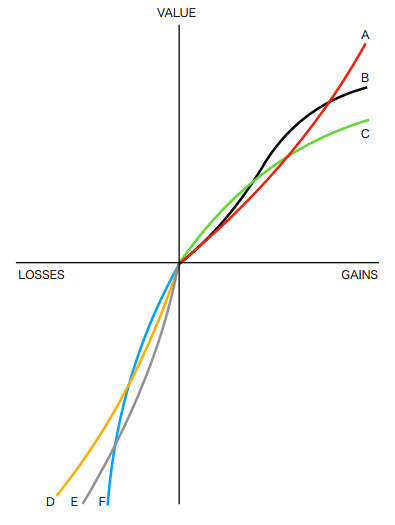
In prospect theory, the value function is typically concave for gains and convex for losses, with losses usually having a steeper slope than gains. The neural system responds differently to losses and gains. Five new studies on neurons related to this issue have examined neuronal responses to losses, gains, and reference points. This study investigated a new concept of the value function. A value function with a neuronal cusp may exhibit variations and behavioral cusps associated with catastrophic events, potentially influencing a trader's decision to close a position. Additionally, we have conducted empirical studies on algorithmic trading strategies that employ different value function specifications.
Citation: Kazuo Sano. New concept for the value function of prospect theory[J]. Quantitative Finance and Economics, 2024, 8(4): 733-756. doi: 10.3934/QFE.2024028
[1] | Abderahman Rejeb, Karim Rejeb, John G. Keogh . Centralized vs. decentralized ledgers in the money supply process: a SWOT analysis. Quantitative Finance and Economics, 2021, 5(1): 40-66. doi: 10.3934/QFE.2021003 |
[2] | Fangzhou Huang, Jiao Song, Nick J. Taylor . The impact of business conditions and commodity market on US stock returns: An asset pricing modelling experiment. Quantitative Finance and Economics, 2022, 6(3): 433-458. doi: 10.3934/QFE.2022019 |
[3] | Salvador Cruz Rambaud, Piedad Ortiz Fernández, Javier Sánchez García, Paula Ortega Perals . A proposal of concentration measures for discount functions. Quantitative Finance and Economics, 2024, 8(2): 347-363. doi: 10.3934/QFE.2024013 |
[4] | Tânia Menezes Montenegro, Pedro Meira, Sónia Silva . The investors' prospects on mandatory auditor rotation: evidence from Euronext Lisbon. Quantitative Finance and Economics, 2023, 7(3): 440-462. doi: 10.3934/QFE.2023022 |
[5] | Andrea Ferrario, Massimo Guidolin, Manuela Pedio . Comparing in- and out-of-sample approaches to variance decomposition-based estimates of network connectedness an application to the Italian banking system. Quantitative Finance and Economics, 2018, 2(3): 661-701. doi: 10.3934/QFE.2018.3.661 |
[6] | Longfei Wei, Lu Liu, Jialong Hou . Pricing hybrid-triggered catastrophe bonds based on copula-EVT model. Quantitative Finance and Economics, 2022, 6(2): 223-243. doi: 10.3934/QFE.2022010 |
[7] | Gabriel Frahm . How often is the financial market going to collapse?. Quantitative Finance and Economics, 2018, 2(3): 590-614. doi: 10.3934/QFE.2018.3.590 |
[8] | Nereida Polovina, Ken Peasnell . The effects of different modes of foreign bank entry in the Turkish banking sector during the 2007–2009 Global financial crisis. Quantitative Finance and Economics, 2023, 7(1): 19-49. doi: 10.3934/QFE.2023002 |
[9] | Oleg S. Sukharev . The restructuring of the investment portfolio: the risk and effect of the emergence of new combinations. Quantitative Finance and Economics, 2019, 3(2): 390-411. doi: 10.3934/QFE.2019.2.390 |
[10] | Vladimir Donskoy . BOMD: Building Optimization Models from Data (Neural Networks based Approach). Quantitative Finance and Economics, 2019, 3(4): 608-623. doi: 10.3934/QFE.2019.4.608 |
In prospect theory, the value function is typically concave for gains and convex for losses, with losses usually having a steeper slope than gains. The neural system responds differently to losses and gains. Five new studies on neurons related to this issue have examined neuronal responses to losses, gains, and reference points. This study investigated a new concept of the value function. A value function with a neuronal cusp may exhibit variations and behavioral cusps associated with catastrophic events, potentially influencing a trader's decision to close a position. Additionally, we have conducted empirical studies on algorithmic trading strategies that employ different value function specifications.
Prospect theory (Tversky and Kahneman (1974); Kahneman and Tversky (1979); Tversky and Kahneman (1992)) is one of the most influential theories of human decision-making (Thaler (1989); Camerer (2004); Ariely et al. (2005); Liberman et al. (2005); Lejarraga and Hertwig (2017); Harinck et al. (2007); Mogiliansky et al. (2009); MacGraw et al. (2010); Goyal et al. (2021); Walther and Münster (2021); Linzmajer et al. (2021); Mengov et al. (1982); Blavatskyy (2021)). According to this theory, individuals assess their losses and gains asymmetrically. Experimental studies have shown that the key behavioral assumption of expected utility theory, the so-called "independence axiom, " is systematically violated in practice (Neumann and Morgenstern (1947)). The value function is typically concave for gains and convex for losses, with losses typically having a steeper slope than gains. Decision weights are often lower than the corresponding probabilities, except in the low-probability range. Given these findings, the empirical relevance of numerous studies on the behavior of economic agents under uncertainty that utilize expected utility analysis has been debated.
In economics, however, it is assumed that economic agents are typically rational (Stigler and Becker (1977)). Economists have long debated the issue of time preference (Strotz (1955); Thaler and Shefrin (1981); Thaler (1981); Weitzman (2001); Nowaihi and Dhami (2006); Haigh and List (2005); Graham and Snower (2013); Andreoni et al. (2015); Larson et al. (2016); Cohen et al. (2020)), as well as the validity of prospect theory. Machina (1982) showed that the basic concepts, tools, and results of his generalized expected utility analysis are independent of the independence axiom but may be derived from a much weaker assumption of smoothness of preferences over alternative probability distributions. He also demonstrated that a simple model of preferences could be constructed to associate a wide body of observed behavior with risk, including the Allais paradox, may be constructed. Thus, the hypothesis that an individual maximizes a fixed preference functional defined over probability distributions may hold true for many apparently independent aspects of risk behavior. However, this may reflect only a part of human innate nature and may represent a mathematical abstraction of human rationality in economic phenomena.
