicals in disease. Aust N Z J Ophthalmol 23: 3–7. doi: 10.1111/j.1442-9071.1995.tb01638.x
![]() |
[3] | Harrison D, Griendling KK, Landmesser U, et al. (2003) Role of oxidative stress in atherosclerosis. Am J Cardiol 91: 7A–11A. |
[4] |
Rahman T, Hosen I, Islam MMT, et al. (2012) Oxidative stress and human health. Adv Biosci Biot 3: 997–1019. doi: 10.4236/abb.2012.327123
![]() |
[5] |
Ríos-Arrabal S, Artacho-Cordón F, León J, et al. (2013) Involvement of free radicals in breast cancer. Springerplus 2: 404. doi: 10.1186/2193-1801-2-404
![]() |
[6] |
Cadenas E, Sies H (1998) The lag phase. Free Radic Res 28: 601–609. doi: 10.3109/10715769809065816
![]() |
[7] |
Kovacic P, Pozos RS, Somanathan R, et al. (2005) Mechanism of mitochondrial uncouplers, inhibitors, and toxins: Focus on electron transfer, free radicals, and structure-activity relationships. Curr Med Chem 12: 2601–2623. doi: 10.2174/092986705774370646
![]() |
[8] |
Valko M, Izakovic M, Mazur M, et al. (2004) Role of oxygen radicals in DNA damage and cancer incidence. Mol Cell Biochem 266: 37–56. doi: 10.1023/B:MCBI.0000049134.69131.89
![]() |
[9] |
Phaniendra A, Jestadi DB, Periyasamy L (2015) Free radicals: properties, sources, targets, and their implication in various diseases. Ind J Clin Biochem 30: 11–26. doi: 10.1007/s12291-014-0446-0
![]() |
[10] |
Valko M, Morris H, Cronin MTD (2005) Metals, toxicity and oxidative stress. Curr Med Chem 12: 1161–1208. doi: 10.2174/0929867053764635
![]() |
[11] |
Poyton RO, Ball KA, Castello PR (2009) Mitochondrial generation of free radicals and hypoxic signaling. Trends Endocrin Met 20: 332–340. doi: 10.1016/j.tem.2009.04.001
![]() |
[12] |
Turrens JF (2003) Mitochondrial formation of reactive oxygen species. J Physiol 552: 335–344. doi: 10.1113/jphysiol.2003.049478
![]() |
[13] |
Sumbayev VV, Yasinska IM (2007) Mechanisms of hypoxic signal transduction regulated by reactive nitrogen species. Scand J Immunol 65: 399–406. doi: 10.1111/j.1365-3083.2007.01919.x
![]() |
[14] |
Reuter S, Gupta SC, Chaturvedi MM, et al. (2010) Oxidative stress, inflammation, and cancer: how are they linked? Free Radic Bio Med 49: 1603–1616. doi: 10.1016/j.freeradbiomed.2010.09.006
![]() |
[15] | Arulselvan P, Fard MT, Tan WS, et al. (2016) Role of Aantioxidants and natural products in inflammation. Oxid Med Cell Longev: 5276130. |
[16] |
Sun J, Trumpower BL (2003) Superoxide anion generation by the cytochrome bc1 complex. ArchBiochem Biophys 419: 198–206. doi: 10.1016/j.abb.2003.08.028
![]() |
[17] | Henson P, Larsen G, Henson J, et al. (1984) Resolution of pulmonary inflammation. Fed Proc 43: 2799–2806. |
[18] |
Schmid-Schonbein GW (2006) Analysis of inflammation. Annu Rev Biomed Eng 8: 93–151. doi: 10.1146/annurev.bioeng.8.061505.095708
![]() |
[19] |
Markiewski MM, Lambris JD (2007) The role of complement in inflammatory diseases from behind the scenes into the spotlight. AM J Pathol 171: 715–727. doi: 10.2353/ajpath.2007.070166
![]() |
[20] | Eaves-Pyles T, Allen CA, Taormina J, et al. (2008) Escherichia coli isolated from a Crohn's disease patient adheres, invades, and induces inflammatory responses in polarized intestinal epithelial cells. Int J MedMicrobiol 298: 397–409. |
[21] |
Ferguson LR (2010) Chronic inflammation and mutagenesis. Mutat Res 690: 3–11. doi: 10.1016/j.mrfmmm.2010.03.007
![]() |
[22] | Weber A, Boege Y, Reisinger F, et al. (2011) Chronic liver inflammation and hepatocellular carcinoma: persistence matters. Swiss Med Wkly 141: w13197. |
[23] |
Gomberg M (1900) An incidence of trivalent carbon trimethylphenyl. J Am Chem Soc 22: 757–771. doi: 10.1021/ja02049a006
![]() |
[24] |
Gerschman R, Gilbert DL, Nye SW, et al. (1954) Oxygen poisoning and x-irradiation-A mechanism in common. Science 119: 623–626. doi: 10.1126/science.119.3097.623
![]() |
[25] | Commoner B, Townsend J, Pake GE (1954) Free radicals in biological materials. Nature 174: 689–691. |
[26] | McCord JM, Fridovich I (1969) Superoxide dismutase an enzymatic function for erythrocuprein (chemocuprein). J Biol Chem 244: 6049–6055. |
[27] | Babior BM, Kipnes RS, Curnutte JT (1973) Biological defense mechanisms. The production by leukocytes of superoxide, a potential bactericidal agent. J Clin Invest 52: 741–744. |
