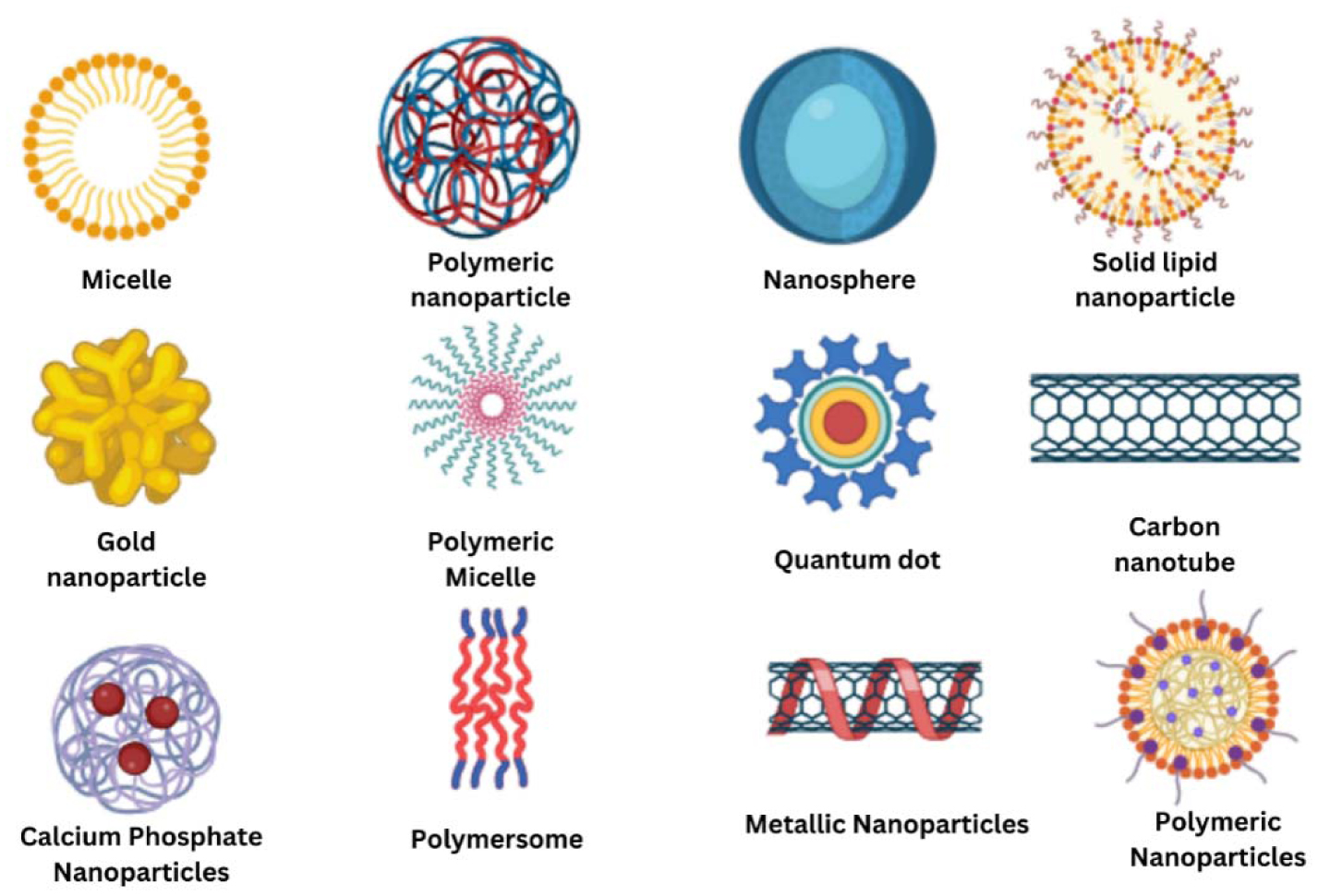
The integration of artificial intelligence (AI) with nanoparticle technology represents a transformative approach in precision oncology, particularly in the development of targeted anticancer drug delivery systems. In our study, we found out that the application of AI in the field of drug delivery enhances the value of nanoparticles in increasing the efficiency of drug delivery and decreasing side effects from specifically targeted mechanisms. Moreover, we considered urgent issues that exist in present-day nanoparticle fabrication such as scalability and regulatory issues and suggest AI solutions that would help overcome these issues. In the same manner, we focused on the utilization of AI in enhancing patient-centered treatment through the establishment of drug delivery systems that will complement the genetic and molecular makeup of patients. Additionally, we present evidence suggesting that AI-designed nanoparticles have the potential to be environmentally sustainable alternatives in cancer therapy. AI is presented as capable of creating synergies with bioengineering methods like CRISPR or gene therapy and able to show potential directions for enhancing the efficiency of nanoparticle-based cancer therapies. In implementing these findings, we systematically discuss and highlight ongoing work and potential development trends in AI-aided nanoparticle research, asserting that it has the potential to greatly enhance oncology therapy.
Citation: Mujibullah Sheikh, Pranita S. Jirvankar. Harnessing artificial intelligence for enhanced nanoparticle design in precision oncology[J]. AIMS Bioengineering, 2024, 11(4): 574-597. doi: 10.3934/bioeng.2024026
[1] | Amin Shakiba, Oussama Zenasni, Maria D. Marquez, T. Randall Lee . Advanced drug delivery via self-assembled monolayer-coated nanoparticles. AIMS Bioengineering, 2017, 4(2): 275-299. doi: 10.3934/bioeng.2017.2.275 |
[2] | Meiling Sun, Changlei Cui . Transforming lung cancer care: Synergizing artificial intelligence and clinical expertise for precision diagnosis and treatment. AIMS Bioengineering, 2023, 10(3): 331-361. doi: 10.3934/bioeng.2023020 |
[3] | P. Mora-Raimundo, M. Manzano, M. Vallet-Regí . Nanoparticles for the treatment of osteoporosis. AIMS Bioengineering, 2017, 4(2): 259-274. doi: 10.3934/bioeng.2017.2.259 |
[4] | Praveen Kumar, Sakshi V. Izankar, Induni N. Weerarathna, David Raymond, Prateek Verma . The evolving landscape: Role of artificial intelligence in cancer detection. AIMS Bioengineering, 2024, 11(2): 147-172. doi: 10.3934/bioeng.2024009 |
[5] | Ana Letícia Braz, Ifty Ahmed . Manufacturing processes for polymeric micro and nanoparticles and their biomedical applications. AIMS Bioengineering, 2017, 4(1): 46-72. doi: 10.3934/bioeng.2017.1.46 |
[6] | David Raymond, Induni Nayodhara Weerarathna, John Kessellie Jallah, Praveen Kumar . Nanoparticles in biomedical implants: Pioneering progress in healthcare. AIMS Bioengineering, 2024, 11(3): 391-438. doi: 10.3934/bioeng.2024019 |
[7] | F. Philipp Seib . Silk nanoparticles—an emerging anticancer nanomedicine. AIMS Bioengineering, 2017, 4(2): 239-258. doi: 10.3934/bioeng.2017.2.239 |
[8] | Dewmini Mututantri-Bastiyange, James C. L. Chow . Imaging dose of cone-beam computed tomography in nanoparticle-enhanced image-guided radiotherapy: A Monte Carlo phantom study. AIMS Bioengineering, 2020, 7(1): 1-11. doi: 10.3934/bioeng.2020001 |
[9] | Gerald Pawlish, Kyle Spivack, Adam Gabriel, Zuyi Huang, Noelle Comolli . Chemotherapeutic loading via tailoring of drug-carrier interactions in poly (sialic acid) micelles. AIMS Bioengineering, 2018, 5(2): 106-132. doi: 10.3934/bioeng.2018.2.106 |
[10] | Daniel Calle, Duygu Yilmaz, Sebastian Cerdan, Armagan Kocer . Drug delivery from engineered organisms and nanocarriers as monitored by multimodal imaging technologies. AIMS Bioengineering, 2017, 4(2): 198-222. doi: 10.3934/bioeng.2017.2.198 |
The integration of artificial intelligence (AI) with nanoparticle technology represents a transformative approach in precision oncology, particularly in the development of targeted anticancer drug delivery systems. In our study, we found out that the application of AI in the field of drug delivery enhances the value of nanoparticles in increasing the efficiency of drug delivery and decreasing side effects from specifically targeted mechanisms. Moreover, we considered urgent issues that exist in present-day nanoparticle fabrication such as scalability and regulatory issues and suggest AI solutions that would help overcome these issues. In the same manner, we focused on the utilization of AI in enhancing patient-centered treatment through the establishment of drug delivery systems that will complement the genetic and molecular makeup of patients. Additionally, we present evidence suggesting that AI-designed nanoparticles have the potential to be environmentally sustainable alternatives in cancer therapy. AI is presented as capable of creating synergies with bioengineering methods like CRISPR or gene therapy and able to show potential directions for enhancing the efficiency of nanoparticle-based cancer therapies. In implementing these findings, we systematically discuss and highlight ongoing work and potential development trends in AI-aided nanoparticle research, asserting that it has the potential to greatly enhance oncology therapy.
Precision oncology is an approach to treating cancer that is tied to the molecular, epigenetic, and phenotypic profiles of a patient's tumor. This approach highlights the importance of comprehending tumor heterogeneity, which is the difference in cells constituting the tumor, within a patient or between two patients. For example, the EPR effect is a foundation for drug delivery in precision oncology, but its variability across tumors confounds nanoparticle-based drug delivery systems [1],[2]. Hence, the effectiveness of therapies should depend on the type of tumor, which contributes to high treatment effectiveness.
The personalized treatment approach is even more important given the recent development of liquid biopsies, which enable the identification of circulating tumor cells and DNA. This technology enables actual time tracking of the interferometric heterogeneity of tumors and their reactions to certain treatments on the basis of the patient's genome [3]. Furthermore, progress in molecular targeted therapy, especially in lung cancer, indicates how precise knowledge of the differences in the biochemical properties of neoplastic cancer cells allows proper targeted therapy [4]. Cancer treatment is challenging because cancer biomarkers are available, AJSW.