Meyer and Pagel (2022) explored the impact of stock price fluctuations on individual investor behavior, drawing on past literature and debates. Standard economic theory suggests that individuals should respond rationally to changes in stock value, but behavioral economics highlights how emotional reactions can influence investment decisions. They analyzed reinvestment behavior following forced liquidations, demonstrating that losses significantly decrease the likelihood of reinvestment. This finding provides empirical support for these theories and contributes to understanding how past investment experiences shape future financial behaviors. The study employs mutual fund liquidations as exogenous shocks to analyze responses to realized gains and losses. They demonstrate that individuals are less likely to reinvest after experiencing losses, indicating a shift in preferences and increased risk aversion. This research enhances our understanding of how past experiences with investments shape future financial behaviors and risk tolerance, ultimately providing insights into broader household finance phenomena, such as stock market non-participation.
If the expected utility theory does not hold for investor's decisions, does the neural system affect the investor trading decisions? Does the neural system respond differently to gains and losses? This study presents hypotheses to address these issues. In general, we examine the outcome of our choice and adjust subsequent choice behavior using the outcome information to choose an appropriate action. Five significant studies on neurons (Kawai et al. (2015); Yamada et al. (2021); Imaizumi et al. (2022); Yang et al. (2022); Ferrari-Toniolo and Schultz (2023)) have examined neuronal responses to loss and gain. These studies suggest that two different neural systems may respond to loss and gain, resulting in a value function with a cusp as a reference point.
This study addresses the reference point and cusps of value functions, and the following questions. When does a trader exit one's position? Why would a trader ever close a position when losses and gains are continuously changing? The reference point is a neuronal cusp, and also, the value function could have other behavior cusps (Rosales-Ruiz and Baer (1997); Bosch and Fuqua (2001); Bosch and Hixson (2004); Smith et al. (2006)) with catastrophe (Whitney (1955); Zeeman (1976)).
This article provides a comprehensive review and synthesis of existing literature on the topic of the neural basis of the value function and decision-making. The article focuses on prospect theory and the neural systems involved in evaluating gains and losses relative to a reference point. Five recent studies that have examined neuronal responses to gains, losses, and reference points are reviewed, and their findings are integrated into a broader framework of decision-making.
The article provides a detailed overview of the neural systems involved in decision-making, including the anterior cingulate cortex (ACC), lateral habenula (LHb), orbitofrontal cortex (OFC), dorsal striatum (DS), ventral striatum (VS), and anterior insular cortex (AIC). It also discusses the role of these regions in the valuation of gains and losses, as well as the effects of reference points and the shape of the value function.
In addition, the article introduces a novel concept of the value function, marked by a neuronal cusp. This concept suggests a potential link between catastrophic behavior and position closure in volatile market conditions. The article includes original data analysis using TOPIX tick data and highlights the need for further research to fully elucidate the implications of this concept for decision-making and financial risk management. We will conduct empirical studies on algorithmic trading strategies that employ different value function specifications.
Recent attention has focused on the critical roles of both the lateral habenula (LHb) and the anterior cingulate cortex (ACC) in monitoring negative outcomes. Kawai et al. (2015) investigated how LHb and ACC contribute to behavioral adjustments using single-unit activity recordings from these areas in monkeys performing a reversal learning task. In this task, monkeys had to switch their choice if their previous choice consistently led to no reward in prior attempts. The researchers found that ACC neurons stored outcome information accumulated across multiple trials, whereas LHb neurons quickly detected ongoing negative outcomes. Only ACC neurons, not LHb neurons, signaled a change in behavior in the subsequent trial. Despite both LHb and ACC signaling negative outcomes, the study indicated that these brain regions influence behavioral adjustments in distinct manners.
Additionally, the researchers observed that ACC neurons associated with negative outcomes consistently integrated the impact of these experiences on their responses to no-reward situations. This integration corresponded to the monkeys' shift from their initial choice to an alternative. They further tested whether neuronal activity reflecting negative outcome experiences predicted behavioral shifts, finding consistency with the loss side of the value function (refer to Figure 4 of Kawai et al. (2015)). From a loss perspective, rapid detection of harm or danger is deemed more adaptive for survival than heightened sensitivity to stimuli.
Although they studied monkeys and the same experiments cannot be repeated on humans, earlier studies have shown strong similarities. Such similarities are seen in human event-related potentials (ERP) and functional magnetic resonance imaging (fMRI) studies (see the comprehensive review by Pammi and Miyapuram (2012)). As Kawai et al. (2015) stated, the ACC is crucial for monitoring and adjustment. Studies using human event-related potentials and functional magnetic resonance imaging (fMRI) have shown that the ACC is activated when subjects receive negative feedback after an inappropriate behavioral response. However, the crucial role played by the LHb in monitoring negative outcomes has evoked interest. As the ACC sends projections directly to the LHb, both structures can communicate through their reciprocal relationship. Therefore, the LHb and ACC may work together to monitor the negative outcome and alter subsequent choice behavior.
Prior to these recent studies, it was already known that the ventromedial prefrontal cortex (vmPFC) and amygdala are involved in losses. The vmPFC plays a crucial role in encoding value representations and decision-making processes. It integrates information about potential losses, influencing how individuals evaluate different options. Its involvement in assessing the emotional significance of outcomes makes it essential for understanding loss-related behavior (Bechara et al. (1994); Padoa-Schioppa and Assad (2006)). The amygdala, on the other hand, is key in processing emotional responses, particularly fear and anxiety associated with losses. It contributes to the urgency and salience of loss experiences, which can significantly impact decision-making and risk assessment (LeDoux (2000); Phelps and LeDoux (2005)).
This can be understood by imagining how humans ceased living in trees and transitioned to bipedal walking. We have chosen survival strategies that enhance the brain's development. Frogs, unlike monkeys and humans, adopted a different evolutionary strategy and aimed to improve by increasing the size of their brains. However, this approach proved unsuccessful, leading them to change their survival strategy by camouflaging, thus saving energy by reducing the size of their brains (Liao et al. (2022)).