[28] | Babior BM, Curnutte JT, Kipnes RS (1975) Biological defense mechanisms. Evidence for the participation of superoxide in bacterial killing by xanthine oxidase. J Lab Clin Med 85: 235–244. |
[29] | Hassett DJ, Britigan BE, Svendsen T, et al. (1987) Bacteria form intracellular free radicals in response to paraquat and streptonigrin. Demonstration of the potency of hydroxyl radical. J Biol Chem 262: 13404–13408. |
[30] | Halliwell B, Gutteridge JMC (1989) Free radicals in biology and medicine, Clarendon Press. |
[31] |
Kohen R, Nyska A (2002) Invited review oxidation of biological systems: oxidative stress phenomena, antioxidants, redox reactions, and methods for their quantification. Toxicol Pathol 30: 620. doi: 10.1080/01926230290166724
![]() |
[32] | Halliwell B (2001) Free Radicals and other reactive species in disease. |
[33] | Mugoni V, Santoro MM (2013) Manipulating redox signaling to block tumor angiogenesis, research directions in tumor angiogenesis, Chai JY, Editor. |
[34] |
Valko M, Leibfritz D, Moncol J, et al. (2007) Free radicals and antioxidants in normal physiological functions and human disease. Int J Biochem Cell Bio 39: 44–84. doi: 10.1016/j.biocel.2006.07.001
![]() |
[35] |
Miller DM, Buettner GR, Aust SD (1990) Transition metals as catalysts of "autoxidation" reactions. Free Radic Bio Med 8: 95–108. doi: 10.1016/0891-5849(90)90148-C
![]() |
[36] | Lushchak VI (2014) Classification of oxidative stress based on its intensity. Excli J 13: 922–937. |
[37] | Nita M, Grzybowski A (2016) The role of the reactive oxygen species and oxidative stress in the pathomechanism of the age-related ocular diseases and other pathologies of the anterior and posterior eye segments in adults. Oxid Med Cell Longev 1–23. |
[38] | Bagheri F, Khori V, Alizadeh AM, et al. (2016) Reactive oxygen species-mediated cardiac-reperfusion injury: Mechanisms and therapies. Life Sci 16: 30570–30577. |
[39] | Lobo V, Patil A, Phatak A, et al. (2010) Free radicals, antioxidants and functional foods: impact on human health, Pharmacogn Rev 4: 118–126. |
[40] |
Inoue M, Sato EF, Nishikawa M, et al. (2003) Mitochondrial generation of reactive oxygen species and its role in aerobic life. Curr Med Chem 10: 2495. doi: 10.2174/0929867033456477
![]() |
[41] | Tandon V, Gupta BM, Tandon R (2005) Free radicals/Reactive oxygen species. JK-Practioner 12: 143–148. |
[42] | Bandyopadhyay U, Das D, Banerjee RK (1999) Reactive oxygen species: Oxidative damage and pathogenesis. Curr Sci 77: 658–665. |
[43] | Ashok S, Jayashree G, Pankaja N (2012) Effect of free radicals & antioxidants on oxidative stress: a review. J D & A Sci 1: 63–66. |
[44] |
Dröse S, Brandt U (2012) Molecular mechanisms of superoxide production by the mitochondrial respiratory chain. Adv Exp Med Bio 748: 145–69. doi: 10.1007/978-1-4614-3573-0_6
![]() |
[45] | Slater TF (1985) Free radical mechanism in tissue injury. J Biochem 222: 1–15. |
[46] |
Valko M, Rhodes CJ, Moncol J, et al. (2006) Free radicals, metals and antioxidants in oxidative stress-induced cancer. Chem Biol Interact 160: 1–40. doi: 10.1016/j.cbi.2005.12.009
![]() |
[47] |
Sies H (1997) Oxidative stress: Oxidants and antioxidants. Exp Physiol 82: 291. doi: 10.1113/expphysiol.1997.sp004024
![]() |
[48] |
Simon HU, Haj-Yehia A, Levi-Schaffer F (2000) Role of reactive oxygen species (ROS) in the apoptosis induction. Apoptosis 5: 415. doi: 10.1023/A:1009616228304
![]() |
[49] |
Halliwell B, Murcia MA, Chirico S, et al. (1995) Free radicals and antioxidants in food and in vivo: what they do and how they work. Crit Rev Food Sci Nutr 35: 7–20. doi: 10.1080/10408399509527682
![]() |
[50] |
Young IS, Woodside JV (2001) Antioxidants in health and disease. J Clin Pathol 54: 176–186. doi: 10.1136/jcp.54.3.176
![]() |
[51] | Sisein EA (2014) Biochemistry of free radicals and antioxidants. Sch Acad J Biosci 2: 110–118. |
[52] |
Shetti N, Patil R (2011) Antioxidants: Its beneficial role against health damaging free radical. World J Sci Technol 1: 46–51. doi: 10.4236/wjnst.2011.12008
![]() |
[53] |
Katakwar P, Metgud R, Naik S, et al. (2016) Oxidative stress marker in oral cancer: a review. J Can Res Ther 12: 438–446. doi: 10.4103/0973-1482.151935
![]() |
[54] |
