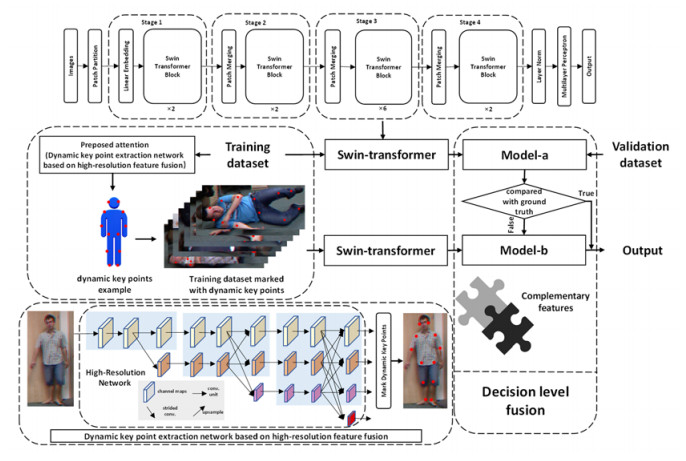
Citation: Samantha B. Gacasan, Daniel L. Baker, Abby L. Parrill. G protein-coupled receptors: the evolution of structural insight[J]. AIMS Biophysics, 2017, 4(3): 491-527. doi: 10.3934/biophy.2017.3.491
[1] | Jiaming Ding, Peigang Jiao, Kangning Li, Weibo Du . Road surface crack detection based on improved YOLOv5s. Mathematical Biosciences and Engineering, 2024, 21(3): 4269-4285. doi: 10.3934/mbe.2024188 |
[2] | Wenjie Liang . Research on a vehicle and pedestrian detection algorithm based on improved attention and feature fusion. Mathematical Biosciences and Engineering, 2024, 21(4): 5782-5802. doi: 10.3934/mbe.2024255 |
[3] | Chen Chen, Guowu Yuan, Hao Zhou, Yi Ma . Improved YOLOv5s model for key components detection of power transmission lines. Mathematical Biosciences and Engineering, 2023, 20(5): 7738-7760. doi: 10.3934/mbe.2023334 |
[4] | Zhijing Xu, Jingjing Su, Kan Huang . A-RetinaNet: A novel RetinaNet with an asymmetric attention fusion mechanism for dim and small drone detection in infrared images. Mathematical Biosciences and Engineering, 2023, 20(4): 6630-6651. doi: 10.3934/mbe.2023285 |
[5] | Cong Lin, Yiquan Huang, Wenling Wang, Siling Feng, Mengxing Huang . Lesion detection of chest X-Ray based on scalable attention residual CNN. Mathematical Biosciences and Engineering, 2023, 20(2): 1730-1749. doi: 10.3934/mbe.2023079 |
[6] | Yueming Huang, Guowu Yuan . AD-DETR: DETR with asymmetrical relation and decoupled attention in crowded scenes. Mathematical Biosciences and Engineering, 2023, 20(8): 14158-14179. doi: 10.3934/mbe.2023633 |
[7] | Shouming Zhang, Yaling Zhang, Yixiao Liao, Kunkun Pang, Zhiyong Wan, Songbin Zhou . Polyphonic sound event localization and detection based on Multiple Attention Fusion ResNet. Mathematical Biosciences and Engineering, 2024, 21(2): 2004-2023. doi: 10.3934/mbe.2024089 |
[8] | Kunpeng Li, Zepeng Wang, Yu Zhou, Sihai Li . Lung adenocarcinoma identification based on hybrid feature selections and attentional convolutional neural networks. Mathematical Biosciences and Engineering, 2024, 21(2): 2991-3015. doi: 10.3934/mbe.2024133 |
[9] | Xiaowen Jia, Jingxia Chen, Kexin Liu, Qian Wang, Jialing He . Multimodal depression detection based on an attention graph convolution and transformer. Mathematical Biosciences and Engineering, 2025, 22(3): 652-676. doi: 10.3934/mbe.2025024 |
[10] | Salamida Daudi, Livingstone Luboobi, Moatlhodi Kgosimore, Dmitry Kuznetsov . Dynamics for a non-autonomous fall armyworm-maize interaction model with a saturation functional response. Mathematical Biosciences and Engineering, 2022, 19(1): 146-168. doi: 10.3934/mbe.2022008 |
Falls, which are unexpected and uncontrolled changes in body posture, commonly occur among the elderly and are a major cause of health problems worldwide. They can lead to various degrees of physical and psychological damage and even death. According to the United Nations World Population Ageing 2020 report, the elderly population is expected to exceed 1.5 billion in 2050, and the percentage of people aged 65 and older is projected to increase from 9.3% in 2020 to approximately 16.0%. With the gradual aging of the global population, more and more elderly people will be exposed to the risk of accidental falls. Fall detection is essential to advanced first aid systems, providing timely assistance to improve the safety of elderly people living alone and avoid injuries caused by falls. The framework proposed by Chen et al. [1] improves the efficiency of first aid for older adults during falls, shortening resuscitation time and reducing the severity of injuries.
In recent years, researchers have conducted numerous studies in the field of fall detection. According to Mubashir [2], fall detection can be mainly divided into three approaches: wearable sensor-based, scene-based sensor-based, and computer vision-based. The wearable sensor-based approach relies on sensors worn by the person to detect falls, while the scene-based sensor-based approach uses sensors installed in the environment to detect falls. The computer vision-based approach uses cameras and computer algorithms to detect falls from video footage.
1) Fall detection using wearable devices is a promising approach, with single-sensor-based systems offering high accuracy and multiple-sensor-based systems offering higher efficiency [3]. Some recent studies have proposed innovative wearable fall detection systems. For example, de Sousa et al. [4] developed a low-power wearable system that integrates a fall detection system with an inflatable airbag to protect the hip. Lin et al. [5] used wireless in-shoe insoles equipped with sensors and a trained reference noise-canceling autoencoder to rapidly identify falls in all four directions. Bet et al. [6] described the latest technology and features for wearable sensors in detecting falls and found that the waist was the most common wearing position. Boudouane et al. [7] proposed a fall detection device using a portable camera worn on the hip, which effectively reduced specificity in fall detection. However, there are challenges to implementing wearable fall detection systems due to the lack of uniform standards for study populations and fall risk assessment, the high cost of sensors, and the cumbersome and forgetful nature of wearing devices for elderly individuals. Additionally, Casilari and Silva [8] found significant differences between simulated falls and real falls when using wearable sensors for fall detection, suggesting that further research is needed in this area.
2) Environmental sensor-based approaches rely on installed sensors that capture information such as sound and pressure to identify fall actions. For instance, Wang et al. [9] proposed a fall detection method that uses Wi-Fi signals based on the theory of indoor propagation of wireless signals, which has several advantages such as non-invasiveness and versatility. Other researchers, such as Madansingh et al. [10], have designed smartphone-based fall detection systems that utilize available embedded sensors such as accelerometer, gyroscope, and magnetometer. Wang et al. [11] proposed a radar-based fall detection method using pattern contour-confined Doppler-time maps that reduce superfluous information to improve detection accuracy. Chaccour et al. [12] summarized the deployment of sensors and proposed a generic fall detection classification scheme. However, such sensors are sensitive to environmental information, such as noise and light, which can result in false alarms and low detection rates.
3) Computer vision-based approaches detect falls by analyzing visual information extracted from images and videos. In contrast to machine vision, recent research by Gutiérrez [13] has reviewed 81 fall detection systems based on computer vision in the last five years, which have improved from simple characterization to sophisticated classification techniques. However, Gutiérrez also indicated that existing public datasets lack a common reference framework for system performance evaluation, which makes it difficult to compare the performance of different systems.
Compared to the first two approaches, computer vision-based systems offer several practical advantages, including lower equipment costs, greater convenience for the elderly, and richer semantic information contained in the video captured by the camera, which can be retrieved and reviewed at any time. These factors make computer vision-based systems more promising for future applications.
This paper introduces three types of methods for vision-based fall detection: threshold-based, machine-learning-based, and deep learning-based. The threshold analysis method uses real-time acceleration or mean square value data after secondary processing as feature values, comparing them with thresholds derived from numerous fall experiments. When the data exceeds or falls below the threshold, it is deemed abnormal, and this method is effective in detecting and identifying fall events from continuous data [14]. The threshold-based method is often used in combination with other methods to enhance performance [15].
Machine learning-based detection algorithms process and describe underlying visual data using manually designed feature operators such as HOG features [16], ORB features [17], and LBP features [18], and these algorithms incorporate classifiers with multiple functions, providing significant classification advantages. However, these algorithms rely excessively on the manual extraction of action features, and any bias in the feature samples significantly degrades the performance of fall detection. Furthermore, these feature operators have difficulty capturing semantic information in complex images, whereas deep learning-based detection algorithms can compensate for this drawback, with feature values automatically derived from sample data to create a learning strategy with strong feature representation capabilities [19]. Liu et al. [20] proposed an accelerometer signal enhancement model based on deep learning as a front-end processor to improve the detection performance of low-resolution fall detection systems. Yu et al. [21] compared the three types of methods: threshold-based, machine-learning-based, and deep learning-based, and the results showed that the deep learning-based methods outperformed the other two.
In summary, vision-based fall detection methods can be divided into threshold-based, machine-learning-based, and deep learning-based approaches. While the threshold-based method is useful for detecting and identifying fall events from continuous data, machine learning-based methods provide significant classification advantages but can be sensitive to biased feature samples. In contrast, deep learning-based methods automatically extract feature values from sample data, compensating for the shortcomings of manually designed feature operators, and have shown excellent performance in detecting falls.