The human body is unique. This heterogeneity is present on the spectrum of different cancer types, in which patients have different molecular profiles and certain driver mutations that contribute to high tumor heterogeneity—a problem for cancer therapy [5],[6]. In this period, diagnostic NPs, including QDs, AuNPs, and PDs, have been used for distinguishing patient-specific diseases [7],[8] (see Figure 1). These enhancements can be carried out further by therapeutic nanotechnologies that could provide improved targeted medical therapies and enhanced personalized medicine. However, the problem of tumor heterogeneity hinders the construction of a systematic diagnostic and therapeutic model that can effectively process patient data. The combination of artificial intelligence (AI) and nanotechnologies can be considered a solution [9]. AI can utilize a large amount of patient information, estimate patient prognosis, assess pharmacodynamics/toxicodynamics in near real time, and help in the detection of cancer biomarkers. In addition, optimization that is intrinsic to AI might improve nanomedicine by fine-tuning the properties of the chosen materials and accelerating the study of drug-pharmacological interactions and molecular recognition with the immune system and cellular membrane. In general, the integration of AI and the concept of precision medicine stems from the complexity involved in cancer therapy and enables the development of better healthcare models that individuals can experience [9]. Most progressive developments in material science have made new formulations that have better characteristics and are important in numerous ways, including catalysis and energy storage [10].
While precision oncology holds much potential in relation to patient treatment, several obstacles exist that may prevent its greater use. A concern that can be raised is the low effectiveness of targeted treatments and the emergence of drug resistance most of its time. It is therefore critical to appreciate the inherent biological pathways that underlie drug resistance in the management of this disease to identify molecular targets for resistance reversal [11]. For example, various molecular changes require distinct treatments; nonetheless, the manageability of such treatment regimens can present substantial challenges in clinical practice [3]. Furthermore, challenges in delivering therapeutic agents beyond biological barriers continue to be a major challenge in precision oncology [12]. Research has demonstrated that new design strategies involving DNA nanostructures can potentially improve targeted drug delivery and reduce side effects, as noted by Prabhakar et al. (2013), who reported that despite the advancements in targeted drug delivery systems employing the EPR effect, the variability of the latter makes it necessary to conduct ongoing research to identify more efficient delivery systems that can increase patient outcomes [2]. Researchers have focused on the interfaces of various systems at the atomic and molecular level. This understanding not only extends to the design of better catalysts but also involves possibilities for the preparation of materials with specific functions. For instance, some researchers investigated the preparation of composite materials incorporating organic structures and metallic colloid, showing higher conversion efficiency than conventional ways [13].
Notably, however, there are many deficiencies in the knowledge base of precision oncology. Much more work remains to be done in ‘painting the complete picture’ of heterogeneity within tumors and multiagent resistance as well as refining approaches to targeting malignant tissues with therapeutic agents. In the same way, the use of AI in processing large aggregates may improve the knowledge of tumor features and contribute to personalized treatments [15].
The research and development of advanced materials and related disciplines have become more popular, with chemistry and material science being the most prominent disciplines. One growing research area that has attracted a lot of interest is the synthesis and investigation of new materials with specific properties. For instance, current research shows that distinct organic and inorganic species contribute to the improvement of the catalytic reactions, which are important for several industries [16]. Further, the analytical methods have been enhanced to provide a clearer comprehension of the structural and functional characteristics of these materials. Methods like spectroscopy and microscopy are used more frequently to explain higher performance of Mercury Cathode Operating Point devices [17].
Future work should also address technologies that are under development, including blood-based diagnostic techniques, also known as liquid biopsies, as well as advanced drug delivery systems.
A comprehensive search strategy was employed to identify relevant sources for this review article, in which we explore harnessing AI-driven nanoparticle design for precision oncology. The literature search was conducted through academic databases, including PubMed, Web of Science, and Scopus, with studies, reviews, and reports conducted between 2010 and the present. To improve the identification of selected studies, search terms such as “AI-Driven Nanoparticle Design,” “Precision Oncology,” “Biomedical Science,” “Robotics,” and “Healthcare Innovation” were used during the identification of the articles. Accordingly, articles, reviews, and reports were chosen by adhering to preset inclusion and exclusion criteria pertinent to the research question. The criteria for the inclusion of those sources were as follows: the sources must be in English, describing the modern achievements in the spheres of AI applied in oncology, the topic of robotics in the sphere of nanoparticle delivery systems, and the sources must be published between 2005 and 2023. This systematic approach to using keywords was intended to provide both the breadth and currency of the literature so that more novel and potential developments in the field could be examined in this review.
In precision oncology, the mode of modifying the size and shape of the nanoparticles for the delivery of drugs becomes critical because the greater efficiency of the specifically oriented drug carriers reduces side effects. This is due to the attributes of engineered nanoparticles (NPs), which are designed to locate cancer cells and deliver therapeutic agents to such cells owing to their size, shape, and surface coatings. Active targeting can be further divided into invasive targeting, which makes use of the known phenomenon of the EPR effect in tumors, and active targeting, where NPs are conjugated with ligands that specifically recognize receptors abundant in cancer cells [18],[19]. Smart nanoparticles can respond to certain biological signals, meaning that drug delivery in tumors is localized and self-administered by patients [1],[18]. This design also facilitates multitreatment regimens through coencapsulation of various treatment molecules or multifunctionality, where diagnostics and treatment are combined in a nanoparticle (theranostics), which can lead to the emergence of drug resistance [20]. The evolving field of research in certain areas of nanoparticle-based systems points toward a sociology revolution in cancer treatment produced by increasing the precision and efficacy profile of CD treatments that are tailored to fit patient types.
Cancer is one of the most significant threats to global health, causing both remarkable economic losses and social stresses at the most sensitive levels. The American Cancer Society estimated that in 2022, there would be approximately 1,918,030 new cancer cases and 609,360 deaths in the United States [21]. More people die from lung cancer and colon or rectal cancer and from breast cancer in women as well as prostate cancer in men (Figure 2). The incidence of cancer is even higher in the European region, as it contributes to approximately 24% of total world cancer cases; however, it affects only 10% of the world's population. Lung cancer is the leading cause of cancer-related deaths, claiming more than 350 lives each day in the United States alone. According to ACS, with present trends persisting, approximately 35 million new cancer cases will occur by 2050, mainly because of population rise and aging. Some of these risks are smoking, consuming too much alcohol, having an unhealthy diet, and doing little or no exercise. The greatest increase is projected among low- and middle-income countries, where cancer is the fourth main cause of mortality in people younger than 70 years and may further deteriorate [22].
Consequently, owing to the adverse side effects of conventional cancer therapies, scientists are considering nanoparticles as potential kids for cancer therapies [23]. Nanoparticles offer operating functions for improving the bioavailability of deliverable agents in drug delivery systems since they help reduce interference from side effects. Because of their properties, they exhibit selective therapy, which is very advantageous in developing nations where most therapies cannot be implemented [24]. The discovery of nanoparticles has revolutionized cancer therapy, especially in drug delivery systems. Lipid nanoparticles have received attention because of their physicochemical properties, which make them superior to conventional therapies, including high stability, improved biocompatibility, and targeted delivery. According to Yao et al. (2020), hybrid nanoparticles combine the characteristics of various nanoparticles; these kinds of nanoparticles have been shown to enhance drug delivery and overcome drug resistance. This remains important, as drug resistance remains a critical issue in the management of cancer through mechanisms such as efflux, which is enhanced by increased efflux transporter proteins and apoptotic dysfunction. This finding demonstrates the ability of nanoparticles to both exert pressure on malignant cells to reveal their mechanisms of drug resistance and, more generally, the ability of nanoparticles in chemotherapy, targeted therapy and immunotherapy as valuable alternatives for the expectant treatment of patients [20].
The subsequent development of nanoparticle technology has led to the incorporation of combination chemotherapy regimens with nanoparticle drug delivery products. According to Hu et al. (2010), nanoparticles enhance the pharmacokinetics of multiple drugs and thus decrease side effects and increase therapeutic effectiveness. This dual approach enhances the delivery of chemotherapeutic agents and solves a series of problems associated with the formulation of nanoparticles that release drugs at the required site. Researchers address the importance of accurately conveying the design nous for these systems toward drug release and targeting, suggesting a future research avenue [25].
The shape and size of nanoparticles also significantly influence their efficiency as drug carriers. Truong et al. (2015) reported that the shape of nanoparticles determines biocirculation and cellular uptake; irregularly shaped nanoparticles provide better drug delivery properties than spherical nanoparticles do. While the other morphologies, e.g., filamental or disk-like nanoparticles, hold much promise, the fact that most nanoparticle-based applications being developed today are spherical indicates that there is room for more research into the creation of nanoparticles that have more diverse morphologies for better cancer treatment [26].
The focus is on examining the complex interplay between nanoparticles and cancer treatments, highlighting their diverse types and the regulatory pathways established by the FDA. The first nanoparticle approved by the FDA in 1995 was PEGylated liposomes with doxorubicin (Doxil), which is intended for treating AIDS-related Kaposi's sarcoma. When formulated in this way, the detrimental reactions of doxorubicin were minimized. Since then, more liposomal formulations have entered the market and been approved for cancer treatment, such as Myocet and DaunoXome (Table 1) [27]–[29]. Two prominent groups of nanocarriers, which are based on phospholipid molecules, are liposomes and micelles; however, the two remain dissimilar in shape and structure. These nanoparticles are mainly used for solubilization of hydrophilic drugs into their central aqueous compartment, mimicking biological cell membranes. Lipophilic drugs can be physically adsorbed on the liposome surface, incorporated within the bilayer, or be chemically conjugated with the liposome [30]. On the other hand, micelles can incorporate hydrophobic medications within the hydrophobic tail portion of the micelles. Other formulations based on polymeric nanoparticles are approved by the FDA, including Abraxane (albumin–paclitaxel particles for pancreatic ductal adenocarcinoma and metastatic breast cancer) and Ontak (recombinant protein of diphtheria toxin with interleukin-2 for non-Hodgkin's peripheral T-cell lymphoma) [31]. These formulations have demonstrated improved circulating toxicity as well as biocompatibility, with certain drawbacks in associated drug content [32],[33].