In their studies on brain reward circuitry, Yamada et al. (2021) and Imaizumi et al. (2022) proposed a neuronal prospect theory model. Using theoretical accuracy equivalent to that of human neuroimaging studies from a gain perspective, they showed that single-neuron activity in four core reward-related cortical and subcortical regions represents a subjective assessment of risky gambles in monkeys. Their studies focused on the central part of the orbitofrontal cortex (cOFC, area 13 M), the medial part of the OFC (mOFC, area 14 O), dorsal striatum (DS, the caudate nucleus), and ventral striatum (VS). Similar to the prospect theory framework, the attractiveness of probabilistic rewards parameterized as a product of utility and probability weighting functions is represented by the activity pattern of single neurons in monkeys passively viewing a lottery. Their study showed that the varied patterns of valuation signals were distributed in most regions of the reward circuitry and not localized. By combining these data, a network model was built to reconstruct the animals' risk preferences and subjective probability weighting as demonstrated by their choices. Distributed neural coding thus explains how subjective valuations under risk are computed. A logistic function was used as the value function. However, the logistic function lacks a reference point, which is obvious because the researchers only focused on neuronal responses on the gain side.
Their findings about the characteristics of the gain side of the value function may be reliable. In contrast to the logistic function of the loss side, that of the gain side may seem appropriate, considering the threshold of response in the region that evokes a response to a stimulus. It is well known that fish can reduce predation risk through learning. Wallerius et al. (2020) showed that fish can acquire social information about a threat not only through privately acquired learned experiences but also by observing the conspecifics' response to threat and using such open information to alter future behavior through learning. This learning from the school of fish also applies to investor groups in the securities market. If you are a smart investor like Buffett, you will wait before buying a stock even if you find it very attractive. It could be a trap. It may be better for survival to avoid unpleasant situations promptly and react slowly to favorable situations.
Yang et al. (2022) used a token gambling task to show that monkeys altered their risk preference across wealth levels and gain/loss contexts. Consistent with the concept suggested by prospect theory, they proposed that the AIC neurons may encode behaviorally relevant value information. The AIC would represent both the subject's current state (the reference point) and reference-dependent value signals that vary in the loss or gain context (asymmetrical value functions in loss and gain), which would together influence a subject's risk attitude in decision-making. To better understand the effect of tokens on different components, they modeled the behavior for each start token number separately. In the gain context, both monkeys were risk-seekers when the starting token number was low; however, both demonstrated risk-neutral or risk-averse behavior when the start token number increased. This result is consistent with Yamada et al. (2021) and Imaizumi et al. (2022). Moreover, Yang et al. (2022) showed, in monkey G, the utility functions (value functions) in monkey G were consistently steeper for losses than for gains. Thus, monkey G showed loss aversion behavior. However, they showed that in monkey O, the utility functions were not consistently steeper for losses than for gains, indicating that monkey O was equally sensitive to gains and losses and thus was not averse to loss. This is similar to the context where there are different types of investors.
A new concept of the value function can be readily derived by integrating these studies on the neural systems' responses to losses and gains. Kahneman and Tversky (1979) proposed that the value function is: (1) defined on deviations from the reference point; (2) concave for gains and convex for losses; and (3) steeper for losses than for gains. However, they have also noted that "most utility functions for gains were concave, most functions for losses were convex, and only three individuals exhibited risk aversion for both gains and losses. With a single exception, utility functions were considerably steeper for losses than for gains" (Kahneman and Tversky (1979), 280). The most important point is that the reference point of the value function is non-differentiable. In fact, it is illustrated that way in Figure 3—a hypothetical value function of their article (279). Although it is not heavily emphasized in economics textbooks, the discovery of a non-differentiable reference point in a value function is remarkable. A Reference point is precisely the neuronal cusp where different nervous systems intersect.
Yang et al. (2022) also observed variations in the value function using two examples. Ferrari-Toniolo and Schultz (2023) explored how signals from individual brain cells involved in behavior can vary. This variation could be attributed to the unreliability of single neurons or to a more complex system where different neurons contribute distinct pieces of information. This concept might also hold true for brain activity related to economic decisions and the associated rewards. The value of a reward is subjective, depending on both its size and the probability of obtaining it. To understand how the brain represents these subjective values, researchers rely on the continuity axiom. This principle is tested by presenting animals with various rewards of different sizes and probabilities. If animals comprehend the choices and make meaningful decisions, their behavior should adhere to the continuity axiom.
Ferrari-Toniolo and Schultz (2023) explored how the brain represents subjective value in economic decision-making. Their work suggests that signals from individual neurons might be unreliable, but the brain seems to overcome this by using a "population code." This code combines information from many neurons to accurately represent subjective value, even if the individual neurons themselves have noisy or inconsistent signals.
They tested this idea in the orbitofrontal cortex (OFC), a brain region crucial for economic decisions in monkeys. They found that while individual neurons in the OFC displayed a variety of value signals, these signals often didn't directly reflect the monkey's choices. However, when they considered the activity of entire populations of neurons, a clear pattern emerged. The combined signal from the population accurately matched the monkey's choices, suggesting that the OFC uses a complex code to represent subjective value despite the limitations of individual neurons.
In this section, we present one theoretical hypothesis regarding traders' decision-making. When do traders exit their position? Why would a trader close a position at some point when losses and gains are continuously changing?
This problem can be addressed using cusp catastrophe theory. In this theory, the concepts of control space and behavior space are crucial for understanding how systems respond to changes. In our example, the variables in the control space are gains and losses, while the behavior space is represented by the surface of a function, illustrating how behavior changes in response to variations in gains and losses. A control space refers to the range of variables or parameters that can be manipulated or controlled within the system. These variables can influence the system's behavior, often leading to different outcomes based on their values. Behavior space, on the other hand, represents the observable outcomes or behaviors of the system as a result of changes in the control variables. It reflects how the system behaves under various conditions defined by the control space. The relationship between these two spaces is significant: changes in the control space can lead to sudden shifts in the behavior space, particularly when critical thresholds are crossed. This dynamic interaction helps to explain why small adjustments in control parameters can sometimes result in abrupt and significant changes in behavior, highlighting the nonlinear nature of the system.