With the rapid development of deep learning, attention mechanisms have become widely used in image detection. Drawing inspiration from human attention, experts and scholars have proposed attention mechanisms that efficiently allocate information processing resources. The structural model, which includes the attention mechanism, not only records the positional relationships between information but also measures the importance of different information features based on their weights. Lu et al. [22] proposed a graph neural network based on the attention mechanism that holistically solves tasks by formulating them as an iterative information fusion process on the data graph. In another study, Abdulwahab et al. [23] classified and analyzed existing feature selection methods and applied them to reduce dimensions when processing high-dimensional data. These methods help to overcome the inefficiencies and ineffectiveness of processing high-dimensional data.
With the advancements in image processing technology and the widespread application of machine learning algorithms, researchers worldwide have conducted numerous studies related to fall detection algorithms. Mrozek et al. [24] developed a new scalable architecture system for remote monitoring, using Random Forest (RF), SVM, Artificial Neural Network (ANN), and Boosted Decision Trees (BDT) classifiers. Cai et al. [25] proposed a vision-based fall detection method using multi-task hourglass convolutional self-coding. The method involved extracting multi-scale features by extending the perceptual field of neurons through hourglass convolutional layers and completing the detection of fall actions and frame reconstruction tasks in parallel using a multi-task mechanism. Vishnu et al. [26] introduced the fall motion mixture model, which effectively recognizes fall events in various scenarios.
Currently, the majority of fall detection algorithms only detect the original image or key points of the human body in the visual dimension, leaving room for further improvement in accuracy. Additionally, some key points of the human body may not be detectable due to environmental factors, and not all key points of the human body may be relevant for fall detection. To address these issues, this paper aims to make the following contributions.
1) A new set of dynamic key points based on high-frequency human key points are proposed for fall detection. These key points are identified during a fall state and automatically labeled to implement an attention capture mechanism using the original data-driven attention mechanism of the depth model. The attention is distinguished by the size of pixel values.
2) The paper also demonstrates that the depth model, which incorporates both human pose and key points, can yield strong complementarity in fall detection results. To this end, a fall detection model is proposed that incorporates dynamic key points and the preposed attention capture mechanism.
The main structure of this paper is as follows: Section 2 introduces the method utilized in this paper, Section 3 shows the experimental results and analysis, Section 4 contains the discussion and outlook, and finally concludes the work of this paper.
In this paper, we utilized Swin Transformer [27] to train the model, as it has demonstrated excellent performance in the field of image analysis. Unlike the original Transformer [28] that employs self-attention as a layer in the network structure and uses self-attention and feedforward networks for encoding and decoding, Swin Transformer introduces the hierarchical construction method typically used in CNNs [29] and conducts self-attention computation in the window region without overlap, which enhances efficiency and reduces computational complexity. The whole model comprises four stages, each of which reduces the resolution of the input feature map and expands the perception field layer by layer like CNN. Figure 1 depicts the system structure. Model-a is the deep learning model without the preposed attention, while Model-b is the deep learning model with the preposed attention, which is trained using labeled key points.
At present, most fall detection algorithms only rely on a single dimension of information, such as visual images or key points. To improve detection accuracy, it is necessary to expand the dimensionality of information properly. In this paper, we propose a preposed attention mechanism to enhance the images fed into the Swin Transformer training network. We achieve this by adding dynamic key points, visually stimulating elements that can attract more attention and cause unconscious attention shifting [30]. Our experiments explore whether there is a complementary fusion between the dynamic key points and the unmarked images, and the results will be presented in this paper.
For labeling the key points, we selected the High-Resolution Network (HRNet) [31], which is based on a multi-resolution feature fusion network. This network changes the link between high and low resolutions from series to parallel, maintains high-resolution representation throughout the network structure, and introduces interaction between high and low resolutions to improve model performance. Some commonly used key point detection datasets include the COCO dataset [32], which represents human key points as 17 joints with up to 300 K samples; the MPII Human Pose Dataset [33], which contains 25 K samples and defines 16 key point coordinates; and the Leeds Sports Pose Dataset contains 2 K samples, with 14 key points per sample [34]. The HRNet network follows the heat map estimation framework and applies it to human pose estimation, and has experimentally demonstrated superior pose estimation performance on the COCO key points detection dataset. Therefore, we trained our model on the COCO key points detection dataset, which can detect a total of 17 human key points.
We calculated the frequency of key points on the UR Fall Detection Dataset (URFD) [35], which includes 30 video segments captured during various daily activities. HRNet was used to label the fall state map for each segment. Due to the fall posture, not all key points may contribute to fall detection. Therefore, we counted the frequency of occurrence of each of the 17 key points in the fall state map and ranked them according to their frequency, as shown in Figure 2.
We selected the 12 points with frequencies higher than 75%: namely 3–8 and 11–16, and defined them as high-frequency key points. A comparison of 17 key points detected by the original HRNet network and the 12 key points is shown in Figure 3.
Fall detection can suffer from incomplete information due to environmental occlusion and obstacles, which often results in missing some of the 12 high-frequency key points identified in the URFD. To obtain as much accurate information as possible, we propose the use of dynamic key points, which correspond to the maximum possible detection of the 12 unobstructed high-frequency key points. The number of dynamic key points varies dynamically based on the detection of the obscured parts, up to a maximum of 12. We present the pseudo-code for dynamic key points extraction in Algorithm 1.
Algorithm 1. Marking dynamic key points with HRNet |
Input: Original images Load the trained 17 human key points models Adopt the top-down detection method, detect the human body first, then mark the key points 1 repeat Extract the features of key points in the human image Locate 17 key points // Use the extracted features to locate the 17 key points on the human body. 2 for i ∈ (3, 8) & (11, 16) do // Iterating on our selected high-frequency key points. 3 if len(pred_boxes) > = 1 then // Check if the model detected the body part corresponding to the key point from the picture 4 marked key points 5 end 6 until all 12 key points are detected // Repeat the process until all 12 key points are detected. Output: Dynamic key points picture marked |
By adding these dynamic key points, we aim to provide additional information that can aid in fall detection. Specifically, we add the dynamic key points to the image before it enters the Swin Transformer network, and we make them more visually stimulating, so they are likely to attract more attention than the unmarked images alone. The Swin Transformer network can then use this information to make more accurate predictions about the fall state.
Overall, the preposed attention mechanism with the dynamic key points aims to provide a complementary fusion between the dynamic key points and the unmarked images. This fusion enhances the images' quality and makes them more informative, leading to improved fall detection performance.
In this paper, we propose the use of dynamic key points for fall detection, which enhances the attentional capture of the model and improves detection accuracy despite incomplete information caused by environmental occlusion and obstacles. To achieve complementary effects, the results of two models are fused at the decision level. Model-a is used to validate the validation set and output error images separately. Then, the error images are validated using Model-b, to achieve decision fusion of the two models. The proposed approach is illustrated in Figure 4.
As shown in Figure 4, Model-a is trained on the data layer composed of original images through the Swin-transformer. Meanwhile, Model-b is trained on the data layer composed of images marked with dynamic key points through the same architecture. Compared with Model-a, the data layer of Model-b added the size and coordinate information of dynamic feature points. Due to being trained on different data, the two models have different feature layers, which allow them to capture different aspects of the data.
At the decision level, Model-a and Model-b are fused to achieve complementary effects. Due to the differences in the data layer, the feature layers of Model-a and Model-b contain different network structure features and weights, which results in different decisions being output for the same data to be verified. In our method, specifically, the error images that Model-a fails to detect are sent to Model-b for further detection, and the final result is based on the detection results of Model-b.
By combining the strengths of both models, our proposed approach achieves improved accuracy in fall detection, even in scenarios with incomplete information caused by environmental occlusion and obstacles.
In this paper, we define missing detection as the model fails to detect images with ground truth as fall, and the MDCR is expressed as (1):
(1) |
where FN represents the total number of false negatives in the dataset that were missed by Model-a. MVm, on the other hand, represents the number of false negatives that were corrected after being processed by Model-b.
Similarly, error detection is defined as the model fails to detect images with safe ground truth, and the EDCR is expressed as (2).
(2) |
where FP represents the total number of false positives in the dataset that were missed by Model-a. MVe, on the other hand, represents the number of false positive that were corrected after being processed by Model-b.
The results of EDCR and MDCR directly reflect the corrective effects of our proposed dynamic key points on the original image, further demonstrating the complementarity between Model-b and Model-a.
In order to compare the effectiveness of different fall detection methods from various aspects, we define the key terms related to fall detection by a confusion matrix as shown in Table 1.
Test results | Positive (factual falls) | Negative (factual safe) | Total |
Positive (Detecting falls) | True Positive (TP) | False Positive (FP) | TP + FP |
Negative (Detecting safe) | False Negative (FN) | True Negative (TN) | FN + TN |
Total | TP + FN | FP + TN | TP + FP + FN + TN |
Note: TP: Samples predicted by the model as fall and ground truth as fall. FN: Samples predicted by the model as safe but ground truth as fall. FP: Samples predicted by the model as fall but ground truth as safe. TN: Samples predicted by the model as fall and ground truth as safe. |
Sensitivity is defined as the proportion of TP to all factual positives of the participating tests. In this paper, sensitivity reflects the sensitivity of the applied method to falls, and experiments are conducted on two datasets.
The specificity of a fall detection method is defined as the proportion of TF to all actual negatives in the tested dataset. In this study, specificity reflects the accuracy of the proposed method for detecting falls safely.