Hayyan M, Hashim MA, AlNashef IM (2016) Superoxide Ion: Generation and Chemical Implications. Chem Rev 116: 3029–3085. doi: 10.1021/acs.chemrev.5b00407
![]() |
[55] | Michelson AM, McCord JM, Fridovich I (1977) Superoxide and Superoxide Dismutases. London: Academic Press, 320. |
[56] |
Afanas'ev I (2015) Mechanisms of superoxide signaling in epigenetic processes: relation to aging and cancer, aging and disease. Aging Dis 6: 216–227. doi: 10.14336/AD.2014.0924
![]() |
[57] |
Ardan T, Kovaceva J, Cejková J (2004) Comparative histochemical and immunohistochemical study on xanthine oxidoreductase/xanthine oxidase in mammalian corneal epithelium. Acta Histochemica 106: 69–75. doi: 10.1016/j.acthis.2003.08.001
![]() |
[58] |
Muller FL, Lustgarten MS, Jang Y, et al. (2007) Trends in oxidative aging theories. Free Radic. Biol. Med 43: 477–503. doi: 10.1016/j.freeradbiomed.2007.03.034
![]() |
[59] |
Kontos HA, Wei EP, Ellis EF, et al. (1985) Appearance of superoxide anion radical in cerebral extracellular space during increased prostaglandin synthesis in cats. Circ Res 57: 142–151. doi: 10.1161/01.RES.57.1.142
![]() |
[60] |
McIntyre M, Bohr DF, Dominiczak AF (1999) Endothelial function in hypertension. Hypertension 34: 539–545. doi: 10.1161/01.HYP.34.4.539
![]() |
[61] | Roy P, Roy SK, Mitra A, et al. (1994) Superoxide generation by lipoxygenase in the presence of NADH and NADPH. Biochim. Biophys. Acta 1214: 171–179. |
[62] |
Montezano AC, Touyz RM (2014) Reactive oxygen species, vascular noxs, and hypertension: focus on translational and clinical research. Antioxid Redox Sig 20: 164–182. doi: 10.1089/ars.2013.5302
![]() |
[63] |
Marchi KC, Ceron CS, Muniz JJ (2016) NADPH oxidase plays a role on ethanol-Induced hypertension and Reactive Oxygen Species generation in the vasculature. Alcohol Alcoholism 51: 522–534. doi: 10.1093/alcalc/agw043
![]() |
[64] | Bielski BHJ, Cabelli DE (1996) Superoxide and hydroxyl radical chemistry in aqueous solution. A Oxy Chem: 66–104. |
[65] |
De Grey AD (2002) HO2*: the forgotten radical. DNA Cell Biol 21: 251-257. doi: 10.1089/104454902753759672
![]() |
[66] |
Cerruti PA (1985) Pro-oxidant states and tumor activation. Science 227: 375–381. doi: 10.1126/science.2981433
![]() |
[67] |
Kelley EE, Khoo NK, Hundley NJ et al. (2010) Hydrogen peroxide is the major oxidant product of xanthine oxidase. Free Radic Biol Med 48: 493–498. doi: 10.1016/j.freeradbiomed.2009.11.012
![]() |
[68] | Chance B, Sies H, Boveris A (1979) Hydroperoxide metabolism in mammalian organs. Physiol Rev 59: 527–605. |
[69] |
Halliwell B, Clement MV, Long LH (2000) Hydrogen peroxide in the human body. FEBS Lett 486: 10–3. doi: 10.1016/S0014-5793(00)02197-9
![]() |
[70] |
Fisher AE, Maxwell SC, Naughton DP (2004) Superoxide and hydrogen peroxide suppression by metal ions and their EDTA complexes. Biochem Biophys Res Commun. 316: 48-51. doi: 10.1016/j.bbrc.2004.02.013
![]() |
[71] |
Mates JM, Perez-Gomez C, Nunez de Castro I (1999) Antioxidant enzymes and human diseases. Clin Biochem 32: 595–603. doi: 10.1016/S0009-9120(99)00075-2
![]() |
[72] |
Chae HZ, Kang SW, Rhee SG (1999) Isoforms of mammalian peroxiredoxin that reduce peroxides in presence of thioredoxin. Meth Enzymol 300: 219–226. doi: 10.1016/S0076-6879(99)00128-7
![]() |
[73] | Halliwell B, Gutteridge JMC (1999) Free radicals in biology and medicine, 3 Eds., Oxford University Press, 1–936. |
[74] |
Dirmeier R, O'Brien K, Engle M, et al. (2004) Measurement of oxidative stress in cells exposed to hypoxia and other changes in oxygen concentration. Meth Enzymol 381: 589–603. doi: 10.1016/S0076-6879(04)81038-3
![]() |
[75] |
Stadtman ER (2004) Role of oxidant species in aging. Curr Med Chem 11: 1105–1112. doi: 10.2174/0929867043365341
![]() |
[76] |
Uchida K (2003) 4-Hydroxy-2-nonenal: a product and mediator of oxidative stress. Prog Lipid Res 42: 318–343. doi: 10.1016/S0163-7827(03)00014-6
![]() |
[77] | Maxwell SRJ, Lip GYH (1997) Free radicals and antioxidants in cardiovascular disease. Brit J Clin Pharmaco 44: 307–317. |
[78] | Menotti A, Kromhout D, Blackburn H, et al. (1999) Food intake patterns and 25 year mortality from coronary heart disease: cross-cultural correlations in the Seven Countries Study. Eur J Epidemiol 15: 507–515. |
[79] |