Recently, cusp catastrophe theory has been applied in various fields. Barnik and Vosvrda (2009) made the first attempt to fit a stochastic cusp catastrophe model to stock market data. The authors took a macroeconomic perspective and applied stochastic cusp catastrophe theory to understand the dynamics of the entire stock market and the mechanisms behind market crashes. Rather than focusing on the behavior or decision-making of individual traders, the research emphasizes the nonlinear dynamics of the market as a whole. Therefore, it is concerned with macroeconomic factors and market movements. Wei et al. (2024) investigated the factors that lead to sudden shifts in online impulsive buying behavior. The authors developed a qualitative cusp catastrophe model integrated with multi-agent simulations to analyze how various elements—such as consumer emotions, social influence, and external triggers—interact to create abrupt changes in purchasing decisions. By simulating different scenarios, the study highlights the conditions under which impulsive buying can escalate dramatically. Key findings indicate that certain thresholds can prompt significant changes in consumer behavior, suggesting that understanding these cusp points can help businesses better predict and respond to shifts in online shopping patterns. The research contributes valuable insights into the psychology of online consumers and the dynamics of e-commerce, offering practical implications for marketers and platform designers. Chen et al. (2014) presented a novel application of the cusp catastrophe model to explore nonlinear health outcomes in nursing contexts. The authors argue that traditional linear models may not adequately capture the complexity of health outcomes influenced by various factors, such as patient characteristics, environmental conditions, and nursing interventions. By employing the cusp catastrophe model, they aim to identify critical thresholds that can lead to sudden changes in health outcomes, providing a more nuanced understanding of patient responses to care. Through simulations and case studies, the research demonstrates how this nonlinear approach can reveal insights into the dynamics of health outcomes, highlighting the importance of recognizing tipping points in patient care. The findings suggest that using the cusp catastrophe model could enhance nursing research and practice by improving the prediction of health outcomes and guiding interventions more effectively. Overall, the paper contributes to the growing body of literature on innovative modeling techniques in healthcare.
The logical connection between psychology and the behavioral cusp model lies in the understanding of how abrupt changes in behavior can be influenced by psychological factors and neural mechanisms. The behavioral cusp model helps explain how small changes in control variables (like emotions or external stimuli) can lead to sudden shifts in behavior, such as impulsive buying or risk-taking. The model posits that there are critical thresholds that, when crossed, result in significant changes in behavior. This concept aligns with psychological theories that emphasize the role of cognitive and emotional thresholds in decision-making.
Neuroscientific research has identified specific brain regions involved in processing rewards and risks. These areas are critical for evaluating control variables and can show changes in activity when individuals approach behavioral thresholds. The role of neurotransmitters like dopamine can illustrate how psychological states (like motivation or pleasure) interact with control parameters in the cusp model. For instance, increased dopamine levels may lower the threshold for engaging in impulsive behaviors.
By integrating insights from psychology and neuroscience, we can better understand how mental states and brain activity influence behavioral responses in the context of the cusp model. This interdisciplinary approach can help in developing interventions or predicting behavior in various settings, such as marketing or clinical psychology.
The connection between psychology and the behavioral cusp model is grounded in the understanding that psychological factors and neural mechanisms interplay to shape behavior. By studying these relationships, we can gain deeper insights into the dynamics of decision-making and the conditions under which sudden behavioral changes occur.
Now, we obtain the 3D image of the value function with cusps near the decision point (Figure 2). The words "anger" and "fear" on the losing side are used in the famous dog example by Zeeman (1976). For example, when a stock trader exits the position, she jumps and fixates on loss or gain. The value function, therefore, should have cusps corresponding to unstable psychology. It is well known that investor psychology is easily affected. We can assume that these cusps are also moving. They could be a type of noise that can be synchronized and influence investors' decisions. Indeed, when viewed individually, there are no such cusps in trading driven by mechanical algorithms. However, when viewed collectively, even trading algorithms driven by AI may involve interactions between individual trades, leading to the potential for instantaneous synchronization that could be modeled as a mathematical catastrophe.
The existence of diverse value functions at the neuronal level implies the existence of diverse types of investors in the market. In this section, we investigate what types of algorithms are advantageous in short-term algorithmic trading unrelated to behavioral cusps. According to the efficient market hypothesis, the existence of consistently advantageous trading algorithms implies the existence of arbitrage opportunities.
Shefrin and Statman (1985) explored the disposition effect, which is the tendency of investors to sell winning stocks too early and hold onto losing stocks for too long. This behavior contradicts rational expectations and tax-efficient strategies. The authors attribute this to prospect theory's value function, where investors are more sensitive to losses than gains. The fear of realizing losses (loss aversion) leads to holding onto losers, while the diminishing sensitivity to gains prompts premature selling of winners. This aligns with the investment wisdom of "cut losses short, let profits run, " emphasizing the importance of swift loss realization and allowing gains to potentially grow further.
The diversity of value functions manifests itself as differences in risk preference near the reference point. For instance, a type that exhibits risk aversion on both the gain and loss sides near the reference point (C and F in Figure 1) would, in reality, refrain from purchasing risky assets. In this analysis, we simplify the characteristics of the value function near the reference point and compare the performance of three types: a type whose position liquidation timing is symmetrical with respect to gains and losses, a type whose gain realization is faster than loss realization, and the reverse type. Specifically, we examine scenarios where:
1. gains and losses are both 1%,
2. gains are 1.5% and losses are 0.5%, and
3. the reverse, where gains are 0.5% and losses are 1.5%.
We will conduct this analysis using TOPIX tick data from the JPX Data Cloud (Figure 4).
Let's explain the analysis method. There are various programming codes to execute this simulation, but considering processing speed, parallel processing is essential. We used R version 4.4.0 along with two libraries, foreach and pforeach (https://github.com/hoxo-m/pforeach). We will randomly extract 1000 ticks from each month's TOPIX tick data. The data period is from August to December in 2021, with data sizes of 377,902 ticks in August, 359,907 ticks in September, 377,899 in October, 359,908 ticks in November, and 395,895 ticks in December. From those, we will select the tick that first reaches a 1% increase or decrease, and calculate the profit or loss if we were to settle at that point (type 1). Similarly, we will calculate the profit and loss for cases where we settle at a 1.5% increase and a 0.5% decrease (type 2), as well as a 0.5% increase and a 1.5% decrease (type 3). We will repeat this process 1000 times and compare the performance of the trades. These parameters represent a first-order approximation of the local shape of the value function near the reference point. Considering that the global properties of the value function are believed to exhibit significant variation, this paper aims to compare the performance of short-term trades based on differences in the local characteristics of the value function near the reference point.
The results of this Monte Carlo simulation are shown in Figures 5 through 9. It is evident, even without statistical analysis, that there is no consistently superior trading algorithm.
As one might easily surmise, in a rising market, delaying profit-taking could lead to greater gains, while in a falling market, quicker stop-losses would curb losses. However, this is impossible without a rational and theoretical method to determine market trends. Therefore, in trading an index like TOPIX, it is not possible to obtain arbitrage profits with a simple mechanical algorithm like the one adopted here. In this sense, the efficient market hypothesis can be considered valid.