Drug Name | Type of Nanoparticle | Active Drug | Type of Cancer Targeted | Year of Approval | Ref |
Doxil | Liposome | Doxorubicin | Breast cancer, ovarian cancer | 2010 | [34] |
Abraxane | Albumin-bound nanoparticle | Paclitaxel | Breast cancer, non-small cell lung cancer | 2012 | [35] |
Onivyde | Liposome | Irinotecan | Pancreatic cancer | 2015 | [36] |
Vyxeos | Liposome | Cytarabine and Daunorubicin | Acute myeloid leukemia | 2017 | [37] |
Epcoritamab | Nanobody | Monoclonal antibody | B-cell malignancies | 2022 | [38] |
Carvykti | CAR-T-cell therapy | Abecma | Multiple myeloma | 2021 | [39] |
Marqibo | Liposome | Vincristine | Acute lymphoblastic leukemia | 2018 | [40] |
Pemetrexed | Polymeric nanoparticle | Pemetrexed | Mesothelioma, non-small cell lung cancer | 2020 | [41] |
Libtayo | Nanobody | Cemiplimab | Cutaneous squamous cell carcinoma | 2022 | [42] |
Recent debates in the creation of new nanoparticles (NPs) have shifted to consider the characterization of NPs and controlling their design and formulation. This optimization particularly aims to achieve optimal biological compatibility and loading capacity in relation to the agent's systemic toxicity. Inorganic NPs, as a class of nanoparticles, have high stability coefficients and low biodegradability [43]. A formula named Nanotherm, in which iron oxide coated with amino silane is preferable, has received approvals for glioblastoma treatment. Another inorganic NP type is quantum dots, which are a group of semiconductor nanocrystals with complementary optical and electrical characteristics [43]. These characteristics make them highly fluorescent together with their relative photobleaching stability, which is essential for imaging and detection. Some analyses have shown that they are conjugated with anti-HER2 antibodies and coated with poly(ethylene glycol) (PEG) to strengthen the targeting selectivity of tumor cells [44]. Gold nanoparticles are also good examples of the versatility of inorganic NPs. These properties, coupled with their optical, electrical, and low toxicity, make them applicable in imaging techniques such as contrast agents in computed tomography and photoacoustic imaging [45]. One of the nanocarriers, AuroShell, is a PEG-coated gold nanoshell with a silica core that was approved by the FDA in 2012 to treat breast cancer through photodynamic therapy [46].
The integration of artificial intelligence with the design of micro- and nanoparticles has become one of the most powerful tools in the field of nanomedicine for improving drug delivery systems. Practical applications of AI technologies include selecting the appropriate nanoparticle size, shape, and surface chemistry to facilitate drug targeting and the controlled release of the drug [47]. Big data and data mining techniques help researchers mimic molecular interactions to understand nanoparticle behavior in biosystems, which enhances the response of customized drugs to complex diseases such as cancer and genetic disorders [48].
Modern partnerships with commercial and academic partners showing how academic research can turn to AI in designing nanoparticles. For example, the Cardiff University and AstraZeneca partnerships revealed how the AI approach can significantly improve the optimization of the plethora of parameters of the synthesis process, resulting in the design of more effective nanoparticles than the initial prototypes. This computational learning application is thus used to fashion nanoparticles to deliver specific therapeutic molecules with improved stability and enhanced drug loading and efficiency at site deposition within the body [49]. Such development not only provides conducive therapeutic gain but also minimizes side effects that are systemically induced, as in conventional dosing systems.
By developing and optimizing the nanomaterial fabrication approach, a combinatorial synthesis strategy guided by high-throughput experimental data is crucial for future research. For example, Chan et al. developed a reproducible approach for high-throughput synthesis of colloidal nanocrystals that allows for a systematic approach involving multiple parameter spaces [50]. By doing so, this approach enables better control over synthesis procedures while also improving reusability and final nanocrystal properties. Similarly, Kajita et al. used an ensemble descriptor that combines high-throughput screening with machine learning for the effective identification of promising SICs from databases of numerous materials. Although they used only a limited number of instances for training, their method succeeded in achieving the best performance in terms of inference power. Both examples reflect the critical contribution of machine learning and high-throughput data for the synthesis of new materials [51]. This method will also be applicable to the large-scale exploration of biomedical nanomaterials if proper and efficient variables are found. For example, Yamankurt et al. described one thousand candidates to optimize spherical nucleic acids (SNAs) via different SNA design parameters. They introduced a high-throughput screening procedure to assess the activity of SNA-based nanoformulations and used the data to develop a classification model for predicting the activity of newly synthesized SNA formulations [52].
Defects play an important role in the properties of nanomaterials and may be categorized as point, line, or surface defects. However, structural defects are very often difficult and tedious to evaluate and visualize, which tends to be accomplished via high-end techniques such as high-resolution transmission electron microscopy. Nevertheless, nanoscale materials that possess defects can possess fantastic optical and physical properties, such as enhanced redox reaction capacity [53]. Wu et al. sought to understand how defects govern the performance of nanomaterials and sought to create a collection of 425 metal–organic frameworks (MOFs) with defects. To better understand the effects of defects on MOF materials, they trained decision tree and logistic regression models on this dataset. Additionally, defect detection and identification during nanomaterial characterization could be achieved more quickly via deep learning methods in the analysis of microscopy images [54]. These breakthroughs have improved the understanding of structural weaknesses and structural phase fluctuations over nanomaterials, which are necessary for developing defensively asymmetric materials [54].
The inverse methodology behind this design approach is transformative for the rational synthesis of nanomaterials, emphasizing functionality-directed features. This method fixes the functional requirements and changes the material structural parameters. For example, Li et al. presented a novel revelation of random forest multiobjective regression for inverse design, which has largely focused on predicting structural features given the desired properties [55]. Using multitarget algorithms, they mapped the vast design space of multifunctional nanoparticles to identify structure–activity relationships that delivered desired performance features.
Similarly, Thomas et al. and colleagues used a decision tree algorithm to identify how the physical properties of nanoparticles, such as size and zeta potential, are essential in relation to the immune response of organisms. In particular, we aimed to understand how the complement system and its reaction to the presence of nanomaterials are affected by specific nanoparticle characteristics. In this way, it is possible to make nanoparticles safer and more biocompatible and ensure that the physical properties of nanomaterials can be regulated to influence the immune response. Specifically, it is crucial for nanoparticles to avoid being detected by the immune system while being active in the organism to achieve the planned therapeutic effects [27],[56]. Boso et al. and colleagues studied how well artificial intelligence can predict whether tiny light-up plastic beads will stick to glass sides depending on how fast the liquid moves and how large the beads are. Moreover, this also means that designing these particles just right can help them stick to places where we need them, such as sick parts of the body. This finding indicates that using an inverse design not only increases performance but also causes therapy to be more effective [57].
The term “precision medicine,” closely linked to the concept of personalized treatment, started to capture considerable interest around the year 2010. In 2015, the National Research Council and President Obama's Precision Medicine Initiative worked to create customized treatments for people on the basis of their unique health information [57]. Although precision medicine and personalized medicine are often viewed as the same, there are slight distinctions between the two. The U.S. National Research Council supports the use of the term “precision medicine,” suggesting that it includes more detailed differences in meaning. This ambiguity has sparked scholarly debates concerning related ideas such as stratified medicine P4. Through the use of extensive patient data, such as genetic details and molecular traits, precision medicine enables healthcare providers to predict disease progression and customize preventive strategies accordingly [58],[59]. Moreover, it assists in the introduction of targeted prevention methods on the basis of personal risk evaluations, potentially reducing the chances of tumor development or progression. This all-encompassing approach positions precision medicine not only as a treatment method but also as a proactive strategy for disease prevention and management [60].
Innovations in tumor biology have enabled scientists to focus on different cancer cell surface proteins, disrupted oncogenes, and signaling pathways for targeted drug delivery. There is an urgent demand for more effective drug delivery systems, which has prompted the investigation of AI-driven nanoparticles. These nanoparticles use artificial intelligence to enhance their design and effectiveness, potentially changing the environment of cancer treatment [61].
Figure 4 shows the two main strategies used by AI-driven nanoparticles (NPs) for delivering treatments in oncology: Passive and active targeting [62]. Passive targeting relies on the enhanced permeability and retention (EPR) effect, which exploits the irregular structure of blood vessels and poor lymphatic drainage often observed in tumors. On the other hand, active targeting involves engineering NPs with ligands that target receptors that are overexpressed on cancer cells, assisting accurate drug delivery [63],[64]. AI algorithms can improve this method by predicting the best ligand–receptor interactions and configurations of nanoparticles, thus increasing their effectiveness and specificity [62].
This illustrates the important role of AI-driven nanoparticles (NPs) in enhancing precision medicine approaches for cancer therapy. NPs incorporate targeting ligands such as aptamers, small molecules, and antibody fragments, which bind to receptors that are overexpressed on cancer cells. This strategy increases the specificity for tumors while minimizing off-target toxicity. In addition, the inclusion of cancer-targeting ligands in NPs improves their uptake and supports receptor-mediated endocytosis.
Antibodies are among the most used ligands for active targeting. They are favored because they are easily accessible, have a high binding affinity, and target cancer cells, including those found in breast cancer. The distinctive Y-shaped structure of these proteins allows their two arms to attach effectively to antigens. In addition, small peptides and proteins can be alternative ligands. These alternatives offer several benefits, such as lower molecular weights, improved diffusion properties, reduced immunogenicity, simpler production, and greater flexibility in chemical conjugation methods [65]. Aptamers are short strands of RNA or DNA that consist of a single chain. These oligonucleotides can assume distinct shapes, enabling them to bind specifically to certain targets. This ability results in the formation of complex three-dimensional structures that attach securely to surface markers [65].
Table 2 provides an overview of different ligand-targeted nanoparticles that are currently in clinical trials. This emphasizes the use of AI-driven techniques to enhance precision in cancer treatment. These nanoparticles are designed to deliver therapeutic agents directly to cancer cells, which improves drug effectiveness while minimizing off-target side effects. The table features various ligands, including antibodies, peptides, and aptamers, that allow for selective binding to receptors that are overexpressed on tumor cells. Additionally, the role of artificial intelligence in optimizing nanoparticle design and functionality is discussed, showing how AI can predict interactions and enhance targeting capabilities. By implementing these advanced strategies, researchers aim to address issues related to tumor heterogeneity and physiological barriers, with the objective of improving treatment outcomes and reducing overall toxicity in cancer patients.