To investigate the potential complementarity between Model-a and Model-b in fall detection, we conducted separate experiments on the Fall Detection Dataset (FDD) [36] and the UP-Fall Detection Dataset (UFDD) [37]. We evaluated the missed detection correction rate, false detection correction rate, and corrected accuracy. The Swin Transformer-based model was trained on the URFD, and the best model was tested on the FDD and UFDD datasets, with the detected erroneous images saved as output. We then labeled all key points and dynamic key points defined in the training set images of the model and categorized them into groups No.1–No.5, as shown in Figure 5, with all 17 key points labeled as an example.
To assess the effectiveness of dynamic key points in improving fall detection accuracy, we created four different training sets comprising different types of data: 1) all key points, 2) all key points and original images, 3) dynamic key points, and 4) dynamic key points and original images. We then trained models on each of these sets and used them to validate the output error images. This allowed us to determine the gain effect of dynamic key points and evaluate their impact on the accuracy of fall detection.
We conducted test experiments on four methods regarding the MDCR are tested. The test results on FDD are shown in Figure 6.
From Figure 6, it can be seen that the MDCR of the No.3 and No.4 key points groups is better than that of the other three groups. In terms of methods, the dynamic key points perform better.
In the field of fall detection, determining the accuracy of the result is important in addition to the final test result. We construct the same dataset for the experiments.
The average correction rate of key points group No.4 was found to be the highest among the four methods. Therefore, key points group No.4 was taken as an example to count the recognition measurement accuracy of the four different methods used to correct false negative (FN) samples. Figure 7 shows the accuracy distribution of the four methods. A–D represent the following respectively: All key points, All key points and original images, Dynamic key points, Dynamic key points and original images. The red line represents the median of the box, and we have labeled the median and interquartile range for each box.
From the box plot, it can be seen that the dynamic key points method has the highest overall distribution of data, as evidenced by both the interquartile range and the median.
The test results of the four methods on UFDD are shown in Figure 8.
From Figure 8, it can be seen that the average EDCR of the No.4 key points group is superior to the other four groups, and the dynamic key points method in No.4 achieves the highest accuracy.
We selected the best group of 5 key points based on the MDCR results, and then determined that the dynamic key points method in Group No.4 had the highest accuracy rate in correcting FN data. In the EDCR section, we found that the Dynamic key points method yielded the highest accuracy. Thus, we used this method as an example and assessed the recognition accuracy of corrected FP samples using five different key point groups. We also marked the median and interquartile range of the box plot in Figure 9. From the box plot, it can be seen that the No.4 key points group yielded the best results.
In this paper Accuracy is defined as the accuracy corrected by Model-b, expressed as (3):
(3) |
where A is All images of the test.
The experimental results are shown in Figures 10 and 11.
From Figures 10 and 11, it can be seen that among the four methods and 5 key point groups, the Dynamic key points method of the No.4 group has the highest accuracy.
The test results of sensitivity on FDD are shown in the Figure 12. From the figure it demonstrated that only the Dynamic key points method of groups No.3 and No.4 outperforms the correction effect of origin.
We evaluated the specificity of our method on both datasets, and the results for the UFDD are shown in Figure 13. From the figure, it can be observed that half of the methods outperform the baseline, and the Dynamic key points method using key points group No.4 achieves the highest specificity.
We compared the training and validation speed of adding preposed attention and not adding preposed attention in Tables 2 and 3. As can be seen from the tables, with preposed attention, the training and validation speed of the data only decreased slightly. Therefore, it can be concluded that our proposed preposed attention did not increase the computation burden.
Model | Average iterations per second (it/s) |
Model-a | 3.07 |
Model-b | 3.01 |
Model | Average iterations per second (it/s) |
Model-a | 8.77 |
Model-b | 8.61 |
Table 4 compares the accuracy of different algorithms on the dataset URFD, and it can be indicated that our method outperforms most algorithms in terms of detection accuracy, slightly lower than the hybrid algorithm [42,43] and Deep network [44] with higher detection cost and time.
Methods | Accuracy (%) |
CNN [38] | 96.43 |
1D CNN [38] | 92.72 |
OF CNN [40] | 83.02 |
OF CNN [41] | 91.40 |
multi-stream DNN [42] | 99.72 |
3D CNN, LSTM [43] | 99.27 |
Deep network [44] | 99.67 |
Our method | 99.20 |
In order to verify the applicability and robustness of the method in this paper, we did cross-library detection. The model is trained on URFD and detected on FDD and UFDD respectively, and the results are shown in Table 5. Based on the accuracy it can be indicated that the robustness of the method in this paper is strong.
Dataset | Accuracy (%) |
FDD | 97.27 |
UFDD | 99.68 |
After analyzing the experimental results, it has been demonstrated that each method has different accuracy improvement effects for different indexes. For instance, the Dynamic key points method of groups No.3 displays the most significant improvement for the correction of missed detections, but the correction effect for false detections is mediocre. On the other hand, the Dynamic key points method of groups No.4 achieves the best improvement in both datasets for the final accuracy index, which represents the comprehensive performance of the proposed method. These results further validate the effectiveness of the proposed method.
Fall detection algorithms play an important role in fall detection and fall prevention. Each fall detection algorithm is capable of achieving very high accuracy, and applying fall detection to real life is an urgent issue to be considered at present. Through the above experiments, it was shown that the performance of the Model-b, compared with the Model-a, did not improve significantly, and even decreased slightly. The Model-b trained with Dynamic key points groups No.4, which has the best results in the above experiments, was selected for comparison, as shown in Table 6.
Model | URFD | FDD | UFDD |
Model-a | 0.987 | 0.926 | 0.931 |
Model-b | 0.982 | 0.938 | 0.986 |
Although the improvement in accuracy with Model-b alone was small, further analysis revealed strong complementarity between Model-a and Model-b. To investigate this, we used the highest-accuracy Model-a trained on URFD in a 5-fold cross-validation approach (defined as K1–K5), where we labeled the validation set images of each fold, sequentially validated and outputted the images with detection errors. We then used Model-a and Model-b in turn to detect the output images. Black dots indicate the error detection result, red dots indicate the corrected error result, and more red dots represent stronger complementarity between the models. The results are shown in Figures 14 and 15.
As an example, we take K1 of Figure 14: in the K1 fold, the images incorrectly detected by the first CNN model are No.1, No.90, No.224, No.225, No.226, and No.227. The No.90 image is corrected after being detected by the Model-a with the next highest accuracy.
: error detection result,
: corrected error result.
The experiments described above have demonstrated the complementarity between the models trained using dynamic key points and original images. To explore whether training the models with dynamic key points first and then using original images for complementary correction would yield similar results, we conducted additional experiments. The results showed that original images do have a correction effect, and the final accuracy is similar. This provides further evidence for the complementarity between the two approaches. However, the correction effect is lower compared to the method proposed in this paper. The results of these additional experiments are summarized in Tables 7 and 8.
FDD | MDER | EDCR | Accuracy | sensitivity | specificity |
Exchange Order | 0.5238 | 0.5 | 0.97 | 0.971 | 0.9687 |
Our method | 0.9225 | 0.847 | 0.973 | 0.9571 | 0.9852 |
UFDD | MDER | EDCR | Accuracy | sensitivity | specificity |
Exchange Order | 0.7673 | 0.867 | 0.997 | 0.9964 | 0.9992 |
Our method | 0.9268 | 0.995 | 0.997 | 0.9848 | 0.9939 |
In the field of biomedical imaging, Optical Coherence Tomography (OCT) is a commonly used non-invasive detection technology. Among them, Swept-Source OCT (SS-OCT) system uses a rapidly scanning laser for imaging in the 1300 nm bio-imaging window [45]. Various automatic calibration schemes have been widely studied to ensure the high-precision imaging of the SS-OCT system. Ratheesh et al. [46] proposed an automatic calibration scheme based on spectral phase. Meleppat et al. [47] proposed an effective wavenumber linearization method that can help SS-OCT systems achieve more accurate imaging. At the same time, dynamic key points detection technology has become an important research direction for real-time monitoring of small structural changes in biological tissue.
In cutting-edge research in this field, Meleppat et al. [48] successfully achieved imaging of the retina and choroid in different types of vertebrate eyes through experiments. Murukeshan et al. [49] introduced a method for quantitative detection of biofilm thickness using an SS-OCT system.
Based on these cutting-edge studies, we can consider new application scenarios, such as whether marking key points at the sclera or iris of the pupil, and combining dynamic key points detection technology, can help the SS-OCT system detect eye or retina structural changes earlier or more quickly, thus achieving more accurate diagnosis and treatment of eye diseases. This is a new direction worth further research.
This paper presents a novel approach for fall detection using dynamic key points and a preposed attention mechanism that fuses this information with original human posture images. We propose a deep learning model that incorporates this attention mechanism, which improves the accuracy of fall detection when compared to models trained with original images and all key points. Our extensive experiments on multiple datasets demonstrate the complementarity of dynamic key points and original images at the decision level, resulting in improved detection accuracy. In the future, we plan to develop more attention capture methods to fuse complementary information from different modalities.
This work was supported in part by Scientific Research Plan of Beijing Education Commission (SZ202110005002), CN.
The authors declare there is no conflict of interest.