Lim SS, Vos T, Flaxman AD, et al. (2012) A comparative risk assessment of burden of disease and injury attributable to 67 risk factors and risk factor clusters in 21 regions, 1990-2010: a systematic analysis for the Global Burden of Disease Study 2010. Lancet 380: 2224–2260. doi: 10.1016/S0140-6736(12)61766-8
![]() |
[80] | Heron M (2013) Deaths: leading causes for 2010. Natl Vital Stat Rep 62: 1–96. |
[81] |
Kadhum M, Sweidan A, Jaffery AE, et al. (2015) A review of the health effects of smoking shisha. Clin Med 15: 263–266. doi: 10.7861/clinmedicine.15-3-263
![]() |
[82] |
Morris PB, Ference BA, Jahangir E, et al. (2015) Cardiovascular effects of exposure to cigarette smoke and electronic cigarettes: clinical perspectives from the prevention of cardiovascular disease section leadership council and early career councils of the American college of cardiology. J Am Col Cardiol 66: 1378–1391. doi: 10.1016/j.jacc.2015.07.037
![]() |
[83] | Jensen IJ, Mæhre HK (2016) Preclinical and clinical studies on antioxidative, antihypertensive and cardioprotective effect of marine proteins and peptides-a review. Mar Drugs14: 211. |
[84] | Reid MB (2001) Redox modulation of skeletal muscle contraction: what we know and what we don't. Brit J Radiol 90: 724–731. |
[85] |
Kukreja RC, Hess ML (1992) The oxygen free-radical system-From equations through membrane–protein interactions to cardiovascular injury and protection. Cardiovasc Res 26: 641–655. doi: 10.1093/cvr/26.7.641
![]() |
[86] | Panth N, Paudel KR, Parajuli K (2016) Reactive oxygen species: a key hallmark of cardiovascular disease. Adv Med: 1–12. |
[87] | Sverdlov AL, Elezaby A, Behring JB, et al. (2015) High fat, high sucrose diet causes cardiac mitochondrial dysfunction due in part to oxidative post-translational modification of mitochondrial complex II. J Mol Cell Cardiol 78: 165–173. |
[88] | Santos CX, Anilkumar N, Zhang M, et al. (2011) Redox signaling in cardiac myocytes. Free Radic Biol Med 50: 777–793. |
[89] | Ku HJ, Ahn Y, Lee JH (2015) IDH2 deficiency promotes mitochondrial dysfunction and cardiac hypertrophy in mice. Free Radic Biol Med 80: 84–92. |
[90] |
Sverdlov AL, Elezaby A, Qin F, et al. (2016) Mitochondrial reactive oxygen species mediate cardiac structural, functional, and mitochondrial consequences of diet‐induced metabolic heart disease. J Am Heart Assoc 5: e002555. doi: 10.1161/JAHA.115.002555
![]() |
[91] | Zhang YB, Meng YH, Chang S, et al. (2016) High fructose causes cardiac hypertrophy via mitochondrial signaling pathway. Am J Transl Res 8: 4869–4880. |
[92] | Mellor K, Ritchie RH, Meredith G, et al. (2010) High-fructose diet elevates myocardial superoxide generation in mice in the absence of cardiac hypertrophy. Nutrition 26: 842–848. |
[93] | Mellor K, Ritchie R, Morris M, et al. (2016) Elevated myocardial superoxide production precedes the cardiac hypertrophy response to a high fructose diet in mice. Am J Physiol Renal Physiol 310: 547–559. |
[94] | Pryor WA, Stone K (1993) Oxidants in cigarette smoke. Radicals, hydrogen peroxide, peroxynitrate, and peroxynitrite. Ann N Y Acad Sci 686: 12–27. |
[95] |
Barnoya J, Glantz SA (2005) Cardiovascular effects of secondhand smoke: nearly as large as smoking. Circulation 111: 2684–2698. doi: 10.1161/CIRCULATIONAHA.104.492215
![]() |
[96] | Jaffa MA, KobeissyF, Al Hariri M et al. (2012) Global renal gene expression profiling analysis in B2-kinin receptor null mice: impact of diabetes. PloS One7: e44714. |
[97] | Al Hariri M, Zibara K, Farhat W, et al. (2016) Cigarette smoking-induced cardiac hypertrophy, vascular inflammation and injury are attenuated by antioxidant supplementation in an animal model. Front Pharmacol 7: 397. |
[98] |
He F, Zuo L (2015) Redox roles of reactive oxygen species in cardiovascular diseases. Int J Mol Sci 16: 27770–27780. doi: 10.3390/ijms161126059
![]() |
[99] |
Maritim A, Dene BA, Sanders RA, et al. (2003) Effects of pycnogenol treatment on oxidative stress in streptozotocin-induced diabetic rats. J Biochem Mol Toxicol 17: 193–199. doi: 10.1002/jbt.10078
![]() |
[100] | Movahedian A, Zolfaghari B, Sajjadi SE, et al. (2010) Antihyperlipidemic effect of Peucedanum pastinacifolium extract in streptozotocin-induced diabetic rats. Clinics (Sao Paulo) 65: 629–933. |