Prostate-specific membrane antigen (PSMA) is highly abundant in prostate cancer. This characteristic makes it a critical target for the direct administration of treatments to prostate tumors [72]. Efforts have been made to use PSMA for directed drug delivery. One example is the development of PSMA-targeted polymeric nanoparticles that contain docetaxel (BIND-014). This formulation has been used in phase I and II clinical trials, demonstrating its ability to specifically target metastatic prostate cancer cells that express the PSMA receptor [73]. Preclinical toxicokinetic studies carried out in different animal models have shown that BIND-014 is located within blood vessels. This has resulted in enhanced effectiveness against several types of cancer, such as cervical cancer and cholangiocarcinoma [74]. Including the PSMA-targeting ligand on these nanoparticles led to increased absorption by cancer cells and new blood vessels. This effect is supported by improved permeation and retention (EPR) [75]. In a phase II clinical trial, BIND-014 demonstrated antitumor effects in patients with metastatic castration-resistant prostate cancer. This led to an improvement in median overall survival and a major reduction in PSA levels for certain patients [76].
Nanoparticle Name | Ligand Type | Targeted Cancer Type | AI Application | Clinical Trial Phase | Ref |
Doxil | Antibody | Breast Cancer | AI algorithms optimize formulation and dosing | Phase III | [20] |
Abraxane | Albumin | Non-Small Cell Lung Cancer | Machine learning predicts patient response | Phase II | [66] |
ONPATTRO | siRNA | Amyloidosis | AI enhances delivery efficiency | Phase III | [67] |
Docetaxel-Loaded NPs | Small Molecule | Prostate Cancer | AI models optimize nanoparticle design | Phase II | [20] |
HER2-Targeted NPs | Monoclonal Antibody | Gastric Cancer | AI assists in biomarker profiling | Phase II | [61] |
RNAi Nanoparticles | Aptamer | Ovarian Cancer | AI predicts interactions with cancer biomarkers | Phase I | [68] |
Paclitaxel-Loaded NPs | Peptide | Breast and Lung Cancer | AI optimizes drug release profiles | Phase II | [66] |
Hybrid Liposome-NPs | Combination of Ligands | Various Cancers | AI aids in hybrid nanoparticle stability | Phase I | [69] |
Silica-Core NPs | Small Molecule | Pancreatic Cancer | AI improves targeting accuracy | Phase II | [70] |
Theranostic NPs | Diagnostic Ligand | Multiple Cancer Types | AI integrates imaging and therapeutic functions | Phase III | [71] |
In precision medicine, the use of certain biomarkers plays an important role. For example, PSMA is used in prostate cancer, whereas HER2 is relevant in breast cancer. This marked an important shift toward personalized treatment methods. HER2 is frequently overexpressed in numerous breast cancers, prompting the attachment of HER2 ligands to various nanoparticles to effectively target these cells [77]. Preliminary clinical trials have indicated that formulations such as MM-302, which are used both on their own and alongside trastuzumab, exhibit enhanced effectiveness and safety for patients with metastatic breast cancer [78]. However, findings from phase II trials revealed no important survival advantage compared with traditional chemotherapy. Other receptors of interest in breast cancer treatment include the transferrin receptor (TfR), epidermal growth factor receptor (EGFR), and folate receptor. For example, rapamycin-loaded PLGA nanoparticles combined with EGFR antibodies have shown improved cellular uptake and increased apoptotic effects in human breast cancer cells [79].
In non-small cell lung carcinoma (NSCLC), there are high levels of epidermal growth factor receptor (EGFR). MUC1 is another glycoprotein that is often overexpressed in both lung and breast cancers [80]. Researchers conducting clinical trials for lung cancer are investigating tecemotide, a synthetic lipopeptide that specifically targets MUC1 [81]. This treatment is being assessed for its effectiveness as maintenance therapy following chemoradiotherapy. Although it has shown a favorable tolerability profile, its effectiveness remains somewhat limited [82]. Other novel strategies include the use of gelatin carriers that are modified with biotinylated EGF to assist in the targeted delivery of cisplatin, which has led to decreased kidney toxicity in preclinical studies. Moreover, mesoporous silica nanocarriers that focus on LHRH receptors have proven to be effective in delivering anticancer agents directly into lung cancer cells [83].
Combining AI with nanoparticle technology is transforming diagnostics, especially in biomedical fields. Numerous studies emphasize that improving the properties of nanoparticles—such as their size, shape, and surface chemistry—is essential for increasing their diagnostic effectiveness. For example, Hoshyar et al. (2016) emphasized the ability of AI-driven machine learning algorithms to predict the optimal arrangements of nanoparticles that enhance cellular interactions and imaging efficiency. This progress creates possibilities for developing customized probes for molecular imaging [84]. The improved ability to predict outcomes enhances both the sensitivity and specificity of disease diagnostics. Additionally, it simplifies the design process, leading to increased efficiency [84]. Quantum dots (QDs) are a major area where AI can greatly improve diagnostic capabilities. Zrazhevskiy et al. (2010) emphasized that AI techniques can enhance the design of QD probes by analyzing their photophysical properties and surface chemistry for specific diagnostic applications. By predicting QD performance in various biological environments, AI has aided in the development of multifunctional nanodevices that can provide real-time imaging, thus accelerating the transition of QD technology from research laboratories to clinical practice [85]. Gold nanoparticles are important because of their use in cancer diagnostics and treatment. Singh et al. (2018) described how AI can enhance the targeting and functionalization of gold nanoparticles, increasing their effectiveness in locating tumor cells. This development eventually leads to more accurate cancer detection. Additionally, AI's capacity to assist in developing personalized treatment plans emphasizes its essential importance in enhancing patient outcomes in cancer therapy [86].
In addition, multifunctional nanoparticles are used for targeted molecular imaging in computed tomography (CT) diagnostics, emphasizing the extensive applications of AI in this field. Kim et al. (2010) demonstrated that AI-driven techniques can enhance nanoparticle design by optimizing particular ligands, which improves targeting accuracy and enriches patient care by merging imaging with therapeutic methods [87]. Magnetic nanoparticles (MNPs) play a major role in both diagnostics and therapy, as noted by Wilson et al. (2012). Employing AI to improve the synthesis and functionalization of MNPs for biosensing applications can lead to the development of highly sensitive diagnostic instruments. These instruments use the unique characteristics of MNPs, which can enhance disease detection and monitoring [88]. In addition, Dias and Torkamani (2019) described how artificial intelligence can enhance the design of nanoparticle–antibody conjugates that are used in LSPR bioassays. This enhancement increases the sensitivity of detection methods and aids in the development of highly sensitive assays for analytes found at low concentrations. The application of AI in this field illustrates its major potential to improve clinical diagnostics [89].
Researchers have emphasized the promise of employing engineered precision nanoparticles for medication delivery. Mitchell et al. (2021) outlined methods for creating nanoparticles that can deliver chemotherapy drugs in a controlled manner, enhancing treatment effectiveness while minimizing overall toxicity. This approach to controlled release is particularly important in precision oncology, where the goal is to personalize treatments to the specific profiles of individual patients [1]. In addition, Aung et al. (2017) emphasized the importance of genomic profiling in advanced pancreatic ductal adenocarcinoma (PDAC). These authors suggested that nanoparticles could be designed to release drugs in response to specific genomic markers. Using AI to analyze genomic and transcriptomic data has the potential to optimize these nanoparticles for targeted delivery, which could considerably improve treatment outcomes [90]. In the area of immunotherapy, Shao et al. (2015) emphasized the potential of smart nanoparticles to improve antitumor immunity through the targeted delivery of antigens or adjuvants to immune cells. Using machine learning algorithms to analyze immune responses can aid in the development of nanoparticles that adapt to the changing features of tumors, thus increasing the effectiveness and personalization of immunotherapy [91]. Similarly, Senapati et al. (2018) focused on controlled drug delivery systems. This emphasized the concept that smart nanoparticles can be personalized for particular therapeutic applications on the basis of the individual requirements of each patient [92].
In addition, researchers have explored the combination of AI and imaging technologies to anticipate clinical outcomes, particularly concerning non-small cell lung cancer (NSCLC). Xu et al. (2019) demonstrated how deep learning networks can assist in the development of nanoparticles that react to imaging biomarkers. This combination allows for real-time adjustments to treatment plans, enhancing the flexibility and precision of therapy [93]. Moreover, research conducted by Sun et al. (2019) on smart hydrogels emphasized how artificial intelligence can adjust to variations in the tumor microenvironment. This emphasizes the adaptable nature of AI-driven designs in drug delivery systems [94].
The challenge of targeting cancer stem-like cells has been specifically addressed by developing doxorubicin-encapsulated polymeric nanoparticles, as noted by Rao et al. (2015). These nanoparticles are created to target CD44 receptors, demonstrating how AI can enhance targeting methods aimed at preventing tumor recurrence [91]. Similarly, Sykes et al. (2016) investigated how nanoparticles move within solid tumors and emphasized the importance of personalized designs that consider the unique features of the tumor microenvironment. AI can support this initiative by modeling the interactions between nanoparticles and tumor tissues, eventually enhancing the effectiveness of treatments [95]. Magnetic nanoparticles (MNPs) have been studied for their various functions in cancer treatment [95]. Bhinder and colleagues (2021) suggested that artificial intelligence can improve the physicochemical characteristics of magnetic nanoparticles (MNPs). This enhancement has led to more efficient targeted drug delivery and imaging, contributing to advancements in precision oncology [96]. A study on manganese dioxide nanoparticles (Zhang et al., 2019) has shown that designs driven by artificial intelligence can increase the oxygen supply to tumors, which is important for the effectiveness of therapies [97].
AI applications in the early stages of nanoparticle development may benefit from more extensive datasets that encompass diverse tumor characteristics and patient demographics. Future studies could concentrate on hybrid nanoparticles that combine different therapeutic methods, using AI for enhanced personalization. In addition, establishing standardized protocols for AI-assisted nanoparticle design could facilitate cooperation among research institutions, leading to faster advancements in precision oncology. Finally, examining the long-term effects of these innovative nanoparticles on tumor development and patient outcomes will be important for demonstrating their effectiveness and safety in clinical settings.