[1] |
Venter JC, Adams MD, Myers EW, et al. (2001) The sequence of the human genome. Science 291: 1304–1351. doi: 10.1126/science.1058040
![]() |
[2] |
Lander ES, Linton LM, Birren B, et al. (2001) Initial sequencing and analysis of the human genome. Nature 409: 860–921. doi: 10.1038/35057062
![]() |
[3] | Fredriksson R, Lagerstrom MC, Lundin LG, et al. (2003) The G-protein-coupled receptors in the human genome form five main families. Phylogenetic analysis, paralogon groups, and fingerprints. Mol Pharmacol 63: 1256–1272. |
[4] |
Pierce KL, Premont RT, Lefkowitz RJ (2002) Seven-transmembrane receptors. Nat Rev Mol Cell Biol 3: 639–650. doi: 10.1038/nrm908
![]() |
[5] |
Lagerstrom MC, Schioth HB (2008) Structural diversity of G protein-coupled receptors and significance for drug discovery. Nat Rev Drug Discov 7: 339–357. doi: 10.1038/nrd2518
![]() |
[6] |
Chien EY, Liu W, Zhao Q, et al. (2010) Structure of the human dopamine D3 receptor in complex with a D2/D3 selective antagonist. Science 330: 1091–1095. doi: 10.1126/science.1197410
![]() |
[7] |
Yang J, Villar VA, Armando I, et al. (2016) G protein-coupled receptor kinases: Crucial regulators of blood pressure. J Am Heart Assoc 5: e003519. doi: 10.1161/JAHA.116.003519
![]() |
[8] |
Bar-Shavit R, Maoz M, Kancharla A, et al. (2016) G protein-coupled receptors in cancer. Int J Mol Sci 17: 1320. doi: 10.3390/ijms17081320
![]() |
[9] |
Flower DR (1999) Modelling G-protein-coupled receptors for drug design. Biochim Biophys Acta 1422: 207–234. doi: 10.1016/S0304-4157(99)00006-4
![]() |
[10] |
Katritch V, Cherezov V, Stevens RC (2012) Diversity and modularity of G protein-coupled receptor structures. Trends Pharmacol Sci 33: 17–27. doi: 10.1016/j.tips.2011.09.003
![]() |
[11] | Gether U, Ballesteros JA, Seifert R, et al. (1997) Structural instability of a constitutively active G protein-coupled receptor. Agonist-independent activation due to conformational flexibility. J Biol Chem 272: 2587–2590. |
[12] |
Seifert R, Wenzel-Seifert K (2002) Constitutive activity of G-protein-coupled receptors: cause of disease and common property of wild-type receptors. Naunyn Schmiedebergs Arch Pharmacol 366: 381–416. doi: 10.1007/s00210-002-0588-0
![]() |
[13] |
Nelson G, Hoon MA, Chandrashekar J, et al. (2001) Mammalian sweet taste receptors. Cell 106: 381–390. doi: 10.1016/S0092-8674(01)00451-2
![]() |
[14] |
Nelson G, Chandrashekar J, Hoon MA, et al. (2002) An amino-acid taste receptor. Nature 416: 199–202. doi: 10.1038/nature726
![]() |
[15] | Milligan G (2004) G protein-coupled receptor dimerization: Function and ligand pharmacology. Mol Pharmacol 66. |
[16] |
Syrovatkina V, Alegre KO, Dey R, et al. (2016) Regulation, signaling, and physiological functions of G-proteins. J Mol Biol 428: 3850–3868. doi: 10.1016/j.jmb.2016.08.002
![]() |
[17] | Wess J (1997) G-protein-coupled receptors: molecular mechanisms involved in receptor activation and selectivity of G-protein recognition. FASEB J 11: 346–354. |
[18] |
Rasumussen SG, DeVree BT, Zou Y, et al. (2011) Crystal structure of the beta2 adrenergic receptor-Gs protein complex. Nature 477: 549–555. doi: 10.1038/nature10361
![]() |
[19] |
Gilman AG (1987) G proteins: transducers of receptor-generated signals. Annu Rev Biochem 56: 615–649. doi: 10.1146/annurev.bi.56.070187.003151
![]() |
[20] | Nie J, Lewis DL (2001) Structural domains of the CB1 cannabinoid receptor that contribute to constitutive activity and G-protein sequestration. J Neurosci 21: 8758–8764. |
[21] |
Kobilka BK, Deupi X (2007) Conformational complexity of G-protein-coupled receptors. Trends Pharmacol Sci 28: 397–406. doi: 10.1016/j.tips.2007.06.003
![]() |
[22] |
Ross EM, Wilkie TM (2000) GTPase-activating proteins for heterotrimeric G proteins: regulators of G protein signaling (RGS) and RGS-like proteins. Annu Rev Biochem 69: 795–827. doi: 10.1146/annurev.biochem.69.1.795
![]() |
[23] |
Claing A, Laporte SA, Caron MG, et al. (2002) Endocytosis of G protein-coupled receptors: roles of G protein-coupled receptor kinases and beta-arrestin proteins. Prog Neurobiol 66: 61–79. doi: 10.1016/S0301-0082(01)00023-5
![]() |
[24] |
Okada T, Le TI, Fox BA, et al. (2000) X-Ray diffraction analysis of three-dimensional crystals of bovine rhodopsin obtained from mixed micelles. J Struct Biol 130: 73–80. doi: 10.1006/jsbi.1999.4209
![]() |
[25] |
Palczewski K, Kumasaka T, Hori T, et al. (2000) Crystal structure of rhodopsin: A G protein-coupled receptor. Science 289: 739–745. doi: 10.1126/science.289.5480.739
![]() |
[26] |
Cherezov V, Rosenbaum DM, Hanson MA, et al. (2007) High resolution crystal structure of an engineered human beta2-adrenergic G protein-couple receptor. Science 318: 1258–1265. doi: 10.1126/science.1150577
![]() |
[27] |
Rasmussen SG, Choi HJ, Rosenbaum DM, et al. (2007) Crystal structure of the human beta2 adrenergic G-protein-coupled receptor. Nature 450: 383–387. doi: 10.1038/nature06325
![]() |
[28] | Stroud RM (2011) New tools in membrane protein determination. F1000 Biol Rep 3: 8. |
[29] |
Ujwal R, Bowie JU (2011) Crystallizing membrane proteins using lipidic bicelles. Methods 55: 337–341. doi: 10.1016/j.ymeth.2011.09.020
![]() |
[30] |
Denisov IG, Sligar SG (2016) Nanodiscs for structural and functional studies of membrane proteins. Nat Struct Mol Biol 23: 481–486. doi: 10.1038/nsmb.3195
![]() |
[31] |
Xiang J, Chun E, Liu C, et al. (2016) Successful strategies to determine high-resolution structures of GPCRs. Trends Pharmacol Sci 37: 1055–1069. doi: 10.1016/j.tips.2016.09.009
![]() |
[32] |
Rawlings AE (2016) Membrane proteins: always an insoluble problem? Biochem Soc Trans 44: 790–795. doi: 10.1042/BST20160025
![]() |
[33] |
Neubig RR, Siderovski DP (2002) Regulators of G-protein signalling as new central nervous system drug targets. Nat Rev Drug Discov 1: 187–197. doi: 10.1038/nrd747
![]() |
[34] |
Bayburt TH, Grinkova YV, Sligar SG (2002) Self-assembly of discoidal phospholipid bilayer nanoparticles with membrane scaffold proteins. Nano Lett 2: 853–856. doi: 10.1021/nl025623k
![]() |
[35] |
Delmar JA, Bolla JR, Su CC, et al. (2015) Crystallization of membrane proteins by vapor diffusion. Methods Enzymol 557: 363–392. doi: 10.1016/bs.mie.2014.12.018
![]() |
[36] | Jaakola VP, Griffith MT, Hanson MA, et al. (2008) The 2.6 angstrom crystal structure of a human A2A adenosine receptor bound to an antagonist. Science 322: 1211–1217. |
[37] |
Dore AS, Okrasa K, Patel JC, et al. (2014) Structure of class C GPCR metabotropic glutamate receptor 5 transmembrane domain. Nature 511: 557–562. doi: 10.1038/nature13396
![]() |
[38] |
Thompson AA, Liu W, Chun E, et al. (2012) Structure of the nociceptin/orphanin FQ receptor in complex with a peptide mimetic. Nature 485: 395–399. doi: 10.1038/nature11085
![]() |
[39] |
Wang C, Wu H, Katritch V, et al. (2013) Structure of the human smoothened receptor bound to an antitumour agent. Nature 497: 338–343. doi: 10.1038/nature12167
![]() |
[40] |
Yin J, Babaoglu K, Brautigam CA, et al. (2016) Structure and ligand-binding mechanism of the human OX1 and OX2 orexin receptors. Nat Struct Mol Biol 23: 293–299. doi: 10.1038/nsmb.3183
![]() |
[41] | Zhang K, Zhang J, Gao Z, et al. (2014) Structure of the human P2Y12 receptor in complex with an antithrombotic drug. Nature 509. |
[42] | Isberg V, Mordalski S, Munk C, et al. (2016) GPCRdb: an information system for G protein-coupled receptors. Nucleic Acids Res. |
[43] |
Berman HM, Westbrook J, Feng Z, et al. (2000) The protein data bank. Nucl Acids Res 28: 235–242. doi: 10.1093/nar/28.1.235
![]() |
[44] |
Teller DC, Okada T, Behnke CA, et al. (2001) Advances in determination of a high-resolution three-dimensional structure of rhodopsin, a model of G-protein-coupled receptors (GPCRs). Biochemistry 40: 7761–7772. doi: 10.1021/bi0155091
![]() |
[45] |
