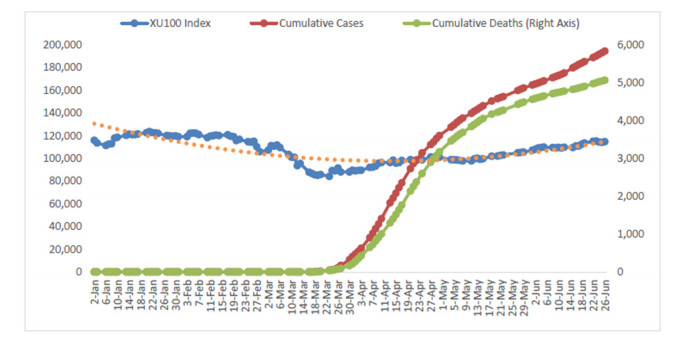
Citation: Morgan Robinson, Brenda Yasie Lee, Zoya Leonenko. Drugs and drug delivery systems targeting amyloid-β in Alzheimer's disease[J]. AIMS Molecular Science, 2015, 2(3): 332-358. doi: 10.3934/molsci.2015.3.332
[1] | Ming-Zhen Xin, Bin-Guo Wang, Yashi Wang . Stationary distribution and extinction of a stochastic influenza virus model with disease resistance. Mathematical Biosciences and Engineering, 2022, 19(9): 9125-9146. doi: 10.3934/mbe.2022424 |
[2] | Tingting Xue, Xiaolin Fan, Zhiguo Chang . Dynamics of a stochastic SIRS epidemic model with standard incidence and vaccination. Mathematical Biosciences and Engineering, 2022, 19(10): 10618-10636. doi: 10.3934/mbe.2022496 |
[3] | Fathalla A. Rihan, Hebatallah J. Alsakaji . Analysis of a stochastic HBV infection model with delayed immune response. Mathematical Biosciences and Engineering, 2021, 18(5): 5194-5220. doi: 10.3934/mbe.2021264 |
[4] | Kangbo Bao, Qimin Zhang, Xining Li . A model of HBV infection with intervention strategies: dynamics analysis and numerical simulations. Mathematical Biosciences and Engineering, 2019, 16(4): 2562-2586. doi: 10.3934/mbe.2019129 |
[5] | Yang Chen, Wencai Zhao . Dynamical analysis of a stochastic SIRS epidemic model with saturating contact rate. Mathematical Biosciences and Engineering, 2020, 17(5): 5925-5943. doi: 10.3934/mbe.2020316 |
[6] | Helong Liu, Xinyu Song . Stationary distribution and extinction of a stochastic HIV/AIDS model with nonlinear incidence rate. Mathematical Biosciences and Engineering, 2024, 21(1): 1650-1671. doi: 10.3934/mbe.2024072 |
[7] | H. J. Alsakaji, F. A. Rihan, K. Udhayakumar, F. El Ktaibi . Stochastic tumor-immune interaction model with external treatments and time delays: An optimal control problem. Mathematical Biosciences and Engineering, 2023, 20(11): 19270-19299. doi: 10.3934/mbe.2023852 |
[8] | Tianfang Hou, Guijie Lan, Sanling Yuan, Tonghua Zhang . Threshold dynamics of a stochastic SIHR epidemic model of COVID-19 with general population-size dependent contact rate. Mathematical Biosciences and Engineering, 2022, 19(4): 4217-4236. doi: 10.3934/mbe.2022195 |
[9] | Divine Wanduku . The stochastic extinction and stability conditions for nonlinear malaria epidemics. Mathematical Biosciences and Engineering, 2019, 16(5): 3771-3806. doi: 10.3934/mbe.2019187 |
[10] | Xiaomeng Wang, Xue Wang, Xinzhu Guan, Yun Xu, Kangwei Xu, Qiang Gao, Rong Cai, Yongli Cai . The impact of ambient air pollution on an influenza model with partial immunity and vaccination. Mathematical Biosciences and Engineering, 2023, 20(6): 10284-10303. doi: 10.3934/mbe.2023451 |
The world has been faced with the Covid-19 common disease phenomenon recently. It originated from China at the end of 2019, spread to other countries, which are close to China in the first months of 2020, and has become pandemic in March 2020 (World Health Organization-WHO, 2020a). The Covid-19 pandemic has been influencing all countries deeply and negatively nowadays.
With the spreading of Covid-19 to countries, most economic and financial indicators have begun to deteriorate. In this context, negative developments have been seen in these indicators such as credit default swap (CDS) spreads, foreign exchange rates (FER), retention share, and net selling amount of foreign investors in equity markets, interest rates, and oil prices. All of these indicators are crucial in terms of roles and effects in economies (Orhan et al., 2019) because they affect foreign investment inflows to countries and investment decisions of foreign investors as well.
All countries pay attention to foreign investments. However, foreign investments have much more significance, especially in emerging countries. Emerging countries can mainly provide financing from external (international/foreign) sources because domestic savings are not sufficient to finance economic activities and growth. Hence, emerging countries try to attract much more foreign investments. By considering that there are two types of foreign investments, which are direct and portfolio (Karikari, 1992; Zengin et al., 2018), attracting more foreign direct investment is not easy because of the geopolitical and political considerations. On the other hand, stimulating foreign portfolio investments, which can be made via bonds, bills, and equities, is much easier concerning foreign direct investments.
Foreign investors consider the riskiness of countries in allocating funds among various asset opportunities to benefit from diversification (Dooley & Hutchison, 2009; Yang et al., 2018; Akhtaruzzaman et al., 2020a) in decreasing risks in the globalizing world. Besides, financial, fiscal, price, and macroeconomic stability in invested countries are significant for foreign investors. All these have a huge role in the financing and development of emerging countries via affecting the improvement of both the real and financial sectors.
Although increasing foreign investments is desired, there are also some arguments against the role of foreign investments in emerging countries. Especially, foreign portfolio investments have been criticized very often. Naturally, it is expected that foreign portfolio investments make effects on the financial markets (i.e. stock exchanges) of countries. Foreign investments affect the development of equity markets, prices, returns, and volatility (Hargis & Ramanlal, 1997; Bekaert & Harvey, 2003). However, the direction of foreign investment effects is not clear and crucial at this point. Levine & Zervos (1998), and Rogoff (1999) conclude that foreign capital inflows make a positive contribution to countries' growth and development. On the other hand, some have argued that retention share and net selling amount in this study have negative effects (Adabag & Ornelas, 2004) if foreign investors decrease the retention share and increase the net selling amount on emerging countries. Also, unfettered activities of foreign investors are evaluated as harmful by some researchers (Rodrik & Subramanian, 2009; Stiglitz, 2010).
On the other hand, there is a Covid-19 pandemic reality in the world that has been affecting all countries. There are nearly 9.9 million cases, and 497 thousand deaths because of the Covid-19 pandemic in the world as of 06.26.2020 (WHO, 2020b). Similarly, the Covid-19 pandemic increased in Turkey. Covid-19 has been determined on 03.10.2020 and the first death has occurred on 03.17.2020 in Turkey. Total cases have become 194.5 thousand and total deaths are 5 thousand in Turkey resulting from Covid-19 as of 06.26.2020 (Ministry of Health of Turkey-MHT, 2020).
While Covid-19 presents and continues to cause deaths, economic and financial indicators have been deteriorating in such an environment. Even, the pandemic causes unprecedented and destructive effects on economies and indicators (Goodell, 2020; Rizwan et al., 2020). Therefore, the Covid-19 pandemic should be considered in making any analysis, especially when examining macroeconomic and financial related issues such as the development of the main stock index. Also, it is seen that the level of economic activities has been decreasing in the time of Covid-19. Therefore, the negative effects of the pandemic have been felt deeply in emerging countries. In this context, Figure 1 presents the development of the XU100 index, cumulative cases, and deaths resulting from the Covid-19 pandemic in Turkey.
As Figure 1 shows, the XU100 index was relatively high before the Covid-19 pandemic times concerning in time of the Covid-19 pandemic. Also, total cumulative cases have reached 194.5 thousand and total deaths have become 5 thousand as of 06.26.2020 in Turkey.
Turkey has been taking measures to decrease the negative effects of the Covid-19 pandemic on the economy, corporations, households, and individuals which is similar to other countries affected by the pandemic (Akhtaruzzaman et al., 2020b; Gherghina et al., 2020; Kartal, 2020; Narayan et al., 2020; Phan & Narayan, 2020). Some of these measures could be summarized as local lockdowns, quarantines on residential areas, ban on flights, lowering the minimum payment requirements of credit cards, cash transfers to families in need, increasing the amount of funding markets by the central bank of the Republic of Turkey including buying governmental bonds, deferred credit payments to banks, deferred governmental (tax and social security premium) payments in various sectors, and minimum wage subsidies (Stanley, 2020; Official Gazette, 2020).
In summary, Turkey has been in the Covid-19 pandemic since 11.03.2020, there have been serious cumulative cases and deaths resulting from the Covid-19 pandemic, there is an important amount of foreign portfolio outflows from Turkey since the beginning of 2020, and the XU100 index has been decreasing and in low-level concerning before the Covid-19 pandemic period. The low-level of the stock exchange index causes the underpricing of Turkish assets and this situation is negative for Turkey. In this context, it could be beneficial to examine the effects of activities of foreign investors on the main stock exchange index in Covid-19 pandemic times and whether the effects of these factors vary according to period whether Turkey is in either before the Covid-19 pandemic period or in Covid-19 pandemic times. This determination could contribute to regulatory bodies to develop policies, take measures, and prevent the negative effects on main stock exchange indices. Hence foreign portfolio investments to Turkey via equities could be stimulated, and Turkey could have the opportunity to increase foreign portfolio inflows, and Turkish assets could be priced at their fair value that all affect the distribution of financial sources among alternative investment instruments including stock exchange.
The study aims to define the effects of activities of foreign investors on the XU100 index by performing the Engle-Granger cointegration test, the Toda-Yamamoto causality test, and MARS analysis. Daily data between 01.02.2020 and 06.26.2020, activities of foreign investors (retention share and net selling amount), and the presence of the Covid-19 pandemic are used for this aim. Turkey is focused on because of being a pioneering and leading emerging country and the main stock exchange index of Turkey decreased on average at an important amount during the Covid-19 pandemic period. Also, the main motivation of the study is that there are approximately Turkish Lira (TRY) 33 billion foreign portfolio outflows from Turkey stock market since 2020 beginning (Central Securities Depositories of Turkey-CSD, 2020) and whether the behavior of foreign investors are influential on the main stock exchange index of Turkey or not.
