Special Issue: Fault Diagnosis: Mathematical Models, Algorithms, and Application
Guest Editors
Dr. Kok Yew NG
Senior Lecturer in Mechatronics and Control Systems, School of Engineering, Ulster University, UK
Email: mark.ng@ulster.ac.uk
Prof. Chee Pin TAN
Professor, School of Engineering, Monash University, Malaysia
Email: tan.chee.pin@monash.edu
Prof. Xianghua WANG
Beijing University of Posts and Telecommunications
Email: xianghuaw@pku.edu.cn
Manuscript Topics
The advancements of Industry 4.0 and autonomous systems in recent years have led to an increase of research interest in the field of fault diagnosis. Using advanced theories and algorithms, researchers seek to detect, isolate, and reconstruct faults in various industries involving automation to enhance overall performance, improve production yield, better predictive maintenance planning, improve safety and reliability, as well as to save cost.
While most established fault diagnosis schemes and algorithms are fundamentally model-based techniques developed using mathematical models of the systems, data-driven techniques using artificial intelligence and machine learning methods have also gained much popularity lately. No matter the method used, mathematics, including statistics and probability theories play critical roles in the design and development of the fault diagnosis schemes.
This Special Issue aims to bring together novel research methodologies using model-based techniques, data-driven methods, or a hybrid of both for fault diagnosis. Contributions of research topics including, but not limited to the following are welcome:
• Fault detection
• Fault isolation
• Fault reconstruction
• Fault diagnosis
• Fault tolerant control
• Predictive maintenance
• Sliding mode
• Model-based design
• Data-driven methods
• Hybrid of model-based and data-driven techniques
Instruction for Authors
http://www.aimspress.com/math/news/solo-detail/instructionsforauthors
Please submit your manuscript to online submission system
https://aimspress.jams.pub/
Paper Submission
All manuscripts will be peer-reviewed before their acceptance for publication. The deadline for manuscript submission is 01 July 2023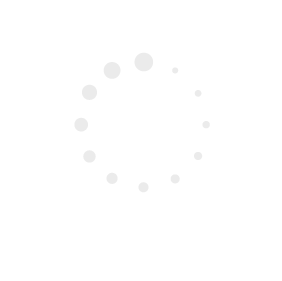