Yeagle PL, Choi G, Albert AD (2001) Studies on the structure of the G-protein-coupled receptor rhodopsin including the putative G-protein binding site in unactivated and activated forms. Biochemistry 40: 11932–11937. doi: 10.1021/bi015543f
![]() |
[46] |
Okada T, Fujiyoshi Y, Silow M, et al. (2002) Functional role of internal water molecules in rhodopsin revealed by X-ray crystallography. Proc Natl Acad Sci USA 99: 5982–5987. doi: 10.1073/pnas.082666399
![]() |
[47] |
Choi G, Landin J, Galan JF, et al. (2002) Structural studies of metarhodopsin II, the activated form of the G-protein coupled receptor, rhodopsin. Biochemistry 41: 7318–7324. doi: 10.1021/bi025507w
![]() |
[48] |
Li J, Edwards PC, Burghammer M, et al. (2004) Structure of bovine rhodopsin in a trigonal crystal form. J Mol Biol 343: 1409–1438. doi: 10.1016/j.jmb.2004.08.090
![]() |
[49] |
Okada T, Sugihara M, Bondar AN, et al. (2004) The retinal conformation and its environment in rhodopsin in light of a new 22 A crystal structure. J Mol Biol 342: 571–583. doi: 10.1016/j.jmb.2004.07.044
![]() |
[50] |
Nakamichi H, Okada T (2006) Crystallographic analysis of primary visual photochemistry. Angew Chem Int Ed Engl 45: 4270–4273. doi: 10.1002/anie.200600595
![]() |
[51] |
Nakamichi H, Okada T (2006) Local peptide movement in the photoreaction intermediate of rhodopsin. Proc Natl Acad Sci USA 103: 12729–12734. doi: 10.1073/pnas.0601765103
![]() |
[52] |
Salom D, Lodowski DT, Stenkamp RE, et al. (2006) Crystal structure of a photoactivated deprotonated intermediate of rhodopsin. Proc Natl Acad Sci USA 103: 16123–16128. doi: 10.1073/pnas.0608022103
![]() |
[53] |
Standfuss J, Xie G, Edwards PC, et al. (2007) Crystal structure of a thermally stable rhodopsin mutant. J Mol Biol 372: 1179–1188. doi: 10.1016/j.jmb.2007.03.007
![]() |
[54] |
Nakamichi H, Buss V, Okada T (2007) Photoisomerization mechanism of rhodopsin and 9-cis-rhodopsin revealed by x-ray crystallography. Biophys J 92: L106–L108. doi: 10.1529/biophysj.107.108225
![]() |
[55] | Stenkamp RE (2008) Alternative models for two crystal structures of bovine rhodopsin. Acta Crystallogr D D64: 902–904. |
[56] |
Park JH, Scheerer P, Hofmann KP, et al. (2008) Crystal structure of the ligand-free G-protein-coupled receptor opsin. Nature 454: 183–187. doi: 10.1038/nature07063
![]() |
[57] |
Scheerer P, Park JH, Hildebrand PW, et al. (2008) Crystal structure of opsin in its G-protein-interacting conformation. Nature 455: 497–502. doi: 10.1038/nature07330
![]() |
[58] |
Standfuss J, Edwards PC, D'Antona A, et al. (2011) The structural basis of agonist-induced activation in constitutively active rhodopsin. Nature 471: 656–660. doi: 10.1038/nature09795
![]() |
[59] |
Makino CL, Riley CK, Looney J, et al. (2010) Binding of more than one retinoid to visual opsins. Biophys J 99: 2366–2373. doi: 10.1016/j.bpj.2010.08.003
![]() |
[60] |
Choe HW, Kim YJ, Park JH, et al. (2011) Crystal structure of metarhodopsin II. Nature 471: 651–655. doi: 10.1038/nature09789
![]() |
[61] |
Deupi X, Edwards P, Singhal A, et al. (2012) Stabilized G protein binding site in the structure of constitutively active metarhodopsin-II. Proc Natl Acad Sci USA 109: 119–124. doi: 10.1073/pnas.1114089108
![]() |
[62] |
Park JH, Morizumi T, Li Y, et al. (2013) Opsin, a structural model for olfactory receptors? Angew Chem Int Ed Engl 52: 11021–11024. doi: 10.1002/anie.201302374
![]() |
[63] |
Singhal A, Ostermaier MK, Vishnivetskiy SA, et al. (2013) Insights into congenital stationary night blindness based on the structure of G90D rhodopsin. EMBO Rep 14: 520–526. doi: 10.1038/embor.2013.44
![]() |
[64] |
Szczepek M, Beyriere F, Hofmann KP, et al. (2014) Crystal structure of a common GPCR-binding interface for G protein and arrestin. Nat Commun 5: 4801. doi: 10.1038/ncomms5801
![]() |
[65] |
Blankenship E, Vahedi-Faridi A, Lodowski DT (2015) The high-resolution structure of activated opsin reveals a conserved solvent network in the transmembrane region essential for activation. Structure 23: 2358–2364. doi: 10.1016/j.str.2015.09.015
![]() |
[66] |
Kang Y, Zhou XE, Gao X, et al. (2015) Crystal structure of rhodopsin bound to arrestin by femtosecond X-ray laser. Nature 523: 561–567. doi: 10.1038/nature14656
![]() |
[67] |
Singhal A, Guo Y, Matkovic M, et al. (2016) Structural role of the T94I rhodopsin mutation in congenital stationary night blindness. EMBO Rep 17: 1431–1440. doi: 10.15252/embr.201642671
![]() |
[68] |
Gulati S, Jastrzebska B, Banerjee S, et al. (2017) Photocyclic behavior of rhodopsin induced by an atypical isomerization mechanism. Proc Natl Acad Sci USA 114: E2608–E2615. doi: 10.1073/pnas.1617446114
![]() |
[69] |
Warne T, Serrano-Vega MJ, Baker JG, et al. (2008) Structure of a beta1-adrenergic G-protein-coupled receptor. Nature 454: 486–491. doi: 10.1038/nature07101
![]() |
[70] |
Warne T, Moukhametzianov R, Baker JG, et al. (2011) The structural basis for agonist and partial agonist action on a beta(1)-adrenergic receptor. Nature 469: 241–244. doi: 10.1038/nature09746
![]() |
[71] |
Moukhametzianov R, Warne T, Edwards PC, et al. (2011) Two distinct conformations of helix 6 observed in antagonist-bound structures of a beta-1 adrenergic receptor. Proc Natl Acad Sci USA 108: 8228–8232. doi: 10.1073/pnas.1100185108
![]() |
[72] |
Christopher JA, Brown J, Dore AS, et al. (2013) Biophysical fragment screening of the beta1-adrenergic receptor: identification of high affinity arylpiperazine leads using structure-based drug design. J Med Chem 56: 3446–3455. doi: 10.1021/jm400140q
![]() |
[73] |
Warne T, Edwards PC, Leslie AG, et al. (2012) Crystal structures of a stabilized beta1-adrenoceptor bound to the biased agonists bucindolol and carvedilol. Structure 20: 841–849. doi: 10.1016/j.str.2012.03.014
![]() |
[74] | Miller-Gallacher JL, Nehme R, Warne T, et al. (2014) The 2.1 A resolution structure of cyanopindolol-bound beta1-adrenoceptor identifies an intramembrane Na+ ion that stabilises the ligand-free receptor. PLoS One 9: e92727. |
[75] |
Huang J, Chen S, Zhang JJ, et al. (2013) Crystal structure of oligomeric beta1-adrenergic G protein-coupled receptors in ligand-free basal state. Nat Struct Mol Biol 20: 419–425. doi: 10.1038/nsmb.2504
![]() |
[76] |
Sato T, Baker J, Warne T, et al. (2015) Pharmacological analysis and structure determination of 7-Methylcyanopindolol-bound beta1-adrenergic receptor. Mol Pharmacol 88: 1024–1034. doi: 10.1124/mol.115.101030
![]() |
[77] |
Leslie AG, Warne T, Tate CG (2015) Ligand occupancy in crystal structure of beta1-adrenergic G protein-coupled receptor. Nat Struct Mol Biol 22: 941–942. doi: 10.1038/nsmb.3130
![]() |
[78] | Hanson MA, Cherezov V, Griffith MT, et al. (2008) A specific cholesterol binding site is established by the 2.8 A structure of the human beta2-adrenergic receptor. Structure 6: 897–905. |
[79] |
Bokoch MP, Zou Y, Rasmussen SG, et al. (2010) Ligand-specific regulation of the extracellular surface of a G-protein-coupled receptor. Nature 463: 108–112. doi: 10.1038/nature08650
![]() |
[80] |
Wacker D, Fenalti G, Brown MA, et al. (2010) Conserved binding mode of human beta2 adrenergic receptor inverse agonists and antagonist revealed by X-ray crystallography. J Am Chem Soc 132: 11443–11445. doi: 10.1021/ja105108q
![]() |
[81] |
Rasmussen SG, Choi HJ, Fung JJ, et al. (2011) Structure of a nanobody-stabilized active state of the beta(2) adrenoceptor. Nature 469: 175–180. doi: 10.1038/nature09648
![]() |
[82] |
Rosenbaum DM, Zhang C, Lyons JA, et al. (2011) Structure and function of an irreversible agonist-beta(2) adrenoceptor complex. Nature 469: 236–240. doi: 10.1038/nature09665
![]() |
[83] | Zou Y, Weis WI, Kobilka BK (2012) N-terminal T4 lysozyme fusion facilitates crystallization of a G protein coupled receptor. PLos One 7. |
[84] |