The main contributions of the study are that the study (ⅰ) is the pioneer study in Turkey examining the effects of foreign investors' activities on the main stock exchange index at Covid-19 age; (ⅱ) examines the XU100 index by focusing on mainly foreign investors' activities with using retention share and net selling amount as dependent variables; (ⅲ) performs Engle-Granger cointegration test and Toda-Yamamoto causality test. Also, the study employs the MARS method which is used for the first time to examine the changes of the XU100 index; (ⅳ) uses daily data between 01.02.2020 and 06.26.2020 which covers before and in time of the Covid-19 pandemic. Data for foreign retention share, which is calculated based on the openness of publicly traded companies in Borsa İstanbul (BIST), is also used for the first time.
The rest of the study is organized as follows. Part 2 explains the variables by benefitting from the literature, data, and methods used in the study. Part 3 presents the empirical results. Part 4 presents a findings-based discussion and policy implications. Part 5 concludes.
By considering that the study focuses on the effects of foreign investors' activities on the main stock exchange index, the XU100 index is used as a dependent variable because the XU100 index is the main stock exchange index in Turkey as in line with the studies of Kesik et al. (2016), and Kartal et al. (2020).
In the literature, some studies examine the causes of the main stock exchange indices' changes. In this context, a variety of macroeconomic and financial variables such as economic growth, foreign direct investments, foreign exchange rates, inflation, interest rates, money supply, production index, etc. are considered in these studies. For example, Kwon & Shin (1999) consider the production index, exchange rate, trade balance, and money supply. However, data for such variables are issued on a quarterly or monthly basis, and the study focuses on the Covid-19 pandemic times which requires working with the most recent (i.e. daily base) data. By considering this fact, Kartal et al. (2020) include CDS spreads, FER, foreign investors' retention share, and net selling amount as variables that have daily data. For these reasons, the mentioned macroeconomic variables are not considered and included in the study. Moreover, it is acknowledged that there is evolving literature concerning the Covid-19 pandemic and its effects on various economic and financial indicators.
On the other hand, foreign portfolio inflows, foreign investors' trading, and net selling amount of foreign investors in emerging economies are examined in various studies. Table 1 summarizes some of these studies.
Authors | Year | Scope | Period | Method | Results |
Clark & Berko | 1997 | Mexico | 1989/1–1996/3 | Regression | The increase in FPI causes an increase in stock prices. |
Bekaert & Harvey | 1998 | 17 Emerging Countries | 1977–1996 | GARCH | Increasing flows have a high correlation with returns. |
Dahlquist & Robertsson | 2004 | Sweden | 1993–1998 | VAR | Net purchases of foreign investors provide a permanent increase of stock prices. |
Baklacı | 2007 | Turkey | 1997/1–2006/4 | Granger causality & OLS | There is a significant bilateral interaction between FPI and stock returns. |
Boyer & Zheng | 2009 | United States | 1952–2004 | VAR & Regression | There is a significant positive relation between stock market returns and flows of foreign investors. |
Sevil et al. | 2012 | Turkey | 2006/1–2010/4 | VECM & Granger causality | There is causality from foreign investors to market index return. |
Vo | 2015 | Vietnam | 2006/2012 | GMM & Regression | Ownership of foreign investors decreases firm stock price volatility. |
Kesik et al. | 2016 | Turkey | 2005/2015 | Granger causality | FPI have an increasing impact on XU100 index. |
Liew et al. | 2018 | Malaysia | 2009/1–2016/12 | VAR & Granger causality | There is a one-way causality from gross inflows. |
Topaloğlu et al. | 2019 | 7 Emerging Countries | 2005–2016 | Panel data analysis | There is a significant and positive nexus between FPI and stock market returns. |
Angelovska | 2020 | Macedonia | 2005/1–2009/12 | Regression | Net inflows are connected with the rise of returns. |
David et al. | 2020 | 11 Countries | 79 Trading Days | Threshold cointegration | Shocks caused by diseases have an important effect on stock indices. |
Gupta & Ahmet | 2020 | India | 2002/1–2016/12 | ARDL | The performance of stock exchanges is one of the most important factors for FPI. |
Kartal et al. | 2020 | Turkey | 2020/1–2020/5 | Machine learning | The retention amount of foreign investors is influential in both the pre-pandemic and the pandemic periods. |
Kotishwar | 2020 | India | 2000/1–2019/12 | ARCH & OLS | FPI influence equity market volatility. |
Note: ARCH = Auto regressive conditional heteroscedasticity; ARDL = Autoregressive distributed lag; FPI = Foreign portfolio inflows; GARCH = Generalized autoregressive conditional heteroscedasticity; GMM = Generalized method of moments; OLS = Ordinary Least Squares; VAR = Vector autoregression; VECM = Vector error correction model. |
As Table 1 summarizes, various studies consider the effects of the foreign investors' activities on stock exchanges. These studies define that some of the foreign trading phenomena are not beneficial; foreign investors make the equity market more efficient and decrease the volatility in the long-run; the equity market index increases when foreign inflows increase. Therefore, it is most likely to make the effect of foreign investors' activities on the XU100 index. In this context, retention share and net selling amount of foreign investors are taken into account in the analysis as dependent variables by considering these studies since the study focuses on the effects of foreign investors' activities XU100 index. Besides, a positive relationship is expected between the XU100 index and retention share of foreign investors; and XU100 and net selling amount of foreign investors.
Moreover, as a black swan case, the Covid-19 pandemic is considered. It is acknowledged that there is a growing literature on the pandemic. By considering that stock market indices have been affected negatively by the pandemic (Adekoya & Kofi Nti, 2020; Al-Awadhi et al., 2020; Ali et al., 2020; Ashraf, 2020; Baker et al., 2020; Corbet et al., 2020; Engelhardt et al., 2020; Erdem, 2020; Gherghina et al., 2020; Liu et al., 2020; Mazur et al., 2020; Zhang et al., 2020), the presence of the pandemic in Turkey, which was seen for the first time on 03.10.2020, is taken into consideration. We use the beginning date of the pandemic in Turkey.
Table 2 summarizes the details of the variables considered in the analysis.
Variable | Symbol | Description | Effect | |
Type | Name | |||
Dependent | Main index | XU100 | The daily closing value of the BIST main index | |
Independent | Retention share | FSHARE | Daily retention share of foreign investors in BIST (%) | + |
Net selling | NETSELL | Daily net selling amount of foreign investors in BIST (TRY denominated amount) | - | |
Covid-19 | COVID | 1: If Covid-19 exists, 0: otherwise | - | |
Note: A positive (+) relationship means that XU100 increases when the independent variable increases. A negative (-) relationship means that XU100 decreases when the independent variable increases. |
This study focuses on Turkey by considering that there is a high amount of foreign portfolio outflows from the stock exchange since the 2020 year beginning and the main stock market index has decreased at an important amount. In this context, the study includes the period between 01.02.2020 and 06.26.2020. This period is selected to focus on the very recent period including Covid-19 pandemic times and the nearest period before the Covid-19 pandemic. Also, data for business days are considered because data of variables can be obtained only for the business day.
Data for the XU100 index are gathered from Bloomberg Terminal. Also, data for foreign investors' retention share and net selling amount are gathered from CSD (2020). Besides, data for Covid-19 situations are gathered from MHT (2020).
The first issue in the analysis is to test the stationarity of the variables to prevent spurious regression and misleading conclusions in turn. Although there is a variety of unit root tests to examine the stationary condition of the data set. Augmented Dickey-Fuller (ADF) is employed in the study to examine the stationarity by considering that this is mostly used in financial time series and studies.
The hypotheses of the ADF test are expressed as follows:
■ H0: Series include unit root (non-stationary).
■ H1: Series include no unit root (stationary).
If test statistics (prob.) of ADF are lower or equal to 0.05, then the H0 hypothesis is rejected which means that the series is stationary.
Engle-Granger cointegration test, which focuses on the long-term relationship among the variables of time-series, includes two steps procedure. In the first step, the stationarity of variables should be provided. After that, regression is applied. Residuals of the regression model are again examined in terms of stationarity by performing unit root tests. If residuals are stationary, then there is a long-term relationship (cointegration) between variables. In other words, these variables move together.
In the second step, the short-term relationship is analyzed with the estimation of an error correction model (ECM). Residual of the regression model could be used to estimate ECM if variables have a long-term relationship (Granger, 1969; Engle & Granger, 1987).
The hypotheses of the Engle-Granger cointegration test are expressed as follows:
■ H0: Variables are not cointegrated (Residuals of OLS include unit root).
■ H1: Variables are cointegrated (Residuals of OLS do not include unit root).
If test statistics (prob.) of the Engle-Granger cointegration test are lower or equal to 0.05, then the H0 hypothesis is rejected which means that there is causality between variables used in the analysis.
Cointegration tests are applied to analyze the long-term relationship between the main stock exchange index and the activities of foreign investors. Cointegration tests are much more appropriate compared to vector autoregression models because they can explore the dynamic co-movements between variables (Mukherjee & Naka, 1995). Therefore, cointegration tests are applied in the study.
Toda & Yamamoto causality test is employed to examine the relationship between variables. Unlike other causality tests, Toda & Yamamoto causality test does not require the stationary situation for variables to be used in the analysis (Dinçer et al., 2020). This is important because using the first difference of variables in the analysis may cause a lack of information and variables may be non-stationary (Tayyar, 2018).
There are two steps in applying the test. In the first step, the unit root test is applied to variables and the maximum cointegration degree (d) is determined. After that, lag lengths (k) are defined. In the second step, "k+dmax" lag formula is applied for estimation (Toda & Yamamoto, 1995).
The hypotheses of the Toda & Yamamoto causality test are expressed as follows:
■ H0: $ {\alpha }_{1i} $ = 0 and $ {\alpha }_{2i} $ = 0 (there is no causality from Y to X).
■ H1: $ {\alpha }_{1i} $ ≠ 0 and $ {\alpha }_{2i} $ ≠ 0 (there is causality from Y to X).