[101] | Iravani S, Zolfaghari B (2011) Pharmaceutical and nutraceutical effects of Pinus pinaster bark extract. Res Pharm Sci 6: 1–11. |
[102] | Golbidi S, Badran M, Laher I (2012) Antioxidant and anti-inflammatory effects of exercise in diabetic patients. Exp Diabetes Res: 1–16. |
[103] | Schönlau F, Rohdewald P (2001) Pycnogenol for diabetic retinopathy. A review. Int Ophthalmol 24: 161–171. |
[104] | Voutilainen S, Nurmi T, Mursu J, et al. (2006) Carotenoids and cardiovascular health. Am J Clin Nutr 83: 1265–1271. |
[105] | Bashan N, Kovsan J, Kachko I (2009) Positive and negative regulation of insulin signaling by reactive oxygen and nitrogen species. Physiol Rev 89: 27–71. |
[106] | Kangralkar VA, Patil SD, Bandivadekar RM (2010) Oxidative stress and diabetes: a review. Int J Pharm Appl 1: 38–45. |
[107] |
Asmat U, Abad K, Ismail K (2016) Diabetes mellitus and oxidative stress-A concise review. Saudi Pharm J 24: 547–553. doi: 10.1016/j.jsps.2015.03.013
![]() |
[108] | Rolo AP, Palmeira CM (2006) Diabetes and mitochondrial function: Role of hyperglycemia and oxidative stress. Toxicol Appl Pharmacol 212: 167–178. |
[109] |
Haidara MA, Yassin HZ, Rateb M, et al. (2006) Role of oxidative stress in development of cardiovascular complications in diabetes mellitus. Curr Vasc Pharmacol 4: 215–227. doi: 10.2174/157016106777698469
![]() |
[110] | Bajaj S, Khan A (2012) Antioxidants and diabetes. Indian J Endocrinol Metab 16: S267–S271. |
[111] | Aghadavod E, Khodadadi S, Baradaran A, et al. (2016) role of oxidative stress and inflammatory factors in diabetic kidney disease. Iran J Kidney Dis 10: 337–343. |
[112] | Perez-Gutierrez RM, Garcia-Campoy AH, Muñiz-Ramirez A (2016) Properties of flavonoids isolated from the bark of Eysenhardtia polystachya and their effect on oxidative stress in streptozotocin-induced diabetes mellitus in mice. Oxid Med Cell Longev 9156510. |
[113] | Elgazzar MA (2007) Thymoquinone suppresses in vitro production of IL-5 and IL-13 by mast cells in response to lipopolysaccharide stimulation.Inflamm Res56:345–351. |
[114] | Golbidi S, Laher I (2010) Antioxidant therapy in human endocrine disorders.Med Sci Monitor16:9–24. |
[115] |
Blumberg RS, Strober W (2001) Prospects for research in inflammatory bowel disease. JAMA-J Am Med Assoc 285: 643–647. doi: 10.1001/jama.285.5.643
![]() |
[116] | Das R, Trafadar B, Das P, et al. (2015) Anti-inflammatory and regenerative potential of probiotics to combat inflammatory bowel disease (IBD). J Biotechnol Biomater 5: 181. |
[117] | Gross V, Arndt H, Andus T, et al. (1994) Free radicals in inflammatory bowel diseases pathophysiology and therapeutic implications. Hepatogastroenterology 41: 320–327. |
[118] | Fang J, Yin H, Liao L, et al. (2016) Water soluble PEG-conjugate of xanthine oxidase inhibitor, PEG-AHPP micelles, as a novel therapeutic for ROS related inflammatory bowel diseases. J Control Release 10: 188–196. |
[119] | Narushima S, Spitz DR, Oberley LW, et al. (2003) Evidence for oxidative stress in NSAID-induced colitis in IL10 mice. Free Radic Biol Med 34: 1153–1166. |
[120] |
Keshavarzian A, Banan A, Farhadi A, et al. (2003) Increases in free radicals and cytoskeletal protein oxidation and nitration in the colon of patients with inflammatory bowel disease. Gut 52: 720–728. doi: 10.1136/gut.52.5.720
![]() |
[121] | Patel MA, Patel PK, Patel MB (2010) Effects of ethanol extract of Ficus bengalensis (bark) on inflammatory bowel disease. Indian J Pharmacol 42: 214–218. |
[122] | Moret-Tatay I, Iborra M, Cerrillo E, et al. (2016) Possible biomarkers in blood for crohn's disease: oxidative stress and micrornas-current evidences and further aspects to unravel. Oxid Med Cell Longev 2325162. |
[123] |
Abraham C, Medzhitov R (2011) Interactions between the host innate immune system and microbes in inflammatory bowel disease. Gastroenterology 140: 1729–1737. doi: 10.1053/j.gastro.2011.02.012
![]() |
[124] |
Cross RK, Wilson KT (2003) Nitric oxide in inflammatory bowel disease. Inflamm Bowel Dis 9: 179–189. doi: 10.1097/00054725-200305000-00006
![]() |
[125] |
Kimura H, Hokari R, Miura S, et al. (1998) Increased expression of an inducible isoform of nitric oxide synthase and the formation of peroxynitrite in colonic mucosa of patients with active ulcerative colitis. Gut 42: 180–187. doi: 10.1136/gut.42.2.180