Ring AM, Manglik A, Kruse AC, et al. (2013) Adrenaline-activated structure of beta2-adrenoreceptor stabilized by an engineered nanobody. Nature 502: 575–579. doi: 10.1038/nature12572
![]() |
[85] |
Weichert D, Kruse AC, Manglik A, et al. (2014) Covalent agonists for studying G protein-coupled receptor activation. Proc Natl Acad Sci USA 111: 10744–10748. doi: 10.1073/pnas.1410415111
![]() |
[86] |
Huang CY, Olieric V, Ma P, et al. (2016) In meso in situ serial X-ray crystallography of soluble and membrane proteins at cryogenic temperatures. Acta Crystallogr D 72: 93–112. doi: 10.1107/S2059798315021683
![]() |
[87] |
Staus DP, Strachan RT, Manglik A, et al. (2016) Allosteric nanobodies reveal the dynamic range and diverse mechanisms of G-protein-coupled receptor activation. Nature 535: 448–452. doi: 10.1038/nature18636
![]() |
[88] |
Shimamura T, Shiroishi M, Weyand S, et al. (2011) Structure of the human histamine H1 receptor complex with doxepin. Nature 475: 65–70. doi: 10.1038/nature10236
![]() |
[89] |
Wang C, Jiang Y, Ma J, et al. (2013) Structural basis for molecular recognition at serotonin receptors. Science 340: 610–614. doi: 10.1126/science.1232807
![]() |
[90] |
Wacker D, Wang C, Katritch V, et al. (2013) Structural features for functional selectivity at serotonin receptors. Science 340: 615–619. doi: 10.1126/science.1232808
![]() |
[91] |
Liu W, Wacker D, Gati C, et al. (2013) Serial femtosecond crystallography of G protein-coupled receptors. Science 342: 1521–1524. doi: 10.1126/science.1244142
![]() |
[92] |
Wacker D, Wang S, McCorvy JD, et al. (2017) Crystal structure of an LSD-bound human serotonin receptor. Cell 168: 377–389. doi: 10.1016/j.cell.2016.12.033
![]() |
[93] |
Thal DM, Sun B, Feng D, et al. (2016) Crystal structures of the M1 and M4 muscarinic acetylcholine receptors. Nature 531: 335–340. doi: 10.1038/nature17188
![]() |
[94] |
Haga K, Kruse AC, Asada H, et al. (2012) Structure of the human M2 muscarinic acetylcholine receptor bound to an antagonist. Nature 482: 547–551. doi: 10.1038/nature10753
![]() |
[95] |
Kruse AC, Ring AM, Manglik A, et al. (2013) Activation and allosteric modulation of a muscarinic acetylcholine receptor. Nature 504: 101–106. doi: 10.1038/nature12735
![]() |
[96] |
Kruse AC, Hu J, Pan AC, et al. (2012) Structure and dynamics of the M3 muscarinic acetylcholine receptor. Nature 482: 552–556. doi: 10.1038/nature10867
![]() |
[97] |
Thorsen TS, Matt R, Weis WI, et al. (2014) Modified T4 lysozyme fusion proteins facilitate G protein-coupled receptor crystallogenesis. Structure 22: 1657–1664. doi: 10.1016/j.str.2014.08.022
![]() |
[98] |
Glukhova A, Thal DM, Nguyen AT, et al. (2017) Structure of the adenosine A1 receptor reveals the basis for subtype selectivity. Cell 168: 867–877. doi: 10.1016/j.cell.2017.01.042
![]() |
[99] |
Dore AS, Robertson N, Errey JC, et al. (2011) Structure of the adenosine A(2A) receptor in complex with ZM241385 and the xanthines XAC and caffeine. Structure 19: 1283–1293. doi: 10.1016/j.str.2011.06.014
![]() |
[100] |
Xu F, Wu H, Katritch V, et al. (2011) Structure of an agonist-bound human A2A adenosine receptor. Science 332: 322–327. doi: 10.1126/science.1202793
![]() |
[101] |
Lebon G, Warne T, Edwards PC, et al. (2011) Agonist-bound adenosine A2A receptor structures reveal common features of GPCR activation. Nature 474: 521–525. doi: 10.1038/nature10136
![]() |
[102] | Hino T, Arakawa T, Iwanari H, et al. (2012) G-protein-coupled receptor inactivation by an allosteric inverse-agonist antibody. Nature 482: 237–240. |
[103] |
Congreve M, Andrews SP, Dore AS, et al. (2012) Discovery of 1,2,4-triazine derivatives as adenosine A(2A) antagonists using structure based drug design. J Med Chem 55: 1898–1903. doi: 10.1021/jm201376w
![]() |
[104] |
Liu W, Chun E, Thompson AA, et al. (2012) Structural basis for allosteric regulation of GPCRs by sodium ions. Science 337: 232–236. doi: 10.1126/science.1219218
![]() |
[105] |
Lebon G, Edwards PC, Leslie AG, et al. (2015) Molecular determinants of CGS21680 binding to the human adenosine A2A receptor. Mol Pharmacol 87: 907–915. doi: 10.1124/mol.114.097360
![]() |
[106] |
Segala E, Guo D, Cheng RK, et al. (2016) Controlling the dissociation of ligands from the adenosine A2A receptor through modulation of salt bridge strength. J Med Chem 59: 6470–6479. doi: 10.1021/acs.jmedchem.6b00653
![]() |
[107] |
Batyuk A, Galli L, Ishchenko A, et al. (2016) Native phasing of x-ray free-electron laser data for a G protein-coupled receptor. Sci Adv 2: e1600292. doi: 10.1126/sciadv.1600292
![]() |
[108] |
Carpenter B, Nehme R, Warne T, et al. (2016) Structure of the adenosine A(2A) receptor bound to an engineered G protein. Nature 536: 104–107. doi: 10.1038/nature18966
![]() |
[109] |
Sun B, Bachhawat P, Chu ML, et al. (2017) Crystal structure of the adenosine A2A receptor bound to an antagonist reveals a potential allosteric pocket. Proc Natl Acad Sci USA 114: 2066–2071. doi: 10.1073/pnas.1621423114
![]() |
[110] |
Hanson MA, Roth CB, Jo E, et al. (2012) Crystal structure of a lipid G protein-coupled receptor. Science 335: 851–855. doi: 10.1126/science.1215904
![]() |
[111] |
Chrencik JE, Roth CB, Terakado M, et al. (2015) Crystal structure of antagonist bound human lysophosphatidic acid receptor 1. Cell 161: 1633–1643. doi: 10.1016/j.cell.2015.06.002
![]() |
[112] |
Hua T, Vemuri K, Pu M, et al. (2016) Crystal structure of the human cannabinoid receptor CB1. Cell 167: 750–762. doi: 10.1016/j.cell.2016.10.004
![]() |
[113] | Shao Z, Yin J, Chapman K, et al. (2016) High-resolution crystal structure of the human CB1 cannabinoid receptor. Nature. |
[114] |
White JF, Noinaj N, Shibata Y, et al. (2012) Structure of the agonist-bound neurotensin receptor. Nature 490: 508–513. doi: 10.1038/nature11558
![]() |
[115] |
Egloff P, Hillenbrand M, Klenk C, et al. (2014) Structure of signaling-competent neurotensin receptor 1 obtained by directed evolution in Escherichia coli. Proc Natl Acad Sci USA 111: E655–E662. doi: 10.1073/pnas.1317903111
![]() |
[116] |
Krumm BE, White JF, Shah P, et al. (2015) Structural prerequisites for G-protein activation by the neurotensin receptor. Nat Commun 6: 7895. doi: 10.1038/ncomms8895
![]() |
[117] |
Krumm BE, Lee S, Bhattacharya S, et al. (2016) Structure and dynamics of a constitutively active neurotensin receptor. Sci Rep 6: 38564. doi: 10.1038/srep38564
![]() |
[118] | Yin J, Mobarec JC, Kolb P, et al. (2015) Crystal structure of the human OX2 orexin receptor bound to the insomnia drug suvorexant. Nature 519: 247–250. |
[119] |
Gayen A, Goswami SK, Mukhopadhyay C (2011) NMR evidence of GM1-induced conformational change of Substance P using isotropic bicelles. Biochim Biophys Acta 1808: 127–139. doi: 10.1016/j.bbamem.2010.09.023
![]() |
[120] |
Shihoya W, Nishizawa T, Okuta A, et al. (2016) Activation mechanism of endothelin ETB receptor by endothelin-1. Nature 537: 363–368. doi: 10.1038/nature19319
![]() |
[121] | Park SH, Das BB, Casagrande F, et al. (2012) Structure of the chemokine receptor CXCR1 in phospholipid bilayers. Nature 491: 779–783. |
[122] |
Zheng Y, Qin L, Zacarias NV, et al. (2016) Structure of CC chemokine receptor 2 with orthosteric and allosteric antagonists. Nature 540: 458–461. doi: 10.1038/nature20605
![]() |
[123] |
Wu B, Chien EY, Mol CD, et al. (2010) Structures of the CXCR4 chemokine GPCR with small-molecule and cyclic peptide antagonists. Science 330: 1066–1071. doi: 10.1126/science.1194396
![]() |
[124] | Qin L, Kufareva I, Holden LG, et al. (2015) Structural biology. Crystal structure of the chemokine receptor CXCR4 in complex with a viral chemokine. Science 347: 1117–1122. |
[125] |
Tan Q, Zhu Y, Li J, et al. (2013) Structure of the CCR5 chemokine receptor-HIV entry inhibitor maraviroc complex. Science 341: 1387–1390. doi: 10.1126/science.1241475
![]() |
[126] |