Type (gain, loss) | Type 1 | (1.0, 1.0) | Type 2 | (1.5, 0.5) | Type 3 | (0.5, 1.5) |
Month (ticks) | Mean | Variance | Mean | Variance | Mean | Variance |
August (377,902) | 4,266.31 | 365,252.1 | 3,083.55 | 342,724.7 | 1,387.24 | 288,850.7 |
September (359,907) | 2,422.43 | 601,216.4 | 6,421.68 | 588,405.1 | 1,261.64 | 429,503.6 |
October (377,899) | 3,649.76 | 408,103.9 | 295,436.6 | 3,370.87 | 342,059.1 | |
November (359,908) | 348,855.9 | 131,341.4 | 422,268.2 | |||
December (395,895) | 7,431.03 | 341,639.2 | 3,452.62 | 322,042.6 | 4,864.27 | 304,590.1 |
Mechanical algorithms, such as those tested in this paper, lack psychological cusps. However, AI-based algorithmic trading might incorporate unforeseen cusps. The synchronized trading of diverse AI algorithms, learning from vast amounts of information concurrently, could significantly impact the market. Therefore, a theoretical examination of the issue of human psychological cusps is deemed crucial.
The concept of behavioral cusps remains a theoretical hypothesis. To fully embrace this new perspective of the value function with cusps, another challenge must be addressed: the necessity of employing terms like "anger" or "fear" to represent these cusps. Since the 19th century, there has been ongoing debate regarding whether "emotions" are the cause or consequence of their associated behaviors. Anderson and Adolphs (2014) contended that emotional behaviors are a class of behaviors that express internal emotional states. These states exhibit general functional and adaptive properties that are consistent across all human emotions, such as fear or anger, and throughout phylogeny. Paul and Mendl (2018) highlighted the complexity of defining emotions in the human context, a difficulty that is magnified when dealing with animals incapable of self-expression. In such cases, notions of internal or consciously experienced states remain inaccessible. Hence, it would be premature to infer the influence of emotions solely from monkeys' neuronal responses that appear evident to us.
The reference point within the value function could potentially be a cusp, representing a behavioral change (Rosales-Ruiz and Baer (1997); Bosch and Fuqua (2001); Bosch and Hixson (2004); Smith et al. (2006)) associated with a mathematical catastrophe (Whitney (1955); Zeeman (1976)). This possibility arises from the substantial differences observed in the neural system's responses to losses and gains. We monitor the outcomes of our choices and adjust subsequent choices using this outcome information to select appropriate actions. Studies by Kawai et al. (2015), Yamada et al. (2021), Imaizumi et al. (2022), Yang et al. (2022), and Ferrari-Toniolo and Schultz (2023) have examined neuronal responses to losses, gains, and reference points. These studies suggest that two distinct neural systems might respond differently to losses and gains, resulting in a value function characterized by a reference point acting as a neuronal cusp.
The introduction of cusps in the value function of prospect theory indeed raises important questions regarding the axiom of continuity. In traditional utility theory, the axiom of continuity states that small changes in outcomes should not lead to abrupt changes in preferences. However, the presence of cusps implies that there are points where the value function has sharp turns, potentially leading to discontinuities in how individuals evaluate changes in outcomes.
Value function characteristics
The value function in prospect theory is typically concave for gains and convex for losses, exhibiting risk aversion in gains and risk-seeking behavior in losses. When cusps are introduced, the function becomes non-differentiable at certain points, which can create scenarios where minor changes in wealth lead to significant shifts in perceived value.
Decision-making under uncertainty
According to prospect theory, individuals evaluate potential outcomes based on perceived gains and losses rather than final wealth. The introduction of cusps means that for certain ranges of outcomes, a small change can drastically affect the decision-maker's valuation. This can lead to inconsistent choices when evaluating similar prospects, as the evaluation process may jump from one valuation to another due to the cusp.
Behavioral implications
The discontinuity introduced by cusps can result in framing effects, where the way a choice is presented influences decision-making. For example, individuals may become overly sensitive to specific outcomes associated with the cusps, leading them to prefer options that avoid loss even if they are not optimal from a utility perspective.
Impact on risk assessment
The presence of cusps could also distort risk perception, causing individuals to overreact to losses near a cusp and underreact to losses further away. This could skew their decisions, making them less stable and predictable.
In summary, while the introduction of cusps in the value function offers a nuanced understanding of decision-making under risk, it challenges the axiom of continuity and suggests that individuals may make choices based on abrupt changes in perceived value rather than smooth transitions. This has significant implications for understanding behavior in economic contexts and the psychological mechanisms behind risk assessment and decision-making.
While the study provides valuable insights into investor behavior, it is crucial to recognize that the findings may not be universally applicable to all contexts or populations. Human behavior is influenced by numerous factors, including psychological, social, and emotional components. The study may not fully account for all these complexities, leading to oversimplifications in the interpretation of results. To enhance the robustness of the findings, future research should aim to include diverse samples and consider longitudinal studies that track changes over time. This would help in better understanding the nuances of investor behavior and the factors influencing it.
Variations in value functions arising from neuron-level diversity can be considered to contribute to the diversity of investors in the market. The presence of diverse investors is likely to lead to the revitalization and stabilization of market transactions. On the other hand, if the psychological cusps of investors synchronize, it will bring extreme price movements to the market, and the same possibility exists for algorithmic trading, including AI. A pertinent issue is the need to clarify how our findings have implications for investors in future markets. Fortunately, an R package for the cusp model has been developed, providing concrete examples of analysis (Grasman et al. (2009)). Therefore, with appropriate data, it is possible to conduct analyses on behavioral cusps. However, such analyses require data on the decision-making processes of multiple traders, and obtaining and utilizing actual trading records may be challenging due to privacy concerns. We encourage future researchers to explore these avenues, as they could open new pathways for understanding market dynamics.
In economics, we assume the rationality of economic agents. For instance, theoretical economics loses relevance without assumptions like utility maximization and profit maximization. Economists have long debated the issue of time preference (Strotz (1955); Thaler and Shefrin (1981); Haigh and List (2005)) and the validity of prospect theory. Machina (1982)'s generalized expected utility analysis can be employed to construct a simple model of preferences that connects various observed risk-related behaviors. However, it remains a mathematical and abstract theory of human rationality within economic phenomena, representing only one facet of our innate nature. The existence of diverse types of investors leads to variations in the valuation of risky assets in the market, which is thought to stimulate trading activity. However, the synchronization of psychological cusps has the potential to generate extreme price movements.