![]() |
[126] | Rachmilewitz D, Eliakim R, Ackerman Z, et al. (1998) Direct determination of colonic nitric oxide level-a sensitive marker of disease activity in ulcerative colitis. Am J Gastroenterol 93: 409–412. |
[127] |
Avdagić N, Zaćiragić A, Babić N, et al. (2013) Nitric oxide as a potential biomarker in inflammatory bowel disease. Bosn J Basic Med Sci 13: 5–9. doi: 10.17305/bjbms.2013.2402
![]() |
[128] |
Soufli I, Toumi R, Rafa H, et al. (2016) Overview of cytokines and nitric oxide involvement in immuno-pathogenesis of inflammatory bowel diseases. World J Gastrointest Pharmacol Ther 7: 353–360. doi: 10.4292/wjgpt.v7.i3.353
![]() |
[129] |
Fatani AJ, Alrojayee FS, Parmar MY, et al. (2016) Myrrh attenuates oxidative and inflammatory processes in acetic acid-induced ulcerative colitis. Exp Ther Med 12: 730–738. doi: 10.3892/etm.2016.3398
![]() |
[130] | Liu YW, Ong WK, Su YW, et al. (2016) Anti-inflammatory effects of Lactobacillus brevis K65 on RAW 264.7 cells and in mice with dextran sulphate sodium-induced ulcerative colitis. Benef Microbes 7: 387–396. |
[131] | Kottova M, Pourova J, Voprsalova M (2007) Oxidative stress and its role in respiratory diseases. Ceska Slov Farm 56: 215–219. |
[132] |
Zinellu A, Fois AG, Sotgia S, et al. (2016) Plasma protein thiols: an early marker of oxidative stress in asthma and chronic obstructive pulmonary disease. Eur J Clin Invest 46: 181–188. doi: 10.1111/eci.12582
![]() |
[133] |
Masoli M, Fabian D, Holt S, et al. (2004) The global burden of asthma: Executive summary of the GINA Dissemination Committee report. Allergy 59: 469–478. doi: 10.1111/j.1398-9995.2004.00526.x
![]() |
[134] |
Lemanske RF, Busse WW (2010) Asthma: Clinical Expression and Molecular Mechanisms. J Allergy Clin Immun 125: S95–S102. doi: 10.1016/j.jaci.2009.10.047
![]() |
[135] |
Chung KF (1986) Role of inflammation in the hyper reactivity of the airways in asthma. Thorax 41: 657–662. doi: 10.1136/thx.41.9.657
![]() |
[136] |
Barnes PJ (1990) Reactive oxygen species and airway inflammation. Free Rad Biol Med 9: 235–243. doi: 10.1016/0891-5849(90)90034-G
![]() |
[137] |
Doelman CJA, Bast A (1990) Oxygen radicals in lung pathology. Free Rad Biol Med 9: 381–400. doi: 10.1016/0891-5849(90)90015-B
![]() |
[138] |
Wood LG, Gibson LG, Garg ML (2003) Biomarkers of lipid peroxidation, airway inflammation and asthma. Eur Respir J 21: 177–186. doi: 10.1183/09031936.03.00017003a
![]() |
[139] |
Terada LS (2006) Specificity in reactive oxidant signaling: Think globally, act locally. J Cell Biol 174: 615–623. doi: 10.1083/jcb.200605036
![]() |
[140] |
Biagioli MC, Kaul P, Singh I, et al. (1999) The role of oxidative stress in rhinovirus induced elaboration of IL‐8 by respiratory epithelial cells. Free Rad Biol Med 26: 454–462. doi: 10.1016/S0891-5849(98)00233-0
![]() |
[141] |
Kanazawa H, Kurihara N, Hirata K, et al. (1991) The role of free radicals in airway obstruction in asthmatic patients. Chest 100: 1319–1322. doi: 10.1378/chest.100.5.1319
![]() |
[142] |
Shanmugasundaram KR, Kumar SS, Rajajee S (2001) Excessive free radical generation in the blood of children suffering from asthma. Clin Chim Acta 305: 107–114. doi: 10.1016/S0009-8981(00)00425-3
![]() |
[143] |
Rahman I, Biswas SK, Kode A (2006) Oxidant and antioxidant balance in the airways and airway diseases. Eur J Pharmacol 533: 222–239. doi: 10.1016/j.ejphar.2005.12.087
![]() |
[144] | Pobed'onna HP (2005) Antioxidant protection, metabolites of nitrogen oxide on the forming of oxidative stress in patients with bronchial asthma. Lik Sprava: 36–40. |
[145] | Rahman I, Morrison D, Donaldson K, et al. (1996) Systemic oxidative stress in asthma, COPD and smokers. Am J Respir Crit Care Med 154: 1055–1060. |
[146] |
Muti AD, Pârvu AE, Muti LA, et al. (2016) Vitamin E effect in a rat model of toluene diisocyanate-induced asthma. Clujul Mel 89: 499–505. doi: 10.15386/cjmed-611
![]() |
[147] |
Sahiner UM, Birben E, Erzurum S et al. (2011) Oxidative stress in asthma. World Allergy Organ J 4: 151–158. doi: 10.1097/WOX.0b013e318232389e
![]() |
[148] | Tohyama Y, Kanazawa H, Fujiwara F, et al. (2005) Role of nitric oxide on airway microvascular permeability in patients with asthma. Osaka City Med J 51: 1–9. |