If test statistics (prob.) of Toda & Yamamoto causality test are lower or equal to 0.05, then the H0 hypothesis is rejected which means that there is causality between variables.
As a nonparametric regression modeling of high dimensional data to estimate underlying functional relationships between variables, the MARS method is firstly introduced by Friedman (1991). The main advantage of MARS is that this method does not require any pre-assumption although linear model regressions require some pre-assumptions.
There are two-steps in the MARS method. In the first step, the algorithm starts from a constant term that is the mean of the dependent values. In the second step, the piecewise linear segments, known as basis functions (BFs), are iteratively added to the model.
BFs are dependent on spline functions that are defined on a given segment and the end points of the segment are called knots. The forward step finds the potential knots to improve the performance and leads to overfitting.
A general MARS model could be formulated as in the equation below (Friedman, 1991):
$ Y\_t = {B}_{0}+{\sum }_{k = 1}^{K}{a}_{n}{B}_{n}\left({X}_{t}\right)+ \epsilon $ | (1) |
in which Y is the dependent variable and X represents independent variables. $ {B}_{0} $ denotes the constant term and $ {B}_{n}\left({X}_{t}\right) $ describes the basis function that is estimated by minimizing the residual sum of squares. $ {a}_{n} $ shows the coefficient of nth basis functions (Friedman, 1991). In the backward stepwise stage (pruning stage), it can be eliminated the redundant knots that have the least contribution to the complex model by using Generalized Cross-Validation (GCV). The best model is selected according to the highest coefficient of determination (R2) and the lowest GCV value (Hastie et al., 2009).
Before Covid-19 pandemic times, daily data includes 48 observations from 01.02.2020 and 03.09.2020. On the other side, daily data includes 74 observations that occurred between 03.10.2020 and 06.26.2020 which is related to Covid-19 pandemic times. Figure 2 shows the development of the retention share and net selling amount of foreign investors in Turkey.
As Figure 2 presents, the retention share of foreign investors has been decreasing from 65.9% to 52.4%. Besides, there are net selling amounts for all days except for a few days. These two figures imply that foreign investors' activities have not been supporting the XU100 index. Especially, foreign investors have been taking back their investments from Borsa İstanbul since the beginning of Covid-19 pandemic times in Turkey. As a result of this approach, retention share has been decreasing constantly.
Table 3 summarizes the descriptive statistics of the variables in both periods.
Period Variable | Before Covid-19 | Covid-19 | |||||
XU1001 | FSHARE2 | NETSELL3 | XU1001 | FSHARE2 | NETSELL3 | ||
Mean | 117,361.9 | 61.01856 | 215.9715 | 100,021.8 | 56.56831 | 234.6904 | |
Median | 119,408.5 | 60.73146 | 258.8678 | 99,069.46 | 57.31724 | 278.9121 | |
Maximum | 123,556.1 | 65.85941 | 786.5698 | 115,338.9 | 60.72814 | 1109.929 | |
Minimum | 103,524.0 | 58.88518 | −1029.742 | 84,246.17 | 52.37000 | -485.1429 | |
Std. Dev. | 4,931.612 | 1.547033 | 324.5663 | 85, 17.130 | 2.988219 | 263.4816 | |
Skewness | -0.982580 | 1.401699 | -1.151931 | 0.041302 | -0.114845 | -0.297241 | |
Kurtosis | 3.089060 | 5.100109 | 5.810881 | 2.087917 | 1.440857 | 4.418486 | |
Jarque-Bera | 7.739573 | 24.53900 | 26.41767 | 2.586051 | 7.658022 | 7.293661 | |
Probability | 0.020863 | 0.000005 | 0.000002 | 0.274439 | 0.021731 | 0.026074 | |
Observations | 48 | 48 | 48 | 74 | 74 | 74 | |
Note: 1 The dependent variable; 2 %; 3 million TRY. |
Before Covid-19 pandemic times, the XU100 index is 117,362 on average but it decreased to 103,524 level at the minimum and has reached 123,556 at the maximum. Besides, the retention share of foreign investors is 61% on average. The share decreased to 58.89% at the minimum and reached 65.86% at the maximum. Besides, the net selling amount of foreign investors became TRY 215.9 million on average. On the other hand, in Covid-19 pandemic times, the XU100 index is 100,021 on average but it decreased to 84,246 level at the minimum and increased 115,339 at the maximum. Besides, the retention share of foreign investors is 56.57% on average. The share decreased to 52.37% at the minimum and reached 60.73% at the maximum. Besides, the net selling amount of foreign investors is TRY 234.7 million on average. The descriptive statistics show that the figures for XU100, FSHARE, and NETSELL vary for the period whether Turkey is in the Covid-19 pandemic or not.
Table 4 presents the results of the ADF unit root test in both periods.
Period | Variable | ADF Test | |
Level (Prob.) | 1st Difference (Prob.) | ||
Before Covid-19 | XU100 | 0.9562 | 0.0002 |
FSHARE | 0.2782 | 0.0002 | |
NETSELL | 0.0003 | 0.0000 | |
In Covid-19 | XU100 | 0.9449 | 0.0000 |
FSHARE | 0.8625 | 0.0089 | |
NETSELL | 0.0231 | 0.0000 |
Before Covid-19 pandemic times, the probability values of the XU100 index and retention share of foreign investors are greater than 0.05. Therefore, these variables have a unit root and are non-stationary. On the other hand, the first difference of the variables has probability values that are lower than 0.05 meaning that they are stationary. In other words, the null hypothesis of one unit root against the alternative of stationarity cannot be rejected in levels of variables, but is rejected in their first differences, I (1). Therefore, the first differences of these variables are used in the analysis. Moreover, the net selling amount of foreign investors are stationary at levels. The stationarity condition of all variables is the same for in Covid-19 pandemic times.
Considering the stationarity results of the dataset which present that variables are stationary at different levels, it is decided to apply Toda & Yamamoto causality test. Moreover, MARS analysis is performed to examine the consistency of results.
In the context of the Engle-Granger cointegration test, the regression model is applied first and the stationarity of residuals is examined secondly. Table 5 shows the results of the unit root test for residuals for the period which is before the Covid-19 pandemic.
Period | Variable | ADF Test (Prob.) |
Before Covid-19 | XU100 & FSHARE | 0.0002 |
XU100 & NETSELL | 0.0009 | |
Covid-19 | XU100 & FSHARE | 0.0000 |
XU100 & NETSELL | 0.0001 |
As can be seen from Table 4, the probability values of the ADF test for residuals are lower than 0.05 which shows that there is a long-term cointegration between the XU100 index and the retention share of foreign investors. Besides, there is a long-term cointegration between the XU100 index and the net selling amount of foreign investors. Therefore, the XU100 index and retention share of foreign investors are cointegrated and move together in the long-run. Similarly, the XU100 index and net selling amount of foreign investors are cointegrated and moved together in the long-run. These findings are similar for both before the Covid-19 pandemic and in Covid-19 pandemic times.
In the context of the Toda-Yamamoto causality test, maximum cointegration degree (d) and lag lengths (k) are determined firstly and estimation is applied by using "k+dmax" formula. Table 6 shows the results of the lag length determination and maximum cointegration degree.
Period | Variable | Lag Lengths (k) | Maximum Cointegration (dmax) | Estimation Degree (k+dmax) | |
Before Covid-19 | XU100 & FSHARE | 1 | 1 | 2 | |
XU100 & NETSELL | 1 | 0 | 1 | ||
Covid-19 | XU100 & FSHARE | 2 | 1 | 3 | |
XU100 & NETSELL | 2 | 0 | 2 |
By considering the results in Table 5, the estimation degree is determined as 2 for retention share and as 1 for the net selling amount of foreign investors in the period which is before the Covid-19 pandemic. On the other hand, the estimation degree is determined as 3 for retention share and as 2 for net selling amount of foreign investors in the period which is in the Covid-19 pandemic.
Table 7 shows the results of the Toda-Yamamoto causality test.
Period | Variable | Estimation Degree (k+dmax) | Prob. | Result |
Before Covid-19 | FSHARE XU100 | 2 | 0.6940 | No Causality |
NETSELL XU100 | 1 | 0.3609 | No Causality | |
Covid-19 | FSHARE XU100 | 3 | 0.4120 | No Causality |
NETSELL XU100 | 2 | 0.2685 | No Causality |
According to the results in Table 6, there is no causality from the retention share of foreign investors and the net selling amount of foreign investors to the XU100 index in both periods. On the other hand, the probability values of the test imply that there is a nexus from the independent variables to the XU100 index at low confidence intervals. Moreover, the probability values of the test prove that there is an increasing role in the index from before the pandemic to the pandemic period. The results are important because the retention share of foreign investors and the net selling amount of foreign investors could be much more influential on the index when they are evaluated with the other indicators that are included in the analysis.
MARS method creates 4 models and BF1 is determined as the best model based on GCV and R2 statistics for the period which is before Covid-19 pandemic times. Table 8 presents the details of the variables' importance and Table 9 shows the details of the best model.
Variable | Importance Level | −GCV |
FSHARE | 100.000 | 0.248383E+08 |
NETSELL | 0.000 | 0.198267E+08 |
Basis Functions | Details | Coefficient |
Constant | 114,549.0783 | |
BF9 | max(0, FSHARE − 59.550) | 1,856.921 |
F Test: 21.274 (0.000) Adjusted R2: 0.301 |
According to the best model, the most significant variable on the XU100 index is the retention share of foreign investors in time which is before the Covid-19 pandemic.
Adjusted R2 of the best MARS model is 0.301 before Covid-19 pandemic times. These results imply that only the variables used in the analysis, which are retention share and net selling amount of foreign investors, explain 30.1% changes in the XU100 index.
On the other hand, MARS creates 17 models and BF2 is selected as the best model based on GCV and R2 statistics for the period which is in Covid-19 pandemic times. Table 10 presents the details of the variables' importance.
Variable | Importance Level | −GCV |
FSHARE | 100.000 | 0.735352E + 08 |
NETSELL | 45.652 | 0.286564E + 08 |
Also, Table 11 presents the details of the best model.