It is clear that humans are both rational and emotional. While these two aspects often conflict, both are essential for maintaining human society. This insight was recognized by Adam Smith in the 18th century. Smith (1759, Smith 1776), considered the father of economics, believed that the pursuit of self-interest should be moderated by a "fellow feeling." However, contemporary economics focuses solely on the rational behavior of self-interested economic agents, neglecting the existence and impact of empathy.
Furthermore, Smith (1759) observation holds significant importance when considering the value function. Human empathy—the ability to share and understand the emotions of others—is another cornerstone of social interaction. Recent research has delved into the mechanisms underlying this remarkable phenomenon, uncovering the intricate interplay of shared emotions, imitation, and learned perceptual-motor links.
Imitation, another pillar of empathy, manifests as the automatic mirroring of observed behaviors. Witnessing someone's actions triggers the activation of corresponding motor representations in our own brains, as if we were preparing to perform those actions ourselves. These internally generated motor representations then guide our own actions, leading to the imitation of observed behaviors.
Recent studies on empathetic systems support Smith's idea (Preston and de Waal FBM (2002); de Wall (2009); Yamamoto (2016)), which include noise synchronization (Sano (2022)). Perhaps it is time to revisit Smith's concepts and rethink economics in terms of justice and empathy. This perspective is lacking in modern microeconomic theory and could introduce a new field of economics.
All data used in this paper can be obtained from JPX Data Cloud (https://db-ec.jpx.co.jp/).
The author declares they have not used Artificial Intelligence (AI) tools in the creation of this article.
The author declares no conflict of interest.
[1] |
Anderson D, Adolphs R (2014) A framework for studying emotions across species. Cell 157: 187–200. https://doi.org/10.1016/j.cell.2014.03.003 doi: 10.1016/j.cell.2014.03.003
![]() |
[2] | Andreoni J, Kuhn M, Sprenger C (2015) Measuring time preferences: A comparison of experimental methods. J Econ Behav Organ 116: 451–464. Available from: https://www.sciencedirect.com/science/article/pii/S0167268115001535. |
[3] |
Ariely D, Huber J, Wertenbroch K (2005) When do losses loom larger than gains? J Market Res 42: 134–138. https://doi.org/10.1509/jmkr.42.2.134.62283 doi: 10.1509/jmkr.42.2.134.62283
![]() |
[4] |
Barnik J, Vosvrda M (2009) Can a stochastic cusp catastrophe model explain stock market crashes? J Econ Dyn Control 33: 1824–1836. https://doi.org/10.1016/j.jedc.2009.04.004 doi: 10.1016/j.jedc.2009.04.004
![]() |
[5] |
Bechara A, Damasio AR, Damasio H, et al. (1994) Insensitivity to future consequences following damage to human prefrontal cortex. Cognition 50: 7–15. https://doi.org/10.1016/0010-0277(94)90018-3 doi: 10.1016/0010-0277(94)90018-3
![]() |
[6] |
Blavatskyy P (2021) A simple non-parametric method for eliciting prospect theory's value function and measuring loss aversion under risk and ambiguity. Theor Decis 91: 403–416. https://doi.org/10.1007/s11238-021-09811-6 doi: 10.1007/s11238-021-09811-6
![]() |
[7] |
Bosch S, Fuqua R (2001) Behavioral cusps: A model for selecting target behaviors. J Appl Behav Anal 34: 123–125. https://doi.org/10.1901/jaba.2001.34-123 doi: 10.1901/jaba.2001.34-123
![]() |
[8] |
Bosch S, Hixson M (2004) The final piece to a complete science of behavior: Behavior development and behavioral cusps. Behav Anal Today 5: 244–254. https://doi.org/10.1037/h0100033 doi: 10.1037/h0100033
![]() |
[9] | Camerer C (2004) Prospect theory in the wild: evidence from the field. Adv Behav Econ, 148–161. https://doi.org/10.1515/9781400829118-008 |
[10] | Chen D, Lin F, Chen XJ, et al. (2014) Cusp Catastrophe Model: A Nonlinear Model for Health Outcomes in Nursing Research. Methods 63: 211–200. https://dx.doi.org/10.1097/NNR.0000000000000034 |
[11] | Cohen J, Ericson K, Laibson D. et al. (2020) Measuring Time Preferences. J Econ Literature 58: 299–347. Available from: https://www.aeaweb.org/articles?id = 10.1257/jel.20191074. |
[12] | de Waal F (2009) The age of empathy : nature's lessons for a kinder society, Three Rivers. |
[13] |
Ferrari-Toniolo S, Schultz W (2023) Reliable population code for subjective economic value from heterogeneous neuronal signals in primate orbitofrontal cortex. Neuron 111: 3683–3696.e7. https://doi.org/10.1016/j.neuron.2023.08.009 doi: 10.1016/j.neuron.2023.08.009
![]() |
[14] |
Goyal P, Guputa P, Yadav V (2021) Antecedents to heuristics: decoding the role of herding and prospect theory for Indian millennial investors. Rev Behav Financ 15: 79–102. http://dx.doi.org/10.1108/RBF-04-2021-0073 doi: 10.1108/RBF-04-2021-0073
![]() |
[15] |
Graham L, Snower D (2013) Hyperbolic discounting and positive optimal inflation. Macroecon Dyn 17: 591–620. https://doi.org/10.1017/S1365100511000393 doi: 10.1017/S1365100511000393
![]() |
[16] |
Grasman R, van der Maas HL, Wagenmakers EJ (2009) Fitting the Cusp Catastrophe in R: A cusp Package Primer. J Stat Softw 32: 1–27. https://doi.org/10.18637/jss.v032.i08 doi: 10.18637/jss.v032.i08
![]() |
[17] |