The integration of AI into the design of nanoparticles and their drug delivery signifies a major change in biomedical applications. Recent research has emphasized the critical role of machine learning models in enhancing the efficiency and effectiveness of these systems. By using computational chemistry alongside AI techniques, researchers can optimize the design of nanoparticles to ensure that they are both effective for drug delivery and safe for clinical use. This partnership between computer science and the physical sciences is important for fully realizing the potential of AI in drug delivery applications [98].
Nanotechnology plays an essential role in cancer treatment, particularly through the use of gold nanoparticles. These nanoparticles are useful for managing hyperthermia, which helps enhance the effectiveness of localized cancer therapies. Additionally, they can detect specific biomarkers linked to various cancer types. The possible integration of artificial intelligence with gold nanoparticle technology may enhance the targeting of treatments by focusing on the molecular signature of a tumor. This approach uses imaging data to identify biomarkers that show how effective a treatment is (Kennedy et al., 2011). This dual capability emphasizes the importance of designing nanoparticles to improve both treatment outcomes and biomarker detection [99]. The investigation of nanoparticles in drug delivery systems reveals the potential of nanocomposite hydrogels. These systems combine nanoparticles and hydrogels to create multifunctional platforms capable of delivering therapeutic agents while detecting cancer biomarkers. By engineering nanoparticles to interact with particular biomarkers, hydrogels can release medications upon the identification of these biomarkers, thereby improving the precision and effectiveness of treatment [100]. This combination signifies a major advancement in personalized medicine. In this approach, treatment strategies are customized not only to the presence of cancer but also to the molecular characteristics of the tumor.
The use of predictive modeling in relation to nanoparticles (NPs) has gained major attention because of its potential to enhance drug delivery systems by increasing cellular uptake and affecting drug release profiles. Researchers have emphasized the detailed interactions between engineered nanoparticles and biological systems, emphasizing the essential role of various physicochemical properties and their impact on therapeutic effectiveness. A major finding in this area is the influence of surface properties, particularly charge, on how cells absorb nanoparticles. Fröhlich (2012) noted that surface charge has a substantial effect on nanoparticle absorption by cells, suggesting that predictive models that include surface modifications can be developed to improve cellular uptake and reduce cytotoxicity. This insight provides a foundation for using AI to analyze datasets that contain different nanoparticle designs and their biological responses, thereby enhancing the efficiency of drug delivery systems [101]. Expanding on these concepts, Sun et al. (2014) investigated advancements in engineered nanoparticles that are specifically created for cancer therapy. These nanoparticles increase drug solubility and optimize drug targeting and distribution within the body. Through predictive modeling, researchers can evaluate extensive datasets to modify nanoparticle characteristics, ensuring that they effectively target cancer cells while overcoming issues such as drug resistance. This ability for prediction is important for accelerating the development of sophisticated nanoparticle platforms [45].
The design of nanoparticles powered by AI is changing the environment of precision oncology. However, it faces several important challenges and limitations that must be resolved to realize its full potential. A primary challenge lies in the complexity of developing nanoparticles for medical applications. Traditional methods often rely on trial-and-error approaches, which can be both time-consuming and inefficient. Despite advances in AI, optimizing nanoparticle formulations requires numerous input factors, making it difficult to achieve optimal results without the use of high-throughput platforms [102]. This aspect not only obstructs the development process but also complicates the design of nanoparticles that are personalized to meet specific therapeutic needs.
An important limitation involves the acquisition and quality of the data. AI algorithms rely on extensive datasets for training, which are critical for producing accurate predictions concerning the properties and behaviors of nanoparticles. Nonetheless, acquiring high-quality data that accurately represent the different interactions of nanoparticles with biological systems presents a considerable challenge [67]. The lack of complete datasets can limit the effectiveness of AI models. This limitation presents challenges when trying to apply findings to various conditions or types of nanoparticles. Data shortfalls can hinder the creation of strong predictive models, which are essential for progressing personalized medicine [47].
Regulatory challenges pose major barriers to the integration of AI in nanoparticle design. Before new technologies can be used in clinical environments, they must undergo comprehensive assessments to ensure their safety and effectiveness. Nevertheless, regulatory frameworks customized for AI-enhanced nanoparticle applications are insufficiently developed. This lack of clear guidelines can delay the approval process and generate uncertainty for researchers and developers [103]. In addition, scalability is also an important issue; although AI can optimize nanoparticle design, maintaining consistent quality during large-scale production is essential yet often challenging to achieve. Finally, there is a considerable shortage of targeted research on how AI can enhance nanoparticle-mediated drug delivery systems specifically for cancer treatment [66]. Most researchers tend to focus on broader uses of AI in drug discovery instead of its precise function in perfecting nanoparticle formulations. Addressing these various challenges is essential for overcoming the revolutionary possibilities of AI-driven nanoparticle design in clinical applications.
The future of AI-driven nanoparticle design for precision oncology is poised for significant advancements, driven by innovative technologies and methodologies that increase therapeutic efficacy and patient outcomes. A promising area of development is the creation of high-throughput formulation platforms that combine AI with microfluidic synthesis technology [104]. This strategy facilitates the fast optimization of nanoparticle formulations, allowing researchers to swiftly identify effective therapeutic options for clinical use, such as mRNA vaccines, gene therapies, and specialized drug delivery systems [105].
A major area that needs more research is the use of AI for real-time monitoring and adaptive synthesis of nanoparticles. AI-powered sensors can quickly adjust during the nanoparticle production process, ensuring consistent quality and performance. This capability not only enhances the efficiency of the manufacturing process but also increases the reproducibility of nanoparticle characteristics that are critical for clinical effectiveness [106]. Additionally, AI can predict how nanoparticles behave within biological systems. This capability allows for more precise targeting of cancer cells while minimizing unwanted side effects. Such predictive modeling can considerably reduce the time and resources required for experimental validation, thereby accelerating the shift from laboratory research to clinical applications [107].
The integration of AI into nanoparticle design represents an important advancement in precision oncology, transforming our approach to cancer treatment. In this review, the two major objectives of focusing on recent advancements in AI and applying new algorithms for biomarker-specific NP design and performance optimization in targeted drug delivery of precision oncology were met. Consequently, we validate the potential of our diagnostic tool to increase the specificity and efficacy of nanoparticle-based therapies via enhancements provided by AI. This is a significant improvement over historic procedures which generally are not very accurate for cancer chemotherapy. Therefore, the novelty of our work is brought by the fact that we for the first time will systematically discuss the integration of AI and nanoparticle technology for cancer treatment with respect to issues like tumor heterogeneity and drug resistance. Although researchers have suggested that nanoparticles have possibilities in oncology treatment, we affirm how AI can refine the nanoparticles characteristics and manufacturing procedures for promoting improved treatment gains. Second, it has helped us delineate how AI may support environmentally sustainable approaches to nanoparticle design, a topic that has been discussed in the literature sparingly. From these investigations, the following directions for future research can be identified. First, researchers should focus on developing a better understanding of the ability of AI to work together with new bioengineering technologies like CRISPR or gene therapy, to improve the performance and stability of nanoparticles. Moreover, further study should be conducted more on mass production and synthesis of AI designed nanoparticles that is safe for human consumption and meets all the relevant FDA requirements while remaining effective in conditions. Finally, we suggest exploring whether liquid biopsies together with AI-based analysis can provide additional information to fine-tune personal patient management even more.
The authors declare that they have not used artificial intelligence (AI) tools in the creation of this article.