Basis Functions | Details | Coefficient |
Constant | 103,278.07 | |
BF1 | max(0, FSHARE − 53.780) | - |
BF2 | max(0, 53.780 − FSHARE) | 7,685.579 |
BF3 | max(0, NETSELL + 485.140) * BF1 | −2.215 |
F Test: 150.531 (0.000) Adjusted R2: 0.804 |
According to the best model, the most significant variable on the XU100 index is the retention share of foreign investors at the time of the Covid-19 pandemic. This result is also similar to the before Covid-19 pandemic times. However, there is an important difference that the net selling amount of foreign investors affects the XU100 index in the time of the Covid-19 period. Moreover, MARS determines that there are interactions between FSHARE and NETSELL in the time of the Covid-19 pandemic.
The adjusted R2 of the best MARS model is 0.804 in the time of the Covid-19 pandemic. These results imply that only the variables used in the analysis, which are retention share and net selling amount of foreign investors, explain 80.4% changes in the XU100 index.
In the study, the Engle-Granger cointegration test, Toda-Yamamoto causality test, MARS analysis are performed to examine the changes in the XU100 index by taking into consideration the retention share and net selling amount of foreign investors as independent variables. Engle-Granger cointegration test shows that the XU100 index is cointegrated with retention share and net selling amount of foreign investors in the long-term. However, the Toda-Yamamoto causality test presents that this relationship between the XU100 index and the independent variables is not at the level of causality. Moreover, MARS analysis defines that although the retention share of foreign investors is influential on the XU100 index for the period which is before the Covid-19 pandemic, however, net selling amount of foreign investors is also influential for the period which is in Covid-19 pandemic.
The analysis results define that the XU100 index is affected by the activities of foreign investors before and in Covid-19 pandemic times. The retention share of foreign investors is influential for both periods according to all methods used. This result is consistent with Kartal et al. (2020). Also, the effect of the net selling amount of foreign investors is increased in Covid-19 pandemic times as expected. However, it was not influential on the XU100 index before the Covid-19 pandemic according to the results of MARS. This result is not consistent with Kartal et al. (2020) that who determine that it is effective on the index although its effect is low. Moreover, there is not a causality relationship between the XU100 index and independent variables according to the Toda-Yamamoto causality test. When we evaluate all these results obtained from the test, they imply that although the activities of foreign investors affect the XU100 index, these are not the sole and main determinants of the XU100 index. Therefore, other determinants, which are not included in the study, should be taken into consideration to determine how the XU100 index change over before the pandemic and the pandemic periods.
By taking into consideration the analysis results, it could be recommended that the critical barriers in independent variables should not be exceeded. In other words, the retention share of foreign investors should be kept above 53.78%, and the net selling amount of foreign investors should be kept under the TRY 485 million so as not to cause a decreasing effect on the XU100 index. However, this should be not provided by increasing legal barriers and restricting the elbowroom of foreign investors such as prohibiting the short-selling. Instead, the attention of foreign investors should be increased by applying structural reforms like a positive real interest rate instead of a negative real interest rate and foreign portfolio inflows should be steered up in turn. By considering that Turkey is in time of Covid-19, and Turkey has not the capacity to lower the negative effects of global factors, Turkey could focus on macroeconomic and market variables which are mostly under the control of the country.
Also, other policies could be developed and applied by Turkish regulatory authorities. In such a process, the relationship between variables should be considered as MARS analysis shows because there is an interaction between the retention share and net selling amount of foreign investors. Therefore, the outcomes of measures to be taken on XU100 should be predicted before implementation.
The study examines the effects of foreign investors' activities on the main stock exchange indices by using Turkey's data before and in the time of the Covid-19 Pandemic. That is why because emerging countries need foreign financing sources, foreign portfolio investments have significance for these countries, equity investments are one of the important foreign portfolio investment tools to be used, and there is a Covid-19 pandemic since 03.10.2020 in Turkey. In this context, the XU100 index as a dependent variable, and retention share and net selling amount of foreign investors as independent variables are used, presence of Covid-19 pandemic is considered, and daily data between 01.02.2020 and 06.26.2020 (including 122 observations) is analyzed with performing Engle-Granger cointegration test, Toda-Yamamoto causality test, and MARS analysis.
As a result of the analysis, the findings reveal that the effects of retention share and net selling amount of foreign investors on the XU100 index vary according to period whether Turkey is in Covid-19 time or not according to the results of MARS. Besides, the power of effects varies according to period. Moreover, the results imply that the Covid-19 pandemic causes the increasing effect of the net selling amount of foreign investors on the XU100 index. On the other hand, there is not a relationship between the index and independent variables at the causality level which is surprising because it is expected that there could be a relationship at the causality level which reflecting a strong effect. Turkey could decrease the outflows of foreign investments from the stock exchange and prevent the underpricing of assets in turn.
In the study, Turkey is examined because there is quite volatile behavior in the XU100 index, retention share, and net selling amount, especially during the Covid-19 pandemic. By taking measures to decrease the negative effects, Turkey could attract more foreign portfolio investments. Also, additional analysis for Turkey at the end of the Covid-19 pandemic would contribute to the literature, and new policy proposals could be recommended based on the results of forthcoming studies. On the other hand, including different country groups like Venezuela, Argentina, Ukraine, which have some problems in terms of foreign investments, could help extend current literature. Similar results are expected for these countries and other emerging countries as well. On the other hand, the cointegration between the variables could be at the causality level which reflects that those countries are much more dependent on foreign portfolio inflows. Moreover, new variables could be included in the new analysis, and other methods like machine learning algorithms, neural networks, threshold cointegration model, and wavelet coherence approach could be applied in forthcoming studies.
The author declares no conflicts of interest in this paper.
[1] | Prince M, Jackson J (2009) Alzheimer's Disease International World Alzheimer Report 2009. London. 1-96 p. |
[2] |
Takeda A, Loveman E, Clegg A, et al. (2006) A systematic review of the clinical effectiveness of donepezil, rivastigmine and galantamine on cognition, quality of life and adverse events in Alzheimer's disease. Int J Geriatr Psychiatry 21: 17-28. doi: 10.1002/gps.1402
![]() |
[3] |
Ong KT, Villemagne VL, Bahar-Fuchs A, et al. (2015) Abeta imaging with 18F-florbetaben in prodromal Alzheimer's disease: a prospective outcome study. J Neurol Neurosurg Psychiatry 86: 431-436. doi: 10.1136/jnnp-2014-308094
![]() |
[4] |
Querfurth HW, LaFerla FM (2010) Alzheimer's disease. N Engl J Med 362: 329-344. doi: 10.1056/NEJMra0909142
![]() |
[5] |
Sperling RA, Aisen PS, Beckett LA, et al. (2011) Toward defining the preclinical stages of Alzheimer's disease: recommendations from the National Institute on Aging-Alzheimer's Association workgroups on diagnostic guidelines for Alzheimer's disease. Alzheimers Dement 7: 280-292. doi: 10.1016/j.jalz.2011.03.003
![]() |
[6] |
Petkova AT, Ishii Y, Balbach JJ, et al. (2002) A structural model for Alzheimer's beta -amyloid fibrils based on experimental constraints from solid state NMR. Proc Natl Acad Sci U S A 99: 16742-16747. doi: 10.1073/pnas.262663499
![]() |