Oswald C, Rappas M, Kean J, et al. (2016) Intracellular allosteric antagonism of the CCR9 receptor. Nature 540: 462–465. doi: 10.1038/nature20606
![]() |
[127] |
Miller RL, Thompson AA, Trapella C, et al. (2015) The importance of ligand-receptor conformational pairs in stabilization: Spotlight on the N/OFQ G protein-coupled receptor. Structure 23: 2291–2299. doi: 10.1016/j.str.2015.07.024
![]() |
[128] |
Wu H, Wacker D, Mileni M, et al. (2012) Structure of the human kappa-opioid receptor in complex with JDTic. Nature 485: 327–332. doi: 10.1038/nature10939
![]() |
[129] |
Manglik A, Kruse AC, Kobilka TS, et al. (2012) Crystal structure of the mu-opioid receptor bound to a morphinan antagonist. Nature 485: 321–326. doi: 10.1038/nature10954
![]() |
[130] |
Huang W, Manglik A, Venkatakrishnan AJ, et al. (2015) Structural insights into micro-opioid receptor activation. Nature 524: 315–321. doi: 10.1038/nature14886
![]() |
[131] |
Granier S, Manglik A, Kruse AC, et al. (2012) Structure of the delta-opioid receptor bound to naltrindole. Nature 485: 400–404. doi: 10.1038/nature11111
![]() |
[132] |
Fenalti G, Giguere PM, Katritch V, et al. (2014) Molecular control of delta-opioid receptor signaling. Nature 506: 191–196. doi: 10.1038/nature12944
![]() |
[133] |
Fenalti G, Zatsepin NA, Betti C, et al. (2015) Structural basis for bifunctional peptide recognition at human delta-opioid receptor. Nat Struct Mol Biol 22: 265–268. doi: 10.1038/nsmb.2965
![]() |
[134] |
Zhang H, Unal H, Gati C, et al. (2015) Structure of the Angiotensin receptor revealed by serial femtosecond crystallography. Cell 161: 833–844. doi: 10.1016/j.cell.2015.04.011
![]() |
[135] |
Zhang H, Unal H, Desnoyer R, et al. (2015) Structural basis for ligand recognition and functional selectivity at angiotensin receptor. J Biol Chem 290: 29127–29139. doi: 10.1074/jbc.M115.689000
![]() |
[136] | Zhang H, Han GW, Batyuk A, et al. (2017) Structural basis for selectivity and diversity in angiotensin II receptors. Nature. |
[137] | Burg JS, Ingram JR, Venkatakrishnan AJ, et al. (2015) Structural biology. Structural basis for chemokine recognition and activation of a viral G protein-coupled receptor. Science 347: 1113–1117. |
[138] |
Zhang D, Gao ZG, Zhang K, et al. (2015) Two disparate ligand-binding sites in the human P2Y1 receptor. Nature 520: 317–321. doi: 10.1038/nature14287
![]() |
[139] |
Zhang J, Zhang K, Gao ZG, et al. (2014) Agonist-bound structure of the human P2Y12 receptor. Nature 509: 119–122. doi: 10.1038/nature13288
![]() |
[140] |
Zhang C, Srinivasan Y, Arlow DH, et al. (2012) High-resolution crystal structure of human protease-activated receptor 1. Nature 492: 387–392. doi: 10.1038/nature11701
![]() |
[141] |
Srivastava A, Yano J, Hirozane Y, et al. (2014) High-resolution structure of the human GPR40 receptor bound to allosteric agonist TAK-875. Nature 513: 124–127. doi: 10.1038/nature13494
![]() |
[142] |
Siu FY, He M, de Graaf C, et al. (2013) Structure of the human glucagon class B G-protein-coupled receptor. Nature 499: 444–449. doi: 10.1038/nature12393
![]() |
[143] |
Jazayeri A, Dore AS, Lamb D, et al. (2016) Extra-helical binding site of a glucagon receptor antagonist. Nature 533: 274–277. doi: 10.1038/nature17414
![]() |
[144] |
Hollenstein K, Kean J, Bortolato A, et al. (2013) Structure of class B GPCR corticotropin-releasing factor receptor 1. Nature 499: 438–443. doi: 10.1038/nature12357
![]() |
[145] | Dore AS, Bortolato A, Hollenstein K, et al. (2017) Decoding corticotropin-releasing factor receptor type 1 crystal structures. Curr Mol Pharmacol: Epub ahead of print, DOI: 10.2174/1874467210666170110114727. |
[146] |
Wu H, Wang C, Gregory KJ, et al. (2014) Structure of a class C GPCR metabotropic glutamate receptor 1 bound to an allosteric modulator. Science 344: 58–64. doi: 10.1126/science.1249489
![]() |
[147] |
Christopher JA, Aves SJ, Bennett KA, et al. (2015) Fragment and structure-based drug discovery for a class C GPCR: Discovery of the mGlu5 negative allosteric modulator HTL14242 (3-Chloro-5-[6-(5-fluoropyridin-2-yl)pyrimidin-4-yl]benzonitrile). J Med Chem 58: 6653–6664. doi: 10.1021/acs.jmedchem.5b00892
![]() |
[148] | Wang C, Wu H, Evron T, et al. (2014) Structural basis for Smoothened receptor modulation and chemoresistance to anticancer drugs. Nat Commun 5: 4355. |
[149] |
Byrne EF, Sircar R, Miller PS, et al. (2016) Structural basis of Smoothened regulation by its extracellular domains. Nature 535: 517–522. doi: 10.1038/nature18934
![]() |
[150] |
Lundstrom K (2006) Latest development in drug discovery on G protein-coupled receptors. Curr Protein Pept Sci 7: 465–470. doi: 10.2174/138920306778559403
![]() |
[151] |
Shonberg J, Kling RC, Gmeiner P, et al. (2015) GPCR crystal structures: Medicinal chemistry in the pocket. Bioorg Med Chem 23: 3880–3906. doi: 10.1016/j.bmc.2014.12.034
![]() |
[152] |
Attwood TK, Findlay JB (1993) Design of a discriminating fingerprint for G-protein-coupled receptors. Protein Eng 6: 167–176. doi: 10.1093/protein/6.2.167
![]() |
[153] |
Chee MS, Satchwell SC, Preddie E, et al. (1990) Human cytomegalovirus encodes three G protein-coupled receptor homologues. Nature 344: 774–777. doi: 10.1038/344774a0
![]() |
[154] |
Attwood TK, Findlay JB (1994) Fingerprinting G-protein-coupled receptors. Protein Eng 7: 195–203. doi: 10.1093/protein/7.2.195
![]() |
[155] | Strader CD, Sigal IS, Dixon RA (1989) Structural basis of beta-adrenergic receptor function. FASEB J 3: 1825–1832. |
[156] | Liapakis G, Ballesteros JA, Papachristou S, et al. (2000) The forgotten serine. A critical role for Ser-2035.42 in ligand binding to and activation of the beta 2-adrenergic receptor. J Biol Chem 275: 37779–37788. |
[157] | Swaminath G, Xiang Y, Lee TW, et al. (2004) Sequential binding of agonists to the beta2 adrenoceptor. Kinetic evidence for intermediate conformational states. J Biol Chem 279: 686–691. |
[158] |
Baldwin JM (1994) Structure and function of receptors coupled to G proteins. Curr Opin Cell Biol 6: 180–190. doi: 10.1016/0955-0674(94)90134-1
![]() |
[159] |
Tyndall JD, Sandilya R (2005) GPCR agonists and antagonists in the clinic. Med Chem 1: 405–421. doi: 10.2174/1573406054368675
![]() |
[160] | Jacoby E, Bouhelal R, Gerspacher M, et al. (2006) The 7 TM G-protein-coupled receptor target family. Chem Med Chem 1: 761–782. |
[161] | Spiss CK, Maze M (1985) Adrenoreceptors. Anaesthesist 34: 1–10. |
[162] |
Civelli O, Reinscheid RK, Zhang Y, et al. (2013) G protein-coupled receptor deorphanizations. Annu Rev Pharmacol Toxicol 53: 127–146. doi: 10.1146/annurev-pharmtox-010611-134548
![]() |
[163] |
Barst RJ, Langleben D, Frost A, et al. (2004) Sitaxsentan therapy for pulmonary arterial hypertension. Am J Respir Crit Care Med 169: 441–447. doi: 10.1164/rccm.200307-957OC
![]() |
[164] |
Kotake T, Usami M, Akaza H, et al. (1999) Goserelin acetate with or without antiandrogen or estrogen in the treatment of patients with advanced prostate cancer: a multicenter, randomized, controlled trial in Japan. Zoladex Study Group. Jpn J Clin Oncol 29: 562–570. doi: 10.1093/jjco/29.11.562
![]() |
[165] |
Onuffer JJ, Horuk R (2002) Chemokines, chemokine receptors and small-molecule antagonists: recent developments. Trends Pharmacol Sci 23: 459–467. doi: 10.1016/S0165-6147(02)02064-3
![]() |
[166] |
Fatkenheuer G, Pozniak AL, Johnson MA, et al. (2005) Efficacy of short-term monotherapy with maraviroc, a new CCR5 antagonist, in patients infected with HIV-1. Nat Med 11: 1170–1172. doi: 10.1038/nm1319
![]() |
[167] |
Lu M, Wu B (2016) Structural studies of G protein-coupled receptors. IUBMB Life 68: 894–903. doi: 10.1002/iub.1578
![]() |
[168] |
Strotmann R, Schrock K, Boselt I, et al. (2011) Evolution of GPCR: change and continuity. Mol Cell Endocrinol 331: 170–178. doi: 10.1016/j.mce.2010.07.012
![]() |