[1] |
Mitchell MJ, Billingsley MM, Haley RM, et al. (2021) Engineering precision nanoparticles for drug delivery. Nat Rev Drug Discov 20: 101-124. https://doi.org/10.1038/s41573-020-0090-8 ![]() |
[2] |
Prabhakar U, Maeda H, Jain RK, et al. (2013) Challenges and key considerations of the enhanced permeability and retention effect for nanomedicine drug delivery in oncology. Cancer Research 73: 2412-2417. https://doi.org/10.1158/0008-5472.CAN-12-4561 ![]() |
[3] |
Zhang Y, Wang X (2020) Targeting the Wnt/β-catenin signaling pathway in cancer. J Hematol Oncol 13: 165. https://doi.org/10.1186/s13045-020-00990-3 ![]() |
[4] |
Malone ER, Oliva M, Sabatini PJB, et al. (2020) Molecular profiling for precision cancer therapies. Genome Med 12: 8. https://doi.org/10.1186/s13073-019-0703-1 ![]() |
[5] |
McGranahan N, Swanton C (2017) Clonal heterogeneity and tumor evolution: past, present, and the future. Cell 168: 613-628. https://doi.org/10.1016/j.cell.2017.01.018 ![]() |
[6] |
Longo DL (2012) Tumor heterogeneity and personalized medicine. N Engl J Med 366: 956-957. https://doi.org/10.1056/NEJMe1200656 ![]() |
[7] |
Adir O, Poley M, Chen G, et al. (2020) Integrating artificial intelligence and nanotechnology for precision cancer medicine. Adv Mater 32: e1901989. https://doi.org/10.1002/adma.201901989 ![]() |
[8] |
Shamay Y, Shah J, Işık M, et al. (2018) Quantitative self-assembly prediction yields targeted nanomedicines. Nat Mater 17: 361-368. https://doi.org/10.1038/s41563-017-0007-z ![]() |
[9] |
Adir O, Poley M, Chen G, et al. (2019) Integrating artificial intelligence and nanotechnology for precision cancer medicine. Adv Mater 32: e1901989. https://doi.org/10.1002/adma.201901989 ![]() |
[10] |
Al-Mohammedawi NA, Zaidan SA, Kashan JS (2024) Bioceramic scaffolds for bone repair and regeneration: a comprehensive review. J Appl Sci Nanotechnol 4: 39-57. https://doi.org/10.53293/jasn.2024.7223.1265 ![]() |
[11] |
Rascio F, Spadaccino F, Rocchetti MT, et al. (2021) The pathogenic role of PI3K/AKT pathway in cancer onset and drug resistance: an updated review. Cancers 13: 3949. https://doi.org/10.3390/cancers13163949 ![]() |
[12] |
Fazio M, Ablain J, Chuan Y, et al. (2020) Zebrafish patient avatars in cancer biology and precision cancer therapy. Nat Rev Cancer 20: 263-273. https://doi.org/10.1038/s41568-020-0252-3 ![]() |
[13] | de Jesus RA, Nascimento VRS, Costa JAS, et al. Empirical modeling, experimental optimization, and artificial intelligence (ANN-GA) as a tool for the efficient dye remediation by the biosilica extracted from sugarcane bagasse ash (2023). https://doi.org/10.1007/s13399-023-04825-2 |
[14] |
Sun W, Chen G, Du F, et al. (2021) Targeted Drug Delivery to Cancer Stem Cells through Nanotechnological Approaches. Curr Stem Cell Re T 16: 367-384. https://doi.org/10.2174/1574888X15999201001204727 ![]() |
[15] |
Lee JK, Liu Z, Sa JK, et al. (2018) Pharmacogenomic landscape of patient-derived tumor cells informs precision oncology therapy. Nat Genet 50: 1399-1411. https://doi.org/10.1038/s41588-018-0209-6 ![]() |
[16] | Sadr S, Hajjafari A, Rahdar A, et al. (2024) Gold nanobiosensors and Machine Learning: Pioneering breakthroughs in precision breast cancer detection. Eur J Med Chem Rep 12: 100238. https://doi.org/10.1016/j.ejmcr.2024.100238 |
[17] |
Fathi-karkan S, Shamsabadipour A, Moradi A, et al. (2024) Four-dimensional printing techniques: a comprehensive review of biomedical and tissue engineering developments. BioNanoSci 14: 4189-4218. https://doi.org/10.1007/s12668-024-01596-6 ![]() |
[18] |
Hristova-Panusheva K, Xenodochidis C, Georgieva M, et al. (2024) Nanoparticle-mediated drug delivery systems for precision targeting in oncology. Pharmaceuticals 17: 677. https://doi.org/10.3390/ph17060677 ![]() |
[19] |
Fu X, Shi Y, Qi T, et al. (2020) Precise design strategies of nanomedicine for improving cancer therapeutic efficacy using subcellular targeting. Sig Transduct Target Ther 5: 1-15. https://doi.org/10.1038/s41392-020-00342-0 ![]() |
[20] |
Yao Y, Zhou Y, Liu L, et al. (2020) Nanoparticle-based drug delivery in cancer therapy and its role in overcoming drug resistance. Front Mol Biosci 7: 193. https://doi.org/10.3389/fmolb.2020.00193 ![]() |
[21] |
Siegel RL, Miller KD, Fuchs HE, et al. (2022) Cancer statistics, 2022. CA Cancer J Clin 72: 7-33. https://doi.org/10.3322/caac.21708 ![]() |
[22] |
Wild CP (2019) The global cancer burden: necessity is the mother of prevention. Nat Rev Cancer 19: 123-124. https://doi.org/10.1038/s41568-019-0110-3 ![]() |
[23] |
Elumalai K, Srinivasan S, Shanmugam A (2024) Review of the efficacy of nanoparticle-based drug delivery systems for cancer treatment. Biomed Technol 5: 109-122. https://doi.org/10.1016/j.bmt.2023.09.001 ![]() |
[24] |
Mundekkad D, Cho WC (2022) Nanoparticles in clinical translation for cancer therapy. Int J Mol Sci 23: 1685. https://doi.org/10.3390/ijms23031685 ![]() |
[25] |
Hu C-MJ, Aryal S, Zhang L (2010) Nanoparticle-assisted combination therapies for effective cancer treatment. Ther Deliv 1: 323-334. https://doi.org/10.4155/tde.10.13 ![]() |
[26] |
Truong NP, Whittaker MR, Mak CW, et al. (2015) The importance of nanoparticle shape in cancer drug delivery. Expert Opin Drug Del 12: 129-142. https://doi.org/10.1517/17425247.2014.950564 ![]() |
[27] |
Markman M (2006) Pegylated liposomal doxorubicin in the treatment of cancers of the breast and ovary. Expert Opin Pharmacother 7: 1469-1474. https://doi.org/10.1517/14656566.7.11.1469 ![]() |
[28] |
Hofheinz R-D, Gnad-Vogt SU, Beyer U, et al. (2005) Liposomal encapsulated anti-cancer drugs. Anticancer Drugs 16: 691-707. https://doi.org/10.1097/01.cad.0000167902.53039.5a ![]() |
[29] |
Malam Y, Loizidou M, Seifalian AM (2009) Liposomes and nanoparticles: nanosized vehicles for drug delivery in cancer. Trends Pharmacol Sci 30: 592-599. https://doi.org/10.1016/j.tips.2009.08.004 ![]() |
[30] |
Gubernator J (2011) Active methods of drug loading into liposomes: recent strategies for stable drug entrapment and increased in vivo activity. Expert Opin Drug Deliv 8: 565-580. https://doi.org/10.1517/17425247.2011.566552 ![]() |
[31] |
Pucci C, Martinelli C, Ciofani G (2019) Innovative approaches for cancer treatment: current perspectives and new challenges. ecancer 13: 961. https://doi.org/10.3332/ecancer.2019.961 ![]() |
[32] |
Karapetis CS, Khambata-Ford S, Jonker DJ, et al. (2008) K-ras mutations and benefit from cetuximab in advanced colorectal cancer. N Engl J Med 359: 1757-1765. https://doi.org/10.1056/NEJMoa0804385 ![]() |
[33] |
Di Nicolantonio F, Martini M, Molinari F, et al. (2008) Wild-type BRAF is required for response to panitumumab or cetuximab in metastatic colorectal cancer. J Clin Oncol 26: 5705-5712. https://doi.org/10.1200/JCO.2008.18.0786 ![]() |
[34] |
Gabizon AA, Patil Y, La-Beck NM (2016) New insights and evolving role of pegylated liposomal doxorubicin in cancer therapy. Drug Resist Update 29: 90-106. https://doi.org/10.1016/j.drup.2016.10.003 ![]() |
[35] | Gupta N, Hatoum H, Dy GK (2014) First line treatment of advanced non-small-cell lung cancer–specific focus on albumin bound paclitaxel. Int J Nanomed 9: 209-221. https://doi.org/10.2147/IJN.S41770 |
[36] |
Passero FC, Grapsa D, Syrigos KN, et al. (2016) The safety and efficacy of Onivyde (irinotecan liposome injection) for the treatment of metastatic pancreatic cancer following gemcitabine-based therapy. Expert Rev Anticanc 16: 697-703. https://doi.org/10.1080/14737140.2016.1192471 ![]() |
[37] |
Kim M, Williams S (2018) Daunorubicin and cytarabine liposome in newly diagnosed therapy-related acute myeloid leukemia (AML) or AML with myelodysplasia-related changes. Ann Pharmacother 52: 792-800. https://doi.org/10.1177/1060028018764923 ![]() |
[38] | Raja A, Kasana A, Verma V, et al. Next-generation therapeutic antibodies for cancer treatment: advancements, applications, and challenges (2024). https://doi.org/10.1007/s12033-024-01270-y |
[39] |
Sheykhhasan M, Ahmadieh-Yazdi A, Vicidomini R, et al. (2024) CAR T therapies in multiple myeloma: unleashing the future. Cancer Gene Ther 31: 667-686. https://doi.org/10.1038/s41417-024-00750-2 ![]() |
[40] |
Harrison TS, Lyseng-Williamson KA (2013) Vincristine sulfate liposome injection. BioDrugs 27: 69-74. https://doi.org/10.1007/s40259-012-0002-5 ![]() |
[41] |
Yang L, Fang H, Jiang J, et al. (2022) EGFR-targeted pemetrexed therapy of malignant pleural mesothelioma. Drug Deliv Transl Res 12: 2527-2536. https://doi.org/10.1007/s13346-021-01094-2 ![]() |
[42] | Raskova Kafkova L, Mierzwicka JM, Chakraborty P, et al. NSCLC: from tumorigenesis, immune checkpoint misuse to current and future targeted therapy (2024). https://doi.org/10.3389/fimmu.2024.1342086 |
[43] |
Sanchez C, Belleville P, Popall M, et al. (2011) Applications of advanced hybrid organic-inorganic nanomaterials: from laboratory to market. Chem Soc Rev 40: 696-753. https://doi.org/10.1039/c0cs00136h ![]() |
[44] |
Gao J, Chen K, Miao Z, et al. (2010) Affibody-based nanoprobes for HER2-expressing cell and tumor imaging. Biomaterials 32: 2141. https://doi.org/10.1016/j.biomaterials.2010.11.053 ![]() |
[45] |