[7] | Matsuzaki K (2014) How do membranes initiate Alzheimer's Disease? Formation of toxic amyloid fibrils by the amyloid beta-protein on ganglioside clusters. Acc Chem Res 47: 2397-2404. |
[8] | Cecchi C, Stefani M (2013) The amyloid-cell membrane system. The interplay between the biophysical features of oligomers/fibrils and cell membrane defines amyloid toxicity. Biophys Chem 182: 30-43. |
[9] |
Drolle E, Gaikwad RM, Leonenko Z (2012) Nanoscale electrostatic domains in cholesterol-laden lipid membranes create a target for amyloid binding. Biophys J 103: L27-29. doi: 10.1016/j.bpj.2012.06.053
![]() |
[10] |
Drolle E, Hane F, Lee B, et al. (2014) Atomic force microscopy to study molecular mechanisms of amyloid fibril formation and toxicity in Alzheimer's disease. Drug Metab Rev 46: 207-223. doi: 10.3109/03602532.2014.882354
![]() |
[11] | Hane F, Drolle E, Gaikwad R, et al. (2011) Amyloid-beta aggregation on model lipid membranes: an atomic force microscopy study. J Alzheimers Dis 26: 485-494. |
[12] |
Lal R, Lin H, Quist AP (2007) Amyloid beta ion channel: 3D structure and relevance to amyloid channel paradigm. Biochim Biophys Acta 1768: 1966-1975. doi: 10.1016/j.bbamem.2007.04.021
![]() |
[13] |
Arispe N, Pollard HB, Rojas E (1993) Giant multilevel cation channels formed by Alzheimer disease amyloid beta-protein [A beta P-(1-40)] in bilayer membranes. Proc Natl Acad Sci U S A 90: 10573-10577. doi: 10.1073/pnas.90.22.10573
![]() |
[14] |
Demuro A, Smith M, Parker I (2011) Single-channel Ca(2+) imaging implicates Abeta1-42 amyloid pores in Alzheimer's disease pathology. J Cell Biol 195: 515-524. doi: 10.1083/jcb.201104133
![]() |
[15] | Plant LD, Boyle JP, Smith IF, et al. (2003) The production of amyloid beta peptide is a critical requirement for the viability of central neurons. J Neurosci 23: 5531-5535. |
[16] |
Giuffrida ML, Caraci F, Pignataro B, et al. (2009) Beta-amyloid monomers are neuroprotective. J Neurosci 29: 10582-10587. doi: 10.1523/JNEUROSCI.1736-09.2009
![]() |
[17] |
Hardy J, Selkoe DJ (2002) The Amyloid Hypothesis of Alzheimer's Disease: Progress and Problems on the Road to Therapeutics. Science 297: 353-356. doi: 10.1126/science.1072994
![]() |
[18] |
Brambilla D, Verpillot R, Le Droumaguet B, et al. (2012) PEGylated nanoparticles bind to and alter amyloid-beta peptide conformation: toward engineering of functional nanomedicines for Alzheimer's disease. ACS Nano 6: 5897-5908. doi: 10.1021/nn300489k
![]() |
[19] |
Gobbi M, Re F, Canovi M, et al. (2010) Lipid-based nanoparticles with high binding affinity for amyloid-beta1-42 peptide. Biomaterials 31: 6519-6529. doi: 10.1016/j.biomaterials.2010.04.044
![]() |
[20] |
Cheng KK, Yeung CF, Ho SW, et al. (2013) Highly stabilized curcumin nanoparticles tested in an in vitro blood-brain barrier model and in Alzheimer's disease Tg2576 mice. Aaps j 15: 324-336. doi: 10.1208/s12248-012-9444-4
![]() |
[21] |
Cheng X, van Breemen RB (2005) Mass Spectrometry-Based Screening for Inhibitors of β-Amyloid Protein Aggregation. Anal Chem 77: 7012-7015. doi: 10.1021/ac050556a
![]() |
[22] |
He H, Dong W, Huang F (2010) Anti-Amyloidogenic and Anti-Apoptotic Role of Melatonin in Alzheimer Disease. Curr Neuropharmacol 8: 211-217. doi: 10.2174/157015910792246137
![]() |
[23] |
Lin L, Huang Q-X, Yang S-S, et al. (2013) Melatonin in Alzheimer's Disease. Int J Mol Sci 14: 14575-14593. doi: 10.3390/ijms140714575
![]() |
[24] | Rosales-Corral S, Acuna-Castroviejo D, Tan DX, et al. (2012) Accumulation of Exogenous Amyloid-Beta Peptide in Hippocampal Mitochondria Causes Their Dysfunction: A Protective Role for Melatonin. Oxid Med Cell Longev 2012: 843649. |
[25] |
Adolfsson O, Pihlgren M, Toni N, et al. (2012) An effector-reduced anti-beta-amyloid (Abeta) antibody with unique abeta binding properties promotes neuroprotection and glial engulfment of Abeta. J Neurosci 32: 9677-9689. doi: 10.1523/JNEUROSCI.4742-11.2012
![]() |
[26] |
DeMattos RB, Bales KR, Cummins DJ, et al. (2001) Peripheral anti-A beta antibody alters CNS and plasma A beta clearance and decreases brain A beta burden in a mouse model of Alzheimer's disease. Proc Natl Acad Sci U S A 98: 8850-8855. doi: 10.1073/pnas.151261398
![]() |
[27] |
Legleiter J, Czilli DL, Gitter B, et al. (2004) Effect of different anti-Abeta antibodies on Abeta fibrillogenesis as assessed by atomic force microscopy. J Mol Biol 335: 997-1006. doi: 10.1016/j.jmb.2003.11.019
![]() |
[28] |
Lemere CA (2013) Immunotherapy for Alzheimer's disease: hoops and hurdles. Mol Neurodegener 8: 36. doi: 10.1186/1750-1326-8-36
![]() |
[29] |
Robert R, Wark KL (2012) Engineered antibody approaches for Alzheimer's disease immunotherapy. Arch Biochem Biophys 526: 132-138. doi: 10.1016/j.abb.2012.02.022
![]() |
[30] |
Wilcock DM, Rojiani A, Rosenthal A, et al. (2004) Passive immunotherapy against Abeta in aged APP-transgenic mice reverses cognitive deficits and depletes parenchymal amyloid deposits in spite of increased vascular amyloid and microhemorrhage. J Neuroinflammation 1: 24. doi: 10.1186/1742-2094-1-24
![]() |
[31] |
Findeis MA, Musso GM, Arico-Muendel CC, et al. (1999) Modified-peptide inhibitors of amyloid beta-peptide polymerization. Biochemistry 38: 6791-6800. doi: 10.1021/bi982824n
![]() |
[32] |
Ghanta J, Shen CL, Kiessling LL, et al. (1996) A strategy for designing inhibitors of beta-amyloid toxicity. J Biol Chem 271: 29525-29528. doi: 10.1074/jbc.271.47.29525
![]() |
[33] |
Hane FT, Lee BY, Petoyan A, et al. (2014) Testing synthetic amyloid-beta aggregation inhibitor using single molecule atomic force spectroscopy. Biosens Bioelectron 54: 492-498. doi: 10.1016/j.bios.2013.10.060
![]() |
[34] |
Pallitto MM, Ghanta J, Heinzelman P, et al. (1999) Recognition sequence design for peptidyl modulators of beta-amyloid aggregation and toxicity. Biochemistry 38: 3570-3578. doi: 10.1021/bi982119e
![]() |
[35] |
Tjernberg LO, Naslund J, Lindqvist F, et al. (1996) Arrest of beta-amyloid fibril formation by a pentapeptide ligand. J Biol Chem 271: 8545-8548. doi: 10.1074/jbc.271.15.8545
![]() |
[36] |
Morgan D, Diamond DM, Gottschall PE, et al. (2000) A beta peptide vaccination prevents memory loss in an animal model of Alzheimer's disease. Nature 408: 982-985. doi: 10.1038/35050116
![]() |
[37] |
Orgogozo JM, Gilman S, Dartigues JF, et al. (2003) Subacute meningoencephalitis in a subset of patients with AD after Abeta42 immunization. Neurology 61: 46-54. doi: 10.1212/01.WNL.0000073623.84147.A8
![]() |
[38] |
Wildsmith KR, Holley M, Savage JC, et al. (2013) Evidence for impaired amyloid beta clearance in Alzheimer's disease. Alzheimers Res Ther 5: 33. doi: 10.1186/alzrt187
![]() |
[39] |
Evin G, Hince C (2013) BACE1 as a therapeutic target in Alzheimer's disease: rationale and current status. Drugs Aging 30: 755-764. doi: 10.1007/s40266-013-0099-3
![]() |
[40] | Wolfe MS (2012) gamma-Secretase inhibitors and modulators for Alzheimer's disease. J Neurochem 120 Suppl 1: 89-98. |
[41] | Atwal JK, Chen Y, Chiu C, et al. (2011) A therapeutic antibody targeting BACE1 inhibits amyloid-beta production in vivo. Sci Transl Med 3: 84ra43. |
[42] |
Kao SC, Krichevsky AM, Kosik KS, et al. (2004) BACE1 suppression by RNA interference in primary cortical neurons. J Biol Chem 279: 1942-1949. doi: 10.1074/jbc.M309219200
![]() |
[43] |
Singer O, Marr RA, Rockenstein E, et al. (2005) Targeting BACE1 with siRNAs ameliorates Alzheimer disease neuropathology in a transgenic model. Nat Neurosci 8: 1343-1349. doi: 10.1038/nn1531
![]() |
[44] |
Vassar R (2014) BACE1 inhibitor drugs in clinical trials for Alzheimer's disease. Alzheimers Res Ther 6: 89. doi: 10.1186/s13195-014-0089-7
![]() |
[45] |
Luo Y, Bolon B, Kahn S, et al. (2001) Mice deficient in BACE1, the Alzheimer's beta-secretase, have normal phenotype and abolished beta-amyloid generation. Nat Neurosci 4: 231-232. doi: 10.1038/85059
![]() |
[46] |
Jacobsen H, Ozmen L, Caruso A, et al. (2014) Combined treatment with a BACE inhibitor and anti-Abeta antibody gantenerumab enhances amyloid reduction in APPLondon mice. J Neurosci 34: 11621-11630. doi: 10.1523/JNEUROSCI.1405-14.2014
![]() |
[47] |
Morgan D (2005) Mechanisms of A beta plaque clearance following passive A beta immunization. Neurodegener Dis 2: 261-266. doi: 10.1159/000090366
![]() |
[48] |
Banks WA, Terrell B, Farr SA, et al. (2002) Passage of amyloid beta protein antibody across the blood-brain barrier in a mouse model of Alzheimer's disease. Peptides 23: 2223-2226. doi: 10.1016/S0196-9781(02)00261-9
![]() |
[49] |
Banks WA (2012) Drug delivery to the brain in Alzheimer's disease: consideration of the blood-brain barrier. Adv Drug Deliv Rev 64: 629-639. doi: 10.1016/j.addr.2011.12.005
![]() |
[50] |
Pfeifer LA, White LR, Ross GW, et al. (2002) Cerebral amyloid angiopathy and cognitive function: the HAAS autopsy study. Neurology 58: 1629-1634. doi: 10.1212/WNL.58.11.1629
![]() |
[51] |