[149] |
Wedes SH, Khatri SB, Zhang R, et al. (2009) Noninvasive markers of airway inflammation in asthma. Clin Transl Sci 2: 112–117. doi: 10.1111/j.1752-8062.2009.00095.x
![]() |
[150] | Sanders SP (1999) Nitric oxide in asthma. Pathogenic, therapeutic, or diagnostic? Am J Respir CellMol Biol 21: 147–149. |
[151] | Edwards CRW, Bouchier IAD, (1994) Davidson's Principles and Practice of Medicine. Chirchill Livingstone UK. 16 Eds. |
[152] |
Kundu S, Ghosh P, Datta S, et al. (2012) Oxidative stress as a potential biomarker for determining disease activity in patients with rheumatoid arthritis. Free Radic Res 46: 1482–1489. doi: 10.3109/10715762.2012.727991
![]() |
[153] |
Feely MG, Erickson A, O'Dell JR (2009) Therapeutic options for rheumatoid arthritis. Expert Opin Pharmacother 10: 2095. doi: 10.1517/14656560903071043
![]() |
[154] | Bala A, Haldar PK (2013) Free radical biology in cellular inflammation related to rheumatoid arthritis. OA Arthritis 1: 15. |
[155] |
Mason AD, McManus AT, Pruitt BA (1986) Association of burn mortality and bacteraemia: A 25-year review. Arch Surg 121: 1027–1031. doi: 10.1001/archsurg.1986.01400090057009
![]() |
[156] | Al-Jawad FH, Sahib AS, Al-Kaisy AA (2008) Role of antioxidants in the treatment of burn lesions. Ann Burns Fire Disasters 21: 186–191. |
[157] |
Ipaktchi K, Mattar A, Niederbichler AD, et al. (2006) Attenuating burn wound inflammatory signaling reduces systemic inflammation and acute lung injury. J Immunol. 177: 8065–8071. doi: 10.4049/jimmunol.177.11.8065
![]() |
[158] |
Sehirli O, Sener E, Sener G, et al. (2008) Ghrelin improves burn-induced multiple organ injury by depressing neutrophil infiltration and the release of pro-inflammatory cytokines. Peptides 29: 1231–1240. doi: 10.1016/j.peptides.2008.02.012
![]() |
[159] | Horton JW, White DJ (1995) Role of xanthine oxidase and leukocytes in post-burn cardiac dysfunction. J Am Coll Surg 181: 129–137. |
[160] |
Saitoh D, Okada Y, Ookawara T, et al. (1994) Prevention of ongoing lipid peroxidation by wound excision and superoxide dismutase treatment in the burned rat. Am J Emerg Med 12: 142–146. doi: 10.1016/0735-6757(94)90233-X
![]() |
[161] |
Piccolo MT, Wang Y, Till GO (1999) Chemotactic mediator requirements in lung injury following skin burns in rats. Exp Mol Pathol 66: 220–226. doi: 10.1006/exmp.1999.2263
![]() |
[162] |
Hosnuter M, Gurel A, Babuccu O, et al. (2004) The effect of CAPE on lipid peroxidation and nitric oxide levels in the plasma of rats following thermal injury. Burns 30: 121–125. doi: 10.1016/j.burns.2003.09.022
![]() |
[163] |
Singh V, Devgan L, Bhat S, et al. (2007) The pathogenesis of burn wound conversion. Ann Plast Surg 59: 109–115. doi: 10.1097/01.sap.0000252065.90759.e6
![]() |
[164] | Rani M, Martin G, Schwacha MG (2012) Aging and the pathogenic response to burn. Aging Dis 3: 23–25. |
[165] | Saez JC, Ward PH, Gunther B, et al. (1984) Superoxide radical involvement in the pathogenesis of burn shock. Circ Shock 12: 229–239. |
[166] |
Ward PA, Till GO, Hatherill JR, et al. (1985) Systemic complement activation, lung injury, and products of lipid peroxidation. J Clin Invest 76: 517–527. doi: 10.1172/JCI112001
![]() |
[167] | Oldham KT, Guice KS, Till GO, et al. (1998) Activation of complement by hydroxyl radical in thermal injury. Surgery 104: 272–279. |
[168] |
Parihar A, Parihar MS, Milner S, et al. (2008) Oxidative stress and anti-oxidative mobilization in burn injury. Burns 34: 6–17. doi: 10.1016/j.burns.2007.04.009
![]() |
[169] |
Nielson CB, Duethman NC, Howard JM, et al. (2017) Burns: pathophysiology of systemic complications and current management. J Burn Care Res 38: e469–e481. doi: 10.1097/BCR.0000000000000355
![]() |
[170] |
Burton LK, Velasco SE, Patt A, et al. (1995) Xanthine oxidase contributes to lung leak in rats subjected to skin burn. Inflammation 19: 31–38. doi: 10.1007/BF01534378
![]() |
[171] |
Trachootham D, Alexandre J, Huang P (2009) Targeting cancer cells by ROS-mediated mechanisms: a radical therapeutic approach? Nat Rev Drug Discov 8: 579–591. doi: 10.1038/nrd2803
![]() |
[172] | Pourahmad J, Salimi A, Seydi E (2016) Role of oxygen free radicals in cancer development and treatment, free radicals and diseases. |