Sun T, Zhang YS, Pang B, et al. (2014) Engineered nanoparticles for drug delivery in cancer therapy. Angew Chem Int Ed Engl 53: 12320-12364. https://doi.org/10.1002/anie.201403036 ![]() |
[46] |
Kim EM, Jeong HJ (2017) Current status and future direction of nanomedicine: focus on advanced biological and medical applications. Nucl Med Mol Imaging 51: 106-117. https://doi.org/10.1007/s13139-016-0435-8 ![]() |
[47] |
Vijaya Lakshmi K (2023) Artificial intelligence and its applications in nanochemistry. IJETMS 7: 385-389. https://doi.org/10.46647/ijetms.2023.v07i05.046 ![]() |
[48] |
Zhu X, Li Y, Gu N (2023) Application of artificial intelligence in the exploration and optimization of biomedical nanomaterials. Nano Biomed Eng 15: 342-353. https://doi.org/10.26599/NBE.2023.9290035 ![]() |
[49] |
Serrano DR, Luciano FC, Anaya BJ, et al. (2024) Artificial intelligence (AI) applications in drug discovery and drug delivery: revolutionizing personalized medicine. Pharmaceutics 16: 1328. https://doi.org/10.3390/pharmaceutics16101328 ![]() |
[50] |
Chan EM, Xu C, Mao AW, et al. (2010) Reproducible, high-throughput synthesis of colloidal nanocrystals for optimization in multidimensional parameter space. Nano Lett 10: 1874-1885. https://doi.org/10.1021/nl100669s ![]() |
[51] |
Kajita S, Ohba N, Suzumura A, et al. (2020) Discovery of superionic conductors by ensemble-scope descriptor. NPG Asia Mater 12: 1-8. https://doi.org/10.1038/s41427-020-0211-1 ![]() |
[52] |
Yamankurt G, Berns EJ, Xue A, et al. (2019) Exploration of the nanomedicine-design space with high-throughput screening and machine learning. Nat Biomed Eng 3: 318-327. https://doi.org/10.1038/s41551-019-0351-1 ![]() |
[53] |
Yuan M, Kermanian M, Agarwal T, et al. (2023) Defect engineering in biomedical sciences. Adv Mater 35: 2304176. https://doi.org/10.1002/adma.202304176 ![]() |
[54] |
Wu Y, Duan H, Xi H (2020) Machine learning-driven insights into defects of zirconium metal–organic frameworks for enhanced ethane–ethylene separation. Chem Mater 32: 2986-2997. https://doi.org/10.1021/acs.chemmater.9b05322 ![]() |
[55] |
Li S, Barnard AS (2022) Inverse design of nanoparticles using multi-target machine learning. Adv Theor Simul 5: 2100414. https://doi.org/10.1002/adts.202100414 ![]() |
[56] |
Thomas DG, Chikkagoudar S, Heredia-Langner A, et al. (2014) Physicochemical signatures of nanoparticle-dependent complement activation. Comput Sci Discov 7: 015003. https://doi.org/10.1088/1749-4699/7/1/015003 ![]() |
[57] |
Boso DP, Lee S-Y, Ferrari M, et al. (2011) Optimizing particle size for targeting diseased microvasculature: from experiments to artificial neural networks. IJN 6: 1517-1526. https://doi.org/10.2147/IJN.S20283 ![]() |
[58] |
Galasso I, Erikainen S, Pickersgill M, et al. (2024) “Different names for the same thing”? novelty, expectations, and performative nominalism in personalized and precision medicine. Soc Theory Health 22: 139-155. https://doi.org/10.1057/s41285-024-00203-8 ![]() |
[59] |
Schleidgen S, Klingler C, Bertram T, et al. (2013) What is personalized medicine: sharpening a vague term based on a systematic literature review. BMC Med Ethics 14: 55. https://doi.org/10.1186/1472-6939-14-55 ![]() |
[60] |
Delpierre C, Lefèvre T (2023) Precision and personalized medicine: What their current definition says and silences about the model of health they promote. Implication for the development of personalized health. Front Sociol 8: 1112159. https://doi.org/10.3389/fsoc.2023.1112159 ![]() |
[61] |
Das KP, J C (2023) Nanoparticles and convergence of artificial intelligence for targeted drug delivery for cancer therapy: current progress and challenges. Front Med Technol 4: 1067144. https://doi.org/10.3389/fmedt.2022.1067144 ![]() |
[62] |
Wang J, Liu G, Zhou C, et al. (2024) Application of artificial intelligence in cancer diagnosis and tumor nanomedicine. Nanoscale 16: 14213-14246. https://doi.org/10.1039/D4NR01832J ![]() |
[63] |
Majumder J, Taratula O, Minko T (2019) Nanocarrier-based systems for targeted and site specific therapeutic delivery. Adv Drug Deliver Rev 144: 57. https://doi.org/10.1016/j.addr.2019.07.010 ![]() |
[64] |
Yook S, Cai Z, Lu Y, et al. (2015) Radiation nanomedicine for EGFR-positive breast cancer: panitumumab-modified gold nanoparticles complexed to the β-particle-emitter, (177)Lu. Mol Pharm 12: 3963-3972. https://doi.org/10.1021/acs.molpharmaceut.5b00425 ![]() |
[65] |
Sharkey RM, Goldenberg DM (2006) Targeted therapy of cancer: new prospects for antibodies and immunoconjugates. CA Cancer J Clin 56: 226-243. https://doi.org/10.3322/canjclin.56.4.226 ![]() |
[66] |
Adir O, Poley M, Chen G, et al. (2019) Integrating artificial intelligence and nanotechnology for precision cancer medicine. Adv Mater 32: e1901989. https://doi.org/10.1002/adma.201901989 ![]() |
[67] | Ori MO, Ekpan FM, Samuel HS, et al. (2024) Integration of artificial intelligence in nanomedicine. Eurasian J Sci Technol 4: 88-104. https://doi.org/10.48309/EJST.2024.422419.1105 |
[68] |
Medhi B, Sharma DH, Kaundal DT, et al. (2024) Artificial intelligence: a catalyst for breakthroughs in nanotechnology and pharmaceutical research. IJPSN 17: 7439-7445. https://doi.org/10.37285/ijpsn.2024.17.4.1 ![]() |
[69] |
Gawel AM, Betkowska A, Gajda E, et al. (2024) Current non-metal nanoparticle-based therapeutic approaches for glioblastoma treatment. Biomedicines 12: 1822. https://doi.org/10.3390/biomedicines12081822 ![]() |
[70] |
Qi G, Shi G, Wang S, et al. (2023) A novel pH-responsive Iron oxide core-shell magnetic mesoporous Silica nanoparticle (M-MSN) system encapsulating doxorubicin (DOX) and glucose oxidase (Gox) for pancreatic cancer treatment. Int J Nanomed 18: 7133-7147. https://doi.org/10.2147/IJN.S436253 ![]() |
[71] |
Choi KY, Liu G, Lee S, et al. (2012) Theranostic nanoplatforms for simultaneous cancer imaging and therapy: current approaches and future perspectives. Nanoscale 4: 330-342. https://doi.org/10.1039/C1NR11277E ![]() |
[72] |
Ristau BT, O'Keefe DS, Bacich DJ (2014) The prostate-specific membrane antigen: lessons and current clinical implications from 20 years of research. Urol Oncol 32: 272-279. https://doi.org/10.1016/j.urolonc.2013.09.003 ![]() |
[73] | Ciccarese C, Bauckneht M, Zagaria L, et al. (2024) Defining the position of [177Lu] Lu-PSMA radioligand therapy in the treatment landscape of metastatic castration-resistant prostate cancer: a meta-analysis of clinical trials. Targ Oncol 2024: 1-10. https://doi.org/10.1007/s11523-024-01117-1 |
[74] |
Von Hoff DD, Mita MM, Ramanathan RK, et al. (2016) Phase I study of PSMA-targeted docetaxel-containing nanoparticle BIND-014 in patients with advanced solid tumors. Clin Cancer Res 22: 3157-3163. https://doi.org/10.1158/1078-0432.CCR-15-2548 ![]() |
[75] |
Ni J, Miao T, Su M, et al. (2021) PSMA-targeted nanoparticles for specific penetration of blood-brain tumor barrier and combined therapy of brain metastases. J Control Release 329: 934-947. https://doi.org/10.1016/j.jconrel.2020.10.023 ![]() |
[76] |
Autio KA, Garcia JA, Alva AS, et al. (2016) A phase 2 study of BIND-014 (PSMA-targeted docetaxel nanoparticle) administered to patients with chemotherapy-naïve metastatic castration-resistant prostate cancer (mCRPC). JCO 34: 233-233. https://doi.org/10.1200/jco.2016.34.2_suppl.233 ![]() |
[77] | Bakht MK, Beltran H (2024) Biological determinants of PSMA expression, regulation and heterogeneity in prostate cancer. Nat Rev Urol 2024: 1-20. https://doi.org/10.1038/s41585-024-00900-z |
[78] |
Zahednezhad F, Allahyari S, Sarfraz M, et al. (2024) Liposomal drug delivery systems for organ-specific cancer targeting: early promises, subsequent problems, and recent breakthroughs. Expert Opinion Drug Del 21: 1363-1384. https://doi.org/10.1080/17425247.2024.2394611 ![]() |
[79] |
Rizwanullah M, Ahmad MZ, Ghoneim MM, et al. (2021) Receptor-mediated targeted delivery of surface-modifiednanomedicine in breast cancer: recent update and challenges. Pharmaceutics 13: 2039. https://doi.org/10.3390/pharmaceutics13122039 ![]() |
[80] |
Guddo F, Giatromanolaki A, Koukourakis MI, et al. (1998) MUC1 (episialin) expression in non-small cell lung cancer is independent of EGFR and c-erbB-2 expression and correlates with poor survival in node positive patients. J Clin Pathol 51: 667-671. https://doi.org/10.1136/jcp.51.9.667 ![]() |
[81] |
Wurz GT, Kao CJ, Wolf M, et al. (2014) Tecemotide: an antigen-specific cancer immunotherapy. Hum Vacc Immunother 10: 3383-3393. https://doi.org/10.4161/hv.29836 ![]() |
[82] |
Cats A, Jansen EPM, Grieken NCT van, et al. (2018) Chemotherapy versus chemoradiotherapy after surgery and preoperative chemotherapy for resectable gastric cancer (CRITICS): an international, open-label, randomised phase 3 trial. Lancet Oncol 19: 616-628. https://doi.org/10.1016/S1470-2045(18)30132-3 ![]() |
[83] |
Sabir F, Qindeel M, Zeeshan M, et al. (2021) Onco-receptors targeting in lung cancer via application of surface-modified and hybrid nanoparticles: a cross-disciplinary review. Processes 9: 621. https://doi.org/10.3390/pr9040621 ![]() |
[84] |