Racke MM, Boone LI, Hepburn DL, et al. (2005) Exacerbation of cerebral amyloid angiopathy-associated microhemorrhage in amyloid precursor protein transgenic mice by immunotherapy is dependent on antibody recognition of deposited forms of amyloid beta. J Neurosci 25: 629-636. doi: 10.1523/JNEUROSCI.4337-04.2005
![]() |
[52] | Wilcock DM, Colton CA (2008) Anti-amyloid-beta immunotherapy in Alzheimer's disease: relevance of transgenic mouse studies to clinical trials. J Alzheimers Dis 15: 555-569. |
[53] |
Doody RS, Thomas RG, Farlow M, et al. (2014) Phase 3 trials of solanezumab for mild-to-moderate Alzheimer's disease. N Engl J Med 370: 311-321. doi: 10.1056/NEJMoa1312889
![]() |
[54] |
Panza F, Solfrizzi V, Imbimbo BP, et al. (2014) Amyloid-based immunotherapy for Alzheimer's disease in the time of prevention trials: the way forward. Expert Rev Clin Immunol 10: 405-419. doi: 10.1586/1744666X.2014.883921
![]() |
[55] |
Salloway S, Sperling R, Fox NC, et al. (2014) Two phase 3 trials of bapineuzumab in mild-to-moderate Alzheimer's disease. N Engl J Med 370: 322-333. doi: 10.1056/NEJMoa1304839
![]() |
[56] |
Tayeb HO, Murray ED, Price BH, et al. (2013) Bapineuzumab and solanezumab for Alzheimer's disease: is the 'amyloid cascade hypothesis' still alive? Expert Opin Biol Ther 13: 1075-1084. doi: 10.1517/14712598.2013.789856
![]() |
[57] |
Garber K (2012) Genentech's Alzheimer's antibody trial to study disease prevention. Nat Biotechnol 30: 731-732. doi: 10.1038/nbt0812-731
![]() |
[58] |
Panza F, Solfrizzi V, Imbimbo BP, et al. (2014) Efficacy and safety studies of gantenerumab in patients with Alzheimer's disease. Expert Rev Neurother 14: 973-986. doi: 10.1586/14737175.2014.945522
![]() |
[59] |
Kumar J, Sim V (2014) D-amino acid-based peptide inhibitors as early or preventative therapy in Alzheimer disease. Prion 8: 119-124. doi: 10.4161/pri.28220
![]() |
[60] |
Porat Y, Mazor Y, Efrat S, et al. (2004) Inhibition of islet amyloid polypeptide fibril formation: a potential role for heteroaromatic interactions. Biochemistry 43: 14454-14462. doi: 10.1021/bi048582a
![]() |
[61] |
Austen BM, Paleologou KE, Ali SA, et al. (2008) Designing peptide inhibitors for oligomerization and toxicity of Alzheimer's beta-amyloid peptide. Biochemistry 47: 1984-1992. doi: 10.1021/bi701415b
![]() |
[62] |
Taylor M, Moore S, Mayes J, et al. (2010) Development of a proteolytically stable retro-inverso peptide inhibitor of beta-amyloid oligomerization as a potential novel treatment for Alzheimer's disease. Biochemistry 49: 3261-3272. doi: 10.1021/bi100144m
![]() |
[63] | Roy S (2010) Designing Novel Peptidic Inhibitors of Beta Amyloid Oligomerization Calgary, Alberta: University of Calgary. 164 p. |
[64] | Gordon DJ, Tappe R, Meredith SC (2002) Design and characterization of a membrane permeable N-methyl amino acid-containing peptide that inhibits Abeta1-40 fibrillogenesis. J Pept Res 60: 37-55. |
[65] |
Parthsarathy V, McClean PL, Holscher C, et al. (2013) A novel retro-inverso peptide inhibitor reduces amyloid deposition, oxidation and inflammation and stimulates neurogenesis in the APPswe/PS1DeltaE9 mouse model of Alzheimer's disease. PLoS One 8: e54769. doi: 10.1371/journal.pone.0054769
![]() |
[66] | Yu YJ, Zhang Y, Kenrick M, et al. (2011) Boosting brain uptake of a therapeutic antibody by reducing its affinity for a transcytosis target. Sci Transl Med 3: 84ra44. |
[67] |
Xiong N, Dong X-Y, Zheng J, et al. (2015) Design of LVFFARK and LVFFARK-functionalized nanoparticles for inhibiting amyloid β-protein fibrillation and cytotoxicity. ACS Appl Mater Interfaces 7: 5650-5662. doi: 10.1021/acsami.5b00915
![]() |
[68] |
Taylor M, Moore S, Mourtas S, et al. (2011) Effect of curcumin-associated and lipid ligand-functionalized nanoliposomes on aggregation of the Alzheimer's Abeta peptide. Nanomedicine 7: 541-550. doi: 10.1016/j.nano.2011.06.015
![]() |
[69] | Armstrong RA (2014) A critical analysis of the 'amyloid cascade hypothesis'. Folia Neuropathol 52: 211-225. |
[70] | Castello MA, Soriano S (2014) On the origin of Alzheimer's disease. Trials and tribulations of the amyloid hypothesis. Ageing Res Rev 13: 10-12. |
[71] |
Chen Y, Liu L (2012) Modern methods for delivery of drugs across the blood-brain barrier. Adv Drug Deliv Rev 64: 640-665. doi: 10.1016/j.addr.2011.11.010
![]() |
[72] |
Pardridge WM (2003) Blood-brain barrier drug targeting: the future of brain drug development. Mol Interv 3: 90-105, 151. doi: 10.1124/mi.3.2.90
![]() |
[73] |
Abbott NJ, Patabendige AA, Dolman DE, et al. (2010) Structure and function of the blood-brain barrier. Neurobiol Dis 37: 13-25. doi: 10.1016/j.nbd.2009.07.030
![]() |
[74] |
Abbott NJ, Ronnback L, Hansson E (2006) Astrocyte-endothelial interactions at the blood-brain barrier. Nat Rev Neurosci 7: 41-53. doi: 10.1038/nrn1824
![]() |
[75] |
Mehta DC, Short JL, Nicolazzo JA (2013) Memantine transport across the mouse blood-brain barrier is mediated by a cationic influx H+ antiporter. Mol Pharm 10: 4491-4498. doi: 10.1021/mp400316e
![]() |
[76] |
Kim MH, Maeng HJ, Yu KH, et al. (2010) Evidence of carrier-mediated transport in the penetration of donepezil into the rat brain. J Pharm Sci 99: 1548-1566. doi: 10.1002/jps.21895
![]() |
[77] |
Cirrito JR, Deane R, Fagan AM, et al. (2005) P-glycoprotein deficiency at the blood-brain barrier increases amyloid-beta deposition in an Alzheimer disease mouse model. J Clin Invest 115: 3285-3290. doi: 10.1172/JCI25247
![]() |
[78] |
Deane R, Wu Z, Zlokovic BV (2004) RAGE (yin) versus LRP (yang) balance regulates alzheimer amyloid beta-peptide clearance through transport across the blood-brain barrier. Stroke 35: 2628-2631. doi: 10.1161/01.STR.0000143452.85382.d1
![]() |
[79] | Guillemin GJ, Brew BJ (2004) Microglia, macrophages, perivascular macrophages, and pericytes: a review of function and identification. J Leukoc Biol 75: 388-397. |
[80] |
Bowman GL, Kaye JA, Moore M, et al. (2007) Blood-brain barrier impairment in Alzheimer disease: stability and functional significance. Neurology 68: 1809-1814. doi: 10.1212/01.wnl.0000262031.18018.1a
![]() |
[81] | Claudio L (1996) Ultrastructural features of the blood-brain barrier in biopsy tissue from Alzheimer's disease patients. Acta Neuropathol 91: 6-14. |
[82] | Kalaria RN (1992) The blood-brain barrier and cerebral microcirculation in Alzheimer disease. Cerebrovasc Brain Metab Rev 4: 226-260. |
[83] |
Marco S, Skaper SD (2006) Amyloid beta-peptide1-42 alters tight junction protein distribution and expression in brain microvessel endothelial cells. Neurosci Lett 401: 219-224. doi: 10.1016/j.neulet.2006.03.047
![]() |
[84] |
Zlokovic BV (2005) Neurovascular mechanisms of Alzheimer's neurodegeneration. Trends Neurosci 28: 202-208. doi: 10.1016/j.tins.2005.02.001
![]() |
[85] |
Wisniewski HM, Vorbrodt AW, Wegiel J (1997) Amyloid angiopathy and blood-brain barrier changes in Alzheimer's disease. Ann N Y Acad Sci 826: 161-172. doi: 10.1111/j.1749-6632.1997.tb48468.x
![]() |
[86] |
Kalaria RN, Sromek SM, Grahovac I, et al. (1992) Transferrin receptors of rat and human brain and cerebral microvessels and their status in Alzheimer's disease. Brain Res 585: 87-93. doi: 10.1016/0006-8993(92)91193-I
![]() |
[87] |
Jones AR, Shusta EV (2007) Blood-brain barrier transport of therapeutics via receptor-mediation. Pharm Res 24: 1759-1771. doi: 10.1007/s11095-007-9379-0
![]() |
[88] |
Gatter KC, Brown G, Trowbridge IS, et al. (1983) Transferrin receptors in human tissues: their distribution and possible clinical relevance. J Clin Pathol 36: 539-545. doi: 10.1136/jcp.36.5.539
![]() |
[89] | Lee HJ, Engelhardt B, Lesley J, et al. (2000) Targeting rat anti-mouse transferrin receptor monoclonal antibodies through blood-brain barrier in mouse. J Pharmacol Exp Ther 292: 1048-1052. |
[90] |
Prades R, Guerrero S, Araya E, et al. (2012) Delivery of gold nanoparticles to the brain by conjugation with a peptide that recognizes the transferrin receptor. Biomaterials 33: 7194-7205. doi: 10.1016/j.biomaterials.2012.06.063
![]() |
[91] | Zhao WQ, De Felice FG, Fernandez S, et al. (2008) Amyloid beta oligomers induce impairment of neuronal insulin receptors. Faseb J 22: 246-260. |
[92] | Xie L, Helmerhorst E, Taddei K, et al. (2002) Alzheimer's beta-amyloid peptides compete for insulin binding to the insulin receptor. J Neurosci 22: Rc221. |
[93] |
Markoutsa E, Papadia K, Giannou AD, et al. (2014) Mono and dually decorated nanoliposomes for brain targeting, in vitro and in vivo studies. Pharm Res 31: 1275-1289. doi: 10.1007/s11095-013-1249-3
![]() |
[94] | Re F, Cambianica I, Sesana S, et al. (2010) Functionalization with ApoE-derived peptides enhances the interaction with brain capillary endothelial cells of nanoliposomes binding amyloid-beta peptide. J Biotechnol 156: 341-346. |
[95] |