[169] |
Ballesteros JA, Weinstein H (1995) Integrated methods for the construction of three-dimensional models and computational probing of structure-function relations in G protein-coupled receptors. Method Neurosci 25: 366–428. doi: 10.1016/S1043-9471(05)80049-7
![]() |
[170] |
Zhang D, Weinstein H (1994) Polarity conserved positions in transmembrane domains of G-protein coupled receptors and bacteriorhodopsin. FEBS Lett 337: 207–212. doi: 10.1016/0014-5793(94)80274-2
![]() |
[171] |
Katritch V, Fenalti G, Abola EE, et al. (2014) Allosteric sodium in class A GPCR signaling. Trends Biochem Sci 39: 233–244. doi: 10.1016/j.tibs.2014.03.002
![]() |
[172] |
Bjarnadottir TK, Geirardsdottir K, Ingemansson M, et al. (2007) Identification of novel splice variants of Adhesion G protein-coupled receptors. Gene 387: 38–48. doi: 10.1016/j.gene.2006.07.039
![]() |
[173] |
Lin HH, Chang GW, Davies JQ, et al. (2004) Autocatalytic cleavage of the EMR2 receptor occurs at a conserved G protein-coupled receptor proteolytic site motif. J Biol Chem 279: 31823–31832. doi: 10.1074/jbc.M402974200
![]() |
[174] |
Isberg V, Vroling B, Van DKR, et al. (2014) GPCRDB: an information system for G protein-coupled receptors. Nucleic Acids Res 42: D422–D425. doi: 10.1093/nar/gkt1255
![]() |
[175] |
Gasparini F, Kuhn R, Pin JP (2002) Allosteric modulators of group I metabotropic glutamate receptors: novel subtype-selective ligands and therapeutic perspectives. Curr Opin Pharmacol 2: 43–49. doi: 10.1016/S1471-4892(01)00119-9
![]() |
[176] |
Malherbe P, Kratochwil N, Zenner MT, et al. (2003) Mutational analysis and molecular modeling of the binding pocket of the metabotropic glutamate 5 receptor negative modulator 2-methyl-6-(phenylethynyl)-pyridine. Mol Pharmacol 64: 823–832. doi: 10.1124/mol.64.4.823
![]() |
[177] | Litschig S, Gasparini F, Rueegg D, et al. (1999) CPCCOEt, a noncompetitive metabotropic glutamate receptor 1 antagonist, inhibits receptor signaling without affecting glutamate binding. Mol Pharmacol 55: 453–461. |
[178] |
Silve C, Petrel C, Leroy C, et al. (2005) Delineating a Ca2+ binding pocket within the venus flytrap module of the human calcium-sensing receptor. J Biol Chem 280: 37917–37923. doi: 10.1074/jbc.M506263200
![]() |
[179] |
Hermans E, Challiss RA (2001) Structural, signalling and regulatory properties of the group I metabotropic glutamate receptors: prototypic family C G-protein-coupled receptors. Biochem J 359: 465–484. doi: 10.1042/bj3590465
![]() |
[180] |
Nakanishi S (1992) Molecular diversity of glutamate receptors and implications for brain function. Science 258: 597–603. doi: 10.1126/science.1329206
![]() |
[181] |
Riedel G, Platt B, Micheau J (2003) Glutamate receptor function in learning and memory. Behav Brain Res 140: 1–47. doi: 10.1016/S0166-4328(02)00272-3
![]() |
[182] |
Kunishima N, Shimada Y, Tsuji Y, et al. (2000) Structural basis of glutamate recognition by a dimeric metabotropic glutamate receptor. Nature 407: 971–977. doi: 10.1038/35039564
![]() |
[183] |
Niswender CM, Conn PJ (2010) Metabotropic glutamate receptors: physiology, pharmacology, and disease. Annu Rev Pharmacol Toxicol 50: 295–322. doi: 10.1146/annurev.pharmtox.011008.145533
![]() |
[184] |
Bhanot P, Brink M, Samos CH, et al. (1996) A new member of the frizzled family from Drosophila functions as a Wingless receptor. Nature 382: 225–230. doi: 10.1038/382225a0
![]() |
[185] |
Murone M, Rosenthal A, de Sauvage FJ (1999) Sonic hedgehog signaling by the patched-smoothened receptor complex. Curr Biol 9: 76–84. doi: 10.1016/S0960-9822(99)80018-9
![]() |
[186] |
Chen CM, Strapps W, Tomlinson A, et al. (2004) Evidence that the cysteine-rich domain of Drosophila Frizzled family receptors is dispensable for transducing Wingless. Proc Natl Acad Sci USA 101: 15961–15966. doi: 10.1073/pnas.0407103101
![]() |
[187] |
Nakano Y, Nystedt S, Shivdasani AA, et al. (2004) Functional domains and sub-cellular distribution of the Hedgehog transducing protein Smoothened in Drosophila. Mech Dev 121: 507–518. doi: 10.1016/j.mod.2004.04.015
![]() |
[188] |
Isberg V, de Graaf C, Bortolato A, et al. (2015) Generic GPCR residue numbers-Aligning topology maps minding the gaps. Trends Pharmacol Sci 36: 22–31. doi: 10.1016/j.tips.2014.11.001
![]() |
1. | Leonard Matheus Wastupranata, Seong G. Kong, Lipo Wang, Deep Learning for Abnormal Human Behavior Detection in Surveillance Videos—A Survey, 2024, 13, 2079-9292, 2579, 10.3390/electronics13132579 | |
2. | Kun Zheng, Li Tian, Zichong Li, Hui Li, Junjie Zhang, Incorporating eyebrow and eye state information for facial expression recognition in mask-obscured scenes, 2024, 32, 2688-1594, 2745, 10.3934/era.2024124 | |
3. | Rongyong Zhao, Wenjie Zhu, Chuanfeng Han, Bingyu Wei, Hao Zhang, Arifur Rahman, Cuiling Li, Pedestrian Fall Detection Methods for Public Traffic Areas: A Literature Review, 2024, 14, 2076-3417, 8934, 10.3390/app14198934 | |
4. | Xiaoqun Yu, Chenfeng Wang, Wenyu Wu, Shuping Xiong, A Real-time skeleton-based fall detection algorithm based on temporal convolutional networks and transformer encoder, 2025, 107, 15741192, 102016, 10.1016/j.pmcj.2025.102016 |
Test results | Positive (factual falls) | Negative (factual safe) | Total |
Positive (Detecting falls) | True Positive (TP) | False Positive (FP) | TP + FP |
Negative (Detecting safe) | False Negative (FN) | True Negative (TN) | FN + TN |
Total | TP + FN | FP + TN | TP + FP + FN + TN |
Note: TP: Samples predicted by the model as fall and ground truth as fall. FN: Samples predicted by the model as safe but ground truth as fall. FP: Samples predicted by the model as fall but ground truth as safe. TN: Samples predicted by the model as fall and ground truth as safe. |
Model | Average iterations per second (it/s) |
Model-a | 3.07 |
Model-b | 3.01 |
Model | Average iterations per second (it/s) |
Model-a | 8.77 |
Model-b | 8.61 |
Dataset | Accuracy (%) |
FDD | 97.27 |
UFDD | 99.68 |
Model | URFD | FDD | UFDD |
Model-a | 0.987 | 0.926 | 0.931 |
Model-b | 0.982 | 0.938 | 0.986 |
FDD | MDER | EDCR | Accuracy | sensitivity | specificity |
Exchange Order | 0.5238 | 0.5 | 0.97 | 0.971 | 0.9687 |
Our method | 0.9225 | 0.847 | 0.973 | 0.9571 | 0.9852 |
UFDD | MDER | EDCR | Accuracy | sensitivity | specificity |
Exchange Order | 0.7673 | 0.867 | 0.997 | 0.9964 | 0.9992 |
Our method | 0.9268 | 0.995 | 0.997 | 0.9848 | 0.9939 |
Test results | Positive (factual falls) | Negative (factual safe) | Total |
Positive (Detecting falls) | True Positive (TP) | False Positive (FP) | TP + FP |
Negative (Detecting safe) | False Negative (FN) | True Negative (TN) | FN + TN |
Total | TP + FN | FP + TN | TP + FP + FN + TN |
Note: TP: Samples predicted by the model as fall and ground truth as fall. FN: Samples predicted by the model as safe but ground truth as fall. FP: Samples predicted by the model as fall but ground truth as safe. TN: Samples predicted by the model as fall and ground truth as safe. |
Model | Average iterations per second (it/s) |
Model-a | 3.07 |
Model-b | 3.01 |
Model | Average iterations per second (it/s) |
Model-a | 8.77 |
Model-b | 8.61 |
Methods | Accuracy (%) |
CNN [38] | 96.43 |
1D CNN [38] | 92.72 |
OF CNN [40] | 83.02 |
OF CNN [41] | 91.40 |
multi-stream DNN [42] | 99.72 |
3D CNN, LSTM [43] | 99.27 |
Deep network [44] | 99.67 |
Our method | 99.20 |
Dataset | Accuracy (%) |
FDD | 97.27 |
UFDD | 99.68 |
Model | URFD | FDD | UFDD |
Model-a | 0.987 | 0.926 | 0.931 |
Model-b | 0.982 | 0.938 | 0.986 |
FDD | MDER | EDCR | Accuracy | sensitivity | specificity |
Exchange Order | 0.5238 | 0.5 | 0.97 | 0.971 | 0.9687 |
Our method | 0.9225 | 0.847 | 0.973 | 0.9571 | 0.9852 |
UFDD | MDER | EDCR | Accuracy | sensitivity | specificity |
Exchange Order | 0.7673 | 0.867 | 0.997 | 0.9964 | 0.9992 |
Our method | 0.9268 | 0.995 | 0.997 | 0.9848 | 0.9939 |