Haigh M, List J (2005) Do professional traders exhibit myopic loss aversion? an experimental analysis. J Financ 60: 523–534. https://doi.org/10.1111/j.1540-6261.2005.00737.x doi: 10.1111/j.1540-6261.2005.00737.x
![]() |
[18] |
Harinck F, Dijk E, Beest I, et al. (2007) When gains loom larger than losses: reversed loss aversion for small amounts of money. Psychol Sci 18: 1099-1105. https://doi.org/10.1111/j.1467-9280.2007.02031.x doi: 10.1111/j.1467-9280.2007.02031.x
![]() |
[19] |
Imaizumi Y, Tymula A, Tsubo Y, et al. (2022) A neuronal prospect theory model in the brain reward circuitry. Nat Commun 13: 5855. https://doi.org/10.1038/s41467-022-33579-0 doi: 10.1038/s41467-022-33579-0
![]() |
[20] |
Kahneman D, Tversky A (1979) Prospect Theory: An analysis of decision under risk. Econometrica 47: 263–291. https://doi.org/10.2307/1914185 doi: 10.2307/1914185
![]() |
[21] | Kahneman D, Tversky A, (Eds.). (2000) Choices, Values, and Frames. Cambridge University Press. https://doi.org/10.1017/CBO9780511803475 |
[22] |
Kawai T, Yamada H, Sato N, et al. (2015) Roles of the lateral habenula and anterior cingulate cortex in negative outcome monitoring and behavioral adjustment in nonhuman primates. Neuron 88: 792–804. https://doi.org/10.1016/j.neuron.2015.09.030 doi: 10.1016/j.neuron.2015.09.030
![]() |
[23] | Larson F, List J, Metcalfe R (2016) Can myopic loss aversion explain the equity premium puzzle? evidence from a natural field experiment with professional traders. Nat Bureau Econ Res Available from: http://www.nber.org/papers/w22605. |
[24] |
LeDoux JE (2000) Emotion circuits in the brain. Annu Rev Neurosci 23: 155–184. https://doi.org/10.1146/annurev.neuro.23.1.155 doi: 10.1146/annurev.neuro.23.1.155
![]() |
[25] |
Lejarraga T, Hertwig R (2017) How the threat of losses makes people explore more than the promise of gains. Psychon Bull & Rev 24: 708–720. https://doi.org/10.3758/s13423-016-1158-7 doi: 10.3758/s13423-016-1158-7
![]() |
[26] | Liao W, Jiang Y, Li D, et al. (2022) Cognition contra camouflage: How the brain mediates predator-driven crypsis evolution. Sci Adv 8. http://dx.doi.org/10.1126/sciadv.abq1878 |
[27] |
Liberman N, Idson L, Higgins E (2005) Predicting the intensity of losses vs. non-gains and non-losses vs. gains in judging fairness and value: A test of the loss aversion explanation. J Exp Soc Psychol 41: 527–534. https://doi.org/10.1016/j.jesp.2004.06.007 doi: 10.1016/j.jesp.2004.06.007
![]() |
[28] |
Linzmajer M, Hubert M, Hubert M (2021) It's about the process, not the result: An fMRI approach to explore the encoding of explicit and implicit price information. J Econ Psychol 86: 102403. https://doi.org/10.1016/j.joep.2021.102403 doi: 10.1016/j.joep.2021.102403
![]() |
[29] |
Machina M (1982) "Expected Utility" analysis without the independence axiom. Econometrica 50: 277–323. https://doi.org/10.2307/1912631 doi: 10.2307/1912631
![]() |
[30] |
McGraw A, Larsen J, Kahneman D, et al. (2010) Comparing gains and losses. Psychol Sci 21: 1438–1445. https://doi.org/10.1177/0956797610381504 doi: 10.1177/0956797610381504
![]() |
[31] |
Mengov G, Georgiev N, Zinovieva I (2022) Virtual social networking increases the individual's economic predictability. J Behav Exp Econ 101: 101944. https://doi.org/10.1016/j.socec.2022.101944 doi: 10.1016/j.socec.2022.101944
![]() |
[32] |
Meyer S, Pagel M (2022) Fully Closed: Individual tesponses to realized gains and losses. J Financ 77: 1529–1585. https://doi.org/10.1111/jofi.13122 doi: 10.1111/jofi.13122
![]() |
[33] |
Mogiliansky LA, Zamir S, Zwirn H (2009) Type indeterminacy: A model of the KT (Kahneman–Tversky) –man. J Math Psychol 53: 349–361. https://doi.org/10.1016/j.jmp.2009.01.001 doi: 10.1016/j.jmp.2009.01.001
![]() |
[34] | Neumann J, Morgenstern O (1947) Theory of games and economic behavior, Princeton University Press, 1947. |
[35] |
Nowaihi A, Dhami S (2006) A simple derivation of Prelec's probability weighting function. J Math Psychol 50: 521–524. https://doi.org/10.1016/j.jmp.2006.07.006 doi: 10.1016/j.jmp.2006.07.006
![]() |
[36] |
Padoa-Schioppa C, Assad JA (2006) Neurons in the orbitofrontal cortex encode economic value. Nature 441: 223–226. https://doi.org/10.1038/nature04676 doi: 10.1038/nature04676
![]() |
[37] | Pammi V, Miyapuram K (2012) Neuroeconomics of individual decision making at multiple levels: a review. In Expanding Horizons Of The Mind Science(s), 159–185. Nova Publications, New York. |
[38] |
Paul E, Mendl M (2018) Animal emotion: Descriptive and prescriptive definitions and their implications for a comparative perspective. Appl Anim Behav Sci 205: 202–209. https://doi.org/10.1016/j.applanim.2018.01.008 doi: 10.1016/j.applanim.2018.01.008
![]() |
[39] |
Phelps EA, LeDoux JE (2005) Contributions of the amygdala to emotion processing: From animal models to human behavior. Neuron 48: 175–187. https://doi.org/10.1016/j.neuron.2005.09.025 doi: 10.1016/j.neuron.2005.09.025
![]() |
[40] |
Preston S, de Waal FBM (2002) Empathy: Its ultimate and proximate bases. Behav Brain Sci 25: 1–20. https://doi.org/10.1017/s0140525x02000018 doi: 10.1017/s0140525x02000018
![]() |
[41] |
Rosales-Ruiz J, Baer D (1997) Behavioral cusps: A developmental and pragmatic concept for behavior analysis. J Appl Behav Anal 30: 533–544. https://doi.org/10.1901/jaba.1997.30-533 doi: 10.1901/jaba.1997.30-533
![]() |