Gaillard PJ, Visser CC, de Boer AG (2005) Targeted delivery across the blood-brain barrier. Expert Opin Drug Deliv 2: 299-309. doi: 10.1517/17425247.2.2.299
![]() |
[96] |
Lentz TL (1990) Rabies virus binding to an acetylcholine receptor alpha-subunit peptide. J Mol Recognit 3: 82-88. doi: 10.1002/jmr.300030205
![]() |
[97] |
Liu Y, Huang R, Han L, et al. (2009) Brain-targeting gene delivery and cellular internalization mechanisms for modified rabies virus glycoprotein RVG29 nanoparticles. Biomaterials 30: 4195-4202. doi: 10.1016/j.biomaterials.2009.02.051
![]() |
[98] | Couch JA, Yu YJ, Zhang Y, et al. (2013) Addressing safety liabilities of TfR bispecific antibodies that cross the blood-brain barrier. Sci Transl Med 5: 183ra157, 181-112. |
[99] |
Bien-Ly N, Yu YJ, Bumbaca D, et al. (2014) Transferrin receptor (TfR) trafficking determines brain uptake of TfR antibody affinity variants. J Exp Med 211: 233-244. doi: 10.1084/jem.20131660
![]() |
[100] | Yu YJ, Atwal JK, Zhang Y, et al. (2014) Therapeutic bispecific antibodies cross the blood-brain barrier in nonhuman primates. Sci Transl Med 6: 261ra154. |
[101] |
Niewoehner J, Bohrmann B, Collin L, et al. (2014) Increased brain penetration and potency of a therapeutic antibody using a monovalent molecular shuttle. Neuron 81: 49-60. doi: 10.1016/j.neuron.2013.10.061
![]() |
[102] |
van Rooy I, Mastrobattista E, Storm G, et al. (2011) Comparison of five different targeting ligands to enhance accumulation of liposomes into the brain. J Control Release 150: 30-36. doi: 10.1016/j.jconrel.2010.11.014
![]() |
[103] |
Zhang C, Wan X, Zheng X, et al. (2014) Dual-functional nanoparticles targeting amyloid plaques in the brains of Alzheimer's disease mice. Biomaterials 35: 456-465. doi: 10.1016/j.biomaterials.2013.09.063
![]() |
[104] |
Zhang C, Zheng X, Wan X, et al. (2014) The potential use of H102 peptide-loaded dual-functional nanoparticles in the treatment of Alzheimer's disease. J Control Release 192: 317-324. doi: 10.1016/j.jconrel.2014.07.050
![]() |
[105] | Salvati E, Re F, Sesana S, et al. (2013) Liposomes functionalized to overcome the blood-brain barrier and to target amyloid-beta peptide: the chemical design affects the permeability across an in vitro model. Int J Nanomedicine 8: 1749-1758. |
[106] |
Markoutsa E, Papadia K, Clemente C, et al. (2012) Anti-Abeta-MAb and dually decorated nanoliposomes: effect of Abeta1-42 peptides on interaction with hCMEC/D3 cells. Eur J Pharm Biopharm 81: 49-56. doi: 10.1016/j.ejpb.2012.02.006
![]() |
[107] |
Herve F, Ghinea N, Scherrmann JM (2008) CNS delivery via adsorptive transcytosis. AAPS J 10: 455-472. doi: 10.1208/s12248-008-9055-2
![]() |
[108] | Pardridge WM, Buciak JL, Kang YS, et al. (1993) Protamine-mediated transport of albumin into brain and other organs of the rat. Binding and endocytosis of protamine-albumin complex by microvascular endothelium. J Clin Invest 92: 2224-2229. |
[109] |
Bickel U (1995) Antibody delivery through the blood-brain barrier. Adv Drug Deliv Rev 15: 53-72. doi: 10.1016/0169-409X(95)00005-R
![]() |
[110] |
Bechara C, Sagan S (2013) Cell-penetrating peptides: 20 years later, where do we stand? FEBS Lett 587: 1693-1702. doi: 10.1016/j.febslet.2013.04.031
![]() |
[111] |
Jaruszewski KM, Ramakrishnan S, Poduslo JF, et al. (2012) Chitosan enhances the stability and targeting of immuno-nanovehicles to cerebro-vascular deposits of Alzheimer's disease amyloid protein. Nanomedicine 8: 250-260. doi: 10.1016/j.nano.2011.06.008
![]() |
[112] |
Parhamifar L, Sime W, Yudina Y, et al. (2010) Ligand-induced tyrosine phosphorylation of cysteinyl leukotriene receptor 1 triggers internalization and signaling in intestinal epithelial cells. PLoS One 5: e14439. doi: 10.1371/journal.pone.0014439
![]() |
[113] |
Malhotra M, Tomaro-Duchesneau C, Prakash S (2013) Synthesis of TAT peptide-tagged PEGylated chitosan nanoparticles for siRNA delivery targeting neurodegenerative diseases. Biomaterials 34: 1270-1280. doi: 10.1016/j.biomaterials.2012.10.013
![]() |
[114] |
Sarvaiya J, Agrawal YK (2015) Chitosan as a suitable nanocarrier material for anti-Alzheimer drug delivery. Int J Biol Macromol 72: 454-465. doi: 10.1016/j.ijbiomac.2014.08.052
![]() |
[115] |
Gao Y, Wang ZY, Zhang J, et al. (2014) RVG-peptide-linked trimethylated chitosan for delivery of siRNA to the brain. Biomacromolecules 15: 1010-1018. doi: 10.1021/bm401906p
![]() |
[116] |
Sharma G, Modgil A, Layek B, et al. (2013) Cell penetrating peptide tethered bi-ligand liposomes for delivery to brain in vivo: Biodistribution and transfection. J Control Release 167: 1-10. doi: 10.1016/j.jconrel.2013.01.016
![]() |
1. | Mustafa Tevfik KARTAL, Özer DEPREN, Serpil KILIÇ DEPREN, The relationship between mobility and COVID-19 pandemic: Daily evidence from an emerging country by causality analysis, 2021, 10, 25901982, 100366, 10.1016/j.trip.2021.100366 | |
2. | Mustafa Tevfik Kartal, Hasan Murat Ertuğrul, Talat Ulussever, The impacts of foreign portfolio flows and monetary policy responses on stock markets by considering COVID-19 pandemic: Evidence from Turkey, 2022, 22, 22148450, 12, 10.1016/j.bir.2021.06.003 | |
3. | Mohammed H. Alamoudi, Omer A. Bafail, BWM—RAPS Approach for Evaluating and Ranking Banking Sector Companies Based on Their Financial Indicators in the Saudi Stock Market, 2022, 15, 1911-8074, 467, 10.3390/jrfm15100467 | |
4. | Tomiwa Sunday Adebayo, Mustafa Tevfik Kartal, Effect of green bonds, oil prices, and COVID-19 on industrial CO2 emissions in the USA: Evidence from novel wavelet local multiple correlation approach, 2023, 0958-305X, 0958305X2311674, 10.1177/0958305X231167463 | |
5. | Herry Subagyo, Hersugondo Hersugondo, Wijaya Marcellino Candra, Kardison Lumban Batu, Dwi Eko Waluyo, Foreign investor portfolio flow and monetary policy response in the Indonesian stock market considering the COVID-19 pandemic, 2024, 21, 18104967, 88, 10.21511/imfi.21(1).2024.08 | |
6. | Abdulrahman T. Alsanousi, Ammar Y. Alqahtani, Anas A. Makki, Majed A. Baghdadi, A Hybrid MCDM Approach Using the BWM and the TOPSIS for a Financial Performance-Based Evaluation of Saudi Stocks, 2024, 15, 2078-2489, 258, 10.3390/info15050258 | |
7. | Mustafa Tevfik Kartal, Talat Ulussever, Ugur Korkut Pata, Serpil Kılıç Depren, Dynamic link between central bank reserves, credit default swap spreads, and foreign exchange rates: Evidence from Turkey by time series econometrics, 2023, 9, 24058440, e16392, 10.1016/j.heliyon.2023.e16392 |
Authors | Year | Scope | Period | Method | Results |
Clark & Berko | 1997 | Mexico | 1989/1–1996/3 | Regression | The increase in FPI causes an increase in stock prices. |
Bekaert & Harvey | 1998 | 17 Emerging Countries | 1977–1996 | GARCH | Increasing flows have a high correlation with returns. |
Dahlquist & Robertsson | 2004 | Sweden | 1993–1998 | VAR | Net purchases of foreign investors provide a permanent increase of stock prices. |
Baklacı | 2007 | Turkey | 1997/1–2006/4 | Granger causality & OLS | There is a significant bilateral interaction between FPI and stock returns. |
Boyer & Zheng | 2009 | United States | 1952–2004 | VAR & Regression | There is a significant positive relation between stock market returns and flows of foreign investors. |
Sevil et al. | 2012 | Turkey | 2006/1–2010/4 | VECM & Granger causality | There is causality from foreign investors to market index return. |
Vo | 2015 | Vietnam | 2006/2012 | GMM & Regression | Ownership of foreign investors decreases firm stock price volatility. |
Kesik et al. | 2016 | Turkey | 2005/2015 | Granger causality | FPI have an increasing impact on XU100 index. |
Liew et al. | 2018 | Malaysia | 2009/1–2016/12 | VAR & Granger causality | There is a one-way causality from gross inflows. |
Topaloğlu et al. | 2019 | 7 Emerging Countries | 2005–2016 | Panel data analysis | There is a significant and positive nexus between FPI and stock market returns. |
Angelovska | 2020 | Macedonia | 2005/1–2009/12 | Regression | Net inflows are connected with the rise of returns. |
David et al. | 2020 | 11 Countries | 79 Trading Days | Threshold cointegration | Shocks caused by diseases have an important effect on stock indices. |
Gupta & Ahmet | 2020 | India | 2002/1–2016/12 | ARDL | The performance of stock exchanges is one of the most important factors for FPI. |
Kartal et al. | 2020 | Turkey | 2020/1–2020/5 | Machine learning | The retention amount of foreign investors is influential in both the pre-pandemic and the pandemic periods. |
Kotishwar | 2020 | India | 2000/1–2019/12 | ARCH & OLS | FPI influence equity market volatility. |
Note: ARCH = Auto regressive conditional heteroscedasticity; ARDL = Autoregressive distributed lag; FPI = Foreign portfolio inflows; GARCH = Generalized autoregressive conditional heteroscedasticity; GMM = Generalized method of moments; OLS = Ordinary Least Squares; VAR = Vector autoregression; VECM = Vector error correction model. |