Hoshyar N, Gray S, Han H, et al. (2016) The effect of nanoparticle size on In Vivo pharmacokinetics and cellular interaction. Nanomedicine (Lond) 11: 673-692. https://doi.org/10.2217/nnm.16.5 ![]() |
[85] |
Zrazhevskiy P, Sena M, Gao X (2010) Designing multifunctional quantum dots for bioimaging, detection, and drug delivery. Chem Soc Rev 39: 4326. https://doi.org/10.1039/b915139g ![]() |
[86] |
Singh P, Pandit S, Mokkapati VRSS, et al. (2018) Gold nanoparticles in diagnostics and therapeutics for human cancer. IJMS 19: 1979. https://doi.org/10.3390/ijms19071979 ![]() |
[87] |
Kim D, Jeong YY, Jon S (2010) A drug-loaded aptamer–gold nanoparticle bioconjugate for combined CT imaging and therapy of prostate cancer. ACS Nano 4: 3689-3696. https://doi.org/10.1021/nn901877h ![]() |
[88] |
Wilson K, Homan K, Emelianov S (2012) Biomedical photoacoustics beyond thermal expansion using triggered nanodroplet vaporization for contrast-enhanced imaging. Nat Commun 3: 618. https://doi.org/10.1038/ncomms1627 ![]() |
[89] |
Dias R, Torkamani A (2019) Artificial intelligence in clinical and genomic diagnostics. Genome Med 11: 70. https://doi.org/10.1186/s13073-019-0689-8 ![]() |
[90] |
Aung KL, Fischer SE, Denroche RE, et al. (2018) Genomics-driven precision medicine for advanced pancreatic cancer: early results from the COMPASS trial. Clin Cancer Res 24: 1344-1354. https://doi.org/10.1158/1078-0432.CCR-17-2994 ![]() |
[91] |
Rao W, Wang H, Han J, et al. (2015) Chitosan-decorated doxorubicin-encapsulated nanoparticle targets and eliminates tumor reinitiating cancer stem-like cells. ACS Nano 9: 5725-5740. https://doi.org/10.1021/nn506928p ![]() |
[92] |
Senapati S, Mahanta AK, Kumar S, et al. (2018) Controlled drug delivery vehicles for cancer treatment and their performance. Sig Transduct Target Ther 3: 7. https://doi.org/10.1038/s41392-017-0004-3 ![]() |
[93] |
Xu Y, Hosny A, Zeleznik R, et al. (2019) Deep learning predicts lung cancer treatment response from serial medical imaging. Clin Cancer Res 25: 3266-3275. https://doi.org/10.1158/1078-0432.CCR-18-2495 ![]() |
[94] | Sun Z, Song C, Wang C, et al. (2020) Hydrogel-based controlled drug delivery for cancer treatment: a review. Mol Pharmaceutics 17: 373-391. https://doi.org/10.1021/acs.molpharmaceut.9b01020 |
[95] |
Sykes EA, Dai Q, Sarsons CD, et al. (2016) Tailoring nanoparticle designs to target cancer based on tumor pathophysiology. Proc Natl Acad Sci USA 113: E1142-E1151. https://doi.org/10.1073/pnas.1521265113 ![]() |
[96] |
Bhinder B, Gilvary C, Madhukar NS, et al. (2021) Artificial intelligence in cancer research and precision medicine. Cancer Discov 11: 900-915. https://doi.org/10.1158/2159-8290.CD-21-0090 ![]() |
[97] |
Zhang Y, Lin R, Li H, et al. (2019) Strategies to improve tumor penetration of nanomedicines through nanoparticle design. WIREs Nanomed Nanobiotechnol 11: e1519. https://doi.org/10.1002/wnan.1519 ![]() |
[98] |
Keith JA, Vassilev-Galindo V, Cheng B, et al. (2021) Combining machine learning and computational chemistry for predictive insights into chemical systems. Chem Rev 121: 9816-9872. https://doi.org/10.1021/acs.chemrev.1c00107 ![]() |
[99] |
Kennedy LC, Bickford LR, Lewinski NA, et al. (2011) A new era for cancer treatment: gold-nanoparticle-mediated thermal therapies. Small 7: 169-183. https://doi.org/10.1002/smll.201000134 ![]() |
[100] |
Merino S, Martín C, Kostarelos K, et al. (2015) Nanocomposite hydrogels: 3D polymer–nanoparticle synergies for on-demand drug delivery. ACS Nano 9: 4686-4697. https://doi.org/10.1021/acsnano.5b01433 ![]() |
[101] |
Fröhlich E (2012) The role of surface charge in cellular uptake and cytotoxicity of medical nanoparticles. IJN 2012: 5577-5591. https://doi.org/10.2147/IJN.S36111 ![]() |
[102] | AI FORM: An AI-Driven Platform for Acceleration of Nanomedicine Development Science Foundation Ireland. Available from: https://www.sfi.ie/challenges/future-digital/ai-form/ |
[103] | The Power of AI-Generated Nanoparticles in Targeted Drug DeliveryNano Magazine - Latest Nanotechnology News (2023). Available from: https://nano-magazine.com/news/2023/7/3/the-power-of-ai-generated-nanoparticles-in-targeted-drug-delivery |
[104] |
Greenberg ZF, Graim KS, He M (2023) Towards artificial intelligence-enabled extracellular vesicle precision drug delivery. Adv Drug Deliver Rev 199: 114974. https://doi.org/10.1016/j.addr.2023.114974 ![]() |
[105] | Weerarathna IN, Kamble AR, Luharia A (2023) Artificial intelligence applications for biomedical cancer research: a review. Cureus 15: e48307. https://doi.org/10.7759/cureus.48307 |
[106] |
Chugh V, Basu A, Kaushik A, et al. (2024) Employing nano-enabled artificial intelligence (AI)-based smart technologies for prediction, screening, and detection of cancer. Nanoscale 16: 5458-5486. https://doi.org/10.1039/D3NR05648A ![]() |
[107] |
Liu J, Du H, Huang L, et al. (2024) AI-powered microfluidics: shaping the future of phenotypic drug discovery. ACS Appl Mater Interfaces 16: 38832-38851. https://doi.org/10.1021/acsami.4c07665 ![]() |
Drug Name | Type of Nanoparticle | Active Drug | Type of Cancer Targeted | Year of Approval | Ref |
Doxil | Liposome | Doxorubicin | Breast cancer, ovarian cancer | 2010 | [34] |
Abraxane | Albumin-bound nanoparticle | Paclitaxel | Breast cancer, non-small cell lung cancer | 2012 | [35] |
Onivyde | Liposome | Irinotecan | Pancreatic cancer | 2015 | [36] |
Vyxeos | Liposome | Cytarabine and Daunorubicin | Acute myeloid leukemia | 2017 | [37] |
Epcoritamab | Nanobody | Monoclonal antibody | B-cell malignancies | 2022 | [38] |
Carvykti | CAR-T-cell therapy | Abecma | Multiple myeloma | 2021 | [39] |
Marqibo | Liposome | Vincristine | Acute lymphoblastic leukemia | 2018 | [40] |
Pemetrexed | Polymeric nanoparticle | Pemetrexed | Mesothelioma, non-small cell lung cancer | 2020 | [41] |
Libtayo | Nanobody | Cemiplimab | Cutaneous squamous cell carcinoma | 2022 | [42] |
Nanoparticle Name | Ligand Type | Targeted Cancer Type | AI Application | Clinical Trial Phase | Ref |
Doxil | Antibody | Breast Cancer | AI algorithms optimize formulation and dosing | Phase III | [20] |
Abraxane | Albumin | Non-Small Cell Lung Cancer | Machine learning predicts patient response | Phase II | [66] |
ONPATTRO | siRNA | Amyloidosis | AI enhances delivery efficiency | Phase III | [67] |
Docetaxel-Loaded NPs | Small Molecule | Prostate Cancer | AI models optimize nanoparticle design | Phase II | [20] |
HER2-Targeted NPs | Monoclonal Antibody | Gastric Cancer | AI assists in biomarker profiling | Phase II | [61] |
RNAi Nanoparticles | Aptamer | Ovarian Cancer | AI predicts interactions with cancer biomarkers | Phase I | [68] |
Paclitaxel-Loaded NPs | Peptide | Breast and Lung Cancer | AI optimizes drug release profiles | Phase II | [66] |
Hybrid Liposome-NPs | Combination of Ligands | Various Cancers | AI aids in hybrid nanoparticle stability | Phase I | [69] |
Silica-Core NPs | Small Molecule | Pancreatic Cancer | AI improves targeting accuracy | Phase II | [70] |
Theranostic NPs | Diagnostic Ligand | Multiple Cancer Types | AI integrates imaging and therapeutic functions | Phase III | [71] |
Drug Name | Type of Nanoparticle | Active Drug | Type of Cancer Targeted | Year of Approval | Ref |
Doxil | Liposome | Doxorubicin | Breast cancer, ovarian cancer | 2010 | [34] |
Abraxane | Albumin-bound nanoparticle | Paclitaxel | Breast cancer, non-small cell lung cancer | 2012 | [35] |
Onivyde | Liposome | Irinotecan | Pancreatic cancer | 2015 | [36] |
Vyxeos | Liposome | Cytarabine and Daunorubicin | Acute myeloid leukemia | 2017 | [37] |
Epcoritamab | Nanobody | Monoclonal antibody | B-cell malignancies | 2022 | [38] |
Carvykti | CAR-T-cell therapy | Abecma | Multiple myeloma | 2021 | [39] |
Marqibo | Liposome | Vincristine | Acute lymphoblastic leukemia | 2018 | [40] |
Pemetrexed | Polymeric nanoparticle | Pemetrexed | Mesothelioma, non-small cell lung cancer | 2020 | [41] |
Libtayo | Nanobody | Cemiplimab | Cutaneous squamous cell carcinoma | 2022 | [42] |
Nanoparticle Name | Ligand Type | Targeted Cancer Type | AI Application | Clinical Trial Phase | Ref |
Doxil | Antibody | Breast Cancer | AI algorithms optimize formulation and dosing | Phase III | [20] |
Abraxane | Albumin | Non-Small Cell Lung Cancer | Machine learning predicts patient response | Phase II | [66] |
ONPATTRO | siRNA | Amyloidosis | AI enhances delivery efficiency | Phase III | [67] |
Docetaxel-Loaded NPs | Small Molecule | Prostate Cancer | AI models optimize nanoparticle design | Phase II | [20] |
HER2-Targeted NPs | Monoclonal Antibody | Gastric Cancer | AI assists in biomarker profiling | Phase II | [61] |
RNAi Nanoparticles | Aptamer | Ovarian Cancer | AI predicts interactions with cancer biomarkers | Phase I | [68] |
Paclitaxel-Loaded NPs | Peptide | Breast and Lung Cancer | AI optimizes drug release profiles | Phase II | [66] |
Hybrid Liposome-NPs | Combination of Ligands | Various Cancers | AI aids in hybrid nanoparticle stability | Phase I | [69] |
Silica-Core NPs | Small Molecule | Pancreatic Cancer | AI improves targeting accuracy | Phase II | [70] |
Theranostic NPs | Diagnostic Ligand | Multiple Cancer Types | AI integrates imaging and therapeutic functions | Phase III | [71] |