[42] |
Sano K (2022) A binary decision model and fat tails in financial market. Appl Sci 12: 7019. https://doi.org/10.1037/0022-3514.81.4.684 doi: 10.1037/0022-3514.81.4.684
![]() |
[43] |
Shefrin H, Statman M (1985) The disposition to sell winners too early and ride losers too long: Theory and evidence. J Financ 40(3): 777–790. https://doi.org/10.1111/j.1540-6261.1985.tb05002.x doi: 10.1111/j.1540-6261.1985.tb05002.x
![]() |
[44] | Smith A (1759) The Theory of Moral Sentiments, 1st ed. (printed for Andrew Millar, in the Strand). |
[45] | Smith A (1776) The Wealth of Nations, 1st ed. (W. Strahan). |
[46] |
Smith G, McDougall D, Edelen-Smith P (2006) Behavioral cusps: A person-centered concept for establishing pivotal individual, family, and community behaviors and repertoires. Focus Autism Dev Dis 21: 223–229. https://doi.org/10.1177/10883576060210040301 doi: 10.1177/10883576060210040301
![]() |
[47] | Stigler G, Becker G (1977) De gustibus non est disputandum. Ame Econ Rev 67: 76–90. https://www.jstor.org/stable/1807222 |
[48] |
Strotz R (1955) Myopia and inconsistency in dynamic utility maximization. Rev Econ Stud 23: 165–180. https://doi.org/10.2307/2295722 doi: 10.2307/2295722
![]() |
[49] | Thaler R, Shefrin H (1981) An economic theory of self-control. J Polit Econ 89: 392–406. https://www.jstor.org/stable/1833317 |
[50] |
Thaler R (1981) Some empirical evidence on dynamic inconsistency. Econ Lett 8: 201–207. https://doi.org/10.1016/0165-1765(81)90067-7 doi: 10.1016/0165-1765(81)90067-7
![]() |
[51] |
Thaler R (1989) Toward a positive theory of consumer choice. J Econ Behav & Organ 1: 39–60. https://doi.org/10.1016/0167-2681(80)90051-7 doi: 10.1016/0167-2681(80)90051-7
![]() |
[52] | Tversky A, Kahneman D (1974) Judgment under Uncertainty: Heuristics and Biases. Science 185: 1124–1131. https://www.jstor.org/stable/1738360 |
[53] | Tversty A, Kahneman D (1992) Advances in prospect theory: Cumulative representation of uncertainty. J Risk Uncertain 5: 297–323. https://www.jstor.org/stable/41755005 |
[54] |
Wallerius ML, Johnsson J, Cooke S, et al. (2020) Hook Avoidance Induced by Private and Social Learning in Common Carp. Transactions Am Fisheries Society 149: 498–511. https://doi.org/10.1002/tafs.10246 doi: 10.1002/tafs.10246
![]() |
[55] |
Walther M, Münster M (2021) Conditional risk premiums and the value function of prospect theory. J Behav Financ 22: 74–83. https://doi.org/10.1080/15427560.2020.1735390 doi: 10.1080/15427560.2020.1735390
![]() |
[56] |
Wei X, Zhang Y, Luo XR, et al. (2024) Qualitative Cusp Catastrophe Multi-Agent Simulation Model to Qualitative Cusp Catastrophe Multi-Agent Simulation Model to Explore Abrupt Changes in Online Impulsive Buying Behavior. J Association Inf Syst 25: 304–340. https://doi.org/10.17705/1jais.00832 doi: 10.17705/1jais.00832
![]() |
[57] |
Weitzman M (2001) Gamma discounting. Ame Econ Rev 91: 260–271. https://doi.org/10.1257/aer.91.1.260 doi: 10.1257/aer.91.1.260
![]() |
[58] |
Whitney H (1955) On singularities of mappings of euclidean spaces. I. Mappings of the plane into the plane. Ann Math 62: 374–410. https://doi.org/10.2307/1970070 doi: 10.2307/1970070
![]() |
[59] |
Yamada H, Imaizumi Y, Matsumoto M (2021) Neural population dynamics underlying expected value computation. J Neurosci 41: 1684–1698. https://doi.org/10.1523/JNEUROSCI.1987-20.2020 doi: 10.1523/JNEUROSCI.1987-20.2020
![]() |
[60] | Yamamoto S (2016) Primate empathy: three factors and their combinations for empathy‐related phenomena. WIREs Cognitive Sci 8. https://doi.org/10.1002/wcs.1431 |
[61] | Yang Y, Li X, Stuphorn V (2022) Primate anterior insular cortex represents economic decision variables proposed by prospect theory. Nat Commun 13. https://doi.org/10.1038/s41467-022-28278-9 |
[62] |
Zablocki-Thomas P, Rogers F, Bales K (2022) Neuroimaging of human and non-human animal emotion and affect in the context of social relationships. Front Behav Neurosci: 16. https://doi.org/10.3389/fnbeh.2022.994504 doi: 10.3389/fnbeh.2022.994504
![]() |
[63] |
Zeeman E (1976) Catastrophe Theory. Sci Am 234: 65–83. https://doi.org/10.1038/scientificamerican0476-65 doi: 10.1038/scientificamerican0476-65
![]() |
Type (gain, loss) | Type 1 | (1.0, 1.0) | Type 2 | (1.5, 0.5) | Type 3 | (0.5, 1.5) |
Month (ticks) | Mean | Variance | Mean | Variance | Mean | Variance |
August (377,902) | 4,266.31 | 365,252.1 | 3,083.55 | 342,724.7 | 1,387.24 | 288,850.7 |
September (359,907) | 2,422.43 | 601,216.4 | 6,421.68 | 588,405.1 | 1,261.64 | 429,503.6 |
October (377,899) | 3,649.76 | 408,103.9 | 295,436.6 | 3,370.87 | 342,059.1 | |
November (359,908) | 348,855.9 | 131,341.4 | 422,268.2 | |||
December (395,895) | 7,431.03 | 341,639.2 | 3,452.62 | 322,042.6 | 4,864.27 | 304,590.1 |
Type (gain, loss) | Type 1 | (1.0, 1.0) | Type 2 | (1.5, 0.5) | Type 3 | (0.5, 1.5) |
Month (ticks) | Mean | Variance | Mean | Variance | Mean | Variance |
August (377,902) | 4,266.31 | 365,252.1 | 3,083.55 | 342,724.7 | 1,387.24 | 288,850.7 |
September (359,907) | 2,422.43 | 601,216.4 | 6,421.68 | 588,405.1 | 1,261.64 | 429,503.6 |
October (377,899) | 3,649.76 | 408,103.9 | 295,436.6 | 3,370.87 | 342,059.1 | |
November (359,908) | 348,855.9 | 131,341.4 | 422,268.2 | |||
December (395,895) | 7,431.03 | 341,639.2 | 3,452.62 | 322,042.6 | 4,864.27 | 304,590.1 |