Variable | Symbol | Description | Effect | |
Type | Name | |||
Dependent | Main index | XU100 | The daily closing value of the BIST main index | |
Independent | Retention share | FSHARE | Daily retention share of foreign investors in BIST (%) | + |
Net selling | NETSELL | Daily net selling amount of foreign investors in BIST (TRY denominated amount) | - | |
Covid-19 | COVID | 1: If Covid-19 exists, 0: otherwise | - | |
Note: A positive (+) relationship means that XU100 increases when the independent variable increases. A negative (-) relationship means that XU100 decreases when the independent variable increases. |
Period Variable | Before Covid-19 | Covid-19 | |||||
XU1001 | FSHARE2 | NETSELL3 | XU1001 | FSHARE2 | NETSELL3 | ||
Mean | 117,361.9 | 61.01856 | 215.9715 | 100,021.8 | 56.56831 | 234.6904 | |
Median | 119,408.5 | 60.73146 | 258.8678 | 99,069.46 | 57.31724 | 278.9121 | |
Maximum | 123,556.1 | 65.85941 | 786.5698 | 115,338.9 | 60.72814 | 1109.929 | |
Minimum | 103,524.0 | 58.88518 | −1029.742 | 84,246.17 | 52.37000 | -485.1429 | |
Std. Dev. | 4,931.612 | 1.547033 | 324.5663 | 85, 17.130 | 2.988219 | 263.4816 | |
Skewness | -0.982580 | 1.401699 | -1.151931 | 0.041302 | -0.114845 | -0.297241 | |
Kurtosis | 3.089060 | 5.100109 | 5.810881 | 2.087917 | 1.440857 | 4.418486 | |
Jarque-Bera | 7.739573 | 24.53900 | 26.41767 | 2.586051 | 7.658022 | 7.293661 | |
Probability | 0.020863 | 0.000005 | 0.000002 | 0.274439 | 0.021731 | 0.026074 | |
Observations | 48 | 48 | 48 | 74 | 74 | 74 | |
Note: 1 The dependent variable; 2 %; 3 million TRY. |
Period | Variable | ADF Test | |
Level (Prob.) | 1st Difference (Prob.) | ||
Before Covid-19 | XU100 | 0.9562 | 0.0002 |
FSHARE | 0.2782 | 0.0002 | |
NETSELL | 0.0003 | 0.0000 | |
In Covid-19 | XU100 | 0.9449 | 0.0000 |
FSHARE | 0.8625 | 0.0089 | |
NETSELL | 0.0231 | 0.0000 |
Period | Variable | ADF Test (Prob.) |
Before Covid-19 | XU100 & FSHARE | 0.0002 |
XU100 & NETSELL | 0.0009 | |
Covid-19 | XU100 & FSHARE | 0.0000 |
XU100 & NETSELL | 0.0001 |
Period | Variable | Lag Lengths (k) | Maximum Cointegration (dmax) | Estimation Degree (k+dmax) | |
Before Covid-19 | XU100 & FSHARE | 1 | 1 | 2 | |
XU100 & NETSELL | 1 | 0 | 1 | ||
Covid-19 | XU100 & FSHARE | 2 | 1 | 3 | |
XU100 & NETSELL | 2 | 0 | 2 |
Period | Variable | Estimation Degree (k+dmax) | Prob. | Result |
Before Covid-19 | FSHARE XU100 | 2 | 0.6940 | No Causality |
NETSELL XU100 | 1 | 0.3609 | No Causality | |
Covid-19 | FSHARE XU100 | 3 | 0.4120 | No Causality |
NETSELL XU100 | 2 | 0.2685 | No Causality |
Variable | Importance Level | −GCV |
FSHARE | 100.000 | 0.248383E+08 |
NETSELL | 0.000 | 0.198267E+08 |
Basis Functions | Details | Coefficient |
Constant | 114,549.0783 | |
BF9 | max(0, FSHARE − 59.550) | 1,856.921 |
F Test: 21.274 (0.000) Adjusted R2: 0.301 |
Variable | Importance Level | −GCV |
FSHARE | 100.000 | 0.735352E + 08 |
NETSELL | 45.652 | 0.286564E + 08 |
Basis Functions | Details | Coefficient |
Constant | 103,278.07 | |
BF1 | max(0, FSHARE − 53.780) | - |
BF2 | max(0, 53.780 − FSHARE) | 7,685.579 |
BF3 | max(0, NETSELL + 485.140) * BF1 | −2.215 |
F Test: 150.531 (0.000) Adjusted R2: 0.804 |
Authors | Year | Scope | Period | Method | Results |
Clark & Berko | 1997 | Mexico | 1989/1–1996/3 | Regression | The increase in FPI causes an increase in stock prices. |
Bekaert & Harvey | 1998 | 17 Emerging Countries | 1977–1996 | GARCH | Increasing flows have a high correlation with returns. |
Dahlquist & Robertsson | 2004 | Sweden | 1993–1998 | VAR | Net purchases of foreign investors provide a permanent increase of stock prices. |
Baklacı | 2007 | Turkey | 1997/1–2006/4 | Granger causality & OLS | There is a significant bilateral interaction between FPI and stock returns. |
Boyer & Zheng | 2009 | United States | 1952–2004 | VAR & Regression | There is a significant positive relation between stock market returns and flows of foreign investors. |
Sevil et al. | 2012 | Turkey | 2006/1–2010/4 | VECM & Granger causality | There is causality from foreign investors to market index return. |
Vo | 2015 | Vietnam | 2006/2012 | GMM & Regression | Ownership of foreign investors decreases firm stock price volatility. |
Kesik et al. | 2016 | Turkey | 2005/2015 | Granger causality | FPI have an increasing impact on XU100 index. |
Liew et al. | 2018 | Malaysia | 2009/1–2016/12 | VAR & Granger causality | There is a one-way causality from gross inflows. |
Topaloğlu et al. | 2019 | 7 Emerging Countries | 2005–2016 | Panel data analysis | There is a significant and positive nexus between FPI and stock market returns. |
Angelovska | 2020 | Macedonia | 2005/1–2009/12 | Regression | Net inflows are connected with the rise of returns. |
David et al. | 2020 | 11 Countries | 79 Trading Days | Threshold cointegration | Shocks caused by diseases have an important effect on stock indices. |
Gupta & Ahmet | 2020 | India | 2002/1–2016/12 | ARDL | The performance of stock exchanges is one of the most important factors for FPI. |
Kartal et al. | 2020 | Turkey | 2020/1–2020/5 | Machine learning | The retention amount of foreign investors is influential in both the pre-pandemic and the pandemic periods. |
Kotishwar | 2020 | India | 2000/1–2019/12 | ARCH & OLS | FPI influence equity market volatility. |
Note: ARCH = Auto regressive conditional heteroscedasticity; ARDL = Autoregressive distributed lag; FPI = Foreign portfolio inflows; GARCH = Generalized autoregressive conditional heteroscedasticity; GMM = Generalized method of moments; OLS = Ordinary Least Squares; VAR = Vector autoregression; VECM = Vector error correction model. |
Variable | Symbol | Description | Effect | |
Type | Name | |||
Dependent | Main index | XU100 | The daily closing value of the BIST main index | |
Independent | Retention share | FSHARE | Daily retention share of foreign investors in BIST (%) | + |
Net selling | NETSELL | Daily net selling amount of foreign investors in BIST (TRY denominated amount) | - | |
Covid-19 | COVID | 1: If Covid-19 exists, 0: otherwise | - | |
Note: A positive (+) relationship means that XU100 increases when the independent variable increases. A negative (-) relationship means that XU100 decreases when the independent variable increases. |
Period Variable | Before Covid-19 | Covid-19 | |||||
XU1001 | FSHARE2 | NETSELL3 | XU1001 | FSHARE2 | NETSELL3 | ||
Mean | 117,361.9 | 61.01856 | 215.9715 | 100,021.8 | 56.56831 | 234.6904 | |
Median | 119,408.5 | 60.73146 | 258.8678 | 99,069.46 | 57.31724 | 278.9121 | |
Maximum | 123,556.1 | 65.85941 | 786.5698 | 115,338.9 | 60.72814 | 1109.929 | |
Minimum | 103,524.0 | 58.88518 | −1029.742 | 84,246.17 | 52.37000 | -485.1429 | |
Std. Dev. | 4,931.612 | 1.547033 | 324.5663 | 85, 17.130 | 2.988219 | 263.4816 | |
Skewness | -0.982580 | 1.401699 | -1.151931 | 0.041302 | -0.114845 | -0.297241 | |
Kurtosis | 3.089060 | 5.100109 | 5.810881 | 2.087917 | 1.440857 | 4.418486 | |
Jarque-Bera | 7.739573 | 24.53900 | 26.41767 | 2.586051 | 7.658022 | 7.293661 | |
Probability | 0.020863 | 0.000005 | 0.000002 | 0.274439 | 0.021731 | 0.026074 | |
Observations | 48 | 48 | 48 | 74 | 74 | 74 | |
Note: 1 The dependent variable; 2 %; 3 million TRY. |
Period | Variable | ADF Test | |
Level (Prob.) | 1st Difference (Prob.) | ||
Before Covid-19 | XU100 | 0.9562 | 0.0002 |
FSHARE | 0.2782 | 0.0002 | |
NETSELL | 0.0003 | 0.0000 | |
In Covid-19 | XU100 | 0.9449 | 0.0000 |
FSHARE | 0.8625 | 0.0089 | |
NETSELL | 0.0231 | 0.0000 |
Period | Variable | ADF Test (Prob.) |
Before Covid-19 | XU100 & FSHARE | 0.0002 |
XU100 & NETSELL | 0.0009 | |
Covid-19 | XU100 & FSHARE | 0.0000 |
XU100 & NETSELL | 0.0001 |
Period | Variable | Lag Lengths (k) | Maximum Cointegration (dmax) | Estimation Degree (k+dmax) | |
Before Covid-19 | XU100 & FSHARE | 1 | 1 | 2 | |
XU100 & NETSELL | 1 | 0 | 1 | ||
Covid-19 | XU100 & FSHARE | 2 | 1 | 3 | |
XU100 & NETSELL | 2 | 0 | 2 |
Period | Variable | Estimation Degree (k+dmax) | Prob. | Result |
Before Covid-19 | FSHARE XU100 | 2 | 0.6940 | No Causality |
NETSELL XU100 | 1 | 0.3609 | No Causality | |
Covid-19 | FSHARE XU100 | 3 | 0.4120 | No Causality |
NETSELL XU100 | 2 | 0.2685 | No Causality |
Variable | Importance Level | −GCV |
FSHARE | 100.000 | 0.248383E+08 |
NETSELL | 0.000 | 0.198267E+08 |
Basis Functions | Details | Coefficient |
Constant | 114,549.0783 | |
BF9 | max(0, FSHARE − 59.550) | 1,856.921 |
F Test: 21.274 (0.000) Adjusted R2: 0.301 |
Variable | Importance Level | −GCV |
FSHARE | 100.000 | 0.735352E + 08 |
NETSELL | 45.652 | 0.286564E + 08 |
Basis Functions | Details | Coefficient |
Constant | 103,278.07 | |
BF1 | max(0, FSHARE − 53.780) | - |
BF2 | max(0, 53.780 − FSHARE) | 7,685.579 |
BF3 | max(0, NETSELL + 485.140) * BF1 | −2.215 |
F Test: 150.531 (0.000) Adjusted R2: 0.804 |