[173] | Ojo OA, Ajiboye B, Fadaka A, et al. (2017) nrf2-keap1 activation, a promising strategy in the prevention of cancer. Free Radic Antioxid 7: 1–7. |
[174] |
Asano H, Horinouchi T, Mai Y, et al. (2012) Nicotine- and tar-free cigarette smoke induces cell damage through reactive oxygen species newly generated by PKC-dependent activation of NADPH oxidase. J Pharmacol Sci 118: 275–287. doi: 10.1254/jphs.11166FP
![]() |
[175] |
Rai S, Malik R, Misra D, et al. (2014) Future prospective and current status of antioxidants in premalignant and malignant lesions of oral cavity. Int J Nutr Pharmacol Neurol Dis 4: 198–202. doi: 10.4103/2231-0738.139399
![]() |
[176] | Weatherall D, Akinyanju O, Fucharoen S, et al. (2006) In disease control priorities in developing countries, In: Jamison DT, Breman JG, Measham AR, et al., Editors, Inherited Disorders of Hemoglobin. Washington: World Bank. |
[177] |
Nisbet RM, Polanco J, Ittner LM, et al. (2015) Tau aggregation and its interplay with amyloid-β. Acta Neuropathol 129: 207–220. doi: 10.1007/s00401-014-1371-2
![]() |
[178] | Bolós M, Perea R, Avila J (2017) Alzheimer's disease as an inflammatory disease. Bio Mol Concepts: 1–7. |
[179] |
Murphy MP (2009) How mitochondria produce reactive oxygen species. Biochem J 417: 1–13. doi: 10.1042/BJ20081386
![]() |
[180] |
Olajide OJ, Yawson EO, Gbadamosi IT, et al. (2017) Ascorbic acid ameliorates behavioural deficits and neuropathologicalalterations in rat model of Alzheimer's disease. Environ Toxicol Phar 50: 200–211. doi: 10.1016/j.etap.2017.02.010
![]() |
[181] |
Kovacic P, Jacintho JD (2001) Mechanisms of carcinogenesis: Focus on oxidative stress and electron transfer. Curr Med Chem 8: 773–796. doi: 10.2174/0929867013373084
![]() |
[182] |
Ridnour LA, Isenberg JS, Espey MG, et al. (2005) Nitric oxide regulates angiogenesis through a functional switch involving thrombospondin-1. Proc Natl Acad Sci USA 102: 13147–13152. doi: 10.1073/pnas.0502979102
![]() |
[183] |
Valko M, Morris H, Mazur M, et al. (2001) Oxygen free radical generating mechanisms in the colon: Do the semiquinones of Vitamin K play a role in the aetiology of colon cancer? Biochim Biophys Acta 1527: 161–166. doi: 10.1016/S0304-4165(01)00163-5
![]() |
[184] | Pham-Huy LA, He H, Pham-Huy C (2008) Free radicals, antioxidants in disease and health. Int J Biomed Sci 4: 89–96. |
[185] |
Cadenas E (1997) Basic mechanisms of antioxidant activity. Biofactors 6: 391–397. doi: 10.1002/biof.5520060404
![]() |
Component | Outer race | Inner race | Ball |
Measured rotation frequency | 14.33 | 14.76 | 14.12 |
Characteristic fault frequency | 43.75 | 73.03 | 56.25 |
Methods | MED | MCKD | First IMF | Original signal | AFETF |
CNN | 88.88 | 86.35 | 91.33 | 61.76 | 92.65 |
WDCNN | 92.36 | 88.74 | 86.63 | 74.52 | 94.26 |
ResNet | 93.12 | 90.32 | 83.75 | 74.26 | 95.98 |
SVM | 90.67 | 91.93 | 85.96 | 73.68 | 92.27 |
RF | 88.49 | 80.06 | 84.53 | 76.12 | 89.34 |
GWO-BiLSTM | 93.64 | 92.25 | 92.81 | 82.32 | 96.82 |
Methods | MED | MCKD | First IMF | Original signal | AFETF |
CNN | 78.98 | 76.63 | 71.23 | 62.26 | 82.26 |
WDCNN | 82.43 | 83.74 | 79.63 | 70.25 | 89.03 |
ResNet | 83.36 | 70.12 | 76.25 | 68.36 | 85.94 |
SVM | 80.17 | 71.13 | 75.36 | 61.98 | 82.20 |
RF | 78.46 | 60.26 | 64.47 | 70.12 | 89.27 |
GWO-BiLSTM | 84.65 | 82.28 | 82.89 | 72.20 | 96.93 |
Component | Outer race | Inner race | Ball |
Measured rotation frequency | 14.33 | 14.76 | 14.12 |
Characteristic fault frequency | 43.75 | 73.03 | 56.25 |
Methods | MED | MCKD | First IMF | Original signal | AFETF |
CNN | 88.88 | 86.35 | 91.33 | 61.76 | 92.65 |
WDCNN | 92.36 | 88.74 | 86.63 | 74.52 | 94.26 |
ResNet | 93.12 | 90.32 | 83.75 | 74.26 | 95.98 |
SVM | 90.67 | 91.93 | 85.96 | 73.68 | 92.27 |
RF | 88.49 | 80.06 | 84.53 | 76.12 | 89.34 |
GWO-BiLSTM | 93.64 | 92.25 | 92.81 | 82.32 | 96.82 |
Methods | MED | MCKD | First IMF | Original signal | AFETF |
CNN | 78.98 | 76.63 | 71.23 | 62.26 | 82.26 |
WDCNN | 82.43 | 83.74 | 79.63 | 70.25 | 89.03 |
ResNet | 83.36 | 70.12 | 76.25 | 68.36 | 85.94 |
SVM | 80.17 | 71.13 | 75.36 | 61.98 | 82.20 |
RF | 78.46 | 60.26 | 64.47 | 70.12 | 89.27 |
GWO-BiLSTM | 84.65 | 82.28 | 82.89 | 72.20 | 96.93 |